Home / Guides / Citation Guides / How to Cite Sources

How to Cite Sources
Here is a complete list for how to cite sources. Most of these guides present citation guidance and examples in MLA, APA, and Chicago.
If you’re looking for general information on MLA or APA citations , the EasyBib Writing Center was designed for you! It has articles on what’s needed in an MLA in-text citation , how to format an APA paper, what an MLA annotated bibliography is, making an MLA works cited page, and much more!
MLA Format Citation Examples
The Modern Language Association created the MLA Style, currently in its 9th edition, to provide researchers with guidelines for writing and documenting scholarly borrowings. Most often used in the humanities, MLA style (or MLA format ) has been adopted and used by numerous other disciplines, in multiple parts of the world.
MLA provides standard rules to follow so that most research papers are formatted in a similar manner. This makes it easier for readers to comprehend the information. The MLA in-text citation guidelines, MLA works cited standards, and MLA annotated bibliography instructions provide scholars with the information they need to properly cite sources in their research papers, articles, and assignments.
- Book Chapter
- Conference Paper
- Documentary
- Encyclopedia
- Google Images
- Kindle Book
- Memorial Inscription
- Museum Exhibit
- Painting or Artwork
- PowerPoint Presentation
- Sheet Music
- Thesis or Dissertation
- YouTube Video
APA Format Citation Examples
The American Psychological Association created the APA citation style in 1929 as a way to help psychologists, anthropologists, and even business managers establish one common way to cite sources and present content.
APA is used when citing sources for academic articles such as journals, and is intended to help readers better comprehend content, and to avoid language bias wherever possible. The APA style (or APA format ) is now in its 7th edition, and provides citation style guides for virtually any type of resource.
Chicago Style Citation Examples
The Chicago/Turabian style of citing sources is generally used when citing sources for humanities papers, and is best known for its requirement that writers place bibliographic citations at the bottom of a page (in Chicago-format footnotes ) or at the end of a paper (endnotes).
The Turabian and Chicago citation styles are almost identical, but the Turabian style is geared towards student published papers such as theses and dissertations, while the Chicago style provides guidelines for all types of publications. This is why you’ll commonly see Chicago style and Turabian style presented together. The Chicago Manual of Style is currently in its 17th edition, and Turabian’s A Manual for Writers of Research Papers, Theses, and Dissertations is in its 8th edition.
Citing Specific Sources or Events
- Declaration of Independence
- Gettysburg Address
- Martin Luther King Jr. Speech
- President Obama’s Farewell Address
- President Trump’s Inauguration Speech
- White House Press Briefing
Additional FAQs
- Citing Archived Contributors
- Citing a Blog
- Citing a Book Chapter
- Citing a Source in a Foreign Language
- Citing an Image
- Citing a Song
- Citing Special Contributors
- Citing a Translated Article
- Citing a Tweet
6 Interesting Citation Facts
The world of citations may seem cut and dry, but there’s more to them than just specific capitalization rules, MLA in-text citations , and other formatting specifications. Citations have been helping researches document their sources for hundreds of years, and are a great way to learn more about a particular subject area.
Ever wonder what sets all the different styles apart, or how they came to be in the first place? Read on for some interesting facts about citations!
1. There are Over 7,000 Different Citation Styles
You may be familiar with MLA and APA citation styles, but there are actually thousands of citation styles used for all different academic disciplines all across the world. Deciding which one to use can be difficult, so be sure to ask you instructor which one you should be using for your next paper.
2. Some Citation Styles are Named After People
While a majority of citation styles are named for the specific organizations that publish them (i.e. APA is published by the American Psychological Association, and MLA format is named for the Modern Language Association), some are actually named after individuals. The most well-known example of this is perhaps Turabian style, named for Kate L. Turabian, an American educator and writer. She developed this style as a condensed version of the Chicago Manual of Style in order to present a more concise set of rules to students.
3. There are Some Really Specific and Uniquely Named Citation Styles
How specific can citation styles get? The answer is very. For example, the “Flavour and Fragrance Journal” style is based on a bimonthly, peer-reviewed scientific journal published since 1985 by John Wiley & Sons. It publishes original research articles, reviews and special reports on all aspects of flavor and fragrance. Another example is “Nordic Pulp and Paper Research,” a style used by an international scientific magazine covering science and technology for the areas of wood or bio-mass constituents.
4. More citations were created on EasyBib.com in the first quarter of 2018 than there are people in California.
The US Census Bureau estimates that approximately 39.5 million people live in the state of California. Meanwhile, about 43 million citations were made on EasyBib from January to March of 2018. That’s a lot of citations.
5. “Citations” is a Word With a Long History
The word “citations” can be traced back literally thousands of years to the Latin word “citare” meaning “to summon, urge, call; put in sudden motion, call forward; rouse, excite.” The word then took on its more modern meaning and relevance to writing papers in the 1600s, where it became known as the “act of citing or quoting a passage from a book, etc.”
6. Citation Styles are Always Changing
The concept of citations always stays the same. It is a means of preventing plagiarism and demonstrating where you relied on outside sources. The specific style rules, however, can and do change regularly. For example, in 2018 alone, 46 new citation styles were introduced , and 106 updates were made to exiting styles. At EasyBib, we are always on the lookout for ways to improve our styles and opportunities to add new ones to our list.

Why Citations Matter
Here are the ways accurate citations can help your students achieve academic success, and how you can answer the dreaded question, “why should I cite my sources?”
They Give Credit to the Right People
Citing their sources makes sure that the reader can differentiate the student’s original thoughts from those of other researchers. Not only does this make sure that the sources they use receive proper credit for their work, it ensures that the student receives deserved recognition for their unique contributions to the topic. Whether the student is citing in MLA format , APA format , or any other style, citations serve as a natural way to place a student’s work in the broader context of the subject area, and serve as an easy way to gauge their commitment to the project.
They Provide Hard Evidence of Ideas
Having many citations from a wide variety of sources related to their idea means that the student is working on a well-researched and respected subject. Citing sources that back up their claim creates room for fact-checking and further research . And, if they can cite a few sources that have the converse opinion or idea, and then demonstrate to the reader why they believe that that viewpoint is wrong by again citing credible sources, the student is well on their way to winning over the reader and cementing their point of view.
They Promote Originality and Prevent Plagiarism
The point of research projects is not to regurgitate information that can already be found elsewhere. We have Google for that! What the student’s project should aim to do is promote an original idea or a spin on an existing idea, and use reliable sources to promote that idea. Copying or directly referencing a source without proper citation can lead to not only a poor grade, but accusations of academic dishonesty. By citing their sources regularly and accurately, students can easily avoid the trap of plagiarism , and promote further research on their topic.
They Create Better Researchers
By researching sources to back up and promote their ideas, students are becoming better researchers without even knowing it! Each time a new source is read or researched, the student is becoming more engaged with the project and is developing a deeper understanding of the subject area. Proper citations demonstrate a breadth of the student’s reading and dedication to the project itself. By creating citations, students are compelled to make connections between their sources and discern research patterns. Each time they complete this process, they are helping themselves become better researchers and writers overall.
When is the Right Time to Start Making Citations?
Make in-text/parenthetical citations as you need them.
As you are writing your paper, be sure to include references within the text that correspond with references in a works cited or bibliography. These are usually called in-text citations or parenthetical citations in MLA and APA formats. The most effective time to complete these is directly after you have made your reference to another source. For instance, after writing the line from Charles Dickens’ A Tale of Two Cities : “It was the best of times, it was the worst of times…,” you would include a citation like this (depending on your chosen citation style):
(Dickens 11).
This signals to the reader that you have referenced an outside source. What’s great about this system is that the in-text citations serve as a natural list for all of the citations you have made in your paper, which will make completing the works cited page a whole lot easier. After you are done writing, all that will be left for you to do is scan your paper for these references, and then build a works cited page that includes a citation for each one.
Need help creating an MLA works cited page ? Try the MLA format generator on EasyBib.com! We also have a guide on how to format an APA reference page .
2. Understand the General Formatting Rules of Your Citation Style Before You Start Writing
While reading up on paper formatting may not sound exciting, being aware of how your paper should look early on in the paper writing process is super important. Citation styles can dictate more than just the appearance of the citations themselves, but rather can impact the layout of your paper as a whole, with specific guidelines concerning margin width, title treatment, and even font size and spacing. Knowing how to organize your paper before you start writing will ensure that you do not receive a low grade for something as trivial as forgetting a hanging indent.
Don’t know where to start? Here’s a formatting guide on APA format .
3. Double-check All of Your Outside Sources for Relevance and Trustworthiness First
Collecting outside sources that support your research and specific topic is a critical step in writing an effective paper. But before you run to the library and grab the first 20 books you can lay your hands on, keep in mind that selecting a source to include in your paper should not be taken lightly. Before you proceed with using it to backup your ideas, run a quick Internet search for it and see if other scholars in your field have written about it as well. Check to see if there are book reviews about it or peer accolades. If you spot something that seems off to you, you may want to consider leaving it out of your work. Doing this before your start making citations can save you a ton of time in the long run.
Finished with your paper? It may be time to run it through a grammar and plagiarism checker , like the one offered by EasyBib Plus. If you’re just looking to brush up on the basics, our grammar guides are ready anytime you are.
How useful was this post?
Click on a star to rate it!
We are sorry that this post was not useful for you!
Let us improve this post!
Tell us how we can improve this post?
Citation Basics
Harvard Referencing
Plagiarism Basics
Plagiarism Checker
Upload a paper to check for plagiarism against billions of sources and get advanced writing suggestions for clarity and style.
Get Started
Purdue Online Writing Lab Purdue OWL® College of Liberal Arts
APA Formatting and Style Guide (7th Edition)
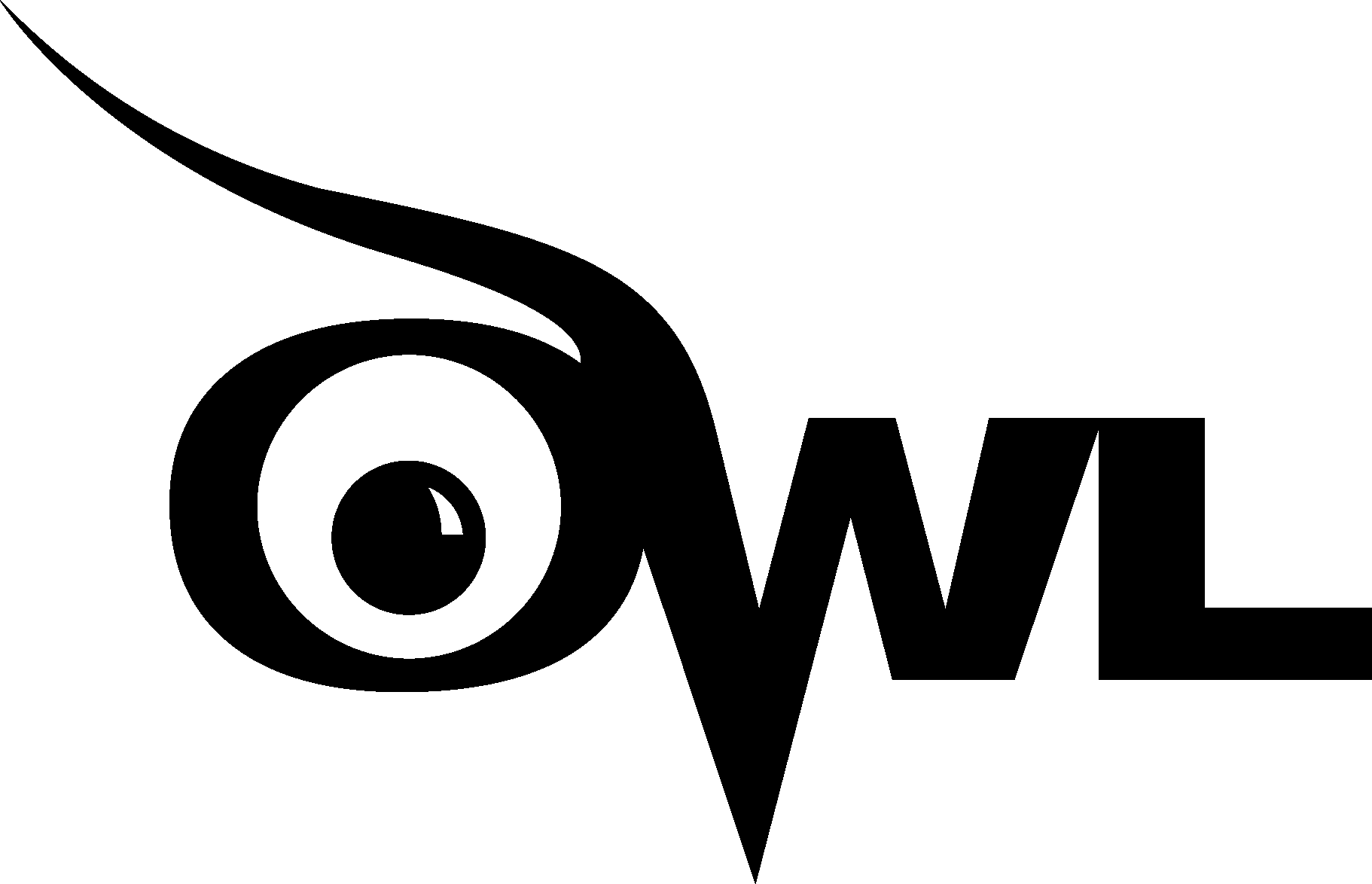
Welcome to the Purdue OWL
This page is brought to you by the OWL at Purdue University. When printing this page, you must include the entire legal notice.
Copyright ©1995-2018 by The Writing Lab & The OWL at Purdue and Purdue University. All rights reserved. This material may not be published, reproduced, broadcast, rewritten, or redistributed without permission. Use of this site constitutes acceptance of our terms and conditions of fair use.
In-Text Citations
Resources on using in-text citations in APA style
Reference List
Resources on writing an APA style reference list, including citation formats
Other APA Resources

IMAGES
VIDEO