Single-Case Design, Analysis, and Quality Assessment for Intervention Research
Affiliation.
- 1 Biomechanics & Movement Science Program, Department of Physical Therapy, University of Delaware, Newark, Delaware (M.A.L., A.B.C., I.B.); and Division of Educational Psychology & Methodology, State University of New York at Albany, Albany, New York (M.M.).
- PMID: 28628553
- PMCID: PMC5492992
- DOI: 10.1097/NPT.0000000000000187
Background and purpose: The purpose of this article is to describe single-case studies and contrast them with case studies and randomized clinical trials. We highlight current research designs, analysis techniques, and quality appraisal tools relevant for single-case rehabilitation research.
Summary of key points: Single-case studies can provide a viable alternative to large group studies such as randomized clinical trials. Single-case studies involve repeated measures and manipulation of an independent variable. They can be designed to have strong internal validity for assessing causal relationships between interventions and outcomes, as well as external validity for generalizability of results, particularly when the study designs incorporate replication, randomization, and multiple participants. Single-case studies should not be confused with case studies/series (ie, case reports), which are reports of clinical management of a patient or a small series of patients.
Recommendations for clinical practice: When rigorously designed, single-case studies can be particularly useful experimental designs in a variety of situations, such as when research resources are limited, studied conditions have low incidences, or when examining effects of novel or expensive interventions. Readers will be directed to examples from the published literature in which these techniques have been discussed, evaluated for quality, and implemented.
- Cohort Studies
- Medical Records*
- Quality Assurance, Health Care*
- Randomized Controlled Trials as Topic
- Research Design*

Grants and funding
- R21 HD076092/HD/NICHD NIH HHS/United States

An official website of the United States government
The .gov means it’s official. Federal government websites often end in .gov or .mil. Before sharing sensitive information, make sure you’re on a federal government site.
The site is secure. The https:// ensures that you are connecting to the official website and that any information you provide is encrypted and transmitted securely.
- Publications
- Account settings
Preview improvements coming to the PMC website in October 2024. Learn More or Try it out now .
- Advanced Search
- Journal List
- HHS Author Manuscripts

Single-Case Design, Analysis, and Quality Assessment for Intervention Research
Michele a. lobo.
1 Biomechanics & Movement Science Program, Department of Physical Therapy, University of Delaware, Newark, DE, USA
Mariola Moeyaert
2 Division of Educational Psychology & Methodology, State University of New York at Albany, Albany, NY, USA
Andrea Baraldi Cunha
Iryna babik, background and purpose.
The purpose of this article is to describe single-case studies, and contrast them with case studies and randomized clinical trials. We will highlight current research designs, analysis techniques, and quality appraisal tools relevant for single-case rehabilitation research.
Summary of Key Points
Single-case studies can provide a viable alternative to large group studies such as randomized clinical trials. Single case studies involve repeated measures, and manipulation of and independent variable. They can be designed to have strong internal validity for assessing causal relationships between interventions and outcomes, and external validity for generalizability of results, particularly when the study designs incorporate replication, randomization, and multiple participants. Single case studies should not be confused with case studies/series (ie, case reports), which are reports of clinical management of one patient or a small series of patients.
Recommendations for Clinical Practice
When rigorously designed, single-case studies can be particularly useful experimental designs in a variety of situations, even when researcher resources are limited, studied conditions have low incidences, or when examining effects of novel or expensive interventions. Readers will be directed to examples from the published literature in which these techniques have been discussed, evaluated for quality, and implemented.
Introduction
The purpose of this article is to present current tools and techniques relevant for single-case rehabilitation research. Single-case (SC) studies have been identified by a variety of names, including “n of 1 studies” and “single-subject” studies. The term “single-case study” is preferred over the previously mentioned terms because previous terms suggest these studies include only one participant. In fact, as will be discussed below, for purposes of replication and improved generalizability, the strongest SC studies commonly include more than one participant.
A SC study should not be confused with a “case study/series “ (also called “case report”. In a typical case study/series, a single patient or small series of patients is involved, but there is not a purposeful manipulation of an independent variable, nor are there necessarily repeated measures. Most case studies/series are reported in a narrative way while results of SC studies are presented numerically or graphically. 1 , 2 This article defines SC studies, contrasts them with randomized clinical trials, discusses how they can be used to scientifically test hypotheses, and highlights current research designs, analysis techniques, and quality appraisal tools that may be useful for rehabilitation researchers.
In SC studies, measurements of outcome (dependent variables) are recorded repeatedly for individual participants across time and varying levels of an intervention (independent variables). 1 – 5 These varying levels of intervention are referred to as “phases” with one phase serving as a baseline or comparison, so each participant serves as his/her own control. 2 In contrast to case studies and case series in which participants are observed across time without experimental manipulation of the independent variable, SC studies employ systematic manipulation of the independent variable to allow for hypothesis testing. 1 , 6 As a result, SC studies allow for rigorous experimental evaluation of intervention effects and provide a strong basis for establishing causal inferences. Advances in design and analysis techniques for SC studies observed in recent decades have made SC studies increasingly popular in educational and psychological research. Yet, the authors believe SC studies have been undervalued in rehabilitation research, where randomized clinical trials (RCTs) are typically recommended as the optimal research design to answer questions related to interventions. 7 In reality, there are advantages and disadvantages to both SC studies and RCTs that should be carefully considered in order to select the best design to answer individual research questions. While there are a variety of other research designs that could be utilized in rehabilitation research, only SC studies and RCTs are discussed here because SC studies are the focus of this article and RCTs are the most highly recommended design for intervention studies. 7
When designed and conducted properly, RCTs offer strong evidence that changes in outcomes may be related to provision of an intervention. However, RCTs require monetary, time, and personnel resources that many researchers, especially those in clinical settings, may not have available. 8 RCTs also require access to large numbers of consenting participants that meet strict inclusion and exclusion criteria that can limit variability of the sample and generalizability of results. 9 The requirement for large participant numbers may make RCTs difficult to perform in many settings, such as rural and suburban settings, and for many populations, such as those with diagnoses marked by lower prevalence. 8 To rely exclusively on RCTs has the potential to result in bodies of research that are skewed to address the needs of some individuals while neglecting the needs of others. RCTs aim to include a large number of participants and to use random group assignment to create study groups that are similar to one another in terms of all potential confounding variables, but it is challenging to identify all confounding variables. Finally, the results of RCTs are typically presented in terms of group means and standard deviations that may not represent true performance of any one participant. 10 This can present as a challenge for clinicians aiming to translate and implement these group findings at the level of the individual.
SC studies can provide a scientifically rigorous alternative to RCTs for experimentally determining the effectiveness of interventions. 1 , 2 SC studies can assess a variety of research questions, settings, cases, independent variables, and outcomes. 11 There are many benefits to SC studies that make them appealing for intervention research. SC studies may require fewer resources than RCTs and can be performed in settings and with populations that do not allow for large numbers of participants. 1 , 2 In SC studies, each participant serves as his/her own comparison, thus controlling for many confounding variables that can impact outcome in rehabilitation research, such as gender, age, socioeconomic level, cognition, home environment, and concurrent interventions. 2 , 11 Results can be analyzed and presented to determine whether interventions resulted in changes at the level of the individual, the level at which rehabilitation professionals intervene. 2 , 12 When properly designed and executed, SC studies can demonstrate strong internal validity to determine the likelihood of a causal relationship between the intervention and outcomes and external validity to generalize the findings to broader settings and populations. 2 , 12 , 13
Single Case Research Designs for Intervention Research
There are a variety of SC designs that can be used to study the effectiveness of interventions. Here we discuss: 1) AB designs, 2) reversal designs, 3) multiple baseline designs, and 4) alternating treatment designs, as well as ways replication and randomization techniques can be used to improve internal validity of all of these designs. 1 – 3 , 12 – 14
The simplest of these designs is the AB Design 15 ( Figure 1 ). This design involves repeated measurement of outcome variables throughout a baseline control/comparison phase (A ) and then throughout an intervention phase (B). When possible, it is recommended that a stable level and/or rate of change in performance be observed within the baseline phase before transitioning into the intervention phase. 2 As with all SC designs, it is also recommended that there be a minimum of five data points in each phase. 1 , 2 There is no randomization or replication of the baseline or intervention phases in the basic AB design. 2 Therefore, AB designs have problems with internal validity and generalizability of results. 12 They are weak in establishing causality because changes in outcome variables could be related to a variety of other factors, including maturation, experience, learning, and practice effects. 2 , 12 Sample data from a single case AB study performed to assess the impact of Floor Play intervention on social interaction and communication skills for a child with autism 15 are shown in Figure 1 .

An example of results from a single-case AB study conducted on one participant with autism; two weeks of observation (baseline phase A) were followed by seven weeks of Floor Time Play (intervention phase B). The outcome measure Circles of Communications (reciprocal communication with two participants responding to each other verbally or nonverbally) served as a behavioral indicator of the child’s social interaction and communication skills (higher scores indicating better performance). A statistically significant improvement in Circles of Communication was found during the intervention phase as compared to the baseline. Note that although a stable baseline is recommended for SC studies, it is not always possible to satisfy this requirement, as you will see in Figures 1 – 4 . Data were extracted from Dionne and Martini (2011) 15 utilizing Rohatgi’s WebPlotDigitizer software. 78
If an intervention does not have carry-over effects, it is recommended to use a Reversal Design . 2 For example, a reversal A 1 BA 2 design 16 ( Figure 2 ) includes alternation of the baseline and intervention phases, whereas a reversal A 1 B 1 A 2 B 2 design 17 ( Figure 3 ) consists of alternation of two baseline (A 1 , A 2 ) and two intervention (B 1 , B 2 ) phases. Incorporating at least four phases in the reversal design (i.e., A 1 B 1 A 2 B 2 or A 1 B 1 A 2 B 2 A 3 B 3 …) allows for a stronger determination of a causal relationship between the intervention and outcome variables, because the relationship can be demonstrated across at least three different points in time – change in outcome from A 1 to B 1 , from B 1 to A 2 , and from A 2 to B 2 . 18 Before using this design, however, researchers must determine that it is safe and ethical to withdraw the intervention, especially in cases where the intervention is effective and necessary. 12

An example of results from a single-case A 1 BA 2 study conducted on eight participants with stable multiple sclerosis (data on three participants were used for this example). Four weeks of observation (baseline phase A 1 ) were followed by eight weeks of core stability training (intervention phase B), then another four weeks of observation (baseline phase A 2 ). Forward functional reach test (the maximal distance the participant can reach forward or lateral beyond arm’s length, maintaining a fixed base of support in the standing position; higher scores indicating better performance) significantly improved during intervention for Participants 1 and 3 without further improvement observed following withdrawal of the intervention (during baseline phase A 2 ). Data were extracted from Freeman et al. (2010) 16 utilizing Rohatgi’s WebPlotDigitizer software. 78

An example of results from a single-case A 1 B 1 A 2 B 2 study conducted on two participants with severe unilateral neglect after a right-hemisphere stroke. Two weeks of conventional treatment (baseline phases A 1, A 2 ) alternated with two weeks of visuo-spatio-motor cueing (intervention phases B 1 , B 2 ). Performance was assessed in two tests of lateral neglect, the Bells Cancellation Test (Figure A; lower scores indicating better performance) and the Line Bisection Test (Figure B; higher scores indicating better performance). There was a statistically significant intervention-related improvement in participants’ performance on the Line Bisection Test, but not on the Bells Test. Data were extracted from Samuel at al. (2000) 17 utilizing Rohatgi’s WebPlotDigitizer software. 78
A recent study used an ABA reversal SC study to determine the effectiveness of core stability training in 8 participants with multiple sclerosis. 16 During the first four weekly data collections, the researchers ensured a stable baseline, which was followed by eight weekly intervention data points, and concluded with four weekly withdrawal data points. Intervention significantly improved participants’ walking and reaching performance ( Figure 2 ). 16 This A 1 BA 2 design could have been strengthened by the addition of a second intervention phase for replication (A 1 B 1 A 2 B 2 ). For instance, a single-case A 1 B 1 A 2 B 2 withdrawal design aimed to assess the efficacy of rehabilitation using visuo-spatio-motor cueing for two participants with severe unilateral neglect after a severe right-hemisphere stroke. 17 Each phase included 8 data points. Statistically significant intervention-related improvement was observed, suggesting that visuo-spatio-motor cueing might be promising for treating individuals with very severe neglect ( Figure 3 ). 17
The reversal design can also incorporate a cross over design where each participant experiences more than one type of intervention. For instance, a B 1 C 1 B 2 C 2 design could be used to study the effects of two different interventions (B and C) on outcome measures. Challenges with including more than one intervention involve potential carry-over effects from earlier interventions and order effects that may impact the measured effectiveness of the interventions. 2 , 12 Including multiple participants and randomizing the order of intervention phase presentations are tools to help control for these types of effects. 19
When an intervention permanently changes an individual’s ability, a return to baseline performance is not feasible and reversal designs are not appropriate. Multiple Baseline Designs (MBDs) are useful in these situations ( Figure 4 ). 20 MBDs feature staggered introduction of the intervention across time: each participant is randomly assigned to one of at least 3 experimental conditions characterized by the length of the baseline phase. 21 These studies involve more than one participant, thus functioning as SC studies with replication across participants. Staggered introduction of the intervention allows for separation of intervention effects from those of maturation, experience, learning, and practice. For example, a multiple baseline SC study was used to investigate the effect of an anti-spasticity baclofen medication on stiffness in five adult males with spinal cord injury. 20 The subjects were randomly assigned to receive 5–9 baseline data points with a placebo treatment prior to the initiation of the intervention phase with the medication. Both participants and assessors were blind to the experimental condition. The results suggested that baclofen might not be a universal treatment choice for all individuals with spasticity resulting from a traumatic spinal cord injury ( Figure 4 ). 20

An example of results from a single-case multiple baseline study conducted on five participants with spasticity due to traumatic spinal cord injury. Total duration of data collection was nine weeks. The first participant was switched from placebo treatment (baseline) to baclofen treatment (intervention) after five data collection sessions, whereas each consecutive participant was switched to baclofen intervention at the subsequent sessions through the ninth session. There was no statistically significant effect of baclofen on viscous stiffness at the ankle joint. Data were extracted from Hinderer at al. (1990) 20 utilizing Rohatgi’s WebPlotDigitizer software. 78
The impact of two or more interventions can also be assessed via Alternating Treatment Designs (ATDs) . In ATDs, after establishing the baseline, the experimenter exposes subjects to different intervention conditions administered in close proximity for equal intervals ( Figure 5 ). 22 ATDs are prone to “carry-over effects” when the effects of one intervention influence the observed outcomes of another intervention. 1 As a result, such designs introduce unique challenges when attempting to determine the effects of any one intervention and have been less commonly utilized in rehabilitation. An ATD was used to monitor disruptive behaviors in the school setting throughout a baseline followed by an alternating treatment phase with randomized presentation of a control condition or an exercise condition. 23 Results showed that 30 minutes of moderate to intense physical activity decreased behavioral disruptions through 90 minutes after the intervention. 23 An ATD was also used to compare the effects of commercially available and custom-made video prompts on the performance of multi-step cooking tasks in four participants with autism. 22 Results showed that participants independently performed more steps with the custom-made video prompts ( Figure 5 ). 22

An example of results from a single case alternating treatment study conducted on four participants with autism (data on two participants were used for this example). After the observation phase (baseline), effects of commercially available and custom-made video prompts on the performance of multi-step cooking tasks were identified (treatment phase), after which only the best treatment was used (best treatment phase). Custom-made video prompts were most effective for improving participants’ performance of multi-step cooking tasks. Data were extracted from Mechling at al. (2013) 22 utilizing Rohatgi’s WebPlotDigitizer software. 78
Regardless of the SC study design, replication and randomization should be incorporated when possible to improve internal and external validity. 11 The reversal design is an example of replication across study phases. The minimum number of phase replications needed to meet quality standards is three (A 1 B 1 A 2 B 2 ), but having four or more replications is highly recommended (A 1 B 1 A 2 B 2 A 3 …). 11 , 14 In cases when interventions aim to produce lasting changes in participants’ abilities, replication of findings may be demonstrated by replicating intervention effects across multiple participants (as in multiple-participant AB designs), or across multiple settings, tasks, or service providers. When the results of an intervention are replicated across multiple reversals, participants, and/or contexts, there is an increased likelihood a causal relationship exists between the intervention and the outcome. 2 , 12
Randomization should be incorporated in SC studies to improve internal validity and the ability to assess for causal relationships among interventions and outcomes. 11 In contrast to traditional group designs, SC studies often do not have multiple participants or units that can be randomly assigned to different intervention conditions. Instead, in randomized phase-order designs , the sequence of phases is randomized. Simple or block randomization is possible. For example, with simple randomization for an A 1 B 1 A 2 B 2 design, the A and B conditions are treated as separate units and are randomly assigned to be administered for each of the pre-defined data collection points. As a result, any combination of A-B sequences is possible without restrictions on the number of times each condition is administered or regard for repetitions of conditions (e.g., A 1 B 1 B 2 A 2 B 3 B 4 B 5 A 3 B 6 A 4 A 5 A 6 ). With block randomization for an A 1 B 1 A 2 B 2 design, two conditions (e.g., A and B) would be blocked into a single unit (AB or BA), randomization of which to different time periods would ensure that each condition appears in the resulting sequence more than two times (e.g., A 1 B 1 B 2 A 2 A 3 B 3 A 4 B 4 ). Note that AB and reversal designs require that the baseline (A) always precedes the first intervention (B), which should be accounted for in the randomization scheme. 2 , 11
In randomized phase start-point designs , the lengths of the A and B phases can be randomized. 2 , 11 , 24 – 26 For example, for an AB design, researchers could specify the number of time points at which outcome data will be collected, (e.g., 20), define the minimum number of data points desired in each phase (e.g., 4 for A, 3 for B), and then randomize the initiation of the intervention so that it occurs anywhere between the remaining time points (points 5 and 17 in the current example). 27 , 28 For multiple-baseline designs, a dual-randomization, or “regulated randomization” procedure has been recommended. 29 If multiple-baseline randomization depends solely on chance, it could be the case that all units are assigned to begin intervention at points not really separated in time. 30 Such randomly selected initiation of the intervention would result in the drastic reduction of the discriminant and internal validity of the study. 29 To eliminate this issue, investigators should first specify appropriate intervals between the start points for different units, then randomly select from those intervals, and finally randomly assign each unit to a start point. 29
Single Case Analysis Techniques for Intervention Research
The What Works Clearinghouse (WWC) single-case design technical documentation provides an excellent overview of appropriate SC study analysis techniques to evaluate the effectiveness of intervention effects. 1 , 18 First, visual analyses are recommended to determine whether there is a functional relation between the intervention and the outcome. Second, if evidence for a functional effect is present, the visual analysis is supplemented with quantitative analysis methods evaluating the magnitude of the intervention effect. Third, effect sizes are combined across cases to estimate overall average intervention effects which contributes to evidence-based practice, theory, and future applications. 2 , 18
Visual Analysis
Traditionally, SC study data are presented graphically. When more than one participant engages in a study, a spaghetti plot showing all of their data in the same figure can be helpful for visualization. Visual analysis of graphed data has been the traditional method for evaluating treatment effects in SC research. 1 , 12 , 31 , 32 The visual analysis involves evaluating level, trend, and stability of the data within each phase (i.e., within-phase data examination) followed by examination of the immediacy of effect, consistency of data patterns, and overlap of data between baseline and intervention phases (i.e., between-phase comparisons). When the changes (and/or variability) in level are in the desired direction, are immediate, readily discernible, and maintained over time, it is concluded that the changes in behavior across phases result from the implemented treatment and are indicative of improvement. 33 Three demonstrations of an intervention effect are necessary for establishing a functional relation. 1
Within-phase examination
Level, trend, and stability of the data within each phase are evaluated. Mean and/or median can be used to report the level, and trend can be evaluated by determining whether the data points are monotonically increasing or decreasing. Within-phase stability can be evaluated by calculating the percentage of data points within 15% of the phase median (or mean). The stability criterion is satisfied if about 85% (80% – 90%) of the data in a phase fall within a 15% range of the median (or average) of all data points for that phase. 34
Between-phase examination
Immediacy of effect, consistency of data patterns, and overlap of data between baseline and intervention phases are evaluated next. For this, several nonoverlap indices have been proposed that all quantify the proportion of measurements in the intervention phase not overlapping with the baseline measurements. 35 Nonoverlap statistics are typically scaled as percent from 0 to 100, or as a proportion from 0 to 1. Here, we briefly discuss the Nonoverlap of All Pairs ( NAP ), 36 the Extended Celeration Line ( ECL ), the Improvement Rate Difference ( IRD) , 37 and the TauU and the TauU-adjusted, TauU adj , 35 as these are the most recent and complete techniques. We also examine the Percentage of Nonoverlapping Data ( PND ) 38 and the Two Standard Deviations Band Method, as these are frequently used techniques. In addition, we include the Percentage of Nonoverlapping Corrected Data ( PNCD ) – an index applying to the PND after controlling for baseline trend. 39
Nonoverlap of all pairs (NAP)
Each baseline observation can be paired with each intervention phase observation to make n pairs (i.e., N = n A * n B ). Count the number of overlapping pairs, n o , counting all ties as 0.5. Then define the percent of the pairs that show no overlap. Alternatively, one can count the number of positive (P), negative (N), and tied (T) pairs 2 , 36 :
Extended Celeration Line (ECL)
ECL or split middle line allows control for a positive Phase A trend. Nonoverlap is defined as the proportion of Phase B ( n b ) data that are above the median trend plotted from Phase A data ( n B< sub > Above Median trend A </ sub > ), but then extended into Phase B: ECL = n B Above Median trend A n b ∗ 100
As a consequence, this method depends on a straight line and makes an assumption of linearity in the baseline. 2 , 12
Improvement rate difference (IRD)
This analysis is conceptualized as the difference in improvement rates (IR) between baseline ( IR B ) and intervention phases ( IR T ). 38 The IR for each phase is defined as the number of “improved data points” divided by the total data points in that phase. IRD, commonly employed in medical group research under the name of “risk reduction” or “risk difference” attempts to provide an intuitive interpretation for nonoverlap and to make use of an established, respected effect size, IR B - IR B , or the difference between two proportions. 37
TauU and TauU adj
Each baseline observation can be paired with each intervention phase observation to make n pairs (i.e., n = n A * n B ). Count the number of positive (P), negative (N), and tied (T) pairs, and use the following formula: TauU = P - N P + N + τ
The TauU adj is an adjustment of TauU for monotonic trend in baseline. Each baseline observation can be paired with each intervention phase observation to make n pairs (i.e., n = n A * n B ). Each baseline observation can be paired with all later baseline observations (n A *(n A -1)/2). 2 , 35 Then the baseline trend can be computed: TauU adf = P - N - S trend P + N + τ ; S trend = P A – NA
Online calculators might assist researchers in obtaining the TauU and TauU adjusted coefficients ( http://www.singlecaseresearch.org/calculators/tau-u ).
Percentage of nonoverlapping data (PND)
If anticipating an increase in the outcome, locate the highest data point in the baseline phase and then calculate the percent of the intervention phase data points that exceed it. If anticipating a decrease in the outcome, find the lowest data point in the baseline phase and then calculate the percent of the treatment phase data points that are below it: PND = n B Overlap A n b ∗ 100 . A PND < 50 would mark no observed effect, PND = 50–70 signifies a questionable effect, and PND > 70 suggests the intervention was effective. 40 The percentage of nonoverlapping (PNDC) corrected was proposed in 2009 as an extension of the PND. 39 Prior to applying the PND, a data correction procedure is applied eliminating pre-existing baseline trend. 38
Two Standard Deviation Band Method
When the stability criterion described above is met within phases, it is possible to apply the two standard deviation band method. 12 , 41 First, the mean of the data for a specific condition is calculated and represented with a solid line. In the next step, the standard deviation of the same data is computed and two dashed lines are represented: one located two standard deviations above the mean and the other – two standard deviations below. For normally distributed data, few points (less than 5%) are expected to be outside the two standard deviation bands if there is no change in the outcome score due to the intervention. However, this method is not considered a formal statistical procedure, as the data cannot typically be assumed to be normal, continuous, or independent. 41
Statistical Analysis
If the visual analysis indicates a functional relationship (i.e., three demonstrations of the effectiveness of the intervention effect), it is recommended to proceed with the quantitative analyses, reflecting the magnitude of the intervention effect. First, effect sizes are calculated for each participant (individual-level analysis). Moreover, if the research interest lies in the generalizability of the effect size across participants, effect sizes can be combined across cases to achieve an overall average effect size estimate (across-case effect size).
Note that quantitative analysis methods are still being developed in the domain of SC research 1 and statistical challenges of producing an acceptable measure of treatment effect remain. 14 , 42 , 43 Therefore, the WWC standards strongly recommend conducting sensitivity analysis and reporting multiple effect size estimators. If consistency across different effect size estimators is identified, there is stronger evidence for the effectiveness of the treatment. 1 , 18
Individual-level effect size analysis
The most common effect sizes recommended for SC analysis are: 1) standardized mean difference Cohen’s d ; 2) standardized mean difference with correction for small sample sizes Hedges’ g ; and 3) the regression-based approach which has the most potential and is strongly recommended by the WWC standards. 1 , 44 , 45 Cohen’s d can be calculated using following formula: d = X A ¯ - X B ¯ s p , with X A ¯ being the baseline mean, X B ¯ being the treatment mean, and s p indicating the pooled within-case standard deviation. Hedges’ g is an extension of Cohen’s d , recommended in the context of SC studies as it corrects for small sample sizes. The piecewise regression-based approach does not only reflect the immediate intervention effect, but also the intervention effect across time:
i stands for the measurement occasion ( i = 0, 1,… I ). The dependent variable is regressed on a time indicator, T , which is centered around the first observation of the intervention phase, D , a dummy variable for the intervention phase, and an interaction term of these variables. The equation shows that the expected score, Ŷ i , equals β 0 + β 1 T i in the baseline phase, and ( β 0 + β 2 ) + ( β 1 + β 3 ) T i in the intervention phase. β 0 , therefore, indicates the expected baseline level at the start of the intervention phase (when T = 0), whereas β 1 marks the linear time trend in the baseline scores. The coefficient β 2 can then be interpreted as an immediate effect of the intervention on the outcome, whereas β 3 signifies the effect of the intervention across time. The e i ’s are residuals assumed to be normally distributed around a mean of zero with a variance of σ e 2 . The assumption of independence of errors is usually not met in the context of SC studies because repeated measures are obtained within a person. As a consequence, it can be the case that the residuals are autocorrelated, meaning that errors closer in time are more related to each other compared to errors further away in time. 46 – 48 As a consequence, a lag-1 autocorrelation is appropriate (taking into account the correlation between two consecutive errors: e i and e i –1 ; for more details see Verbeke & Molenberghs, (2000). 49 In Equation 1 , ρ indicates the autocorrelation parameter. If ρ is positive, the errors closer in time are more similar; if ρ is negative, the errors closer in time are more different, and if ρ equals zero, there is no correlation between the errors.
Across-case effect sizes
Two-level modeling to estimate the intervention effects across cases can be used to evaluate across-case effect sizes. 44 , 45 , 50 Multilevel modeling is recommended by the WWC standards because it takes the hierarchical nature of SC studies into account: measurements are nested within cases and cases, in turn, are nested within studies. By conducting a multilevel analysis, important research questions can be addressed (which cannot be answered by single-level analysis of SC study data), such as: 1) What is the magnitude of the average treatment effect across cases? 2) What is the magnitude and direction of the case-specific intervention effect? 3) How much does the treatment effect vary within cases and across cases? 4) Does a case and/or study level predictor influence the treatment’s effect? The two-level model has been validated in previous research using extensive simulation studies. 45 , 46 , 51 The two-level model appears to have sufficient power (> .80) to detect large treatment effects in at least six participants with six measurements. 21
Furthermore, to estimate the across-case effect sizes, the HPS (Hedges, Pustejovsky, and Shadish) , or single-case educational design ( SCEdD)-specific mean difference, index can be calculated. 52 This is a standardized mean difference index specifically designed for SCEdD data, with the aim of making it comparable to Cohen’s d of group-comparison designs. The standard deviation takes into account both within-participant and between-participant variability, and is typically used to get an across-case estimator for a standardized change in level. The advantage of using the HPS across-case effect size estimator is that it is directly comparable with Cohen’s d for group comparison research, thus enabling the use of Cohen’s (1988) benchmarks. 53
Valuable recommendations on SC data analyses have recently been provided. 54 , 55 They suggest that a specific SC study data analytic technique can be chosen based on: (1) the study aims and the desired quantification (e.g., overall quantification, between-phase quantifications, randomization, etc.), (2) the data characteristics as assessed by visual inspection and the assumptions one is willing to make about the data, and (3) the knowledge and computational resources. 54 , 55 Table 1 lists recommended readings and some commonly used resources related to the design and analysis of single-case studies.
Recommend readings and resources related to the design and analysis of single-case studies.
Quality Appraisal Tools for Single-Case Design Research
Quality appraisal tools are important to guide researchers in designing strong experiments and conducting high-quality systematic reviews of the literature. Unfortunately, quality assessment tools for SC studies are relatively novel, ratings across tools demonstrate variability, and there is currently no “gold standard” tool. 56 Table 2 lists important SC study quality appraisal criteria compiled from the most common scales; when planning studies or reviewing the literature, we recommend readers consider these criteria. Table 3 lists some commonly used SC quality assessment and reporting tools and references to resources where the tools can be located.
Summary of important single-case study quality appraisal criteria.
Quality assessment and reporting tools related to single-case studies.
When an established tool is required for systematic review, we recommend use of the What Works Clearinghouse (WWC) Tool because it has well-defined criteria and is developed and supported by leading experts in the SC research field in association with the Institute of Education Sciences. 18 The WWC documentation provides clear standards and procedures to evaluate the quality of SC research; it assesses the internal validity of SC studies, classifying them as “Meeting Standards”, “Meeting Standards with Reservations”, or “Not Meeting Standards”. 1 , 18 Only studies classified in the first two categories are recommended for further visual analysis. Also, WWC evaluates the evidence of effect, classifying studies into “Strong Evidence of a Causal Relation”, “Moderate Evidence of a Causal Relation”, or “No Evidence of a Causal Relation”. Effect size should only be calculated for studies providing strong or moderate evidence of a causal relation.
The Single-Case Reporting Guideline In BEhavioural Interventions (SCRIBE) 2016 is another useful SC research tool developed recently to improve the quality of single-case designs. 57 SCRIBE consists of a 26-item checklist that researchers need to address while reporting the results of SC studies. This practical checklist allows for critical evaluation of SC studies during study planning, manuscript preparation, and review.
Single-case studies can be designed and analyzed in a rigorous manner that allows researchers strength in assessing causal relationships among interventions and outcomes, and in generalizing their results. 2 , 12 These studies can be strengthened via incorporating replication of findings across multiple study phases, participants, settings, or contexts, and by using randomization of conditions or phase lengths. 11 There are a variety of tools that can allow researchers to objectively analyze findings from SC studies. 56 While a variety of quality assessment tools exist for SC studies, they can be difficult to locate and utilize without experience, and different tools can provide variable results. The WWC quality assessment tool is recommended for those aiming to systematically review SC studies. 1 , 18
SC studies, like all types of study designs, have a variety of limitations. First, it can be challenging to collect at least five data points in a given study phase. This may be especially true when traveling for data collection is difficult for participants, or during the baseline phase when delaying intervention may not be safe or ethical. Power in SC studies is related to the number of data points gathered for each participant so it is important to avoid having a limited number of data points. 12 , 58 Second, SC studies are not always designed in a rigorous manner and, thus, may have poor internal validity. This limitation can be overcome by addressing key characteristics that strengthen SC designs ( Table 2 ). 1 , 14 , 18 Third, SC studies may have poor generalizability. This limitation can be overcome by including a greater number of participants, or units. Fourth, SC studies may require consultation from expert methodologists and statisticians to ensure proper study design and data analysis, especially to manage issues like autocorrelation and variability of data. 2 Fifth, while it is recommended to achieve a stable level and rate of performance throughout the baseline, human performance is quite variable and can make this requirement challenging. Finally, the most important validity threat to SC studies is maturation. This challenge must be considered during the design process in order to strengthen SC studies. 1 , 2 , 12 , 58
SC studies can be particularly useful for rehabilitation research. They allow researchers to closely track and report change at the level of the individual. They may require fewer resources and, thus, can allow for high-quality experimental research, even in clinical settings. Furthermore, they provide a tool for assessing causal relationships in populations and settings where large numbers of participants are not accessible. For all of these reasons, SC studies can serve as an effective method for assessing the impact of interventions.
Acknowledgments
This research was supported by the National Institute of Health, Eunice Kennedy Shriver National Institute of Child Health & Human Development (1R21HD076092-01A1, Lobo PI) and the Delaware Economic Development Office (Grant #109).
Some of the information in this manuscript was presented at the IV Step Meeting in Columbus, OH, June 2016.
Evidence-Based Instructional Practices
Single Case Analysis and Review Framework
To download an Excel file that can be used for scoring via SCARF, click below:
To download an Excel file that can be used to automatically generate inter-assessor agreement (IAA), click below. See the bottom of the page for citations that should be used for the SCARF and the accompanying IAA tool.
The SCARF is intended as a tool to assess the quality and outcomes of single case design studies. For the purposes of the tool, “study” refers to any single design, which may include a single participant (e.g., A-B-A-B designs) or multiple participants (e.g., multiple baseline design across participants). Each design should be evaluated separately, even if multiple designs are present in a single source.
Note: This tool is designed for the assessment of groups of articles for the purposes of answering the question: To what extent are studies sufficient and to what extent are outcomes consistent and replicated for Intervention X for changing Behavior Y for Participants with Z inclusion characteristics? Studies should be included or excluded based on your research questions. Coding Study Characteristics and Primary Outcomes
Internal Validity : The first scale is for assessing the extent to which a study has adequate internal validity. There are 13 items, for a “best” internal validity score of 13, and a “worst” of 0. The higher the validity score, the more confident you can generally be in conclusions drawn from the study, although all items may not be equally important.
External Validity : The second scale is for assessing the extent to which study outcomes may be important outside of study contexts (e.g., the extent to which studies include generalization data, evidence of social validity, maintenance data, endogenous implementers, typical contexts). Some of these indicators may not be relevant in a given review.
Reporting: The third scale is for assessing the extent to which study authors reported necessary information for replication.
Outcomes : Primary outcomes are coded based on the consistency of outcomes across potential demonstrations. A score of 4 is given for consistent positive effects, a score of 0 is given for counter-therapeutic effects. Scores of 1, 2, and 3 indicate null, inconsistent, or weak positive effects. Scores of 3 and 4 are consistent with functional relation identification.
Graphical Representations
Internal Validity : This is a raw score denoting the number of internal validity features are present. Scores closer to the right side of the graph represent high-quality studies, which scores closer to the left axis represent studies with fewer internal validity features. These studies (to the left) may provide less convincing evidence, although if all evidence across internal validity scores (e.g., low and high quality studies) is similar, it may suggest these studies may include valid results.
Primary Outcomes : (range 0-4) = Score coded by reviewer, based on visual analysis
Generalization Measurement: Reviewers code the nature of generalization measurement, with more consistent data collection throughout the study resulting in more confidence in outcomes.
Generalization Outcomes : Similar to primary outcomes, reviewers score generalized outcomes for strong, weak, inconsistent, null, or counter-therapeutic effects.
Maintenance Measurement : We evaluate the extent to which maintenance measurement is separated in time from intervention including no measurement, immediate measurement (i.e., maintenance data are collected right after intervention is withdrawn), or data collected following specific periods of time after intervention is withdrawn.
Maintenance Outcomes : Similar to primary outcomes, reviewers score maintained outcomes for strong, weak, inconsistent, null, or counter-therapeutic effects.
Published Manuscripts using the SCARF
Barton, E. E., Murray, R., O’Flaherty, C., Sweeney, E. M., & Gossett, S. (2020). Teaching Object Play to Young Children With Disabilities: A Systematic Review of Methods and Rigor. American Journal on Intellectual and Developmental Disabilities , 125 (1), 14-36.
Chazin, K. T., Ledford, J. R., & Pak, N. (2020) Does it work, for whom, and under what conditions: A systematic review of moderators in augmented input interventions. Available at: https://osf.io/b3urx/
Chazin, K. T., Velez, M. S., & Ledford. J. R. (2020) Interventions without escape extinction to treat escape-maintained behavior: A systematic review and meta-analysis. Available at: https://osf.io/4nxvc/
Dubin, A. H., & Lieberman-Betz, R. G. (2020). Naturalistic Interventions to Improve Prelinguistic Communication for Children with Autism Spectrum Disorder: A Systematic Review. Review Journal of Autism and Developmental Disorders , 1-17.
Gibbs, A. R., & Tullis, C. A. (2020). The Emergence of Untrained Relations in Individuals with Autism and Other Intellectual and Developmental Disabilities: a Systematic Review of the Recent Literature. Review Journal of Autism and Developmental Disorders , 1-26.
Hardy, J. K., McLeod, R. H., Sweigart, C. A., & Landrum, T. (2022). Comparing and Contrasting Quality Frameworks Using Research on High-Probability Requests With Young Children. Infants & Young Children , 35 (4), 267-284.
Herrod, J. L., Snyder, S. K., Hart, J. B., Frantz, S. J., & Ayres, K. M. (2023). Applications of the Premack Principle: A review of the literature. Behavior Modification , 47 (1), 219-246.
Ledford, J. R., & Pustejovsky, J. E. (2020). Stay-play-talk Meta-analysis. Available at: https://osf.io/u7cph
Ledford, J. R., Trump, C., Chazin, K. T., Windsor, S. A., Eyler, P. B., & Wunderlich, K. (2023). Systematic review of interruption and redirection procedures for autistic individuals. Behavioral Interventions , 38 (1), 198-218.
Ledford, J. R., & Windsor, S. A. (2022). Systematic review of interventions designed to teach imitation to young children with disabilities. Topics in Early Childhood Special Education , 42 (2), 202-214. Available at: https://osf.io/wsjn4
Trump, C. E., Ayres, K. M., Quinland, K. K., & Zabala, K. A. (2019). Differential reinforcement without extinction: A review of the literature. Behavior Analysis: Research and Practice .
Zimmerman, K. N., & Ledford, J. R. (2017). Beyond ASD: Evidence for the effectiveness of social narratives. Journal of Early Intervention , 39 (3), 199-217.
Zimmerman, K. N., Ledford, J. R., Severini, K. E., Pustejovsky, J. E., Barton, E. E., & Lloyd, B. P. (2018). Single-case synthesis tools I: Comparing tools to evaluate SCD quality and rigor. Research in Developmental Disabilities , 79 , 19-32.
To cite the SCARF (v 3.1):
Ledford, J. R., Chazin, K. T., Lane, J. D., Zimmerman, K. N., Bennett, P. B., & Ayres, K. A. (2023, May). Single case analysis and review framework (SCARF). Retrieved from: http://ebip.vkcsites.org/scarfv2
To cite the SCARF IAA tool:
Todt, M. J. (2023, May). Single case analysis and review framework (SCARF) inter-assessor agreement tool. Retrieved from: http://ebip.vkcsites.org/scarfv2
Europe PMC requires Javascript to function effectively.
Either your web browser doesn't support Javascript or it is currently turned off. In the latter case, please turn on Javascript support in your web browser and reload this page.
Search life-sciences literature (44,160,791 articles, preprints and more)
- Free full text
- Citations & impact
- Similar Articles
Single-Case Design, Analysis, and Quality Assessment for Intervention Research.
Author information, affiliations, orcids linked to this article.
- Baraldi Cunha A | 0000-0003-0473-5402
- Babik I | 0000-0003-0574-4482
- Lobo MA | 0000-0003-2892-7687
Journal of Neurologic Physical Therapy : JNPT , 01 Jul 2017 , 41(3): 187-197 https://doi.org/10.1097/npt.0000000000000187 PMID: 28628553 PMCID: PMC5492992
Free full text in Europe PMC
Abstract
Background and purpose, summary of key points, recommendations for clinical practice, free full text .

Single-Case Design, Analysis, and Quality Assessment for Intervention Research
Michele a. lobo.
1 Biomechanics & Movement Science Program, Department of Physical Therapy, University of Delaware, Newark, DE, USA
Mariola Moeyaert
2 Division of Educational Psychology & Methodology, State University of New York at Albany, Albany, NY, USA
Andrea Baraldi Cunha
Iryna babik, background and purpose.
The purpose of this article is to describe single-case studies, and contrast them with case studies and randomized clinical trials. We will highlight current research designs, analysis techniques, and quality appraisal tools relevant for single-case rehabilitation research.
Summary of Key Points
Single-case studies can provide a viable alternative to large group studies such as randomized clinical trials. Single case studies involve repeated measures, and manipulation of and independent variable. They can be designed to have strong internal validity for assessing causal relationships between interventions and outcomes, and external validity for generalizability of results, particularly when the study designs incorporate replication, randomization, and multiple participants. Single case studies should not be confused with case studies/series (ie, case reports), which are reports of clinical management of one patient or a small series of patients.

Recommendations for Clinical Practice
When rigorously designed, single-case studies can be particularly useful experimental designs in a variety of situations, even when researcher resources are limited, studied conditions have low incidences, or when examining effects of novel or expensive interventions. Readers will be directed to examples from the published literature in which these techniques have been discussed, evaluated for quality, and implemented.
- Introduction
The purpose of this article is to present current tools and techniques relevant for single-case rehabilitation research. Single-case (SC) studies have been identified by a variety of names, including “n of 1 studies” and “single-subject” studies. The term “single-case study” is preferred over the previously mentioned terms because previous terms suggest these studies include only one participant. In fact, as will be discussed below, for purposes of replication and improved generalizability, the strongest SC studies commonly include more than one participant.
A SC study should not be confused with a “case study/series “ (also called “case report”. In a typical case study/series, a single patient or small series of patients is involved, but there is not a purposeful manipulation of an independent variable, nor are there necessarily repeated measures. Most case studies/series are reported in a narrative way while results of SC studies are presented numerically or graphically. 1 , 2 This article defines SC studies, contrasts them with randomized clinical trials, discusses how they can be used to scientifically test hypotheses, and highlights current research designs, analysis techniques, and quality appraisal tools that may be useful for rehabilitation researchers.
In SC studies, measurements of outcome (dependent variables) are recorded repeatedly for individual participants across time and varying levels of an intervention (independent variables). 1 – 5 These varying levels of intervention are referred to as “phases” with one phase serving as a baseline or comparison, so each participant serves as his/her own control. 2 In contrast to case studies and case series in which participants are observed across time without experimental manipulation of the independent variable, SC studies employ systematic manipulation of the independent variable to allow for hypothesis testing. 1 , 6 As a result, SC studies allow for rigorous experimental evaluation of intervention effects and provide a strong basis for establishing causal inferences. Advances in design and analysis techniques for SC studies observed in recent decades have made SC studies increasingly popular in educational and psychological research. Yet, the authors believe SC studies have been undervalued in rehabilitation research, where randomized clinical trials (RCTs) are typically recommended as the optimal research design to answer questions related to interventions. 7 In reality, there are advantages and disadvantages to both SC studies and RCTs that should be carefully considered in order to select the best design to answer individual research questions. While there are a variety of other research designs that could be utilized in rehabilitation research, only SC studies and RCTs are discussed here because SC studies are the focus of this article and RCTs are the most highly recommended design for intervention studies. 7
When designed and conducted properly, RCTs offer strong evidence that changes in outcomes may be related to provision of an intervention. However, RCTs require monetary, time, and personnel resources that many researchers, especially those in clinical settings, may not have available. 8 RCTs also require access to large numbers of consenting participants that meet strict inclusion and exclusion criteria that can limit variability of the sample and generalizability of results. 9 The requirement for large participant numbers may make RCTs difficult to perform in many settings, such as rural and suburban settings, and for many populations, such as those with diagnoses marked by lower prevalence. 8 To rely exclusively on RCTs has the potential to result in bodies of research that are skewed to address the needs of some individuals while neglecting the needs of others. RCTs aim to include a large number of participants and to use random group assignment to create study groups that are similar to one another in terms of all potential confounding variables, but it is challenging to identify all confounding variables. Finally, the results of RCTs are typically presented in terms of group means and standard deviations that may not represent true performance of any one participant. 10 This can present as a challenge for clinicians aiming to translate and implement these group findings at the level of the individual.
SC studies can provide a scientifically rigorous alternative to RCTs for experimentally determining the effectiveness of interventions. 1 , 2 SC studies can assess a variety of research questions, settings, cases, independent variables, and outcomes. 11 There are many benefits to SC studies that make them appealing for intervention research. SC studies may require fewer resources than RCTs and can be performed in settings and with populations that do not allow for large numbers of participants. 1 , 2 In SC studies, each participant serves as his/her own comparison, thus controlling for many confounding variables that can impact outcome in rehabilitation research, such as gender, age, socioeconomic level, cognition, home environment, and concurrent interventions. 2 , 11 Results can be analyzed and presented to determine whether interventions resulted in changes at the level of the individual, the level at which rehabilitation professionals intervene. 2 , 12 When properly designed and executed, SC studies can demonstrate strong internal validity to determine the likelihood of a causal relationship between the intervention and outcomes and external validity to generalize the findings to broader settings and populations. 2 , 12 , 13
- Single Case Research Designs for Intervention Research
There are a variety of SC designs that can be used to study the effectiveness of interventions. Here we discuss: 1) AB designs, 2) reversal designs, 3) multiple baseline designs, and 4) alternating treatment designs, as well as ways replication and randomization techniques can be used to improve internal validity of all of these designs. 1 – 3 , 12 – 14
The simplest of these designs is the AB Design 15 ( Figure 1 ). This design involves repeated measurement of outcome variables throughout a baseline control/comparison phase (A ) and then throughout an intervention phase (B). When possible, it is recommended that a stable level and/or rate of change in performance be observed within the baseline phase before transitioning into the intervention phase. 2 As with all SC designs, it is also recommended that there be a minimum of five data points in each phase. 1 , 2 There is no randomization or replication of the baseline or intervention phases in the basic AB design. 2 Therefore, AB designs have problems with internal validity and generalizability of results. 12 They are weak in establishing causality because changes in outcome variables could be related to a variety of other factors, including maturation, experience, learning, and practice effects. 2 , 12 Sample data from a single case AB study performed to assess the impact of Floor Play intervention on social interaction and communication skills for a child with autism 15 are shown in Figure 1 .

An example of results from a single-case AB study conducted on one participant with autism; two weeks of observation (baseline phase A) were followed by seven weeks of Floor Time Play (intervention phase B). The outcome measure Circles of Communications (reciprocal communication with two participants responding to each other verbally or nonverbally) served as a behavioral indicator of the child’s social interaction and communication skills (higher scores indicating better performance). A statistically significant improvement in Circles of Communication was found during the intervention phase as compared to the baseline. Note that although a stable baseline is recommended for SC studies, it is not always possible to satisfy this requirement, as you will see in Figures 1 – 4 . Data were extracted from Dionne and Martini (2011) 15 utilizing Rohatgi’s WebPlotDigitizer software. 78
If an intervention does not have carry-over effects, it is recommended to use a Reversal Design . 2 For example, a reversal A 1 BA 2 design 16 ( Figure 2 ) includes alternation of the baseline and intervention phases, whereas a reversal A 1 B 1 A 2 B 2 design 17 ( Figure 3 ) consists of alternation of two baseline (A 1 , A 2 ) and two intervention (B 1 , B 2 ) phases. Incorporating at least four phases in the reversal design (i.e., A 1 B 1 A 2 B 2 or A 1 B 1 A 2 B 2 A 3 B 3 …) allows for a stronger determination of a causal relationship between the intervention and outcome variables, because the relationship can be demonstrated across at least three different points in time – change in outcome from A 1 to B 1 , from B 1 to A 2 , and from A 2 to B 2 . 18 Before using this design, however, researchers must determine that it is safe and ethical to withdraw the intervention, especially in cases where the intervention is effective and necessary. 12

An example of results from a single-case A 1 BA 2 study conducted on eight participants with stable multiple sclerosis (data on three participants were used for this example). Four weeks of observation (baseline phase A 1 ) were followed by eight weeks of core stability training (intervention phase B), then another four weeks of observation (baseline phase A 2 ). Forward functional reach test (the maximal distance the participant can reach forward or lateral beyond arm’s length, maintaining a fixed base of support in the standing position; higher scores indicating better performance) significantly improved during intervention for Participants 1 and 3 without further improvement observed following withdrawal of the intervention (during baseline phase A 2 ). Data were extracted from Freeman et al. (2010) 16 utilizing Rohatgi’s WebPlotDigitizer software. 78

An example of results from a single-case A 1 B 1 A 2 B 2 study conducted on two participants with severe unilateral neglect after a right-hemisphere stroke. Two weeks of conventional treatment (baseline phases A 1, A 2 ) alternated with two weeks of visuo-spatio-motor cueing (intervention phases B 1 , B 2 ). Performance was assessed in two tests of lateral neglect, the Bells Cancellation Test (Figure A; lower scores indicating better performance) and the Line Bisection Test (Figure B; higher scores indicating better performance). There was a statistically significant intervention-related improvement in participants’ performance on the Line Bisection Test, but not on the Bells Test. Data were extracted from Samuel at al. (2000) 17 utilizing Rohatgi’s WebPlotDigitizer software. 78
A recent study used an ABA reversal SC study to determine the effectiveness of core stability training in 8 participants with multiple sclerosis. 16 During the first four weekly data collections, the researchers ensured a stable baseline, which was followed by eight weekly intervention data points, and concluded with four weekly withdrawal data points. Intervention significantly improved participants’ walking and reaching performance ( Figure 2 ). 16 This A 1 BA 2 design could have been strengthened by the addition of a second intervention phase for replication (A 1 B 1 A 2 B 2 ). For instance, a single-case A 1 B 1 A 2 B 2 withdrawal design aimed to assess the efficacy of rehabilitation using visuo-spatio-motor cueing for two participants with severe unilateral neglect after a severe right-hemisphere stroke. 17 Each phase included 8 data points. Statistically significant intervention-related improvement was observed, suggesting that visuo-spatio-motor cueing might be promising for treating individuals with very severe neglect ( Figure 3 ). 17
The reversal design can also incorporate a cross over design where each participant experiences more than one type of intervention. For instance, a B 1 C 1 B 2 C 2 design could be used to study the effects of two different interventions (B and C) on outcome measures. Challenges with including more than one intervention involve potential carry-over effects from earlier interventions and order effects that may impact the measured effectiveness of the interventions. 2 , 12 Including multiple participants and randomizing the order of intervention phase presentations are tools to help control for these types of effects. 19
When an intervention permanently changes an individual’s ability, a return to baseline performance is not feasible and reversal designs are not appropriate. Multiple Baseline Designs (MBDs) are useful in these situations ( Figure 4 ). 20 MBDs feature staggered introduction of the intervention across time: each participant is randomly assigned to one of at least 3 experimental conditions characterized by the length of the baseline phase. 21 These studies involve more than one participant, thus functioning as SC studies with replication across participants. Staggered introduction of the intervention allows for separation of intervention effects from those of maturation, experience, learning, and practice. For example, a multiple baseline SC study was used to investigate the effect of an anti-spasticity baclofen medication on stiffness in five adult males with spinal cord injury. 20 The subjects were randomly assigned to receive 5–9 baseline data points with a placebo treatment prior to the initiation of the intervention phase with the medication. Both participants and assessors were blind to the experimental condition. The results suggested that baclofen might not be a universal treatment choice for all individuals with spasticity resulting from a traumatic spinal cord injury ( Figure 4 ). 20

An example of results from a single-case multiple baseline study conducted on five participants with spasticity due to traumatic spinal cord injury. Total duration of data collection was nine weeks. The first participant was switched from placebo treatment (baseline) to baclofen treatment (intervention) after five data collection sessions, whereas each consecutive participant was switched to baclofen intervention at the subsequent sessions through the ninth session. There was no statistically significant effect of baclofen on viscous stiffness at the ankle joint. Data were extracted from Hinderer at al. (1990) 20 utilizing Rohatgi’s WebPlotDigitizer software. 78
The impact of two or more interventions can also be assessed via Alternating Treatment Designs (ATDs) . In ATDs, after establishing the baseline, the experimenter exposes subjects to different intervention conditions administered in close proximity for equal intervals ( Figure 5 ). 22 ATDs are prone to “carry-over effects” when the effects of one intervention influence the observed outcomes of another intervention. 1 As a result, such designs introduce unique challenges when attempting to determine the effects of any one intervention and have been less commonly utilized in rehabilitation. An ATD was used to monitor disruptive behaviors in the school setting throughout a baseline followed by an alternating treatment phase with randomized presentation of a control condition or an exercise condition. 23 Results showed that 30 minutes of moderate to intense physical activity decreased behavioral disruptions through 90 minutes after the intervention. 23 An ATD was also used to compare the effects of commercially available and custom-made video prompts on the performance of multi-step cooking tasks in four participants with autism. 22 Results showed that participants independently performed more steps with the custom-made video prompts ( Figure 5 ). 22

An example of results from a single case alternating treatment study conducted on four participants with autism (data on two participants were used for this example). After the observation phase (baseline), effects of commercially available and custom-made video prompts on the performance of multi-step cooking tasks were identified (treatment phase), after which only the best treatment was used (best treatment phase). Custom-made video prompts were most effective for improving participants’ performance of multi-step cooking tasks. Data were extracted from Mechling at al. (2013) 22 utilizing Rohatgi’s WebPlotDigitizer software. 78
Regardless of the SC study design, replication and randomization should be incorporated when possible to improve internal and external validity. 11 The reversal design is an example of replication across study phases. The minimum number of phase replications needed to meet quality standards is three (A 1 B 1 A 2 B 2 ), but having four or more replications is highly recommended (A 1 B 1 A 2 B 2 A 3 …). 11 , 14 In cases when interventions aim to produce lasting changes in participants’ abilities, replication of findings may be demonstrated by replicating intervention effects across multiple participants (as in multiple-participant AB designs), or across multiple settings, tasks, or service providers. When the results of an intervention are replicated across multiple reversals, participants, and/or contexts, there is an increased likelihood a causal relationship exists between the intervention and the outcome. 2 , 12
Randomization should be incorporated in SC studies to improve internal validity and the ability to assess for causal relationships among interventions and outcomes. 11 In contrast to traditional group designs, SC studies often do not have multiple participants or units that can be randomly assigned to different intervention conditions. Instead, in randomized phase-order designs , the sequence of phases is randomized. Simple or block randomization is possible. For example, with simple randomization for an A 1 B 1 A 2 B 2 design, the A and B conditions are treated as separate units and are randomly assigned to be administered for each of the pre-defined data collection points. As a result, any combination of A-B sequences is possible without restrictions on the number of times each condition is administered or regard for repetitions of conditions (e.g., A 1 B 1 B 2 A 2 B 3 B 4 B 5 A 3 B 6 A 4 A 5 A 6 ). With block randomization for an A 1 B 1 A 2 B 2 design, two conditions (e.g., A and B) would be blocked into a single unit (AB or BA), randomization of which to different time periods would ensure that each condition appears in the resulting sequence more than two times (e.g., A 1 B 1 B 2 A 2 A 3 B 3 A 4 B 4 ). Note that AB and reversal designs require that the baseline (A) always precedes the first intervention (B), which should be accounted for in the randomization scheme. 2 , 11
In randomized phase start-point designs , the lengths of the A and B phases can be randomized. 2 , 11 , 24 – 26 For example, for an AB design, researchers could specify the number of time points at which outcome data will be collected, (e.g., 20), define the minimum number of data points desired in each phase (e.g., 4 for A, 3 for B), and then randomize the initiation of the intervention so that it occurs anywhere between the remaining time points (points 5 and 17 in the current example). 27 , 28 For multiple-baseline designs, a dual-randomization, or “regulated randomization” procedure has been recommended. 29 If multiple-baseline randomization depends solely on chance, it could be the case that all units are assigned to begin intervention at points not really separated in time. 30 Such randomly selected initiation of the intervention would result in the drastic reduction of the discriminant and internal validity of the study. 29 To eliminate this issue, investigators should first specify appropriate intervals between the start points for different units, then randomly select from those intervals, and finally randomly assign each unit to a start point. 29
- Single Case Analysis Techniques for Intervention Research
The What Works Clearinghouse (WWC) single-case design technical documentation provides an excellent overview of appropriate SC study analysis techniques to evaluate the effectiveness of intervention effects. 1 , 18 First, visual analyses are recommended to determine whether there is a functional relation between the intervention and the outcome. Second, if evidence for a functional effect is present, the visual analysis is supplemented with quantitative analysis methods evaluating the magnitude of the intervention effect. Third, effect sizes are combined across cases to estimate overall average intervention effects which contributes to evidence-based practice, theory, and future applications. 2 , 18
Visual Analysis
Traditionally, SC study data are presented graphically. When more than one participant engages in a study, a spaghetti plot showing all of their data in the same figure can be helpful for visualization. Visual analysis of graphed data has been the traditional method for evaluating treatment effects in SC research. 1 , 12 , 31 , 32 The visual analysis involves evaluating level, trend, and stability of the data within each phase (i.e., within-phase data examination) followed by examination of the immediacy of effect, consistency of data patterns, and overlap of data between baseline and intervention phases (i.e., between-phase comparisons). When the changes (and/or variability) in level are in the desired direction, are immediate, readily discernible, and maintained over time, it is concluded that the changes in behavior across phases result from the implemented treatment and are indicative of improvement. 33 Three demonstrations of an intervention effect are necessary for establishing a functional relation. 1
Within-phase examination
Level, trend, and stability of the data within each phase are evaluated. Mean and/or median can be used to report the level, and trend can be evaluated by determining whether the data points are monotonically increasing or decreasing. Within-phase stability can be evaluated by calculating the percentage of data points within 15% of the phase median (or mean). The stability criterion is satisfied if about 85% (80% – 90%) of the data in a phase fall within a 15% range of the median (or average) of all data points for that phase. 34
Between-phase examination
Immediacy of effect, consistency of data patterns, and overlap of data between baseline and intervention phases are evaluated next. For this, several nonoverlap indices have been proposed that all quantify the proportion of measurements in the intervention phase not overlapping with the baseline measurements. 35 Nonoverlap statistics are typically scaled as percent from 0 to 100, or as a proportion from 0 to 1. Here, we briefly discuss the Nonoverlap of All Pairs ( NAP ), 36 the Extended Celeration Line ( ECL ), the Improvement Rate Difference ( IRD) , 37 and the TauU and the TauU-adjusted, TauU adj , 35 as these are the most recent and complete techniques. We also examine the Percentage of Nonoverlapping Data ( PND ) 38 and the Two Standard Deviations Band Method, as these are frequently used techniques. In addition, we include the Percentage of Nonoverlapping Corrected Data ( PNCD ) – an index applying to the PND after controlling for baseline trend. 39
Nonoverlap of all pairs (NAP)
Each baseline observation can be paired with each intervention phase observation to make n pairs (i.e., N = n A * n B ). Count the number of overlapping pairs, n o , counting all ties as 0.5. Then define the percent of the pairs that show no overlap. Alternatively, one can count the number of positive (P), negative (N), and tied (T) pairs 2 , 36 :
Extended Celeration Line (ECL)
ECL or split middle line allows control for a positive Phase A trend. Nonoverlap is defined as the proportion of Phase B ( n b ) data that are above the median trend plotted from Phase A data ( n B< sub > Above Median trend A </ sub > ), but then extended into Phase B: ECL = n B Above Median trend A n b ∗ 100
As a consequence, this method depends on a straight line and makes an assumption of linearity in the baseline. 2 , 12
Improvement rate difference (IRD)
This analysis is conceptualized as the difference in improvement rates (IR) between baseline ( IR B ) and intervention phases ( IR T ). 38 The IR for each phase is defined as the number of “improved data points” divided by the total data points in that phase. IRD, commonly employed in medical group research under the name of “risk reduction” or “risk difference” attempts to provide an intuitive interpretation for nonoverlap and to make use of an established, respected effect size, IR B - IR B , or the difference between two proportions. 37
TauU and TauU adj
Each baseline observation can be paired with each intervention phase observation to make n pairs (i.e., n = n A * n B ). Count the number of positive (P), negative (N), and tied (T) pairs, and use the following formula: TauU = P - N P + N + τ
The TauU adj is an adjustment of TauU for monotonic trend in baseline. Each baseline observation can be paired with each intervention phase observation to make n pairs (i.e., n = n A * n B ). Each baseline observation can be paired with all later baseline observations (n A *(n A -1)/2). 2 , 35 Then the baseline trend can be computed: TauU adf = P - N - S trend P + N + τ ; S trend = P A – NA
Online calculators might assist researchers in obtaining the TauU and TauU adjusted coefficients ( http://www.singlecaseresearch.org/calculators/tau-u ).
Percentage of nonoverlapping data (PND)
If anticipating an increase in the outcome, locate the highest data point in the baseline phase and then calculate the percent of the intervention phase data points that exceed it. If anticipating a decrease in the outcome, find the lowest data point in the baseline phase and then calculate the percent of the treatment phase data points that are below it: PND = n B Overlap A n b ∗ 100 . A PND < 50 would mark no observed effect, PND = 50–70 signifies a questionable effect, and PND > 70 suggests the intervention was effective. 40 The percentage of nonoverlapping (PNDC) corrected was proposed in 2009 as an extension of the PND. 39 Prior to applying the PND, a data correction procedure is applied eliminating pre-existing baseline trend. 38
Two Standard Deviation Band Method
When the stability criterion described above is met within phases, it is possible to apply the two standard deviation band method. 12 , 41 First, the mean of the data for a specific condition is calculated and represented with a solid line. In the next step, the standard deviation of the same data is computed and two dashed lines are represented: one located two standard deviations above the mean and the other – two standard deviations below. For normally distributed data, few points (less than 5%) are expected to be outside the two standard deviation bands if there is no change in the outcome score due to the intervention. However, this method is not considered a formal statistical procedure, as the data cannot typically be assumed to be normal, continuous, or independent. 41
Statistical Analysis
If the visual analysis indicates a functional relationship (i.e., three demonstrations of the effectiveness of the intervention effect), it is recommended to proceed with the quantitative analyses, reflecting the magnitude of the intervention effect. First, effect sizes are calculated for each participant (individual-level analysis). Moreover, if the research interest lies in the generalizability of the effect size across participants, effect sizes can be combined across cases to achieve an overall average effect size estimate (across-case effect size).
Note that quantitative analysis methods are still being developed in the domain of SC research 1 and statistical challenges of producing an acceptable measure of treatment effect remain. 14 , 42 , 43 Therefore, the WWC standards strongly recommend conducting sensitivity analysis and reporting multiple effect size estimators. If consistency across different effect size estimators is identified, there is stronger evidence for the effectiveness of the treatment. 1 , 18
Individual-level effect size analysis
The most common effect sizes recommended for SC analysis are: 1) standardized mean difference Cohen’s d ; 2) standardized mean difference with correction for small sample sizes Hedges’ g ; and 3) the regression-based approach which has the most potential and is strongly recommended by the WWC standards. 1 , 44 , 45 Cohen’s d can be calculated using following formula: d = X A ¯ - X B ¯ s p , with X A ¯ being the baseline mean, X B ¯ being the treatment mean, and s p indicating the pooled within-case standard deviation. Hedges’ g is an extension of Cohen’s d , recommended in the context of SC studies as it corrects for small sample sizes. The piecewise regression-based approach does not only reflect the immediate intervention effect, but also the intervention effect across time:
i stands for the measurement occasion ( i = 0, 1,… I ). The dependent variable is regressed on a time indicator, T , which is centered around the first observation of the intervention phase, D , a dummy variable for the intervention phase, and an interaction term of these variables. The equation shows that the expected score, Ŷ i , equals β 0 + β 1 T i in the baseline phase, and ( β 0 + β 2 ) + ( β 1 + β 3 ) T i in the intervention phase. β 0 , therefore, indicates the expected baseline level at the start of the intervention phase (when T = 0), whereas β 1 marks the linear time trend in the baseline scores. The coefficient β 2 can then be interpreted as an immediate effect of the intervention on the outcome, whereas β 3 signifies the effect of the intervention across time. The e i ’s are residuals assumed to be normally distributed around a mean of zero with a variance of σ e 2 . The assumption of independence of errors is usually not met in the context of SC studies because repeated measures are obtained within a person. As a consequence, it can be the case that the residuals are autocorrelated, meaning that errors closer in time are more related to each other compared to errors further away in time. 46 – 48 As a consequence, a lag-1 autocorrelation is appropriate (taking into account the correlation between two consecutive errors: e i and e i –1 ; for more details see Verbeke & Molenberghs, (2000). 49 In Equation 1 , ρ indicates the autocorrelation parameter. If ρ is positive, the errors closer in time are more similar; if ρ is negative, the errors closer in time are more different, and if ρ equals zero, there is no correlation between the errors.
Across-case effect sizes
Two-level modeling to estimate the intervention effects across cases can be used to evaluate across-case effect sizes. 44 , 45 , 50 Multilevel modeling is recommended by the WWC standards because it takes the hierarchical nature of SC studies into account: measurements are nested within cases and cases, in turn, are nested within studies. By conducting a multilevel analysis, important research questions can be addressed (which cannot be answered by single-level analysis of SC study data), such as: 1) What is the magnitude of the average treatment effect across cases? 2) What is the magnitude and direction of the case-specific intervention effect? 3) How much does the treatment effect vary within cases and across cases? 4) Does a case and/or study level predictor influence the treatment’s effect? The two-level model has been validated in previous research using extensive simulation studies. 45 , 46 , 51 The two-level model appears to have sufficient power (> .80) to detect large treatment effects in at least six participants with six measurements. 21
Furthermore, to estimate the across-case effect sizes, the HPS (Hedges, Pustejovsky, and Shadish) , or single-case educational design ( SCEdD)-specific mean difference, index can be calculated. 52 This is a standardized mean difference index specifically designed for SCEdD data, with the aim of making it comparable to Cohen’s d of group-comparison designs. The standard deviation takes into account both within-participant and between-participant variability, and is typically used to get an across-case estimator for a standardized change in level. The advantage of using the HPS across-case effect size estimator is that it is directly comparable with Cohen’s d for group comparison research, thus enabling the use of Cohen’s (1988) benchmarks. 53
Valuable recommendations on SC data analyses have recently been provided. 54 , 55 They suggest that a specific SC study data analytic technique can be chosen based on: (1) the study aims and the desired quantification (e.g., overall quantification, between-phase quantifications, randomization, etc.), (2) the data characteristics as assessed by visual inspection and the assumptions one is willing to make about the data, and (3) the knowledge and computational resources. 54 , 55 Table 1 lists recommended readings and some commonly used resources related to the design and analysis of single-case studies.
Recommend readings and resources related to the design and analysis of single-case studies.
- Quality Appraisal Tools for Single-Case Design Research
Quality appraisal tools are important to guide researchers in designing strong experiments and conducting high-quality systematic reviews of the literature. Unfortunately, quality assessment tools for SC studies are relatively novel, ratings across tools demonstrate variability, and there is currently no “gold standard” tool. 56 Table 2 lists important SC study quality appraisal criteria compiled from the most common scales; when planning studies or reviewing the literature, we recommend readers consider these criteria. Table 3 lists some commonly used SC quality assessment and reporting tools and references to resources where the tools can be located.
Summary of important single-case study quality appraisal criteria.
Quality assessment and reporting tools related to single-case studies.
When an established tool is required for systematic review, we recommend use of the What Works Clearinghouse (WWC) Tool because it has well-defined criteria and is developed and supported by leading experts in the SC research field in association with the Institute of Education Sciences. 18 The WWC documentation provides clear standards and procedures to evaluate the quality of SC research; it assesses the internal validity of SC studies, classifying them as “Meeting Standards”, “Meeting Standards with Reservations”, or “Not Meeting Standards”. 1 , 18 Only studies classified in the first two categories are recommended for further visual analysis. Also, WWC evaluates the evidence of effect, classifying studies into “Strong Evidence of a Causal Relation”, “Moderate Evidence of a Causal Relation”, or “No Evidence of a Causal Relation”. Effect size should only be calculated for studies providing strong or moderate evidence of a causal relation.
The Single-Case Reporting Guideline In BEhavioural Interventions (SCRIBE) 2016 is another useful SC research tool developed recently to improve the quality of single-case designs. 57 SCRIBE consists of a 26-item checklist that researchers need to address while reporting the results of SC studies. This practical checklist allows for critical evaluation of SC studies during study planning, manuscript preparation, and review.
Single-case studies can be designed and analyzed in a rigorous manner that allows researchers strength in assessing causal relationships among interventions and outcomes, and in generalizing their results. 2 , 12 These studies can be strengthened via incorporating replication of findings across multiple study phases, participants, settings, or contexts, and by using randomization of conditions or phase lengths. 11 There are a variety of tools that can allow researchers to objectively analyze findings from SC studies. 56 While a variety of quality assessment tools exist for SC studies, they can be difficult to locate and utilize without experience, and different tools can provide variable results. The WWC quality assessment tool is recommended for those aiming to systematically review SC studies. 1 , 18
SC studies, like all types of study designs, have a variety of limitations. First, it can be challenging to collect at least five data points in a given study phase. This may be especially true when traveling for data collection is difficult for participants, or during the baseline phase when delaying intervention may not be safe or ethical. Power in SC studies is related to the number of data points gathered for each participant so it is important to avoid having a limited number of data points. 12 , 58 Second, SC studies are not always designed in a rigorous manner and, thus, may have poor internal validity. This limitation can be overcome by addressing key characteristics that strengthen SC designs ( Table 2 ). 1 , 14 , 18 Third, SC studies may have poor generalizability. This limitation can be overcome by including a greater number of participants, or units. Fourth, SC studies may require consultation from expert methodologists and statisticians to ensure proper study design and data analysis, especially to manage issues like autocorrelation and variability of data. 2 Fifth, while it is recommended to achieve a stable level and rate of performance throughout the baseline, human performance is quite variable and can make this requirement challenging. Finally, the most important validity threat to SC studies is maturation. This challenge must be considered during the design process in order to strengthen SC studies. 1 , 2 , 12 , 58
SC studies can be particularly useful for rehabilitation research. They allow researchers to closely track and report change at the level of the individual. They may require fewer resources and, thus, can allow for high-quality experimental research, even in clinical settings. Furthermore, they provide a tool for assessing causal relationships in populations and settings where large numbers of participants are not accessible. For all of these reasons, SC studies can serve as an effective method for assessing the impact of interventions.
- Acknowledgments
This research was supported by the National Institute of Health, Eunice Kennedy Shriver National Institute of Child Health & Human Development (1R21HD076092-01A1, Lobo PI) and the Delaware Economic Development Office (Grant #109).
Some of the information in this manuscript was presented at the IV Step Meeting in Columbus, OH, June 2016.
Full text links
Read article at publisher's site: https://doi.org/10.1097/npt.0000000000000187
Citations & impact
Impact metrics, citations of article over time, alternative metrics.

Smart citations by scite.ai Smart citations by scite.ai include citation statements extracted from the full text of the citing article. The number of the statements may be higher than the number of citations provided by EuropePMC if one paper cites another multiple times or lower if scite has not yet processed some of the citing articles. Explore citation contexts and check if this article has been supported or disputed. https://scite.ai/reports/10.1097/npt.0000000000000187
Article citations, assessing the effectiveness of stapp@work, a self-management mobile app, in reducing work stress and preventing burnout: single-case experimental design study..
Demirel S , Roke Y , Hoogendoorn AW , Hoefakker J , Hoeberichts K , van Harten PN
J Med Internet Res , 26:e48883, 29 Feb 2024
Cited by: 0 articles | PMID: 38275128 | PMCID: PMC10940977
Mixed methods, single case design, feasibility trial of a motivational conversational agent for rehabilitation for adults with traumatic brain injury.
Hocking J , Maeder A , Powers D , Perimal-Lewis L , Dodd B , Lange B
Clin Rehabil , 38(3):322-336, 06 Dec 2023
Cited by: 0 articles | PMID: 38058144 | PMCID: PMC10829423
Case report: Maintaining altered states of consciousness over repeated ketamine infusions may be key to facilitate long-lasting antidepressant effects: some initial lessons from a personalized-dosing single-case study.
Reissmann S , Hartmann M , Kist A , Liechti ME , Stocker K
Front Psychiatry , 14:1197697, 25 Oct 2023
Cited by: 0 articles | PMID: 37953937 | PMCID: PMC10634239
The Permutation Distancing Test for dependent single-case observational AB-phase design data: A Monte Carlo simulation study.
Vroegindeweij A , Nijhof LN , Onghena P , van de Putte EM , Nijhof SL , Houtveen J
Behav Res Methods , 56(3):2569-2580, 01 Aug 2023
Cited by: 0 articles | PMID: 37528291 | PMCID: PMC10991042
Intramuscular Electrical Stimulation for the Treatment of Trigger Points in Patients with Chronic Migraine: A Protocol for a Pilot Study Using a Single-Case Experimental Design.
Perreault T , Arendt-Nielson L , Fernández-de-Las-Peñas C , Dommerholt J , Herrero P , Hubbard R
Medicina (Kaunas) , 59(8):1380, 28 Jul 2023
Cited by: 0 articles | PMID: 37629671 | PMCID: PMC10456716
Similar Articles
To arrive at the top five similar articles we use a word-weighted algorithm to compare words from the Title and Abstract of each citation.
How has the impact of 'care pathway technologies' on service integration in stroke care been measured and what is the strength of the evidence to support their effectiveness in this respect?
Allen D , Rixson L
Int J Evid Based Healthc , 6(1):78-110, 01 Mar 2008
Cited by: 28 articles | PMID: 21631815
Single case studies in psychology and psychiatry.
Scand J Gastroenterol Suppl , 147:11-21, 01 Jan 1988
Cited by: 0 articles | PMID: 3059452
Case studies, single-subject research, and N of 1 randomized trials: comparisons and contrasts.
Backman CL , Harris SR
Am J Phys Med Rehabil , 78(2):170-176, 01 Mar 1999
Cited by: 49 articles | PMID: 10088595
Behavioral and Pharmacotherapy Weight Loss Interventions to Prevent Obesity-Related Morbidity and Mortality in Adults: An Updated Systematic Review for the U.S. Preventive Services Task Force
LeBlanc EL , Patnode CD , Webber EM , Redmond N , Rushkin M , O’Connor EA
Agency for Healthcare Research and Quality (US), Rockville (MD) , 26 Oct 2018
Cited by: 0 articles | PMID: 30354042
Review Books & documents Free full text in Europe PMC
Trial design and reporting standards for intra-arterial cerebral thrombolysis for acute ischemic stroke.
Higashida RT , Furlan AJ , Roberts H , Tomsick T , Connors B , Barr J , Dillon W , Warach S , Broderick J , Tilley B , Sacks D , Technology Assessment Committee of the American Society of Interventional and Therapeutic Neuroradiology , Technology Assessment Committee of the Society of Interventional Radiology
Stroke , 34(8):e109-37, 17 Jul 2003
Cited by: 785 articles | PMID: 12869717
Funding
Funders who supported this work.
NICHD NIH HHS (1)
Grant ID: R21 HD076092
12 publication s
Europe PMC is part of the ELIXIR infrastructure
Single-Case Design, Analysis, and Quality Assessment for Intervention Research.
M. Lobo , Mariola Moeyaert , Andrea Baraldi Cunha + 1 more authors
Jul 1, 2017
Influential Citations
Quality indicators
Journal of Neurologic Physical Therapy
Key takeaway
Single-case studies can provide a viable alternative to large group studies, with strong internal validity and external validity for assessing causal relationships and generalizability of results..
BACKGROUND AND PURPOSE The purpose of this article is to describe single-case studies and contrast them with case studies and randomized clinical trials. We highlight current research designs, analysis techniques, and quality appraisal tools relevant for single-case rehabilitation research. SUMMARY OF KEY POINTS Single-case studies can provide a viable alternative to large group studies such as randomized clinical trials. Single-case studies involve repeated measures and manipulation of an independent variable. They can be designed to have strong internal validity for assessing causal relationships between interventions and outcomes, as well as external validity for generalizability of results, particularly when the study designs incorporate replication, randomization, and multiple participants. Single-case studies should not be confused with case studies/series (ie, case reports), which are reports of clinical management of a patient or a small series of patients. RECOMMENDATIONS FOR CLINICAL PRACTICE When rigorously designed, single-case studies can be particularly useful experimental designs in a variety of situations, such as when research resources are limited, studied conditions have low incidences, or when examining effects of novel or expensive interventions. Readers will be directed to examples from the published literature in which these techniques have been discussed, evaluated for quality, and implemented.
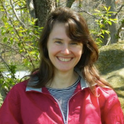
- Michele A. Lobo , University of Delaware
- Mariola Moeyaert , State University of New York at Albany
- Andrea Baraldi Cunha , University of Delaware
- Iryna Babik , University of Delaware
- n-of-1 studies,
- quality assessment,
- research design,
- single-subject research

Exploring Single-Case Research Design With Individualized Anxiety-Based Neurofeedback Protocols and Session Data
- J. Claire Gregory The University of Texas at San Antonio
- Devon E. Romero
- Mark S. Jones
Individuals’ experiences of anxiety differ in manifestation, development, and severity. Using retrospective neurofeedback session data which included quantitative electroencephalography (qEEG)-based anxiety protocols, we examined four participants’ data. We employed a single-case research design (SCRD) methodology to highlight the individual variations or change across participants’ neurofeedback session data. We assessed effect size using visual analysis, nonoverlap of all pairs, and simulation modeling analysis. Considering the novel concept of applying SCRD to physiological data, we compare and contrast our findings while also suggesting limitations and future areas for research.
American Psychiatric Association. (2022). Diagnostic and statistical manual of mental disorders (5th ed., text rev.). https://doi.org/10.1176/appi.books.9780890425787
Borckardt, J. J. (2006). SMA time series analysis program for short time series data streams (Version 8.3.3.) [MacOS 64-Bit]. http://www.clinicalresearcher.org/software.htm
Carpenter, J. K., Andrews, L. A., Witcraft, S. M., Powers, M. B., Smits, J. A. J., & Hofmann, S. G. (2018). Cognitive behavioral therapy for anxiety and related disorders: A meta-analysis of randomized placebo-controlled trials. Depression and Anxiety, 35(6), 502–514. https://doi.org/10.1002/da.22728
Cheon, E.-J., Koo, B.-H., Seo, W.-S., Lee, J.-Y., Choi, J.-H., & Song, S.-H. (2015). Effects of neurofeedback on adult patients with psychiatric disorders in a naturalistic setting. Applied Psychophysiology and Biofeedback, 40(1), 17–24. https://doi.org/10.1007/s10484-015-9269-x
Delpino, F. M., da Silva, C. N., Jerônimo, J. S., Mulling, E. S., da Cunha, L. L., Weymar, M. K., Alt, R., Caputo, E. L., & Feter, N. (2022). Prevalence of anxiety during the COVID-19 pandemic: A systematic review and meta-analysis of over 2 million people. Journal of Affective Disorders, 318, 272–282. https://doi.org/10.1016/j.jad.2022.09.003
Fielenbach, S., Donkers, F., Spreen, M., & Bogaerts, S. (2019). The ability of forensic psychiatric patients with substance use disorder to learn neurofeedback. International Journal of Forensic Mental Health, 18(3), 187–199. https://doi.org/10.1080/14999013.2018.1485187
Ganz, J. B., & Ayres, K. M. (2018). Methodological standards in single-case experimental design: Raising the bar. Research in Developmental Disabilities, 79, 3-9. https://doi.org/10.1016/j.ridd.2018.03.003
Gregory, J. C. (2022). Using a neuro-ecological approach: Individualized neurofeedback for the treatment of alcohol use disorder. [Doctoral dissertation, University of Texas at San Antonio].
Gregory, J. C., Romero, D. E., & Jones, M. S. (2020). Predictors of neurofeedback outcomes following qEEG individualized protocols for anxiety. NeuroRegulation, 7(1), 18–25. https://doi.org/10.15540/nr.7.1.18
Jones, M. S. (2015). Comparing DC offset and impedance readings in the assessment of electrode connection quality. NeuroRegulation, 2(1), 29–36. https://doi.org/10.15540/nr.2.1.29
Kazdin, A. E. (2021). Single‐case experimental designs: Characteristics, changes, and challenges. Journal of the Experimental Analysis of Behavior, 115(1), 56–85. https://doi.org/10.1002/jeab.638
Kratochwill, T. R., Horner, R. H., Levin, J. R., Machalicek, W., Ferron, J., & Johnson, A. (2023). Single-case intervention research design standards: Additional proposed upgrades and future directions. Journal of School Psychology, 97, 192–216. https://doi.org/10.1016/j.jsp.2022.12.002
La Vaque, T. J., Hammond, D. C., Trudeau, D., Monastra, V., Perry, J., Lehrer, P., Matheson, D., & Sherman, R. (2002). Template for developing guidelines for the evaluation of the clinical efficacy of psychophysiological interventions. Applied Psychophysiology and Biofeedback, 27(4), 273–281. https://doi.org/10.1023/A:1021061318355
Ledford, J., Lane, J., & Severini, K. (2018). Systematic use of visual analysis for assessing outcomes in single case design studies. Brain Impairment, 19(1), 4–17. https://doi.org/10.1017/BrImp.2017.16
Lenz, A. S. (2015). Using single-case research designs to demonstrate evidence for counseling practices. Journal of Counseling and Development, 93(4), 387–393. https://doi.org/10.1002/jcad.12036
Lobo, M. A., Moeyaert, M., Cunha, A. B., & Babik, I. (2017). Single-case design, analysis, and quality assessment for intervention research. Journal of Neurologic Physical Therapy, 41(3), 187–197. https://doi.org/10.1097/NPT.0000000000000187
Manolov, R., & Solanas, A. (2018). Analytical options for single-case experimental designs: Review and application to brain impairment. Brain Impairment, 19(1), 18–32. https://doi.org/10.1017/BrImp.2017.17
National Institute of Mental Health. (2017). Any anxiety disorder. https://www.nimh.nih.gov/health/statistics/any-anxiety-disorder#part_2576
National Institute of Mental Health. (2023, April). Anxiety disorders. https://www.nimh.nih.gov/health/topics/anxiety-disorders#part_2222
Parker, R. I, & Vannest, K. (2009). An improved effect size for single-case research: Nonoverlap of all pairs. Behavior Therapy, 40(4), 357–367. https://doi.org/10.1016/j.beth.2008.10.006
Tanaka-Matsumi, J., & Kameoka, V. A. (1986). Reliabilities and concurrent validities of popular self-report measures of depression, anxiety, and social desirability. Journal of Consultation and Clinical Psychology, 54(3), 328–333. https://doi.org/10.1037/0022-006X.54.3.328
Walker, J. E. (2012). Remediation of enuresis using QEEG-guided neurofeedback training. Biofeedback, 40(3), 109–112. https://doi.org/10.5298/1081-5937-40.3.04
Wigton, N. L., & Krigbaum, G. (2015). A Review of qEEG-guided neurofeedback. NeuroRegulation, 2(3), 149–155. https://doi.org/10.15540/nr.2.3.149
Zung, W. (1971). A rating instrument for anxiety disorders. Psychosomatics, 12(6), 371–179. https://doi.org/10.1016/S0033-3182(71)71479-0
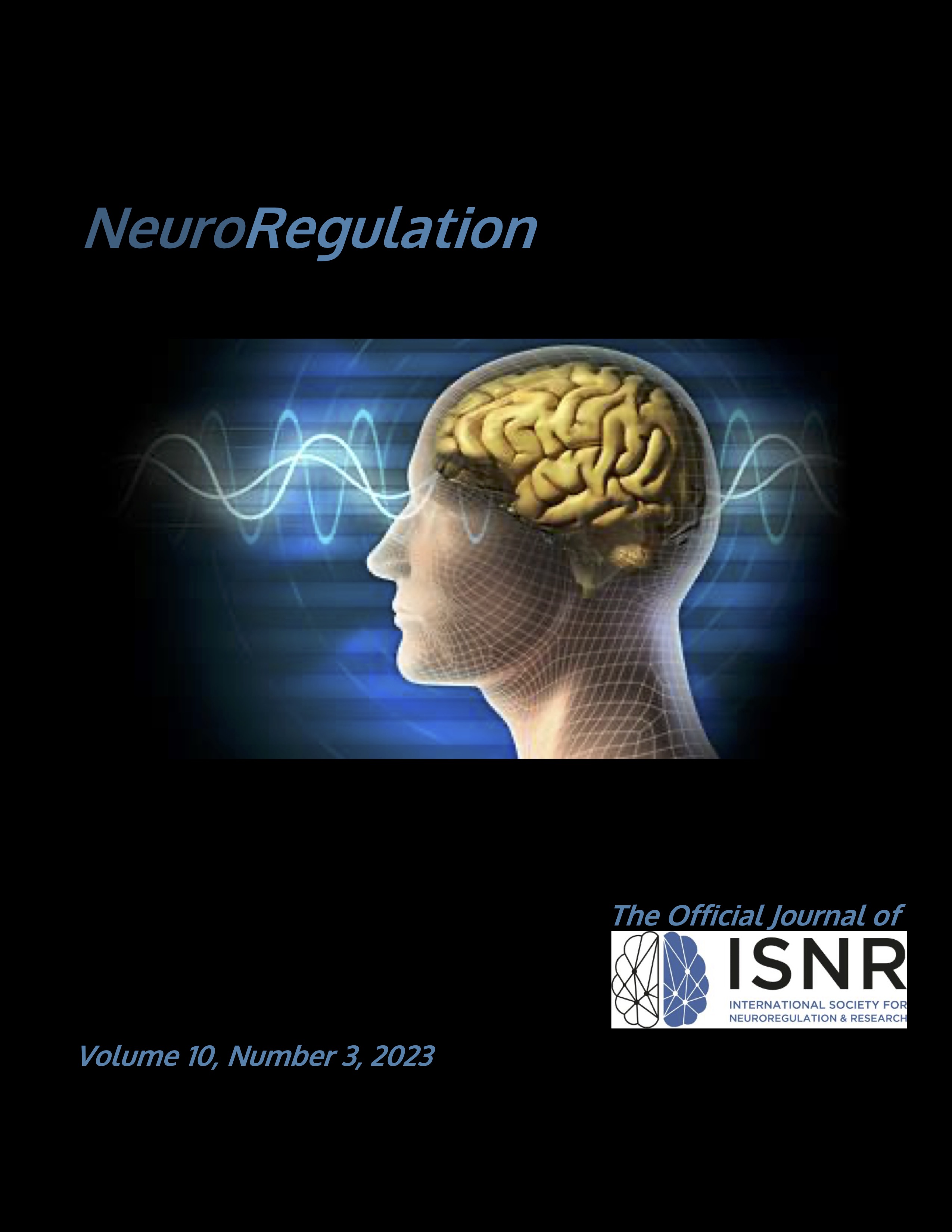
Copyright (c) 2023 J. Claire Gregory, Devon E. Romero, Mark S. Jones

This work is licensed under a Creative Commons Attribution 4.0 International License .
- Authors retain copyright and grant the journal right of first publication with the work simultaneously licensed under a Creative Commons Attribution License (CC-BY) that allows others to share the work with an acknowledgement of the work's authorship and initial publication in this journal.
- Authors are able to enter into separate, additional contractual arrangements for the non-exclusive distribution of the journal's published version of the work (e.g., post it to an institutional repository or publish it in a book), with an acknowledgement of its initial publication in this journal.
- Authors are permitted and encouraged to post their work online (e.g., in institutional repositories or on their website) prior to and during the submission process, as it can lead to productive exchanges, as well as earlier and greater citation of published work (See The Effect of Open Access ).
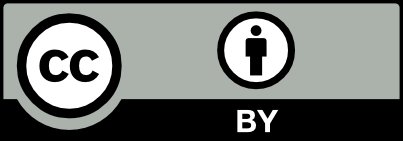
Make a Submission
Information.
- For Readers
- For Authors
- For Librarians

We thank the generous sponsors of the current issue:
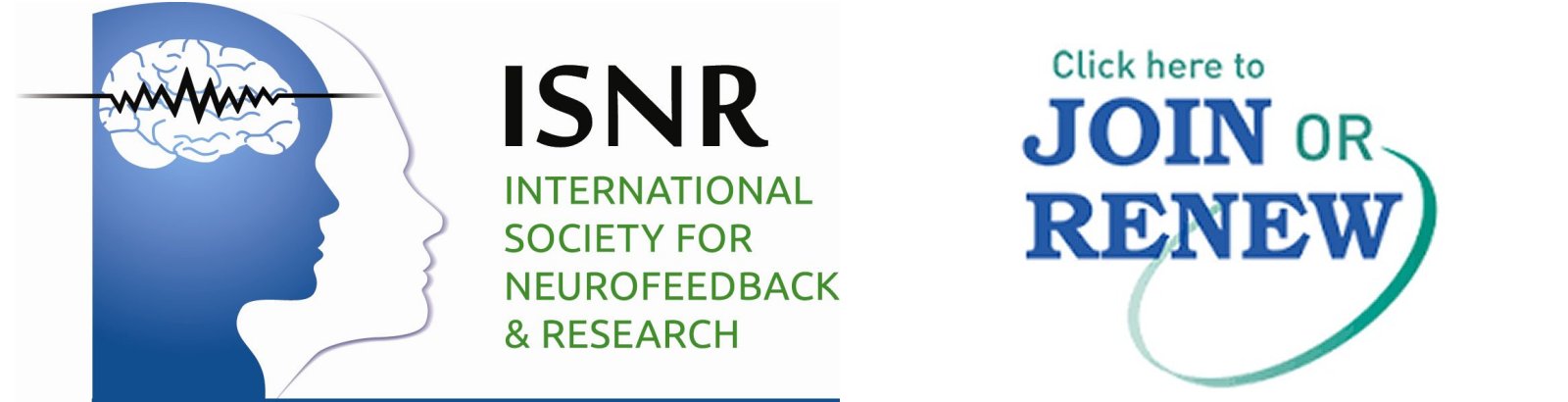
The official journal of the International Society of NeuroRegulation and Research (ISNR ) ; (c) 2021, all rights reserved.
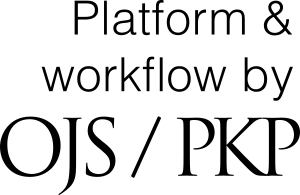

IMAGES
VIDEO
COMMENTS
We highlight current research designs, analysis techniques, and quality appraisal tools relevant for single-case rehabilitation research. Summary of key points: Single-case studies can provide a viable alternative to large group studies such as randomized clinical trials. Single-case studies involve repeated measures and manipulation of an ...
Single-case studies can provide a viable alternative to large group studies such as randomized clinical trials. Single case studies involve repeated measures, and manipulation of and independent variable. They can be designed to have strong internal validity for assessing causal relationships between interventions and outcomes, and external ...
This study made use of a multiple single-case design, which is used to evaluate the effect of a (personalized) treatment or intervention in individuals based on repeated measurement data (Barlow ...
Current research designs, analysis techniques, and quality appraisal tools relevant for single-case rehabilitation research are highlighted and examples from the published literature in which these techniques have been discussed, evaluated for quality, and implemented are directed. BACKGROUND AND PURPOSE The purpose of this article is to describe single-case studies and contrast them with case ...
This book is a compendium of tools and information for researchers considering single-case design (SCD) research, a newly viable and often essential methodology in applied psychology, education, and related fields. ... Visual Analysis of Single-Case Intervention Research: Conceptual and Methodological Issues Thomas R. Kratochwill, Joel R. Levin ...
intervention research, we introduce a valuable design-and-analysis methodology that may be of consequence to health sciences intervention researchers. In 2010, a lengthy article appeared in Psychological Methods on the value of con-ducting randomized experiments in an area of quantitative experimental research known as single-case intervention
The purpose of this article is to describe single-case studies and contrast them with case studies and randomized clinical trials. We highlight current research designs, analysis techniques, and quality appraisal tools relevant for single-case rehabilitation research. Summary of Key Points:
A mixed methods single-case design research (MMSCDR) approach may be particularly well-suited to investigating questions about participant engagement/completion of the intervention, fidelity of implementation, and the contextual fit or social validity of the intervention (Hitchcock et al., 2010; Onghena, Maes, & Heyvaert, 2019; Van Ness et al ...
Single-case research design and analysis: New directions for psychology and education. Hillsdale, NJ: Lawrence Erlbaum. ... Evidence-Based Communication Assessment and Intervention, 3, 188-196. Crossref. Google Scholar. ... A systematic quality review of high-tech AAC interventions as an evide...
Recommendations for Clinical Practice:When rigorously designed, single-case studies can be particularly useful experimental designs in a variety of situations, such as when rese...
SCARF.May23.IRA_ Download. The SCARF is intended as a tool to assess the quality and outcomes of single case design studies. For the purposes of the tool, "study" refers to any single design, which may include a single participant (e.g., A-B-A-B designs) or multiple participants (e.g., multiple baseline design across participants).
The What Works Clearinghouse (WWC) single-case design technical documentation provides an excellent overview of appropriate SC study analysis techniques to evaluate the effectiveness of intervention effects. 1, 18 First, visual analyses are recommended to determine whether there is a functional relationship between the intervention and the ...
The What Works Clearinghouse (WWC) single-case design technical documentation provides an excellent overview of appropriate SC study analysis techniques to evaluate the effectiveness of intervention effects. 1,18 First, visual analyses are recommended to determine whether there is a functional relation between the intervention and the outcome ...
Key takeaway: 'Single-case studies can provide a viable alternative to large group studies, with strong internal validity and external validity for assessing causal relationships and generalizability of results.'
In this paper, we provide a critique focused on the What Works Clearinghouse (WWC) Standards for Single-Case Research Design (Standards 4.1).Specifically, we (a) recommend the use of visual-analysis to verify a single-case intervention study's design standards and to examine the study's operational issues, (b) identify limitations of the design-comparable effect-size measure and discuss ...
Single-case intervention research has a rich tradition of providing evidence about the efficacy of interventions applied both to solving a diverse range of human problems and to enriching the knowledge base established in many fields of science (Kratochwill, 1978; Kratochwill & Levin, 1992, 2010). In the social sciences the randomized ...
Now thoroughly updated in its second edition, acclaimed author Alan E. Kazdin's Single-Case Research Designs provides a notable contrast to the quantitative methodology approach that pervades the biological and social sciences. Single-case research has played an important role in developing and evaluating interventions that are designed to alter a particular facet of human functioning. Now ...
Article: Single-Case Design, Analysis, and Quality Assessment for Intervention Research The purpose of this article is to describe single-case studies and contrast them with case studies and randomized clinical trials.
We highlight current research designs, analysis techniques, and quality appraisal tools relevant for single-case rehabilitation research. </jats:sec> <jats:sec> Summary of Key Points: Single-case studies can provide a viable alternative to large group studies such as randomized clinical trials. Single-case studies involve repeated measures and….
Single-case studies involve repeated measures and manipulation of an independent variable. They can be designed to have strong internal validity for assessing causal relationships between interventions and outcomes, as well as external validity for generalizability of results, particularly when the study designs incorporate replication ...
Individuals' experiences of anxiety differ in manifestation, development, and severity. Using retrospective neurofeedback session data which included quantitative electroencephalography (qEEG)-based anxiety protocols, we examined four participants' data. We employed a single-case research design (SCRD) methodology to highlight the individual variations or change across participants ...
Keywords n-of-one studies; single-subject research; research design; quality assessment Introduction The purpose of this article is to present current tools and techniques relevant for single-case rehabilitation research. Single-case (SC) studies have been identified by a variety of names, Correspondence regarding this article should be ...