Subscribe to the PwC Newsletter
Join the community, trending research, scalable matmul-free language modeling.

Our experiments show that our proposed MatMul-free models achieve performance on-par with state-of-the-art Transformers that require far more memory during inference at a scale up to at least 2. 7B parameters.


Autoregressive Model Beats Diffusion: Llama for Scalable Image Generation
(3) A text-conditional image generation model with 775M parameters, from two-stage training on LAION-COCO and high aesthetics quality images, demonstrating competitive performance of visual quality and text alignment.

"Do Anything Now": Characterizing and Evaluating In-The-Wild Jailbreak Prompts on Large Language Models
We hope that our study can facilitate the research community and LLM vendors in promoting safer and regulated LLMs.

TextGrad: Automatic "Differentiation" via Text
Without modifying the framework, TextGrad improves the zero-shot accuracy of GPT-4o in Google-Proof Question Answering from $51\%$ to $55\%$, yields $20\%$ relative performance gain in optimizing LeetCode-Hard coding problem solutions, improves prompts for reasoning, designs new druglike small molecules with desirable in silico binding, and designs radiation oncology treatment plans with high specificity.
Matching Anything by Segmenting Anything
siyuanliii/masa • CVPR 2024
The robust association of the same objects across video frames in complex scenes is crucial for many applications, especially Multiple Object Tracking (MOT).
X-LoRA: Mixture of Low-Rank Adapter Experts, a Flexible Framework for Large Language Models with Applications in Protein Mechanics and Molecular Design
ericlbuehler/mistral.rs • 11 Feb 2024
Starting with a set of pre-trained LoRA adapters, our gating strategy uses the hidden states to dynamically mix adapted layers, allowing the resulting X-LoRA model to draw upon different capabilities and create never-before-used deep layer-wise combinations to solve tasks.
Less is More: Removing Text-regions Improves CLIP Training Efficiency and Robustness

In this paper, we discuss two effective approaches to improve the efficiency and robustness of CLIP training: (1) augmenting the training dataset while maintaining the same number of optimization steps, and (2) filtering out samples that contain text regions in the image.
Revisiting MoE and Dense Speed-Accuracy Comparisons for LLM Training
In this work, we revisit the settings by adopting step time as a more accurate measure of model complexity, and by determining the total compute budget under the Chinchilla compute-optimal settings.
LibriTTS-P: A Corpus with Speaking Style and Speaker Identity Prompts for Text-to-Speech and Style Captioning
line/libritts-p • 12 Jun 2024
We employ a hybrid approach to construct prompt annotations: (1) manual annotations that capture human perceptions of speaker characteristics and (2) synthetic annotations on speaking style.
OmniCorpus: A Unified Multimodal Corpus of 10 Billion-Level Images Interleaved with Text
opengvlab/omnicorpus • 12 Jun 2024
In this paper, we introduce OmniCorpus, a 10 billion-scale image-text interleaved dataset.
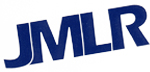
Frequently Asked Questions
Journal of Machine Learning Research
The Journal of Machine Learning Research (JMLR), established in 2000 , provides an international forum for the electronic and paper publication of high-quality scholarly articles in all areas of machine learning. All published papers are freely available online.
- 2024.02.18 : Volume 24 completed; Volume 25 began.
- 2023.01.20 : Volume 23 completed; Volume 24 began.
- 2022.07.20 : New special issue on climate change .
- 2022.02.18 : New blog post: Retrospectives from 20 Years of JMLR .
- 2022.01.25 : Volume 22 completed; Volume 23 began.
- 2021.12.02 : Message from outgoing co-EiC Bernhard Schölkopf .
- 2021.02.10 : Volume 21 completed; Volume 22 began.
- More news ...
Latest papers
Optimal Locally Private Nonparametric Classification with Public Data Yuheng Ma, Hanfang Yang , 2024. [ abs ][ pdf ][ bib ] [ code ]
Learning to Warm-Start Fixed-Point Optimization Algorithms Rajiv Sambharya, Georgina Hall, Brandon Amos, Bartolomeo Stellato , 2024. [ abs ][ pdf ][ bib ] [ code ]
Nonparametric Regression Using Over-parameterized Shallow ReLU Neural Networks Yunfei Yang, Ding-Xuan Zhou , 2024. [ abs ][ pdf ][ bib ]
Nonparametric Copula Models for Multivariate, Mixed, and Missing Data Joseph Feldman, Daniel R. Kowal , 2024. [ abs ][ pdf ][ bib ] [ code ]
An Analysis of Quantile Temporal-Difference Learning Mark Rowland, Rémi Munos, Mohammad Gheshlaghi Azar, Yunhao Tang, Georg Ostrovski, Anna Harutyunyan, Karl Tuyls, Marc G. Bellemare, Will Dabney , 2024. [ abs ][ pdf ][ bib ]
Conformal Inference for Online Prediction with Arbitrary Distribution Shifts Isaac Gibbs, Emmanuel J. Candès , 2024. [ abs ][ pdf ][ bib ] [ code ]
More Efficient Estimation of Multivariate Additive Models Based on Tensor Decomposition and Penalization Xu Liu, Heng Lian, Jian Huang , 2024. [ abs ][ pdf ][ bib ]
A Kernel Test for Causal Association via Noise Contrastive Backdoor Adjustment Robert Hu, Dino Sejdinovic, Robin J. Evans , 2024. [ abs ][ pdf ][ bib ] [ code ]
Assessing the Overall and Partial Causal Well-Specification of Nonlinear Additive Noise Models Christoph Schultheiss, Peter Bühlmann , 2024. [ abs ][ pdf ][ bib ] [ code ]
Simple Cycle Reservoirs are Universal Boyu Li, Robert Simon Fong, Peter Tino , 2024. [ abs ][ pdf ][ bib ]
On the Computational Complexity of Metropolis-Adjusted Langevin Algorithms for Bayesian Posterior Sampling Rong Tang, Yun Yang , 2024. [ abs ][ pdf ][ bib ]
Generalization and Stability of Interpolating Neural Networks with Minimal Width Hossein Taheri, Christos Thrampoulidis , 2024. [ abs ][ pdf ][ bib ]
Statistical Optimality of Divide and Conquer Kernel-based Functional Linear Regression Jiading Liu, Lei Shi , 2024. [ abs ][ pdf ][ bib ]
Identifiability and Asymptotics in Learning Homogeneous Linear ODE Systems from Discrete Observations Yuanyuan Wang, Wei Huang, Mingming Gong, Xi Geng, Tongliang Liu, Kun Zhang, Dacheng Tao , 2024. [ abs ][ pdf ][ bib ]
Robust Black-Box Optimization for Stochastic Search and Episodic Reinforcement Learning Maximilian Hüttenrauch, Gerhard Neumann , 2024. [ abs ][ pdf ][ bib ]
Kernel Thinning Raaz Dwivedi, Lester Mackey , 2024. [ abs ][ pdf ][ bib ] [ code ]
Optimal Algorithms for Stochastic Bilevel Optimization under Relaxed Smoothness Conditions Xuxing Chen, Tesi Xiao, Krishnakumar Balasubramanian , 2024. [ abs ][ pdf ][ bib ]
Variational Estimators of the Degree-corrected Latent Block Model for Bipartite Networks Yunpeng Zhao, Ning Hao, Ji Zhu , 2024. [ abs ][ pdf ][ bib ]
Statistical Inference for Fairness Auditing John J. Cherian, Emmanuel J. Candès , 2024. [ abs ][ pdf ][ bib ] [ code ]
Adjusted Wasserstein Distributionally Robust Estimator in Statistical Learning Yiling Xie, Xiaoming Huo , 2024. [ abs ][ pdf ][ bib ]
DoWhy-GCM: An Extension of DoWhy for Causal Inference in Graphical Causal Models Patrick Blöbaum, Peter Götz, Kailash Budhathoki, Atalanti A. Mastakouri, Dominik Janzing , 2024. (Machine Learning Open Source Software Paper) [ abs ][ pdf ][ bib ] [ code ]
Flexible Bayesian Product Mixture Models for Vector Autoregressions Suprateek Kundu, Joshua Lukemire , 2024. [ abs ][ pdf ][ bib ]
A Variational Approach to Bayesian Phylogenetic Inference Cheng Zhang, Frederick A. Matsen IV , 2024. [ abs ][ pdf ][ bib ] [ code ]
Fat-Shattering Dimension of k-fold Aggregations Idan Attias, Aryeh Kontorovich , 2024. [ abs ][ pdf ][ bib ]
Unified Binary and Multiclass Margin-Based Classification Yutong Wang, Clayton Scott , 2024. [ abs ][ pdf ][ bib ]
Neural Feature Learning in Function Space Xiangxiang Xu, Lizhong Zheng , 2024. [ abs ][ pdf ][ bib ] [ code ]
PyGOD: A Python Library for Graph Outlier Detection Kay Liu, Yingtong Dou, Xueying Ding, Xiyang Hu, Ruitong Zhang, Hao Peng, Lichao Sun, Philip S. Yu , 2024. (Machine Learning Open Source Software Paper) [ abs ][ pdf ][ bib ] [ code ]
Blessings and Curses of Covariate Shifts: Adversarial Learning Dynamics, Directional Convergence, and Equilibria Tengyuan Liang , 2024. [ abs ][ pdf ][ bib ]
Fixed points of nonnegative neural networks Tomasz J. Piotrowski, Renato L. G. Cavalcante, Mateusz Gabor , 2024. [ abs ][ pdf ][ bib ] [ code ]
Learning with Norm Constrained, Over-parameterized, Two-layer Neural Networks Fanghui Liu, Leello Dadi, Volkan Cevher , 2024. [ abs ][ pdf ][ bib ]
A Survey on Multi-player Bandits Etienne Boursier, Vianney Perchet , 2024. [ abs ][ pdf ][ bib ]
Transport-based Counterfactual Models Lucas De Lara, Alberto González-Sanz, Nicholas Asher, Laurent Risser, Jean-Michel Loubes , 2024. [ abs ][ pdf ][ bib ] [ code ]
Adaptive Latent Feature Sharing for Piecewise Linear Dimensionality Reduction Adam Farooq, Yordan P. Raykov, Petar Raykov, Max A. Little , 2024. [ abs ][ pdf ][ bib ] [ code ]
Topological Node2vec: Enhanced Graph Embedding via Persistent Homology Yasuaki Hiraoka, Yusuke Imoto, Théo Lacombe, Killian Meehan, Toshiaki Yachimura , 2024. [ abs ][ pdf ][ bib ] [ code ]
Granger Causal Inference in Multivariate Hawkes Processes by Minimum Message Length Katerina Hlaváčková-Schindler, Anna Melnykova, Irene Tubikanec , 2024. [ abs ][ pdf ][ bib ] [ code ]
Representation Learning via Manifold Flattening and Reconstruction Michael Psenka, Druv Pai, Vishal Raman, Shankar Sastry, Yi Ma , 2024. [ abs ][ pdf ][ bib ] [ code ]
Bagging Provides Assumption-free Stability Jake A. Soloff, Rina Foygel Barber, Rebecca Willett , 2024. [ abs ][ pdf ][ bib ] [ code ]
Fairness guarantees in multi-class classification with demographic parity Christophe Denis, Romuald Elie, Mohamed Hebiri, François Hu , 2024. [ abs ][ pdf ][ bib ]
Regimes of No Gain in Multi-class Active Learning Gan Yuan, Yunfan Zhao, Samory Kpotufe , 2024. [ abs ][ pdf ][ bib ]
Learning Optimal Dynamic Treatment Regimens Subject to Stagewise Risk Controls Mochuan Liu, Yuanjia Wang, Haoda Fu, Donglin Zeng , 2024. [ abs ][ pdf ][ bib ]
Margin-Based Active Learning of Classifiers Marco Bressan, Nicolò Cesa-Bianchi, Silvio Lattanzi, Andrea Paudice , 2024. [ abs ][ pdf ][ bib ]
Random Subgraph Detection Using Queries Wasim Huleihel, Arya Mazumdar, Soumyabrata Pal , 2024. [ abs ][ pdf ][ bib ]
Classification with Deep Neural Networks and Logistic Loss Zihan Zhang, Lei Shi, Ding-Xuan Zhou , 2024. [ abs ][ pdf ][ bib ]
Spectral learning of multivariate extremes Marco Avella Medina, Richard A Davis, Gennady Samorodnitsky , 2024. [ abs ][ pdf ][ bib ]
Sum-of-norms clustering does not separate nearby balls Alexander Dunlap, Jean-Christophe Mourrat , 2024. [ abs ][ pdf ][ bib ] [ code ]
An Algorithm with Optimal Dimension-Dependence for Zero-Order Nonsmooth Nonconvex Stochastic Optimization Guy Kornowski, Ohad Shamir , 2024. [ abs ][ pdf ][ bib ]
Linear Distance Metric Learning with Noisy Labels Meysam Alishahi, Anna Little, Jeff M. Phillips , 2024. [ abs ][ pdf ][ bib ] [ code ]
OpenBox: A Python Toolkit for Generalized Black-box Optimization Huaijun Jiang, Yu Shen, Yang Li, Beicheng Xu, Sixian Du, Wentao Zhang, Ce Zhang, Bin Cui , 2024. (Machine Learning Open Source Software Paper) [ abs ][ pdf ][ bib ] [ code ]
Generative Adversarial Ranking Nets Yinghua Yao, Yuangang Pan, Jing Li, Ivor W. Tsang, Xin Yao , 2024. [ abs ][ pdf ][ bib ] [ code ]
Predictive Inference with Weak Supervision Maxime Cauchois, Suyash Gupta, Alnur Ali, John C. Duchi , 2024. [ abs ][ pdf ][ bib ]
Functions with average smoothness: structure, algorithms, and learning Yair Ashlagi, Lee-Ad Gottlieb, Aryeh Kontorovich , 2024. [ abs ][ pdf ][ bib ]
Differentially Private Data Release for Mixed-type Data via Latent Factor Models Yanqing Zhang, Qi Xu, Niansheng Tang, Annie Qu , 2024. [ abs ][ pdf ][ bib ]
The Non-Overlapping Statistical Approximation to Overlapping Group Lasso Mingyu Qi, Tianxi Li , 2024. [ abs ][ pdf ][ bib ] [ code ]
Faster Rates of Differentially Private Stochastic Convex Optimization Jinyan Su, Lijie Hu, Di Wang , 2024. [ abs ][ pdf ][ bib ]
Nonasymptotic analysis of Stochastic Gradient Hamiltonian Monte Carlo under local conditions for nonconvex optimization O. Deniz Akyildiz, Sotirios Sabanis , 2024. [ abs ][ pdf ][ bib ]
Finite-time Analysis of Globally Nonstationary Multi-Armed Bandits Junpei Komiyama, Edouard Fouché, Junya Honda , 2024. [ abs ][ pdf ][ bib ] [ code ]
Stable Implementation of Probabilistic ODE Solvers Nicholas Krämer, Philipp Hennig , 2024. [ abs ][ pdf ][ bib ]
More PAC-Bayes bounds: From bounded losses, to losses with general tail behaviors, to anytime validity Borja Rodríguez-Gálvez, Ragnar Thobaben, Mikael Skoglund , 2024. [ abs ][ pdf ][ bib ]
Neural Hilbert Ladders: Multi-Layer Neural Networks in Function Space Zhengdao Chen , 2024. [ abs ][ pdf ][ bib ]
QDax: A Library for Quality-Diversity and Population-based Algorithms with Hardware Acceleration Felix Chalumeau, Bryan Lim, Raphaël Boige, Maxime Allard, Luca Grillotti, Manon Flageat, Valentin Macé, Guillaume Richard, Arthur Flajolet, Thomas Pierrot, Antoine Cully , 2024. (Machine Learning Open Source Software Paper) [ abs ][ pdf ][ bib ] [ code ]
Random Forest Weighted Local Fréchet Regression with Random Objects Rui Qiu, Zhou Yu, Ruoqing Zhu , 2024. [ abs ][ pdf ][ bib ] [ code ]
PhAST: Physics-Aware, Scalable, and Task-Specific GNNs for Accelerated Catalyst Design Alexandre Duval, Victor Schmidt, Santiago Miret, Yoshua Bengio, Alex Hernández-García, David Rolnick , 2024. [ abs ][ pdf ][ bib ] [ code ]
Unsupervised Anomaly Detection Algorithms on Real-world Data: How Many Do We Need? Roel Bouman, Zaharah Bukhsh, Tom Heskes , 2024. [ abs ][ pdf ][ bib ] [ code ]
Multi-class Probabilistic Bounds for Majority Vote Classifiers with Partially Labeled Data Vasilii Feofanov, Emilie Devijver, Massih-Reza Amini , 2024. [ abs ][ pdf ][ bib ]
Information Processing Equalities and the Information–Risk Bridge Robert C. Williamson, Zac Cranko , 2024. [ abs ][ pdf ][ bib ]
Nonparametric Regression for 3D Point Cloud Learning Xinyi Li, Shan Yu, Yueying Wang, Guannan Wang, Li Wang, Ming-Jun Lai , 2024. [ abs ][ pdf ][ bib ] [ code ]
AMLB: an AutoML Benchmark Pieter Gijsbers, Marcos L. P. Bueno, Stefan Coors, Erin LeDell, Sébastien Poirier, Janek Thomas, Bernd Bischl, Joaquin Vanschoren , 2024. [ abs ][ pdf ][ bib ] [ code ]
Materials Discovery using Max K-Armed Bandit Nobuaki Kikkawa, Hiroshi Ohno , 2024. [ abs ][ pdf ][ bib ]
Semi-supervised Inference for Block-wise Missing Data without Imputation Shanshan Song, Yuanyuan Lin, Yong Zhou , 2024. [ abs ][ pdf ][ bib ]
Adaptivity and Non-stationarity: Problem-dependent Dynamic Regret for Online Convex Optimization Peng Zhao, Yu-Jie Zhang, Lijun Zhang, Zhi-Hua Zhou , 2024. [ abs ][ pdf ][ bib ]
Scaling Speech Technology to 1,000+ Languages Vineel Pratap, Andros Tjandra, Bowen Shi, Paden Tomasello, Arun Babu, Sayani Kundu, Ali Elkahky, Zhaoheng Ni, Apoorv Vyas, Maryam Fazel-Zarandi, Alexei Baevski, Yossi Adi, Xiaohui Zhang, Wei-Ning Hsu, Alexis Conneau, Michael Auli , 2024. [ abs ][ pdf ][ bib ] [ code ]
MAP- and MLE-Based Teaching Hans Ulrich Simon, Jan Arne Telle , 2024. [ abs ][ pdf ][ bib ]
A General Framework for the Analysis of Kernel-based Tests Tamara Fernández, Nicolás Rivera , 2024. [ abs ][ pdf ][ bib ]
Overparametrized Multi-layer Neural Networks: Uniform Concentration of Neural Tangent Kernel and Convergence of Stochastic Gradient Descent Jiaming Xu, Hanjing Zhu , 2024. [ abs ][ pdf ][ bib ]
Sparse Representer Theorems for Learning in Reproducing Kernel Banach Spaces Rui Wang, Yuesheng Xu, Mingsong Yan , 2024. [ abs ][ pdf ][ bib ]
Exploration of the Search Space of Gaussian Graphical Models for Paired Data Alberto Roverato, Dung Ngoc Nguyen , 2024. [ abs ][ pdf ][ bib ]
The good, the bad and the ugly sides of data augmentation: An implicit spectral regularization perspective Chi-Heng Lin, Chiraag Kaushik, Eva L. Dyer, Vidya Muthukumar , 2024. [ abs ][ pdf ][ bib ] [ code ]
Stochastic Approximation with Decision-Dependent Distributions: Asymptotic Normality and Optimality Joshua Cutler, Mateo Díaz, Dmitriy Drusvyatskiy , 2024. [ abs ][ pdf ][ bib ]
Minimax Rates for High-Dimensional Random Tessellation Forests Eliza O'Reilly, Ngoc Mai Tran , 2024. [ abs ][ pdf ][ bib ]
Nonparametric Estimation of Non-Crossing Quantile Regression Process with Deep ReQU Neural Networks Guohao Shen, Yuling Jiao, Yuanyuan Lin, Joel L. Horowitz, Jian Huang , 2024. [ abs ][ pdf ][ bib ]
Spatial meshing for general Bayesian multivariate models Michele Peruzzi, David B. Dunson , 2024. [ abs ][ pdf ][ bib ] [ code ]
A Semi-parametric Estimation of Personalized Dose-response Function Using Instrumental Variables Wei Luo, Yeying Zhu, Xuekui Zhang, Lin Lin , 2024. [ abs ][ pdf ][ bib ]
Learning Non-Gaussian Graphical Models via Hessian Scores and Triangular Transport Ricardo Baptista, Rebecca Morrison, Olivier Zahm, Youssef Marzouk , 2024. [ abs ][ pdf ][ bib ] [ code ]
On the Learnability of Out-of-distribution Detection Zhen Fang, Yixuan Li, Feng Liu, Bo Han, Jie Lu , 2024. [ abs ][ pdf ][ bib ]
Win: Weight-Decay-Integrated Nesterov Acceleration for Faster Network Training Pan Zhou, Xingyu Xie, Zhouchen Lin, Kim-Chuan Toh, Shuicheng Yan , 2024. [ abs ][ pdf ][ bib ] [ code ]
On the Eigenvalue Decay Rates of a Class of Neural-Network Related Kernel Functions Defined on General Domains Yicheng Li, Zixiong Yu, Guhan Chen, Qian Lin , 2024. [ abs ][ pdf ][ bib ]
Tight Convergence Rate Bounds for Optimization Under Power Law Spectral Conditions Maksim Velikanov, Dmitry Yarotsky , 2024. [ abs ][ pdf ][ bib ]
ptwt - The PyTorch Wavelet Toolbox Moritz Wolter, Felix Blanke, Jochen Garcke, Charles Tapley Hoyt , 2024. (Machine Learning Open Source Software Paper) [ abs ][ pdf ][ bib ] [ code ]
Choosing the Number of Topics in LDA Models – A Monte Carlo Comparison of Selection Criteria Victor Bystrov, Viktoriia Naboka-Krell, Anna Staszewska-Bystrova, Peter Winker , 2024. [ abs ][ pdf ][ bib ] [ code ]
Functional Directed Acyclic Graphs Kuang-Yao Lee, Lexin Li, Bing Li , 2024. [ abs ][ pdf ][ bib ]
Unlabeled Principal Component Analysis and Matrix Completion Yunzhen Yao, Liangzu Peng, Manolis C. Tsakiris , 2024. [ abs ][ pdf ][ bib ] [ code ]
Distributed Estimation on Semi-Supervised Generalized Linear Model Jiyuan Tu, Weidong Liu, Xiaojun Mao , 2024. [ abs ][ pdf ][ bib ]
Towards Explainable Evaluation Metrics for Machine Translation Christoph Leiter, Piyawat Lertvittayakumjorn, Marina Fomicheva, Wei Zhao, Yang Gao, Steffen Eger , 2024. [ abs ][ pdf ][ bib ]
Differentially private methods for managing model uncertainty in linear regression Víctor Peña, Andrés F. Barrientos , 2024. [ abs ][ pdf ][ bib ]
Data Summarization via Bilevel Optimization Zalán Borsos, Mojmír Mutný, Marco Tagliasacchi, Andreas Krause , 2024. [ abs ][ pdf ][ bib ]
Pareto Smoothed Importance Sampling Aki Vehtari, Daniel Simpson, Andrew Gelman, Yuling Yao, Jonah Gabry , 2024. [ abs ][ pdf ][ bib ] [ code ]
Policy Gradient Methods in the Presence of Symmetries and State Abstractions Prakash Panangaden, Sahand Rezaei-Shoshtari, Rosie Zhao, David Meger, Doina Precup , 2024. [ abs ][ pdf ][ bib ] [ code ]
Scaling Instruction-Finetuned Language Models Hyung Won Chung, Le Hou, Shayne Longpre, Barret Zoph, Yi Tay, William Fedus, Yunxuan Li, Xuezhi Wang, Mostafa Dehghani, Siddhartha Brahma, Albert Webson, Shixiang Shane Gu, Zhuyun Dai, Mirac Suzgun, Xinyun Chen, Aakanksha Chowdhery, Alex Castro-Ros, Marie Pellat, Kevin Robinson, Dasha Valter, Sharan Narang, Gaurav Mishra, Adams Yu, Vincent Zhao, Yanping Huang, Andrew Dai, Hongkun Yu, Slav Petrov, Ed H. Chi, Jeff Dean, Jacob Devlin, Adam Roberts, Denny Zhou, Quoc V. Le, Jason Wei , 2024. [ abs ][ pdf ][ bib ]
Tangential Wasserstein Projections Florian Gunsilius, Meng Hsuan Hsieh, Myung Jin Lee , 2024. [ abs ][ pdf ][ bib ] [ code ]
Learnability of Linear Port-Hamiltonian Systems Juan-Pablo Ortega, Daiying Yin , 2024. [ abs ][ pdf ][ bib ] [ code ]
Off-Policy Action Anticipation in Multi-Agent Reinforcement Learning Ariyan Bighashdel, Daan de Geus, Pavol Jancura, Gijs Dubbelman , 2024. [ abs ][ pdf ][ bib ] [ code ]
On Unbiased Estimation for Partially Observed Diffusions Jeremy Heng, Jeremie Houssineau, Ajay Jasra , 2024. (Machine Learning Open Source Software Paper) [ abs ][ pdf ][ bib ] [ code ]
Improving Lipschitz-Constrained Neural Networks by Learning Activation Functions Stanislas Ducotterd, Alexis Goujon, Pakshal Bohra, Dimitris Perdios, Sebastian Neumayer, Michael Unser , 2024. [ abs ][ pdf ][ bib ] [ code ]
Mathematical Framework for Online Social Media Auditing Wasim Huleihel, Yehonathan Refael , 2024. [ abs ][ pdf ][ bib ]
An Embedding Framework for the Design and Analysis of Consistent Polyhedral Surrogates Jessie Finocchiaro, Rafael M. Frongillo, Bo Waggoner , 2024. [ abs ][ pdf ][ bib ]
Low-rank Variational Bayes correction to the Laplace method Janet van Niekerk, Haavard Rue , 2024. [ abs ][ pdf ][ bib ] [ code ]
Scaling the Convex Barrier with Sparse Dual Algorithms Alessandro De Palma, Harkirat Singh Behl, Rudy Bunel, Philip H.S. Torr, M. Pawan Kumar , 2024. [ abs ][ pdf ][ bib ] [ code ]
Causal-learn: Causal Discovery in Python Yujia Zheng, Biwei Huang, Wei Chen, Joseph Ramsey, Mingming Gong, Ruichu Cai, Shohei Shimizu, Peter Spirtes, Kun Zhang , 2024. (Machine Learning Open Source Software Paper) [ abs ][ pdf ][ bib ] [ code ]
Decomposed Linear Dynamical Systems (dLDS) for learning the latent components of neural dynamics Noga Mudrik, Yenho Chen, Eva Yezerets, Christopher J. Rozell, Adam S. Charles , 2024. [ abs ][ pdf ][ bib ] [ code ]
Existence and Minimax Theorems for Adversarial Surrogate Risks in Binary Classification Natalie S. Frank, Jonathan Niles-Weed , 2024. [ abs ][ pdf ][ bib ]
Data Thinning for Convolution-Closed Distributions Anna Neufeld, Ameer Dharamshi, Lucy L. Gao, Daniela Witten , 2024. [ abs ][ pdf ][ bib ] [ code ]
A projected semismooth Newton method for a class of nonconvex composite programs with strong prox-regularity Jiang Hu, Kangkang Deng, Jiayuan Wu, Quanzheng Li , 2024. [ abs ][ pdf ][ bib ]
Revisiting RIP Guarantees for Sketching Operators on Mixture Models Ayoub Belhadji, Rémi Gribonval , 2024. [ abs ][ pdf ][ bib ]
Monotonic Risk Relationships under Distribution Shifts for Regularized Risk Minimization Daniel LeJeune, Jiayu Liu, Reinhard Heckel , 2024. [ abs ][ pdf ][ bib ] [ code ]
Polygonal Unadjusted Langevin Algorithms: Creating stable and efficient adaptive algorithms for neural networks Dong-Young Lim, Sotirios Sabanis , 2024. [ abs ][ pdf ][ bib ]
Axiomatic effect propagation in structural causal models Raghav Singal, George Michailidis , 2024. [ abs ][ pdf ][ bib ]
Optimal First-Order Algorithms as a Function of Inequalities Chanwoo Park, Ernest K. Ryu , 2024. [ abs ][ pdf ][ bib ] [ code ]
Resource-Efficient Neural Networks for Embedded Systems Wolfgang Roth, Günther Schindler, Bernhard Klein, Robert Peharz, Sebastian Tschiatschek, Holger Fröning, Franz Pernkopf, Zoubin Ghahramani , 2024. [ abs ][ pdf ][ bib ]
Trained Transformers Learn Linear Models In-Context Ruiqi Zhang, Spencer Frei, Peter L. Bartlett , 2024. [ abs ][ pdf ][ bib ]
Adam-family Methods for Nonsmooth Optimization with Convergence Guarantees Nachuan Xiao, Xiaoyin Hu, Xin Liu, Kim-Chuan Toh , 2024. [ abs ][ pdf ][ bib ]
Efficient Modality Selection in Multimodal Learning Yifei He, Runxiang Cheng, Gargi Balasubramaniam, Yao-Hung Hubert Tsai, Han Zhao , 2024. [ abs ][ pdf ][ bib ]
A Multilabel Classification Framework for Approximate Nearest Neighbor Search Ville Hyvönen, Elias Jääsaari, Teemu Roos , 2024. [ abs ][ pdf ][ bib ] [ code ]
Probabilistic Forecasting with Generative Networks via Scoring Rule Minimization Lorenzo Pacchiardi, Rilwan A. Adewoyin, Peter Dueben, Ritabrata Dutta , 2024. [ abs ][ pdf ][ bib ] [ code ]
Multiple Descent in the Multiple Random Feature Model Xuran Meng, Jianfeng Yao, Yuan Cao , 2024. [ abs ][ pdf ][ bib ]
Mean-Square Analysis of Discretized Itô Diffusions for Heavy-tailed Sampling Ye He, Tyler Farghly, Krishnakumar Balasubramanian, Murat A. Erdogdu , 2024. [ abs ][ pdf ][ bib ]
Invariant and Equivariant Reynolds Networks Akiyoshi Sannai, Makoto Kawano, Wataru Kumagai , 2024. (Machine Learning Open Source Software Paper) [ abs ][ pdf ][ bib ] [ code ]
Personalized PCA: Decoupling Shared and Unique Features Naichen Shi, Raed Al Kontar , 2024. [ abs ][ pdf ][ bib ] [ code ]
Survival Kernets: Scalable and Interpretable Deep Kernel Survival Analysis with an Accuracy Guarantee George H. Chen , 2024. [ abs ][ pdf ][ bib ] [ code ]
On the Sample Complexity and Metastability of Heavy-tailed Policy Search in Continuous Control Amrit Singh Bedi, Anjaly Parayil, Junyu Zhang, Mengdi Wang, Alec Koppel , 2024. [ abs ][ pdf ][ bib ]
Convergence for nonconvex ADMM, with applications to CT imaging Rina Foygel Barber, Emil Y. Sidky , 2024. [ abs ][ pdf ][ bib ] [ code ]
Distributed Gaussian Mean Estimation under Communication Constraints: Optimal Rates and Communication-Efficient Algorithms T. Tony Cai, Hongji Wei , 2024. [ abs ][ pdf ][ bib ]
Sparse NMF with Archetypal Regularization: Computational and Robustness Properties Kayhan Behdin, Rahul Mazumder , 2024. [ abs ][ pdf ][ bib ] [ code ]
Deep Network Approximation: Beyond ReLU to Diverse Activation Functions Shijun Zhang, Jianfeng Lu, Hongkai Zhao , 2024. [ abs ][ pdf ][ bib ]
Effect-Invariant Mechanisms for Policy Generalization Sorawit Saengkyongam, Niklas Pfister, Predrag Klasnja, Susan Murphy, Jonas Peters , 2024. [ abs ][ pdf ][ bib ]
Pygmtools: A Python Graph Matching Toolkit Runzhong Wang, Ziao Guo, Wenzheng Pan, Jiale Ma, Yikai Zhang, Nan Yang, Qi Liu, Longxuan Wei, Hanxue Zhang, Chang Liu, Zetian Jiang, Xiaokang Yang, Junchi Yan , 2024. (Machine Learning Open Source Software Paper) [ abs ][ pdf ][ bib ] [ code ]
Heterogeneous-Agent Reinforcement Learning Yifan Zhong, Jakub Grudzien Kuba, Xidong Feng, Siyi Hu, Jiaming Ji, Yaodong Yang , 2024. [ abs ][ pdf ][ bib ] [ code ]
Sample-efficient Adversarial Imitation Learning Dahuin Jung, Hyungyu Lee, Sungroh Yoon , 2024. [ abs ][ pdf ][ bib ]
Stochastic Modified Flows, Mean-Field Limits and Dynamics of Stochastic Gradient Descent Benjamin Gess, Sebastian Kassing, Vitalii Konarovskyi , 2024. [ abs ][ pdf ][ bib ]
Rates of convergence for density estimation with generative adversarial networks Nikita Puchkin, Sergey Samsonov, Denis Belomestny, Eric Moulines, Alexey Naumov , 2024. [ abs ][ pdf ][ bib ]
Additive smoothing error in backward variational inference for general state-space models Mathis Chagneux, Elisabeth Gassiat, Pierre Gloaguen, Sylvain Le Corff , 2024. [ abs ][ pdf ][ bib ]
Optimal Bump Functions for Shallow ReLU networks: Weight Decay, Depth Separation, Curse of Dimensionality Stephan Wojtowytsch , 2024. [ abs ][ pdf ][ bib ]
Numerically Stable Sparse Gaussian Processes via Minimum Separation using Cover Trees Alexander Terenin, David R. Burt, Artem Artemev, Seth Flaxman, Mark van der Wilk, Carl Edward Rasmussen, Hong Ge , 2024. [ abs ][ pdf ][ bib ] [ code ]
On Tail Decay Rate Estimation of Loss Function Distributions Etrit Haxholli, Marco Lorenzi , 2024. [ abs ][ pdf ][ bib ] [ code ]
Deep Nonparametric Estimation of Operators between Infinite Dimensional Spaces Hao Liu, Haizhao Yang, Minshuo Chen, Tuo Zhao, Wenjing Liao , 2024. [ abs ][ pdf ][ bib ]
Post-Regularization Confidence Bands for Ordinary Differential Equations Xiaowu Dai, Lexin Li , 2024. [ abs ][ pdf ][ bib ]
On the Generalization of Stochastic Gradient Descent with Momentum Ali Ramezani-Kebrya, Kimon Antonakopoulos, Volkan Cevher, Ashish Khisti, Ben Liang , 2024. [ abs ][ pdf ][ bib ]
Pursuit of the Cluster Structure of Network Lasso: Recovery Condition and Non-convex Extension Shotaro Yagishita, Jun-ya Gotoh , 2024. [ abs ][ pdf ][ bib ]
Iterate Averaging in the Quest for Best Test Error Diego Granziol, Nicholas P. Baskerville, Xingchen Wan, Samuel Albanie, Stephen Roberts , 2024. [ abs ][ pdf ][ bib ] [ code ]
Nonparametric Inference under B-bits Quantization Kexuan Li, Ruiqi Liu, Ganggang Xu, Zuofeng Shang , 2024. [ abs ][ pdf ][ bib ]
Black Box Variational Inference with a Deterministic Objective: Faster, More Accurate, and Even More Black Box Ryan Giordano, Martin Ingram, Tamara Broderick , 2024. [ abs ][ pdf ][ bib ] [ code ]
On Sufficient Graphical Models Bing Li, Kyongwon Kim , 2024. [ abs ][ pdf ][ bib ]
Localized Debiased Machine Learning: Efficient Inference on Quantile Treatment Effects and Beyond Nathan Kallus, Xiaojie Mao, Masatoshi Uehara , 2024. [ abs ][ pdf ][ bib ] [ code ]
On the Effect of Initialization: The Scaling Path of 2-Layer Neural Networks Sebastian Neumayer, Lénaïc Chizat, Michael Unser , 2024. [ abs ][ pdf ][ bib ]
Improving physics-informed neural networks with meta-learned optimization Alex Bihlo , 2024. [ abs ][ pdf ][ bib ]
A Comparison of Continuous-Time Approximations to Stochastic Gradient Descent Stefan Ankirchner, Stefan Perko , 2024. [ abs ][ pdf ][ bib ]
Critically Assessing the State of the Art in Neural Network Verification Matthias König, Annelot W. Bosman, Holger H. Hoos, Jan N. van Rijn , 2024. [ abs ][ pdf ][ bib ]
Estimating the Minimizer and the Minimum Value of a Regression Function under Passive Design Arya Akhavan, Davit Gogolashvili, Alexandre B. Tsybakov , 2024. [ abs ][ pdf ][ bib ]
Modeling Random Networks with Heterogeneous Reciprocity Daniel Cirkovic, Tiandong Wang , 2024. [ abs ][ pdf ][ bib ]
Exploration, Exploitation, and Engagement in Multi-Armed Bandits with Abandonment Zixian Yang, Xin Liu, Lei Ying , 2024. [ abs ][ pdf ][ bib ]
On Efficient and Scalable Computation of the Nonparametric Maximum Likelihood Estimator in Mixture Models Yangjing Zhang, Ying Cui, Bodhisattva Sen, Kim-Chuan Toh , 2024. [ abs ][ pdf ][ bib ] [ code ]
Decorrelated Variable Importance Isabella Verdinelli, Larry Wasserman , 2024. [ abs ][ pdf ][ bib ]
Model-Free Representation Learning and Exploration in Low-Rank MDPs Aditya Modi, Jinglin Chen, Akshay Krishnamurthy, Nan Jiang, Alekh Agarwal , 2024. [ abs ][ pdf ][ bib ]
Seeded Graph Matching for the Correlated Gaussian Wigner Model via the Projected Power Method Ernesto Araya, Guillaume Braun, Hemant Tyagi , 2024. [ abs ][ pdf ][ bib ] [ code ]
Fast Policy Extragradient Methods for Competitive Games with Entropy Regularization Shicong Cen, Yuting Wei, Yuejie Chi , 2024. [ abs ][ pdf ][ bib ]
Power of knockoff: The impact of ranking algorithm, augmented design, and symmetric statistic Zheng Tracy Ke, Jun S. Liu, Yucong Ma , 2024. [ abs ][ pdf ][ bib ]
Lower Complexity Bounds of Finite-Sum Optimization Problems: The Results and Construction Yuze Han, Guangzeng Xie, Zhihua Zhang , 2024. [ abs ][ pdf ][ bib ]
On Truthing Issues in Supervised Classification Jonathan K. Su , 2024. [ abs ][ pdf ][ bib ]
2024. |
Help | Advanced Search
Machine Learning
Authors and titles for recent submissions.
- Mon, 17 Jun 2024
- Fri, 14 Jun 2024
- Thu, 13 Jun 2024
- Wed, 12 Jun 2024
- Tue, 11 Jun 2024
See today's new changes
Mon, 17 Jun 2024 (showing 15 of 15 entries )
Fri, 14 jun 2024 (showing first 10 of 34 entries ).

- Advanced Search
The Journal of Machine Learning Research
Volume 24, Issue 1
January 2023
- new algorithms with empirical, theoretical, psychological, or biological justification;
- experimental and/or theoretical studies yielding new insight into the design and behavior of learning in intelligent systems;
- accounts of applications of existing techniques that shed light on the strengths and weaknesses of the methods;
- formalization of new learning tasks (e.g., in the context of new applications) and of methods for assessing performance on those tasks;
- development of new analytical frameworks that advance theoretical studies of practical learning methods;
- computational models of data from natural learning systems at the behavioral or neural level; or
- extremely well-written surveys of existing work.
JMLR has a commitment to rigorous yet rapid reviewing. Final versions are published electronically (ISSN 1533-7928) immediately upon receipt. Printed volumes (ISSN: 1532-4435) are now published by Microtome Publishing and available for sale .
Subject Areas
Announcements.
ACM Updates Its Peer Review Policy
ACM is pleased to announce that its Publications Board has approved an updated Peer Review Policy . If you have any questions regarding the update, the associated FAQ addresses topics such as confidentiality, the use of large language models in the peer review process, conflicts of interest, and several other relevant concerns. If there are any issues that are not addressed in the FAQ, please contact ACM’s Director of Publications, Scott Delman .
New ACM Policy on Authorship ACM has a new Policy on Authorship , covering a range of key topics, including the use of generative AI tools. Please familiarize yourself with the new policy and the associated list of Frequently Asked Questions .
Most Frequent Affiliations
Most cited authors, latest issue.
- Volume 24, Issue 1 January 2023 ISSN: 1532-4435 EISSN: 1533-7928 View Table of Contents
The measure and mismeasure of fairness
Department of Statistics, Harvard University, Cambridge, MA
Department of Computer Science, Stanford University, Stanford, CA
Department of Applied Statistics, Social Science, and Humanities, New York University, New York, NY
Harvard Kennedy School, Harvard University, Cambridge, MA
Weisfeiler and Leman go machine learning: the story so far
Department of Computer Science, RWTH Aachen University, Aachen, Germany
Meta AI Research, Department of Computer Science and Applied Mathematics, Weizmann Institute of Science, Rehovot, Israel
NVIDIA Research, Tel Aviv, Israel

AIDOS Lab, Institute of AI for Health Helmholtz Zentrum München and Technical University of Munich Munich, Germany
Faculty of Computer Science and Research Network Data Science, University of Vienna, Vienna, Austria
Kumo.AI, Mountain View, CA
Machine Learning & Computational Biology Lab, Department of Biosystems Science and Engineering, ETH Zürich, Basel, Switzerland and Swiss Institute of Bioinformatics, Lausanne, Switzerland
Recent Award Winners
Most popular, other acm journals.

Volume 2, Issue 2

Volume 3, Issue 2
April-June 2024

Volume 56, Issue 10
October 2024

Volume 5, Issue 1

Volume 3, Issue 1

Volume 36, Issue 1
Export Citations
- Please download or close your previous search result export first before starting a new bulk export. Preview is not available. By clicking download, a status dialog will open to start the export process. The process may take a few minutes but once it finishes a file will be downloadable from your browser. You may continue to browse the DL while the export process is in progress. Download
- Download citation
- Copy citation
We are preparing your search results for download ...
We will inform you here when the file is ready.
Your file of search results citations is now ready.
Your search export query has expired. Please try again.
machine learning Recently Published Documents
Total documents.
- Latest Documents
- Most Cited Documents
- Contributed Authors
- Related Sources
- Related Keywords
An explainable machine learning model for identifying geographical origins of sea cucumber Apostichopus japonicus based on multi-element profile
A comparison of machine learning- and regression-based models for predicting ductility ratio of rc beam-column joints, alexa, is this a historical record.
Digital transformation in government has brought an increase in the scale, variety, and complexity of records and greater levels of disorganised data. Current practices for selecting records for transfer to The National Archives (TNA) were developed to deal with paper records and are struggling to deal with this shift. This article examines the background to the problem and outlines a project that TNA undertook to research the feasibility of using commercially available artificial intelligence tools to aid selection. The project AI for Selection evaluated a range of commercial solutions varying from off-the-shelf products to cloud-hosted machine learning platforms, as well as a benchmarking tool developed in-house. Suitability of tools depended on several factors, including requirements and skills of transferring bodies as well as the tools’ usability and configurability. This article also explores questions around trust and explainability of decisions made when using AI for sensitive tasks such as selection.
Automated Text Classification of Maintenance Data of Higher Education Buildings Using Text Mining and Machine Learning Techniques
Data-driven analysis and machine learning for energy prediction in distributed photovoltaic generation plants: a case study in queensland, australia, modeling nutrient removal by membrane bioreactor at a sewage treatment plant using machine learning models, big five personality prediction based in indonesian tweets using machine learning methods.
<span lang="EN-US">The popularity of social media has drawn the attention of researchers who have conducted cross-disciplinary studies examining the relationship between personality traits and behavior on social media. Most current work focuses on personality prediction analysis of English texts, but Indonesian has received scant attention. Therefore, this research aims to predict user’s personalities based on Indonesian text from social media using machine learning techniques. This paper evaluates several machine learning techniques, including <a name="_Hlk87278444"></a>naive Bayes (NB), K-nearest neighbors (KNN), and support vector machine (SVM), based on semantic features including emotion, sentiment, and publicly available Twitter profile. We predict the personality based on the big five personality model, the most appropriate model for predicting user personality in social media. We examine the relationships between the semantic features and the Big Five personality dimensions. The experimental results indicate that the Big Five personality exhibit distinct emotional, sentimental, and social characteristics and that SVM outperformed NB and KNN for Indonesian. In addition, we observe several terms in Indonesian that specifically refer to each personality type, each of which has distinct emotional, sentimental, and social features.</span>
Compressive strength of concrete with recycled aggregate; a machine learning-based evaluation
Temperature prediction of flat steel box girders of long-span bridges utilizing in situ environmental parameters and machine learning, computer-assisted cohort identification in practice.
The standard approach to expert-in-the-loop machine learning is active learning, where, repeatedly, an expert is asked to annotate one or more records and the machine finds a classifier that respects all annotations made until that point. We propose an alternative approach, IQRef , in which the expert iteratively designs a classifier and the machine helps him or her to determine how well it is performing and, importantly, when to stop, by reporting statistics on a fixed, hold-out sample of annotated records. We justify our approach based on prior work giving a theoretical model of how to re-use hold-out data. We compare the two approaches in the context of identifying a cohort of EHRs and examine their strengths and weaknesses through a case study arising from an optometric research problem. We conclude that both approaches are complementary, and we recommend that they both be employed in conjunction to address the problem of cohort identification in health research.
Export Citation Format
Share document.

Advertisement
Artificial intelligence and machine learning research: towards digital transformation at a global scale
- Published: 17 April 2021
- Volume 13 , pages 3319–3321, ( 2022 )
Cite this article
- Akila Sarirete 1 ,
- Zain Balfagih 1 ,
- Tayeb Brahimi 1 ,
- Miltiadis D. Lytras 1 , 2 &
- Anna Visvizi 3 , 4
15k Accesses
13 Citations
Explore all metrics
Avoid common mistakes on your manuscript.
Artificial intelligence (AI) is reshaping how we live, learn, and work. Until recently, AI used to be a fanciful concept, more closely associated with science fiction rather than with anything else. However, driven by unprecedented advances in sophisticated information and communication technology (ICT), AI today is synonymous technological progress already attained and the one yet to come in all spheres of our lives (Chui et al. 2018 ; Lytras et al. 2018 , 2019 ).
Considering that Machine Learning (ML) and AI are apt to reach unforeseen levels of accuracy and efficiency, this special issue sought to promote research on AI and ML seen as functions of data-driven innovation and digital transformation. The combination of expanding ICT-driven capabilities and capacities identifiable across our socio-economic systems along with growing consumer expectations vis-a-vis technology and its value-added for our societies, requires multidisciplinary research and research agenda on AI and ML (Lytras et al. 2021 ; Visvizi et al. 2020 ; Chui et al. 2020 ). Such a research agenda should oscilate around the following five defining issues (Fig. 1 ):
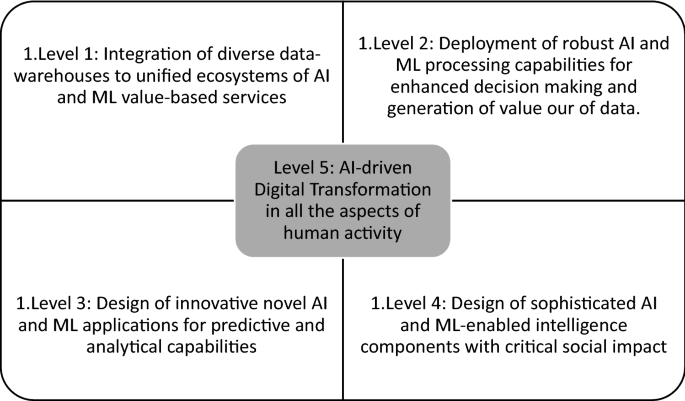
Source: The Authors
An AI-Driven Digital Transformation in all aspects of human activity/
Integration of diverse data-warehouses to unified ecosystems of AI and ML value-based services
Deployment of robust AI and ML processing capabilities for enhanced decision making and generation of value our of data.
Design of innovative novel AI and ML applications for predictive and analytical capabilities
Design of sophisticated AI and ML-enabled intelligence components with critical social impact
Promotion of the Digital Transformation in all the aspects of human activity including business, healthcare, government, commerce, social intelligence etc.
Such development will also have a critical impact on government, policies, regulations and initiatives aiming to interpret the value of the AI-driven digital transformation to the sustainable economic development of our planet. Additionally the disruptive character of AI and ML technology and research will required further research on business models and management of innovation capabilities.
This special issue is based on submissions invited from the 17th Annual Learning and Technology Conference 2019 that was held at Effat University and open call jointly. Several very good submissions were received. All of them were subjected a rigorous peer review process specific to the Ambient Intelligence and Humanized Computing Journal.
A variety of innovative topics are included in the agenda of the published papers in this special issue including topics such as:
Stock market Prediction using Machine learning
Detection of Apple Diseases and Pests based on Multi-Model LSTM-based Convolutional Neural Networks
ML for Searching
Machine Learning for Learning Automata
Entity recognition & Relation Extraction
Intelligent Surveillance Systems
Activity Recognition and K-Means Clustering
Distributed Mobility Management
Review Rating Prediction with Deep Learning
Cybersecurity: Botnet detection with Deep learning
Self-Training methods
Neuro-Fuzzy Inference systems
Fuzzy Controllers
Monarch Butterfly Optimized Control with Robustness Analysis
GMM methods for speaker age and gender classification
Regression methods for Permeability Prediction of Petroleum Reservoirs
Surface EMG Signal Classification
Pattern Mining
Human Activity Recognition in Smart Environments
Teaching–Learning based Optimization Algorithm
Big Data Analytics
Diagnosis based on Event-Driven Processing and Machine Learning for Mobile Healthcare
Over a decade ago, Effat University envisioned a timely platform that brings together educators, researchers and tech enthusiasts under one roof and functions as a fount for creativity and innovation. It was a dream that such platform bridges the existing gap and becomes a leading hub for innovators across disciplines to share their knowledge and exchange novel ideas. It was in 2003 that this dream was realized and the first Learning & Technology Conference was held. Up until today, the conference has covered a variety of cutting-edge themes such as Digital Literacy, Cyber Citizenship, Edutainment, Massive Open Online Courses, and many, many others. The conference has also attracted key, prominent figures in the fields of sciences and technology such as Farouq El Baz from NASA, Queen Rania Al-Abdullah of Jordan, and many others who addressed large, eager-to-learn audiences and inspired many with unique stories.
While emerging innovations, such as Artificial Intelligence technologies, are seen today as promising instruments that could pave our way to the future, these were also the focal points around which fruitful discussions have always taken place here at the L&T. The (AI) was selected for this conference due to its great impact. The Saudi government realized this impact of AI and already started actual steps to invest in AI. It is stated in the Kingdome Vision 2030: "In technology, we will increase our investments in, and lead, the digital economy." Dr. Ahmed Al Theneyan, Deputy Minister of Technology, Industry and Digital Capabilities, stated that: "The Government has invested around USD 3 billion in building the infrastructure so that the country is AI-ready and can become a leader in AI use." Vision 2030 programs also promote innovation in technologies. Another great step that our country made is establishing NEOM city (the model smart city).
Effat University realized this ambition and started working to make it a reality by offering academic programs that support the different sectors needed in such projects. For example, the master program in Energy Engineering was launched four years ago to support the energy sector. Also, the bachelor program of Computer Science has tracks in Artificial Intelligence and Cyber Security which was launched in Fall 2020 semester. Additionally, Energy & Technology and Smart Building Research Centers were established to support innovation in the technology and energy sectors. In general, Effat University works effectively in supporting the KSA to achieve its vision in this time of national transformation by graduating skilled citizen in different fields of technology.
The guest editors would like to take this opportunity to thank all the authors for the efforts they put in the preparation of their manuscripts and for their valuable contributions. We wish to express our deepest gratitude to the referees, who provided instrumental and constructive feedback to the authors. We also extend our sincere thanks and appreciation for the organizing team under the leadership of the Chair of L&T 2019 Conference Steering Committee, Dr. Haifa Jamal Al-Lail, University President, for her support and dedication.
Our sincere thanks go to the Editor-in-Chief for his kind help and support.
Chui KT, Lytras MD, Visvizi A (2018) Energy sustainability in smart cities: artificial intelligence, smart monitoring, and optimization of energy consumption. Energies 11(11):2869
Article Google Scholar
Chui KT, Fung DCL, Lytras MD, Lam TM (2020) Predicting at-risk university students in a virtual learning environment via a machine learning algorithm. Comput Human Behav 107:105584
Lytras MD, Visvizi A, Daniela L, Sarirete A, De Pablos PO (2018) Social networks research for sustainable smart education. Sustainability 10(9):2974
Lytras MD, Visvizi A, Sarirete A (2019) Clustering smart city services: perceptions, expectations, responses. Sustainability 11(6):1669
Lytras MD, Visvizi A, Chopdar PK, Sarirete A, Alhalabi W (2021) Information management in smart cities: turning end users’ views into multi-item scale development, validation, and policy-making recommendations. Int J Inf Manag 56:102146
Visvizi A, Jussila J, Lytras MD, Ijäs M (2020) Tweeting and mining OECD-related microcontent in the post-truth era: A cloud-based app. Comput Human Behav 107:105958
Download references
Author information
Authors and affiliations.
Effat College of Engineering, Effat Energy and Technology Research Center, Effat University, P.O. Box 34689, Jeddah, Saudi Arabia
Akila Sarirete, Zain Balfagih, Tayeb Brahimi & Miltiadis D. Lytras
King Abdulaziz University, Jeddah, 21589, Saudi Arabia
Miltiadis D. Lytras
Effat College of Business, Effat University, P.O. Box 34689, Jeddah, Saudi Arabia
Anna Visvizi
Institute of International Studies (ISM), SGH Warsaw School of Economics, Aleja Niepodległości 162, 02-554, Warsaw, Poland
You can also search for this author in PubMed Google Scholar
Corresponding author
Correspondence to Akila Sarirete .
Additional information
Publisher's note.
Springer Nature remains neutral with regard to jurisdictional claims in published maps and institutional affiliations.
Rights and permissions
Reprints and permissions
About this article
Sarirete, A., Balfagih, Z., Brahimi, T. et al. Artificial intelligence and machine learning research: towards digital transformation at a global scale. J Ambient Intell Human Comput 13 , 3319–3321 (2022). https://doi.org/10.1007/s12652-021-03168-y
Download citation
Published : 17 April 2021
Issue Date : July 2022
DOI : https://doi.org/10.1007/s12652-021-03168-y
Share this article
Anyone you share the following link with will be able to read this content:
Sorry, a shareable link is not currently available for this article.
Provided by the Springer Nature SharedIt content-sharing initiative
- Find a journal
- Publish with us
- Track your research
Suggestions or feedback?
MIT News | Massachusetts Institute of Technology
- Machine learning
- Social justice
- Black holes
- Classes and programs
Departments
- Aeronautics and Astronautics
- Brain and Cognitive Sciences
- Architecture
- Political Science
- Mechanical Engineering
Centers, Labs, & Programs
- Abdul Latif Jameel Poverty Action Lab (J-PAL)
- Picower Institute for Learning and Memory
- Lincoln Laboratory
- School of Architecture + Planning
- School of Engineering
- School of Humanities, Arts, and Social Sciences
- Sloan School of Management
- School of Science
- MIT Schwarzman College of Computing
New hardware offers faster computation for artificial intelligence, with much less energy
Press contact :, media download.
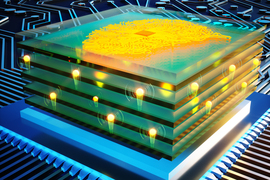
*Terms of Use:
Images for download on the MIT News office website are made available to non-commercial entities, press and the general public under a Creative Commons Attribution Non-Commercial No Derivatives license . You may not alter the images provided, other than to crop them to size. A credit line must be used when reproducing images; if one is not provided below, credit the images to "MIT."
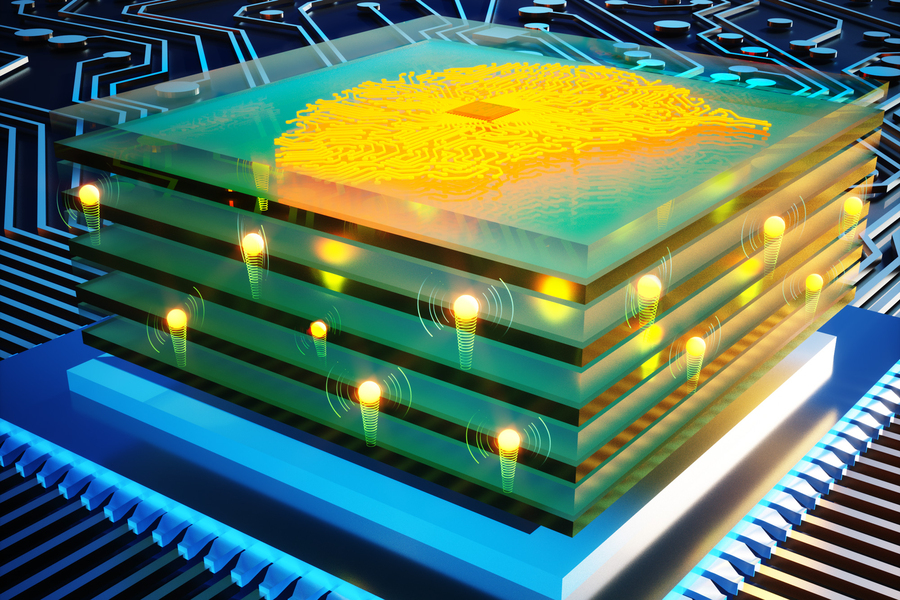
Previous image Next image
As scientists push the boundaries of machine learning, the amount of time, energy, and money required to train increasingly complex neural network models is skyrocketing. A new area of artificial intelligence called analog deep learning promises faster computation with a fraction of the energy usage.
Programmable resistors are the key building blocks in analog deep learning, just like transistors are the core elements for digital processors. By repeating arrays of programmable resistors in complex layers, researchers can create a network of analog artificial “neurons” and “synapses” that execute computations just like a digital neural network. This network can then be trained to achieve complex AI tasks like image recognition and natural language processing.
A multidisciplinary team of MIT researchers set out to push the speed limits of a type of human-made analog synapse that they had previously developed . They utilized a practical inorganic material in the fabrication process that enables their devices to run 1 million times faster than previous versions, which is also about 1 million times faster than the synapses in the human brain.
Moreover, this inorganic material also makes the resistor extremely energy-efficient. Unlike materials used in the earlier version of their device, the new material is compatible with silicon fabrication techniques. This change has enabled fabricating devices at the nanometer scale and could pave the way for integration into commercial computing hardware for deep-learning applications.
“With that key insight, and the very powerful nanofabrication techniques we have at MIT.nano , we have been able to put these pieces together and demonstrate that these devices are intrinsically very fast and operate with reasonable voltages,” says senior author Jesús A. del Alamo, the Donner Professor in MIT’s Department of Electrical Engineering and Computer Science (EECS). “This work has really put these devices at a point where they now look really promising for future applications.”
“The working mechanism of the device is electrochemical insertion of the smallest ion, the proton, into an insulating oxide to modulate its electronic conductivity. Because we are working with very thin devices, we could accelerate the motion of this ion by using a strong electric field, and push these ionic devices to the nanosecond operation regime,” explains senior author Bilge Yildiz, the Breene M. Kerr Professor in the departments of Nuclear Science and Engineering and Materials Science and Engineering.
“The action potential in biological cells rises and falls with a timescale of milliseconds, since the voltage difference of about 0.1 volt is constrained by the stability of water,” says senior author Ju Li, the Battelle Energy Alliance Professor of Nuclear Science and Engineering and professor of materials science and engineering, “Here we apply up to 10 volts across a special solid glass film of nanoscale thickness that conducts protons, without permanently damaging it. And the stronger the field, the faster the ionic devices.”
These programmable resistors vastly increase the speed at which a neural network is trained, while drastically reducing the cost and energy to perform that training. This could help scientists develop deep learning models much more quickly, which could then be applied in uses like self-driving cars, fraud detection, or medical image analysis.
“Once you have an analog processor, you will no longer be training networks everyone else is working on. You will be training networks with unprecedented complexities that no one else can afford to, and therefore vastly outperform them all. In other words, this is not a faster car, this is a spacecraft,” adds lead author and MIT postdoc Murat Onen.
Co-authors include Frances M. Ross, the Ellen Swallow Richards Professor in the Department of Materials Science and Engineering; postdocs Nicolas Emond and Baoming Wang; and Difei Zhang, an EECS graduate student. The research is published today in Science .
Accelerating deep learning
Analog deep learning is faster and more energy-efficient than its digital counterpart for two main reasons. “First, computation is performed in memory, so enormous loads of data are not transferred back and forth from memory to a processor.” Analog processors also conduct operations in parallel. If the matrix size expands, an analog processor doesn’t need more time to complete new operations because all computation occurs simultaneously.
The key element of MIT’s new analog processor technology is known as a protonic programmable resistor. These resistors, which are measured in nanometers (one nanometer is one billionth of a meter), are arranged in an array, like a chess board.
In the human brain, learning happens due to the strengthening and weakening of connections between neurons, called synapses. Deep neural networks have long adopted this strategy, where the network weights are programmed through training algorithms. In the case of this new processor, increasing and decreasing the electrical conductance of protonic resistors enables analog machine learning.
The conductance is controlled by the movement of protons. To increase the conductance, more protons are pushed into a channel in the resistor, while to decrease conductance protons are taken out. This is accomplished using an electrolyte (similar to that of a battery) that conducts protons but blocks electrons.
To develop a super-fast and highly energy efficient programmable protonic resistor, the researchers looked to different materials for the electrolyte. While other devices used organic compounds, Onen focused on inorganic phosphosilicate glass (PSG).
PSG is basically silicon dioxide, which is the powdery desiccant material found in tiny bags that come in the box with new furniture to remove moisture. It is studied as a proton conductor under humidified conditions for fuel cells. It is also the most well-known oxide used in silicon processing. To make PSG, a tiny bit of phosphorus is added to the silicon to give it special characteristics for proton conduction.
Onen hypothesized that an optimized PSG could have a high proton conductivity at room temperature without the need for water, which would make it an ideal solid electrolyte for this application. He was right.
Surprising speed
PSG enables ultrafast proton movement because it contains a multitude of nanometer-sized pores whose surfaces provide paths for proton diffusion. It can also withstand very strong, pulsed electric fields. This is critical, Onen explains, because applying more voltage to the device enables protons to move at blinding speeds.
“The speed certainly was surprising. Normally, we would not apply such extreme fields across devices, in order to not turn them into ash. But instead, protons ended up shuttling at immense speeds across the device stack, specifically a million times faster compared to what we had before. And this movement doesn’t damage anything, thanks to the small size and low mass of protons. It is almost like teleporting,” he says.
“The nanosecond timescale means we are close to the ballistic or even quantum tunneling regime for the proton, under such an extreme field,” adds Li.
Because the protons don’t damage the material, the resistor can run for millions of cycles without breaking down. This new electrolyte enabled a programmable protonic resistor that is a million times faster than their previous device and can operate effectively at room temperature, which is important for incorporating it into computing hardware.
Thanks to the insulating properties of PSG, almost no electric current passes through the material as protons move. This makes the device extremely energy efficient, Onen adds.
Now that they have demonstrated the effectiveness of these programmable resistors, the researchers plan to reengineer them for high-volume manufacturing, says del Alamo. Then they can study the properties of resistor arrays and scale them up so they can be embedded into systems.
At the same time, they plan to study the materials to remove bottlenecks that limit the voltage that is required to efficiently transfer the protons to, through, and from the electrolyte.
“Another exciting direction that these ionic devices can enable is energy-efficient hardware to emulate the neural circuits and synaptic plasticity rules that are deduced in neuroscience, beyond analog deep neural networks. We have already started such a collaboration with neuroscience, supported by the MIT Quest for Intelligence ,” adds Yildiz.
“The collaboration that we have is going to be essential to innovate in the future. The path forward is still going to be very challenging, but at the same time it is very exciting,” del Alamo says.
“Intercalation reactions such as those found in lithium-ion batteries have been explored extensively for memory devices. This work demonstrates that proton-based memory devices deliver impressive and surprising switching speed and endurance,” says William Chueh, associate professor of materials science and engineering at Stanford University, who was not involved with this research. “It lays the foundation for a new class of memory devices for powering deep learning algorithms.”
“This work demonstrates a significant breakthrough in biologically inspired resistive-memory devices. These all-solid-state protonic devices are based on exquisite atomic-scale control of protons, similar to biological synapses but at orders of magnitude faster rates,” says Elizabeth Dickey, the Teddy & Wilton Hawkins Distinguished Professor and head of the Department of Materials Science and Engineering at Carnegie Mellon University, who was not involved with this work. “I commend the interdisciplinary MIT team for this exciting development, which will enable future-generation computational devices.”
This research is funded, in part, by the MIT-IBM Watson AI Lab.
Share this news article on:
Press mentions.
MIT researchers have developed a new hardware that offers faster computation for artificial intelligence with less energy, reports Kyle Wiggers for TechCrunch . “The researchers’ processor uses ‘protonic programmable resistors’ arranged in an array to ‘learn’ skills” explains Wiggers.
New Scientist
Postdoctoral researcher Murat Onen and his colleagues have created “a nanoscale resistor that transmits protons from one terminal to another,” reports Alex Wilkins for New Scientist . “The resistor uses powerful electric fields to transport protons at very high speeds without damaging or breaking the resistor itself, a problem previous solid-state proton resistors had suffered from,” explains Wilkins.
Previous item Next item
Related Links
- Jesús del Alamo
- Bilge Yildiz
- Frances Ross
- Microsystems Technology Laboratories
- MIT-IBM Watson AI Lab
- Department of Materials Science and Engineering
- Department of Nuclear Science and Engineering
- Department of Electrical Engineering and Computer Science
Related Topics
- Electronics
- Materials science and engineering
- Nanoscience and nanotechnology
- Computer science and technology
- Artificial intelligence
- Electrical Engineering & Computer Science (eecs)
- Nuclear science and engineering
- Quest for Intelligence
Related Articles
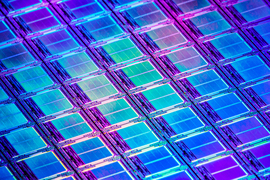
Discovery suggests new promise for nonsilicon computer transistors
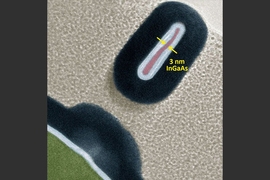
Engineers produce smallest 3-D transistor yet
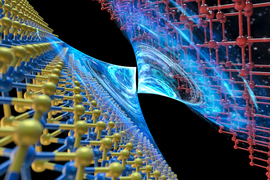
Advance may enable “2D” transistors for tinier microchip components
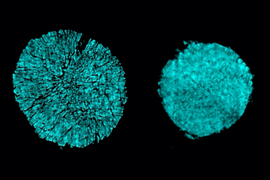
Design could enable longer lasting, more powerful lithium batteries
More mit news.
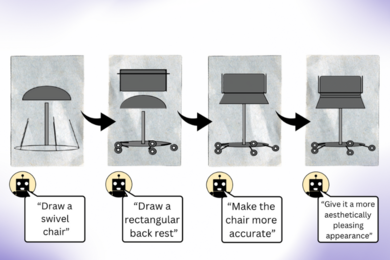
Understanding the visual knowledge of language models
Read full story →
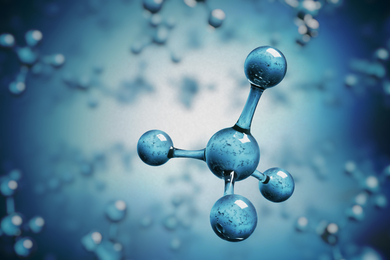
A smarter way to streamline drug discovery
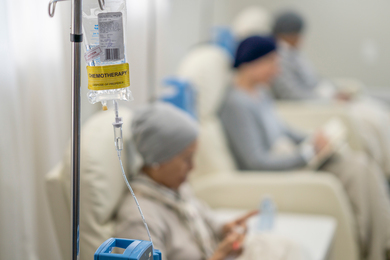
A new way to spot life-threatening infections in cancer patients
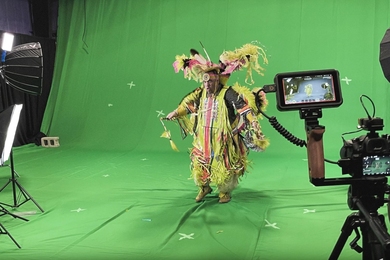
A creation story told through immersive technology
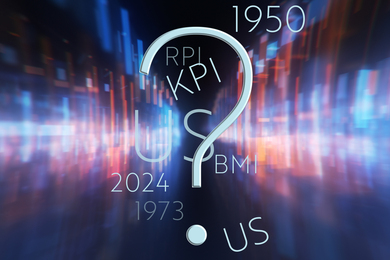
Technique improves the reasoning capabilities of large language models
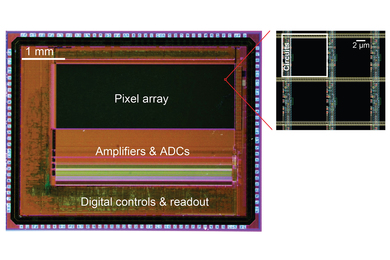
With programmable pixels, novel sensor improves imaging of neural activity
- More news on MIT News homepage →
Massachusetts Institute of Technology 77 Massachusetts Avenue, Cambridge, MA, USA
- Map (opens in new window)
- Events (opens in new window)
- People (opens in new window)
- Careers (opens in new window)
- Accessibility
- Social Media Hub
- MIT on Facebook
- MIT on YouTube
- MIT on Instagram

- Conferences
- MachineHack
- Last updated November 18, 2021
- In AI Origins & Evolution
Top Machine Learning Research Papers Released In 2021
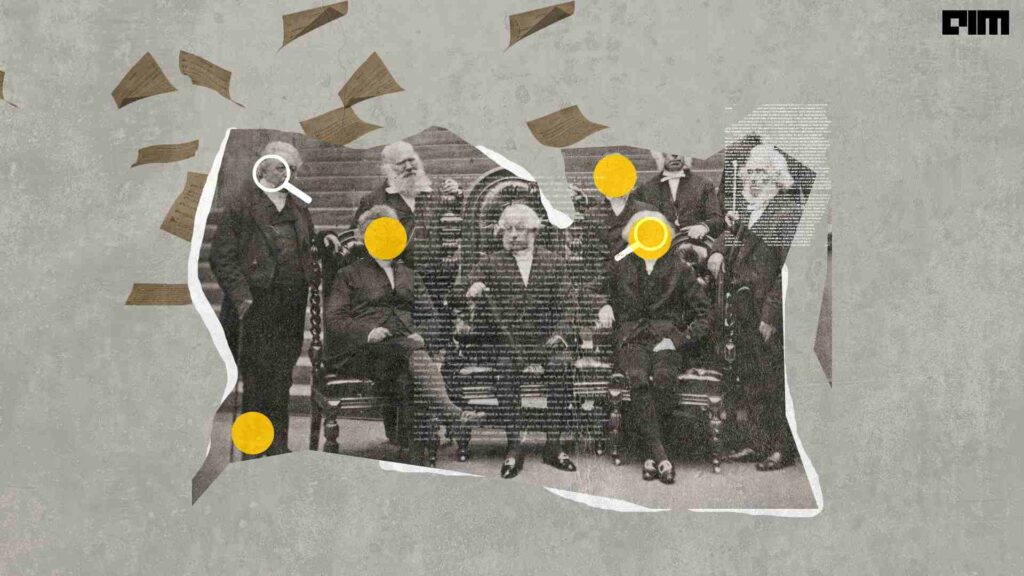
- Published on November 18, 2021
- by Dr. Nivash Jeevanandam
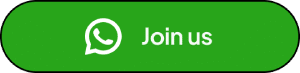
Advances in machine learning and deep learning research are reshaping our technology. Machine learning and deep learning have accomplished various astounding feats this year in 2021, and key research articles have resulted in technical advances used by billions of people. The research in this sector is advancing at a breakneck pace and assisting you to keep up. Here is a collection of the most important recent scientific study papers.
Rebooting ACGAN: Auxiliary Classifier GANs with Stable Training
The authors of this work examined why ACGAN training becomes unstable as the number of classes in the dataset grows. The researchers revealed that the unstable training occurs due to a gradient explosion problem caused by the unboundedness of the input feature vectors and the classifier’s poor classification capabilities during the early training stage. The researchers presented the Data-to-Data Cross-Entropy loss (D2D-CE) and the Rebooted Auxiliary Classifier Generative Adversarial Network to alleviate the instability and reinforce ACGAN (ReACGAN). Additionally, extensive tests of ReACGAN demonstrate that it is resistant to hyperparameter selection and is compatible with a variety of architectures and differentiable augmentations.
This article is ranked #1 on CIFAR-10 for Conditional Image Generation.
For the research paper, read here .
For code, see here .
Dense Unsupervised Learning for Video Segmentation
The authors presented a straightforward and computationally fast unsupervised strategy for learning dense spacetime representations from unlabeled films in this study. The approach demonstrates rapid convergence of training and a high degree of data efficiency. Furthermore, the researchers obtain VOS accuracy superior to previous results despite employing a fraction of the previously necessary training data. The researchers acknowledge that the research findings may be utilised maliciously, such as for unlawful surveillance, and that they are excited to investigate how this skill might be used to better learn a broader spectrum of invariances by exploiting larger temporal windows in movies with complex (ego-)motion, which is more prone to disocclusions.
This study is ranked #1 on DAVIS 2017 for Unsupervised Video Object Segmentation (val).
Temporally-Consistent Surface Reconstruction using Metrically-Consistent Atlases
The authors offer an atlas-based technique for producing unsupervised temporally consistent surface reconstructions by requiring a point on the canonical shape representation to translate to metrically consistent 3D locations on the reconstructed surfaces. Finally, the researchers envisage a plethora of potential applications for the method. For example, by substituting an image-based loss for the Chamfer distance, one may apply the method to RGB video sequences, which the researchers feel will spur development in video-based 3D reconstruction.
This article is ranked #1 on ANIM in the category of Surface Reconstruction.
EdgeFlow: Achieving Practical Interactive Segmentation with Edge-Guided Flow
The researchers propose a revolutionary interactive architecture called EdgeFlow that uses user interaction data without resorting to post-processing or iterative optimisation. The suggested technique achieves state-of-the-art performance on common benchmarks due to its coarse-to-fine network design. Additionally, the researchers create an effective interactive segmentation tool that enables the user to improve the segmentation result through flexible options incrementally.
This paper is ranked #1 on Interactive Segmentation on PASCAL VOC
Learning Transferable Visual Models From Natural Language Supervision
The authors of this work examined whether it is possible to transfer the success of task-agnostic web-scale pre-training in natural language processing to another domain. The findings indicate that adopting this formula resulted in the emergence of similar behaviours in the field of computer vision, and the authors examine the social ramifications of this line of research. CLIP models learn to accomplish a range of tasks during pre-training to optimise their training objective. Using natural language prompting, CLIP can then use this task learning to enable zero-shot transfer to many existing datasets. When applied at a large scale, this technique can compete with task-specific supervised models, while there is still much space for improvement.
This research is ranked #1 on Zero-Shot Transfer Image Classification on SUN
CoAtNet: Marrying Convolution and Attention for All Data Sizes
The researchers in this article conduct a thorough examination of the features of convolutions and transformers, resulting in a principled approach for combining them into a new family of models dubbed CoAtNet. Extensive experiments demonstrate that CoAtNet combines the advantages of ConvNets and Transformers, achieving state-of-the-art performance across a range of data sizes and compute budgets. Take note that this article is currently concentrating on ImageNet classification for model construction. However, the researchers believe their approach is relevant to a broader range of applications, such as object detection and semantic segmentation.
This paper is ranked #1 on Image Classification on ImageNet (using extra training data).
SwinIR: Image Restoration Using Swin Transformer
The authors of this article suggest the SwinIR image restoration model, which is based on the Swin Transformer . The model comprises three modules: shallow feature extraction, deep feature extraction, and human-recognition reconstruction. For deep feature extraction, the researchers employ a stack of residual Swin Transformer blocks (RSTB), each formed of Swin Transformer layers, a convolution layer, and a residual connection.
This research article is ranked #1 on Image Super-Resolution on Manga109 – 4x upscaling.
Access all our open Survey & Awards Nomination forms in one place

Dr. Nivash Jeevanandam

AI Can Never Replace Excel

6 Incredible Ways LLMs are Transforming Healthcare
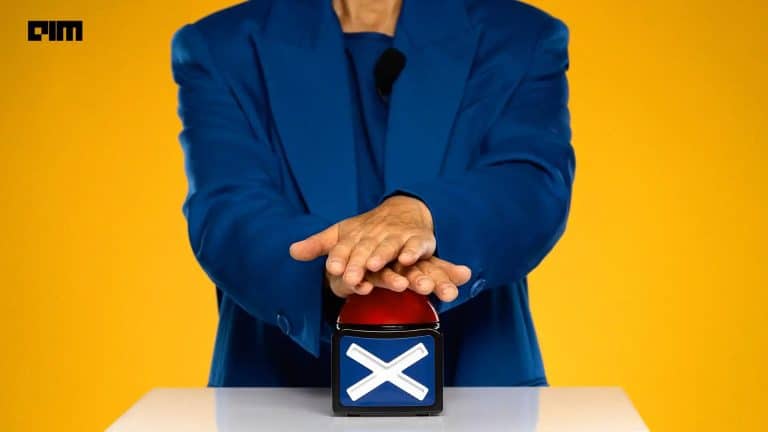
Could AI have Prevented the Exit Poll Mess in India?
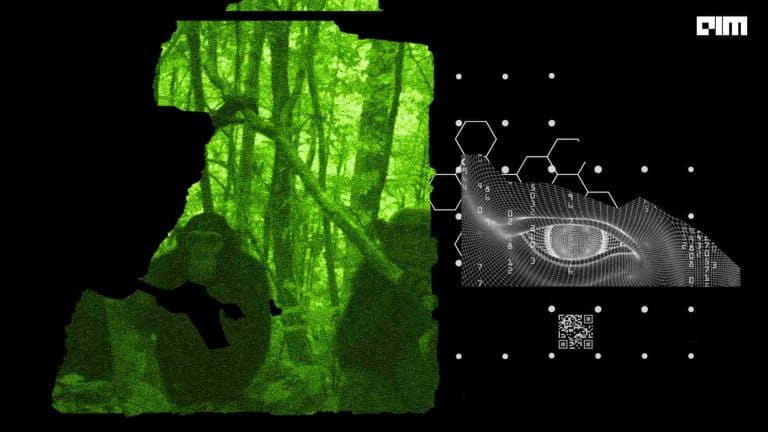
6 Incredible Ways AI is Helping Wildlife Conservation

AI Whistleblowers Stand in the Way of AGI?
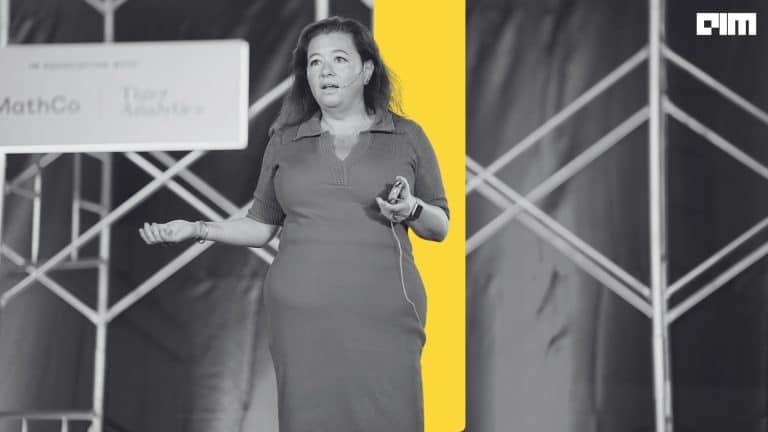
EPAM’s Elaina Shekhter Envisions a Future with Human-AI Agents

OpenAI’s GPT-4 Shows Prowess in Picking Stocks
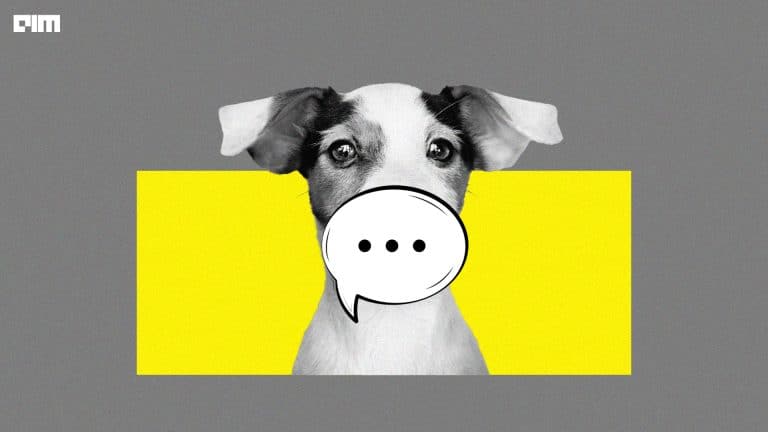
Soon, LLMs Can Help Humans Communicate with Animals
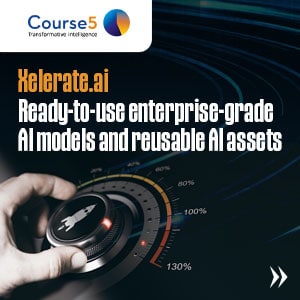
The sentiment that education in India is not adequately preparing students for real-world applications is echoed widely.
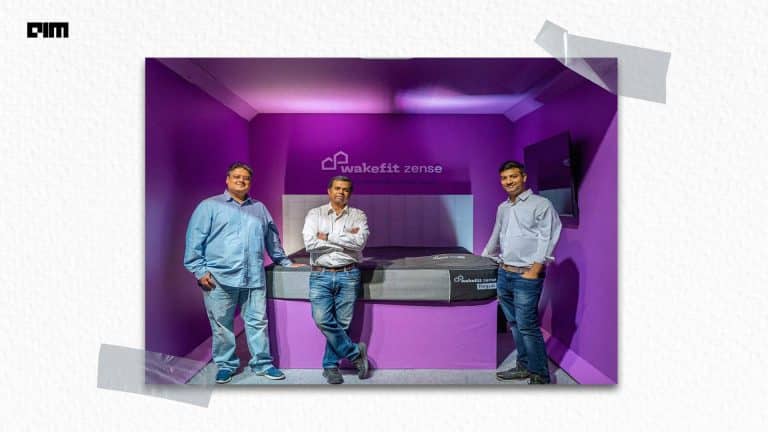
Top Editorial Picks
Bill Gates Advises Indians to Build AI Models on Google, Microsoft and OpenAI Vandana Nair
Milestone Systems Unveils Cloud-Based Video Surveillance System Shyam Nandan Upadhyay
MIT Prof Calls for AI Experts to Revamp Kumbh Mela Tarunya S
EPAM Acquires Health Data Analytics Company Odysseus Data Services Pritam Bordoloi
Transformers Can Now Work Pixel by Pixel, Says Meta AI’s New Study Gopika Raj
NVIDIA Rolls Out HelpSteer2 Dataset to Align LLMs Gopika Raj
AWS Teams Up with Accel to Support GenAI Startups in APJ Gopika Raj
Subscribe to The Belamy: Our Weekly Newsletter
Biggest ai stories, delivered to your inbox every week., "> "> flagship events.
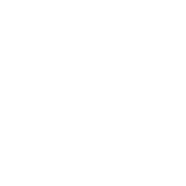
Explore the transformative journey of Global Capability Centers at MachineCon GCC Summit 2024, where innovation meets strategic growth.
© Analytics India Magazine Pvt Ltd & AIM Media House LLC 2024
- Terms of use
- Privacy Policy
Subscribe to Our Newsletter
The Belamy, our weekly Newsletter is a rage. Just enter your email below.
Subscribe to Our Youtube channel
Introducing Apple’s On-Device and Server Foundation Models
At the 2024 Worldwide Developers Conference , we introduced Apple Intelligence, a personal intelligence system integrated deeply into iOS 18, iPadOS 18, and macOS Sequoia.
Apple Intelligence is comprised of multiple highly-capable generative models that are specialized for our users’ everyday tasks, and can adapt on the fly for their current activity. The foundation models built into Apple Intelligence have been fine-tuned for user experiences such as writing and refining text, prioritizing and summarizing notifications, creating playful images for conversations with family and friends, and taking in-app actions to simplify interactions across apps.
In the following overview, we will detail how two of these models — a ~3 billion parameter on-device language model, and a larger server-based language model available with Private Cloud Compute and running on Apple silicon servers — have been built and adapted to perform specialized tasks efficiently, accurately, and responsibly. These two foundation models are part of a larger family of generative models created by Apple to support users and developers; this includes a coding model to build intelligence into Xcode, as well as a diffusion model to help users express themselves visually, for example, in the Messages app. We look forward to sharing more information soon on this broader set of models.
Our Focus on Responsible AI Development
Apple Intelligence is designed with our core values at every step and built on a foundation of groundbreaking privacy innovations.
Additionally, we have created a set of Responsible AI principles to guide how we develop AI tools, as well as the models that underpin them:
- Empower users with intelligent tools : We identify areas where AI can be used responsibly to create tools for addressing specific user needs. We respect how our users choose to use these tools to accomplish their goals.
- Represent our users : We build deeply personal products with the goal of representing users around the globe authentically. We work continuously to avoid perpetuating stereotypes and systemic biases across our AI tools and models.
- Design with care : We take precautions at every stage of our process, including design, model training, feature development, and quality evaluation to identify how our AI tools may be misused or lead to potential harm. We will continuously and proactively improve our AI tools with the help of user feedback.
- Protect privacy : We protect our users' privacy with powerful on-device processing and groundbreaking infrastructure like Private Cloud Compute. We do not use our users' private personal data or user interactions when training our foundation models.
These principles are reflected throughout the architecture that enables Apple Intelligence, connects features and tools with specialized models, and scans inputs and outputs to provide each feature with the information needed to function responsibly.
In the remainder of this overview, we provide details on decisions such as: how we develop models that are highly capable, fast, and power-efficient; how we approach training these models; how our adapters are fine-tuned for specific user needs; and how we evaluate model performance for both helpfulness and unintended harm.
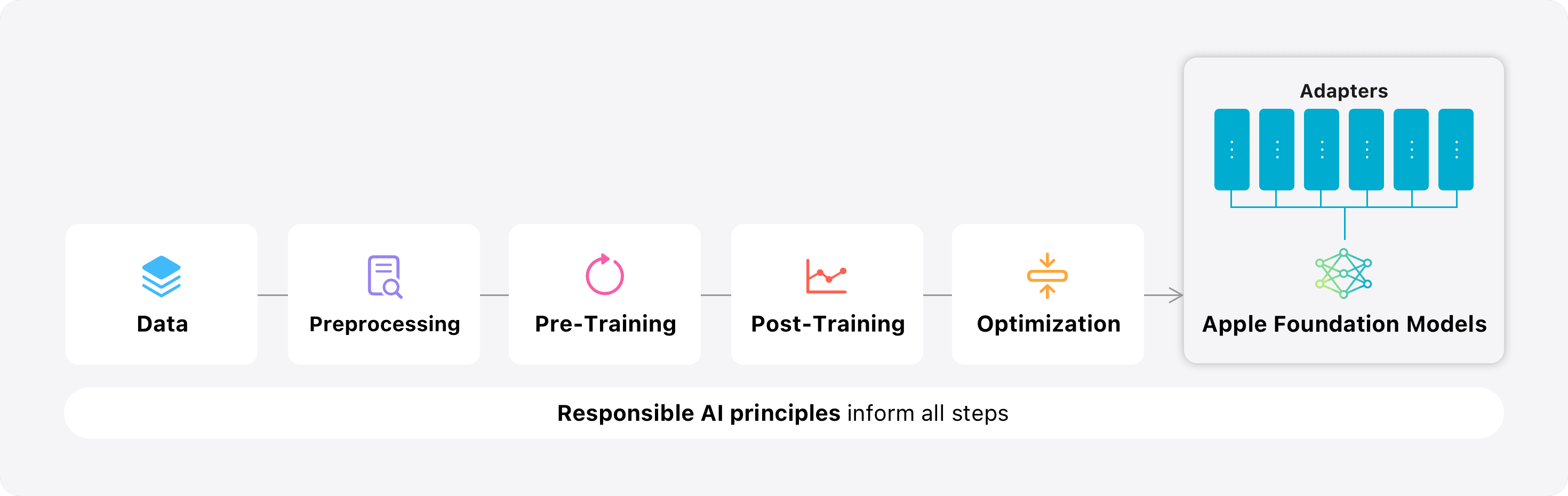
Pre-Training
Our foundation models are trained on Apple's AXLearn framework , an open-source project we released in 2023. It builds on top of JAX and XLA, and allows us to train the models with high efficiency and scalability on various training hardware and cloud platforms, including TPUs and both cloud and on-premise GPUs. We used a combination of data parallelism, tensor parallelism, sequence parallelism, and Fully Sharded Data Parallel (FSDP) to scale training along multiple dimensions such as data, model, and sequence length.
We train our foundation models on licensed data, including data selected to enhance specific features, as well as publicly available data collected by our web-crawler, AppleBot. Web publishers have the option to opt out of the use of their web content for Apple Intelligence training with a data usage control.
We never use our users’ private personal data or user interactions when training our foundation models, and we apply filters to remove personally identifiable information like social security and credit card numbers that are publicly available on the Internet. We also filter profanity and other low-quality content to prevent its inclusion in the training corpus. In addition to filtering, we perform data extraction, deduplication, and the application of a model-based classifier to identify high quality documents.
Post-Training
We find that data quality is essential to model success, so we utilize a hybrid data strategy in our training pipeline, incorporating both human-annotated and synthetic data, and conduct thorough data curation and filtering procedures. We have developed two novel algorithms in post-training: (1) a rejection sampling fine-tuning algorithm with teacher committee, and (2) a reinforcement learning from human feedback (RLHF) algorithm with mirror descent policy optimization and a leave-one-out advantage estimator. We find that these two algorithms lead to significant improvement in the model’s instruction-following quality.
Optimization
In addition to ensuring our generative models are highly capable, we have used a range of innovative techniques to optimize them on-device and on our private cloud for speed and efficiency. We have applied an extensive set of optimizations for both first token and extended token inference performance.
Both the on-device and server models use grouped-query-attention. We use shared input and output vocab embedding tables to reduce memory requirements and inference cost. These shared embedding tensors are mapped without duplications. The on-device model uses a vocab size of 49K, while the server model uses a vocab size of 100K, which includes additional language and technical tokens.
For on-device inference, we use low-bit palletization, a critical optimization technique that achieves the necessary memory, power, and performance requirements. To maintain model quality, we developed a new framework using LoRA adapters that incorporates a mixed 2-bit and 4-bit configuration strategy — averaging 3.5 bits-per-weight — to achieve the same accuracy as the uncompressed models.
Additionally, we use an interactive model latency and power analysis tool, Talaria , to better guide the bit rate selection for each operation. We also utilize activation quantization and embedding quantization, and have developed an approach to enable efficient Key-Value (KV) cache update on our neural engines.
With this set of optimizations, on iPhone 15 Pro we are able to reach time-to-first-token latency of about 0.6 millisecond per prompt token, and a generation rate of 30 tokens per second. Notably, this performance is attained before employing token speculation techniques, from which we see further enhancement on the token generation rate.
Model Adaptation
Our foundation models are fine-tuned for users’ everyday activities, and can dynamically specialize themselves on-the-fly for the task at hand. We utilize adapters, small neural network modules that can be plugged into various layers of the pre-trained model, to fine-tune our models for specific tasks. For our models we adapt the attention matrices, the attention projection matrix, and the fully connected layers in the point-wise feedforward networks for a suitable set of the decoding layers of the transformer architecture.
By fine-tuning only the adapter layers, the original parameters of the base pre-trained model remain unchanged, preserving the general knowledge of the model while tailoring the adapter layers to support specific tasks.
We represent the values of the adapter parameters using 16 bits, and for the ~3 billion parameter on-device model, the parameters for a rank 16 adapter typically require 10s of megabytes. The adapter models can be dynamically loaded, temporarily cached in memory, and swapped — giving our foundation model the ability to specialize itself on the fly for the task at hand while efficiently managing memory and guaranteeing the operating system's responsiveness.
To facilitate the training of the adapters, we created an efficient infrastructure that allows us to rapidly retrain, test, and deploy adapters when either the base model or the training data gets updated. The adapter parameters are initialized using the accuracy-recovery adapter introduced in the Optimization section.
Performance and Evaluation
Our focus is on delivering generative models that can enable users to communicate, work, express themselves, and get things done across their Apple products. When benchmarking our models, we focus on human evaluation as we find that these results are highly correlated to user experience in our products. We conducted performance evaluations on both feature-specific adapters and the foundation models.
To illustrate our approach, we look at how we evaluated our adapter for summarization. As product requirements for summaries of emails and notifications differ in subtle but important ways, we fine-tune accuracy-recovery low-rank (LoRA) adapters on top of the palletized model to meet these specific requirements. Our training data is based on synthetic summaries generated from bigger server models, filtered by a rejection sampling strategy that keeps only the high quality summaries.
To evaluate the product-specific summarization, we use a set of 750 responses carefully sampled for each use case. These evaluation datasets emphasize a diverse set of inputs that our product features are likely to face in production, and include a stratified mixture of single and stacked documents of varying content types and lengths. As product features, it was important to evaluate performance against datasets that are representative of real use cases. We find that our models with adapters generate better summaries than a comparable model.
As part of responsible development, we identified and evaluated specific risks inherent to summarization. For example, summaries occasionally remove important nuance or other details in ways that are undesirable. However, we found that the summarization adapter did not amplify sensitive content in over 99% of targeted adversarial examples. We continue to adversarially probe to identify unknown harms and expand our evaluations to help guide further improvements.
In addition to evaluating feature specific performance powered by foundation models and adapters, we evaluate both the on-device and server-based models’ general capabilities. We utilize a comprehensive evaluation set of real-world prompts to test the general model capabilities. These prompts are diverse across different difficulty levels and cover major categories such as brainstorming, classification, closed question answering, coding, extraction, mathematical reasoning, open question answering, rewriting, safety, summarization, and writing.
We compare our models with both open-source models (Phi-3, Gemma, Mistral, DBRX) and commercial models of comparable size (GPT-3.5-Turbo, GPT-4-Turbo) 1 . We find that our models are preferred by human graders over most comparable competitor models. On this benchmark, our on-device model, with ~3B parameters, outperforms larger models including Phi-3-mini, Mistral-7B, and Gemma-7B. Our server model compares favorably to DBRX-Instruct, Mixtral-8x22B, and GPT-3.5-Turbo while being highly efficient.
We use a set of diverse adversarial prompts to test the model performance on harmful content, sensitive topics, and factuality. We measure the violation rates of each model as evaluated by human graders on this evaluation set, with a lower number being desirable. Both the on-device and server models are robust when faced with adversarial prompts, achieving violation rates lower than open-source and commercial models.
Our models are preferred by human graders as safe and helpful over competitor models for these prompts. However, considering the broad capabilities of large language models, we understand the limitation of our safety benchmark. We are actively conducting both manual and automatic red-teaming with internal and external teams to continue evaluating our models' safety.
To further evaluate our models, we use the Instruction-Following Eval (IFEval) benchmark to compare their instruction-following capabilities with models of comparable size. The results suggest that both our on-device and server model follow detailed instructions better than the open-source and commercial models of comparable size.
We evaluate our models’ writing ability on our internal summarization and composition benchmarks, consisting of a variety of writing instructions. These results do not refer to our feature-specific adapter for summarization (seen in Figure 3 ), nor do we have an adapter focused on composition.
The Apple foundation models and adapters introduced at WWDC24 underlie Apple Intelligence, the new personal intelligence system that is integrated deeply into iPhone, iPad, and Mac, and enables powerful capabilities across language, images, actions, and personal context. Our models have been created with the purpose of helping users do everyday activities across their Apple products, and developed responsibly at every stage and guided by Apple’s core values. We look forward to sharing more information soon on our broader family of generative models, including language, diffusion, and coding models.
[1] We compared against the following model versions: gpt-3.5-turbo-0125, gpt-4-0125-preview, Phi-3-mini-4k-instruct, Mistral-7B-Instruct-v0.2, Mixtral-8x22B-Instruct-v0.1, Gemma-1.1-2B, and Gemma-1.1-7B. The open-source and Apple models are evaluated in bfloat16 precision.
Related readings and updates.
Advancing speech accessibility with personal voice.
A voice replicator is a powerful tool for people at risk of losing their ability to speak, including those with a recent diagnosis of amyotrophic lateral sclerosis (ALS) or other conditions that can progressively impact speaking ability. First introduced in May 2023 and made available on iOS 17 in September 2023, Personal Voice is a tool that creates a synthesized voice for such users to speak in FaceTime, phone calls, assistive communication apps, and in-person conversations.
Apple Natural Language Understanding Workshop 2023
Earlier this year, Apple hosted the Natural Language Understanding workshop. This two-day hybrid event brought together Apple and members of the academic research community for talks and discussions on the state of the art in natural language understanding.
In this post, we share highlights from workshop discussions and recordings of select workshop talks.
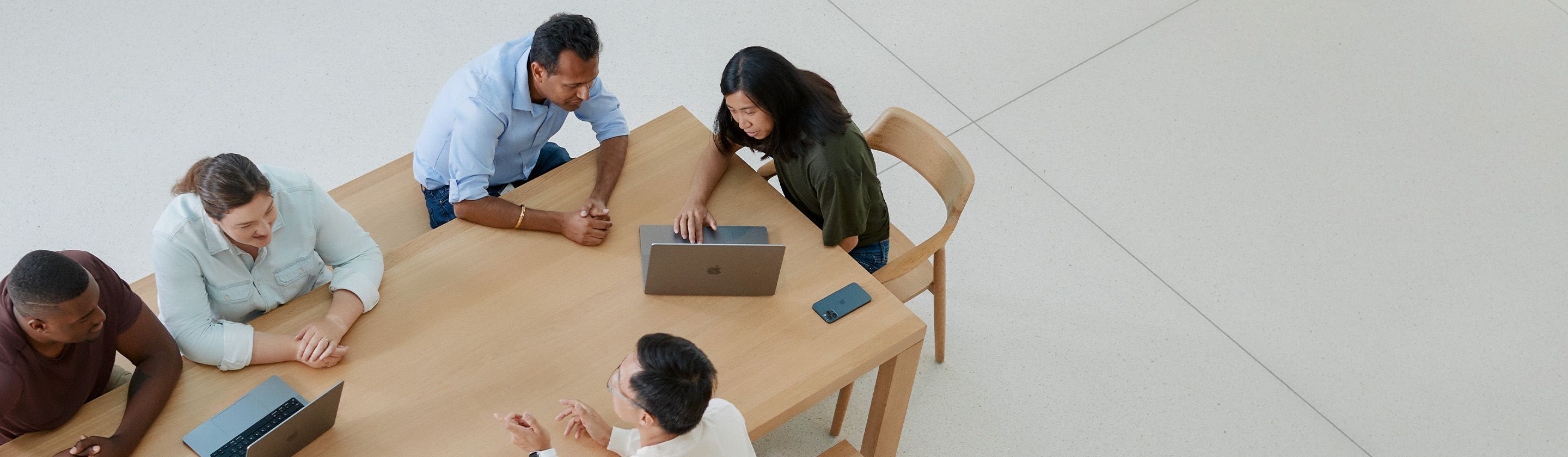
Discover opportunities in Machine Learning.
Our research in machine learning breaks new ground every day.
Work with us
More From Forbes
5 online courses with certificates for high-income ai skills in 2024.
- Share to Facebook
- Share to Twitter
- Share to Linkedin
AI skills are now non-negotiable for career success
New research shows that having AI as part of your professional toolkit is now a non-negotiable for career success. A recent Amazon study revealed that employers are willing to pay up to 47% more than the base salary for employees with AI skills, while similarly, an Oxford University study conducted in late 2023 discovered that employers were willing to offer a pay increase of up to 40% more when workers possessed in-demand AI skills.
This demonstrates just how significant and valuable AI skills are. For many executives, how best to implement and adopt AI into their processes, workflows, and work culture is top of mind in 2024, and having AI allies in their current and would-be employees is essential.
Perhaps, this serves to explain why employers are willing to pay higher salaries for workers who demonstrate strong AI skills proficiency, especially since 73% of employers consider hiring talent with AI skills and experience a priority, but nearly three in four cannot find the talent they need, leading to an AI skills gap.
Whether you're a working professional or you're currently unemployed, it's a wise move to acquire this hot commodity—AI skills—before the hype and labor demand begins to fade out.
Here are five online courses, that also come with certificates, to help you learn and build in-demand AI skills this year:
IMF Issues Serious U.S. Dollar Collapse Warning As The Fed Primes Bitcoin, Ethereum And XRP For A Crypto Price Boom
Biden vs. trump 2024 election polls: independents less likely to support trump post-conviction, house of the dragon season 2 episode 1 recap and review blood and cheese, 1. aws certified ai practitioner.
In response to the growing demand, Amazon Web Services has recently created two courses—one of them being the AWS Certified AI Practitioner—which is aimed at enhancing professionals' AI skills. This particular certification is foundation-level and is suitable to anyone regardless of their industry background. If you're a novice to artificial intelligence, studying for this certificate can help you master basic concepts related to ethical AI usage and generative AI.
2. AWS Certified Machine Learning Engineer—Associate
Like it's twin, this AWS-provided certification helps you enhance AI skills—but is more focused on professionals who have "at least one year of experience building, deploying, and maintaining AI and ML solutions on AWS," the website says. "It is beneficial for people who want to demonstrate their ability to make AI models available for real-time usage."
3. Google's Machine Learning And AI Learning Path
Next up is the machine learning and AI learning path which you can take up with Google Cloud, which walks you through several areas including big data, and AI in Google Cloud. Upon completion of the learning path you can earn skills badges and take the professional machine learning engineer exam, to obtain your certificate.
4. The AI Awakening: Implications for the Economy and Society, by Stanford University
This certificate would be good for you to consider if you work in leadership or management, or are considering climbing the corporate ladder into leadership and management roles, including program and project management. It also might be useful to consider if you work within HR. This course by Stanford University (via Coursera) teaches about AI capabilities and its benefits to the workforce and to the global economy through start-ups, as well as the potential risks and important ethical factors to be aware of.
5. IBM AI Developer Professional Certificate
This professional certification offered via Coursera by IBM, teaches you AI skills at the foundational, beginner level, and according to the website, equips you for the workforce in as little as six months. Some of the job-readiness AI skills it covers include large language models (LLMS), AI prompting, and Python.
Three in four employers cannot find talented employees with AI skills, leading to an AI skills gap
These courses range from basic and foundational, to more complex, and most are suitable for you to upskill regardless of your background. This year, take your professional development seriously, and commit to polishing your AI skills and knowledge so you can have access to more career opportunities and land higher-paying jobs.
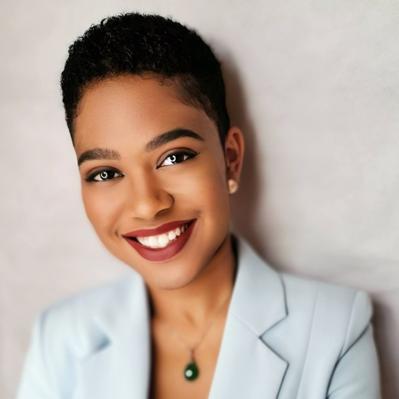
- Editorial Standards
- Reprints & Permissions
Join The Conversation
One Community. Many Voices. Create a free account to share your thoughts.

Forbes Community Guidelines
Our community is about connecting people through open and thoughtful conversations. We want our readers to share their views and exchange ideas and facts in a safe space.
In order to do so, please follow the posting rules in our site's Terms of Service. We've summarized some of those key rules below. Simply put, keep it civil.
Your post will be rejected if we notice that it seems to contain:
- False or intentionally out-of-context or misleading information
- Insults, profanity, incoherent, obscene or inflammatory language or threats of any kind
- Attacks on the identity of other commenters or the article's author
- Content that otherwise violates our site's terms.
User accounts will be blocked if we notice or believe that users are engaged in:
- Continuous attempts to re-post comments that have been previously moderated/rejected
- Racist, sexist, homophobic or other discriminatory comments
- Attempts or tactics that put the site security at risk
- Actions that otherwise violate our site's terms.
So, how can you be a power user?
- Stay on topic and share your insights
- Feel free to be clear and thoughtful to get your point across
- ‘Like’ or ‘Dislike’ to show your point of view.
- Protect your community.
- Use the report tool to alert us when someone breaks the rules.
Thanks for reading our community guidelines. Please read the full list of posting rules found in our site's Terms of Service.
- AI technologies
TechTarget's guide to machine learning is a primer on this important field of computer science, further explaining what machine learning is, how to do it and how it is applied in business. You'll find information on the various types of machine learning algorithms, the challenges and best practices associated with developing and deploying ML models, and what the future holds for machine learning. Throughout the guide, there are hyperlinks to related articles that cover the topics in greater depth.
Top 12 machine learning use cases and business applications, machine learning applications are increasing the efficiency and improving the accuracy of business functions ranging from decision-making to maintenance to service delivery..
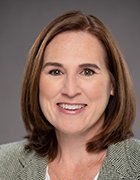
- Mary K. Pratt
Executives across all business sectors have been making substantial investments in machine learning, saying it is a critical technology for competing in today's fast-paced digital economy.
"Machine learning is the backbone of today's business, turning data into insights and insights into action and predictability. That's why machine learning is highly useful," said Adnan Masood, chief AI architect at UST, a digital transformation solutions company.
The proof? Masood pointed to the fact that machine learning ( ML ) supports a large swath of business processes -- from decision-making to maintenance to service delivery.
That, in turn, is driving the ongoing adoption of machine learning, with technology and business leaders implementing ML capabilities throughout their operations.
This article is part of
What is machine learning and how does it work? In-depth guide
- Which also includes:
- The different types of machine learning explained
- How to build a machine learning model in 7 steps
- CNN vs. RNN: How are they different?
Machine learning, a subset of AI , features software systems capable of analyzing data and offering actionable insights based on that analysis. Moreover, it continuously learns from that work to produce more refined and accurate insights over time.
It is a powerful, prolific technology that powers many of the services people encounter every day, from online product recommendations to customer service chatbots.
In fact, experts said that many of the AI capabilities used by companies today are specifically associated with machine learning.
The benefits of machine learning can be grouped into the following four major categories, said Vishal Gupta, partner at research firm Everest Group.
- Efficiency. This is achieved primarily through increased productivity or optimized processes.
- Effectiveness. Machine learning can improve the quality of work done.
- Experience. Workers, customers and other stakeholders have an overall better interaction using machine learning.
- Evolution of the business itself. Machine learning enables new products, services and market opportunities.
The "2024 IT Outlook Report" -- commissioned by Rackspace Technology in partnership with Dell Technologies and VMware -- found that 34% of the 1,420 IT professionals surveyed said machine learning will be a priority at their organizations in 2024.
"There are many use cases across most businesses where machine learning is in place today and can still be put in place tomorrow, even in a world where generative AI exists," said Ryan Gross, partner in the data practice at consulting firm Credera. "In fact, machine learning is often the right solution. It is still the more effective technology, and the most cost-effective technology, for most use cases."
Common machine learning use cases
Although there are myriad use cases for machine learning, experts highlighted the following 12 as the top applications of machine learning in business today.
1. Chatbots
The majority of people have had direct interactions with machine learning at work in the form of chatbots .
Aptly named, these software programs use machine learning and natural language processing ( NLP ) to mimic human conversation. They work off preprogrammed scripts to engage individuals and respond to their questions by accessing company databases to provide answers to those queries.
Early generations of chatbots followed scripted rules that told the bots what actions to take based on keywords. However, ML enables chatbots to be more interactive and productive, and thereby more responsive to a user's needs, more accurate with its responses and ultimately more humanlike in its conversation.
Digital assistants such as Apple's Siri and Amazon's Alexa are everyday examples of chatbots, as are the chatbots that provide the first point of contact for most customer call centers today.
2. Recommendation engines
Machine learning also powers recommendation engines , which are most commonly used in online retail and streaming services.
Here, algorithms process data -- such as a customer's past purchases along with data about a company's current inventory and other customers' buying history -- to determine what products or services to recommend to customers.
Recommendation engines let companies personalize a customer's experience , which helps with customer retention, and enables companies to increase sales by offering products and services that more accurately match what each customer likes and wants.
"That recommendation engine is profiling you and saying, 'People like you bought these other things,' and so then you end up buying them, too," explained Rod Fontecilla, who served as partner and chief innovation officer at consultancy Guidehouse until March 2024.
3. Dynamic pricing
Machine learning also enables companies to adjust the prices they charge for products and services in near real time based on changing market conditions, a practice known as dynamic pricing.
"You look at consumer behavior and buying patterns to move your pricing up and down; it's a very valuable use of machine learning for companies," said Vikas Agarwal, a financial services risk and regulatory leader at professional services firm PwC.
Machine learning systems typically use numerous data sets, such as macro-economic and social media data, to set and reset prices. This is commonly done for airline tickets, hotel room rates and ride-sharing fares. Uber's surge pricing, where prices increase when demand goes up, is a prominent example of how companies use ML algorithms to adjust prices as circumstances change.
4. Customer churn modeling, customer segmentation, targeted marketing and sales forecasting
In many organizations, sales and marketing teams are the most prolific users of machine learning, as the technology supports much of their everyday activities. The ML capabilities are typically built into the enterprise software that supports those departments, such as customer relationship management systems.
So much so, that "these have become table stakes," Gross said. "And if you're not using these, you're probably behind the competition."
Machine learning supports multiple marketing activities.
First, there's customer churn modeling, where machine learning is used to identify which customers might be souring on the company, when that might happen and how that situation could be turned around. To do that, algorithms pinpoint patterns in huge volumes of historical, demographic and sales data to identify and understand why a company loses customers. The company can then use machine learning capabilities to analyze behaviors among existing customers to alert it to which ones are at risk of taking their business elsewhere, identify the reasons why they're leaving and then determine what steps to take to retain them. "Think of it as a recommendation engine built for retail," Masood said.
Companies also use machine learning for customer segmentation , a business practice in which companies categorize customers into specific segments based on common characteristics such as similar ages, incomes or education levels. This lets marketing and sales tune their services, products, advertisements and messaging to each segment.
Additionally, machine learning supports sales by helping customers set the optimal prices for their products and ensures they deliver the right products and services to the right areas at the right time through predictive inventory planning and customer segmentation. Retailers, for example, use machine learning to predict what inventory will sell best in which of its stores based on seasonal factors affecting a particular store, the demographics of that region, what's trending on social media and other data points, Masood explained.
5. Fraud detection
Another prominent use of machine learning in business is in fraud detection, particularly in banking and financial services , where institutions use it to alert customers of potentially fraudulent use of their credit and debit cards.
Machine learning's capacity to understand patterns, and instantly see anomalies that fall outside those patterns, makes this technology a valuable tool for detecting fraudulent activity.
This is how it works: Data scientists use machine learning to understand an individual customer's typical behavior, such as when and where the customer uses a credit card. Machine learning takes that information along with other data to accurately determine in mere milliseconds which transactions fall within the normal range and are therefore legitimate versus which transactions are outside expected norms and therefore are likely fraudulent.
Although this application of machine learning is most common in the financial services sector, travel institutions, gaming companies and retailers are also big users of machine learning for fraud detection.
6. Cyberthreat detection
Machine learning's capacity to analyze complex patterns within high volumes of activities to both determine normal behaviors and identify anomalies also makes it a powerful tool for detecting cyberthreats .
Moreover, its capacity to learn lets it continually refine its understanding of an organization's IT environment, network traffic and usage patterns. So even as the IT environment expands and cyberattacks grow in number and complexity, ML algorithms can continually improve its ability to detect unusual activity that could indicate an intrusion or threat.
7. Optimization
Another use case that cuts across industries and business functions is the use of specific machine learning algorithms to optimize processes . Companies can have the algorithms analyze data and run simulations to determine optimal or near-optimal solutions, or they can use algorithms to offer next best actions -- predictions and recommendations the technology has determined will lead to the best result.
Management advisers said they see ML for optimization used across all areas of enterprise operations, from finance to software development, with the technology speeding up work and reducing human error.
They further noted that its use in logistics, manufacturing and supply chain has delivered particularly significant benefits.
"Machine learning and graph machine learning techniques specifically have been shown to dramatically improve those networks as a whole. They optimize operations while also increasing resiliency," Gross said.
8. Decision support
Organizations also use machine learning to help them make better decisions.
For its survey, Rackspace asked respondents what benefits they expect to see from their AI and ML initiatives. Improved decision-making ranked fourth after improved innovation, reduced costs and enhanced performance.
Experts noted that a decision support system (DSS) can also help cut costs and enhance performance by ensuring workers make the best decisions.
To support decision-making, ML algorithms are trained on historical and other relevant data sets, enabling them to then analyze new information and run through multiple possible scenarios at a scale and speed impossible for humans to match. The algorithms then offer up recommendations on the best course of action to take.
In the healthcare sector, a DSS can assist clinicians in diagnosing patients, reading and interpreting medical imaging and diagnostic scans, and developing treatment options.
In agriculture, machine learning-enabled decision support tools incorporate data on climate, energy, water, resources and other elements to guide farmers on their crop management decisions.
In business operations, a DSS can help management teams anticipate trends, identify problems and speed up decisions.
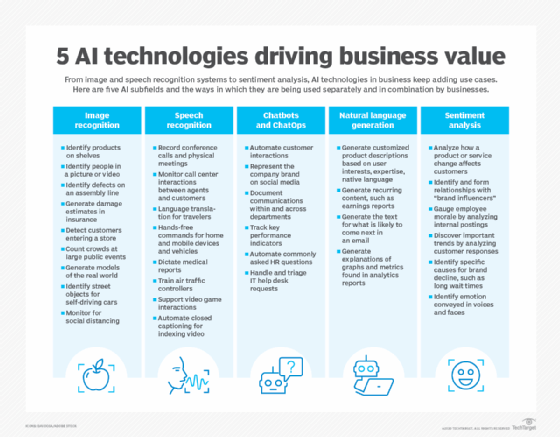
9. Predictive maintenance
Powering predictive maintenance is another longstanding use of machine learning, Gross said.
Company machine learning systems take data from numerous disparate sources -- historical operational data, performance data coming from IoT devices, supply chain data and market prediction information -- to predict the optimal time to perform maintenance on equipment.
Predictive maintenance differs from preventive maintenance in that predictive maintenance can precisely identify what maintenance should be done at what time based on multiple factors. It can, for example, incorporate market conditions and worker availability to determine the optimal time to perform maintenance.
This minimizes the effect of any equipment downtime while maximizing investments in the equipment by not scheduling unnecessary maintenance or scheduling work unnecessarily early in the equipment lifecycle.
Airliners, farmers, mining companies and transportation firms all use ML for predictive maintenance , Gross said.
Meanwhile, some companies are using predictive maintenance to create new services, for example, by offering predictive maintenance scheduling services to customers who buy their equipment.
10. Monitoring and quality assurance
Machine learning's capacity to understand and distinguish patterns in data at a scale, speed and level unmatched by humans makes the technology particularly useful for monitoring needs and quality assurance, said Nicolas Avila, CTO for North America at IT services firm Globant.
As an example, he pointed to the use of machine learning to monitor supply chain operations, with the technology continually analyzing patterns to identify anything that diverts from normal parameters and, thus, could indicate an issue that needs attention.
"It's able to highlight anything that doesn't seem right," Avila said.
Meanwhile, ML technology types such as deep learning, neural networks and computer vision can be used to more effectively and efficiently monitor production lines and other workplace outputs to ensure products meet established quality standards .
11. Sentiment analysis
With sentiment analysis, machine learning models scan and analyze human language to determine whether the emotional tone exhibited is positive, negative or neutral. ML models can also be programmed to rate sentiment on a scale, for example, from 1 to 5.
Companies often use sentiment analysis tools to analyze the text of customer reviews and to evaluate the emotions exhibited by customers in their interactions with the company.
Sentiment analysis also lets companies react more appropriately to customers' needs, Fontecilla said.
For example, the use of sentiment analysis in a call center can help identify a customer's tone and share that analysis with other systems -- such as a chatbot or a human agent's DSS -- to adjust responses or recommended scripts based on those emotions.
12. Information extraction
Information retrieval and information extraction systems -- built using ML technologies such as NLP, optical character recognition and intelligent character recognition -- automatically identify key pieces of structured data from documents even if the information is held in unstructured or semistructured formats.
The technology can also be used with voice-to-text processes, Fontecilla said.
This use of machine learning brings increased efficiency and improved accuracy to documentation processing. It also frees human talent from what can often be mundane and repetitive work.
Editor's note: This article was updated in June 2024 to provide updated research figures and to improve the reader experience.
Mary K. Pratt is an award-winning freelance journalist with a focus on covering enterprise IT, cybersecurity management and strategy.
History and evolution of machine learning: A timeline
What is a machine learning engineer (ML engineer)?
What is machine learning bias (AI bias)?
What is adversarial machine learning?
Popular machine learning certificates
Related Resources
- Buying an AI Infrastructure: What You Should Know –Data Science Central
- How AI in the workplace is changing ERP systems –TechTarget ComputerWeekly.com
- Human-like AI quest drives general AI development efforts –TechTarget ComputerWeekly.com
- Optimising content management workflows with AI –TechTarget ComputerWeekly.com
Dig Deeper on AI technologies
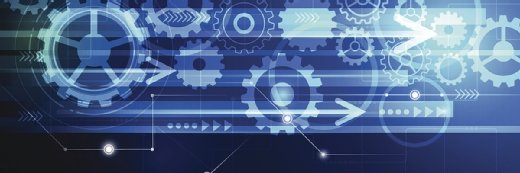
Compare 6 top MLOps platforms
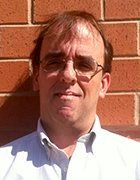
How to get started with machine learning
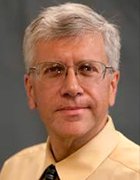
adversarial machine learning
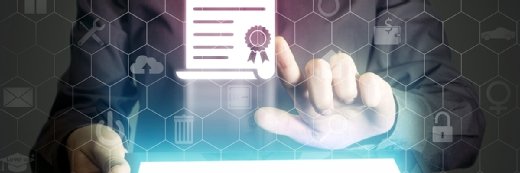
How to become an MLOps engineer
The longtime BI and data integration vendor's tools enable C40 Cities, a climate leadership consortium of nearly 100 cities, to ...
With trusted data as a foundation, the longtime analytics and data integration vendor has been pragmatic in its creation of an ...
The longtime analytics vendor's latest new features include data integration capabilities targeting data quality and a GenAI ...
While there is disagreement in Congress over how to diversify the critical materials supply chain, there is bipartisan agreement ...
As U.S. states like Colorado pass their own AI laws, businesses will need to prepare compliance measures if they do business in ...
Digital transformation success requires cross-organizational alignment, actionable goals and top-notch project management. Here's...
The vendor's latest update adds new customer insight capabilities, including an AI assistant, and industry-specific tools all ...
The data quality specialist's capabilities will enable customers to monitor unstructured text to ensure the health of data used ...
Graph databases offer plenty of advantages for enterprises, but relational databases still top the market. Both emphasize ...
Epicor continues its buying spree with PIM vendor Kyklo, enabling manufacturers and distributors to gain visibility into what ...
Supply chains have a range of connection points -- and vulnerabilities. Learn which vulnerabilities hackers look for first and ...
As supplier relationships become increasingly complex and major disruptions continue, it pays to understand the top supply chain ...
Thank you for visiting nature.com. You are using a browser version with limited support for CSS. To obtain the best experience, we recommend you use a more up to date browser (or turn off compatibility mode in Internet Explorer). In the meantime, to ensure continued support, we are displaying the site without styles and JavaScript.
- View all journals
- Explore content
- About the journal
- Publish with us
- Sign up for alerts
- Review Article
- Open access
- Published: 05 April 2022
Recent advances and applications of deep learning methods in materials science
- Kamal Choudhary ORCID: orcid.org/0000-0001-9737-8074 1 , 2 , 3 ,
- Brian DeCost ORCID: orcid.org/0000-0002-3459-5888 4 ,
- Chi Chen ORCID: orcid.org/0000-0001-8008-7043 5 ,
- Anubhav Jain ORCID: orcid.org/0000-0001-5893-9967 6 ,
- Francesca Tavazza ORCID: orcid.org/0000-0002-5602-180X 1 ,
- Ryan Cohn ORCID: orcid.org/0000-0002-7898-0059 7 ,
- Cheol Woo Park 8 ,
- Alok Choudhary 9 ,
- Ankit Agrawal 9 ,
- Simon J. L. Billinge ORCID: orcid.org/0000-0002-9734-4998 10 ,
- Elizabeth Holm 7 ,
- Shyue Ping Ong ORCID: orcid.org/0000-0001-5726-2587 5 &
- Chris Wolverton ORCID: orcid.org/0000-0003-2248-474X 8
npj Computational Materials volume 8 , Article number: 59 ( 2022 ) Cite this article
69k Accesses
258 Citations
37 Altmetric
Metrics details
- Atomistic models
- Computational methods
Deep learning (DL) is one of the fastest-growing topics in materials data science, with rapidly emerging applications spanning atomistic, image-based, spectral, and textual data modalities. DL allows analysis of unstructured data and automated identification of features. The recent development of large materials databases has fueled the application of DL methods in atomistic prediction in particular. In contrast, advances in image and spectral data have largely leveraged synthetic data enabled by high-quality forward models as well as by generative unsupervised DL methods. In this article, we present a high-level overview of deep learning methods followed by a detailed discussion of recent developments of deep learning in atomistic simulation, materials imaging, spectral analysis, and natural language processing. For each modality we discuss applications involving both theoretical and experimental data, typical modeling approaches with their strengths and limitations, and relevant publicly available software and datasets. We conclude the review with a discussion of recent cross-cutting work related to uncertainty quantification in this field and a brief perspective on limitations, challenges, and potential growth areas for DL methods in materials science.
Similar content being viewed by others
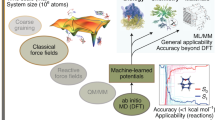
Machine-learned potentials for next-generation matter simulations
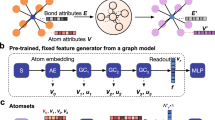
AtomSets as a hierarchical transfer learning framework for small and large materials datasets
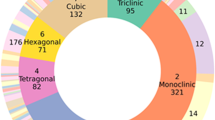
Automated classification of big X-ray diffraction data using deep learning models
Introduction.
“Processing-structure-property-performance” is the key mantra in Materials Science and Engineering (MSE) 1 . The length and time scales of material structures and phenomena vary significantly among these four elements, adding further complexity 2 . For instance, structural information can range from detailed knowledge of atomic coordinates of elements to the microscale spatial distribution of phases (microstructure), to fragment connectivity (mesoscale), to images and spectra. Establishing linkages between the above components is a challenging task.
Both experimental and computational techniques are useful to identify such relationships. Due to rapid growth in automation in experimental equipment and immense expansion of computational resources, the size of public materials datasets has seen exponential growth. Several large experimental and computational datasets 3 , 4 , 5 , 6 , 7 , 8 , 9 , 10 have been developed through the Materials Genome Initiative (MGI) 11 and the increasing adoption of Findable, Accessible, Interoperable, Reusable (FAIR) 12 principles. Such an outburst of data requires automated analysis which can be facilitated by machine learning (ML) techniques 13 , 14 , 15 , 16 , 17 , 18 , 19 , 20 .
Deep learning (DL) 21 , 22 is a specialized branch of machine learning (ML). Originally inspired by biological models of computation and cognition in the human brain 23 , 24 , one of DL’s major strengths is its potential to extract higher-level features from the raw input data.
DL applications are rapidly replacing conventional systems in many aspects of our daily lives, for example, in image and speech recognition, web search, fraud detection, email/spam filtering, financial risk modeling, and so on. DL techniques have been proven to provide exciting new capabilities in numerous fields (such as playing Go 25 , self-driving cars 26 , navigation, chip design, particle physics, protein science, drug discovery, astrophysics, object recognition 27 , etc).
Recently DL methods have been outperforming other machine learning techniques in numerous scientific fields, such as chemistry, physics, biology, and materials science 20 , 28 , 29 , 30 , 31 , 32 . DL applications in MSE are still relatively new, and the field has not fully explored its potential, implications, and limitations. DL provides new approaches for investigating material phenomena and has pushed materials scientists to expand their traditional toolset.
DL methods have been shown to act as a complementary approach to physics-based methods for materials design. While large datasets are often viewed as a prerequisite for successful DL applications, techniques such as transfer learning, multi-fidelity modelling, and active learning can often make DL feasible for small datasets as well 33 , 34 , 35 , 36 .
Traditionally, materials have been designed experimentally using trial and error methods with a strong dose of chemical intuition. In addition to being a very costly and time-consuming approach, the number of material combinations is so huge that it is intractable to study experimentally, leading to the need for empirical formulation and computational methods. While computational approaches (such as density functional theory, molecular dynamics, Monte Carlo, phase-field, finite elements) are much faster and cheaper than experiments, they are still limited by length and time scale constraints, which in turn limits their respective domains of applicability. DL methods can offer substantial speedups compared to conventional scientific computing, and, for some applications, are reaching an accuracy level comparable to physics-based or computational models.
Moreover, entering a new domain of materials science and performing cutting-edge research requires years of education, training, and the development of specialized skills and intuition. Fortunately, we now live in an era of increasingly open data and computational resources. Mature, well-documented DL libraries make DL research much more easily accessible to newcomers than almost any other research field. Testing and benchmarking methodologies such as underfitting/overfitting/cross-validation 15 , 16 , 37 are common knowledge, and standards for measuring model performance are well established in the community.
Despite their many advantages, DL methods have disadvantages too, the most significant one being their black-box nature 38 which may hinder physical insights into the phenomena under examination. Evaluating and increasing the interpretability and explainability of DL models remains an active field of research. Generally a DL model has a few thousand to millions of parameters, making model interpretation and direct generation of scientific insight difficult.
Although there are several good recent reviews of ML applications in MSE 15 , 16 , 17 , 19 , 39 , 40 , 41 , 42 , 43 , 44 , 45 , 46 , 47 , 48 , 49 , DL for materials has been advancing rapidly, warranting a dedicated review to cover the explosion of research in this field. This article discusses some of the basic principles in DL methods and highlights major trends among the recent advances in DL applications for materials science. As the tools and datasets for DL applications in materials keep evolving, we provide a github repository ( https://github.com/deepmaterials/dlmatreview ) that can be updated as new resources are made publicly available.
General machine learning concepts
It is beyond the scope of this article to give a detailed hands-on introduction to Deep Learning. There are many materials for this purpose, for example, the free online book “Neural Networks and Deep Learning” by Michael Nielsen ( http://neuralnetworksanddeeplearning.com ), Deep Learning by Goodfellow et al. 21 , and multiple online courses at Coursera, Udemy, and so on. Rather, this article aims to motivate materials scientist researchers in the types of problems that are amenable to DL, and to introduce some of the basic concepts, jargon, and materials-specific databases and software (at the time of writing) as a helpful on-ramp to help get started. With this in mind, we begin with a very basic introduction to Deep learning.
Artificial intelligence (AI) 13 is the development of machines and algorithms that mimic human intelligence, for example, by optimizing actions to achieve certain goals. Machine learning (ML) is a subset of AI, and provides the ability to learn without explicitly being programmed for a given dataset such as playing chess, social network recommendation etc. DL, in turn, is the subset of ML that takes inspiration from biological brains and uses multilayer neural networks to solve ML tasks. A schematic of AI-ML-DL context and some of the key application areas of DL in the materials science and engineering field are shown in Fig. 1 .
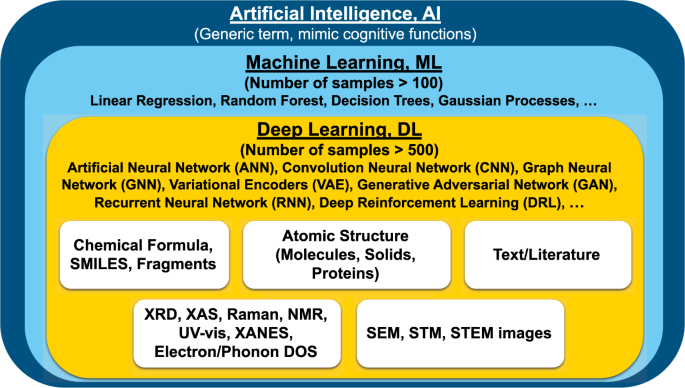
Deep learning is considered a part of machine learning, which is contained in an umbrella term artificial intelligence.
Some of the commonly used ML technologies are linear regression, decision trees, and random forest in which generalized models are trained to learn coefficients/weights/parameters for a given dataset (usually structured i.e., on a grid or a spreadsheet).
Applying traditional ML techniques to unstructured data (such as pixels or features from an image, sounds, text, and graphs) is challenging because users have to first extract generalized meaningful representations or features themselves (such as calculating pair-distribution for an atomic structure) and then train the ML models. Hence, the process becomes time-consuming, brittle, and not easily scalable. Here, deep learning (DL) techniques become more important.
DL methods are based on artificial neural networks and allied techniques. According to the “universal approximation theorem” 50 , 51 , neural networks can approximate any function to arbitrary accuracy. However, it is important to note that the theorem doesn’t guarantee that the functions can be learnt easily 52 .
Neural networks
A perceptron or a single artificial neuron 53 is the building block of artificial neural networks (ANNs) and performs forward propagation of information. For a set of inputs [ x 1 , x 2 , . . . , x m ] to the perceptron, we assign floating number weights (and biases to shift wights) [ w 1 , w 2 , . . . , w m ] and then we multiply them correspondingly together to get a sum of all of them. Some of the common software packages allowing NN trainings are: PyTorch 54 , Tensorflow 55 , and MXNet 56 . Please note that certain commercial equipment, instruments, or materials are identified in this paper in order to specify the experimental procedure adequately. Such identification is not intended to imply recommendation or endorsement by NIST, nor is it intended to imply that the materials or equipment identified are necessarily the best available for the purpose.
Activation function
Activation functions (such as sigmoid, hyperbolic tangent (tanh), rectified linear unit (ReLU), leaky ReLU, Swish) are the critical nonlinear components that enable neural networks to compose many small building blocks to learn complex nonlinear functions. For example, the sigmoid activation maps real numbers to the range (0, 1); this activation function is often used in the last layer of binary classifiers to model probabilities. The choice of activation function can affect training efficiency as well as final accuracy 57 .
Loss function, gradient descent, and normalization
The weight matrices of a neural network are initialized randomly or obtained from a pre-trained model. These weight matrices are multiplied with the input matrix (or output from a previous layer) and subjected to a nonlinear activation function to yield updated representations, which are often referred to as activations or feature maps. The loss function (also known as an objective function or empirical risk) is calculated by comparing the output of the neural network and the known target value data. Typically, network weights are iteratively updated via stochastic gradient descent algorithms to minimize the loss function until the desired accuracy is achieved. Most modern deep learning frameworks facilitate this by using reverse-mode automatic differentiation 58 to obtain the partial derivatives of the loss function with respect to each network parameter through recursive application of the chain rule. Colloquially, this is also known as back-propagation.
Common gradient descent algorithms include: Stochastic Gradient Descent (SGD), Adam, Adagrad etc. The learning rate is an important parameter in gradient descent. Except for SGD, all other methods use adaptive learning parameter tuning. Depending on the objective such as classification or regression, different loss functions such as Binary Cross Entropy (BCE), Negative Log likelihood (NLLL) or Mean Squared Error (MSE) are used.
The inputs of a neural network are generally scaled i.e., normalized to have zero mean and unit standard deviation. Scaling is also applied to the input of hidden layers (using batch or layer normalization) to improve the stability of ANNs.
Epoch and mini-batches
A single pass of the entire training data is called an epoch, and multiple epochs are performed until the weights converge. In DL, datasets are usually large and computing gradients for the entire dataset and network becomes challenging. Hence, the forward passes are done with small subsets of the training data called mini-batches.
Underfitting, overfitting, regularization, and early stopping
During an ML training, the dataset is split into training, validation, and test sets. The test set is never used during the training process. A model is said to be underfitting if the model performs poorly on the training set and lacks the capacity to fully learn the training data. A model is said to overfit if the model performs too well on the training data but does not perform well on the validation data. Overfitting is controlled with regularization techniques such as L2 regularization, dropout, and early stopping 37 .
Regularization discourages the model from simply memorizing the training data, resulting in a model that is more generalizable. Overfitting models are often characterized by neurons that have weights with large magnitudes. L2 regularization reduces the possibility of overfitting by adding an additional term to the loss function that penalizes the large weight values, keeping the values of the weights and biases small during training. Another popular regularization is dropout 59 in which we randomly set the activations for an NN layer to zero during training. Similar to bagging 60 , the use of dropout brings about the same effect of training a collection of randomly chosen models which prevents the co-adaptations among the neurons, consequently reducing the likelihood of the model from overfitting. In early stopping, further epochs for training are stopped before the model overfits i.e., accuracy on the validation set flattens or decreases.
Convolutional neural networks
Convolutional neural networks (CNN) 61 can be viewed as a regularized version of multilayer perceptrons with a strong inductive bias for learning translation-invariant image representations. There are four main components in CNNs: (a) learnable convolution filterbanks, (b) nonlinear activations, (c) spatial coarsening (via pooling or strided convolution), (d) a prediction module, often consisting of fully connected layers that operate on a global instance representation.
In CNNs we use convolution functions with multiple kernels or filters with trainable and shared weights or parameters, instead of general matrix multiplication. These filters/kernels are matrices with a relatively small number of rows and columns that convolve over the input to automatically extract high-level local features in the form of feature maps. The filters slide/convolve (element-wise multiply) across the input with a fixed number of strides to produce the feature map and the information thus learnt is passed to the hidden/fully connected layers. Depending on the input data, these filters can be one, two, or three-dimensional.
Similar to the fully connected NNs, nonlinearities such as ReLU are then applied that allows us to deal with nonlinear and complicated data. The pooling operation preserves spatial invariance, downsamples and reduces the dimension of each feature map obtained after convolution. These downsampling/pooling operations can be of different types such as maximum-pooling, minimum-pooling, average pooling, and sum pooling. After one or more convolutional and pooling layers, the outputs are usually reduced to a one-dimensional global representation. CNNs are especially popular for image data.
Graph neural networks
Graphs and their variants.
Classical CNNs as described above are based on a regular grid Euclidean data (such as 2D grid in images). However, real-life data structures, such as social networks, segments of images, word vectors, recommender systems, and atomic/molecular structures, are usually non-Euclidean. In such cases, graph-based non-Euclidean data structures become especially important.
Mathematically, a graph G is defined as a set of nodes/vertices V , a set of edges/links, E and node features, X : G = ( V , E , X ) 62 , 63 , 64 and can be used to represent non-Euclidean data. An edge is formed between a pair of two nodes and contains the relation information between the nodes. Each node and edge can have attributes/features associated with it. An adjacency matrix A is a square matrix indicating connections between the nodes or not in the form of 1 (connected) and 0 (unconnected). A graph can be of various types such as: undirected/directed, weighted/unweighted, homogeneous/heterogeneous, static/dynamic.
An undirected graph captures symmetric relations between nodes, while a directed one captures asymmetric relations such that A i j ≠ A j i . In a weighted graph, each edge is associated with a scalar weight rather than just 1s and 0s. In a homogeneous graph, all the nodes represent instances of the same type, and all the edges capture relations of the same type while in a heterogeneous graph, the nodes and edges can be of different types. Heterogeneous graphs provide an easy interface for managing nodes and edges of different types as well as their associated features. When input features or graph topology vary with time, they are called dynamic graphs otherwise they are considered static. If a node is connected to another node more than once it is termed a multi-graph.
Types of GNNs
At present, GNNs are probably the most popular AI method for predicting various materials properties based on structural information 33 , 65 , 66 , 67 , 68 , 69 . Graph neural networks (GNNs) are DL methods that operate on graph domain and can capture the dependence of graphs via message passing between the nodes and edges of graphs. There are two key steps in GNN training: (a) we first aggregate information from neighbors and (b) update the nodes and/or edges. Importantly, aggregation is permutation invariant. Similar to the fully connected NNs, the input node features, X (with embedding matrix) are multiplied with the adjacency matrix and the weight matrices and then multiplied with the nonlinear activation function to provide outputs for the next layer. This method is called the propagation rule.
Based on the propagation rule and aggregation methodology, there could be different variants of GNNs such as Graph convolutional network (GCN) 70 , Graph attention network (GAT) 71 , Relational-GCN 72 , graph recurrent network (GRN) 73 , Graph isomerism network (GIN) 74 , and Line graph neural network (LGNN) 75 . Graph convolutional neural networks are the most popular GNNs.
Sequence-to-sequence models
Traditionally, learning from sequential inputs such as text involves generating a fixed-length input from the data. For example, the “bag-of-words” approach simply counts the number of instances of each word in a document and produces a fixed-length vector that is the size of the overall vocabulary.
In contrast, sequence-to-sequence models can take into account sequential/contextual information about each word and produce outputs of arbitrary length. For example, in named entity recognition (NER), an input sequence of words (e.g., a chemical abstract) is mapped to an output sequence of “entities” or categories where every word in the sequence is assigned a category.
An early form of sequence-to-sequence model is the recurrent neural network, or RNN. Unlike the fully connected NN architecture, where there is no connection between hidden nodes in the same layer, but only between nodes in adjacent layers, RNN has feedback connections. Each hidden layer can be unfolded and processed similarly to traditional NNs sharing the same weight matrices. There are multiple types of RNNs, of which the most common ones are: gated recurrent unit recurrent neural network (GRURNN), long short-term memory (LSTM) network, and clockwork RNN (CW-RNN) 76 .
However, all such RNNs suffer from some drawbacks, including: (i) difficulty of parallelization and therefore difficulty in training on large datasets and (ii) difficulty in preserving long-range contextual information due to the “vanishing gradient” problem. Nevertheless, as we will later describe, LSTMs have been successfully applied to various NER problems in the materials domain.
More recently, sequence-to-sequence models based on a “transformer” architecture, such as Google’s Bidirectional Encoder Representations from Transformers (BERT) model 77 , have helped address some of the issues of traditional RNNs. Rather than passing a state vector that is iterated word-by-word, such models use an attention mechanism to allow access to all previous words simultaneously without explicit time steps. This mechanism facilitates parallelization and also better preserves long-term context.
Generative models
While the above DL frameworks are based on supervised machine learning (i.e., we know the target or ground truth data such as in classification and regression) and discriminative (i.e., learn differentiating features between various datasets), many AI tasks are based on unsupervised (such as clustering) and are generative (i.e., aim to learn underlying distributions) 78 .
Generative models are used to (a) generate data samples similar to the training set with variations i.e., augmentation and for synthetic data, (b) learn good generalized latent features, (c) guide mixed reality applications such as virtual try-on. There are various types of generative models, of which the most common are: (a) variational encoders (VAE), which explicitly define and learn likelihood of data, (b) Generative adversarial networks (GAN), which learn to directly generate samples from model’s distribution, without defining any density function.
A VAE model has two components: namely encoder and decoder. A VAE’s encoder takes input from a target distribution and compresses it into a low-dimensional latent space. Then the decoder takes that latent space representation and reproduces the original image. Once the network is trained, we can generate latent space representations of various images, and interpolate between these before forwarding them through the decoder which produces new images. A VAE is similar to a principal component analysis (PCA) but instead of linear data assumption in PCA, VAEs work in nonlinear domain. A GAN model also has two components: namely generator, and discriminator. GAN’s generator generates fake/synthetic data that could fool the discriminator. Its discriminator tries to distinguish fake data from real ones. This process is also termed as “min-max two-player game.” We note that VAE models learn the hidden state distributions during the training process, while GAN’s hidden state distributions are predefined. Rather GAN generators serve to generate images that could fool the discriminator. These techniques are widely used for images and spectra and have also been recently applied to atomic structures.
Deep reinforcement learning
Reinforcement learning (RL) deals with tasks in which a computational agent learns to make decisions by trial and error. Deep RL uses DL into the RL framework, allowing agents to make decisions from unstructured input data 79 . In traditional RL, Markov decision process (MDP) is used in which an agent at every timestep takes action to receive a scalar reward and transitions to the next state according to system dynamics to learn policy in order to maximize returns. However, in deep RL, the states are high-dimensional (such as continuous images or spectra) which act as an input to DL methods. DRL architectures can be either model-based or model-free.
Scientific machine learning
The nascent field of scientific machine learning (SciML) 80 is creating new opportunities across all paradigms of machine learning, and deep learning in particular. SciML is focused on creating ML systems that incorporate scientific knowledge and physical principles, either directly in the specific form of the model or indirectly through the optimization algorithms used for training. This offers potential improvements in sample and training complexity, robustness (particularly under extrapolation), and model interpretability. One prominent theme can be found in ref. 57 . Such implementations usually involve applying multiple physics-based constraints while training a DL model 81 , 82 , 83 . One of the key challenges of universal function approximation is that a NN can quickly learn spurious features that have nothing to do with the features that a researcher could be actually interested in, within the data. In this sense, physics-based regularization can assist. Physics-based deep learning can also aid in inverse design problems, a challenging but important task 84 , 85 . On the flip side, deep Learning using Graph Neural Nets and symbolic regression (stochastically building symbolic expressions) has even been used to “discover” symbolic equations from data that capture known (and unknown) physics behind the data 86 , i.e., to deep learn a physics model rather than to use a physics model to constrain DL.
Overview of applications
Some aspects of successful DL application that require materials-science-specific considerations are:
acquiring large, balanced, and diverse datasets (often on the order of 10,000 data points or more),
determing an appropriate DL approach and suitable vector or graph representation of the input samples, and
selecting appropriate performance metrics relevant to scientific goals.
In the following sections we discuss some of the key areas of materials science in which DL has been applied with available links to repositories and datasets that help in the reproducibility and extensibility of the work. In this review we categorize materials science applications at a high level by the type of input data considered: 11 atomistic, 12 stoichiometric, 13 spectral, 14 image, and 15 text. We summarize prevailing machine learning tasks and their impact on materials research and development within each broad materials data modality.
Applications in atomistic representations
In this section, we provide a few examples of solving materials science problems with DL methods trained on atomistic data. The atomic structure of material usually consists of atomic coordinates and atomic composition information of material. An arbitrary number of atoms and types of elements in a system poses a challenge to apply traditional ML algorithms for atomistic predictions. DL-based methods are an obvious strategy to tackle this problem. There have been several previous attempts to represent crystals and molecules using fixed-size descriptors such as Coulomb matrix 87 , 88 , 89 , classical force field inspired descriptors (CFID) 90 , 91 , 92 , pair-distribution function (PRDF), Voronoi tessellation 93 , 94 , 95 . Recently graph neural network methods have been shown to surpass previous hand-crafted feature set 28 .
DL for atomistic materials applications include: (a) force-field development, (b) direct property predictions, (c) materials screening. In addition to the above points, we also elucidate upon some of the recent generative adversarial network and complimentary methods to atomistic aproaches.
Databases and software libraries
In Table 1 we provide some of the commonly used datasets used for atomistic DL models for molecules, solids, and proteins. We note that the computational methods used for different datasets are different and many of them are continuously evolving. Generally it takes years to generate such databases using conventional methods such as density functional theory; in contrast, DL methods can be used to make predictions with much reduced computational cost and reasonable accuracy.
Table 1 we provide DL software packages used for atomistic materials design. The type of models includes general property (GP) predictors and interatomic force fields (FF). The models have been demonstrated in molecules (Mol), solid-state materials (Sol), or proteins (Prot). For some force fields, high-performance large-scale implementations (LSI) that leverage paralleling computing exist. Some of these methods mainly used interatomic distances to build graphs while others use distances as well as bond-angle information. Recently, including bond angle within GNN has been shown to drastically improve the performance with comparable computational timings.
Force-field development
The first application includes the development of DL-based force fields (FF) 96 , 97 /interatomic potentials. Some of the major advantages of such applications are that they are very fast (on the order of hundreds to thousands times 64 ) for making predictions and solving the tenuous development of FFs, but the disadvantage is they still require a large dataset using computationally expensive methods to train.
Models such as Behler-Parrinello neural network (BPNN) and its variants 98 , 99 are used for developing interatomic potentials that can be used beyond just 0 K temperature and time-dependent behavior using molecular dynamics simulations such as for nanoparticles 100 . Such FF models have been developed for molecular systems, such as water, methane, and other organic molecules 99 , 101 as well as solids such as silicon 98 , sodium 102 , graphite 103 , and titania ( T i O 2 ) 104 .
While the above works are mainly based on NNs, there has also been the development of graph neural network force-field (GNNFF) framework 105 , 106 that bypasses both computational bottlenecks. GNNFF can predict atomic forces directly using automatically extracted structural features that are not only translationally invariant, but rotationally-covariant to the coordinate space of the atomic positions, i.e., the features and hence the predicted force vectors rotate the same way as the rotation of coordinates. In addition to the development of pure NN-based FFs, there have also been recent developments of combining traditional FFs such as bond-order potentials with NNs and ReaxFF with message passing neural network (MPNN) that can help mitigate the NNs issue for extrapolation 82 , 107 .
Direct property prediction from atomistic configurations
DL methods can be used to establish a structure-property relationship between atomic structure and their properties with high accuracy 28 , 108 . Models such as SchNet, crystal graph convolutional neural network (CGCNN), improved crystal graph convolutional neural network (iCGCNN), directional message passing neural network (DimeNet), atomistic line graph neural network (ALIGNN) and materials graph neural network (MEGNet) shown in Table 1 have been used to predict up to 50 properties of crystalline and molecular materials. These property datasets are usually obtained from ab-initio calculations. A schematic of such models shown in Fig. 2 . While SchNet, CGCNN, MEGNet are primarily based on atomic distances, iCGCNN, DimeNet, and ALIGNN models capture many-body interactions using GCNN.
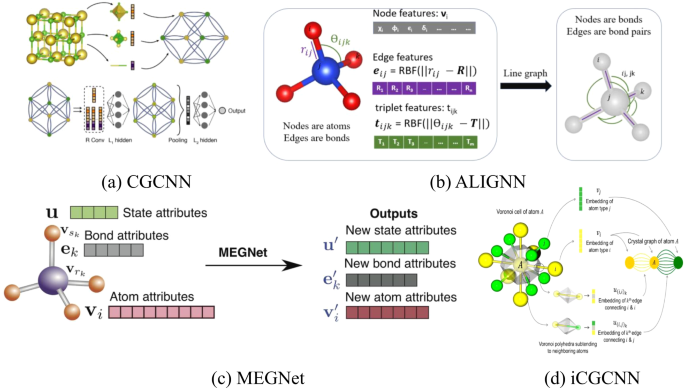
a CGCNN model in which crystals are converted to graphs with nodes representing atoms in the unit cell and edges representing atom connections. Nodes and edges are characterized by vectors corresponding to the atoms and bonds in the crystal, respectively [Reprinted with permission from ref. 67 Copyright 2019 American Physical Society], b ALIGNN 65 model in which the convolution layer alternates between message passing on the bond graph and its bond-angle line graph. c MEGNet in which the initial graph is represented by the set of atomic attributes, bond attributes and global state attributes [Reprinted with permission from ref. 33 Copyright 2019 American Chemical Society] model, d iCGCNN model in which multiple edges connect a node to neighboring nodes to show the number of Voronoi neighbors [Reprinted with permission from ref. 122 Copyright 2019 American Physical Society].
Some of these properties include formation energies, electronic bandgaps, solar-cell efficiency, topological spin-orbit spillage, dielectric constants, piezoelectric constants, 2D exfoliation energies, electric field gradients, elastic modulus, Seebeck coefficients, power factors, carrier effective masses, highest occupied molecular orbital, lowest unoccupied molecular orbital, energy gap, zero-point vibrational energy, dipole moment, isotropic polarizability, electronic spatial extent, internal energy.
For instance, the current state-of-the-art mean absolute error for formation energy for solids at 0 K is 0.022 eV/atom as obtained by the ALIGNN model 65 . DL is also heavily being used for predicting catalytic behavior of materials such as the Open Catalyst Project 109 which is driven by the DL methods materials design. There is an ongoing effort to continuously improve the models. Usually energy-based models such as formation and total energies are more accurate than electronic property-based models such as bandgaps and power factors.
In addition to molecules and solids, property predictions models have also been used for bio-materials such as proteins, which can be viewed as large molecules. There have been several efforts for predicting protein-based properties, such as binding affinity 66 and docking predictions 110 .
There have also been several applications for identifying reasonable chemical space using DL methods such as autoencoders 111 and reinforcement learning 112 , 113 , 114 for inverse materials design. Inverse materials design with techniques such as GAN deals with finding chemical compounds with suitable properties and act as complementary to forward prediction models. While such concepts have been widely applied to molecular systems, 115 , recently these methods have been applied to solids as well 116 , 117 , 118 , 119 , 120 .
Fast materials screening
DFT-based high-throughput methods are usually limited to a few thousands of compounds and take a long time for calculations, DL-based methods can aid this process and allow much faster predictions. DL-based property prediction models mentioned above can be used for pre-screening chemical compounds. Hence, DL-based tools can be viewed as a pre-screening tool for traditional methods such as DFT. For example, Xie et al. used CGCNN model to screen stable perovskite materials 67 as well hierarchical visualization of materials space 121 . Park et al. 122 used iCGCNN to screen T h C r 2 S i 2 -type materials. Lugier et al. used DL methods to predict thermoelectric properties 123 . Rosen et al. 124 used graph neural network models to predict the bandgaps of metal-organic frameworks. DL for molecular materials has been used to predict technologically important properties such as aqueous solubility 125 and toxicity 126 .
It should be noted that the full atomistic representations and the associated DL models are only possible if the crystal structure and atom positions are available. In practice, the precise atom positions are only available from DFT structural relaxations or experiments, and are one of the goals for materials discovery instead of the starting point. Hence, alternative methods have been proposed to bypass the necessity for atom positions in building DL models. For example, Jain and Bligaard 127 proposed the atomic position-independent descriptors and used a CNN model to learn the energies of crystals. Such descriptors include information based only on the symmetry (e.g., space group and Wyckoff position). In principle, the method can be applied universally in all crystals. Nevertheless, the model errors tend to be much higher than graph-based models. Similar coarse-grained representation using Wyckoff representation was also used by Goodall et al. 128 . Alternatively, Zuo et al. 129 started from the hypothetical structures without precise atom positions, and used a Bayesian optimization method coupled with a MEGNet energy model as an energy evaluator to perform direct structural relaxation. Applying the Bayesian optimization with symmetry relaxation (BOWSR) algorithm successfully discovered ReWB (Pca2 1 ) and MoWC 2 (P6 3 /mmc) hard materials, which were then experimentally synthesized.
Applications in chemical formula and segment representations
One of the earliest applications for DL included SMILES for molecules, elemental fractions and chemical descriptors for solids, and sequence of protein names as descriptors. Such descriptors lack explicit inclusion of atomic structure information but are still useful for various pre-screening applications for both theoretical and experimental data.
SMILES and fragment representation
The simplified molecular-input line-entry system (SMILES) is a method to represent elemental and bonding for molecular structures using short American Standard Code for Information Interchange (ASCII) strings. SMILES can express structural differences including the chirality of compounds, making it more useful than a simply chemical formula. A SMILES string is a simple grid-like (1-D grid) structure that can represent molecular sequences such as DNA, macromolecules/polymers, protein sequences also 130 , 131 . In addition to the chemical constituents as in the chemical formula, bondings (such as double and triple bondings) are represented by special symbols (such as ’=’ and ’#’). The presence of a branch point indicated using a left-hand bracket “(” while the right-hand bracket “)” indicates that all the atoms in that branch have been taken into account. SMILES strings are represented as a distributed representation termed a SMILES feature matrix (as a sparse matrix), and then we can apply DL to the matrix similar to image data. The length of the SMILES matrix is generally kept fixed (such as 400) during training and in addition to the SMILES multiple elemental attributes and bonding attributes (such as chirality, aromaticity) can be used. Key DL tasks for molecules include (a) novel molecule design, (b) molecule screening.
Novel molecules with target properties can designed using VAE, GAN and RNN based methods 132 , 133 , 134 . These DL-generated molecules might not be physically valid, but the goal is to train the model to learn the patterns in SMILES strings such that the output resembles valid molecules. Then chemical intuitions can be further used to screen the molecules. DL for SMILES can also be used for molecularscreening such as to predict molecular toxicity. Some of the common SMILES datasets are: ZINC 135 , Tox21 136 , and PubChem 137 .
Due to the limitations to enforce the generation of valid molecular structures from SMILES, fragment-based models are developed such as DeepFrag and DeepFrag-K 138 , 139 . In fragment-based models, a ligand/receptor complex is removed and then a DL model is trained to predict the most suitable fragment substituent. A set of useful tools for SMILES and fragment representations are provided in Table 2 .
Chemical formula representation
There are several ways of using the chemical formula-based representations for building ML/DL models, beginning with a simple vector of raw elemental fractions 140 , 141 or of weight percentages of alloying compositions 142 , 143 , 144 , 145 , as well as more sophisticated hand-crafted descriptors or physical attributes to add known chemistry knowledge (e.g., electronegativity, valency, etc. of constituent elements) to the feature representations 146 , 147 , 148 , 149 , 150 , 151 . Statistical and mathematical operations such as average, max, min, median, mode, and exponentiation can be carried out on elemental properties of the constituent elements to get a set of descriptors for a given compound. The number of such composition-based features can range from a few dozens to a few hundreds. One of the commonly used representations that have been shown to work for a variety of different use-cases is the materials agnostic platform for informatics and exploration (MagPie) 150 . All these composition-based representations can be used with both traditional ML methods such as Random Forest as well as DL.
It is relevant to note that ElemNet 141 , which is a 17-layer neural network composed of fully connected layers and uses only raw elemental fractions as input, was found to significantly outperform traditional ML methods such as Random Forest, even when they were allowed to use more sophisticated physical attributes based on MagPie as input. Although no periodic table information was provided to the model, it was found to self-learn some interesting chemistry, like groups (element similarity) and charge balance (element interaction). It was also able to predict phase diagrams on unseen materials systems, underscoring the power of DL for representation learning directly from raw inputs without explicit feature extraction. Further increasing the depth of the network was found to adversely affect the model accuracy due to the vanishing gradient problem. To address this issue, Jha et al. 152 developed IRNet, which uses individual residual learning to allow a smoother flow of gradients and enable deeper learning for cases where big data is available. IRNet models were tested on a variety of big and small materials datasets, such as OQMD, AFLOW, Materials Project, JARVIS, using different vector-based materials representations (element fractions, MagPie, structural) and were found to not only successfully alleviate the vanishing gradient problem and enable deeper learning, but also lead to significantly better model accuracy as compared to plain deep neural networks and traditional ML techniques for a given input materials representation in the presence of big data 153 . Further, graph-based methods such as Roost 154 have also been developed which can outperform many similar techniques.
Such methods have been used for diverse DFT datasets mentioned above in Table 1 as well as experimental datasets such as SuperCon 155 , 156 for quick pre-screening applications. In terms of applications, they have been applied for predicting properties such as formation energy 141 , bandgap, and magnetization 152 , superconducting temperatures 156 , bulk, and shear modulus 153 . They have also been used for transfer learning across datasets for enhanced predictive accuracy on small data 34 , even for different source and target properties 157 , which is especially useful to build predictive models for target properties for which big source datasets may not be readily available.
There have been libraries of such descriptors developed such as MatMiner 151 and DScribe 158 . Some examples of such models are given in Table 2 . Such representations are especially useful for experimental datasets such as those for superconducting materials where the atomic structure is not tabulated. However, these representations cannot distinguish different polymorphs of a system with different point groups and space groups. It has been recently shown that although composition-based representations can help build ML/DL models to predict some properties like formation energy with remarkable accuracy, it does not necessarily translate to accurate predictions of other properties such as stability, when compared to DFT’s own accuracy 159 .
Spectral models
When electromagnetic radiation hits materials, the interaction between the radiation and matter measured as a function of the wavelength or frequency of the radiation produces a spectroscopic signal. By studying spectroscopy, researchers can gain insights into the materials’ composition, structural, and dynamic properties. Spectroscopic techniques are foundational in materials characterization. For instance, X-ray diffraction (XRD) has been used to characterize the crystal structure of materials for more than a century. Spectroscopic analysis can involve fitting quantitative physical models (for example, Rietveld refinement) or more empirical approaches such as fitting linear combinations of reference spectra, such as with x-ray absorption near-edge spectroscopy (XANES). Both approaches require a high degree of researcher expertise through careful design of experiments; specification, revision, and iterative fitting of physical models; or the availability of template spectra of known materials. In recent years, with the advances in high-throughput experiments and computational data, spectroscopic data has multiplied, giving opportunities for researchers to learn from the data and potentially displace the conventional methods in analyzing such data. This section covers emerging DL applications in various modes of spectroscopic data analysis, aiming to offer practice examples and insights. Some of the applications are shown in Fig. 3 .
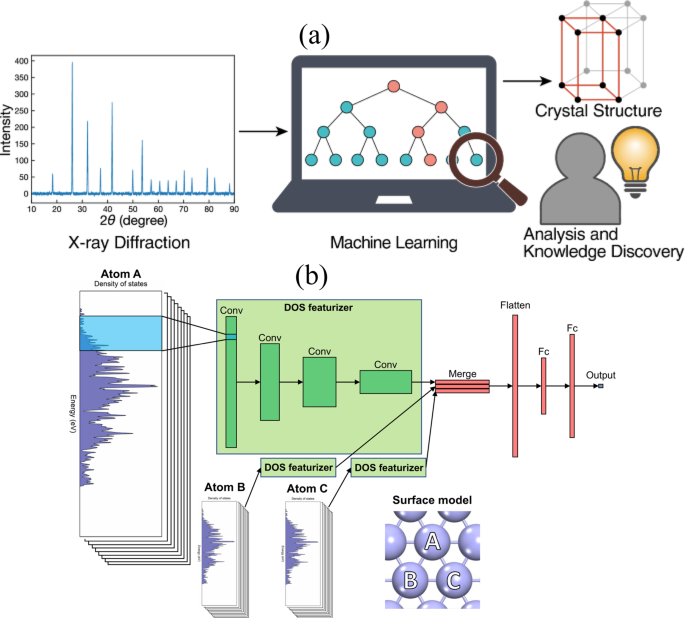
a Predicting structure information from the X-ray diffraction 374 , Reprinted according to the terms of the CC-BY license 374 . Copyright 2020. b Predicting catalysis properties from computational electronic density of states data. Reprinted according to the terms of the CC-BY license 202 . Copyright 2021.
Currently, large-scale and element-diverse spectral data mainly exist in computational databases. For example, in ref. 160 , the authors calculated the infrared spectra, piezoelectric tensor, Born effective charge tensor, and dielectric response as a part of the JARVIS-DFT DFPT database. The Materials Project has established the largest computational X-ray absorption database (XASDb), covering the K-edge X-ray near-edge fine structure (XANES) 161 , 162 and the L-edge XANES 163 of a large number of material structures. The database currently hosts more than 400,000 K-edge XANES site-wise spectra and 90,000 L-edge XANES site-wise spectra of many compounds in the Materials Project. There are considerably fewer experimental XAS spectra, being on the order of hundreds, as seen in the EELSDb and the XASLib. Collecting large experimental spectra databases that cover a wide range of elements is a challenging task. Collective efforts focused on curating data extracted from different sources, as found in the RRUFF Raman, XRD and chemistry database 164 , the open Raman database 165 , and the SOP spectra library 166 . However, data consistency is not guaranteed. It is also now possible for contributors to share experimental data in a Materials Project curated database, MPContribs 167 . This database is supported by the US Department of Energy (DOE) providing some expectation of persistence. Entries can be kept private or published and are linked to the main materials project computational databases. There is an ongoing effort to capture data from DOE-funded synchrotron light sources ( https://lightsources.materialsproject.org/ ) into MPContribs in the future.
Recent advances in sources, detectors, and experimental instrumentation have made high-throughput measurements of experimental spectra possible, giving rise to new possibilities for spectral data generation and modeling. Such examples include the HTEM database 10 that contains 50,000 optical absorption spectra and the UV-Vis database of 180,000 samples from the Joint Center for Artificial Photosynthesis. Some of the common spectra databases for spectra data are shown in Table 3 . There are beginning to appear cloud-based software as a service platforms for high-throughput data analysis, for example, pair-distribution function (PDF) in the cloud ( https://pdfitc.org ) 168 which are backed by structured databases, where data can be kept private or made public. This transition to the cloud from data analysis software installed and run locally on a user’s computer will facilitate the sharing and reuse of data by the community.
Applications
Due to the widespread deployment of XRD across many materials technologies, XRD spectra became one of the first test grounds for DL models. Phase identification from XRD can be mapped into a classification task (assuming all phases are known) or an unsupervised clustering task. Unlike the traditional analysis of XRD data, where the spectra are treated as convolved, discrete peak positions and intensities, DL methods treat the data as a continuous pattern similar to an image. Unfortunately, a significant number of experimental XRD datasets in one place are not readily available at the moment. Nevertheless, extensive, high-quality crystal structure data makes creating simulated XRD trivial.
Park et al. 169 calculated 150,000 XRD patterns from the Inorganic Crystal Structure Database (ICSD) structural database 170 and then used CNN models to predict structural information from the simulated XRD patterns. The accuracies of the CNN models reached 81.14%, 83.83%, and 94.99% for space-group, extinction-group, and crystal-system classifications, respectively.
Liu et al. 95 obtained similar accuracies by using a CNN for classifying atomic pair-distribution function (PDF) data into space groups. The PDF is obtained by Fourier transforming XRD into real space and is particularly useful for studying the local and nanoscale structure of materials. In the case of the PDF, models were trained, validated, and tested on simulated data from the ICSD. However, the trained model showed excellent performance when given experimental data, something that can be a challenge in XRD data because of the different resolutions and line-shapes of the diffraction data depending on specifics of the sample and experimental conditions. The PDF seems to be more robust against these aspects.
Similarly, Zaloga et al. 171 also used the ICSD database for XRD pattern generation and CNN models to classify crystals. The models achieved 90.02% and 79.82% accuracy for crystal systems and space groups, respectively.
It should be noted that the ICSD database contains many duplicates, and such duplicates should be filtered out to avoid information leakage. There is also a large difference in the number of structures represented in each space group (the label) in the database resulting in data normalization challenges.
Lee et al. 172 developed a CNN model for phase identification from samples consisting of a mixture of several phases in a limited chemical space relevant for battery materials. The training data are mixed patterns consisting of 1,785,405 synthetic XRD patterns from the Sr-Li-Al-O phase space. The resulting CNN can not only identify the phases but also predict the compound fraction in the mixture. A similar CNN was utilized by Wang et al. 173 for fast identification of metal-organic frameworks (MOFs), where experimental spectral noise was extracted and then synthesized into the theoretical XRD for training data augmentation.
An alternative idea was proposed by Dong et al. 174 . Instead of recognizing only phases from the CNN, a proposed “parameter quantification network” (PQ-Net) was able to extract physico-chemical information. The PQ-Net yields accurate predictions for scale factors, crystallite size, and lattice parameters for simulated and experimental XRD spectra. The work by Aguiar et al. 175 took a step further and proposed a modular neural network architecture that enables the combination of diffraction patterns and chemistry data and provided a ranked list of predictions. The ranked list predictions provide user flexibility and overcome some aspects of overconfidence in model predictions. In practical applications, AI-driven XRD identification can be beneficial for high-throughput materials discovery, as shown by Maffettone et al. 176 . In their work, an ensemble of 50 CNN models was trained on synthetic data reproducing experimental variations (missing peaks, broadening, peaking shifting, noises). The model ensemble is capable of predicting the probability of each category label. A similar data augmentation idea was adopted by Oviedo et al. 177 , where experimental XRD data for 115 thin-film metal-halides were measured, and CNN models trained on the augmented XRD data achieved accuracies of 93% and 89% for classifying dimensionality and space group, respectively.
Although not a DL method, an unsupervised machine learning approach, non-negative matrix factorization (NMF), is showing great promise for yielding chemically relevant XRD spectra from time- or spatially-dependent sets of diffraction patterns. NMF is closely related to principle component analysis in that it takes a set of patterns as a matrix and then compresses the data by reducing the dimensionality by finding the most important components. In NMF a constraint is applied that all the components and their weights must be strictly positive. This often corresponds to a real physical situation (for example, spectra tend to be positive, as are the weights of chemical constituents). As a result, it appears that the mathematical decomposition often results in interpretable, physically meaningful, components and weights, as shown by Liu et al. for PDF data 178 . An extension of this showed that in a spatially resolved study, NMF could be used to extract chemically resolved differential PDFs (similar to the information in EXAFS) from non-chemically resolved PDF measurements 179 . NMF is very quick and easy to apply and can be applied to just about any set of spectra. It is likely to become widely used and is being implemented in the PDFitc.org website to make it more accessible to potential users.
Other than XRD, the XAS, Raman, and infrared spectra, also contain rich structure-dependent spectroscopic information about the material. Unlike XRD, where relatively simple theories and equations exist to relate structures to the spectral patterns, the relationships between general spectra and structures are somewhat elusive. This difficulty has created a higher demand for machine learning models to learn structural information from other spectra.
For instance, the case of X-ray absorption spectroscopy (XAS), including the X-ray absorption near-edge spectroscopy (XANES) and extended X-ray absorption fine structure (EXAFS), is usually used to analyze the structural information on an atomic level. However, the high signal-to-noise XANES region has no equation for data fitting. DL modeling of XAS data is fascinating and offers unprecedented insights. Timoshenko et al. used neural networks to predict the coordination numbers of Pt 180 and Cu 181 in nanoclusters from the XANES. Aside from the high accuracies, the neural network also offers high prediction speed and new opportunities for quantitative XANES analysis. Timoshenko et al. 182 further carried out a novel analysis of EXAFS using DL. Although EXAFS analysis has an explicit equation to fit, the study is limited to the first few coordination shells and on relatively ordered materials. Timoshenko et al. 182 first transformed the EXAFS data into 2D maps with a wavelet transform and then supplied the 2D data to a neural network model. The model can instantly predict relatively long-range radial distribution functions, offering in situ local structure analysis of materials. The advent of high-throughput XAS databases has recently unveiled more possibilities for machine learning models to be deployed using XAS data. For example, Zheng et al. 161 used an ensemble learning method to match and fast search new spectra in the XASDb. Later, the same authors showed that random forest models outperform DL models such as MLPs or CNNs in directly predicting atomic environment labels from the XANES spectra 183 . Similar approaches were also adopted by Torrisi et al. 184 In practical applications, Andrejevic et al. 185 used the XASDb data together with the topological materials database. They constructed CNN models to classify the topology of materials from the XANES and symmetry group inputs. The model correctly predicted 81% topological and 80% trivial cases and achieved 90% accuracy in material classes containing certain elements.
Raman, infrared, and other vibrational spectroscopies provide structural fingerprints and are usually used to discriminate and estimate the concentration of components in a mixture. For example, Madden et al. 186 have used neural network models to predict the concentration of illicit materials in a mixture using the Raman spectra. Interestingly, several groups have independently found that DL models outperform chemometrics analysis in vibrational spectroscopies 187 , 188 . For learning vibrational spectra, the number of training spectra is usually less than or on the order of the number of features (intensity points), and the models can easily overfit. Hence, dimensional reduction strategies are commonly used to compress the information dimension using, for example, principal component analysis (PCA) 189 , 190 . DL approaches do not have such concerns and offer elegant and unified solutions. For example, Liu et al. 191 applied CNN models to the Raman spectra in the RRUFF spectral database and show that CNN models outperform classical machine learning models such as SVM in classification tasks. More DL applications in vibrational spectral analysis can be found in a recent review by Yang et al. 192 .
Although most current DL work focuses on the inverse problem, i.e., predicting structural information from the spectra, some innovative approaches also solve the forward problems by predicting the spectra from the structure. In this case, the spectroscopy data can be viewed simply as a high-dimensional material property of the structure. This is most common in molecular science, where predicting the infrared spectra 193 , molecular excitation spectra 194 , is of particular interest. In the early 2000s, Selzer et al. 193 and Kostka et al. 195 attempted predicting the infrared spectra directly from the molecular structural descriptors using neural networks. Non-DL models can also perform such tasks to a reasonable accuracy 196 . For DL models, Chen et al. 197 used a Euclidean neural network (E(3)NN) to predict the phonon density of state (DOS) spectra 198 from atom positions and element types. The E(3)NN model captures symmetries of the crystal structures, with no need to perform data augmentation to achieve target invariances. Hence the E(3)NN model is extremely data-efficient and can give reliable DOS spectra prediction and heat capacity using relatively sparse data of 1200 calculation results on 65 elements. A similar idea was also used to predict the XAS spectra. Carbone et al. 199 used a message passing neural network (MPNN) to predict the O and N K-edge XANES spectra from the molecular structures in the QM9 database 7 . The training XANES data were generated using the FEFF package 200 . The trained MPNN model reproduced all prominent peaks in the predicted XANES, and 90% of the predicted peaks are within 1 eV of the FEFF calculations. Similarly, Rankine et al. 201 started from the two-body radial distribution function (RDC) and used a deep neural network model to predict the Fe K-edge XANES spectra for arbitrary local environments.
In addition to learn the structure-spectra or spectra-structure relationships, a few works have also explored the possibility of relating spectra to other material properties in a non-trivial way. The DOSnet proposed by Fung et al. 202 (Fig. 3 b) uses the electronic DOS spectra calculated from DFT as inputs to a CNN model to predict the adsorption energies of H, C, N, O, S and their hydrogenated counterparts, CH, CH 2 , CH 3 , NH, OH, and SH, on bimetallic alloy surfaces. This approach extends the previous d-band theory 203 , where only the d-band center, a scalar, was used to correlate with the adsorption energy on transition metals. Similarly, Kaundinya et al. 204 used Atomistic Line Graph Neural Network (ALIGNN) to predict DOS for 56,000 materials in the JARVIS-DFT database using a direct discretized spectrum (D-ALIGNN), and a compressed low-dimensional representation using an autoencoder (AE-ALIGNN). Stein et al. 205 tried to learn the mapping between the image and the UV-vis spectrum of the material using the conditional variational encoder (cVAE) with neural network models as the backbone. Such models can generate the UV-vis spectrum directly from a simple material image, offering much faster material characterizations. Predicting gas adsorption isotherms for direct air capture (DAC) are also an important application of spectra-based DL models. There have been several important works 206 , 207 for CO 2 capture with high-performance metal-organic frameworks (MOFs) which are important for mitigating climate change issues.
Image-based models
Computer vision is often credited as precipitating the current wave of mainstream DL applications a decade ago 208 . Naturally, materials researchers have developed a broad portfolio of applications of computer vision for accelerating and improving image-based material characterization techniques. High-level microscopy vision tasks can be organized as follows: image classification (and material property regression), auto-tuning experimental imaging hyperparameters, pixelwise learning (e.g., semantic segmentation), super-resolution imaging, object/entity recognition, localization, and tracking, microstructure representation learning.
Often these tasks generalize across many different imaging modalities, spanning optical microscopy (OM), scanning electron microscopy (SEM) techniques, scanning probe microscopy (SPM, as in scanning tunneling microscopy (STM) or atomic force microscopy (AFM), and transmission electron microscopy (TEM) variants, including scanning transmission electron microscopy (STEM).
The images obtained with these techniques range from capturing local atomic to mesoscale structures (microstructure), the distribution and type of defects, and their dynamics which are critically linked to the functionality and performance of the materials. Over the past few decades, atomic-scale imaging has become widespread and near-routine due to aberration-corrected STEM 209 . The collection of large image datasets is increasingly presenting an analysis bottleneck in the materials characterization pipeline, and the immediate need for automated image analysis becomes important. Non-DL image analysis methods have driven tremendous progress in quantitative microscopy, but often image processing pipelines are brittle and require too much manual identification of image features to be broadly applicable. Thus, DL is currently the most promising solution for high-performance, high-throughput automated analysis of image datasets. For a good overview of applications in microstructure characterization specifically, see 210 .
Image datasets for materials can come from either experiments or simulations. Software libraries mentioned above can be used to generate images such as STM/STEM. Images can also be obtained from the literature. A few common examples for image datasets are shown below in Table 4 . Recently, there has been a rapid development in the field of image learning tasks for materials leading to several useful packages. We list some of them in Table 4 .
Applications in image classification and regression
DL for images can be used to automatically extract information from images or transform images into a more useful state. The benefits of automated image analysis include higher throughput, better consistency of measurements compared to manual analysis, and even the ability to measure signals in images that humans cannot detect. The benefits of altering images include image super-resolution, denoising, inferring 3D structure from 2D images, and more. Examples of the applications of each task are summarized below.
Image classification and regression
Classification and regression are the processes of predicting one or more values associated with an image. In the context of DL the only difference between the two methods is that the outputs of classification are discrete while the outputs of regression models are continuous. The same network architecture may be used for both classification and regression by choosing the appropriate activation function (i.e., linear for regression or Softmax for classification) for the output of the network. Due to its simplicity image classification is one of the most established DL techniques available in the materials science literature. Nonetheless, this technique remains an area of active research.
Modarres et al. applied DL with transfer learning to automatically classify SEM images of different material systems 211 . They demonstrated how a single approach can be used to identify a wide variety of features and material systems such as particles, fibers, Microelectromechanical systems (MEMS) devices, and more. The model achieved 90% accuracy on a test set. Misclassifications resulted from images containing objects from multiple classes, which is an inherent limitation of single-class classification. More advanced techniques such as those described in subsequent sections can be applied to avoid these limitations. Additionally, they developed a system to deploy the trained model at scale to process thousands of images in parallel. This approach is essential for large-scale, high-throughput experiments or industrial applications of classification. ImageNet-based deep transfer learning has also been successfully applied for crack detection in macroscale materials images 212 , 213 , as well as for property prediction on small, noisy, and heterogeneous industrial datasets 214 , 215 .
DL has also been applied to characterize the symmetries of simulated measurements of samples. In ref. 216 , Ziletti et al. obtained a large database of perfect crystal structures, introduced defects into the perfect lattices, and simulated diffraction patterns for each structure. DL models were trained to identify the space group of each diffraction patterns. The model achieved high classification performance, even on crystals with significant numbers of defects, surpassing the performance of conventional algorithms for detecting symmetries from diffraction patterns.
DL has also been applied to classify symmetries in simulated STM measurements of 2D material systems 217 . DFT was used to generate simulated STM images for a variety of material systems. A convolutional neural network was trained to identify which of the five 2D Bravais lattices each material belonged to using the simulated STM image as input. The model achieved an average F1 score of around 0.9 for each lattice type.
DL has also been used to improve the analysis of electron backscatter diffraction (EBSD) data, with Liu et al. 218 presenting one of the first DL-based solution for EBSD indexing capable of taking an EBSD image as input and predicting the three Euler angles representing the orientation that would have led to the given EBSD pattern. However, they considered the three Euler angles to be independent of each other, creating separate CNNs for each angle, although the three angles should be considered together. Jha et al. 219 built upon that work to train a single DL model to predict the three Euler angles in simulated EBSD patterns of polycrystalline Ni while directly minimizing the misorientation angle between the true and predicted orientations. When tested on experimental EBSD patterns, the model achieved 16% lower disorientation error than dictionary-based indexing. Similarly, Kaufman et al. trained a CNN to predict the corresponding space group for a given diffraction pattern 220 . This enables EBSD to be used for phase identification in samples where the existing phases are unknown, providing a faster or more cost-effective method of characterizing than X-ray or neutron diffraction. The results from these studies demonstrate the promise of applying DL to improve the performance and utility of EBSD experiments.
Recently, DL has also been to learn crystal plasticity using images of strain profiles as input 221 , 222 . The work in ref. 221 used domain knowledge integration in the form of two-point auto-correlation to enhance the predictive accuracy, while 222 applied residual learning to learn crystal plasticity at nanoscale. It used strain profiles of materials of varying sample widths ranging from 2 μm down to 62.5 nm obtained from discrete dislocation dynamics to build a deep residual network capable of identifying prior deformation history of the sample as low, medium, or high. Compared to the correlation function-based method (68.24% accuracy), the DL model was found to be significantly more accurate (92.48%) and also capable of predicting stress-strain curves of test samples. This work additionally used saliency maps to try to interpret the developed DL model.
Pixelwise learning
DL can also be applied to generate one or more predictions for every pixel in an image. This can provide more detailed information about the size, position, orientation, and morphology of features of interest in images. Thus, pixelwise learning has been a significant area of focus with many recent studies appearing in materials science literature.
Azimi et al. applied an ensemble of fully convolutional neural networks to segment martensite, tempered martensite, bainite, and pearlite in SEM images of carbon steels. Their model achieved 94% accuracy, demonstrating a significant improvement over previous efforts to automate the segmentation of different phases in SEM images. Decost, Francis, and Holm applied PixelNet to segment microstructural constituents in the UltraHigh Carbon Steel Database 223 , 224 . In contrast to fully convolutional neural networks, which encode and decode visual signals using a series of convolution layers, PixelNet constructs “hypercolumns”, or concatenations of feature representations corresponding to each pixel at different layers in a neural network. The hypercolumns are treated as individual feature vectors, which can then be classified using any typical classification approach, like a multilayer perceptron. This approach achieved phase segmentation precision and recall scores of 86.5% and 86.5%, respectively. Additionally, this approach was used to segment spheroidite particles in the matrix, achieving precision and recall scores of 91.1% and 91.1%, respectively.
Pixelwise DL has also been applied to automatically segment dislocations in Ni superalloys 210 . Dislocations are visually similar to \(\gamma -{\gamma }^{\prime}\) and dislocation in Ni superalloys. With limited training data, a single segmentation model could not distinguish between these features. To overcome this, a second model was trained to generate a coarse mask corresponding to the deformed region in the material. Overlaying this mask with predictions from the first model selects the dislocations, enabling them to be distinguished from \(\gamma -{\gamma }^{\prime}\) interfaces.
Stan, Thompson, and Voorhees applied Pixelwise DL to characterize dendritic growth from serial sectioning and synchrotron computed tomography data 225 . Both of these techniques generate large amounts of data, making manual analysis impractical. Conventional image processing approaches, utilizing thresholding, edge detectors, or other hand-crafted filters, cannot effectively deal with noise, contrast gradients, and other artifacts that are present in the data. Despite having a small training set of labeled images, SegNet automatically segmented these images with much higher performance.
Object/entity recognition, localization, and tracking
Object detection or localization is needed when individual instances of recognized objects in a given image need to be distinguished from each other. In cases where instances do not overlap each other by a significant amount, individual instances can be resolved through post-processing of semantic segmentation outputs. This technique has been applied extensively to detect individual atoms and defects in microstructural images.
Madsen et al. applied pixelwise DL to detect atoms in simulated atomic-resolution TEM images of graphene 226 . A neural network was trained to detect the presence of each atom as well as predict its column height. Pixelwise results are used as seeds for watershed segmentation to achieve instance-level detection. Analysis of the arrangement of the atoms led to the autonomous characterization of defects in the lattice structure of the material. Interestingly, despite being trained only on simulations, the model successfully detected atomic positions in experimental images.
Maksov et al. demonstrated atomistic defect recognition and tracking across sequences of atomic-resolution STEM images of WS 2 227 . The lattice structure and defects existing in the first frame were characterized through a physics-based approach utilizing Fourier transforms. The positions of atoms and defects in the first frame were used to train a segmentation model. Despite only using the first frame for training, the model successfully identified and tracked defects in the subsequent frames for each sequence, even when the lattice underwent significant deformation. Similarly, Yang et al. 228 used U-net architecture (as shown in Fig. 4 ) to detect vacancies and dopants in WSe 2 in STEM images with model accuracy of up to 98%. They classified the possible atomic sites based on experimental observations into five different types: tungsten, vanadium substituting for tungsten, selenium with no vacancy, mono-vacancy of selenium, and di-vacancy of selenium.
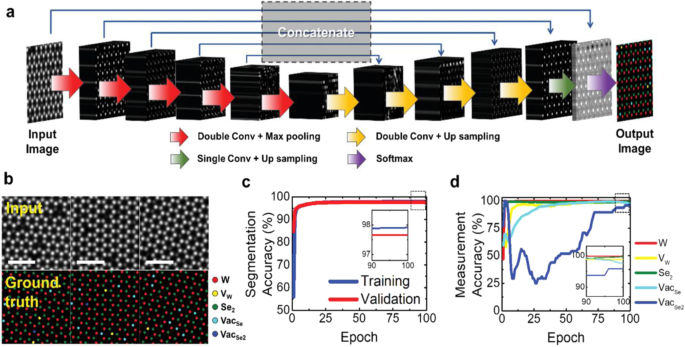
a Deep neural networks U-Net model constructed for quantification analysis of annular dark-field in the scanning transmission electron microscope (ADF-STEM) image of V-WSe 2 . b Examples of training dataset for deep learning of atom segmentation model for five different species. c Pixel-level accuracy of the atom segmentation model as a function of training epoch. d Measurement accuracy of the segmentation model compared with human-based measurements. Scale bars are 1 nm [Reprinted according to the terms of the CC-BY license ref. 228 ].
Roberts et al. developed DefectSegNet to automatically identify defects in transmission and STEM images of steel including dislocations, precipitates, and voids 229 . They provide detailed information on the model’s design, training, and evaluation. They also compare measurements generated from the model to manual measurements performed by several different human experts, demonstrating that the measurements generated by DL are quantitatively more accurate and consistent.
Kusche et al. applied DL to localize defects in panoramic SEM images of dual-phase steel 230 . Manual thresholding was applied to identify dark defects against the brighter matrix. Regions containing defects were classified via two neural networks. The first neural network distinguished between inclusions and ductile damage in the material. The second classified the type of ductile damage (i.e., notching, martensite cracking, etc.) Each defect was also segmented via a watershed algorithm to obtain detailed information on its size, position, and morphology.
Applying DL to localize defects and atomic structures is a popular area in materials science research. Thus, several other recent studies on these applications can be found in the literature 231 , 232 , 233 , 234 .
In the above examples pixelwise DL, or classification models are combined with image analysis to distinguish individual instances of detected objects. However, when several adjacent objects of the same class touch or overlap each other in the image, this approach will falsely detect them to be a single, larger object. In this case, DL models designed for the detection or instance segmentation can be used to resolve overlapping instances. In one such study, Cohn and Holm applied DL for instance-level segmentation of individual particles and satellites in dense powder images 235 . Segmenting each particle allows for computer vision to generate detailed size and morphology information which can be used to supplement experimental powder characterization for additive manufacturing. Additionally, overlaying the powder and satellite masks yielded the first method for quantifying the satellite content of powder samples, which cannot be measured experimentally.
Super-resolution imaging and auto-tuning experimental parameters
The studies listed so far focus on automating the analysis of existing data after it has been collected experimentally. However, DL can also be applied during experiments to improve the quality of the data itself. This can reduce the time for data collection or improve the amount of information captured in each image. Super-resolution and other DL techniques can also be applied in situ to autonomously adjust experimental parameters.
Recording high-resolution electron microscope images often require large dwell times, limiting the throughput of microscopy experiments. Additionally, during imaging, interactions between the electron beam and a microscopy sample can result in undesirable effects, including charging of non-conductive samples and damage to sensitive samples. Thus, there is interest in using DL to artificially increase the resolution of images without introducing these artifacts. One method of interest is applying generative adversarial networks (GANs) for this application.
De Haan et al. recorded SEM images of the same regions of interest in carbon samples containing gold nanoparticles at two resolutions 236 . Low-resolution images recorded were used as inputs to a GAN. The corresponding images with twice the resolution were used as the ground truth. After training the GAN reduced the number of undetected gaps between nanoparticles from 13.9 to 3.7%, indicating that super-resolution was successful. Thus, applying DL led to a four-fold reduction of the interaction time between the electron beam and the sample.
Ede and Beanland collected a dataset of STEM images of different samples 237 . Images were subsampled with spiral and ‘jittered’ grid masks to obtain partial images with resolutions reduced by a factor up to 100. A GAN was trained to reconstruct full images from their corresponding partial images. The results indicated that despite a significant reduction in the sampling area, this approach successfully reconstructed high-resolution images with relatively small errors.
DL has also been applied to automated tip conditioning for SPM experiments. Rashidi and Wolkow trained a model to detect artifacts in SPM measurements resulting from degradation in tip quality 238 . Using an ensemble of convolutional neural networks resulted in 99% accuracy. After detecting that a tip has degraded, the SPM was configured to automatically recondition the tip in situ until the network indicated that the atomic sharpness of the tip has been restored. Monitoring and reconditioning the tip is the most time and labor-intensive part of conducting SPM experiments. Thus, automating this process through DL can increase the throughput and decrease the cost of collecting data through SPM.
In addition to materials characterization, DL can be applied to autonomously adjust parameters during manufacturing. Scime et al. mounted a camera to multiple 3D printers 239 . Images of the build plate were recorded throughout the printing process. A dynamic segmentation convolutional neural network was trained to recognize defects such as recoater streaking, incomplete spreading, spatter, porosity, and others. The trained model achieved high performance and was transferable to multiple printers from three different methods of additive manufacturing. This work is the first step to enabling smart additive manufacturing machines that can correct defects and adjust parameters during printing.
There is also growing interest in establishing instruments and laboratories for autonomous experimentation. Eppel et al. trained multiple models to detect chemicals, materials, and transparent vessels in a chemistry lab setting 240 . This study provides a rigorous analysis of several different approaches for scene understanding. Models were trained to characterize laboratory scenes with different methods including semantic segmentation and instance segmentation, both with and without overlapping instances. The models successfully detected individual vessels and materials in a variety of settings. Finer-grained understanding of the contents of vessels, such as segmentation of individual phases in multi-phase systems, was limited, outlining the path for future work in this area. The results represent an important step towards realizing automated experimentation for laboratory-scale experiments.
Microstructure representation learning
Materials microstructure is often represented in the form of multi-phase high-dimensional 2D/3D images and thus can readily leverage image-based DL methods to learn robust, low-dimensional microstructure representations, which can subsequently be used for building predictive and generative models to learn forward and inverse structure-property linkages, which are typically studied across different length scales (multi-scale modeling). In this context, homogenization and localization refer to the transfer of information from lower length scales to higher length scales and vice-versa. DL using customized CNNs has been used both for homogenization, i.e., predicting the macroscale property of material given its microstructure information 221 , 241 , 242 , as well as for localization, i.e., predicting the strain distribution across a given microstructure for a loading condition 243 .
Transfer learning has also been widely used for analyzing materials microstructure images; methods for improving the use of transfer learning to materials science applications remain an area of active research. Goetz et al. investigated the use of unsupervised domain adaptation as an alternative to simply fine-tuning a pre-trained model 244 . In this technique a model is first trained on a labeled dataset in the source domain. Next, a discriminator model is used to train the model to generate domain-agnostic features. Compared to simple fine-tuning, unsupervised domain adaptation improved the performance of classification and segmentation neural networks on materials science datasets. However, it was determined that the highest performance was achieved when the source domain was more visually similar to the target (for example, using a different set of microstructural images instead of ImageNet.) This highlights the utility of establishing large, publicly available datasets of annotated images in materials science.
Kitaraha and Holm used the output of an intermediate layer of a pre-trained convolutional neural network as a feature representation for images of steel surface defects and Inconnel fracture surfaces 245 . Images were classified by defect type or fracture surface orientation using unsupervised DL. Even though no labeled data was used to train the neural network or the unsupervised classifier, the model found natural decision boundaries that achieved a classification performance of 98% and 88% for the defect classes and fracture surface orientations, respectively. Visualization of the representations through principal component analysis (PCA) and t-distributed stochastic neighborhood embedding (t-SNE) provided qualitative insights into the representations. Although the detailed physical interpretation of the representations is still a distant goal, this study provides tools for investigating patterns in visual signals contained in image-based datasets in materials science.
Larmuseau et al. investigated the use of triplet networks to obtain consistent representations for visually similar images of materials 246 . Triplet networks are trained with three images at a time. The first image, the reference, is classified by the network. The second image, called the positive, is another image with the same class label. The last image, called the negative, is an image from a separate class. During training the loss function includes errors in predicting the class of the reference image, the difference in representations of the reference and positive images, and the similarity in representations of the reference and negative images. This process allows the network to learn consistent representations for images in the same class while distinguishing images from different classes. The triple network outperformed an ordinary convolutional neural network trained for image classification on the same dataset.
In addition to investigating representations used to analyze existing images, DL can generate synthetic images of materials systems. Generative Adversarial Networks (GANs) are currently the predominant method for synthetic microstructure generation. GANs consist of a generator, which creates a synthetic microstructure image, and a discriminator, which attempts to predict if a given input image is real or synthetic. With careful application, GANs can be a powerful tool for microstructure representation learning and design.
Yang and Li et al. 247 , 248 developed a GAN-based model for learning a low-dimensional embedding of microstructures, which could then be easily sampled and used with the generator of the GAN model to generate realistic, statistically similar microstructure images, thus enabling microstructural materials design. The model was able to capture complex, nonlinear microstructure characteristics and learn the mapping between the latent design variables and microstructures. In order to close the loop, the method was combined with a Bayesian optimization approach to design microstructures with optimal optical absorption performance. The discovered microstructures were found to have up to 17% better property than randomly sampled microstructures. The unique architecture of their GAN model also facilitated generator scalability to generate arbitrary-sized microstructure images and discriminator transferability to build structure-property prediction models. Yang et al. 249 recently combined GANs with MDNs (mixture density networks) to enable inverse modeling in microstructural materials design, i.e., generate the microstructure for a given desired property.
Hsu et al. constructed a GAN to generate 3D synthetic solid oxide fuel cell microstructures 250 . These microstructures were compared to other synthetic microstructures generated by DREAM.3D as well as experimentally observed microstructures measured via sectioning and imaging with PFIB-SEM. Synthetic microstructures generated from the GAN were observed to qualitatively show better agreement to the experimental microstructures than the DREAM.3D microstructures, as evidenced by the more realistic phase connectivity and lower amount of agglomeration of solid phases. Additionally, a statistical analysis of various features such as volume fraction, particle size, and several other quantities demonstrated that the GAN microstructures were quantitatively more similar to the real microstructures than the DREAM.3D microstructures.
In a similar study, Chun et al. generated synthetic microstructures of high energy materials using a GAN 251 . Once again, a synthetic microstructure generated via GAN showed better qualitative visual similarity to an experimentally observed microstructure compared to a synthetic microstructure generated via a transfer learning approach, with sharper phase boundaries and fewer computational artifacts. Additionally, a statistical analysis of the void size, aspect ratio, and orientation distributions indicated that the GAN produced microstructures that were quantitatively more similar to real materials.
Applications of DL to microstructure representation learning can help researchers improve the performance of predictive models used for the applications listed above. Additionally, using generative models can generate more realistic simulated microstructures. This can help researchers develop more accurate models for predicting material properties and performance without needing to synthesize and process these materials, significantly increasing the throughput of materials selection and screening experiments.
Mesoscale modeling applications
In addition to image-based characterization, deep learning methods are increasingly used in mesoscale modeling. Dai et al. 252 trained a GNN successfully trained to predict magnetostriction in a wide range of synthetic polycrystalline systems with around 10% prediction error. The microstructure is represented by a graph where each node corresponds to a single grain, and the edges between nodes indicate an interface between neighboring grains. Five node features (3 Euler angles, volume, and the number of neighbors) were associated with each grain. The GNN outperformed other machine learning approaches for property prediction of polycrystalline materials by accounting for interactions between neighboring grains.
Similarly, Cohn and Holm present preliminary work applying GNNs to predict the occurrence of abnormal grain growth (AGG) in Monte Carlo simulations of microstructure evolution 253 . AGG appears to be stochastic, making it notoriously difficult to predict, control, and even observe experimentally in some materials. AGG has been reproduced in Monte Carlo simulations of material systems, but a model that can predict which initial microstructures will undergo AGG has not been established before. A dataset of Monte Carlo simulations was created using SPPARKS 254 , 255 . A microstructure GNN was trained to predict AGG in individual simulations, with 75% classification accuracy. In comparison, an image-based only achieved 60% accuracy. The GNN also provided physical insight to understanding AGG and indicated that only 2 neighborhood shells are needed to achieve the maximum performance achieved in the study. These early results motivate additional work on applying GNNs to predict the occurrence in both simulated and real materials during processing.
Natural language processing
Most of the existing knowledge in the materials domain is currently unavailable as structured information and only exists as unstructured text, tables, or images in various publications. There exists a great opportunity to use natural language processing (NLP) techniques to convert text to structured data or to directly learn and make inferences from the text information. However, as a relatively new field within materials science, many challenges remain unsolved in this domain, such as resolving dependencies between words and phrases across multiple sentences and paragraphs.
Datasets for NLP
Datasets relevant to natural language processing include peer-reviewed journal articles, articles published on preprint servers such as arXiv or ChemRxiv, patents, and online material such as Wikipedia. Unfortunately, accessing or parsing most such datasets remains difficult. Peer-reviewed journal articles are typically subject to copyright restrictions and thus difficult to obtain, especially in the large numbers required for machine learning. Many publishers now offer text and data mining (TDM) agreements that can be signed online, allowing at least a limited, restricted amount of work to be performed. However, gaining access to the full text of many publications still typically requires strict and dedicated agreements with each publisher. The major advantage of working with publishers is that they have often already converted the articles from a document format such as PDF into an easy-to-parse format such as HyperText Markup Language (HTML). In contrast, articles on preprint servers and patents are typically available with fewer restrictions, but are commonly available only as PDF files. It remains difficult to properly parse text from PDF files in a reliable manner, even when the text is embedded in the PDF. Therefore, new tools that can easily and automatically convert such content into well-structured HTML format with few residual errors would likely have a major impact on the field. Finally, online sources of information such as Wikipedia can serve as another type of data source. However, such online sources are often more difficult to verify in terms of accuracy and also do not contain as much domain-specific information as the research literature.
Software libraries for NLP
Applying NLP to a raw dataset involves multiple steps. These steps include retrieving the data, various forms of “pre-processing” (sentence and word tokenization, word stemming and lemmatization, featurization such as word vectors or part of speech tagging), and finally machine learning for information extraction (e.g., named entity recognition, entity-relationship modeling, question and answer, or others). Multiple software libraries exist to aid in materials NLP, as described in Table 5 . We note that although many of these steps can in theory be performed by general-purpose NLP libraries such as NLTK 256 , SpaCy 257 , or AllenNLP 258 , the specialized nature of chemistry and materials science text (including the presence of complex chemical formulas) often leads to errors. For example, researchers have developed specialized codes to perform preprocessing that better detect chemical formulas (and not split them into separate tokens or apply stemming/lemmatization to them) and scientific phrases and notation such as oxidation states or symbols for physical units.
Similarly, chemistry-specific codes for extracting entities are better at extracting the names of chemical elements (e.g., recognizing that “He” likely represents helium and not a male pronoun) and abbreviations for chemical formulas. Finally, word embeddings that convert words such as “manganese” into numerical vectors for further data mining are more informative when trained specifically on materials science text versus more generic texts, even when the latter datasets are larger 259 . Thus, domain-specific tools for NLP are required in nearly all aspects of the pipeline. The main exception is that the architecture of the specific neural network models used for information extraction (e.g., LSTM, BERT, or architectures used to generate word embeddings such as word2vec or GloVe) are typically not modified specifically for the materials domain. Thus, much of the materials and chemistry-centric work currently regards data retrieval and appropriate preprocessing. A longer discussion of this topic, with specific examples, can be found in refs. 260 , 261 .
NLP methods for materials have been applied for information extraction and search (particularly as applied to synthesis prediction) as well as materials discovery. As the domain is rapidly growing, we suggest dedicated reviews on this topic by Olivetti et al. 261 and Kononova et al. 260 for more information.
One of the major uses of NLP methods is to extract datasets from the text in published studies. Conventionally, such datasets required manual entry of datasets by researchers combing the literature, a laborious and time-consuming process. Recently, software tools such as ChemDataExtractor 262 and other methods 263 based on more conventional machine learning and rule-based approaches have enabled automated or semi-automated extraction of datasets such as Curie and Néel magnetic phase transition temperatures 264 , battery properties 265 , UV-vis spectra 266 , and surface and pore characteristics of metal-organic frameworks 267 . In the past few years, DL approaches such as LSTMs and transformer-based models have been employed to extract various categories of information 268 , and in particular materials synthesis information 269 , 270 , 271 from text sources. Such data have been used to predict synthesis maps for titania nanotubes 272 , various binary and ternary oxides 273 , and perovskites 274 .
Databases based on natural language processing have also been used to train machine learning models to identify materials with useful functional properties, such as the recent discovery of the large magnetocaloric properties of HoBe 2 275 . Similarly, Cooper et al. 276 demonstrated a “design to device approach” for designing dye-sensitized solar cells that are co-sensitized with two dyes 276 . This study used automated text mining to compile a list of candidate dyes for the application along with measured properties such as maximum absorption wavelengths and extinction coefficients. The resulting list of 9431 dyes extracted from the literature was downselected to 309 candidates using various criteria such as molecular structure and ability to absorb in the solar spectrum. These candidates were evaluated for suitable combinations for co-sensitization, yielding 33 dyes that were further downselected using density functional theory calculations and experimental constraints. The resulting 5 dyes were evaluated experimentally, both individually and in combinations, resulting in a combination of dyes that not only outperformed any of the individual dyes but demonstrated performance comparable to existing standard material. This study demonstrates the possibility of using literature-based extraction to identify materials candidates for new applications from the vast body of published work, which may have never tested those materials for the desired application.
It is even possible that natural language processing can directly make materials predictions without intermediary models. In a study reported by Tshitoyan et al. 259 (as shown in Fig. 5 ), word embeddings (i.e., numerical vectors representing distinct words) trained on materials science literature could directly predict materials applications through a simple dot product between the trained embedding for a composition word (such as PbTe) and an application words (such as thermoelectrics). The researchers demonstrated that such an approach, if applied in the past using historical data, may have subsequently predicted many recently reported thermoelectric materials; they also presented a list of potentially interesting thermoelectric compositions using the known literature at the time. Since then, several of these predictions have been tested either computationally 277 , 278 , 279 , 280 , 281 , 282 or experimentally 283 as potential thermoelectrics. Such approaches have recently been applied to search for understudied areas of metallocene catalysis 284 , although challenges still remain in such direct approaches to materials prediction.
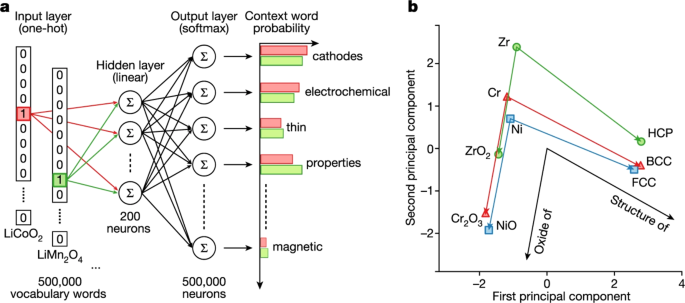
a Network for training word embeddings for natural language processing application. A one-hot encoded vector at left represents each distinct word in the corpus; the role of a hidden layer is to predict the probability of neighboring words in the corpus. This network structure trains a relatively small hidden layer of 100–200 neurons to contain information on the context of words in the entire corpus, with the result that similar words end up with similar hidden layer weights (word embeddings). Such word embeddings can transform wordsin text form into numerical vectors that may be useful for a variety of applications. b projection of word embeddings for various materials science words, as trained on a corpus scientific abstracts, into two dimensions using principle components analysis. Without any explicit training, the word embeddings naturally preserve relationships between chemical formulas, their common oxides, and their ground state structures. [Reprinted according to the terms of the CC-BY license ref. 259 ].
Uncertainty quantification
Uncertainty quantification (UQ) is an essential step in evaluating the robustness of DL. Specifically, DL models have been criticized for lack of robustness, interpretability, and reliability and the addition of carefully quantified uncertainties would go a long way towards addressing such shortcomings. While most of the focus in the DL field currently goes into developing new algorithms or training networks to high accuracy, there is increasing attention to UQ, as exemplified by the detailed review of Abdar et al. 285 . However, determining the uncertainty associated with DL predictions is still challenging and far from a completely solved problem.
The main drawback to estimating UQ when performing DL is the fact that most of the currently available UQ implementations do not work for arbitrary, off-the-shelf models, without retraining or redesigning. Bayesian NNs are the exception; however, they require significant modifications to the training procedure, are computationally expensive compared to non-Bayesian NNs, and become increasingly inefficient the larger the datasize gets. A considerable fraction of the current research in DL UQ focuses exactly on such an issue: how to evaluate uncertainty without requiring computationally expensive retraining or DL code modifications. An example of such an effort is the work of Mi et al. 286 , where three scalable methods are explored, to evaluate the variance of output from trained NN, without requiring any amount of retraining. Another example is Teye, Azizpour, and Smith’s exploration of the use of batch normalization as a way to approximate inference in Bayesian models 287 .
Before reviewing the most common methods used to evaluate uncertainty in DL, let us briefly point out key reasons to add UQ to DL modeling. Reaching high accuracy when training DL models implicitly assume the availability of a sufficiently large and diverse training dataset. Unfortunately, this rarely occurs in material discovery applications 288 . ML/DL models are prone to perform poorly on extrapolation 289 . It is also extremely difficult for ML/DL models to recognize ambiguous samples 290 . In general, determining the amount of data necessary to train a DL to achieve the required accuracy is a challenging problem. Careful evaluation of the uncertainty associated with DL predictions would not only increase reliability in predicted results but would also provide guidance on estimating the needed training dataset size as well as suggesting what new data should be added to reach the target accuracy (uncertainty-guided decision). Zhang, Kailkhura, and Han’s work emphasizes how including a UQ-motivated reject option into the DL model substantially improves the performance of the remaining material data 288 . Such a reject option is associated with the detection of out-of-distribution samples, which is only possible through UQ analysis of the predicted results.
Two different uncertainty types are associated with each ML prediction: epistemic uncertainty and aleatory uncertainty. Epistemic uncertainty is related to insufficient training data in part of the input domain. As mentioned above, while DL is very effective at interpolation tasks, they can have more difficulty in extrapolation. Therefore, it is vital to quantify the lack of accuracy due to localized, insufficient training data. The aleatory uncertainty, instead, is related to parameters not included in the model. It relates to the possibility of training on data that our DL perceives as very similar but that are associated with different outputs because of missing features in the model. Ideally, we would like UQ methodologies to distinguish and quantify both types of uncertainties separately.
The most common approaches to evaluate uncertainty using DL are Dropout methods, Deep Ensemble methods, Quantile regression, and Gaussian Processes. Dropout methods are commonly used to avoid overfitting. In this type of approach, network nodes are disabled randomly during training, resulting in the evaluation of a different subset of the network at each training step. When a similar randomization procedure is also applied to the prediction procedure, the methodology becomes Monte-Carlo dropout 291 . Repeating such randomization multiple times produces a distribution over the outputs, from which mean and variance are determined for each prediction. Another example of using a dropout approach to approximate Bayesian inference in deep Gaussian processes is the work of Gal and Ghahramani 292 .
Deep ensemble methodologies 293 , 294 , 295 , 296 combine deep learning modelling with ensemble learning. Ensemble methods utilize multiple models and different random initializations to improve predictability. Because of the multiple predictions, statistical distributions of the outputs are generated. Combining such results into a Gaussian distribution, confidence intervals are obtained through variance evaluation. Such a multi-model strategy allows the evaluation of aleatory uncertainty when sufficient training data are provided. For areas without sufficient data, the predicted mean and variance will not be accurate, but the expectation is that a very large variance should be estimated, clearly indicating non-trustable predictions. Monte-Carlo Dropout and Deep Ensembles approaches can be combined to further improve confidence in the predicted outputs.
Quantile regression can be utilized with DL 297 . In this approach, the loss function is used in a way that allows to predict for the chosen quantile a (between 0 and 1). A choice of a = 0.5 corresponds to evaluating the Mean Absolute Error (MAE) and predicting the median of the distribution. Predicting for two more quantile values (amin and amax) determines confidence intervals of width amax − amin. For instance, predicting for amin = 0.1 and amax = 0.8 produces confidence intervals covering 70% of the population. The largest drawback of using quantile to estimate prediction intervals is the need to run the model three times, one for each quantile needed. However, a recent implementation in TensorFlow allows to simultaneously obtain multiple quantiles in one run.
Lastly, Gaussian Processes (GP) can be used within a DL approach as well and have the side benefit of providing UQ information at no extra cost. Gaussian processes are a family of infinite-dimensional multivariate Gaussian distributions completely specified by a mean function and a flexible kernel function (prior distribution). By optimizing such functions to fit the training data, the posterior distribution is determined, which is later used to predict outputs for inputs not included in the training set. Because the prior is a Gaussian process, the posterior distribution is Gaussian as well 298 , thus providing mean and variance information for each predicted data. However, in practice standard kernels under-perform 299 . In 2016, Wilson et al. 300 suggested processing inputs through a neural network prior to a Gaussian process model. This procedure could extract high-level patterns and features, but required careful design and optimization. In general, Deep Gaussian processes improve the performance of Gaussian processes by mapping the inputs through multiple Gaussian process ‘layers’. Several groups have followed this avenue and further perfected such an approach (ref. 299 and references within). A common drawback of Bayesian methods is a prohibitive computational cost if dealing with large datasets 292 .
Limitations and challenges
Although DL methods have various fascinating opportunities for materials design, they have several limitations and there is much room to improve. Reliability and quality assessment of datasets used in DL tasks are challenging because there is either a lack of ground truth data, or there are not enough metrics for global comparison, or datasets using similar or identical set-ups may not be reproducible 301 . This poses an important challenge in relying upon DL-based prediction.
Material representations based on chemical formula alone by definition do not consider structure, which on the one hand makes them more amenable to work for new compounds for which structure information may not be available, but on the other hand, makes it impossible for them to capture phenomena such as phase transitions. Properties of materials depend sensitively on structure to the extent that their properties can be quite opposite depending on the atomic arrangement, like a diamond (hard, wide-band-gap insulator) and graphite (soft, semi-metal). It is thus not a surprise that chemical formula-based methods may not be adequate in some cases 159 .
Atomistic graph-based predictions, although considered a full atomistic description, are tested on bulk materials only and not for defective systems or for multi-dimensional phases of space exploration such as using genetic algorithms. In general, this underscores that the input features must be predictive for the output labels and not be missing some key information. Although atomistic graph neural network models such as atomistic line graph neural network (ALIGNN) have achieved remarkable accuracy compared to previous atomistic based models, the model errors still need to be further brought down to reach something resembling deep learning ‘chemical-accuracies.’
In terms of images and spectra, the experimental data are too noisy most of the time and require much manipulation before applying DL. In contrast, theory-based simulated data represent an alternate path forward but may not capture realistic scenarios such as the presence of structured noise 217 .
Uncertainty quantification for deep learning for materials science is important, yet only a few works have been published in this field. To alleviate the black-box 38 nature of the DL methods, a package such as GNNExplainer 302 has been tried in the context of the material. Such attempts at greater interpretability will be important moving forward to gain the trust of the materials community.
While training-validation-test split strategies were primarily designed in DL for image classification tasks with a certain number of classes, the same for regression models in materials science may not be the best approach. This is because it is possible that during the training the model is seeing a material very similar to the test set material and in reality it is difficult to generalize the model. Best practices need to be developed for data split, normalization, and augmentation to avoid such issues 289 .
Finally, we note an important technological challenge is to make a closed-loop autonomous materials design and synthesis process 303 , 304 that can include both machine learning and experimental components in a self-driving laboratory 305 . For an overview of early proof of principle attempts see 306 . For example, in an autonomous synthesis experiment the oxidation state of copper (and therefore the oxide phase) was varied in a sample of copper oxide by automatically flowing more oxidizing or more reducing gas over the sample and monitoring the charge state of the copper using XANES. An algorithmic decision policy was then used to automatically change the gas composition for a subsequent experiment based on the prior experiments, with no human in the loop, in such a way as to autonomously move towards a target copper oxidation state 307 . This simple proof of principle experiment provides just a glimpse of what is possible moving forward.
Data availability
The data from new figures are available on reasonable request from the corresponding author. Data from other publishers are not available from the corresponding author of this work but may be available by reaching the corresponding author of the cited work.
Code availability
Software packages mentioned in the article (whichever made available by the authors) can be found at https://github.com/deepmaterials/dlmatreview . Software for other packages can be obtained by reaching the corresponding author of the cited work.
Callister, W. D. et al. Materials Science and Engineering: An Introduction (Wiley, 2021).
Saito, T. Computational Materials Design, Vol. 34 (Springer Science & Business Media, 2013).
Choudhary, K. et al. The joint automated repository for various integrated simulations (jarvis) for data-driven materials design. npj Comput. Mater. 6 , 1–13 (2020).
Article Google Scholar
Kirklin, S. et al. The open quantum materials database (oqmd): assessing the accuracy of dft formation energies. npj Comput. Mater. 1 , 1–15 (2015).
Jain, A. et al. Commentary: The materials project: A materials genome approach to accelerating materials innovation. APL Mater. 1 , 011002 (2013).
Curtarolo, S. et al. Aflow: An automatic framework for high-throughput materials discovery. Comput. Mater. Sci. 58 , 218–226 (2012).
Article CAS Google Scholar
Ramakrishnan, R., Dral, P. O., Rupp, M. & Von Lilienfeld, O. A. Quantum chemistry structures and properties of 134 kilo molecules. Sci. Data 1 , 1–7 (2014).
Draxl, C. & Scheffler, M. Nomad: The fair concept for big data-driven materials science. MRS Bull. 43 , 676–682 (2018).
Wang, R., Fang, X., Lu, Y., Yang, C.-Y. & Wang, S. The pdbbind database: methodologies and updates. J. Med. Chem. 48 , 4111–4119 (2005).
Zakutayev, A. et al. An open experimental database for exploring inorganic materials. Sci. Data 5 , 1–12 (2018).
de Pablo, J. J. et al. New frontiers for the materials genome initiative. npj Comput. Mater. 5 , 1–23 (2019).
Wilkinson, M. D. et al. The fair guiding principles for sci. data management and stewardship. Sci. Data 3 , 1–9 (2016).
Friedman, J. et al. The Elements of Statistical Learning, Vol. 1 (Springer series in statistics New York, 2001).
Agrawal, A. & Choudhary, A. Perspective: Materials informatics and big data: Realization of the “fourth paradigm” of science in materials science. APL Mater. 4 , 053208 (2016).
Vasudevan, R. K. et al. Materials science in the artificial intelligence age: high-throughput library generation, machine learning, and a pathway from correlations to the underpinning physics. MRS Commun. 9 , 821–838 (2019).
Schmidt, J., Marques, M. R., Botti, S. & Marques, M. A. Recent advances and applications of machine learning in solid-state materials science. npj Comput. Mater. 5 , 1–36 (2019).
Butler, K. T., Davies, D. W., Cartwright, H., Isayev, O. & Walsh, A. Machine learning for molecular and materials science. Nature 559 , 547–555 (2018).
Xu, Y. et al. Deep dive into machine learning models for protein engineering. J. Chem. Inf. Model. 60 , 2773–2790 (2020).
Schleder, G. R., Padilha, A. C., Acosta, C. M., Costa, M. & Fazzio, A. From dft to machine learning: recent approaches to materials science–a review. J. Phys. Mater. 2 , 032001 (2019).
Agrawal, A. & Choudhary, A. Deep materials informatics: applications of deep learning in materials science. MRS Commun. 9 , 779–792 (2019).
Goodfellow, I., Bengio, Y. & Courville, A. Deep Learning (MIT Press, 2016).
LeCun, Y., Bengio, Y. & Hinton, G. Deep learning. Nature 521 , 436–444 (2015).
McCulloch, W. S. & Pitts, W. A logical calculus of the ideas immanent in nervous activity. Bull. Math. Biophys. 5 , 115–133 (1943).
Rosenblatt, F. The perceptron: A probabilistic model for information storage and organization in the brain. Psychol. Rev. 65 , 386–408 (1958).
Gibney, E. Google ai algorithm masters ancient game of go. Nat. News 529 , 445 (2016).
Ramos, S., Gehrig, S., Pinggera, P., Franke, U. & Rother, C. Detecting unexpected obstacles for self-driving cars: Fusing deep learning and geometric modeling. in 2017 IEEE Intelligent Vehicles Symposium (IV) , 1025–1032 (IEEE, 2017).
Buduma, N. & Locascio, N. Fundamentals of deep learning: Designing next-generation machine intelligence algorithms (O’Reilly Media, Inc., O’Reilly, 2017).
Kearnes, S., McCloskey, K., Berndl, M., Pande, V. & Riley, P. Molecular graph convolutions: moving beyond fingerprints. J. Computer Aided Mol. Des. 30 , 595–608 (2016).
Albrecht, T., Slabaugh, G., Alonso, E. & Al-Arif, S. M. R. Deep learning for single-molecule science. Nanotechnology 28 , 423001 (2017).
Ge, M., Su, F., Zhao, Z. & Su, D. Deep learning analysis on microscopic imaging in materials science. Mater. Today Nano 11 , 100087 (2020).
Agrawal, A., Gopalakrishnan, K. & Choudhary, A. In Handbook on Big Data and Machine Learning in the Physical Sciences: Volume 1. Big Data Methods in Experimental Materials Discovery World Scientific Series on Emerging Technologies, 205–230 (“World Scientific, 2020).
Erdmann, M., Glombitza, J., Kasieczka, G. & Klemradt, U. Deep Learning for Physics Research (World Scientific, 2021).
Chen, C., Ye, W., Zuo, Y., Zheng, C. & Ong, S. P. Graph networks as a universal machine learning framework for molecules and crystals. Chem. Mater. 31 , 3564–3572 (2019).
Jha, D. et al. Enhancing materials property prediction by leveraging computational and experimental data using deep transfer learning. Nat. Commun . 10 , 1–12 (2019).
Cubuk, E. D., Sendek, A. D. & Reed, E. J. Screening billions of candidates for solid lithium-ion conductors: a transfer learning approach for small data. J. Chem. Phys. 150 , 214701 (2019).
Chen, C., Zuo, Y., Ye, W., Li, X. & Ong, S. P. Learning properties of ordered and disordered materials from multi-fidelity data. Nat. Comput. Sci. 1 , 46–53 (2021).
Artrith, N. et al. Best practices in machine learning for chemistry. Nat. Chem. 13 , 505–508 (2021).
Holm, E. A. In defense of the black box. Science 364 , 26–27 (2019).
Mueller, T., Kusne, A. G. & Ramprasad, R. Machine learning in materials science: Recent progress and emerging applications. Rev. Comput. Chem. 29 , 186–273 (2016).
CAS Google Scholar
Wei, J. et al. Machine learning in materials science. InfoMat 1 , 338–358 (2019).
Liu, Y. et al. Machine learning in materials genome initiative: a review. J. Mater. Sci. Technol. 57 , 113–122 (2020).
Wang, A. Y.-T. et al. Machine learning for materials scientists: an introductory guide toward best practices. Chem. Mater. 32 , 4954–4965 (2020).
Morgan, D. & Jacobs, R. Opportunities and challenges for machine learning in materials science. Annu. Rev. Mater. Res. 50 , 71–103 (2020).
Himanen, L., Geurts, A., Foster, A. S. & Rinke, P. Data-driven materials science: status, challenges, and perspectives. Adv. Sci. 6 , 1900808 (2019).
Rajan, K. Informatics for materials science and engineering: data-driven discovery for accelerated experimentation and application (Butterworth-Heinemann, 2013).
Montáns, F. J., Chinesta, F., Gómez-Bombarelli, R. & Kutz, J. N. Data-driven modeling and learning in science and engineering. Comptes Rendus Mécanique 347 , 845–855 (2019).
Aykol, M. et al. The materials research platform: defining the requirements from user stories. Matter 1 , 1433–1438 (2019).
Stanev, V., Choudhary, K., Kusne, A. G., Paglione, J. & Takeuchi, I. Artificial intelligence for search and discovery of quantum materials. Commun. Mater. 2 , 1–11 (2021).
Chen, C. et al. A critical review of machine learning of energy materials. Adv. Energy Mater. 10 , 1903242 (2020).
Cybenko, G. Approximation by superpositions of a sigmoidal function. Math. Control Signals Syst. 2 , 303–314 (1989).
Kidger, P. & Lyons, T. Universal approximation with deep narrow networks . in Conference on learning theory , 2306–2327 (PMLR, 2020).
Lin, H. W., Tegmark, M. & Rolnick, D. Why does deep and cheap learning work so well? J. Stat. Phys. 168 , 1223–1247 (2017).
Minsky, M. & Papert, S. A. Perceptrons: An introduction to computational geometry (MIT press, 2017).
Paszke, A. et al. Pytorch: An imperative style, high-performance deep learning library. Adv. Neural Inf. Process. Syst. 32 , 8026–8037 (2019).
Google Scholar
Abadi et al., TensorFlow: A system for large-scale machine learning. arXiv:1605.08695, Preprint at https://arxiv.org/abs/1605.08695 (2006).
Chen, T. et al. Mxnet: A flexible and efficient machine learning library for heterogeneous distributed systems. arXiv . https://arxiv.org/abs/1512.01274 (2015).
Nwankpa, C., Ijomah, W., Gachagan, A. & Marshall, S. Activation functions: comparison of trends in practice and research for deep learning. arXiv . https://arxiv.org/abs/1811.03378 (2018).
Baydin, A. G., Pearlmutter, B. A., Radul, A. A. & Siskind, J. M. Automatic differentiation in machine learning: a survey. J. Machine Learn. Res. 18 , 1–43 (2018).
Hinton, G. E., Srivastava, N., Krizhevsky, A., Sutskever, I. & Salakhutdinov, R. R. Improving neural networks by preventing co-adaptation of feature detectors. arXiv. https://arxiv.org/abs/1207.0580 (2012).
Breiman, L. Bagging predictors. Machine Learn. 24 , 123–140 (1996).
LeCun, Y. et al. The Handbook of Brain Theory and Neural Networks vol. 3361 (MIT press Cambridge, MA, USA 1995).
Wilson, R. J. Introduction to Graph Theory (Pearson Education India, 1979).
West, D. B. et al. Introduction to Graph Theory Vol. 2 (Prentice hall Upper Saddle River, 2001).
Wang, M. et al. Deep graph library: A graph-centric, highly-performant package for graph neural networks. arXiv . https://arxiv.org/abs/1909.01315 (2019).
Choudhary, K. & DeCost, B. Atomistic line graph neural network for improved materials property predictions. npj Comput. Mater. 7 , 1–8 (2021).
Li, M. et al. Dgl-lifesci: An open-source toolkit for deep learning on graphs in life science. arXiv . https://arxiv.org/abs/2106.14232 (2021).
Xie, T. & Grossman, J. C. Crystal graph convolutional neural networks for an accurate and interpretable prediction of material properties. Phys. Rev. Lett. 120 , 145301 (2018).
Klicpera, J., Groß, J. & Günnemann, S. Directional message passing for molecular graphs. arXiv . https://arxiv.org/abs/2003.03123 (2020).
Schutt, K. et al. Schnetpack: A deep learning toolbox for atomistic systems. J. Chem. Theory Comput. 15 , 448–455 (2018).
Kipf, T. N. & Welling, M. Semi-supervised classification with graph convolutional networks. arXiv . https://arxiv.org/abs/1609.02907 (2016).
Veličković, P. et al. Graph attention networks. arXiv . https://arxiv.org/abs/1710.10903 (2017).
Schlichtkrull, M. et al. Modeling relational data with graph convolutional networks. arXiv. https://arxiv.org/abs/1703.06103 (2017).
Song, L., Zhang, Y., Wang, Z. & Gildea, D. A graph-to-sequence model for AMR-to-text generation . In Proceedings of the 56th Annual Meeting of the Association for Computational Linguistics (Volume 1: Long Papers) , 1616–1626 (Association for Computational Linguistics, 2018).
Xu, K., Hu, W., Leskovec, J. & Jegelka, S. How powerful are graph neural networks? arXiv . https://arxiv.org/abs/1810.00826 (2018).
Chen, Z., Li, X. & Bruna, J. Supervised community detection with line graph neural networks. arXiv . https://arxiv.org/abs/1705.08415 (2017).
Jing, Y., Bian, Y., Hu, Z., Wang, L. & Xie, X.-Q. S. Deep learning for drug design: an artificial intelligence paradigm for drug discovery in the big data era. AAPS J. 20 , 1–10 (2018).
Devlin, J., Chang, M.-W., Lee, K. & Toutanova, K. Bert: Pre-training of deep bidirectional transformers for language understanding. arXiv. https://arxiv.org/abs/1810.04805 (2018).
De Cao, N. & Kipf, T. Molgan: An implicit generative model for small molecular graphs. arXiv . https://arxiv.org/abs/1805.11973 (2018).
Pereira, T., Abbasi, M., Ribeiro, B. & Arrais, J. P. Diversity oriented deep reinforcement learning for targeted molecule generation. J. Cheminformatics 13 , 1–17 (2021).
Baker, N. et al. Workshop report on basic research needs for scientific machine learning: core technologies for artificial intelligence. Tech. Rep . https://doi.org/10.2172/1478744 . (2019).
Chan, H. et al. Rapid 3d nanoscale coherent imaging via physics-aware deep learning. Appl. Phys. Rev. 8 , 021407 (2021).
Pun, G. P., Batra, R., Ramprasad, R. & Mishin, Y. Physically informed artificial neural networks for atomistic modeling of materials. Nat. Commun. 10 , 1–10 (2019).
Onken, D. et al. A neural network approach for high-dimensional optimal control. arXiv. https://arxiv.org/abs/2104.03270 (2021).
Zunger, A. Inverse design in search of materials with target functionalities. Nat. Rev. Chem. 2 , 1–16 (2018).
Chen, L., Zhang, W., Nie, Z., Li, S. & Pan, F. Generative models for inverse design of inorganic solid materials. J. Mater. Inform. 1 , 4 (2021).
Cranmer, M. et al. Discovering symbolic models from deep learning with inductive biases. arXiv . https://arxiv.org/abs/2006.11287 (2020).
Rupp, M., Tkatchenko, A., Müller, K.-R. & Von Lilienfeld, O. A. Fast and accurate modeling of molecular atomization energies with machine learning. Phys. Rev. Lett. 108 , 058301 (2012).
Bartók, A. P., Kondor, R. & Csányi, G. On representing chemical environments. Phys. Rev. B 87 , 184115 (2013).
Faber, F. A. et al. Prediction errors of molecular machine learning models lower than hybrid dft error. J. Chem. Theory Comput. 13 , 5255–5264 (2017).
Choudhary, K., DeCost, B. & Tavazza, F. Machine learning with force-field-inspired descriptors for materials: Fast screening and mapping energy landscape. Phys. Rev. Mater. 2 , 083801 (2018).
Choudhary, K., Garrity, K. F., Ghimire, N. J., Anand, N. & Tavazza, F. High-throughput search for magnetic topological materials using spin-orbit spillage, machine learning, and experiments. Phys. Rev. B 103 , 155131 (2021).
Choudhary, K., Garrity, K. F. & Tavazza, F. Data-driven discovery of 3d and 2d thermoelectric materials. J. Phys. Condens. Matter 32 , 475501 (2020).
Ward, L. et al. Including crystal structure attributes in machine learning models of formation energies via voronoi tessellations. Phys. Rev. B 96 , 024104 (2017).
Isayev, O. et al. Universal fragment descriptors for predicting properties of inorganic crystals. Nat. Commun. 8 , 1–12 (2017).
Liu, C.-H., Tao, Y., Hsu, D., Du, Q. & Billinge, S. J. Using a machine learning approach to determine the space group of a structure from the atomic pair distribution function. Acta Crystallogr. Sec. A 75 , 633–643 (2019).
Smith, J. S., Isayev, O. & Roitberg, A. E. Ani-1: an extensible neural network potential with dft accuracy at force field computational cost. Chem. Sci. 8 , 3192–3203 (2017).
Behler, J. Atom-centered symmetry functions for constructing high-dimensional neural network potentials. J. Chem. Phys. 134 , 074106 (2011).
Behler, J. & Parrinello, M. Generalized neural-network representation of high-dimensional potential-energy surfaces. Phys. Rev. Lett. 98 , 146401 (2007).
Ko, T. W., Finkler, J. A., Goedecker, S. & Behler, J. A fourth-generation high-dimensional neural network potential with accurate electrostatics including non-local charge transfer. Nat. Commun. 12 , 398 (2021).
Weinreich, J., Romer, A., Paleico, M. L. & Behler, J. Properties of alpha-brass nanoparticles. 1. neural network potential energy surface. J. Phys. Chem C 124 , 12682–12695 (2020).
Wang, H., Zhang, L., Han, J. & E, W. Deepmd-kit: A deep learning package for many-body potential energy representation and molecular dynamics. Computer Phys. Commun. 228 , 178–184 (2018).
Eshet, H., Khaliullin, R. Z., Kühne, T. D., Behler, J. & Parrinello, M. Ab initio quality neural-network potential for sodium. Phys. Rev. B 81 , 184107 (2010).
Khaliullin, R. Z., Eshet, H., Kühne, T. D., Behler, J. & Parrinello, M. Graphite-diamond phase coexistence study employing a neural-network mapping of the ab initio potential energy surface. Phys. Rev. B 81 , 100103 (2010).
Artrith, N. & Urban, A. An implementation of artificial neural-network potentials for atomistic materials simulations: Performance for tio2. Comput. Mater. Sci. 114 , 135–150 (2016).
Park, C. W. et al. Accurate and scalable graph neural network force field and molecular dynamics with direct force architecture. npj Comput. Mater. 7 , 1–9 (2021).
Chmiela, S., Sauceda, H. E., Müller, K.-R. & Tkatchenko, A. Towards exact molecular dynamics simulations with machine-learned force fields. Nat. Commun. 9 , 1–10 (2018).
Xue, L.-Y. et al. Reaxff-mpnn machine learning potential: a combination of reactive force field and message passing neural networks. Phys. Chem. Chem. Phys. 23 , 19457–19464 (2021).
Gilmer, J., Schoenholz, S. S., Riley, P. F., Vinyals, O. & Dahl, G. E. Neural message passing for quantum chemistry. arXiv . https://arxiv.org/abs/1704.01212 (2017).
Zitnick, C. L. et al. An introduction to electrocatalyst design using machine learning for renewable energy storage. arXiv. https://arxiv.org/abs/2010.09435 (2020).
McNutt, A. T. et al. Gnina 1 molecular docking with deep learning. J. Cheminformatics 13 , 1–20 (2021).
Jin, W., Barzilay, R. & Jaakkola, T. Junction tree variational autoencoder for molecular graph generation. in International conference on machine learning , 2323–2332 (PMLR, 2018).
Olivecrona, M., Blaschke, T., Engkvist, O. & Chen, H. Molecular de-novo design through deep reinforcement learning. J. Cheminformatics 9 , 1–14 (2017).
You, J., Liu, B., Ying, R., Pande, V. & Leskovec, J. Graph convolutional policy network for goal-directed molecular graph generation. arXiv. https://arxiv.org/abs/1806.02473 (2018).
Putin, E. et al. Reinforced adversarial neural computer for de novo molecular design. J. Chem. Inf. Model. 58 , 1194–1204 (2018).
Sanchez-Lengeling, B., Outeiral, C., Guimaraes, G. L. & Aspuru-Guzik, A. Optimizing distributions over molecular space. an objective-reinforced generative adversarial network for inverse-design chemistry (organic). ChemRxiv https://doi.org/10.26434/chemrxiv.5309668.v3 (2017).
Nouira, A., Sokolovska, N. & Crivello, J.-C. Crystalgan: learning to discover crystallographic structures with generative adversarial networks. arXiv. https://arxiv.org/abs/1810.11203 (2018).
Long, T. et al. Constrained crystals deep convolutional generative adversarial network for the inverse design of crystal structures. npj Comput. Mater. 7 , 66 (2021).
Noh, J. et al. Inverse design of solid-state materials via a continuous representation. Matter 1 , 1370–1384 (2019).
Kim, S., Noh, J., Gu, G. H., Aspuru-Guzik, A. & Jung, Y. Generative adversarial networks for crystal structure prediction. ACS Central Sci. 6 , 1412–1420 (2020).
Long, T. et al. Inverse design of crystal structures for multicomponent systems. arXiv. https://arxiv.org/abs/2104.08040 (2021).
Xie, T. & Grossman, J. C. Hierarchical visualization of materials space with graph convolutional neural networks. J. Chem. Phys. 149 , 174111 (2018).
Park, C. W. & Wolverton, C. Developing an improved crystal graph convolutional neural network framework for accelerated materials discovery. Phys. Rev. Mater. 4 , 063801 (2020).
Laugier, L. et al. Predicting thermoelectric properties from crystal graphs and material descriptors-first application for functional materials. arXiv. https://arxiv.org/abs/1811.06219 (2018).
Rosen, A. S. et al. Machine learning the quantum-chemical properties of metal–organic frameworks for accelerated materials discovery. Matter 4 , 1578–1597 (2021).
Lusci, A., Pollastri, G. & Baldi, P. Deep architectures and deep learning in chemoinformatics: the prediction of aqueous solubility for drug-like molecules. J. Chem. Inf. Model. 53 , 1563–1575 (2013).
Xu, Y. et al. Deep learning for drug-induced liver injury. J. Chem. Inf. Model. 55 , 2085–2093 (2015).
Jain, A. & Bligaard, T. Atomic-position independent descriptor for machine learning of material properties. Phys. Rev. B 98 , 214112 (2018).
Goodall, R. E., Parackal, A. S., Faber, F. A., Armiento, R. & Lee, A. A. Rapid discovery of novel materials by coordinate-free coarse graining. arXiv . https://arxiv.org/abs/2106.11132 (2021).
Zuo, Y. et al. Accelerating Materials Discovery with Bayesian Optimization and Graph Deep Learning. arXiv . https://arxiv.org/abs/2104.10242 (2021).
Lin, T.-S. et al. Bigsmiles: a structurally-based line notation for describing macromolecules. ACS Central Sci. 5 , 1523–1531 (2019).
Tyagi, A. et al. Cancerppd: a database of anticancer peptides and proteins. Nucleic Acids Res. 43 , D837–D843 (2015).
Krenn, M., Häse, F., Nigam, A., Friederich, P. & Aspuru-Guzik, A. Self-referencing embedded strings (selfies): a 100% robust molecular string representation. Machine Learn. Sci. Technol. 1 , 045024 (2020).
Lim, J., Ryu, S., Kim, J. W. & Kim, W. Y. Molecular generative model based on conditional variational autoencoder for de novo molecular design. J. Cheminformatics 10 , 1–9 (2018).
Krasnov, L., Khokhlov, I., Fedorov, M. V. & Sosnin, S. Transformer-based artificial neural networks for the conversion between chemical notations. Sci. Rep. 11 , 1–10 (2021).
Irwin, J. J., Sterling, T., Mysinger, M. M., Bolstad, E. S. & Coleman, R. G. Zinc: a free tool to discover chemistry for biology. J. Chem. Inf. Model. 52 , 1757–1768 (2012).
Dix, D. J. et al. The toxcast program for prioritizing toxicity testing of environmental chemicals. Toxicol. Sci. 95 , 5–12 (2007).
Kim, S. et al. Pubchem 2019 update: improved access to chemical data. Nucleic Acids Res. 47 , D1102–D1109 (2019).
Hirohara, M., Saito, Y., Koda, Y., Sato, K. & Sakakibara, Y. Convolutional neural network based on smiles representation of compounds for detecting chemical motif. BMC Bioinformatics 19 , 83–94 (2018).
Gómez-Bombarelli, R. et al. Automatic chemical design using a data-driven continuous representation of molecules. ACS Central Sci. 4 , 268–276 (2018).
Liu, R. et al. Deep learning for chemical compound stability prediction . In Proceedings of ACM SIGKDD workshop on large-scale deep learning for data mining (DL-KDD) , 1–7. https://rosanneliu.com/publication/kdd/ (ACM SIGKDD, 2016).
Jha, D. et al. Elemnet: Deep learning the chem. mater. from only elemental composition. Sci. Rep. 8 , 1–13 (2018).
Agrawal, A. et al. Exploration of data science techniques to predict fatigue strength of steel from composition and processing parameters. Integr. Mater. Manuf. Innov. 3 , 90–108 (2014).
Agrawal, A. & Choudhary, A. A fatigue strength predictor for steels using ensemble data mining: steel fatigue strength predictor . In Proceedings of the 25th ACM International on Conference on information and knowledge management , 2497–2500. https://doi.org/10.1145/2983323.2983343 (2016).
Agrawal, A. & Choudhary, A. An online tool for predicting fatigue strength of steel alloys based on ensemble data mining. Int. J. Fatigue 113 , 389–400 (2018).
Agrawal, A., Saboo, A., Xiong, W., Olson, G. & Choudhary, A. Martensite start temperature predictor for steels using ensemble data mining . in 2019 IEEE International Conference on Data Science and Advanced Analytics (DSAA) , 521–530 (IEEE, 2019).
Meredig, B. et al. Combinatorial screening for new materials in unconstrained composition space with machine learning. Phys. Rev. B 89 , 094104 (2014).
Agrawal, A., Meredig, B., Wolverton, C. & Choudhary, A. A formation energy predictor for crystalline materials using ensemble data mining . in 2016 IEEE 16th International Conference on Data Mining Workshops (ICDMW) , 1276–1279 (IEEE, 2016).
Furmanchuk, A., Agrawal, A. & Choudhary, A. Predictive analytics for crystalline materials: bulk modulus. RSC Adv. 6 , 95246–95251 (2016).
Furmanchuk, A. et al. Prediction of seebeck coefficient for compounds without restriction to fixed stoichiometry: A machine learning approach. J. Comput. Chem. 39 , 191–202 (2018).
Ward, L., Agrawal, A., Choudhary, A. & Wolverton, C. A general-purpose machine learning framework for predicting properties of inorganic materials. npj Comput. Mater. 2 , 1–7 (2016).
Ward, L. et al. Matminer: An open source toolkit for materials data mining. Comput. Mater. Sci. 152 , 60–69 (2018).
Jha, D. et al. Irnet: A general purpose deep residual regression framework for materials discovery . In Proceedings of the 25th ACM SIGKDD International Conference on Knowledge Discovery & Data Mining , 2385–2393. https://arxiv.org/abs/1907.03222 (2019).
Jha, D. et al. Enabling deeper learning on big data for materials informatics applications. Sci. Rep. 11 , 1–12 (2021).
Goodall, R. E. & Lee, A. A. Predicting materials properties without crystal structure: Deep representation learning from stoichiometry. Nat. Commun. 11 , 1–9 (2020).
NIMS. Superconducting material database (supercon) . https://supercon.nims.go.jp/ (2021).
Stanev, V. et al. Machine learning modeling of superconducting critical temperature. npj Comput. Mater. 4 , 1–14 (2018).
Gupta, V. et al. Cross-property deep transfer learning framework for enhanced predictive analytics on small materials data. Nat. Commun . 12 , 1–10 (2021).
Himanen, L. et al. Dscribe: Library of descriptors for machine learning in materials science. Computer Phys. Commun. 247 , 106949 (2020).
Bartel, C. J. et al. A critical examination of compound stability predictions from machine-learned formation energies. npj Comput. Mater. 6 , 1–11 (2020).
Choudhary, K. et al. High-throughput density functional perturbation theory and machine learning predictions of infrared, piezoelectric, and dielectric responses. npj Comput. Mater. 6 , 1–13 (2020).
Zheng, C. et al. Automated generation and ensemble-learned matching of X-ray absorption spectra. npj Comput. Mater. 4 , 1–9 (2018).
Mathew, K. et al. High-throughput computational x-ray absorption spectroscopy. Sci. Data 5 , 1–8 (2018).
Chen, Y. et al. Database of ab initio l-edge x-ray absorption near edge structure. Sci. Data 8 , 1–8 (2021).
Lafuente, B., Downs, R. T., Yang, H. & Stone, N. In Highlights in mineralogical crystallography 1–30 (De Gruyter (O), 2015).
El Mendili, Y. et al. Raman open database: first interconnected raman–x-ray diffraction open-access resource for material identification. J. Appl. Crystallogr. 52 , 618–625 (2019).
Fremout, W. & Saverwyns, S. Identification of synthetic organic pigments: the role of a comprehensive digital raman spectral library. J. Raman Spectrosc. 43 , 1536–1544 (2012).
Huck, P. & Persson, K. A. Mpcontribs: user contributed data to the materials project database . https://docs.mpcontribs.org/ (2019).
Yang, L. et al. A cloud platform for atomic pair distribution function analysis: Pdfitc. Acta Crystallogr. A 77 , 2–6 (2021).
Park, W. B. et al. Classification of crystal structure using a convolutional neural network. IUCrJ 4 , 486–494 (2017).
Hellenbrandt, M. The Inorganic Crystal Structure Database (ICSD)—present and future. Crystallogr. Rev. 10 , 17–22 (2004).
Zaloga, A. N., Stanovov, V. V., Bezrukova, O. E., Dubinin, P. S. & Yakimov, I. S. Crystal symmetry classification from powder X-ray diffraction patterns using a convolutional neural network. Mater. Today Commun. 25 , 101662 (2020).
Lee, J.-W., Park, W. B., Lee, J. H., Singh, S. P. & Sohn, K.-S. A deep-learning technique for phase identification in multiphase inorganic compounds using synthetic XRD powder patterns. Nat. Commun. 11 , 86 (2020).
Wang, H. et al. Rapid identification of X-ray diffraction patterns based on very limited data by interpretable convolutional neural networks. J. Chem. Inf. Model. 60 , 2004–2011 (2020).
Dong, H. et al. A deep convolutional neural network for real-time full profile analysis of big powder diffraction data. npj Comput. Mater. 7 , 1–9 (2021).
Aguiar, J. A., Gong, M. L. & Tasdizen, T. Crystallographic prediction from diffraction and chemistry data for higher throughput classification using machine learning. Comput. Mater. Sci. 173 , 109409 (2020).
Maffettone, P. M. et al. Crystallography companion agent for high-throughput materials discovery. Nat. Comput. Sci. 1 , 290–297 (2021).
Oviedo, F. et al. Fast and interpretable classification of small X-ray diffraction datasets using data augmentation and deep neural networks. npj Comput. Mater. 5 , 1–9 (2019).
Liu, C.-H. et al. Validation of non-negative matrix factorization for rapid assessment of large sets of atomic pair-distribution function (pdf) data. J. Appl. Crystallogr. 54 , 768–775 (2021).
Rakita, Y. et al. Studying heterogeneities in local nanostructure with scanning nanostructure electron microscopy (snem). arXiv https://arxiv.org/abs/2110.03589 (2021).
Timoshenko, J., Lu, D., Lin, Y. & Frenkel, A. I. Supervised machine-learning-based determination of three-dimensional structure of metallic nanoparticles. J. Phys. Chem Lett. 8 , 5091–5098 (2017).
Timoshenko, J. et al. Subnanometer substructures in nanoassemblies formed from clusters under a reactive atmosphere revealed using machine learning. J. Phys. Chem C 122 , 21686–21693 (2018).
Timoshenko, J. et al. Neural network approach for characterizing structural transformations by X-ray absorption fine structure spectroscopy. Phys. Rev. Lett. 120 , 225502 (2018).
Zheng, C., Chen, C., Chen, Y. & Ong, S. P. Random forest models for accurate identification of coordination environments from X-ray absorption near-edge structure. Patterns 1 , 100013 (2020).
Torrisi, S. B. et al. Random forest machine learning models for interpretable X-ray absorption near-edge structure spectrum-property relationships. npj Comput. Mater. 6 , 1–11 (2020).
Andrejevic, N., Andrejevic, J., Rycroft, C. H. & Li, M. Machine learning spectral indicators of topology. arXiv preprint at https://arxiv.org/abs/2003.00994 (2020).
Madden, M. G. & Ryder, A. G. Machine learning methods for quantitative analysis of raman spectroscopy data . in Opto-Ireland 2002: Optics and Photonics Technologies and Applications , Vol. 4876, 1130–1139 (International Society for Optics and Photonics, 2003).
Conroy, J., Ryder, A. G., Leger, M. N., Hennessey, K. & Madden, M. G. Qualitative and quantitative analysis of chlorinated solvents using Raman spectroscopy and machine learning . in Opto-Ireland 2005: Optical Sensing and Spectroscopy, Vol. 5826, 131–142 (International Society for Optics and Photonics, 2005).
Acquarelli, J. et al. Convolutional neural networks for vibrational spectroscopic data analysis. Anal. Chim. Acta 954 , 22–31 (2017).
O’Connell, M.-L., Howley, T., Ryder, A. G., Leger, M. N. & Madden, M. G. Classification of a target analyte in solid mixtures using principal component analysis, support vector machines, and Raman spectroscopy . in Opto-Ireland 2005: Optical Sensing and Spectroscopy , Vol. 5826, 340–350 (International Society for Optics and Photonics, 2005).
Zhao, J., Chen, Q., Huang, X. & Fang, C. H. Qualitative identification of tea categories by near infrared spectroscopy and support vector machine. J. Pharm. Biomed. Anal. 41 , 1198–1204 (2006).
Liu, J. et al. Deep convolutional neural networks for Raman spectrum recognition: a unified solution. Analyst 142 , 4067–4074 (2017).
Yang, J. et al. Deep learning for vibrational spectral analysis: Recent progress and a practical guide. Anal. Chim. Acta 1081 , 6–17 (2019).
Selzer, P., Gasteiger, J., Thomas, H. & Salzer, R. Rapid access to infrared reference spectra of arbitrary organic compounds: scope and limitations of an approach to the simulation of infrared spectra by neural networks. Chem. Euro. J. 6 , 920–927 (2000).
Ghosh, K. et al. Deep learning spectroscopy: neural networks for molecular excitation spectra. Adv. Sci. 6 , 1801367 (2019).
Kostka, T., Selzer, P. & Gasteiger, J. A combined application of reaction prediction and infrared spectra simulation for the identification of degradation products of s-triazine herbicides. Chemistry 7 , 2254–2260 (2001).
Mahmoud, C. B., Anelli, A., Csányi, G. & Ceriotti, M. Learning the electronic density of states in condensed matter. Phys. Rev. B 102 , 235130 (2020).
Chen, Z. et al. Direct prediction of phonon density of states with Euclidean neural networks. Adv. Sci. 8 , 2004214 (2021).
Kong, S. et al. Density of states prediction for materials discovery via contrastive learning from probabilistic embeddings. arXiv . https://arxiv.org/abs/2110.11444 (2021).
Carbone, M. R., Topsakal, M., Lu, D. & Yoo, S. Machine-learning X-ray absorption spectra to quantitative accuracy. Phys. Rev. Lett. 124 , 156401 (2020).
Rehr, J. J., Kas, J. J., Vila, F. D., Prange, M. P. & Jorissen, K. Parameter-free calculations of X-ray spectra with FEFF9. Phys. Chem. Chem. Phys. 12 , 5503–5513 (2010).
Rankine, C. D., Madkhali, M. M. M. & Penfold, T. J. A deep neural network for the rapid prediction of X-ray absorption spectra. J. Phys. Chem A 124 , 4263–4270 (2020).
Fung, V., Hu, G., Ganesh, P. & Sumpter, B. G. Machine learned features from density of states for accurate adsorption energy prediction. Nat. Commun. 12 , 88 (2021).
Hammer, B. & Nørskov, J. Theoretical surface science and catalysis-calculations and concepts. Adv. Catal. Impact Surface Sci. Catal. 45 , 71–129 (2000).
Kaundinya, P. R., Choudhary, K. & Kalidindi, S. R. Prediction of the electron density of states for crystalline compounds with atomistic line graph neural networks (alignn). arXiv. https://arxiv.org/abs/2201.08348 (2022).
Stein, H. S., Soedarmadji, E., Newhouse, P. F., Guevarra, D. & Gregoire, J. M. Synthesis, optical imaging, and absorption spectroscopy data for 179072 metal oxides. Sci. Data 6 , 9 (2019).
Choudhary, A. et al. Graph neural network predictions of metal organic framework co2 adsorption properties. arXiv . https://arxiv.org/abs/2112.10231 (2021).
Anderson, R., Biong, A. & Gómez-Gualdrón, D. A. Adsorption isotherm predictions for multiple molecules in mofs using the same deep learning model. J. Chem. Theory Comput. 16 , 1271–1283 (2020).
Krizhevsky, A., Sutskever, I. & Hinton, G. E. Imagenet classification with deep convolutional neural networks. Adv. Neural Inf. Process. Syst. 25 , 1097–1105 (2012).
Varela, M. et al. Materials characterization in the aberration-corrected scanning transmission electron microscope. Annu. Rev. Mater. Res. 35 , 539–569 (2005).
Holm, E. A. et al. Overview: Computer vision and machine learning for microstructural characterization and analysis. Metal. Mater Trans. A 51 , 5985–5999 (2020).
Modarres, M. H. et al. Neural network for nanoscience scanning electron microscope image recognition. Sci. Rep. 7 , 1–12 (2017).
Gopalakrishnan, K., Khaitan, S. K., Choudhary, A. & Agrawal, A. Deep convolutional neural networks with transfer learning for computer vision-based data-driven pavement distress detection. Construct. Build. Mater. 157 , 322–330 (2017).
Gopalakrishnan, K., Gholami, H., Vidyadharan, A., Choudhary, A. & Agrawal, A. Crack damage detection in unmanned aerial vehicle images of civil infrastructure using pre-trained deep learning model. Int. J. Traffic Transp. Eng . 8 , 1–14 (2018).
Yang, Z. et al. Data-driven insights from predictive analytics on heterogeneous experimental data of industrial magnetic materials . In IEEE International Conference on Data Mining Workshops (ICDMW) , 806–813. https://doi.org/10.1109/ICDMW.2019.00119 (IEEE Computer Society, 2019).
Yang, Z. et al. Heterogeneous feature fusion based machine learning on shallow-wide and heterogeneous-sparse industrial datasets . In 25th International Conference on Pattern Recognition Workshops, ICPR 2020 , 566–577. https://doi.org/10.1007/978-3-030-68799-1_41 (Springer Science and Business Media Deutschland GmbH, 2021).
Ziletti, A., Kumar, D., Scheffler, M. & Ghiringhelli, L. M. Insightful classification of crystal structures using deep learning. Nat. Commun. 9 , 2775 (2018).
Choudhary, K. et al. Computational scanning tunneling microscope image database. Sci. Data 8 , 1–9 (2021).
Liu, R., Agrawal, A., Liao, W.-k., Choudhary, A. & De Graef, M. Materials discovery: Understanding polycrystals from large-scale electron patterns . in 2016 IEEE International Conference on Big Data (Big Data) , 2261–2269 (IEEE, 2016).
Jha, D. et al. Extracting grain orientations from EBSD patterns of polycrystalline materials using convolutional neural networks. Microsc. Microanal. 24 , 497–502 (2018).
Kaufmann, K., Zhu, C., Rosengarten, A. S. & Vecchio, K. S. Deep neural network enabled space group identification in EBSD. Microsc. Microanal. 26 , 447–457 (2020).
Yang, Z. et al. Deep learning based domain knowledge integration for small datasets: Illustrative applications in materials informatics . in 2019 International Joint Conference on Neural Networks (IJCNN) , 1–8 (IEEE, 2019).
Yang, Z. et al. Learning to predict crystal plasticity at the nanoscale: Deep residual networks and size effects in uniaxial compression discrete dislocation simulations. Sci. Rep. 10 , 1–14 (2020).
Decost, B. L. et al. Uhcsdb: Ultrahigh carbon steel micrograph database. Integr. Mater. Manuf. Innov. 6 , 197–205 (2017).
Decost, B. L., Lei, B., Francis, T. & Holm, E. A. High throughput quantitative metallography for complex microstructures using deep learning: a case study in ultrahigh carbon steel. Microsc. Microanal. 25 , 21–29 (2019).
Stan, T., Thompson, Z. T. & Voorhees, P. W. Optimizing convolutional neural networks to perform semantic segmentation on large materials imaging datasets: X-ray tomography and serial sectioning. Materials Characterization 160 , 110119 (2020).
Madsen, J. et al. A deep learning approach to identify local structures in atomic-resolution transmission electron microscopy images. Adv. Theory Simulations 1 , 1800037 (2018).
Maksov, A. et al. Deep learning analysis of defect and phase evolution during electron beam-induced transformations in ws 2. npj Comput. Mater. 5 , 1–8 (2019).
Yang, S.-H. et al. Deep learning-assisted quantification of atomic dopants and defects in 2d materials. Adv. Sci. https://doi.org/10.1002/advs.202101099 (2021).
Roberts, G. et al. Deep learning for semantic segmentation of defects in advanced stem images of steels. Sci. Rep. 9 , 1–12 (2019).
Kusche, C. et al. Large-area, high-resolution characterisation and classification of damage mechanisms in dual-phase steel using deep learning. PLoS ONE 14 , e0216493 (2019).
Vlcek, L. et al. Learning from imperfections: predicting structure and thermodynamics from atomic imaging of fluctuations. ACS Nano 13 , 718–727 (2019).
Ziatdinov, M., Maksov, A. & Kalinin, S. V. Learning surface molecular structures via machine vision. npj Comput. Mater. 3 , 1–9 (2017).
Ovchinnikov, O. S. et al. Detection of defects in atomic-resolution images of materials using cycle analysis. Adv. Struct. Chem. Imaging 6 , 3 (2020).
Li, W., Field, K. G. & Morgan, D. Automated defect analysis in electron microscopic images. npj Comput. Mater. 4 , 1–9 (2018).
Cohn, R. et al. Instance segmentation for direct measurements of satellites in metal powders and automated microstructural characterization from image data. JOM 73 , 2159–2172 (2021).
de Haan, K., Ballard, Z. S., Rivenson, Y., Wu, Y. & Ozcan, A. Resolution enhancement in scanning electron microscopy using deep learning. Sci. Rep. 9 , 1–7 (2019).
Ede, J. M. & Beanland, R. Partial scanning transmission electron microscopy with deep learning. Sci. Rep. 10 , 1–10 (2020).
Rashidi, M. & Wolkow, R. A. Autonomous scanning probe microscopy in situ tip conditioning through machine learning. ACS Nano 12 , 5185–5189 (2018).
Scime, L., Siddel, D., Baird, S. & Paquit, V. Layer-wise anomaly detection and classification for powder bed additive manufacturing processes: A machine-agnostic algorithm for real-time pixel-wise semantic segmentation. Addit. Manufact. 36 , 101453 (2020).
Eppel, S., Xu, H., Bismuth, M. & Aspuru-Guzik, A. Computer vision for recognition of materials and vessels in chemistry lab settings and the Vector-LabPics Data Set. ACS Central Sci. 6 , 1743–1752 (2020).
Yang, Z. et al. Deep learning approaches for mining structure-property linkages in high contrast composites from simulation datasets. Comput. Mater. Sci. 151 , 278–287 (2018).
Cecen, A., Dai, H., Yabansu, Y. C., Kalidindi, S. R. & Song, L. Material structure-property linkages using three-dimensional convolutional neural networks. Acta Mater. 146 , 76–84 (2018).
Yang, Z. et al. Establishing structure-property localization linkages for elastic deformation of three-dimensional high contrast composites using deep learning approaches. Acta Mater. 166 , 335–345 (2019).
Goetz, A. et al. Addressing materials’ microstructure diversity using transfer learning. arXiv . arXiv-2107. https://arxiv.org/abs/2107.13841 (2021).
Kitahara, A. R. & Holm, E. A. Microstructure cluster analysis with transfer learning and unsupervised learning. Integr. Mater. Manuf. Innov. 7 , 148–156 (2018).
Larmuseau, M. et al. Compact representations of microstructure images using triplet networks. npj Comput. Mater. 2020 6:1 6 , 1–11 (2020).
Li, X. et al. A deep adversarial learning methodology for designing microstructural material systems . in International Design Engineering Technical Conferences and Computers and Information in Engineering Conference , Vol. 51760, V02BT03A008 (American Society of Mechanical Engineers, 2018).
Yang, Z. et al. Microstructural materials design via deep adversarial learning methodology. J. Mech. Des. 140 , 111416 (2018).
Yang, Z. et al. A general framework combining generative adversarial networks and mixture density networks for inverse modeling in microstructural materials design. arXiv . https://arxiv.org/abs/2101.10553 (2021).
Hsu, T. et al. Microstructure generation via generative adversarial network for heterogeneous, topologically complex 3d materials. JOM 73 , 90–102 (2020).
Chun, S. et al. Deep learning for synthetic microstructure generation in a materials-by-design framework for heterogeneous energetic materials. Sci. Rep. 10 , 1–15 (2020).
Dai, M., Demirel, M. F., Liang, Y. & Hu, J.-M. Graph neural networks for an accurate and interpretable prediction of the properties of polycrystalline materials. npj Comput. Mater. 7 , 1–9 (2021).
Cohn, R. & Holm, E. Neural message passing for predicting abnormal grain growth in Monte Carlo simulations of microstructural evolution. arXiv. https://arxiv.org/abs/2110.09326v1 (2021).
Plimpton, S. et al. SPPARKS Kinetic Monte Carlo Simulator . https://spparks.github.io/index.html . (2021).
Plimpton, S. et al. Crossing the mesoscale no-man’s land via parallel kinetic Monte Carlo. Tech. Rep . https://doi.org/10.2172/966942 (2009).
Xue, N. Steven bird, evan klein and edward loper. natural language processing with python. oreilly media, inc.2009. isbn: 978-0-596-51649-9. Nat. Lang. Eng. 17 , 419–424 (2010).
Honnibal, M. & Montani, I. spaCy 2: Natural language understanding with Bloom embeddings, convolutional neural networks and incremental parsing. https://doi.org/10.5281/zenodo.3358113 (2017).
Gardner, M. et al. Allennlp: A deep semantic natural language processing platform. arXiv. https://arxiv.org/abs/1803.07640 (2018).
Tshitoyan, V. et al. Unsupervised word embeddings capture latent knowledge from materials science literature. Nature 571 , 95–98 (2019).
Kononova, O. et al. Opportunities and challenges of text mining in aterials research. iScience 24 , 102155 (2021).
Olivetti, E. A. et al. Data-driven materials research enabled by natural language processing and information extraction. Appl. Phys. Rev. 7 , 041317 (2020).
Swain, M. C. & Cole, J. M. Chemdataextractor: a toolkit for automated extraction of chemical information from the scientific literature. J. Chem. Inf. Model. 56 , 1894–1904 (2016).
Park, S. et al. Text mining metal–organic framework papers. J. Chem. Inf. Model. 58 , 244–251 (2018).
Court, C. J. & Cole, J. M. Auto-generated materials database of curie and néel temperatures via semi-supervised relationship extraction. Sci. Data 5 , 1–12 (2018).
Huang, S. & Cole, J. M. A database of battery materials auto-generated using chemdataextractor. Sci. Data 7 , 1–13 (2020).
Beard, E. J., Sivaraman, G., Vázquez-Mayagoitia, Á., Vishwanath, V. & Cole, J. M. Comparative dataset of experimental and computational attributes of uv/vis absorption spectra. Sci. Data 6 , 1–11 (2019).
Tayfuroglu, O., Kocak, A. & Zorlu, Y. In silico investigation into h2 uptake in mofs: combined text/data mining and structural calculations. Langmuir 36 , 119–129 (2019).
Weston, L. et al. Named entity recognition and normalization applied to large-scale information extraction from the materials science literature. J. Chem. Inf. Model. 59 , 3692–3702 (2019).
Vaucher, A. C. et al. Automated extraction of chemical synthesis actions from experimental procedures. Nat. Commun. 11 , 1–11 (2020).
He, T. et al. Similarity of precursors in solid-state synthesis as text-mined from scientific literature. Chem. Mater. 32 , 7861–7873 (2020).
Kononova, O. et al. Text-mined dataset of inorganic materials synthesis recipes. Sci. Data 6 , 1–11 (2019).
Kim, E. et al. Materials synthesis insights from scientific literature via text extraction and machine learning. Chem. Mater. 29 , 9436–9444 (2017).
Kim, E., Huang, K., Jegelka, S. & Olivetti, E. Virtual screening of inorganic materials synthesis parameters with deep learning. npj Comput. Mater. 3 , 1–9 (2017).
Kim, E. et al. Inorganic materials synthesis planning with literature-trained neural networks. J. Chem. Inf. Model. 60 , 1194–1201 (2020).
de Castro, P. B. et al. Machine-learning-guided discovery of the gigantic magnetocaloric effect in hob 2 near the hydrogen liquefaction temperature. NPG Asia Mater. 12 , 1–7 (2020).
Cooper, C. B. et al. Design-to-device approach affords panchromatic co-sensitized solar cells. Adv. Energy Mater. 9 , 1802820 (2019).
Yang, X., Dai, Z., Zhao, Y., Liu, J. & Meng, S. Low lattice thermal conductivity and excellent thermoelectric behavior in li3sb and li3bi. J. Phys. Condens. Matter 30 , 425401 (2018).
Wang, Y., Gao, Z. & Zhou, J. Ultralow lattice thermal conductivity and electronic properties of monolayer 1t phase semimetal site2 and snte2. Phys. E 108 , 53–59 (2019).
Jong, U.-G., Yu, C.-J., Kye, Y.-H., Hong, S.-N. & Kim, H.-G. Manifestation of the thermoelectric properties in ge-based halide perovskites. Phys. Rev. Mater. 4 , 075403 (2020).
Yamamoto, K., Narita, G., Yamasaki, J. & Iikubo, S. First-principles study of thermoelectric properties of mixed iodide perovskite cs (b, b’) i3 (b, b’= ge, sn, and pb). J. Phys. Chem. Solids 140 , 109372 (2020).
Viennois, R. et al. Anisotropic low-energy vibrational modes as an effect of cage geometry in the binary barium silicon clathrate b a 24 s i 100. Phys. Rev. B 101 , 224302 (2020).
Haque, E. Effect of electron-phonon scattering, pressure and alloying on the thermoelectric performance of tmcu _3 ch _4(tm= v, nb, ta; ch= s, se, te). arXiv . https://arxiv.org/abs/2010.08461 (2020).
Yahyaoglu, M. et al. Phase-transition-enhanced thermoelectric transport in rickardite mineral cu3–x te2. Chem. Mater. 33 , 1832–1841 (2021).
Ho, D., Shkolnik, A. S., Ferraro, N. J., Rizkin, B. A. & Hartman, R. L. Using word embeddings in abstracts to accelerate metallocene catalysis polymerization research. Computers Chem. Eng. 141 , 107026 (2020).
Abdar, M. et al. A review of uncertainty quantification in deep learning: techniques, applications and challenges. Inf. Fusion . 76 , 243–297 (2021).
Mi, Lu, et al. Training-free uncertainty estimation for dense regression: Sensitivityas a surrogate. arXiv . preprint at arXiv:1910.04858. https://arxiv.org/abs/1910.04858 (2019).
Teye, M., Azizpour, H. & Smith, K. Bayesian uncertainty estimation for batch normalized deep networks . in International Conference on Machine Learning , 4907–4916 (PMLR, 2018).
Zhang, J., Kailkhura, B. & Han, T. Y.-J. Leveraging uncertainty from deep learning for trustworthy material discovery workflows. ACS Omega 6 , 12711–12721 (2021).
Meredig, B. et al. Can machine learning identify the next high-temperature superconductor? examining extrapolation performance for materials discovery. Mol. Syst. Des. Eng. 3 , 819–825 (2018).
Zhang, J., Kailkhura, B. & Han, T. Y.-J. Mix-n-match: Ensemble and compositional methods for uncertainty calibration in deep learning . in International Conference on Machine Learning , 11117–11128 (PMLR, 2020).
Seoh, R. Qualitative analysis of monte carlo dropout. arXiv. https://arxiv.org/abs/2007.01720 (2020).
Gal, Y. & Ghahramani, Z. Dropout as a bayesian approximation: Representing model uncertainty in deep learning . in international conference on machine learning , 1050–1059 (PMLR, 2016).
Jain, S., Liu, G., Mueller, J. & Gifford, D. Maximizing overall diversity for improved uncertainty estimates in deep ensembles . In Proceedings of the AAAI Conference on Artificial Intelligence , 34 , 4264–4271. https://doi.org/10.1609/aaai.v34i04.5849 (2020).
Ganaie, M. et al. Ensemble deep learning: a review. arXiv . https://arxiv.org/abs/2104.02395 (AAAI Technical Track: Machine Learning, 2021).
Fort, S., Hu, H. & Lakshminarayanan, B. Deep ensembles: a loss landscape perspective. arXiv. https://arxiv.org/abs/1912.02757 (2019).
Lakshminarayanan, B., Pritzel, A. & Blundell, C. Simple and scalable predictive uncertainty estimation using deep ensembles. arXiv. https://arxiv.org/abs/1612.01474 (2016).
Moon, S. J., Jeon, J.-J., Lee, J. S. H. & Kim, Y. Learning multiple quantiles with neural networks. J. Comput. Graph. Stat. 30 , 1–11. https://doi.org/10.1080/10618600.2021.1909601 (2021).
Rasmussen, C. E. Summer School on Machine Learning , 63–71 (Springer, 2003).
Hegde, P., Heinonen, M., Lähdesmäki, H. & Kaski, S. Deep learning with differential gaussian process flows. arXiv. https://arxiv.org/abs/1810.04066 (2018).
Wilson, A. G., Hu, Z., Salakhutdinov, R. & Xing, E. P. Deep kernel learning. in Artificial intelligence and statistics , 370–378 (PMLR, 2016).
Hegde, V. I. et al. Reproducibility in high-throughput density functional theory: a comparison of aflow, materials project, and oqmd. arXiv. https://arxiv.org/abs/2007.01988 (2020).
Ying, R., Bourgeois, D., You, J., Zitnik, M. & Leskovec, J. Gnnexplainer: Generating explanations for graph neural networks. Adv. Neural Inf. Process. Syst. 32 , 9240 (2019).
Roch, L. M. et al. Chemos: orchestrating autonomous experimentation. Sci. Robot. 3 , eaat5559 (2018).
Szymanski, N. et al. Toward autonomous design and synthesis of novel inorganic materials. Mater. Horiz. 8 , 2169–2198. https://doi.org/10.1039/D1MH00495F (2021).
MacLeod, B. P. et al. Self-driving laboratory for accelerated discovery of thin-film materials. Sci. Adv. 6 , eaaz8867 (2020).
Stach, E. A. et al. Autonomous experimentation systems for materials development: a community perspective. Matter https://www.cell.com/matter/fulltext/S2590-2385(21)00306-4 (2021).
Rakita, Y. et al. Active reaction control of cu redox state based on real-time feedback from i n situ synchrotron measurements. J. Am. Chem. Soc. 142 , 18758–18762 (2020).
Chmiela, S. et al. Machine learning of accurate energy-conserving molecular force fields. Sci. Adv. 3 , e1603015 (2017).
Thomas, R. S. et al. The us federal tox21 program: a strategic and operational plan for continued leadership. Altex 35 , 163 (2018).
Russell Johnson, N. Nist computational chemistry comparison and benchmark database . In The 4th Joint Meeting of the US Sections of the Combustion Institute . https://ci.confex.com/ci/2005/techprogram/P1309.HTM (2005).
Lopez, S. A. et al. The harvard organic photovoltaic dataset. Sci. Data 3 , 1–7 (2016).
Johnson, R. D. et al. Nist computational chemistry comparison and benchmark database . http://srdata.nist.gov/cccbdb (2006).
Mobley, D. L. & Guthrie, J. P. Freesolv: a database of experimental and calculated hydration free energies, with input files. J. Computer Aided Mol. Des. 28 , 711–720 (2014).
Andersen, C. W. et al. Optimade: an api for exchanging materials data. arXiv. https://arxiv.org/abs/2103.02068 (2021).
Chanussot, L. et al. Open catalyst 2020 (oc20) dataset and community challenges. ACS Catal. 11 , 6059–6072 (2021).
Dunn, A., Wang, Q., Ganose, A., Dopp, D. & Jain, A. Benchmarking materials property prediction methods: the matbench test set and automatminer reference algorithm. npj Comput. Mater. 6 , 1–10 (2020).
Talirz, L. et al. Materials cloud, a platform for open computational science. Sci. Data 7 , 1–12 (2020).
Chung, Y. G. et al. Advances, updates, and analytics for the computation-ready, experimental metal–organic framework database: Core mof 2019. J. Chem. Eng. Data 64 , 5985–5998 (2019).
Sussman, J. L. et al. Protein data bank (pdb): database of three-dimensional structural information of biological macromolecules. Acta Crystallogr. Sec. D Biol. Crystallogr. 54 , 1078–1084 (1998).
Benson, M. L. et al. Binding moad, a high-quality protein–ligand database. Nucleic Acids Res. 36 , D674–D678 (2007).
Fung, V., Zhang, J., Juarez, E. & Sumpter, B. G. Benchmarking graph neural networks for materials chemistry. npj Comput. Mater. 7 , 1–8 (2021).
Louis, S.-Y. et al. Graph convolutional neural networks with global attention for improved materials property prediction. Phys. Chem. Chem. Phys. 22 , 18141–18148 (2020).
Khorshidi, A. & Peterson, A. A. Amp: A modular approach to machine learning in atomistic simulations. Computer Phys. Commun. 207 , 310–324 (2016).
Yao, K., Herr, J. E., Toth, D. W., Mckintyre, R. & Parkhill, J. The tensormol-0.1 model chemistry: a neural network augmented with long-range physics. Chem. Sci. 9 , 2261–2269 (2018).
Doerr, S. et al. Torchmd: A deep learning framework for molecular simulations. J. Chem. Theory Comput. 17 , 2355–2363 (2021).
Kolb, B., Lentz, L. C. & Kolpak, A. M. Discovering charge density functionals and structure-property relationships with prophet: A general framework for coupling machine learning and first-principles methods. Sci. Rep. 7 , 1–9 (2017).
Zhang, L., Han, J., Wang, H., Car, R. & Weinan, E. Deep potential molecular dynamics: a scalable model with the accuracy of quantum mechanics. Phys. Rev. Lett. 120 , 143001 (2018).
Geiger, M. et al. e3nn/e3nn: 2021-06-21 . https://doi.org/10.5281/zenodo.5006322 (2021).
Duvenaud, D. K. et al. Convolutional networks on graphs for learning molecular fingerprints (eds. Cortes, C., Lawrence, N. D., Lee, D. D., Sugiyama, M. & Garnett, R.) in Adv. Neural Inf. Process. Syst. 28 2224–2232 (Curran Associates, Inc., 2015).
Li, X. et al. Deepchemstable: Chemical stability prediction with an attention-based graph convolution network. J. Chem. Inf. Model. 59 , 1044–1049 (2019).
Wu, Z. et al. MoleculeNet: A benchmark for molecular machine learning. Chem. Sci. 9 , 513–530 (2018).
Wang, A. Y.-T., Kauwe, S. K., Murdock, R. J. & Sparks, T. D. Compositionally restricted attention-based network for materials property predictions. npj Comput. Mater. 7 , 77 (2021).
Zhou, Q. et al. Learning atoms for materials discovery. Proc. Natl Acad. Sci. USA 115 , E6411–E6417 (2018).
O’Boyle, N. & Dalke, A. Deepsmiles: An adaptation of smiles for use in machine-learning of chemical structures. ChemRxiv https://doi.org/10.26434/chemrxiv.7097960.v1 (2018).
Green, H., Koes, D. R. & Durrant, J. D. Deepfrag: a deep convolutional neural network for fragment-based lead optimization. Chem. Sci. 12 , 8036–8047. https://doi.org/10.1039/D1SC00163A (2021).
Elhefnawy, W., Li, M., Wang, J. & Li, Y. Deepfrag-k: a fragment-based deep learning approach for protein fold recognition. BMC Bioinformatics 21 , 203 (2020).
Paul, A. et al. Chemixnet: Mixed dnn architectures for predicting chemical properties using multiple molecular representations. arXiv . https://arxiv.org/abs/1811.08283 (2018).
Paul, A. et al. Transfer learning using ensemble neural networks for organic solar cell screening . in 2019 International Joint Conference on Neural Networks (IJCNN) , 1–8 (IEEE, 2019).
Choudhary, K. et al. Computational screening of high-performance optoelectronic materials using optb88vdw and tb-mbj formalisms. Sci. Data 5 , 1–12 (2018).
Wong-Ng, W., McMurdie, H., Hubbard, C. & Mighell, A. D. Jcpds-icdd research associateship (cooperative program with nbs/nist). J. Res. Natl Inst. Standards Technol. 106 , 1013 (2001).
Belsky, A., Hellenbrandt, M., Karen, V. L. & Luksch, P. New developments in the inorganic crystal structure database (icsd): accessibility in support of materials research and design. Acta Crystallogr. Sec. B Struct. Sci. 58 , 364–369 (2002).
Gražulis, S. et al. Crystallography Open Database—an open-access collection of crystal structures. J. Appl. Crystallogr. 42 , 726–729 (2009).
Linstrom, P. J. & Mallard, W. G. The nist chemistry webbook: a chemical data resource on the internet. J. Chem. Eng. Data 46 , 1059–1063 (2001).
Saito, T. et al. Spectral database for organic compounds (sdbs). (National Institute of Advanced Industrial Science and Technology (AIST), 2006).
Steinbeck, C., Krause, S. & Kuhn, S. Nmrshiftdb constructing a free chemical information system with open-source components. J. Chem. inf. Computer Sci. 43 , 1733–1739 (2003).
Fung, V., Hu, G., Ganesh, P. & Sumpter, B. G. Machine learned features from density of states for accurate adsorption energy prediction. Nat. Commun. 12 , 1–11 (2021).
Kong, S., Guevarra, D., Gomes, C. P. & Gregoire, J. M. Materials representation and transfer learning for multi-property prediction. arXiv . https://arxiv.org/abs/2106.02225 (2021).
Bang, K., Yeo, B. C., Kim, D., Han, S. S. & Lee, H. M. Accelerated mapping of electronic density of states patterns of metallic nanoparticles via machine-learning. Sci. Rep . 11 , 1–11 (2021).
Chen, D. et al. Automating crystal-structure phase mapping by combining deep learning with constraint reasoning. Nat. Machine Intell. 3 , 812–822 (2021).
Ophus, C. A fast image simulation algorithm for scanning transmission electron microscopy. Adv. Struct. Chem. imaging 3 , 1–11 (2017).
Aversa, R., Modarres, M. H., Cozzini, S., Ciancio, R. & Chiusole, A. The first annotated set of scanning electron microscopy images for nanoscience. Sci. Data 5 , 1–10 (2018).
Ziatdinov, M. et al. Causal analysis of competing atomistic mechanisms in ferroelectric materials from high-resolution scanning transmission electron microscopy data. npj Comput. Mater. 6 , 1–9 (2020).
Souza, A. L. F. et al. Deepfreak: Learning crystallography diffraction patterns with automated machine learning. arXiv. http://arxiv.org/abs/1904.11834 (2019).
Scime, L. et al. Layer-wise imaging dataset from powder bed additive manufacturing processes for machine learning applications (peregrine v2021-03). Tech. Rep . https://www.osti.gov/biblio/1779073 (2021).
Somnath, S., Smith, C. R., Laanait, N., Vasudevan, R. K. & Jesse, S. Usid and pycroscopy–open source frameworks for storing and analyzing imaging and spectroscopy data. Microsc. Microanal. 25 , 220–221 (2019).
Savitzky, B. H. et al. py4dstem: A software package for multimodal analysis of four-dimensional scanning transmission electron microscopy datasets. arXiv. https://arxiv.org/abs/2003.09523 (2020).
Madsen, J. & Susi, T. The abtem code: transmission electron microscopy from first principles. Open Res. Euro. 1 , 24 (2021).
Koch, C. T. Determination of core structure periodicity and point defect density along dislocations . (Arizona State University, 2002).
Allen, L. J. et al. Modelling the inelastic scattering of fast electrons. Ultramicroscopy 151 , 11–22 (2015).
Maxim, Z., Jesse, S., Sumpter, B. G., Kalinin, S. V. & Dyck, O. Tracking atomic structure evolution during directed electron beam induced si-atom motion in graphene via deep machine learning. Nanotechnology 32 , 035703 (2020).
Khadangi, A., Boudier, T. & Rajagopal, V. Em-net: Deep learning for electron microscopy image segmentation . in 2020 25th International Conference on Pattern Recognition (ICPR) , 31–38 (IEEE, 2021).
Meyer, C. et al. Nion swift: Open source image processing software for instrument control, data acquisition, organization, visualization, and analysis using python. Microsc. Microanal. 25 , 122–123 (2019).
Kim, J., Tiong, L. C. O., Kim, D. & Han, S. S. Deep learning-based prediction of material properties using chemical compositions and diffraction patterns as experimentally accessible inputs. J. Phys. Chem Lett. 12 , 8376–8383 (2021).
Von Chamier, L. et al. Zerocostdl4mic: an open platform to simplify access and use of deep-learning in microscopy. BioRxiv. https://www.biorxiv.org/content/10.1101/2020.03.20.000133v4 (2020).
Jha, D. et al. Peak area detection network for directly learning phase regions from raw x-ray diffraction patterns . in 2019 International Joint Conference on Neural Networks (IJCNN) , 1–8 (IEEE, 2019).
Hawizy, L., Jessop, D. M., Adams, N. & Murray-Rust, P. Chemicaltagger: A tool for semantic text-mining in chemistry. J. Cheminformatics 3 , 1–13 (2011).
Corbett, P. & Boyle, J. Chemlistem: chemical named entity recognition using recurrent neural networks. J. Cheminformatics 10 , 1–9 (2018).
Rocktäschel, T., Weidlich, M. & Leser, U. Chemspot: a hybrid system for chemical named entity recognition. Bioinformatics 28 , 1633–1640 (2012).
Jessop, D. M., Adams, S. E., Willighagen, E. L., Hawizy, L. & Murray-Rust, P. Oscar4: a flexible architecture for chemical text-mining. J. Cheminformatics 3 , 1–12 (2011).
Leaman, R., Wei, C.-H. & Lu, Z. tmchem: a high performance approach for chemical named entity recognition and normalization. J. Cheminformatics 7 , 1–10 (2015).
Suzuki, Y. et al. Symmetry prediction and knowledge discovery from X-ray diffraction patterns using an interpretable machine learning approach. Sci. Rep. 10 , 21790 (2020).
Download references
Acknowledgements
Contributions from K.C. were supported by the financial assistance award 70NANB19H117 from the U.S. Department of Commerce, National Institute of Standards and Technology. E.A.H. and R.C. (CMU) were supported by the National Science Foundation under grant CMMI-1826218 and the Air Force D3OM2S Center of Excellence under agreement FA8650-19-2-5209. A.J., C.C., and S.P.O. were supported by the Materials Project, funded by the U.S. Department of Energy, Office of Science, Office of Basic Energy Sciences, Materials Sciences and Engineering Division under contract no. DE-AC02-05-CH11231: Materials Project program KC23MP. S.J.L.B. was supported by the U.S. National Science Foundation through grant DMREF-1922234. A.A. and A.C. were supported by NIST award 70NANB19H005 and NSF award CMMI-2053929.
Author information
Authors and affiliations.
Materials Science and Engineering Division, National Institute of Standards and Technology, Gaithersburg, MD, 20899, USA
Kamal Choudhary & Francesca Tavazza
Theiss Research, La Jolla, CA, 92037, USA
Kamal Choudhary
DeepMaterials LLC, Silver Spring, MD, 20906, USA
Material Measurement Science Division, National Institute of Standards and Technology, Gaithersburg, MD, 20899, USA
Brian DeCost
Department of NanoEngineering, University of California San Diego, San Diego, CA, 92093, USA
Chi Chen & Shyue Ping Ong
Energy Technologies Area, Lawrence Berkeley National Laboratory, Berkeley, CA, USA
Anubhav Jain
Department of Materials Science and Engineering, Carnegie Mellon University, Pittsburgh, PA, 15213, USA
Ryan Cohn & Elizabeth Holm
Department of Materials Science and Engineering, Northwestern University, Evanston, IL, 60208, USA
Cheol Woo Park & Chris Wolverton
Department of Electrical and Computer Engineering, Northwestern University, Evanston, IL, 60208, USA
Alok Choudhary & Ankit Agrawal
Department of Applied Physics and Applied Mathematics and the Data Science Institute, Fu Foundation School of Engineering and Applied Sciences, Columbia University, New York, NY, 10027, USA
Simon J. L. Billinge
You can also search for this author in PubMed Google Scholar
Contributions
The authors contributed equally to the search as well as analysis of the literature and writing of the manuscript.
Corresponding author
Correspondence to Kamal Choudhary .
Ethics declarations
Competing interests.
The authors declare no competing interests.
Additional information
Publisher’s note Springer Nature remains neutral with regard to jurisdictional claims in published maps and institutional affiliations.
Rights and permissions
Open Access This article is licensed under a Creative Commons Attribution 4.0 International License, which permits use, sharing, adaptation, distribution and reproduction in any medium or format, as long as you give appropriate credit to the original author(s) and the source, provide a link to the Creative Commons license, and indicate if changes were made. The images or other third party material in this article are included in the article’s Creative Commons license, unless indicated otherwise in a credit line to the material. If material is not included in the article’s Creative Commons license and your intended use is not permitted by statutory regulation or exceeds the permitted use, you will need to obtain permission directly from the copyright holder. To view a copy of this license, visit http://creativecommons.org/licenses/by/4.0/ .
Reprints and permissions
About this article
Cite this article.
Choudhary, K., DeCost, B., Chen, C. et al. Recent advances and applications of deep learning methods in materials science. npj Comput Mater 8 , 59 (2022). https://doi.org/10.1038/s41524-022-00734-6
Download citation
Received : 25 October 2021
Accepted : 24 February 2022
Published : 05 April 2022
DOI : https://doi.org/10.1038/s41524-022-00734-6
Share this article
Anyone you share the following link with will be able to read this content:
Sorry, a shareable link is not currently available for this article.
Provided by the Springer Nature SharedIt content-sharing initiative
This article is cited by
Analysis of solar energy potentials of five selected south-east cities in nigeria using deep learning algorithms.
- Samuel Ikemba
- Kim Song-hyun
- Akeeb Adepoju Fawole
Sustainable Energy Research (2024)
Correlative, ML-based and non-destructive 3D-analysis of intergranular fatigue cracking in SAC305-Bi solder balls
- Charlotte Cui
- Fereshteh Falah Chamasemani
- Roland Brunner
npj Materials Degradation (2024)
Uncertainty quantification in multivariable regression for material property prediction with Bayesian neural networks
- Jiang Chang
Scientific Reports (2024)
Uncertainty-aware particle segmentation for electron microscopy at varied length scales
- Luca Rettenberger
- Nathan J. Szymanski
- Markus Reischl
npj Computational Materials (2024)
Application of digital twins for simulation based tailoring of laser induced graphene
- José Carlos Santos-Ceballos
- Foad Salehnia
- Xavier Vilanova
Quick links
- Explore articles by subject
- Guide to authors
- Editorial policies
Sign up for the Nature Briefing newsletter — what matters in science, free to your inbox daily.

- Top Courses
- Online Degrees
- Find your New Career
- Join for Free
Machine Learning Models: What They Are and How to Build Them
Machine learning models are the backbone of innovations in everything from finance to retail. Read on to find out more.
![latest research on machine learning [Featured Image]: A woman with long dark hair and wearing a gray turtleneck sweater. She is sitting in front of two computer screens and is wearing white headphones.](https://d3njjcbhbojbot.cloudfront.net/api/utilities/v1/imageproxy/https://images.ctfassets.net/wp1lcwdav1p1/NlyyW0zofW1t3DomgmJK7/8eab2b74a46e93da5679051b3b977324/GettyImages-1332378553.jpg?w=1500&h=680&q=60&fit=fill&f=faces&fm=jpg&fl=progressive&auto=format%2Ccompress&dpr=1&w=1000)
Machine learning models are critical for everything from data science to marketing , finance, retail, and even more. Today there are few industries untouched by the machine learning revolution that has changed not only how businesses operate, but entire industries too.
But what are machine learning models? And how are they built?
In this article, you'll learn how machine learning models are created and find a list of popular algorithms that act as their foundation. You'll also find suggested courses and articles to guide you toward machine learning mastery.
What is a machine learning model?
Machine learning models are computer programs that are used to recognize patterns in data or make predictions.
Machine learning models are created from machine learning algorithms , which undergo a training process using either labeled, unlabeled, or mixed data. Different machine learning algorithms are suited to different goals, such as classification or prediction modeling, so data scientists use different algorithms as the basis for different models. As data is introduced to a specific algorithm, it is modified to better manage a specific task and becomes a machine learning model.
For example, a decision tree is a common algorithm used for both classification and prediction modeling. A data scientist looking to create a machine learning model that identifies different animal species might train a decision tree algorithm with various animal images. Over time, the algorithm would become modified by the data and become increasingly better at classifying animal images. In turn, this would eventually become a machine learning model.
Read more: Decision Trees in Machine Learning: Two Types (+ Examples)
Machine learning models vs. machine learning algorithms
Despite their differences, these two terms are often used interchangeably. Machine learning algorithms are programming procedures. They are methods created to solve a problem or complete a task. Machine learning models are the output of these procedures, containing the data and the procedural guidelines for using that data to predict new data.
How to create a machine learning model
Machine learning models are created by training algorithms with either labeled data, unlabeled data, or a mix of both. Four primary machine learning algorithms exist:
Supervised learning: Supervised learning occurs when an algorithm is trained using “labeled data,” or data that is tagged with a label so that an algorithm can successfully learn from it. Training labels help the eventual machine learning model know how to classify data in the manner that the researcher desires.
Unsupervised learning: Unsupervised algorithms use unlabeled data to train an algorithm. In this process, the algorithm finds patterns in the data itself and creates its own data clusters. Unsupervised learning and pattern recognition are helpful for researchers who are looking to find patterns in data that are currently unknown to them.
Semi-supervised learning: Semi-supervised learning uses a mix of labeled and unlabeled data to train an algorithm. In this process, the algorithm is first trained with a small amount of labeled data before being trained with a much larger amount of unlabeled data.
Reinforcement learning: Reinforcement learning is a machine learning technique in which positive and negative values are assigned to desired and undesired actions. The goal is to encourage programs to avoid the negative training examples and seek out the positive, learning how to maximize rewards through trial and error. Reinforcement learning can be used to direct unsupervised machine learning.
Read more : 7 Machine Learning Algorithms to Know
What are parameters in machine learning?
Before machine learning engineers train a machine learning algorithm, they must first set the hyperparameters for the algorithm, which act as external guides that inform the decision process and direct how the algorithm will learn. For instance, the number of branches on a regression tree, the learning rate, and the number of clusters in a clustering algorithm are all examples of hyperparameters.
As the algorithm is trained and directed by the hyperparameters, parameters begin to form in response to the training data. These parameters include the weights and biases formed by the algorithm as it is being trained. The final parameters for a machine learning model are called the model parameters, which ideally fit a data set without going over or under.
While a machine learning model’s parameters can be identified, the hyperparameters used to create it cannot.
Types of machine learning models
There are two types of problems that dominate machine learning: classification and prediction.
These problems are approached using models derived from algorithms designed for either classification or regression (a method used for predictive modeling). Occasionally, the same algorithm can be used to create either classification or regression models, depending on how it is trained.
Below you will find a list of popular algorithms used to create classification and regression models.
Classification models
Logistic regression
Naive Bayes
Decision trees
Random forest
K-nearest neighbor (KNN)
Support vector machine
Regression models
Linear regression
Ridge regression
Decision trees
Neural network regression
Learn more about ML with expert-level guidance on Coursera
Whether you’re looking to become a data scientist or simply want to deepen your understanding of the field of machine learning, enrolling in an online course can help you advance your career.
In Stanford and DeepLearning.AI's Machine Learning Specialization , you'll master fundamental AI concepts and develop practical machine learning skills in a beginner-friendly, three-course program by AI visionary Andrew Ng.
DeepLearning.AI’s Deep Learning Specialization , meanwhile, teaches you how to build and train neural network architecture and contribute to developing machine learning systems.
Keep reading
Coursera staff.
Editorial Team
Coursera’s editorial team is comprised of highly experienced professional editors, writers, and fact...
This content has been made available for informational purposes only. Learners are advised to conduct additional research to ensure that courses and other credentials pursued meet their personal, professional, and financial goals.

IMAGES
VIDEO
COMMENTS
Machine learning articles from across Nature Portfolio. Machine learning is the ability of a machine to improve its performance based on previous results. Machine learning methods enable computers ...
srameo/le3d • 10 Jun 2024. Volumetric rendering based methods, like NeRF, excel in HDR view synthesis from RAWimages, especially for nighttime scenes. 2k Novel View Synthesis +1. 53. 1.08 stars / hour. Paper. Code. Papers With Code highlights trending Machine Learning research and the code to implement it.
Learning to grok: Emergence of in-context learning and skill composition in modular arithmetic tasks. Tianyu He, Darshil Doshi, Aritra Das, Andrey Gromov. Comments: 21 pages, 19 figures. Subjects: Machine Learning (cs.LG); Disordered Systems and Neural Networks (cond-mat.dis-nn); High Energy Physics - Theory (hep-th); Machine Learning (stat.ML)
Read the latest Research articles in Machine learning from Nature. ... New research unveils the workings of one of the most complex bio-mechanical structures in the natural world.
To discuss the applicability of machine learning-based solutions in various real-world application domains. To highlight and summarize the potential research directions within the scope of our study for intelligent data analysis and services. The rest of the paper is organized as follows.
The Journal of Machine Learning Research (JMLR), , provides an international forum for the electronic and paper publication of high-quality scholarly articles in all areas of machine learning. All published papers are freely available online. JMLR has a commitment to rigorous yet rapid reviewing. Final versions are (ISSN 1533-7928) immediately ...
A data-driven approach to making better choices. In the new economics course 14.163 (Algorithms and Behavioral Science), students investigate the deployment of machine-learning tools and their potential to understand people, reduce bias, and improve society. June 6, 2024. Read full story.
Many of the new developments in machine learning are connected with gradient-based optimization methods. Recently, these methods have been studied using a variational perspective (Betancourt et al., 2018). This has opened up the possibility of ...
Comments: A new versatile family of sequence models that can be used for both generative modeling and supervised learning. The codebase will be made available upon publication. ... Subjects: Machine Learning (cs.LG); Disordered Systems and Neural Networks (cond-mat.dis-nn); Artificial Intelligence (cs.AI); Machine Learning (stat.ML)
The Journal of Machine Learning Research (JMLR) provides an international forum for the electronic and paper publication of high-quality scholarly articles in all areas of machine learning.JMLR seeks previously unpublished papers that contain:new algorithms with empirical, theoretical, psychological, or biological justification; experimental and/or theoretical studies yielding new insight into ...
Overview. Machine Learning is an international forum focusing on computational approaches to learning. Reports substantive results on a wide range of learning methods applied to various learning problems. Provides robust support through empirical studies, theoretical analysis, or comparison to psychological phenomena.
Find the latest published documents for machine learning, Related hot topics, top authors, the most cited documents, and related journals. Find the latest published documents for machine learning, Related hot topics, top authors, the most cited documents, and related journals ... this research aims to predict user's personalities based on ...
In this work, we address the ambitious vision of developing a data-driven approach to predict future research directions 1.As new research ideas often emerge from connecting seemingly unrelated ...
Artificial intelligence (AI), and in particular, Machine Learning (ML), have progressed remarkably in recent years as key instruments to intelligently analyze such data and to develop the corresponding real-world applications (Koteluk et al., 2021; Sarker, 2021b).For instance, ML has emerged as the method of choice for developing practical software for computer vision, speech recognition, and ...
Discover opportunities in Machine Learning. Our research in machine learning breaks new ground every day. Work with us. Explore advancements in state of the art machine learning research in speech and natural language, privacy, computer vision, health, and more.
As computers and the concept of artificial intelligence (AI) were almost simultaneously developed in the 1940s and 1950s, the field of medicine was quick to see their potential relevance and ...
Chui KT, Fung DCL, Lytras MD, Lam TM (2020) Predicting at-risk university students in a virtual learning environment via a machine learning algorithm. Comput Human Behav 107:105584. Article Google Scholar Lytras MD, Visvizi A, Daniela L, Sarirete A, De Pablos PO (2018) Social networks research for sustainable smart education.
MIT researchers created protonic programmable resistors — building blocks of analog deep learning systems — that can process data 1 million times faster than synapses in the human brain. These ultrafast, low-energy resistors could enable analog deep learning systems that can train new and more powerful neural networks rapidly, which could be used for areas like self-driving cars, fraud ...
Top Machine Learning Research Papers Released In 2021. Advances in the machine and deep learning in 2021 could lead to new technologies utilised by billions of people worldwide. Published on November 18, 2021. by Dr. Nivash Jeevanandam. Advances in machine learning and deep learning research are reshaping our technology.
Here are the top 10 AI and machine learning trends to prepare for in 2024. 1. Multimodal AI. Multimodal AI goes beyond traditional single-mode data processing to encompass multiple input types, such as text, images and sound -- a step toward mimicking the human ability to process diverse sensory information.
Research Topics. Core Machine Learning. Related Publications. June 07, 2024. CORE MACHINE LEARNING SYSTEMS RESEARCH. Beyond Efficiency: Scaling AI Sustainably. ... Latest News. Hardware. MSVP: Meta's first ASIC for video transcoding. May 18, 2023. Help Us Pioneer The Future of AI.
Figure 1: Modeling overview for the Apple foundation models. Pre-Training. Our foundation models are trained on Apple's AXLearn framework, an open-source project we released in 2023.It builds on top of JAX and XLA, and allows us to train the models with high efficiency and scalability on various training hardware and cloud platforms, including TPUs and both cloud and on-premise GPUs.
One of the latest tests is the Graduate-Level Google-Proof Q&A Benchmark (GPQA) 1, developed last year by a team including machine-learning researcher David Rein at New York University.
Zico Kolter, recently named director of CMU's Machine Learning Department. Elan Mizrahi. By Jake Dabkowski - Reporter, Pittsburgh Business Times. Jun 10, 2024. Listen to this article 3 min. Last ...
More information: Erkan Caner Ozkat et al, Machine learning driven optimization and parameter selection of multi-surface HTS Maglev, Physica C: Superconductivity and its Applications (2023). DOI ...
New research shows that having AI skills can now boost your salary up to 47%. Here are five AI certifications to upskill for high-income skills in 2024. ... Next up is the machine learning and AI ...
Machine learning enables new products, services and market opportunities. ... Editor's note: This article was updated in June 2024 to provide updated research figures and to improve the reader experience. Mary K. Pratt is an award-winning freelance journalist with a focus on covering enterprise IT, cybersecurity management and strategy. ...
Deep learning (DL) is one of the fastest-growing topics in materials data science, with rapidly emerging applications spanning atomistic, image-based, spectral, and textual data modalities. DL ...
Machine learning models are computer programs that are used to recognize patterns in data or make predictions. Machine learning models are created from machine learning algorithms, which undergo a training process using either labeled, unlabeled, or mixed data. Different machine learning algorithms are suited to different goals, such as ...
There is some good news, however. Medical advances are accelerating the battle against cancer. Here are 11 recent developments. Personalized cancer vaccines. Thousands of NHS cancer patients in England could soon access trials of a new vaccine treatment. It's designed to prime the immune system to target cancer cells and reduce recurrence risk.