An official website of the United States government
Official websites use .gov A .gov website belongs to an official government organization in the United States.
Secure .gov websites use HTTPS A lock ( Lock Locked padlock icon ) or https:// means you've safely connected to the .gov website. Share sensitive information only on official, secure websites.
- Publications
- Account settings
- Advanced Search
- Journal List


Limitations of COVID-19 testing and case data for evidence-informed health policy and practice
Elizabeth alvarez, iwona a bielska, stephanie hopkins, ahmed a belal, donna m goldstein, sureka pavalagantharajah, anna wynfield, shruthi dakey, marie-carmel gedeon, katrina bouzanis.
- Author information
- Article notes
- Copyright and License information
Corresponding author.
Received 2021 Mar 27; Accepted 2023 Jan 15; Collection date 2023.
Open Access This article is licensed under a Creative Commons Attribution 4.0 International License, which permits use, sharing, adaptation, distribution and reproduction in any medium or format, as long as you give appropriate credit to the original author(s) and the source, provide a link to the Creative Commons licence, and indicate if changes were made. The images or other third party material in this article are included in the article's Creative Commons licence, unless indicated otherwise in a credit line to the material. If material is not included in the article's Creative Commons licence and your intended use is not permitted by statutory regulation or exceeds the permitted use, you will need to obtain permission directly from the copyright holder. To view a copy of this licence, visit http://creativecommons.org/licenses/by/4.0/ . The Creative Commons Public Domain Dedication waiver ( http://creativecommons.org/publicdomain/zero/1.0/ ) applies to the data made available in this article, unless otherwise stated in a credit line to the data.
Coronavirus disease 2019 (COVID-19) became a pandemic within a matter of months. Analysing the first year of the pandemic, data and surveillance gaps have subsequently surfaced. Yet, policy decisions and public trust in their country’s strategies in combating COVID-19 rely on case numbers, death numbers and other unfamiliar metrics. There are many limitations on COVID-19 case counts internationally, which make cross-country comparisons of raw data and policy responses difficult.
Purpose and conclusions
This paper presents and describes steps in the testing and reporting process, with examples from a number of countries of barriers encountered in each step, all of which create an undercount of COVID-19 cases. This work raises factors to consider in COVID-19 data and provides recommendations to inform the current situation with COVID-19 as well as issues to be aware of in future pandemics.
Keywords: COVID-19, Testing, Epidemiology, Case count, Physical distancing policy
Since the emergence of coronavirus disease 2019 (COVID-19) in Wuhan, China, the world has faced serious data issues, ranging from a lack of transparency on the emergence, spread and nature of the virus to an absence of grounded comparative analyses, with temporal differences considered, about emerging social and economic challenges [ 1 , 2 ]. Most critically, scientists have lacked data to conduct analyses on non-pharmaceutical interventions (NPIs), including policies and strategies that governments have engaged to mitigate the situation, and how these have varied across regions, presumably affecting both short- and long-term outcomes [ 1 , 2 ].
Out of all the strategies implemented to date, physical distancing policies have emerged as one of the more effective NPIs to battle COVID-19 [ 3 , 4 ]. While physical distancing policies have been the mainstay in the battle against COVID-19, there has been a call to understand which forms of physical distancing policies are effective so that targeted and less disruptive measures can be taken in further waves of this pandemic and future pandemics [ 1 , 2 , 5 , 6 ]. The best time to institute physical distancing policies and what happens when and how they are eased remain unclear. There are many aspects of distancing, such as recommendations for maintaining a physical distance in public, banning group gatherings (the maximum number and where they take place), or complete lockdowns, that complicate their assessment. Timing and synergies of policies and sociodemographic and political factors play a role in the effectiveness of these policies [ 7 – 13 ]. Some hypothesized sociodemographic factors for increased exposure and severity of COVID-19 include living in a long-term care facility or being institutionalized, age (older), gender (mixed findings), having comorbidities (including high blood pressure, diabetes, obesity, immunocompromised status, tobacco smoking) and social vulnerabilities including race or ethnicity. Also relevant is the carrying capacity and infrastructure of health systems. These factors pose challenges for comparison among countries. Comparison is a prime requisite for evaluating the effectiveness of implementation of various policies between countries. Policymakers and the public have been using metrics such as number of cases, number of deaths and testing capacity to make policy or programme decisions or to decide whether to trust the actions of their governments, respectively.
An international team of researchers has been collecting data on physical distancing policies and contextual factors, such as health and political systems and demographics, to expedite knowledge translation (which means applying high-quality research evidence to processes of decision making) on the effect of policies and their influence on the epidemiology of COVID-19 [ 14 – 16 ]. Through this work, we identified gaps in the accuracy of reported numbers of COVID-19 cases and deaths, which make cross-country comparisons of the raw data, indexes using the raw data, and policy outcomes challenging [ 7 , 17 ]. While the work of this team is ongoing, this paper limits the findings from the inception of the pandemic to the end of 2020. It is important to understand the limitations of available COVID-19 data in order to properly inform decision making, especially at the outset as a novel infectious disease. This paper focuses on the testing and reporting cycle (Fig. 1 ) and provides examples from a number of countries of possible barriers leading to inaccurate data on reported COVID-19 cases. It also describes other cross-cutting implications of COVID-19 data for policy, practice and research, including reported deaths, missing information, implementation of policy, and unpredictable population behaviour. Furthermore, it calls into question analyses performed to date, which do not account for a number of known data gaps.
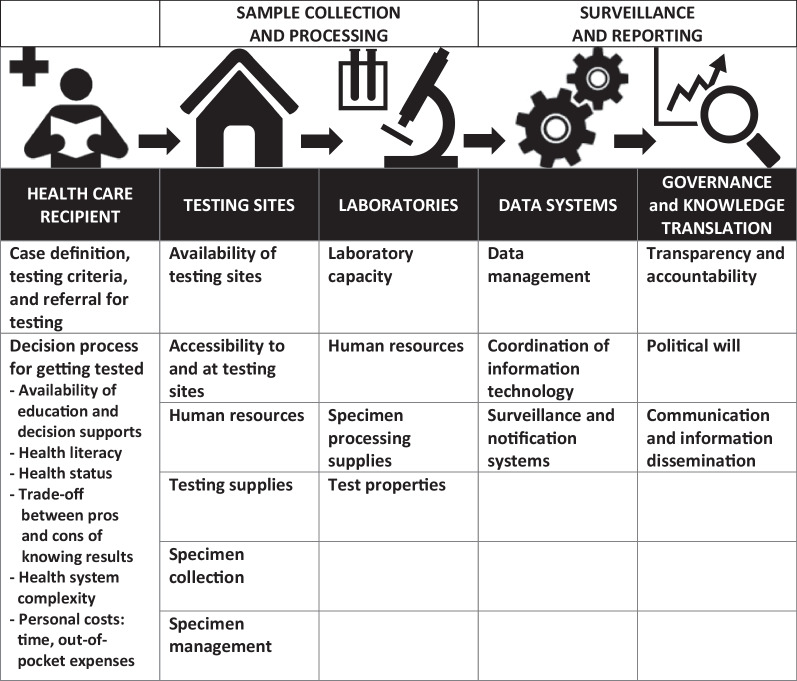
COVID-19 testing and reporting cycle. *The icons in this figure are in the public domain (Creative Commons CC0 1.0 Universal Public Domain) and were obtained from Wikimedia Commons at: https://commons.wikimedia.org/wiki/File:Medical_Library_-_The_Noun_Project.svg ; https://commons.wikimedia.org/wiki/File:Home_(85251)_-_The_Noun_Project.svg ; https://commons.wikimedia.org/wiki/File:Laboratory_-_The_Noun_Project.svg ; https://commons.wikimedia.org/wiki/File:Noun_project_1063.svg ; https://commons.wikimedia.org/wiki/File:Analysis_-_The_Noun_Project.svg
It is important to note that Fig. 1 only represents the testing and reporting cycle, which leads to counting of cases, and it does not include COVID-19 contact tracing and case management; however, we recognize that testing, contact tracing and case management are intricately linked to each other in the spread of COVID-19 [ 18 , 19 ]. As ‘Our World in Data’ states, “Without testing there is no data.” [ 20 ]. Understanding the links between testing, data and action underlies country responses to the pandemic. Ultimately, this work serves to provide a basis to improve pandemic planning, surveillance and reporting systems, and communications.
In Fig. 1 , the first level of testing is at the healthcare recipient level (Sect. “ Healthcare recipient level ”), followed by sample collection and processing (Sect. “ Sample collection and processing ”) and surveillance and reporting (Sect. “ Surveillance and reporting ”). Each level will be further explained below and examples provided as to potential or actual barriers at each level. These descriptions are not exhaustive, and nuanced understanding of the context will be needed to evaluate these steps and potential barriers in different settings.
Healthcare recipient level
Testing starts with individuals getting tested. There may be times when it is predetermined who gets tested and when, such as health workers getting tested prior to starting work in a long-term care facility or travellers returning from overseas [ 21 , 22 ]. However, most individuals are tested in the community, where a number of steps predicate individuals’ decisions to seek out testing. First, case definition, testing criteria and referral for testing influence our understanding of what the disease entity is and whether people are encouraged or discouraged to get tested. Given the novel status of COVID-19, there were challenges at the onset of this pandemic in establishing a working case definition. In China, arguably the leader in COVID-19 knowledge at the time, the case definition for reporting changed over time and between places [ 23 ]. These definitions were not always consistent with one another. Between 22 January and 12 February 2020, China’s National Health Commission had revised the COVID-19 outbreak response guidelines at least six times, resulting in significant differences in the daily counts due to changes over time in the definition of a case [ 24 ]. Adding to the uncertainty, the World Health Organization did not publish case definition guidelines until 16 April 2020, long after many countries had created their own working case definitions [ 25 ]. Although changes in methodology are expected as we learn more about the disease and as new variants emerge, these changes have implications for case counts [ 23 , 25 ]. Yet, communication does need to be flexible during a crisis. For example, little was known about asymptomatic COVID-19 spread at the beginning of the pandemic. As more evidence was garnered on this topic, information about precautions and testing criteria needed to be flexible to keep up with what was known [ 26 ].
Not only have case definitions changed over time, criteria for testing have changed over time and across jurisdictions on the basis of a number of factors, such as better understanding of the disease process, availability and capacity for testing, and national and local strategies for addressing the pandemic [ 27 ]. In some places, testing criteria were narrow, which discouraged people from getting tested because they did not fit the criteria. In its early response, Canada only tested symptomatic people returning from specific countries known to have high numbers of cases of COVID-19 [ 18 ]. Given that there were no treatments and media reported that the hospitals were overwhelmed, people were also discouraged from seeking medical attention unless they warranted hospitalization. If people were feeling unwell, but not needing to be on a ventilator, testing might not have been deemed necessary. Shifting testing criteria and differences in referral channels for testing, such as going through public health or needing a physician referral versus self-referrals, could create additional barriers.
Depending on the testing strategy, whether based on specific criteria or population-based, will make a difference for number of COVID-19 cases identified. Changes in criteria for testing sometimes led to increased demand without a corresponding increase in the availability of testing resources, which then led to delays in accessing tests [ 28 ]. Additionally, as different sectors, such as schools, resumed in-person activity, there was an increased demand for testing within certain population groups. Again, testing capacity could not always keep up with demand, leading, in some instances, to further limitations of who could be tested to prioritize resources for testing [ 27 ].
In the case that an individual has a choice to get tested, once a person is determined to be eligible for testing, that person has to decide whether or not to get tested, following a decisional process for getting tested, which can be affected by factors such as the availability of education and decision supports, health literacy, health status, trade-offs between knowing their results and potential economic and social consequences, health system complexity, and personal costs, such as time and out-of-pocket expenses [ 29 , 30 ]. Availability of education and decision support is needed for people to understand that there is a pandemic, what that means, how it might impact them, how and where to get tested (if available) and why getting tested is important for them or their loved ones. This relies on accurate and timely information, which is discussed in more detail in Sect. “ Governance and knowledge translation ”.
Furthermore, health literacy can involve a general understanding of factors that affect health or it can be specific to a disease entity, such as the virus that causes COVID-19. Health status can decrease the number of people seeking testing if they have mild symptoms and decide it is not worthwhile to seek testing or care, or they may not fit the testing criteria. On the other hand, some people with severe symptoms may not have the physical resources to go to a testing centre.
Of course, even individual-level factors are affected by broader systematic determinants of health. As the gravity of the pandemic took hold, jurisdictions began implementing more robust isolation policies to prevent the spread of COVID-19. These policies included self-isolation or a quarantine period for those who tested positive or who had come in contact with a known case. In many countries, governments provided economic relief to support people who were unable to work [ 31 , 32 ]. However, in countries such as Brazil and Mexico there were limited social safety nets, and in many other countries such as the USA, COVID-19 exposed gaps in these nets [ 33 , 34 ]. This created an economic barrier for people to access testing, as a positive test would force them to stay home without adequate financial means to survive. On 28 April 2020, the French Prime Minister, Edouard Philippe, urged the population of France to “protect-test-isolate”; meanwhile, containment measures generated a “disaffiliation process” among migrants and asylum seekers. Absence of work, isolation from French society, and fear of being checked by the police brought individuals into a “disaffiliation zone” marked by social non-existence, in a context of global health crisis [ 35 ].
Health systems themselves created a barrier to testing through their slow response to testing requests, causing some individuals to abandon testing [ 36 ]. In some countries, testing was expensive and not offered in the poorest communities [ 37 ]. For those travelling, mandatory testing, with varying requirements between different countries and potential out-of-pocket costs, increased the complexity of getting tested. Furthermore, competing crises may have lowered the number of people seeking testing due to other, more immediate, priorities, such as floods or wildfires [ 38 – 41 ].
Sample collection and processing
Once a person decides to seek testing, tests must be available and accessible and there must also be sufficient test processing centres. While these factors are often lumped together, it is important to distinguish these two steps in the testing cycle as they often require different structural and/or operational components.
Tests and testing sites
For an individual to get tested, there must be availability of testing sites and accessibility to these testing sites. Testing sites may include already available clinic, hospital or community sites, or assessment centres which are created for the purpose of testing. Having separate assessment centres can ease conflicting burdens on already overwhelmed health systems, and they can allow for efficiency in the process of testing and in keeping potentially infectious individuals separate from those who are seen for other ailments. Not only do testing sites have to be available, they have to be accessible. Times of operation, parking and other accessibility considerations are important. Testing sites can be centralized in one or several locations, where people have to find transportation to the sites, or can be mobile sites, which can increase access to those in rural/remote areas or those with mobility or transportation issues. Drive-thru testing has been showcased in countries such as South Korea [ 42 ]. However, limitations also exist with drive-thru sites for those who do not own a vehicle, or those who have to drive long distances or endure long wait times [ 43 ]. In areas with poor health system infrastructure, lack of access can exacerbate inequities in testing.
Operational components include the need for adequate human resources and testing supplies. In Ontario, Canada, assessment centres were slow to set up and there was a lack of swabs and other testing supplies [ 18 ]. In France, laboratories struggled to keep up with testing demands due to delays in receiving chemicals and testing kits produced abroad, given France’s reliance on global supply chains [ 44 ]. Bangladesh had a very limited number of case testing capacity in the beginning of the outbreak. The country conducted fewer than 3000 tests in the first four weeks of the outbreak between 8 March and 5 April 2020 for its 164 million population as well as 155,898 overseas passengers, some arriving from hard-hit countries such as Italy, allowing for community transmission [ 45 ].
The method of specimen collection and specimen management for processing are also important considerations. Specimen collection has varied between contexts and over time [ 46 ]. Nasopharyngeal, nasal and throat swabs have been used in community settings. Saliva tests and blood samples, mainly for hospitalized patients, are other methods of obtaining specimens. Each of these testing modalities has different properties, but none is 100% sensitive or able to pick up all positive cases of COVID-19. There are reports of very ill patients testing negative on multiple occasions on nasopharyngeal samples but subsequently testing positive from lung samples [ 47 , 48 ]. Specimen management requires the proper labelling, storage and transportation of samples from the testing site to the laboratory for processing.
Laboratories
Laboratory preparedness and laboratory capacity played crucial roles in COVID-19 testing globally [ 27 , 49 ]. Issues with this preparedness and capacity, along with lack of testing supplies, resulted in “lack of testing” as a prime factor for not having accurate numbers of COVID-19 cases, especially at the beginning of the pandemic. Laboratory capacity includes human resources and specimen processing supplies, often called the testing kits, which require specific reagents and equipment. Over time, countries with low laboratory preparedness focused on improving their testing capacities [ 49 ]. Since the start of the pandemic, Germany was touted as testing widely and therefore having a robust ability to contact trace in order to find people who may transmit the virus causing COVID-19. However, other countries struggled to get testing in place. In the USA, initial tests developed were invalid, which delayed the ability to distribute and complete tests [ 50 ]. This was further exacerbated by bureaucratic/institutional red tape which centralized testing to the Centers for Disease Control and Prevention (CDC) and prohibited local public health and commercial laboratories from developing or administering more effective tests [ 51 ]. Supply chain management issues for swabs, transport media and reagents slowed down early testing in multiple countries [ 27 ].
Once testing methods have been established, there are a number of tests available for COVID-19 [ 52 ]. Test properties include the sensitivity and specificity of a test, among others, and these can vary by test. Therefore, the type of test used can also influence case counts. Recent studies have highlighted the need to validate laboratory tests and share the results during a pandemic. Evidence from a study in Alberta, Canada suggested that variations in test sensitivity for the virus causing COVID-19, particularly earlier in a pandemic, can result in “an undercounting of cases by nearly a factor of two” (p. 398) [ 53 ]. With rapid tests and home-approved testing kits available during the course of the pandemic, testing properties can vary even more greatly [ 52 , 54 , 55 ].
Surveillance and reporting
Once individuals have been tested and the results are processed, surveillance and reporting systems must be in place to communicate that information back to individuals, public health officials or others involved in case management or treatment, and to politicians and other stakeholders to act on this information and prevent further spread.
Data systems
Data management refers to the inputting and tracking of data. However, because of the need to quickly and accurately inform the public and decision makers in the time of a crisis, coordination of information technology is needed to align all the various data management systems within a jurisdiction and internationally. For example, each hospital system, clinic or laboratory may have separate electronic medical record or data management systems. Not many countries maintain a common database system for COVID-19-related management (testing, response, etc.). Even if database management systems are in place, lack of trained professionals, serious lags in updating data, challenges with interdepartmental coordination among various task force members, and new innovations such as artificial intelligence, health tracking apps, telemedicine and big data, which are suddenly in place, can lead to disrupted transparency. An exception is China, which developed a highly responsive national notifiable disease reporting system (NNDRS) in the aftermath of severe acute respiratory syndrome (SARS) [ 56 , 57 ]. The United Nations Department of Economic and Social Affairs statistics division launched a common website for improving the data capacities of countries [ 58 ]. This information has to be further coordinated to create larger and more robust surveillance and notification systems. Robust surveillance systems help decision makers know what is happening locally or how a disease is moving through populations. Notification systems are needed for sharing information between the testing site, laboratory and public health or local health agencies for case management and contact tracing and for letting people know their test results in a timely manner to help prevent further spread. The COVID Tracking Project has highlighted many discrepancies in USA reporting and surveillance, demonstrating unreliability of the data [ 59 ]. For example, hospitals were required to change how COVID-19 data were relayed to the federal government, and the switch from reporting through the CDC to the Health and Human Services (HHS) system resulted in misreporting of data and administrative lags across several states. Countries’ national-level CDCs collect information from state and local sources. The time lag can hence be one of the reasons for misleading the overall comprehensive pandemic impact. Lastly, with rapid, point-of-care and home tests available, keeping track of positive cases may be even more difficult, and COVID-19 case counts could be even further artificially decreased [ 60 ]. These tests could make contact tracing even more difficult if there is a lack of disclosure from the user end. It is important to note that, while there are many available sites for international COVID-19 data comparisons, including John’s Hopkins COVID-19 Dashboard [ 61 ], Worldometer [ 62 ], Our World in Data [ 20 ] and the World Health Organization (WHO) COVID-19 Dashboard [ 63 ], these all rely on locally-acquired data for their reporting, and therefore fall into and potentially augment the same fallacies discussed in this paper.
Governance and knowledge translation
Even with robust surveillance and notification systems, transparency and accountability are important for informing decision makers and the public. Decision makers need to know what the health and laboratory systems are finding so that evidence-informed policy and practice decisions can be made for the public good. At the same time, trust in government and government responses rely in part on perceived transparency of government by the public [ 64 , 65 ]. Accountability spans all through the spectrum discussed in the testing and reporting cycle, in a whole-of-society approach. Individuals are accountable for knowing when to get tested, getting tested and following public health guidelines and other policies. The public health and healthcare systems are accountable for planning testing and sharing information. Decision makers are accountable for transparency in sharing information, communicating appropriately with the public and relevant stakeholders, and making decisions for those they represent. In parts of Russia, there were two separate reports for those who died from COVID-19 and those who were positive but died from other causes [ 25 ]. In Florida, state officials instructed medical examiners to remove causes of death in their lists [ 66 ]. In China, despite having a highly responsive national data surveillance and reporting system, at the beginning of the pandemic, cases were only reported to the system once they had been approved by local members of government who only allowed cases with a direct connection to the original source of the outbreak, the seafood market, to be recorded [ 67 ].
Political will has been shown to be a barrier or facilitator in the fight against COVID-19. Examples of good leadership and political will can be found in places like New Zealand, where decisions were made early on, implemented, supported and continued to be informed by emerging evidence, or as described, following “science and empathy”[ 68 ]. Poor leadership has also come through clearly during this pandemic. Tanzania, Iran, the USA, Brazil and Egypt are only a handful of countries demonstrating the impact of political will on the course of the pandemic, in some cases resulting from subversion and corruption. Communication in these countries was often not transparent or mixed, and accountability for the lack of decision making or poor decision making was limited or non-existent in the pandemic’s outset. Tanzania stopped reporting cases due to political optics [ 30 , 69 ]. Iran’s Health Ministry reported 14,405 deaths due to COVID-19 through July 2020, which was a significant discrepancy from the 42,000 deaths recorded through government records [ 70 ]. The number of cases was also almost double those reported, 451,024 as compared with 278,827. One main reason for releasing underestimated information about the cases was considered to be upcoming parliamentary elections [ 70 , 71 ]. The former president of the USA, Donald Trump, often flouted public health and healthcare expert advice [ 72 ]. The Washington Post reported that Brazil was testing 12 times fewer people than Iran and 32 times fewer people than the USA, and hospitalized patients and some healthcare professionals were not tested in an effort to lower the case numbers [ 73 ]. Hiding numbers of deaths from COVID-19, whether intentionally or inadvertently, shored up far-right supporters of Brazil’s President Bolsonaro at a time when he was facing possible charges of impeachment for corruption and helped bolster the President’s messaging that the pandemic was under control. This further enabled a large swath of the population to call for less strict rules around COVID-19 and a quick reopening of the economy. Similarly, in July 2020, it was reported that at least eight doctors and six journalists had been arrested because they criticized the Egyptian government’s response to the pandemic [ 74 ].
Lastly, communication and information dissemination link to every piece of this process. Why, when and how people seek testing, how and where to set up testing sites, supply chain management, setting up and managing data systems, and policy decision making all work in a cycle. Good communication between systems and dissemination of information to the public and relevant stakeholders is imperative during a crisis, such as the COVID-19 pandemic. The amount of information available and rapid change in information creates an infodemic problem. ‘Infodemic’ is a term used by the WHO in the context of COVID-19 and refers to informational problems, such as misinformation and fake news, that accompany the pandemic [ 75 ]. Addressing the infodemic issue was highlighted as one of the prominent factors needed to improve future global mitigation efforts [ 76 ]. A report published in the second week of April 2020 by the Reuters Institute for the Study of Journalism at the University of Oxford found that roughly one-third of social media users across the USA, as well as Argentina, Germany, South Korea, Spain and the UK, reported seeing false or misleading information about COVID-19 [ 77 ]. The presidents of Brazil and the USA were themselves sources of misinformation, as they were seen in public without masks and touting the benefits of hydroxychloroquine after it was largely known that harms outweighed benefits of its use [ 72 , 78 , 79 ]. Having clear public health communications, from trusted sources, and breaking down silos between systems could be helpful in combating ever-changing information during a pandemic.
Other implications of COVID-19 data for policy, practice and research
There are several cross-cutting issues separate, but related, to the testing and reporting cycle which arose during this work. These issues also affect COVID-19 case counts and optimal timing of policies: how deaths are reported, missing information, implementation of policies, and unpredictable population behaviour.
Reported deaths
Deaths from COVID-19 tend to occur weeks after infection; therefore, assessments of policy changes using death counts need to account for this timing. However, reported death counts from COVID-19 carry many similar limitations given lack of testing for those who are deceased, attributing cause of death to COVID-19-related complications, processes for declaring deaths and causes of deaths, and lack of transparency [ 80 ]. In Brazil, hospitalized patients were not being tested, and deaths were attributed to respiratory ailments [ 73 ]. Further, COVID-19 deaths from the City of Rio de Janeiro’s dashboard were blacked out for 4 days in May (22–26 May 2020) [ 81 ]. When the dashboard was restarted, the death count was artificially lowered by changing the cause of death from COVID-19 to its comorbidities. Additional changes included requiring a confirmed COVID-19 test at the time of death in order for the death certificate to list COVID-19 as the cause; however, the results of the test often came after the death certificates were issued [ 81 ]. In Italy, the reverse occurred where only those in hospital were counted as COVID-19 deaths, while many people died at home or in care homes without being tested [ 82 , 83 ]. In Ireland, early discrepancies in reported deaths were noted between official government figures and an increase in deaths noted on the website Rip.ie, which has served as a public forum disclosing deaths and wake information in line with Irish funeral traditions. Information from this forum was used to re-assess mortality and in some cases aid epidemiological modelling [ 84 ].
Missing information
Given the lack of access to treatments at the beginning of the pandemic, understanding who was at highest risk of obtaining or dying from COVID-19 was important to know in order to develop appropriate policies that balanced health with social and economic impacts of the pandemic. Early data showed a sex and age gradient for COVID-19 cases and deaths. However, not all countries report data by sex and/or age. Race/ethnicity and sociodemographic findings were not collected or reported early in the pandemic [ 85 ]. France has been criticized for laws which prohibit the collection of race and ethnicity data, since they lack data which demonstrate whether certain groups are overrepresented in COVID-19 cases and deaths [ 86 , 87 ]. Another aspect of missing data early in the pandemic was that of asymptomatic spread. Due to limited testing early in the pandemic, asymptomatic cases were not picked up. Population-based studies are being conducted to better understand the role of asymptomatic and pre-symptomatic spread of COVID-19 in different population groups, such as children [ 26 ].
Implementation of policy
Population-level strategies since the start of the pandemic and reported findings in the literature go hand and hand. Cause and effect are difficult to attribute. For example, early literature looking at the role of children on the spread of COVID-19 found that children played a small role. This was to be expected given that many schools around the world closed, and children would not be exposed through transportation and workplaces as adults would be. Therefore, family spread would naturally flow from adults to children given these circumstances. In addition, many places were not testing mild to asymptomatic cases, which were more commonly found in children. Publications early on related to the few severe COVID-19 cases in children or to school-related cases in places that had low community transmission rates of COVID-19 and were following public health guidelines [ 88 ]. Limitations of these data have been described, yet findings have been used to justify specific policies in places that were dissimilar, with expected results ensuing, such as an increase in community transmission and school closures due to COVID-19 infections [ 89 ]. Therefore, it is even more important to understand the context of policies before applying them to various jurisdictions.
Unpredictable population behaviour
There is a difference between stated policy, implementation and enforcement. To understand which policies worked to combat COVID-19, it is important to consider the level of compliance with stated policies. Some people may follow recommended approaches for protective actions while others may not comply and see these recommendations as problematic [ 90 ]. For example, people may change their behaviours in anticipation of an announced change; for example, individuals may start working from home even before it is enforced or if it is never officially mandated, or people may go on a shopping spree prior to known closures [ 91 , 92 ]. Of course, people’s behaviours may also be dependent on a disconnect between policy messages at different levels of government and exacerbated by rapid updates in a fast-moving pandemic of unknown properties and the associated information overload. Therefore, communication management and clarity are of utmost importance during a crisis.
Discussion and recommendations
The need for cross-country comparison is necessary for understanding the effectiveness of policies in various countries. Policy decisions are being made and judged on the basis of case numbers, deaths and testing, among others. Understanding the steps and barriers in testing and reporting data related to COVID-19 case numbers can help address the limitations of data to strengthen these systems for future pandemics and can also help in the interpretation of findings across jurisdictions. Robust and timely public health measures are needed to decrease the health, social and economic ramifications of the pandemic. Even with available vaccines, it will still take time to have sufficient population coverage internationally.
There are a few assumptions considered in this paper. First, we assume that the reported numbers for each country are not inflated. There could be some cases that are counted more than once if repeated tests are taken and the person continues to test positive. Most data do not disclose how often this occurs, but it is likely not a significant issue for population reports, at least at from the beginning of the pandemic [ 20 ]. Next, ideally COVID-19 case counts are accurate. This is the assumption that is made by policymakers and the public in judging their decisions and their outcomes. We argue that the reported COVID-19 data are likely an undercount of actual cases. The reasons are highlighted in this paper.
Future global discussions will continue around who is most affected by COVID-19 and how to best prepare for pandemics, among others. COVID-19 case and death counts will be used in determining successful approaches. It is important to understand the context of COVID-19 data in these discussions, especially with respect to other global indicators that may look to COVID-19 data, such as the Sustainable Development Goals (SDGs) through improvement of early warning, risk reduction and management of national and global health risks [ 93 ]. Specifically, SDG 3 (good health and wellbeing) with an emphasis on highlighting the lacunas in informed data tying policy and epidemiology, SDG 10 to reduce inequalities within and among countries, and SDG 16 (peace, justice and strong institutions) with a goal to build effective and accountable institutions at all levels. This research also contributes to the Sendai Framework for Disaster Risk Reduction, specifically priority 2, strengthening disaster risk governance to manage disaster risk, and priority 3, investing in disaster risk reduction for resilience [ 94 ]. Unfortunately, there is little published on good governance in reporting systems during COVID-19, and our findings in this area are limited to media and news sources. Future research could focus on this critical aspect.
Decision makers could consider the following overarching recommendations, contextualized to their individual jurisdictions (i.e. regional, country, province, territory, state), to evaluate the testing and reporting cycle and improve accuracy and comparability of COVID-19 data:
Understand barriers to accurate testing and reporting —This paper lays out the steps in the testing and reporting process and components of these steps. Barriers are described at each of these steps, and examples are provided.
Address barriers to testing and reporting —Understanding barriers in the testing and reporting process can uncover facilitators. Each setting will deal with different barriers. Ultimately, political will, capacity building and robust information systems will be needed to address any of these barriers.
Transparency and accountability for surveillance and reporting —Any attempt to assign causality to these policies must take into account the timing and quality of surveillance data. Data quality issues, such as completeness, accuracy, timeliness, reliability, relevance and consistency, are important for surveillance and reporting [ 95 , 96 ].
Invest in health system strengthening, including surveillance and all-hazards emergency response plans —COVID-19, as this and past pandemics have shown, is not just a health issue, and instead requires community, health systems, social systems and policy approaches to mitigate its effects. Preparing for infectious disease outbreaks and other crises needs to incorporate all-hazards emergency response plans in order to have all the necessary resources in place at the time of the events.
Identify promising communication strategies —Research is needed to understand how messages conveyed at all stages of a pandemic are received and understood at the micro-level and used by the public [ 97 ]. Development of communication strategies aimed at promoting good understanding of information may defer inappropriate behaviours.
Invest in research to further understand data reporting systems and policy strategies and implementation . Research could compare global COVID-19 data reporting platforms mentioned in this article to see from where they obtained their raw data to further understand data reporting accuracy and comparability of data over time and whether any limitations of data were noted. Further research could address what policy and implementation strategies worked in a variety of settings to strengthen future recommendations for emerging pandemics.
The use and effectiveness of government responses, specifically pertaining to physical distancing policies in the COVID-19 pandemic, has been evolving constantly. Testing is a measure of response performance and becomes a focal point during an infectious disease pandemic as all countries are faced with a similar situation. COVID-19 represents a unique opportunity to evaluate and measure success by countries to control its spread and address social and economic impacts of interventions. Understanding limitations of COVID-19 case counts by addressing factors related to testing and reporting will strengthen country responses to this and future pandemics and increase the reliability of knowledge gained by cross-country comparisons. Alarmingly, with COVID-19 having asymptomatic spread, lack of testing can discredit the efforts of an entire community, not to say an entire population.
Acknowledgements
The authors acknowledge Dr. Neil Abernethy’s contributions to the COVID-19 Policies and Epidemiology Working Group.
Abbreviations
Centers for Disease Control and Prevention
Coronavirus disease 2019
Health and Human Services
National notifiable disease reporting system
Non-pharmaceutical interventions
Severe acute respiratory syndrome
Sustainable Development Goals
World Health Organization
Author contributions
E.A. drafted the manuscript, and all other authors contributed to the content. All authors read and approved the final manuscript.
No funding was received for this manuscript.
Availability of data and materials
All data generated or analysed during this study are included in this published article.
Declarations
Ethics approval and consent to participate.
Not applicable as publicly available information was used.
Consent for publication
Not applicable as no individual-level data are used in this manuscript.
Competing interests
The authors declare that they have no competing interests.
Publisher’s Note
Springer Nature remains neutral with regard to jurisdictional claims in published maps and institutional affiliations.
- 1. Pueyo T. Coronavirus: the hammer and the dance. Medium. 2020. https://medium.com/@tomaspueyo/coronavirus-the-hammer-and-the-dance-be9337092b56 . Accessed 5 Sep 2020.
- 2. Ferguson N, Laydon D, Nedjati-Gilani G, et al. Report 9—impact of non-pharmaceutical interventions (NPIs) to reduce COVID-19 mortality and healthcare demand. WHO Collaborating Centre for Infectious Disease Modelling; MRC Centre for Global Infectious Disease Analysis; Abdul Latif Jameel Institute for Disease and Emergency Analytics; Imperial College London, UK; 2020. http://www.imperial.ac.uk/medicine/departments/school-public-health/infectious-disease-epidemiology/mrc-global-infectious-disease-analysis/covid-19/report-9-impact-of-npis-on-covid-19/ . Accessed 14 Dec 2020.
- 3. Chu DK, Akl EA, Duda S, Solo K, Yaacoub S, Schünemann HJ, et al. Physical distancing, face masks, and eye protection to prevent person-to-person transmission of SARS-CoV-2 and COVID-19: a systematic review and meta-analysis. Lancet. 2020;395(10242):1973–1987. doi: 10.1016/S0140-6736(20)31142-9. [ DOI ] [ PMC free article ] [ PubMed ] [ Google Scholar ]
- 4. Islam N, Sharp SJ, Chowell G, Shabnam S, Kawachi I, Lacey B, et al. Physical distancing interventions and incidence of coronavirus disease 2019: natural experiment in 149 countries. BMJ. 2020;15(370):m2743. doi: 10.1136/bmj.m2743. [ DOI ] [ PMC free article ] [ PubMed ] [ Google Scholar ]
- 5. WHO. Archived: WHO Timeline—COVID-19. https://www.who.int/news/item/27-04-2020-who-timeline---covid-19 . Accessed 14 Dec 2020.
- 6. Report of the WHO-China Joint Mission on Coronavirus Disease 2019 (COVID-19). WHO; 2020. https://www.who.int/docs/default-source/coronaviruse/who-china-joint-mission-on-covid-19-final-report.pdf . Accessed 7 May 2020.
- 7. Ho S. Breaking down the COVID-19 numbers: should we be comparing countries? CTV News. 2020. https://www.ctvnews.ca/health/coronavirus/breaking-down-the-covid-19-numbers-should-we-be-comparing-countries-1.4874552 . Accessed 14 Dec 2020.
- 8. D’Adamo H, Yoshikawa T, Ouslander JG. Coronavirus Disease 2019 in geriatrics and long-term care: the ABCDs of COVID-19. J Am Geriatr Soc. 2020;68(5):912–917. doi: 10.1111/jgs.16445. [ DOI ] [ PubMed ] [ Google Scholar ]
- 9. Kluge HHP. Statement—older people are at highest risk from COVID-19, but all must act to prevent community spread. WHO, Regional Office for Europe. 2020. https://www.euro.who.int/en/health-topics/health-emergencies/coronavirus-covid-19/statements/statement-older-people-are-at-highest-risk-from-covid-19,-but-all-must-act-to-prevent-community-spread . Accessed 14 Dec 2020.
- 10. Jin JM, Bai P, He W, Wu F, Liu XF, Han DM, et al. Gender differences in patients with COVID-19: focus on severity and mortality. Front Public Health. 2020 doi: 10.3389/fpubh.2020.00152/full. [ DOI ] [ PMC free article ] [ PubMed ] [ Google Scholar ]
- 11. Canadian Institutes of Health Research. Why sex and gender need to be considered in COVID-19 research—CIHR. Government of Canada. 2020. https://cihr-irsc.gc.ca/e/51939.html . Accessed 14 Dec 2020.
- 12. Gaynor TS, Wilson ME. Social vulnerability and equity: the disproportionate impact of COVID-19. Public Adm Rev. 2020;80(5):832–838. doi: 10.1111/puar.13264. [ DOI ] [ PMC free article ] [ PubMed ] [ Google Scholar ]
- 13. Karaye IM, Horney JA. The impact of social vulnerability on COVID-19 in the US: an analysis of spatially varying relationships. Am J Prev Med. 2020;59(3):317–325. doi: 10.1016/j.amepre.2020.06.006. [ DOI ] [ PMC free article ] [ PubMed ] [ Google Scholar ]
- 14. Alvarez E. Policy frameworks and impacts on the epidemiology of COVID-19. CONVERGE. 2020. https://converge.colorado.edu/resources/covid-19/working-groups/issues-impacts-recovery/policy-frameworks-and-impacts-on-the-epidemiology-of-covid-19 . Accessed 14 Dec 2020.
- 15. Alvarez E. Physical distancing policies and their effect on the epidemiology of COVID-19: a multi-national comparative study. World Pandemic Research Network. 2020. https://wprn.org/item/457852 . Accessed 14 Dec 2020.
- 16. COVID-19 Policies & Epidemiology Research Project. Home. https://covid19-policies.healthsci.mcmaster.ca/ . Accessed 20 Oct 2020.
- 17. CDC. Coronavirus Disease 2019 (COVID-19)—Transmission. Centers for Disease Control and Prevention. 2020. https://www.cdc.gov/coronavirus/2019-ncov/covid-data/faq-surveillance.html . Accessed 2 Sep 2020.
- 18. Crowe K. Why it’s so difficult to get tested for COVID-19 in Canada | CBC News. CBC News. 2020. https://www.cbc.ca/news/health/covid-testing-shortages-1.5503926 . Accessed 5 Sep 2020.
- 19. Confused about COVID-19 testing guidelines? Find out if you should get tested | CBC News. CBC News. 2020. https://www.cbc.ca/news/canada/toronto/covid-19-testing-ontario-1.5737683 . Accessed 14 Dec 2020.
- 20. Ritchie H, Ortiz-Ospina E, Beltekian D, Mathieu E, Hasell J, Macdonald B, et al. Coronavirus (COVID-19) Testing—our world in data. Our world in data. n.d. https://ourworldindata.org/coronavirus-testing . Accessed 14 Dec 2020. [ DOI ] [ PMC free article ] [ PubMed ]
- 21. Fox C. Workers at long-term care homes in COVID-19 hot spots will now be required to get tested weekly. CP24. 2020. https://www.cp24.com/news/workers-at-long-term-care-homes-in-covid-19-hot-spots-will-now-be-required-to-get-tested-weekly-1.5194380?cache=%3FclipId%3D89926%3FautoPlay%3DtrueSC . Accessed 8 Jan 2021.
- 22. Mzezewa T. Travel and coronavirus testing: your questions answered. The New York Times. 2020. https://www.nytimes.com/article/virus-covid-travel-questions.html . Accessed 8 Jan 2021.
- 23. Tsang TK, Wu P, Lin Y, Lau EHY, Leung GM, Cowling BJ. Effect of changing case definitions for COVID-19 on the epidemic curve and transmission parameters in mainland China: a modelling study. Lancet Public Health. 2020;5(5):e289–e296. doi: 10.1016/S2468-2667(20)30089-X. [ DOI ] [ PMC free article ] [ PubMed ] [ Google Scholar ]
- 24. Feuer W. “Confusion breeds distrust:” China keeps changing how it counts coronavirus cases. CNBC. 2020. https://www.cnbc.com/2020/02/26/confusion-breeds-distrust-china-keeps-changing-how-it-counts-coronavirus-cases.html . Accessed 14 Dec 2020.
- 25. Sauer P, Gershkovich E. Russia is boasting about low coronavirus deaths. The numbers are deceiving. The Moscow Times. 2020. https://www.themoscowtimes.com/2020/05/08/russia-is-boasting-about-low-coronavirus-deaths-the-numbers-are-deceiving-a70220 . Accessed 14 Dec 2020.
- 26. Gandhi M, Yokoe DS, Havlir DV. Asymptomatic transmission, the Achilles’ heel of current strategies to control COVID-19. N Engl J Med. 2020;382(22):2158–2160. doi: 10.1056/NEJMe2009758. [ DOI ] [ PMC free article ] [ PubMed ] [ Google Scholar ]
- 27. Pabbaraju K, Wong AA, Douesnard M, Ma R, Gill K, Dieu P, et al. A public health laboratory response to the pandemic. J Clin Microbiol. 2020;58(8):e01110–20. [ DOI ] [ PMC free article ] [ PubMed ]
- 28. Sherriff-Scott I. Ontario is changing how it tests for COVID. Here’s what we know so far. iPolitics. 2020. https://ipolitics.ca/2020/10/05/ontario-is-changing-how-it-tests-for-covid-heres-what-we-know-so-far/ . Accessed 16 Dec 2020.
- 29. Cousins S. Bangladesh’s COVID-19 testing criticised. Lancet. 2020;396(10251):591. doi: 10.1016/S0140-6736(20)31819-5. [ DOI ] [ PMC free article ] [ PubMed ] [ Google Scholar ]
- 30. Maclean R. COVID-19 outbreak in Nigeria is just one of Africa’s alarming hot spots. The New York Times. 2020. https://www.nytimes.com/2020/05/17/world/africa/coronavirus-kano-nigeria-hotspot.html . Accessed 30 Oct 2020.
- 31. Government of Singapore. Budget 2020. 2020. http://www.gov.sg/features/budget2020 . Accessed 8 Jan 2021.
- 32. Hapuarachchi P. President announces relief measures to the people amid COVID-19. 2020. https://www.newsfirst.lk/2020/03/23/president-grants-concessions-for-the-people-amid-covid-19/ . Accessed 8 Jan 2021.
- 33. Rauls L. COVID-19 is exposing the holes in Latin America’s safety nets. Americas Quarterly. 2020. https://americasquarterly.org/article/covid-19-is-exposing-the-holes-in-latin-americas-safety-nets/ . Accessed 8 Jan 2021.
- 34. Shafer P, Avila CJ. 4 ways COVID-19 has exposed gaps in the US social safety net. The Conversation. 2020. http://theconversation.com/4-ways-covid-19-has-exposed-gaps-in-the-us-social-safety-net-138233 . Accessed 16 Dec 2021.
- 35. Carillon S, Gosselin A, Coulibaly K, Ridde V, Desgrées du Loû A. Immigrants facing COVID 19 containment in France: an ordinary hardship of disaffiliation. J Migr Health. 2020;1–2:100032. doi: 10.1016/j.jmh.2020.100032. [ DOI ] [ PMC free article ] [ PubMed ] [ Google Scholar ]
- 36. Charbonneau D. Ottawa residents frustrated with multi-day wait for COVID-19 test results. CTV News. 2020. https://ottawa.ctvnews.ca/ottawa-residents-frustrated-with-multi-day-wait-for-covid-19-test-results-1.5122365 . Accessed 14 Dec 2020.
- 37. Faust L, Zimmer AJ, Kohli M, Saha S, Boffa J, Bayot ML, et al. SARS-CoV-2 testing in low- and middle-income countries: availability and affordability in the private health sector. Microbes Infect. 2020;22(10):511–514. doi: 10.1016/j.micinf.2020.10.005. [ DOI ] [ PMC free article ] [ PubMed ] [ Google Scholar ]
- 38. FloodList. India—over 60 dead after more rain and floods hit Telangana—FloodList. 2020. http://floodlist.com/asia/india-floods-telangana-october-2020 . Accessed 15 Dec 2020.
- 39. Al Jazeera. India: cyclone Nivar makes landfall bringing rains, flood | Weather News. 2020. https://www.aljazeera.com/news/2020/11/26/cyclone-nivar-makes-landfall-bringing-rains-flood . Accessed 15 Dec 2020.
- 40. Niles N, Hauck G, Aretakis R, Shannon J. Tropical storm sally: hurricane watch, evacuations for New Orleans; storm expected to strengthen. USA TODAY. 2020. https://www.usatoday.com/story/news/nation/2020/09/12/tropical-storm-update-florida-gulf-path-paulette-path-bermuda/5778321002/ . Accessed 15 Dec 2020.
- 41. Flaccus G, Cline S. Wildfires raging across Oregon, Washington may cause historic destruction: officials. Global News. 2020. https://globalnews.ca/news/7325865/oregon-washington-wildfires/ . Accessed 15 Dec 2020.
- 42. Watson I, Jeong S. South Korea pioneers coronavirus drive-through testing station—CNN. CNN . 2020. https://www.cnn.com/2020/03/02/asia/coronavirus-drive-through-south-korea-hnk-intl/index.html . Accessed 15 Dec 2020.
- 43. Herhalt C. “This is torture”: Ontario mom waits 6.5 hours for COVID-19 test. CTV News. 2020. https://toronto.ctvnews.ca/this-is-torture-ontario-mom-waits-6-5-hours-for-covid-19-test-1.5109045 . Accessed 15 Dec 2020.
- 44. Lough R. French labs show how global supply bottlenecks thwart effort to ramp up testing. Reuters. 2020. https://www.reuters.com/article/us-health-coronavirus-france-reagents-idINKCN26G2EP . Accessed 8 Jan 2021.
- 45. Huq S, Biswas RK. COVID-19 in Bangladesh: data deficiency to delayed decision. J Glob Health. 2020;10(1):010342. doi: 10.7189/jogh.10.010342. [ DOI ] [ PMC free article ] [ PubMed ] [ Google Scholar ]
- 46. Alberta Precision Laboratories. Major changes in COVID-19 specimen collection recommendations. 2020. https://www.albertahealthservices.ca/assets/wf/lab/wf-lab-bulletin-major-changes-in-covid-19-specimen-collection-recommendations.pdf .
- 47. Ramos KJ, Kapnadak SG, Collins BF, Pottinger PS, Wall R, Mays JA, et al. Detection of SARS-CoV-2 by bronchoscopy after negative nasopharyngeal testing: stay vigilant for COVID-19. Respir Med Case Rep. 2020;30:101120. doi: 10.1016/j.rmcr.2020.101120. [ DOI ] [ PMC free article ] [ PubMed ] [ Google Scholar ]
- 48. Chen LD, Li H, Ye YM, Wu Z, Huang YP, Zhang WL, et al. A COVID-19 patient with multiple negative results for PCR assays outside Wuhan, China: a case report. BMC Infect Dis. 2020;20(1):517. doi: 10.1186/s12879-020-05245-7. [ DOI ] [ PMC free article ] [ PubMed ] [ Google Scholar ]
- 49. Gupta N, Potdar V, Praharaj I, Giri S, Sapkal G, Yadav P, et al. Laboratory preparedness for SARS-CoV-2 testing in India: harnessing a network of virus research & diagnostic laboratories. Indian J Med Res. 2020;151(2 & 3):216–225. doi: 10.4103/ijmr.IJMR_594_20. [ DOI ] [ PMC free article ] [ PubMed ] [ Google Scholar ]
- 50. Johnson CY, McGinley L. What went wrong with the coronavirus tests in the U.S. Washington Post. https://www.washingtonpost.com/health/what-went-wrong-with-the-coronavirus-tests/2020/03/07/915f5dea-5d82-11ea-b29b-9db42f7803a7_story.html . Accessed 5 Sep 2020.
- 51. Resnick B, Scott D. America’s shamefully slow coronavirus testing threatens all of us . Vox. 2020. https://www.vox.com/science-and-health/2020/3/12/21175034/coronavirus-covid-19-testing-usa . Accessed 14 Dec 2020.
- 52. Health Canada. Authorized medical devices for uses related to COVID-19: List of authorized testing devices. AEM. 2020. https://www.canada.ca/en/health-canada/services/drugs-health-products/covid19-industry/medical-devices/authorized/list.html . Accessed 15 Dec 2020.
- 53. Burstyn I, Goldstein ND, Gustafson P. It can be dangerous to take epidemic curves of COVID-19 at face value. Can J Public Health. 2020;111(3):397–400. doi: 10.17269/s41997-020-00367-6. [ DOI ] [ PMC free article ] [ PubMed ] [ Google Scholar ]
- 54. Bachelet VC. Do we know the diagnostic properties of the tests used in COVID-19? A rapid review of recently published literature. Medwave. 2020;20(3):e7890. doi: 10.5867/medwave.2020.03.7891. [ DOI ] [ PubMed ] [ Google Scholar ]
- 55. Mina MJ, Parker R, Larremore DB. Rethinking COVID-19 test sensitivity— a strategy for containment. N Engl J Med. 2020;383(22):e120. doi: 10.1056/NEJMp2025631. [ DOI ] [ PubMed ] [ Google Scholar ]
- 56. Jia P, Yang S. China needs a national intelligent syndromic surveillance system. Nat Med. 2020;26(7):990–990. doi: 10.1038/s41591-020-0921-5. [ DOI ] [ PubMed ] [ Google Scholar ]
- 57. Jia P, Yang S. Early warning of epidemics: towards a national intelligent syndromic surveillance system (NISSS) in China. BMJ Glob Health. 2020;5(10):e002925. doi: 10.1136/bmjgh-2020-002925. [ DOI ] [ PMC free article ] [ PubMed ] [ Google Scholar ]
- 58. United Nations Department of Economic and Social Affairs. Statistics, COVID-19 response. https://covid-19-response.unstatshub.org/https:/covid-19-response.unstatshub.org/ . Accessed 20 Oct 2022.
- 59. The Atlantic Monthly Group. The COVID tracking project. The COVID tracking project. https://covidtracking.com/ . Accessed 21 Oct 2021.
- 60. Erdman SL. FDA authorizes first rapid COVID-19 self-testing kit for at-home diagnosis. CNN. https://www.cnn.com/2020/11/18/health/covid-home-self-test/index.html . Accessed 15 Dec 2020.
- 61. Johns Hopkins Coronavirus Resource Center. COVID-19 Map. https://coronavirus.jhu.edu/map.html . Accessed 20 Oct 2022.
- 62. Worldometer. COVID Live—Coronavirus Statistics. https://www.worldometers.info/coronavirus/ . Accessed 20 Oct 2022.
- 63. World Health Organization. WHO Coronavirus (COVID-19) Dashboard. https://covid19.who.int . Accessed 20 Oct 2022.
- 64. Enria L, Waterlow N, Rogers NT, Brindle H, Lal S, Eggo RM, et al. Trust and transparency in times of crisis: results from an online survey during the first wave (April 2020) of the COVID-19 epidemic in the UK. PLoS ONE. 2021;16(2):e0239247. doi: 10.1371/journal.pone.0239247. [ DOI ] [ PMC free article ] [ PubMed ] [ Google Scholar ]
- 65. OECD. Transparency, communication and trust: The role of public communication in responding to the wave of disinformation about the new Coronavirus. OECD. https://www.oecd.org/coronavirus/policy-responses/transparency-communication-and-trust-the-role-of-public-communication-in-responding-to-the-wave-of-disinformation-about-the-new-coronavirus-bef7ad6e/ . Accessed 21 Oct 2022.
- 66. McGrory K, Wollington R. Florida medical examiners were releasing coronavirus death data. The state made them stop. Tampa Bay Times. 2020. https://www.tampabay.com/news/health/2020/04/29/florida-medical-examiners-were-releasing-coronavirus-death-data-the-state-made-them-stop/ . Accessed 14 Dec 2020.
- 67. Myers SL. China created a fail-safe system to track contagions. It failed. The New York Times. 2020. https://www.nytimes.com/2020/03/29/world/asia/coronavirus-china.html . Accessed 16 Dec 2020.
- 68. Coronavirus: how New Zealand relied on science and empathy—BBC News. BBC News. 2020. https://www.bbc.com/news/world-asia-52344299 . Accessed 15 Dec 2020.
- 69. Wasike A. Tanzanian president claims “country free of COVID-19”. Anadolu Agency. 2020. https://www.aa.com.tr/en/africa/tanzanian-president-claims-country-free-of-covid-19/1869961 . Accessed 30 Oct 2020.
- 70. Coronavirus: Iran cover-up of deaths revealed by data leak. BBC News. 2020. https://www.bbc.com/news/world-middle-east-53598965 . Accessed 15 Dec 2020.
- 71. Hosseini K. Is Iran covering up its outbreak? BBC News. 2020. https://www.bbc.com/news/world-middle-east-51930856 . Accessed 15 Dec 2020.
- 72. Pazzanesse C. Calculating possible fallout of Trump’s face mask remarks. Harvard Gazette. 2020. https://news.harvard.edu/gazette/story/2020/10/possible-fallout-from-trumps-dismissal-of-face-masks/ . Accessed 15 Dec 2020.
- 73. McCoy T, Traiano H. Limits on coronavirus testing in Brazil are hiding the true dimensions of Latin America’s largest outbreak. Washington Post. https://www.washingtonpost.com/world/the_americas/coronavirus-brazil-testing-bolsonaro-cemetery-gravedigger/2020/04/22/fe757ee4-83cc-11ea-878a-86477a724bdb_story.html . Accessed 14 Dec 2020.
- 74. Dyer O. Covid-19: at least eight doctors in Egypt arrested for criticising government response. BMJ. 2020;370:m2850. doi: 10.1136/bmj.m2850. [ DOI ] [ PubMed ] [ Google Scholar ]
- 75. Joint statement by WHO, UN, UNICEF, UNDP, UNESCO, UNAIDS, ITU, UN Global Pulse, and IFRC. Managing the COVID-19 infodemic: Promoting healthy behaviours and mitigating the harm from misinformation and disinformation. WHO. https://www.who.int/news/item/23-09-2020-managing-the-covid-19-infodemic-promoting-healthy-behaviours-and-mitigating-the-harm-from-misinformation-and-disinformation . Accessed 30 Oct 2020.
- 76. Hua J, Shaw R. Corona Virus (COVID-19) “Infodemic” and emerging issues through a data lens: the case of China. Int J Environ Res Public Health. 2020;17(7):2309. [ DOI ] [ PMC free article ] [ PubMed ]
- 77. Nielsen RK, Fletcher R, Newman N, Brennen JS, Howard PN. Navigating the ‘Infodemic’: how people in six countries access and rate news and information about coronavirus. Reuters Institute for the Study of Journalism as part of the Oxford Martin Programme on Misinformation, Science and Media, a three-year research collaboration between the Reuters Institute, the Oxford Internet Institute, and the Oxford Martin School; 2020.
- 78. Gittleson B, Phelps J, Cathey L. Trump doubles down on defense of hydroxychloroquine to treat COVID-19 despite efficacy concerns. ABC News. 2020. https://abcnews.go.com/Politics/trump-doubles-defense-hydroxychloroquine-treat-covid-19-efficacy/story?id=72039824 . Accessed 15 Dec 2020.
- 79. De Sousa M, Biller D. Brazil’s president, infected with virus, touts malaria drug. CTVNews. 2020. https://www.ctvnews.ca/world/brazil-s-president-infected-with-virus-touts-malaria-drug-1.5015608 . Accessed 15 Dec 2020.
- 80. COVID-19 INED. Key issues. Ined—Institut national d’études démographiques. n.d. https://dc-covid.site.ined.fr/en/presentation/ . Accessed 14 Dec 2020.
- 81. Lima T. Following Data Blackout, Rio City Government’s “Updated” Covid-19 dashboard excludes 40% of deaths. RioOnWatch. 2020. https://www.rioonwatch.org/?p=59883 . Accessed 15 Dec 2020.
- 82. Ciminelli G, Garcia-Mandicó S. COVID-19 in Italy: an analysis of death registry data. J Public Health Oxf Engl. 2020;42(4):723–730. doi: 10.1093/pubmed/fdaa165. [ DOI ] [ PMC free article ] [ PubMed ] [ Google Scholar ]
- 83. Italy’s coronavirus death toll is likely much higher: “Most deaths simply aren’t counted.” CBS News . 2020. https://www.cbsnews.com/news/italy-coronavirus-deaths-likely-much-higher/ . Accessed 15 Dec 2020.
- 84. McCarthy G, Dempsey R, Parnell A, MacCarron. Just a “bad flu”? How death notices debunk that Covid-19 myth. 2020. https://www.rte.ie/brainstorm/2020/0928/1167878-covid-19-rip-death-notices-bad-flu-excess-mortality/ . Accessed 10 Jan 2021.
- 85. Osman L. Canada still only considering gathering race-based COVID-19 data. CTV News . 2020. https://www.ctvnews.ca/health/coronavirus/canada-still-only-considering-gathering-race-based-covid-19-data-1.4927648 . Accessed 15 Dec 2020.
- 86. Gilbert J, Keane D. How French law makes minorities invisible. The Independent . 2016. https://www.independent.co.uk/news/world/politics/how-french-law-makes-minorities-invisible-a7416656.html . Accessed 15 Dec 2020.
- 87. Timsit A. France’s data collection rules obscure the racial disparities of COVID-19. Quartz. 2020. https://qz.com/1864274/france-doesnt-track-how-race-affects-covid-19-outcomes/ . Accessed 15 Dec 2020.
- 88. Vogel L. Have we misjudged the role of children in spreading COVID-19? CMAJ. 2020;192(38):E1102–E1103. doi: 10.1503/cmaj.1095897. [ DOI ] [ PMC free article ] [ PubMed ] [ Google Scholar ]
- 89. Aguilar B. TDSB closes six more schools because of COVID-19. Toronto. 2020. https://toronto.ctvnews.ca/tdsb-closes-six-more-schools-because-of-covid-19-1.5229597 . Accessed 15 Dec 2020.
- 90. Kleitman S, Fullerton DJ, Zhang LM, Blanchard MD, Lee J, Stankov L, et al. To comply or not comply? A latent profile analysis of behaviours and attitudes during the COVID-19 pandemic. PLoS ONE. 2021;16(7):e0255268. doi: 10.1371/journal.pone.0255268. [ DOI ] [ PMC free article ] [ PubMed ] [ Google Scholar ]
- 91. Savage M. Could the Swedish lifestyle help fight coronavirus? BBC . 2020. https://www.bbc.com/worklife/article/20200328-how-to-self-isolate-what-we-can-learn-from-sweden . Accessed 15 Dec 2020.
- 92. Green K. Worried about possible lockdown, Alberta shoppers flood the stores. CTV News . 2020. https://calgary.ctvnews.ca/worried-about-possible-lockdown-alberta-shoppers-flood-the-stores-1.5201682 . Accessed 15 Dec 2020.
- 93. Department of Economic and Social Affairs. The 17 GOALS | sustainable development. United Nations. n.d. https://sdgs.un.org/goals . Accessed 14 Dec 2020.
- 94. United Nations Office for Disaster Risk Reduction. Sendai Framework for Disaster Risk Reduction 2015–2030. 2015. https://www.undrr.org/publication/sendai-framework-disaster-risk-reduction-2015-2030 . Accessed 14 Dec 2020.
- 95. Chen H, Hailey D, Wang N, Yu P. A review of data quality assessment methods for public health information systems. Int J Environ Res Public Health. 2014;11(5):5170–5207. doi: 10.3390/ijerph110505170. [ DOI ] [ PMC free article ] [ PubMed ] [ Google Scholar ]
- 96. Rajan D, Koch K, Rohrer K, Bajnoczki C, Socha A, Voss M, et al. Governance of the Covid-19 response: a call for more inclusive and transparent decision-making. BMJ Glob Health. 2020;5(5):e002655. [ DOI ] [ PMC free article ] [ PubMed ]
- 97. Sherbrooke U de. Study by the Université de Sherbrooke on the psychosocial impacts of the pandemic. https://www.newswire.ca/news-releases/study-by-the-universite-de-sherbrooke-on-the-psychosocial-impacts-of-the-pandemic-886218205.html . Accessed 30 Jan 2021.
Associated Data
This section collects any data citations, data availability statements, or supplementary materials included in this article.
Data Availability Statement
- View on publisher site
- PDF (1.1 MB)
- Collections
Similar articles
Cited by other articles, links to ncbi databases.
- Download .nbib .nbib
- Format: AMA APA MLA NLM
Add to Collections
Thank you for visiting nature.com. You are using a browser version with limited support for CSS. To obtain the best experience, we recommend you use a more up to date browser (or turn off compatibility mode in Internet Explorer). In the meantime, to ensure continued support, we are displaying the site without styles and JavaScript.
- View all journals
- My Account Login
- Explore content
- About the journal
- Publish with us
- Sign up for alerts
- Open access
- Published: 11 February 2021
Methodological quality of COVID-19 clinical research
- Richard G. Jung ORCID: orcid.org/0000-0002-8570-6736 1 , 2 , 3 na1 ,
- Pietro Di Santo 1 , 2 , 4 , 5 na1 ,
- Cole Clifford 6 ,
- Graeme Prosperi-Porta 7 ,
- Stephanie Skanes 6 ,
- Annie Hung 8 ,
- Simon Parlow 4 ,
- Sarah Visintini ORCID: orcid.org/0000-0001-6966-1753 9 ,
- F. Daniel Ramirez ORCID: orcid.org/0000-0002-4350-1652 1 , 4 , 10 , 11 ,
- Trevor Simard 1 , 2 , 3 , 4 , 12 &
- Benjamin Hibbert ORCID: orcid.org/0000-0003-0906-1363 2 , 3 , 4
Nature Communications volume 12 , Article number: 943 ( 2021 ) Cite this article
14k Accesses
96 Citations
234 Altmetric
Metrics details
- Infectious diseases
- Public health
The COVID-19 pandemic began in early 2020 with major health consequences. While a need to disseminate information to the medical community and general public was paramount, concerns have been raised regarding the scientific rigor in published reports. We performed a systematic review to evaluate the methodological quality of currently available COVID-19 studies compared to historical controls. A total of 9895 titles and abstracts were screened and 686 COVID-19 articles were included in the final analysis. Comparative analysis of COVID-19 to historical articles reveals a shorter time to acceptance (13.0[IQR, 5.0–25.0] days vs. 110.0[IQR, 71.0–156.0] days in COVID-19 and control articles, respectively; p < 0.0001). Furthermore, methodological quality scores are lower in COVID-19 articles across all study designs. COVID-19 clinical studies have a shorter time to publication and have lower methodological quality scores than control studies in the same journal. These studies should be revisited with the emergence of stronger evidence.
Similar content being viewed by others
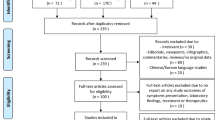
Clinical presentations, laboratory and radiological findings, and treatments for 11,028 COVID-19 patients: a systematic review and meta-analysis
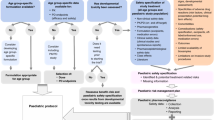
Improving clinical paediatric research and learning from COVID-19: recommendations by the Conect4Children expert advice group
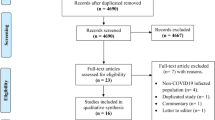
The effect of influenza vaccine in reducing the severity of clinical outcomes in patients with COVID-19: a systematic review and meta-analysis
Introduction.
The severe acute respiratory syndrome coronavirus 2 (SARS-CoV-2) pandemic spread globally in early 2020 with substantial health and economic consequences. This was associated with an exponential increase in scientific publications related to the coronavirus disease 2019 (COVID-19) in order to rapidly elucidate the natural history and identify diagnostic and therapeutic tools 1 .
While a need to rapidly disseminate information to the medical community, governmental agencies, and general public was paramount—major concerns have been raised regarding the scientific rigor in the literature 2 . Poorly conducted studies may originate from failure at any of the four consecutive research stages: (1) choice of research question relevant to patient care, (2) quality of research design 3 , (3) adequacy of publication, and (4) quality of research reports. Furthermore, evidence-based medicine relies on a hierarchy of evidence, ranging from the highest level of randomized controlled trials (RCT) to the lowest level of case series and case reports 4 .
Given the implications for clinical care, policy decision making, and concerns regarding methodological and peer-review standards for COVID-19 research 5 , we performed a formal evaluation of the methodological quality of published COVID-19 literature. Specifically, we undertook a systematic review to identify COVID-19 clinical literature and matched them to historical controls to formally evaluate the following: (1) the methodological quality of COVID-19 studies using established quality tools and checklists, (2) the methodological quality of COVID-19 studies, stratified by median time to acceptance, geographical regions, and journal impact factor and (3) a comparison of COVID-19 methodological quality to matched controls.
Herein, we show that COVID-19 articles are associated with lower methodological quality scores. Moreover, in a matched cohort analysis with control articles from the same journal, we reveal that COVID-19 articles are associated with lower quality scores and shorter time from submission to acceptance. Ultimately, COVID-19 clinical studies should be revisited with the emergence of stronger evidence.
Article selection
A total of 14787 COVID-19 papers were identified as of May 14, 2020 and 4892 duplicate articles were removed. In total, 9895 titles and abstracts were screened, and 9101 articles were excluded due to the study being pre-clinical in nature, case report, case series <5 patients, in a language other than English, reviews (including systematic reviews), study protocols or methods, and other coronavirus variants with an overall inter-rater study inclusion agreement of 96.7% ( κ = 0.81; 95% CI, 0.79–0.83). A total number of 794 full texts were reviewed for eligibility. Over 108 articles were excluded for ineligible study design or publication type (such as letter to the editors, editorials, case reports or case series <5 patients), wrong patient population, non-English language, duplicate articles, wrong outcomes and publication in a non-peer-reviewed journal. Ultimately, 686 articles were identified with an inter-rater agreement of 86.5% ( κ = 0.68; 95% CI, 0.67–0.70) (Fig. 1 ).
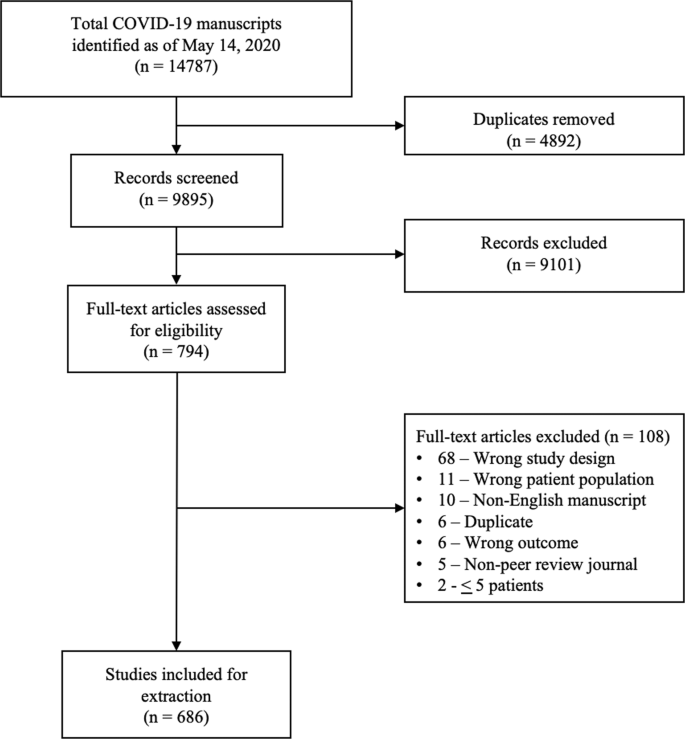
A total of 14787 articles were identified and 4892 duplicate articles were removed. Overall, 9895 articles were screened by title and abstract leaving 794 articles for full-text screening. Over 108 articles were excluded, leaving a total of 686 articles that underwent methodological quality assessment.
COVID-19 literature methodological quality
Most studies originated from Asia/Oceania with 469 (68.4%) studies followed by Europe with 139 (20.3%) studies, and the Americas with 78 (11.4%) studies. Of included studies, 380 (55.4%) were case series, 199 (29.0%) were cohort, 63 (9.2%) were diagnostic, 38 (5.5%) were case–control, and 6 (0.9%) were RCTs. Most studies (590, 86.0%) were retrospective in nature, 620 (90.4%) reported the sex of patients, and 7 (2.3%) studies excluding case series calculated their sample size a priori. The method of SARS-CoV-2 diagnosis was reported in 558 studies (81.3%) and ethics approval was obtained in 556 studies (81.0%). Finally, journal impact factor of COVID-19 manuscripts was 4.7 (IQR, 2.9–7.6) with a time to acceptance of 13.0 (IQR, 5.0–25.0) days (Table 1 ).
Overall, when COVID-19 articles were stratified by study design, a mean case series score (out of 5) (SD) of 3.3 (1.1), mean NOS cohort study score (out of 8) of 5.8 (1.5), mean NOS case–control study score (out of 8) of 5.5 (1.9), and low bias present in 4 (6.4%) diagnostic studies was observed (Table 2 and Fig. 2 ). Furthermore, in the 6 RCTs in the COVID-19 literature, there was a high risk of bias with little consideration for sequence generation, allocation concealment, blinding, incomplete outcome data, and selective outcome reporting (Table 2 ).
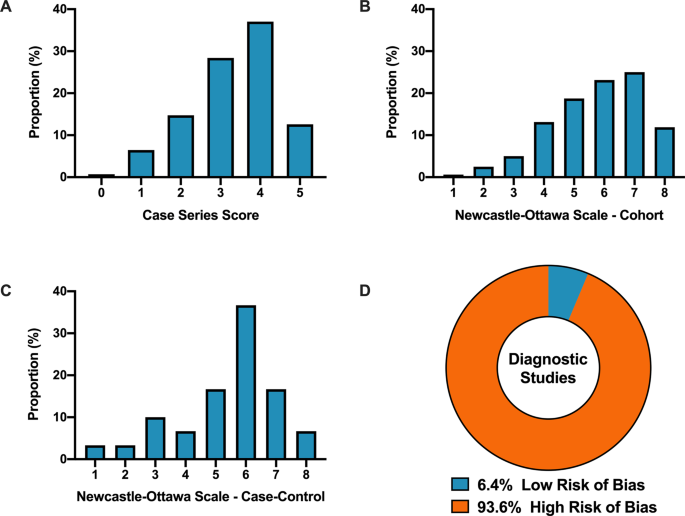
A Distribution of COVID-19 case series studies scored using the Murad tool ( n = 380). B Distribution of COVID-19 cohort studies scored using the Newcastle–Ottawa Scale ( n = 199). C Distribution of COVID-19 case–control studies scored using the Newcastle–Ottawa Scale ( n = 38). D Distribution of COVID-19 diagnostic studies scored using the QUADAS-2 tool ( n = 63). In panel D , blue represents low risk of bias and orange represents high risk of bias.
For secondary outcomes, rapid time from submission to acceptance (stratified by median time of acceptance of <13.0 days) was associated with lower methodological quality scores for case series and cohort study designs but not for case–control nor diagnostic studies (Fig. 3A–D ). Low journal impact factor (<10) was associated with lower methodological quality scores for case series, cohort, and case–control designs (Fig. 3E–H ). Finally, studies originating from different geographical regions had no differences in methodological quality scores with the exception of cohort studies (Fig. 3I–L ). When dichotomized by high vs. low methodological quality scores, a similar trend was observed with rapid time from submission to acceptance (34.4% vs. 46.3%, p = 0.01, Supplementary Fig. 1B ), low impact factor journals (<10) was associated with lower methodological quality score (38.8% vs. 68.0%, p < 0.0001, Supplementary Fig. 1C ). Finally, studies originating in either Americas or Asia/Oceania was associated with higher methodological quality scores than Europe (Supplementary Fig. 1D ).
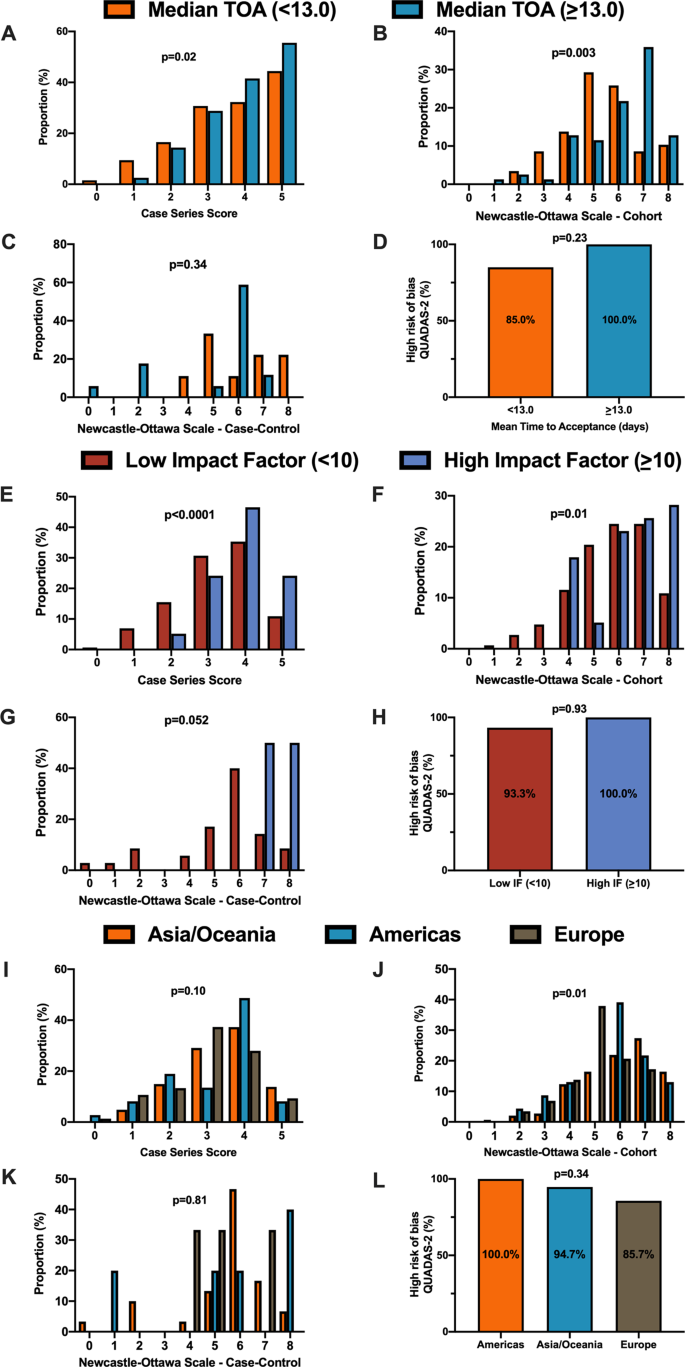
A When stratified by time of acceptance (13.0 days), increased time of acceptance was associated with higher case series score ( n = 186 for <13 days and n = 193 for >=13 days; p = 0.02). B Increased time of acceptance was associated with higher NOS cohort score ( n = 112 for <13 days and n = 144 for >=13 days; p = 0.003). C No difference in time of acceptance and case–control score was observed ( n = 18 for <13 days and n = 27 for >=13 days; p = 0.34). D No difference in time of acceptance and diagnostic risk of bias (QUADAS-2) was observed ( n = 43 for <13 days and n = 33 for >=13 days; p = 0.23). E When stratified by impact factor (IF ≥10), high IF was associated with higher case series score ( n = 466 for low IF and n = 60 for high IF; p < 0.0001). F High IF was associated with higher NOS cohort score ( n = 262 for low IF and n = 68 for high IF; p = 0.01). G No difference in IF and case–control score was observed ( n = 62 for low IF and n = 2 for high IF; p = 0.052). H No difference in IF and QUADAS-2 was observed ( n = 101 for low IF and n = 2 for high IF; p = 0.93). I When stratified by geographical region, no difference in geographical region and case series score was observed ( n = 276 Asia/Oceania, n = 135 Americas, and n = 143 Europe/Africa; p = 0.10). J Geographical region was associated with differences in cohort score ( n = 177 Asia/Oceania, n = 81 Americas, and n = 89 Europe/Africa; p = 0.01). K No difference in geographical region and case–control score was observed ( n = 37 Asia/Oceania, n = 13 Americas, and n = 14 Europe/Africa; p = 0.81). L No difference in geographical region and QUADAS-2 was observed ( n = 49 Asia/Oceania, n = 28 Americas, and n = 28 Europe/Africa; p = 0.34). In panels A – D , orange represents lower median time of acceptance and blue represents high median time of acceptance. In panels E – H , red is low impact factor and blue is high impact factor. In panels I – L , orange represents Asia/Oceania, blue represents Americas, and brown represents Europe. Differences in distributions were analysed by two-sided Kruskal–Wallis test. Differences in diagnostic risk of bias were quantified by Chi-squares test. p < 0.05 was considered statistically significant.
Methodological quality score differences in COVID-19 versus historical control
We matched 539 historical control articles to COVID-19 articles from the same journal with identical study designs in the previous year for a final analysis of 1078 articles (Table 1 ). Overall, 554 (51.4%) case series, 348 (32.3%) cohort, 64 (5.9%) case–control, 106 (9.8%) diagnostic and 6 (0.6%) RCTs were identified from the 1078 total articles. Differences exist between COVID-19 and historical control articles in geographical region of publication, retrospective study design, and sample size calculation (Table 1 ). Time of acceptance was 13.0 (IQR, 5.0–25.0) days in COVID-19 articles vs. 110.0 (IQR, 71.0–156.0) days in control articles (Table 1 and Fig. 4A , p < 0.0001). Case-series methodological quality score was lower in COVID-19 articles compared to the historical control (3.3 (1.1) vs. 4.3 (0.8); n = 554; p < 0.0001; Table 2 and Fig. 4B ). Furthermore, NOS score was lower in COVID-19 cohort studies (5.8 (1.6) vs. 7.1 (1.0); n = 348; p < 0.0001; Table 2 and Fig. 4C ) and case–control studies (5.4 (1.9) vs. 6.6 (1.0); n = 64; p = 0.003; Table 2 and Fig. 4D ). Finally, lower risk of bias in diagnostic studies was in 12 COVID-19 articles (23%; n = 53) compared to 24 control articles (45%; n = 53; p = 0.02; Table 2 and Fig. 4E ). A similar trend was observed between COVID-19 and historical control articles when dichotomized by good vs. low methodological quality scores (Supplementary Fig. 2 ).
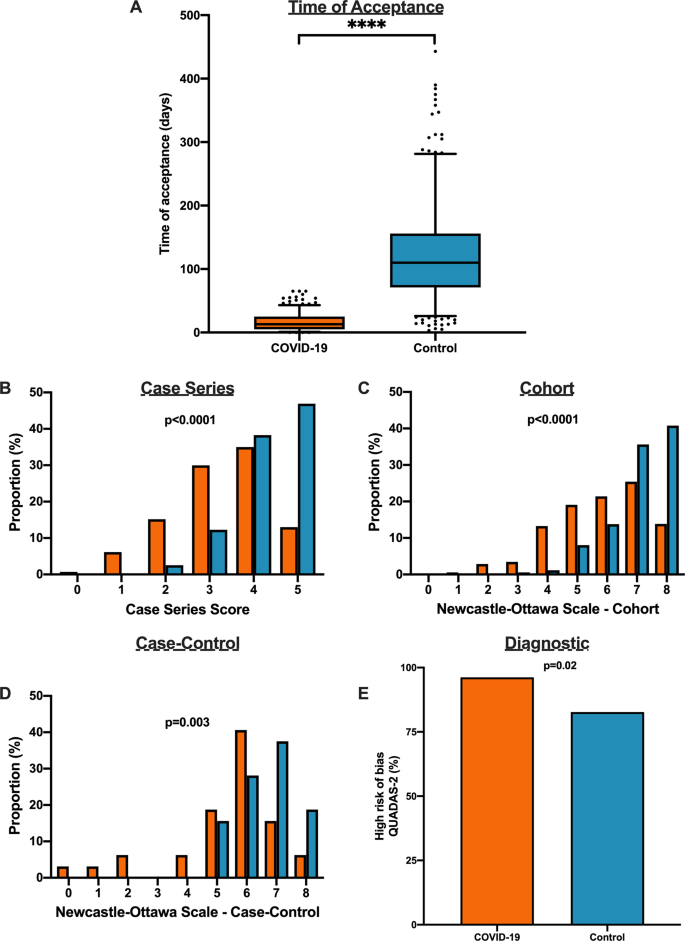
A Time to acceptance was reduced in COVID-19 articles compared to control articles (13.0 [IQR, 5.0–25.0] days vs. 110.0 [IQR, 71.0–156.0] days, n = 347 for COVID-19 and n = 414 for controls; p < 0.0001). B When compared to historical control articles, COVID-19 articles were associated with lower case series score ( n = 277 for COVID-19 and n = 277 for controls; p < 0.0001). C COVID-19 articles were associated with lower NOS cohort score compared to historical control articles ( n = 174 for COVID-19 and n = 174 for controls; p < 0.0001). D COVID-19 articles were associated with lower NOS case–control score compared to historical control articles ( n = 32 for COVID-19 and n = 32 for controls; p = 0.003). E COVID-19 articles were associated with higher diagnostic risk of bias (QUADAS-2) compared to historical control articles ( n = 53 for COVID-19 and n = 53 for controls; p = 0.02). For panel A , boxplot captures 5, 25, 50, 75 and 95% from the first to last whisker. Orange represents COVID-19 articles and blue represents control articles. Two-sided Mann–Whitney U-test was conducted to evaluate differences in time to acceptance between COVID-19 and control articles. Differences in study quality scores were evaluated by two-sided Kruskal–Wallis test. Differences in diagnostic risk of bias were quantified by Chi-squares test. p < 0.05 was considered statistically significant.
In this systematic evaluation of methodological quality, COVID-19 clinical research was primarily observational in nature with modest methodological quality scores. Not only were the study designs low in the hierarchy of scientific evidence, we found that COVID-19 articles were associated with a lower methodological quality scores when published with a shorter time of publication and in lower impact factor journals. Furthermore, in a matched cohort analysis with historical control articles identified from the same journal of the same study design, we demonstrated that COVID-19 articles were associated with lower quality scores and shorter time from submission to acceptance.
The present study demonstrates comparative differences in methodological quality scores between COVID-19 literature and historical control articles. Overall, the accelerated publication of COVID-19 research was associated with lower study quality scores compared to previously published historical control studies. Our research highlights major differences in study quality between COVID-19 and control articles, possibly driven in part by a combination of more thorough editorial and/or peer-review process as suggested by the time to publication, and robust study design with questions which are pertinent for clinicians and patient management 3 , 6 , 7 , 8 , 9 , 10 , 11 .
In the early stages of the COVID-19 pandemic, we speculate that an urgent need for scientific data to inform clinical, social and economic decisions led to shorter time to publication and explosion in publication of COVID-19 studies in both traditional peer-reviewed journals and preprint servers 1 , 12 . The accelerated scientific process in the COVID-19 pandemic allowed a rapid understanding of natural history of COVID-19 symptomology and prognosis, identification of tools including RT-PCR to diagnose SARS-CoV-2 13 , and identification of potential therapeutic options such as tocilizumab and convalescent plasma which laid the foundation for future RCTs 14 , 15 , 16 . A delay in publication of COVID-19 articles due to a slower peer-review process may potentially delay dissemination of pertinent information against the pandemic. Despite concerns of slow peer review, major landmark trials (i.e. RECOVERY and ACTT-1 trial) 17 , 18 published their findings in preprint servers and media releases to allow for rapid dissemination. Importantly, the data obtained in these initial studies should be revisited as stronger data emerges as lower quality studies may fundamentally risk patient safety, resource allocation and future scientific research 19 .
Unfortunately, poor evidence begets poor clinical decisions 20 . Furthermore, lower quality scientific evidence potentially undermines the public’s trust in science during this time and has been evident through misleading information and high-profile retractions 12 , 21 , 22 , 23 . For example, the benefits of hydroxychloroquine, which were touted early in the pandemic based on limited data, have subsequently failed to be replicated in multiple observational studies and RCTs 5 , 24 , 25 , 26 , 27 , 28 , 29 , 30 . One poorly designed study combined with rapid publication led to considerable investment of both the scientific and medical community—akin to quinine being sold to the public as a miracle drug during the 1918 Spanish Influenza 31 , 32 . Moreover, as of June 30, 2020, ClinicalTrials.gov listed an astonishing 230 COVID-19 trials with hydroxychloroquine/plaquenil, and a recent living systematic review of observational studies and RCTs of hydroxychloroquine or chloroquine for COVID-19 demonstrated no evidence of benefit nor harm with concerns of severe methodological flaws in the included studies 33 .
Our study has important limitations. We evaluated the methodological quality of existing studies using established checklists and tools. While it is tempting to associate methodological quality scores with reproducibility or causal inferences of the intervention, it is not possible to ascertain the impact on the study design and conduct of research nor results or conclusions in the identified reports 34 . Second, although the methodological quality scales and checklists used for the manuscript are commonly used for quality assessment in systematic reviews and meta-analyses 35 , 36 , 37 , 38 , they can only assess the methodology without consideration for causal language and are prone to limitations 39 , 40 . Other tools such as the ROBINS-I and GRADE exist to evaluate methodological quality of identified manuscripts, although no consensus currently exists for critical appraisal of non-randomized studies 41 , 42 , 43 . Furthermore, other considerations of quality such as sample size calculation, sex reporting or ethics approval are not considered in these quality scores. As such, the quality scores measured using these checklists only reflect the patient selection, comparability, diagnostic reference standard and methods to ascertain the outcome of the study. Third, the 1:1 ratio to identify our historical control articles may affect the precision estimates of our findings. Interestingly, a simulation of an increase from 1:1 to 1:4 control ratio tightened the precision estimates but did not significantly alter the point estimate 44 . Furthermore, the decision for 1:1 ratio in our study exists due to limitations of available historical control articles from the identical journal in the restricted time period combined with a large effect size and sample size in the analysis. Finally, our analysis includes early publications on COVID-19 and there is likely to be an improvement in quality of related studies and study design as the field matures and higher-quality studies. Accordingly, our findings are limited to the early body of research as it pertains to the pandemic and it is likely that over time research quality will improve over time.
In summary, the early body of peer-reviewed COVID-19 literature was composed primarily of observational studies that underwent shorter peer-review evaluation and were associated with lower methodological quality scores than comparable studies. COVID-19 clinical studies should be revisited with the emergence of stronger evidence.
A systematic literature search was conducted on May 14, 2020 (registered on June 3, 2020 at PROSPERO: CRD42020187318) and reported according to the Preferred Reporting Items for Systematic Reviews and Meta-Analyses. Furthermore, the cohort study was reported according to the Strengthening The Reporting of Observational Studies in Epidemiology checklist. The data supporting the findings of this study is available as Supplementary Data 1 – 2 .
Data sources and searches
The search was created in MEDLINE by a medical librarian with expertise in systematic reviews (S.V.) using a combination of key terms and index headings related to COVID-19 and translated to the remaining bibliographic databases (Supplementary Tables 1 – 3 ). The searches were conducted in MEDLINE (Ovid MEDLINE(R) ALL 1946–), Embase (Ovid Embase Classic + Embase 1947–) and the Cochrane Central Register of Controlled Trials (from inception). Search results were limited to English-only publications, and a publication date limit of January 1, 2019 to present was applied. In addition, a Canadian Agency for Drugs and Technologies in Health search filter was applied in MEDLINE and Embase to remove animal studies, and commentary, newspaper article, editorial, letter and note publication types were also eliminated. Search results were exported to Covidence (Veritas Health Innovation, Melbourne, Australia) and duplicates were eliminated using the platform’s duplicate identification feature.
Study selection, data extraction and methodological quality assessment
We included all types of COVID-19 clinical studies, including case series, observational studies, diagnostic studies and RCTs. For diagnostic studies, the reference standard for COVID-19 diagnosis was defined as a nasopharyngeal swab followed by reverse transcriptase-polymerase chain reaction in order to detect SARS-CoV-2. We excluded studies that were exploratory or pre-clinical in nature (i.e. in vitro or animal studies), case reports or case series of <5 patients, studies published in a language other than English, reviews, methods or protocols, and other coronavirus variants such as the Middle East respiratory syndrome.
The review team consisted of trained research staff with expertise in systematic reviews and one trainee. Title and abstracts were evaluated by two independent reviewers using Covidence and all discrepancies were resolved by consensus. Articles that were selected for full review were independently evaluated by two reviewers for quality assessment using a standardized case report form following the completion of a training period where all reviewers were trained with the original manuscripts which derived the tools or checklists along with examples for what were deemed high scores 35 , 36 , 37 , 38 . Following this, reviewers completed thirty full-text extractions and the two reviewers had to reach consensus and the process was repeated for the remaining manuscripts independently. When two independent reviewers were not able reach consensus, a third reviewer (principal investigator) provided oversight in the process to resolve the conflicted scores.
First and corresponding author names, date of publication, title of manuscript and journal of publication were collected for all included full-text articles. Journal impact factor was obtained from the 2018 InCites Journal Citation Reports from Clarivate Analytics. Submission and acceptance dates were collected in manuscripts when available. Other information such as study type, prospective or retrospective study, sex reporting, sample size calculation, method of SARS-CoV-2 diagnosis and ethics approval was collected by the authors. Methodological quality assessment was conducted using the Newcastle–Ottawa Scale (NOS) for case–control and cohort studies 37 , QUADAS-2 tool for diagnostic studies 38 , Cochrane risk of bias for RCTs 35 and a score derived by Murad et al. for case series studies 36 .
Identification of historical control from identified COVID-19 articles
Following the completion of full-text extraction of COVID-19 articles, we obtained a historical control group by identifying reports matched in a 1:1 fashion. From the eligible COVID-19 article, historical controls were identified by searching the same journal in a systematic fashion by matching the same study design (“case series”, “cohort”, “case control” or “diagnostic”) starting in the journal edition 12 months prior to the COVID-19 article publication on the publisher website (i.e. COVID-19 article published on April 2020, going backwards to April 2019) and proceeding forward (or backward if a specific article type was not identified) in a temporal fashion until the first matched study was identified following abstract screening by two independent reviewers. If no comparison article was found by either reviewers, the corresponding COVID-19 article was excluded from the comparison analysis. Following the identification of the historical control, data extraction and quality assessment was conducted on the identified articles using the standardized case report forms by two independent reviewers and conflicts resolved by consensus. The full dataset has been made available as Supplementary Data 1 – 2 .
Data synthesis and statistical analysis
Continuous variables were reported as mean (SD) or median (IQR) as appropriate, and categorical variables were reported as proportions (%). Continuous variables were compared using Student t -test or Mann–Whitney U-test and categorical variables including quality scores were compared by χ 2 , Fisher’s exact test, or Kruskal–Wallis test.
The primary outcome of interest was to evaluate the methodological quality of COVID-19 clinical literature by study design using the Newcastle–Ottawa Scale (NOS) for case–control and cohort studies, QUADAS-2 tool for diagnostic studies 38 , Cochrane risk of bias for RCTs 35 , and a score derived by Murad et al. for case series studies 36 . Pre-specified secondary outcomes were comparison of methodological quality scores of COVID-19 articles by (i) median time to acceptance, (ii) impact factor, (iii) geographical region and (iv) historical comparator. Time of acceptance was defined as the time between submission to acceptance which captures peer review and editorial decisions. Geographical region was stratified into continents including Asia/Oceania, Europe/Africa and Americas (North and South America). Post hoc comparison analysis between COVID-19 and historical control article quality scores were evaluated using Kruskal–Wallis test. Furthermore, good quality of NOS was defined as 3+ on selection and 1+ on comparability, and 2+ on outcome/exposure domains and high-quality case series scores was defined as a score ≥3.5. Due to a small sample size of identified RCTs, they were not included in the comparison analysis.
The finalized dataset was collected on Microsoft Excel v16.44. All statistical analyses were performed using SAS v9.4 (SAS Institute, Inc., Cary, NC, USA). Statistical significance was defined as P < 0.05. All figures were generated using GraphPad Prism v8 (GraphPad Software, La Jolla, CA, USA).
Reporting summary
Further information on research design is available in the Nature Research Reporting Summary linked to this article.

Data availability
The authors can confirm that all relevant data are included in the paper and in Supplementary Data 1 – 2 . The original search was conducted on MEDLINE, Embase and Cochrane Central Register of Controlled Trials.
Chen, Q., Allot, A. & Lu, Z. Keep up with the latest coronavirus research. Nature 579 , 193 (2020).
Article ADS CAS Google Scholar
Mahase, E. Covid-19: 146 researchers raise concerns over chloroquine study that halted WHO trial. BMJ https://doi.org/10.1136/bmj.m2197 (2020).
Chalmers, I. & Glasziou, P. Avoidable waste in the production and reporting of research evidence. Lancet 374 , 86–89 (2009).
Article Google Scholar
Burns, P. B., Rohrich, R. J. & Chung, K. C. The levels of evidence and their role in evidence-based medicine. Plast. Reconstr. Surg. 128 , 305–310 (2011).
Article CAS Google Scholar
Alexander, P. E. et al. COVID-19 coronavirus research has overall low methodological quality thus far: case in point for chloroquine/hydroxychloroquine. J. Clin. Epidemiol. 123 , 120–126 (2020).
Barakat, A. F., Shokr, M., Ibrahim, J., Mandrola, J. & Elgendy, I. Y. Timeline from receipt to online publication of COVID-19 original research articles. Preprint at medRxiv https://doi.org/10.1101/2020.06.22.20137653 (2020).
Chan, A.-W. et al. Increasing value and reducing waste: addressing inaccessible research. Lancet 383 , 257–266 (2014).
Ioannidis, J. P. A. et al. Increasing value and reducing waste in research design, conduct, and analysis. Lancet 383 , 166–175 (2014).
Chalmers, I. et al. How to increase value and reduce waste when research priorities are set. Lancet 383 , 156–165 (2014).
Salman, R. A.-S. et al. Increasing value and reducing waste in biomedical research regulation and management. Lancet 383 , 176–185 (2014).
Glasziou, P. et al. Reducing waste from incomplete or unusable reports of biomedical research. Lancet 383 , 267–276 (2014).
Bauchner, H. The rush to publication: an editorial and scientific mistake. JAMA 318 , 1109–1110 (2017).
He, X. et al. Temporal dynamics in viral shedding and transmissibility of COVID-19. Nat. Med. 26 , 672–675 (2020).
Guaraldi, G. et al. Tocilizumab in patients with severe COVID-19: a retrospective cohort study. Lancet Rheumatol. 2 , e474–e484 (2020).
Duan, K. et al. Effectiveness of convalescent plasma therapy in severe COVID-19 patients. Proc. Natl Acad. Sci. USA 117 , 9490–9496 (2020).
Shen, C. et al. Treatment of 5 critically Ill patients with COVID-19 with convalescent plasma. JAMA 323 , 1582–1589 (2020).
Beigel, J. H. et al. Remdesivir for the treatment of covid-19—final report. N. Engl. J. Med. 383 , 1813–1826 (2020).
Group, R. C. et al. Dexamethasone in hospitalized patients with Covid-19—preliminary report. N. Engl. J. Med. https://doi.org/10.1056/NEJMoa2021436 (2020).
Ramirez, F. D. et al. Methodological rigor in preclinical cardiovascular studies: targets to enhance reproducibility and promote research translation. Circ. Res 120 , 1916–1926 (2017).
Heneghan, C. et al. Evidence based medicine manifesto for better healthcare. BMJ 357 , j2973 (2017).
Mehra, M. R., Desai, S. S., Ruschitzka, F. & Patel, A. N. RETRACTED: hydroxychloroquine or chloroquine with or without a macrolide for treatment of COVID-19: a multinational registry analysis. Lancet https://doi.org/10.1016/S0140-6736(20)31180-6 (2020).
Servick, K. & Enserink, M. The pandemic’s first major research scandal erupts. Science 368 , 1041–1042 (2020).
Mehra, M. R., Desai, S. S., Kuy, S., Henry, T. D. & Patel, A. N. Retraction: Cardiovascular disease, drug therapy, and mortality in Covid-19. N. Engl. J. Med. 382 , 2582–2582, https://doi.org/10.1056/NEJMoa2007621. (2020).
Article PubMed Google Scholar
Boulware, D. R. et al. A randomized trial of hydroxychloroquine as postexposure prophylaxis for Covid-19. N. Engl. J. Med. 383 , 517–525 (2020).
Gautret, P. et al. Clinical and microbiological effect of a combination of hydroxychloroquine and azithromycin in 80 COVID-19 patients with at least a six-day follow up: a pilot observational study. Travel Med. Infect. Dis. 34 , 101663–101663 (2020).
Geleris, J. et al. Observational study of hydroxychloroquine in hospitalized patients with Covid-19. N. Engl. J. Med. 382 , 2411–2418 (2020).
Borba, M. G. S. et al. Effect of high vs low doses of chloroquine diphosphate as adjunctive therapy for patients hospitalized with severe acute respiratory syndrome coronavirus 2 (SARS-CoV-2) infection: a randomized clinical trial. JAMA Netw. Open 3 , e208857–e208857 (2020).
Mercuro, N. J. et al. Risk of QT interval prolongation associated with use of hydroxychloroquine with or without concomitant azithromycin among hospitalized patients testing positive for coronavirus disease 2019 (COVID-19). JAMA Cardiol. 5 , 1036–1041 (2020).
Molina, J. M. et al. No evidence of rapid antiviral clearance or clinical benefit with the combination of hydroxychloroquine and azithromycin in patients with severe COVID-19 infection. Médecine et. Maladies Infectieuses 50 , 384 (2020).
Group, R. C. et al. Effect of hydroxychloroquine in hospitalized patients with Covid-19. N. Engl. J. Med . 383, 2030–2040 (2020).
Shors, T. & McFadden, S. H. 1918 influenza: a Winnebago County, Wisconsin perspective. Clin. Med. Res. 7 , 147–156 (2009).
Stolberg, S. A Mad Scramble to Stock Millions of Malaria Pills, Likely for Nothing (The New York Times, 2020).
Hernandez, A. V., Roman, Y. M., Pasupuleti, V., Barboza, J. J. & White, C. M. Hydroxychloroquine or chloroquine for treatment or prophylaxis of COVID-19: a living systematic review. Ann. Int. Med. 173 , 287–296 (2020).
Glasziou, P. & Chalmers, I. Research waste is still a scandal—an essay by Paul Glasziou and Iain Chalmers. BMJ 363 , k4645 (2018).
Higgins, J. P. T. et al. The Cochrane Collaboration’s tool for assessing risk of bias in randomised trials. BMJ 343 , d5928 (2011).
Murad, M. H., Sultan, S., Haffar, S. & Bazerbachi, F. Methodological quality and synthesis of case series and case reports. BMJ Evid. Based Med. 23 , 60–63 (2018).
Wells, G. S. B. et al. The Newcastle-Ottawa Scale (NOS) for assessing the quality of nonrandomised studies in meta-analysis. http://wwwohrica/programs/clinical_epidemiology/oxfordasp (2004).
Whiting, P. F. et al. QUADAS-2: a revised tool for the quality assessment of diagnostic accuracy studies. Ann. Intern. Med. 155 , 529–536 (2011).
Sanderson, S., Tatt, I. D. & Higgins, J. P. Tools for assessing quality and susceptibility to bias in observational studies in epidemiology: a systematic review and annotated bibliography. Int. J. Epidemiol. 36 , 666–676 (2007).
Stang, A. Critical evaluation of the Newcastle-Ottawa scale for the assessment of the quality of nonrandomized studies in meta-analyses. Eur. J. Epidemiol. 25 , 603–605 (2010).
Guyatt, G. et al. GRADE guidelines: 1. Introduction-GRADE evidence profiles and summary of findings tables. J. Clin. Epidemiol. 64 , 383–394 (2011).
Quigley, J. M., Thompson, J. C., Halfpenny, N. J. & Scott, D. A. Critical appraisal of nonrandomized studies-A review of recommended and commonly used tools. J. Evaluation Clin. Pract. 25 , 44–52 (2019).
Sterne, J. A. et al. ROBINS-I: a tool for assessing risk of bias in non-randomised studies of interventions. BMJ 355 , i4919 (2016).
Hamajima, N. et al. Case-control studies: matched controls or all available controls? J. Clin. Epidemiol. 47 , 971–975 (1994).
Download references
Acknowledgements
This study received no specific funding or grant from any agency in the public, commercial, or not-for-profit sectors. R.G.J. was supported by the Vanier CIHR Canada Graduate Scholarship. F.D.R. was supported by a CIHR Banting Postdoctoral Fellowship and a Royal College of Physicians and Surgeons of Canada Detweiler Travelling Fellowship. The funder/sponsor(s) had no role in design and conduct of the study, collection, analysis and interpretation of the data.
Author information
These authors contributed equally: Richard G. Jung, Pietro Di Santo.
Authors and Affiliations
CAPITAL Research Group, University of Ottawa Heart Institute, Ottawa, Ontario, Canada
Richard G. Jung, Pietro Di Santo, F. Daniel Ramirez & Trevor Simard
Vascular Biology and Experimental Medicine Laboratory, University of Ottawa Heart Institute, Ottawa, Ontario, Canada
Richard G. Jung, Pietro Di Santo, Trevor Simard & Benjamin Hibbert
Department of Cellular and Molecular Medicine, Faculty of Medicine, University of Ottawa, Ottawa, Ontario, Canada
Richard G. Jung, Trevor Simard & Benjamin Hibbert
Division of Cardiology, University of Ottawa Heart Institute, Ottawa, Ontario, Canada
Pietro Di Santo, Simon Parlow, F. Daniel Ramirez, Trevor Simard & Benjamin Hibbert
School of Epidemiology and Public Health, University of Ottawa, Ottawa, Ontario, Canada
Pietro Di Santo
Faculty of Medicine, University of Ottawa, Ontario, Canada
Cole Clifford & Stephanie Skanes
Department of Medicine, Cumming School of Medicine, Calgary, Alberta, Canada
Graeme Prosperi-Porta
Division of Internal Medicine, The Ottawa Hospital, Ottawa, Ontario, Canada
Berkman Library, University of Ottawa Heart Institute, Ottawa, Ontario, Canada
Sarah Visintini
Hôpital Cardiologique du Haut-Lévêque, CHU Bordeaux, Bordeaux-Pessac, France
F. Daniel Ramirez
L’Institut de Rythmologie et Modélisation Cardiaque (LIRYC), University of Bordeaux, Bordeaux, France
Department of Cardiovascular Medicine, Mayo Clinic, Rochester, MN, USA
Trevor Simard
You can also search for this author in PubMed Google Scholar
Contributions
R.G.J., P.D.S., S.V., F.D.R., T.S. and B.H. participated in the study conception and design. Data acquisition, analysis and interpretation were performed by R.G.J., P.D.S., C.C., G.P.P., S.P., S.S., A.H., F.D.R., T.S. and B.H. Statistical analysis was performed by R.G.J., P.D.S. and B.H. The manuscript was drafted by R.G.J., P.D.S., F.D.R., T.S. and B.H. All authors approved the final version of the manuscript and agree to be accountable to all aspects of the work.
Corresponding author
Correspondence to Benjamin Hibbert .
Ethics declarations
Competing interests.
B.H. reports funding as a clinical trial investigator from Abbott, Boston Scientific and Edwards Lifesciences outside of the submitted work. The remaining authors declare no competing interests.
Additional information
Peer review information Nature Communications Ian White and the other, anonymous, reviewer(s) for their contribution to the peer review of this work. Peer reviewer reports are available.
Publisher’s note Springer Nature remains neutral with regard to jurisdictional claims in published maps and institutional affiliations.
Supplementary information
Supplementary information, peer review file, description of additional supplementary files, supplementary data 1, supplementary data 2, reporting summary, rights and permissions.
Open Access This article is licensed under a Creative Commons Attribution 4.0 International License, which permits use, sharing, adaptation, distribution and reproduction in any medium or format, as long as you give appropriate credit to the original author(s) and the source, provide a link to the Creative Commons license, and indicate if changes were made. The images or other third party material in this article are included in the article’s Creative Commons license, unless indicated otherwise in a credit line to the material. If material is not included in the article’s Creative Commons license and your intended use is not permitted by statutory regulation or exceeds the permitted use, you will need to obtain permission directly from the copyright holder. To view a copy of this license, visit http://creativecommons.org/licenses/by/4.0/ .
Reprints and permissions
About this article
Cite this article.
Jung, R.G., Di Santo, P., Clifford, C. et al. Methodological quality of COVID-19 clinical research. Nat Commun 12 , 943 (2021). https://doi.org/10.1038/s41467-021-21220-5
Download citation
Received : 16 July 2020
Accepted : 13 January 2021
Published : 11 February 2021
DOI : https://doi.org/10.1038/s41467-021-21220-5
Share this article
Anyone you share the following link with will be able to read this content:
Sorry, a shareable link is not currently available for this article.
Provided by the Springer Nature SharedIt content-sharing initiative
This article is cited by
The quality of covid-19 systematic reviews during the coronavirus 2019 pandemic: an exploratory comparison.
- Kevin T. McDermott
- Jos Kleijnen
Systematic Reviews (2024)
Gatekeeping should be conserved in the open science era
- Hugh Desmond
Synthese (2024)
Exploring COVID-19 research credibility among Spanish scientists
- Eduardo Garcia-Garzon
- Ariadna Angulo-Brunet
- Guido Corradi
Current Psychology (2024)
Primary health care research in COVID-19: analysis of the protocols reviewed by the ethics committee of IDIAPJGol, Catalonia
- Anna Moleras-Serra
- Rosa Morros-Pedros
- Ainhoa Gómez-Lumbreras
BMC Primary Care (2023)
Identifying patterns of reported findings on long-term cardiac complications of COVID-19: a systematic review and meta-analysis
- Chenya Zhao
BMC Medicine (2023)
Quick links
- Explore articles by subject
- Guide to authors
- Editorial policies
Sign up for the Nature Briefing newsletter — what matters in science, free to your inbox daily.

An official website of the United States government
Official websites use .gov A .gov website belongs to an official government organization in the United States.
Secure .gov websites use HTTPS A lock ( Lock Locked padlock icon ) or https:// means you've safely connected to the .gov website. Share sensitive information only on official, secure websites.
- Publications
- Account settings
- Advanced Search
- Journal List
Potential limitations in systematic review studies assessing the effect of the main intervention for treatment/therapy of COVID-19 patients: An overview
Mahsa mohseni, hosein ameri, morteza arab-zozani.
- Author information
- Article notes
- Copyright and License information
Edited by: Faris Lami, University of Baghdad, Iraq
Reviewed by: Tinku Basu, Amity University, India; Naina Mohamed Pakkir Maideen, Dubai Health Authority, United Arab Emirates; Edit Szabó, Hungarian Academy of Sciences (MTA), Hungary
*Correspondence: Morteza Arab-Zozani, [email protected] ; orcid.org/0000-0001-7223-6707
This article was submitted to Infectious Diseases – Surveillance, Prevention and Treatment, a section of the journal Frontiers in Medicine
Received 2022 Jun 11; Accepted 2022 Aug 30; Collection date 2022.
This is an open-access article distributed under the terms of the Creative Commons Attribution License (CC BY). The use, distribution or reproduction in other forums is permitted, provided the original author(s) and the copyright owner(s) are credited and that the original publication in this journal is cited, in accordance with accepted academic practice. No use, distribution or reproduction is permitted which does not comply with these terms.
Although several studies have assessed the safety, efficacy, and effectiveness of interventions in treating the COVID-19, many of them have limitations that can have an immense impact on their results. This study aims to assess the potential limitations in systematic reviews (SRs) that evaluate the effect of interventions on the treatment of the COVID-19.
PubMed, Scopus, and Web of Sciences (WOS) databases were searched from inception to January 1, 2022. All systematic reviews investigated the effectiveness, efficacy, safety, and outcome of the main intervention (Favipiravir, Remdesivir, Hydroxychloroquine, Ivermectin, Lopinavir/Ritonavir, or Tocilizumab) for the treatment of COVID-19 patients and reported the potential limitations of the included studies. We assessed the quality of the included studies using the Quality Assessment Tool (QAT) for review articles. We conducted a content analysis and prepared a narrative summary of the limitations.
Forty-six studies were included in this review. Ninety one percent of the included studies scored as strong quality and the remaining (9%) as moderate quality. Only 29.7% of the included systematic reviews have a registered protocol. 26% of the included studies mentioned a funding statement. The main limitations of the included studies were categorized in 10 domains: sample size, heterogeneity, follow-up, treatment, including studies, design, definitions, synthesis, quality, and search.
Various limitations have been reported in all the included studies. Indeed, the existence of limitations in studies can affect their results, therefore, identifying these limitations can help researchers design better studies. As a result, stronger studies with more reliable results will be reported and disseminated. Further research on COVID-19 SRs is essential to improve research quality and also, efficiency among scientists across the world.
Keywords: COVID-19, systematic review, limitations, intervention, treatment
The COVID-19 pandemic began in early 2020 with major health consequences ( 1 ). According to live data from Worldometer website, the total number of coronavirus cases and the number of deaths so far is 595,494,252 and 6,455,301, respectively (Tue, 16 Aug 2022). Numerous studies have assessed the effects of the different interventions on the treatment of the COVID-19 patients ( 2 – 6 ). These studies differ in many ways, including the type of treatment, follow-up time, study design, patient type, and disease severity, each of which can have a positive or negative effect on the results of these studies ( 7 ).
As the global community eagerly awaits credible scientific solutions for this pandemic, researchers and scientists are under much pressure to identify effective therapeutic and preventive strategies for COVID-19. Also, there are many unknowns, and the massive demand for evidence on the treatment of a novel disease such as COVID-19 may be unintentionally affecting studies’ design and conduct. Furthermore, it may inadvertently affect the peer-review and publication process, leading to significant methodology gaps and overall lower quality evidence on COVID-19. These gaps lead to less-informative studies, loss of precious time, and valuable resources ( 8 ).
With the growth of evidence in this area ( 9 ), there is a need for studies that report the results of these individual studies in general. Systematic reviews objectively summarize large amounts of information, identifying gaps in medical research, and identifying beneficial or harmful interventions which will be useful for clinicians, researchers, and even for public and policymakers. The value of a systematic review depends on what was done, what was found, and the clarity of reporting ( 10 ). The results of a systematic review are influenced by the quality of the primary studies included. Methodologically, poor studies tend to exaggerate the overall estimate of treatment effect and may lead to incorrect inferences ( 11 ).
While a need to disseminate information to the medical community and general public was paramount, concerns have been raised regarding the scientific rigor, quality, and limitations in published reports which may potentially effect on the systematic reviews and meta-analysis results ( 1 ). In this study, we aim to identify the potential limitations in systematic reviews that evaluated the effect of interventions on the treatment of the COVID-19 which can help to improve and make the result of studies more accurate in the future.
Methodology
Protocol and registration.
We conducted this overview based on Smith et al. guideline for conducting a systematic review of systematic reviews of healthcare interventions ( 12 ). We also followed the PRISMA guideline for reporting the methods and results of this study ( 13 ).
Eligibility criteria
All systematic reviews with available full text and in EN languages investigated the effectiveness, efficacy, safety, and outcome of the main intervention (Favipiravir, Remdesivir, Hydroxychloroquine, Ivermectin, Lopinavir/Ritonavir, or Tocilizumab) for treatment of COVID-19 patients and reported the potential limitation of the study were included.
We exclude articles that are full-text not available or used other treatment options than mentioned drugs. For example, acupuncture or traditional medicine, or supplement therapy. Preprint and without peer review articles also was excluded.
Information sources and search strategy
We searched PubMed, Scopus, and Web of Sciences (WOS) databases from inception to January 1, 2022, for the keywords COVID-19, “SARS-CoV-2,” “novel coronavirus,” “systematic review,” OR limitation in the title, abstract, or main text of the published article. There was no limitation regarding time or language. We also conducted a manual search in Google Scholar for potential missing articles. In addition to database searches, we screened reference lists of included studies after screening records were retrieved via databases and also contacted the corresponding authors of the included studies. The full search strategy for all databases is presented in Supplementary Table 1 .
Selection process
After the search was completed, all retrieved records were imported in EndNote, version X7, and duplicate removed. Two independent reviewers (HA, MM) screened the records based on the title, abstract, and full text. For increasing the agreement between reviewers we piloted a set of 30 studies before the screening. Discrepancies at this stage were resolved by consensus with a third reviewer (MA-Z).
Data collection process and data items
Two independent reviewers (HA, MM) extracted the data. We designed a data extraction table for this study, which was piloted by two reviewers (5 studies). we extracted the following data: first author name, corresponding authors name and email, Publication year, number of authors, study design, number of included studies in each included systematic review, investigated drug, country, language limitation, time of the search, number of the investigated outcome, sample size, limitations, funding statement, mean age, gender (%), protocol and registration information. Discrepancies at this stage were resolved by consensus with a third reviewer (MA-Z).
Quality appraisal
Two reviewers (HA and MM) independently assessed the quality of the included studies. We assessed the quality of the included studies using the Quality Assessment Tool (QAT) for review articles developed by healthevidence.org , which was piloted by two reviewers (5 studies) including ten quality criteria. A final review quality rating for each review is assigned: strong (8–10/10), moderate (5–7/10), or weak (1–4/10). Any discrepancies were resolved upon consultation with a third reviewer (MA-Z).
QAT tool available at: https://www.healthevidence.org/our-appraisal-tools.aspx .
Synthesis of results
For data synthesis, we prepared a table summarizing systematic review information. We also used graphs for presenting some information. We then conducted a content analysis and prepared a narrative summary of the limitations. Two authors (HA, MM) read and reread the results reported in published articles to extract limitations. The coding frame and final categories were developed by 3 authors (HA, MM, and MA-Z) using these data.
Study selection
A total of 773 records were retrieved from the database search. After removing duplicates, 525 records were screened by title, abstract, and full-text based on eligibility criteria, of which 46 studies were included in the final review ( 14 – 58 , 59 ). Twenty-seven studies were excluded after Full-text screening. The reasons for exclusion were as follows: Protocol (5 records), Preprint (6 records), Full-text not available after contacting the corresponding author (2 records), Not reporting limitation (5 records), and not investigating our target intervention (9 records). The PRISMA flow diagram for the complete study selection process is presented in Figure 1 .
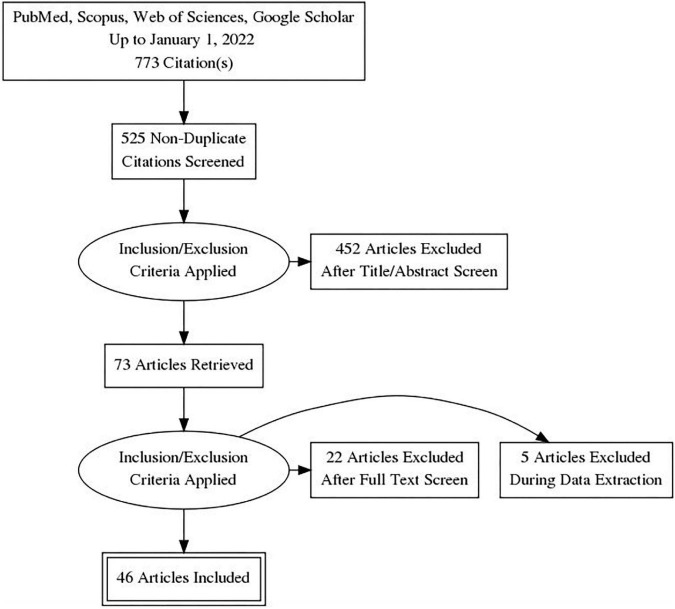
PRISMA flow diagram.
Study characteristics
The number of authors of the included systematic reviews varied between 3 and 58 people. Most studies were from Asia (46%), America (31%), and Europe (17%). Also, by country, most studies were reported from the United States and India ( Figure 2 ). 80.4% of the included systematic review conducted a meta-analysis. The number of included studies in the included systematic reviews varied between 2 and 136. Only 29.7% of the included systematic reviews have a registered protocol. Also, 26% of the included systematic reviews mentioned a funding statement. More details about the characteristics of included systematic reviews are presented in Table 1 . The most studied drug in the included studies was Remdesivir (17.37%) ( Figure 3 ).
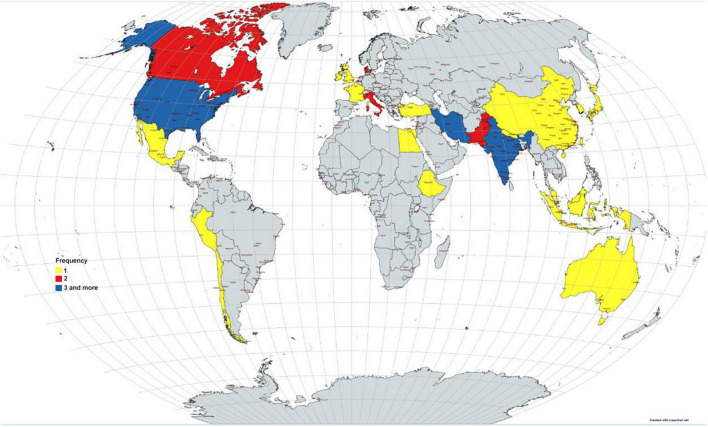
Distribution of the included studies by countries.
Summary characteristics of the included studies.
*MC, Multi Country.
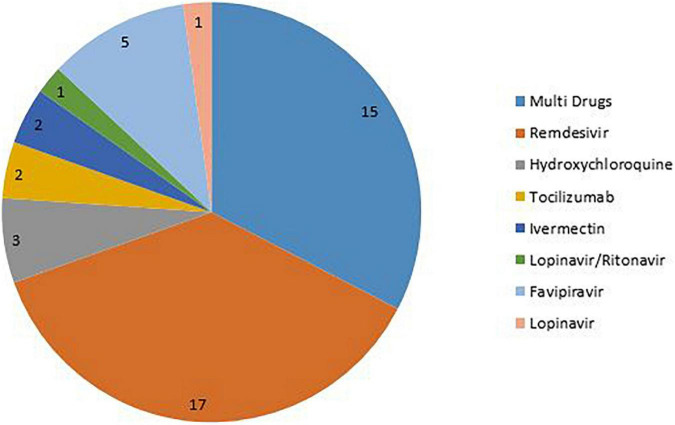
Distribution of the drugs in the included studies.
The overall mean quality score of the included studies was 9.5. Overall, 91% of the included studies were scored as strong quality and the remaining (9%) as moderate quality. The overall scores ranged between 7 and 10. About 74% of the included studies had a score of 10, 11% had a score of 9, 6% had a score of 8, and the remaining (9%) had a score of 7 ( Figure 4 ) (for more details about items on the QAT checklist see Supplementary Table 2 ).
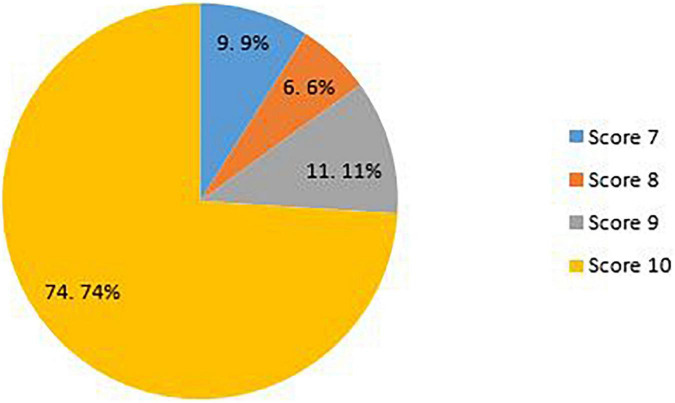
Quality scores of the included studies.
Results of synthesis
Potential limitations of the included studies.
Various studies have listed different limitations for the studies, some related to how the systematic review was conducted and some related to the studies included in these systematic reviews. The main limitations of the included studies were categorized in 10 domains: Heterogeneity (4 sub-categories), sample size (2 sub-categories), follow-up (2 sub-categories), treatment (7 sub-categories), included studies (4 sub-categories), design (10sub-categories), definitions (3 sub-categories), synthesis (4 sub-categories), quality (2 sub-categories), and search (4 sub-categories). The highest frequencies reported in the included studies related to the heterogeneity in sample population, small sample size, and database searches.
Heterogeneity in studies has been reported for a variety of reasons, including differences in the sample population regarding age, gender, ethnicity, and racial groups; different level of disease severity in the included patients; different control group; and difference in the investigated outcome. Treatment-related limitations were mostly related to differences regarding the administration of drug, dose, duration of treatment, and different standard protocols and guidelines. Also, there are differences related to discontinuation, combination therapy, and supportive care which obscure the effect of the main treatment.
The studies had several design shortcomings. Many studies have suffered from a lack of randomization, placebo, blinding, and comparator arm. Selection bias, and publication bias, confounding bias were also reported in the studies. Also, different strategies regarding search were another limitation. Different databases, using pre-print and un-published data, limitations on language, and missing some eligible studies were the important limitation in this regard.
More details about the potential limitations of the included systematic reviews are presented in Table 2 .
Potential limitation of the included studies.
With the spread of the COVID-19 pandemic and has many consequences, the need arose to conduct studies and disseminate their findings ( 1 ).
Systematic reviews are a valuable resource in academia and practice. Well-done systematic reviews, which include but are not limited to meta-analyses, offer an efficient way to evaluate a large amount of information for decision-makers in areas of research, policy, and patient care. Systematic reviews can help us know what we know about a topic, and what is not yet known, often to a greater extent than the findings of a single study ( 60 – 64 ). Systemic review studies on the safety and efficacy of COVID-19 have grown in numbers. Regarding the growing number of studies and rapid publication time, there are concerns about accuracy, quality, and limitations. Richard et al. performed a systematic review to evaluate the methodological quality of currently available COVID-19 studies compared to historical controls. This research showed that COVID-19 clinical studies have a shorter time to publication and have lower methodological quality scores than control studies in the same journal ( 1 ). We tried to identify the potential limitations of COVID-19 systematic reviews which can improve and make the result of studies more accurate.
The current review examined 46 systematic reviews and all of them were conducted on COVID-19 patients. These studies differ in many aspects, including the type of treatment, follow-up time, study design, patient type, and disease severity. Most of them (80.4%) have conducted meta-analyses. Overall, 91% of the included studies were scored as strong quality, and the rest of them were moderate. The number of studies in the included systematic reviews ranged from 2 to 136.
In this study, we classified the reported limitations into 10 categories and 42 sub-categories. Heterogeneity, sample size, follow-up, treatment, including studies, design, definitions, synthesis, quality, and search are identified as the main limitation of included studies. These limitations were attributed to the included systematic reviews or due to primary studies in these systematic reviews. Among all the limitations, sample population, sample size, and database search were found to be the most-mentioned limitations with frequencies of 22, 21, and 18 in the studies, respectively. It seems that a greater number of limitations could be due to primary studies in the systematic reviews including heterogeneity, small sample size, short follow-up time, and low quality of included studies. Limitations in systematic review studies result from selection of studies, choice of relevant outcomes, methods of analysis, interpretation of heterogeneity, and generalization, application of results, and proper search ( 65 ).
Heterogeneity contains four subcategories including differences in the sample population, differences in disease severity of patients, different control group, and different measured outcomes. Differences in the sample population in terms of age, gender, race, and comorbidities in the participants are the most reported limitation. Heterogeneity across the studies may affect the study results ( 65 ). For instance, pooling the data of the original articles would be highly difficult due to heterogeneity in the study design and reported outcomes ( 25 ), and heterogeneity in disease severity could affect the treatment output.
The small sample size is the second most frequently reported limitation in 21 studies. The number of participants in the included studies was small which could decrease the power of the studies, furthermore comparing the interventions regarding the efficacy would not be incontrovertible. Therefore, the findings need to be interpreted with caution.
Database search is another important item that belongs to the search category and is reported in 18 studies. It may potentially limit access to eligible trials for inclusion and miss some data.
Treatment-related limitations are mostly associated with differences regarding the administration of drugs, dose, duration of treatment, and different standard protocols and guidelines. The lack of uniform guidelines for administering additional treatments and providing supportive care for COVID-19 patients in clinical trials may lead to inaccurate and unreliable outcomes. These limitations can generate confounding bias ( 36 ). Also, there are other items belonging to this category such as differences related to discontinuation, combination therapy, and supportive care which obscure the effect of the main treatment.
In addition to the above-mentioned limitations, different follow-up times, low quality of the included studies, pre-publish and unpublished studies, different comparator arms, and heterogeneity in control groups are the other highly reported items. The lack of a comparison/control group can limit the validity of the meta-analysis.
As mentioned, although systematic reviews are considered the gold standard of evidence for clinical decision-making, one should keep in mind that meta-analyses should neither be a replacement for well-designed large-scale randomized studies nor a justification for conducting small underpowered studies ( 65 ). As other studies reported, the quality of the methodology and reporting of present COVID-19 SR is far from optimal. In addition, Differences in disease definition and heterogeneity in studies are important factors influencing the results of these studies. Following existing guidelines and proper study design can be one of the factors reducing the limitations of these studies ( 66 , 67 ). Taken together, poor designs and various limitations of the studies render them ineffective in gaging the full extent of its safety and efficacy and thus warrant further research into the use of interventions in COVID-19 patient treatment. Our study further highlighted the importance of conducting quality studies so that the results can be trusted with more certainty.
Implications for future research
Our results can be used as a guide for designing and reporting the future studies in this field. Undoubtedly, awareness of the limitations of articles in this field can reduce bias and on the other hand increase the power of studies. Considering these issues helps researchers to report studies in a more integrated way, which can also help readers to better understand the results of studies and prevent the repetition of errors and mistakes or limitations reported in previous studies. It is recommended that researchers interested in research related to COVID-19, as well as those interested in investigating the effectiveness of treatments for this disease, must consider the points mentioned in this study when designing, implementing, and reporting their studies. In addition, respected researchers can design similar studies for other fields related to this disease and report their results. Designing such studies can greatly contribute to evidence-based decision making.
Strengths and limitations
Although this study is an overview, and the quality appraisal is optional, but the quality of the articles has been evaluated in it, which is one of the strong points of the study. Also, we conducted this overview based on Smith et al. guideline for conducting a systematic review of systematic reviews and report the results of this study based on PRISMA guideline. All the steps of this study were done by two independent reviewers, which reduces errors and increases the power of the study. There are many potential limitations to this overview. First, a literature search was conducted in the three major electronic databases, Scopus, Pubmed, and WOS, but no other databases were searched, as was the “gray” literature. Therefore, additional relevant studies might have been missed. Second, we included all systematic reviews with available full text and in EN languages investigated the effectiveness, efficacy, safety, and outcome of the main intervention (Favipiravir, Remdesivir, Hydroxychloroquine, Ivermectin, Lopinavir/Ritonavir, or Tocilizumab) for treatment of COVID-19 patients. However, there are other interventions for the treatment of this disease, which can be investigated in other studies, but due to the small number of them, they were not included in this study and only the main interventions were used. Third, we excluded articles published in preprint databases due to lack of peer review.
Data availability statement
The original contributions presented in this study are included in the article/ Supplementary material , further inquiries can be directed to the corresponding author.
Author contributions
MA-Z: idea, design, analyses, writing the first draft, and revising. MM and HA: data extraction, quality appraisal, writing the first draft, and revising. All authors read and approved the final draft before submission.
Conflict of interest
The authors declare that the research was conducted in the absence of any commercial or financial relationships that could be construed as a potential conflict of interest.
Publisher’s note
All claims expressed in this article are solely those of the authors and do not necessarily represent those of their affiliated organizations, or those of the publisher, the editors and the reviewers. Any product that may be evaluated in this article, or claim that may be made by its manufacturer, is not guaranteed or endorsed by the publisher.
Supplementary material
The Supplementary Material for this article can be found online at: https://www.frontiersin.org/articles/10.3389/fmed.2022.966632/full#supplementary-material
- 1. Jung RG, Di Santo P, Clifford C, Prosperi-Porta G, Skanes S, Hung A, et al. Methodological quality of COVID-19 clinical research. Nat Commun. (2021) 12:1–10. 10.1038/s41467-021-21220-5 [ DOI ] [ PMC free article ] [ PubMed ] [ Google Scholar ]
- 2. Ansarin K, Tolouian R, Ardalan M, Taghizadieh A, Varshochi M, Teimouri S, et al. Effect of bromhexine on clinical outcomes and mortality in COVID-19 patients: a randomized clinical trial. Bioimpacts. (2020) 10:209. 10.34172/bi.2020.27 [ DOI ] [ PMC free article ] [ PubMed ] [ Google Scholar ]
- 3. Doi Y, Hibino M, Hase R, Yamamoto M, Kasamatsu Y, Hirose M, et al. A prospective, randomized, open-label trial of early versus late favipiravir therapy in hospitalized patients with COVID-19. Antimicrob Agents Chemother. (2020) 64:e01897–20. 10.1128/AAC.01897-20 [ DOI ] [ PMC free article ] [ PubMed ] [ Google Scholar ]
- 4. Salvarani C, Dolci G, Massari M, Merlo DF, Cavuto S, Savoldi L, et al. Effect of tocilizumab vs standard care on clinical worsening in patients hospitalized with COVID-19 pneumonia: a randomized clinical trial. JAMA Internal Med. (2021) 181:24–31. 10.1001/jamainternmed.2020.6615 [ DOI ] [ PMC free article ] [ PubMed ] [ Google Scholar ]
- 5. Chen Z, Hu J, Zhang Z, Jiang S, Han S, Yan D, et al. Efficacy of hydroxychloroquine in patients with COVID-19: results of a randomized clinical trial. medRxiv [Preprint]. (2020). 10.1101/2020.03.22.20040758 [ DOI ] [ Google Scholar ]
- 6. Davoudi-Monfared E, Rahmani H, Khalili H, Hajiabdolbaghi M, Salehi M, Abbasian L, et al. A randomized clinical trial of the efficacy and safety of interferon β-1a in treatment of severe COVID-19. Antimicrob Agents Chemother. (2020) 64:e01061–20. 10.1128/AAC.01061-20 [ DOI ] [ PMC free article ] [ PubMed ] [ Google Scholar ]
- 7. Barceló MA, Saez M. Methodological limitations in studies assessing the effects of environmental and socioeconomic variables on the spread of COVID-19: a systematic review. Environ Sci Eur. (2021) 33:1–18. 10.1186/s12302-021-00550-7 [ DOI ] [ PMC free article ] [ PubMed ] [ Google Scholar ]
- 8. Alexander PE, Debono VB, Mammen MJ, Iorio A, Aryal K, Deng D, et al. COVID-19 coronavirus research has overall low methodological quality thus far: case in point for chloroquine/hydroxychloroquine. J Clin Epidemiol. (2020) 123:120–6. 10.1016/j.jclinepi.2020.04.016 [ DOI ] [ PMC free article ] [ PubMed ] [ Google Scholar ]
- 9. Spinner CD, Gottlieb RL, Criner GJ, López JRA, Cattelan AM, Viladomiu AS, et al. Effect of remdesivir vs standard care on clinical status at 11 days in patients with moderate COVID-19: a randomized clinical trial. JAMA. (2020) 324:1048–57. 10.1001/jama.2020.16349 [ DOI ] [ PMC free article ] [ PubMed ] [ Google Scholar ]
- 10. Gopalakrishnan S, Ganeshkumar P. Systematic reviews and meta-analysis: understanding the best evidence in primary healthcare. J Fam Med Prim Care. (2013) 2:9. 10.4103/2249-4863.109934 [ DOI ] [ PMC free article ] [ PubMed ] [ Google Scholar ]
- 11. Khan KS, Daya S, Jadad AR. The importance of quality of primary studies in producing unbiased systematic reviews. Arch Internal Med. (1996) 156:661–6. 10.1001/archinte.156.6.661 [ DOI ] [ PubMed ] [ Google Scholar ]
- 12. Smith V, Devane D, Begley CM, Clarke M. Methodology in conducting a systematic review of systematic reviews of healthcare interventions. BMC Med Res Methodol. (2011) 11:15. 10.1186/1471-2288-11-15 [ DOI ] [ PMC free article ] [ PubMed ] [ Google Scholar ]
- 13. Page ME, McKenzie J, Bossuyt PM, Boutron I, Hoffmann TC, Mulrow CD, et al. Updating guidance for reporting systematic reviews: development of the PRISMA 2020 statement. J Clin Epidemiol. (2021) 134:103–12. 10.1016/j.jclinepi.2021.02.003 [ DOI ] [ PubMed ] [ Google Scholar ]
- 14. Wilt TJ, Kaka AS, MacDonald R, Greer N, Obley A, Duan-Porter W. Remdesivir for adults with COVID-19 : a living systematic review for American College of Physicians Practice Points. Ann Internal Med. (2021) 174:209–20. 10.7326/M20-5752 [ DOI ] [ PMC free article ] [ PubMed ] [ Google Scholar ]
- 15. Verdugo-Paiva F, Izcovich A, Ragusa M, Rada G. Lopinavir-ritonavir for COVID-19: a living systematic review. Medwave. (2020) 20:e7967. 10.5867/medwave.2020.06.7966 [ DOI ] [ PubMed ] [ Google Scholar ]
- 16. Vegivinti CTR, Pederson JM, Saravu K, Gupta N, Barrett A, Davis AR, et al. Remdesivir therapy in patients with COVID-19: a systematic review and meta-analysis of randomized controlled trials. Ann Med Surg. (2021) 62:43–8. 10.1016/j.amsu.2020.12.051 [ DOI ] [ PMC free article ] [ PubMed ] [ Google Scholar ]
- 17. Thoguluva Chandrasekar V, Venkatesalu B, Patel HK, Spadaccini M, Manteuffel J, Ramesh M. Systematic review and meta-analysis of effectiveness of treatment options against SARS-CoV-2 infection. J Med Virol. (2021) 93:775–85. 10.1002/jmv.26302 [ DOI ] [ PMC free article ] [ PubMed ] [ Google Scholar ]
- 18. Thiruchelvam K, Kow CS, Hadi MA, Hasan SS. The use of remdesivir for the management of patients with moderate-to-severe COVID-19: a systematic review. Exp Rev Anti Infect Ther. (2021) 20:211–29. 10.1080/14787210.2021.1949984 [ DOI ] [ PMC free article ] [ PubMed ] [ Google Scholar ]
- 19. Singh S, Khera D, Chugh A, Khera PS, Chugh VK. Efficacy and safety of remdesivir in COVID-19 caused by SARS-CoV-2: a systematic review and meta-analysis. BMJ Open. (2021) 11:e048416. 10.1136/bmjopen-2020-048416 [ DOI ] [ PMC free article ] [ PubMed ] [ Google Scholar ]
- 20. Siemieniuk RAC, Bartoszko JJ, Ge L, Zeraatkar D, Izcovich A, Pardo-Hernandez H, et al. Drug treatments for covid-19: living systematic review and network meta-analysis. BMJ. (2020) 370:m2980. [ DOI ] [ PMC free article ] [ PubMed ] [ Google Scholar ]
- 21. Shrestha DB, Budhathoki P, Rawal E, Raut S, Khadka S. Remdesivir: a potential game-changer or just a myth? A systematic review and meta-analysis. Life Sci. (2021) 264:118663. 10.1016/j.lfs.2020.118663 [ DOI ] [ PMC free article ] [ PubMed ] [ Google Scholar ]
- 22. Shrestha DB, Budhathoki P, Khadka S, Shah PB, Pokharel N, Rashmi P. Favipiravir versus other antiviral or standard of care for COVID-19 treatment: a rapid systematic review and meta-analysis. Virol J. (2020) 17:141. 10.1186/s12985-020-01412-z [ DOI ] [ PMC free article ] [ PubMed ] [ Google Scholar ]
- 23. Sarfraz A, Sarfraz Z, Marcos Sanchez-Gonzalez JM, Michel G, Frontela O, Posada J, et al. Randomized controlled trials of remdesivir in hospitalized coronavirus disease 2019 patients: a meta-analysis. Turk J Emerg Med. (2021) 21:43. 10.4103/2452-2473.309139 [ DOI ] [ PMC free article ] [ PubMed ] [ Google Scholar ]
- 24. Santenna C, Vidyasagar K, Amarneni KC, Ghanta SN, Sadasivam B, Pathan S, et al. The safety, tolerability and mortality reduction efficacy of remdesivir; based on randomized clinical trials, observational and case studies reported safety outcomes: an updated systematic review and meta-analysis. Therap Adv Drug Saf. (2021) 12:20420986211042517. 10.1177/20420986211042517 [ DOI ] [ PMC free article ] [ PubMed ] [ Google Scholar ]
- 25. Roshanshad A, Kamalipour A, Ashraf MA, Roshanshad R, Jafari S, Nazemi P, et al. The efficacy of remdesivir in coronavirus disease 2019 (COVID-19): a systematic review. Iran J Microbiol. (2020) 12:376. 10.18502/ijm.v12i5.4597 [ DOI ] [ PMC free article ] [ PubMed ] [ Google Scholar ]
- 26. Rezagholizadeh A, Khiali S, Sarbakhsh P, Entezari-Maleki T. Remdesivir for treatment of COVID-19; an updated systematic review and meta-analysis. Eur J Pharmacol. (2021) 897:173926. 10.1016/j.ejphar.2021.173926 [ DOI ] [ PMC free article ] [ PubMed ] [ Google Scholar ]
- 27. Qomara WF, Primanissa DN, Amalia SH, Purwadi FV, Zakiyah N. Effectiveness of remdesivir, lopinavir/ritonavir, and favipiravir for COVID-19 treatment: a systematic review. Int J Gen Med. (2021) 14:8557–71. 10.2147/IJGM.S332458 [ DOI ] [ PMC free article ] [ PubMed ] [ Google Scholar ]
- 28. Prakash A, Singh H, Kaur H, Semwal A, Sarma P, Bhattacharyya A, et al. Systematic review and meta-analysis of effectiveness and safety of favipiravir in the management of novel coronavirus (COVID-19) patients. Indian J Pharmacol. (2020) 52:414–21. 10.4103/ijp.ijp_998_20 [ DOI ] [ PMC free article ] [ PubMed ] [ Google Scholar ]
- 29. Piscoya A, Ng-Sueng LF, Parra del Riego A, Cerna-Viacava R, Pasupuleti V, Roman YM, et al. Efficacy and harms of remdesivir for the treatment of COVID-19: a systematic review and meta-analysis. PLoS One. (2020) 15:e0243705. 10.1371/journal.pone.0243705 [ DOI ] [ PMC free article ] [ PubMed ] [ Google Scholar ]
- 30. Padhy BM, Mohanty RR, Das S, Meher BR. Therapeutic potential of ivermectin as add on treatment in COVID 19: a systematic review and meta-analysis. J Pharm Pharmaceut Sci. (2020) 23:462–9. 10.18433/jpps31457 [ DOI ] [ PubMed ] [ Google Scholar ]
- 31. Özlüşen B, Kozan ş, Akcan RE, Kalender M, Yaprak D, Peltek ÝB, et al. Effectiveness of favipiravir in COVID-19: a live systematic review. Eur J Clin Microbiol Infect Dis. (2021) 40:2575–83. 10.1007/s10096-021-04307-1 [ DOI ] [ PMC free article ] [ PubMed ] [ Google Scholar ]
- 32. Okoli GN, Rabbani R, Copstein L, Al-Juboori A, Askin N, Abou-Setta AM. Remdesivir for coronavirus disease 2019 (COVID-19): a systematic review with meta-analysis and trial sequential analysis of randomized controlled trials. Infect Dis. (2021) 53:691–9. 10.1080/23744235.2021.1923799 [ DOI ] [ PMC free article ] [ PubMed ] [ Google Scholar ]
- 33. Manzo-Toledo A, Torres-Rosas R, Mendieta-Zerón H, Arriaga-Pizano L, Argueta-Figueroa L. Hydroxychloroquine in the treatment of covid-19 disease: a systematic review and meta-analysis. Med J Indonesia. (2021) 30:20–32. 10.13181/mji.oa.205012 [ DOI ] [ Google Scholar ]
- 34. Manabe T, Kambayashi D, Akatsu H, Kudo K. Favipiravir for the treatment of patients with COVID-19: a systematic review and meta-analysis. BMC Infect Dis. (2021) 21:489. 10.1186/s12879-021-06164-x [ DOI ] [ PMC free article ] [ PubMed ] [ Google Scholar ]
- 35. Lai C-C, Chen C-H, Wang C-Y, Chen K-H, Wang Y-H, Hsueh P-R. Clinical efficacy and safety of remdesivir in patients with COVID-19: a systematic review and network meta-analysis of randomized controlled trials. J Antimicrob Chemother. (2021) 76:1962–8. 10.1093/jac/dkab093 [ DOI ] [ PMC free article ] [ PubMed ] [ Google Scholar ]
- 36. Kotak S, Khatri M, Malik M, Malik M, Hassan W, Amjad A, et al. Use of tocilizumab in COVID-19: a systematic review and meta-analysis of current evidence. Cureus. (2020) 12:e10869. 10.7759/cureus.10869 [ DOI ] [ PMC free article ] [ PubMed ] [ Google Scholar ]
- 37. Kim MS, An MH, Kim WJ, Hwang TH. Comparative efficacy and safety of pharmacological interventions for the treatment of COVID-19: a systematic review and network meta-analysis. PLoS Med. (2020) 17:e1003501. 10.1371/journal.pmed.1003501 [ DOI ] [ PMC free article ] [ PubMed ] [ Google Scholar ]
- 38. Kaka AS, MacDonald R, Greer N, Vela K, Duan-Porter W, Obley A, et al. Major update: remdesivir for adults with COVID-19 : a living systematic review and meta-analysis for the American College of Physicians Practice Points. Ann Internal Med. (2021) 174:663–72. 10.7326/M20-8148 [ DOI ] [ PMC free article ] [ PubMed ] [ Google Scholar ]
- 39. Juul S, Nielsen EE, Feinberg J, Siddiqui F, Jørgensen CK, Barot E, et al. Interventions for treatment of COVID-19: a living systematic review with meta-analyses and trial sequential analyses (The LIVING Project). PLoS Med. (2020) 17:e1003293. 10.1371/journal.pmed.1003293 [ DOI ] [ PMC free article ] [ PubMed ] [ Google Scholar ]
- 40. Juul S, Nielsen EE, Feinberg J, Siddiqui F, Jørgensen CK, Barot E, et al. Interventions for treatment of COVID-19: second edition of a living systematic review with meta-analyses and trial sequential analyses (The LIVING Project). PLoS One. (2021) 16:e0248132. 10.1371/journal.pone.0248132 [ DOI ] [ PMC free article ] [ PubMed ] [ Google Scholar ]
- 41. Jankelson L, Karam G, Becker ML, Chinitz LA, Tsai MC. QT prolongation, torsades de pointes, and sudden death with short courses of chloroquine or hydroxychloroquine as used in COVID-19: a systematic review. Heart Rhythm. (2020) 17:1472–9. 10.1016/j.hrthm.2020.05.008 [ DOI ] [ PMC free article ] [ PubMed ] [ Google Scholar ]
- 42. Hussain N, Yoganathan A, Hewage S, Alom S, Harky A. The effect of antivirals on COVID-19: a systematic review. Expert Rev Anti Infect Ther. (2021) 19:473–86. 10.1080/14787210.2021.1823832 [ DOI ] [ PubMed ] [ Google Scholar ]
- 43. Hernandez AV, Roman YM, Pasupuleti V, Barboza JJ, White CM. Hydroxychloroquine or chloroquine for treatment or prophylaxis of COVID-19: a living systematic review. Ann Internal Med. (2020) 173:287–96. 10.7326/M20-2496 [ DOI ] [ PubMed ] [ Google Scholar ]
- 44. Hassanipour S, Arab-Zozani M, Amani B, Heidarzad F, Fathalipour M, Martinez-de-Hoyo R. The efficacy and safety of favipiravir in treatment of COVID-19: a systematic review and meta-analysis of clinical trials. Sci Rep. (2021) 11:11022. 10.1038/s41598-021-90551-6 [ DOI ] [ PMC free article ] [ PubMed ] [ Google Scholar ]
- 45. Gholamhoseini MT, Yazdi-Feyzabadi V, Goudarzi R, Mehrolhassani MH. Safety and efficacy of remdesivir for the treatment of COVID-19: a systematic review and meta-analysis. J Pharm Pharmaceut Sci. (2021) 24:237–45. 10.18433/jpps31870 [ DOI ] [ PubMed ] [ Google Scholar ]
- 46. Fiolet T, Guihur A, Rebeaud ME, Mulot M, Peiffer-Smadja N, Mahamat-Saleh Y. Effect of hydroxychloroquine with or without azithromycin on the mortality of coronavirus disease 2019 (COVID-19) patients: a systematic review and meta-analysis. Clin Microbiol Infect. (2021) 27:19–27. 10.1016/j.cmi.2020.08.022 [ DOI ] [ PMC free article ] [ PubMed ] [ Google Scholar ]
- 47. Elsawah HK, Elsokary MA, Abdallah MS, ElShafie AH. Efficacy and safety of remdesivir in hospitalized Covid-19 patients: systematic review and meta-analysis including network meta-analysis. Rev Med Virol. (2021) 31:e2187. 10.1002/rmv.2187 [ DOI ] [ PubMed ] [ Google Scholar ]
- 48. Diaz-Arocutipa C, Brañez-Condorena A, Hernandez AV. QTc prolongation in COVID-19 patients treated with hydroxychloroquine, chloroquine, azithromycin, or lopinavir/ritonavir: a systematic review and meta-analysis. Pharmacoepidemiol Drug Saf. (2021) 30:694–706. 10.1002/pds.5234 [ DOI ] [ PMC free article ] [ PubMed ] [ Google Scholar ]
- 49. Das S, Bhowmick S, Tiwari S, Sen S. An updated systematic review of the therapeutic role of hydroxychloroquine in coronavirus disease-19 (COVID-19). Clin Drug Invest. (2020) 40:591–601. 10.1007/s40261-020-00927-1 [ DOI ] [ PMC free article ] [ PubMed ] [ Google Scholar ]
- 50. Cruciani M, Pati I, Masiello F, Malena M, Pupella S, De Angelis V. Ivermectin for prophylaxis and treatment of COVID-19: a systematic review and meta-analysis. Diagnostics. (2021) 11:1645. 10.3390/diagnostics11091645 [ DOI ] [ PMC free article ] [ PubMed ] [ Google Scholar ]
- 51. Conti V, Corbi G, Sellitto C, Sabbatino F, Maci C, Bertini N, et al. Effect of tocilizumab in reducing the mortality rate in covid-19 patients: a systematic review with meta-analysis. J Pers Med. (2021) 11:628. 10.3390/jpm11070628 [ DOI ] [ PMC free article ] [ PubMed ] [ Google Scholar ]
- 52. Bhattacharyya A, Kumar S, Sarma P, Kaur H, Prajapat M, Shekhar N, et al. Safety and efficacy of lopinavir/ritonavir combination in COVID-19: a systematic review, meta-analysis, and meta-regression analysis. Indian J Pharmacol. (2020) 52:313–23. [ DOI ] [ PMC free article ] [ PubMed ] [ Google Scholar ]
- 53. Bartoszko JJ, Siemieniuk RAC, Kum E, Qasim A, Zeraatkar D, Ge L, et al. Prophylaxis against covid-19: living systematic review and network meta-analysis. BMJ. (2021) 373:n949. 10.1136/bmj.n949 [ DOI ] [ PMC free article ] [ PubMed ] [ Google Scholar ]
- 54. Bansal V, Mahapure KS, Bhurwal A, Gupta I, Hassanain S, Makadia J, et al. Mortality benefit of remdesivir in COVID-19: a systematic review and meta-analysis. Front Med. (2021) 7:606429. 10.3389/fmed.2020.606429 [ DOI ] [ PMC free article ] [ PubMed ] [ Google Scholar ]
- 55. Ayele Mega T, Feyissa TM, Dessalegn Bosho D, Kumela Goro K, Zeleke Negera G. The outcome of hydroxychloroquine in patients treated for COVID-19: systematic review and meta-analysis. Can Respir J. (2020) 2020:4312519. 10.1155/2020/4312519 [ DOI ] [ PMC free article ] [ PubMed ] [ Google Scholar ]
- 56. Angamo MT, Mohammed MA, Peterson GM. Efficacy and safety of remdesivir in hospitalised COVID-19 patients: a systematic review and meta-analysis. Infection. (2021) 50:27–41. 10.1007/s15010-021-01671-0 [ DOI ] [ PMC free article ] [ PubMed ] [ Google Scholar ]
- 57. Al-Abdouh A, Bizanti A, Barbarawi M, Jabri A, Kumar A, Fashanu OE, et al. Remdesivir for the treatment of COVID-19: a systematic review and meta-analysis of randomized controlled trials. Contemp Clin Trials. (2021) 101:106272. 10.1016/j.cct.2021.106272 [ DOI ] [ PMC free article ] [ PubMed ] [ Google Scholar ]
- 58. Abdelrahman Z, Liu Q, Jiang S, Li M, Sun Q, Zhang Y, et al. Evaluation of the current therapeutic approaches for COVID-19: a systematic review and a meta-analysis. Front Pharmacol. (2021) 12:607408. 10.3389/fphar.2021.607408 [ DOI ] [ PMC free article ] [ PubMed ] [ Google Scholar ]
- 59. O Murchu E, Spillane S, Byrne P, O’Neill M, Harrington P, Ryan M. Interventions in an ambulatory setting to prevent progression to severe disease in patients with COVID-19: a systematic review. Ann. Pharmacother. (2021) 56:309–18. [ DOI ] [ PubMed ] [ Google Scholar ]
- 60. Baker KA, Weeks SM. An overview of systematic review. J Perianesth Nurs. (2014) 29:454–8. 10.1016/j.jopan.2014.07.002 [ DOI ] [ PubMed ] [ Google Scholar ]
- 61. Leucht S, Kissling W, Davis J. How to read and understand and use systematic reviews and meta-analyses. Acta Psychiatr Scand. (2009) 119:443–50. 10.1111/j.1600-0447.2009.01388.x [ DOI ] [ PubMed ] [ Google Scholar ]
- 62. Mulrow CD. Systematic reviews: rationale for systematic reviews. BMJ. (1994) 309:597–9. 10.1136/bmj.309.6954.597 [ DOI ] [ PMC free article ] [ PubMed ] [ Google Scholar ]
- 63. Cook DJ, Mulrow CD, Haynes RB. Systematic reviews: synthesis of best evidence for clinical decisions. Ann Internal Med. (1997) 126:376–80. 10.7326/0003-4819-126-5-199703010-00006 [ DOI ] [ PubMed ] [ Google Scholar ]
- 64. Owens JK. Systematic reviews: brief overview of methods, limitations, and resources. Nurse Author Ed. (2021) 31:69–72. 10.1111/nae2.28 [ DOI ] [ Google Scholar ]
- 65. Bartolucci AA, Hillegass WB. Overview, strengths, and limitations of systematic reviews and meta-analyses. In: Chiappelli F. editor. Evidence-Based Practice: Toward Optimizing Clinical Outcomes. Berlin: Springer; (2010). p. 17–33. 10.1007/978-3-642-05025-1_2 [ DOI ] [ Google Scholar ]
- 66. Wurth R, Hajdenberg M, Barrera FJ, Shekhar S, Copacino CE, Moreno-Peña PJ, et al. Scoping review of COVID-19-related systematic reviews and meta-analyses: can we really have confidence in their results? Postgrad Med J. (2022) 98:372–9. 10.1136/postgradmedj-2020-139392 [ DOI ] [ PMC free article ] [ PubMed ] [ Google Scholar ]
- 67. Nothacker J, Stadelmaier J, Siemens W, Meerpohl JJ, Schmucker C. Characteristics of registered and published systematic reviews focusing on the prevention of COVID-19: a meta-research study. BMJ Open. (2022) 12:e060255. 10.1136/bmjopen-2021-060255 [ DOI ] [ PMC free article ] [ PubMed ] [ Google Scholar ]
Associated Data
This section collects any data citations, data availability statements, or supplementary materials included in this article.
Supplementary Materials
Data availability statement.
- View on publisher site
- PDF (1.3 MB)
- Collections
Similar articles
Cited by other articles, links to ncbi databases.
- Download .nbib .nbib
- Format: AMA APA MLA NLM
Add to Collections
