Journal of Educational Data Mining
About the journal.
The Journal of Educational Data Mining (JEDM; ISSN: 2157-2100; see indexing ) is published by the International Educational Data Mining Society (IEDMS) . It is an international and interdisciplinary forum of research on computational approaches for analyzing electronic repositories of student data to answer educational questions. It is completely and permanently free and open-access to both authors and readers .
Educational Data Mining is an emerging discipline dedicated to developing methods that explore the unique data generated in educational settings. The goal is to deepen our understanding of students and their learning environments through innovative and impactful research. Key data sources in EDM include:
- Student interactions within interactive learning environments.
- Learner test data and assessment artifacts.
- Digital didactic materials.
- Usage patterns in learning management systems.
Types of Submissions:
The journal seeks high-quality original work that emphasizes novelty and impact in the field. Accepted submissions should extend beyond mere application and must include elements that contribute to broader knowledge, such as generalizable methodologies or comparative analyses. Specific areas of interest include, but are not limited to:
- Innovative Processes or Methodologies: Developing and detailing new processes or methodologies for analyzing educational data.
- Integration with Pedagogical Theories: Research that advances pedagogical theories through data-driven insights.
- Broader Applicability of Educational Software: Work that not only improves educational software but also demonstrates the generalizable applicability of findings across different contexts.
- Advancing Understanding of Learner Cognition: Research that enhances our understanding of learners' domain representations and cognitive processes.
- Comparative Assessment of Learner Engagement: Studies that compare different approaches to assessing learner engagement and effectiveness.
The journal also welcomes survey articles, theoretical articles, and position papers, provided they build on existing research and offer significant contributions to the field. Please look here for additional information.
Former Editors:

Current Issue
- Archive Issues
- Announcements
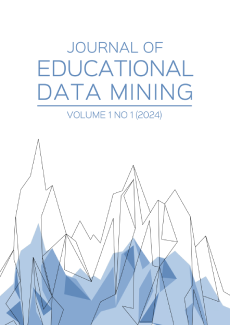
Published: 2024-06-27
Editorial Acknowledgment
Edm 2024 journal track, the knowledge component attribution problem for programming: methods and tradeoffs with limited labeled data, automated evaluation of classroom instructional support with llms and bows: connecting global predictions to specific feedback, an approach to improve k-anonymization practices in educational data mining, exploring the impact of symbol spacing and problem sequencing on arithmetic performance: an educational data mining approach.
Page 84-111
Effect of Gamification on Gamers: Evaluating Interventions for Students Who Game the System
Page 112-140
LearnSphere: A Learning Data and Analytics CyberInfrastructure
Page 141-163
Session-based Methods for Course Recommendation
Page 164-196
Analyzing Transitions in Sequential Data with Marginal Models
Page 197-232
Supercharging BKT with Multidimensional Generalizable IRT and Skill Discovery
Page 233-278
Structural Neural Networks Meet Piecewise Exponential Models for Interpretable College Dropout Prediction
Page 279-302
Extended Articles from the EDM 2023 Conference
Investigating concept definition and skill modeling for cognitive diagnosis in language learning.
Page 303-329
A Course Recommender System Built on Success to Support Students at Risk in Higher Education
Page 330-364
A Comprehensive Study on Evaluating and Mitigating Algorithmic Unfairness with the MADD Metric
Page 365-409
- Vol 16, No 1 (2024)
- Vol 15, No 3 (2023)
- Vol 15, No 2 (2023)
- Vol 15, No 1 (2023)
- Vol 14, No 3 (2022)
- Vol 14, No 2 (2022)
- Vol 14, No 1 (2022)
- Vol 13, No 4 (2021)
- Vol 13, No 3 (2021)
- Vol 13, No 2 (2021)
- Vol 13, No 1 (2021)
- Vol 12, No 4 (2020)
- Vol 12, No 3 (2020)
- Vol 12, No 2 (2020)
- Vol 12, No 1 (2020)
- Vol 11, No 3 (2019)
- Vol 11, No 2 (2019)
- Vol 11, No 1 (2019)
- Vol 10, No 3 (2018)
- Vol 10, No 2 (2018)
- Vol 10, No 1 (2018)
- Vol 9, No 2 (2017)
- Vol 9, No 1 (2017)
- Vol 8, No 2 (2016)
- Vol 8, No 1 (2016)
- Vol 7, No 3 (2015)
- Vol 7, No 2 (2015)
- Vol 7, No 1 (2015)
- Vol 6, No 1 (2014)
- Vol 5, No 2 (2013)
- Vol 5, No 1 (2013)
- Vol 4, No 1 (2012)
- Vol 3, No 1 (2011)
- Vol 2, No 1 (2010)
- Vol 1, No 1 (2009)
To read this content please select one of the options below:
Please note you do not have access to teaching notes, educational data mining: a systematic review of research and emerging trends.
Information Discovery and Delivery
ISSN : 2398-6247
Article publication date: 19 May 2020
Issue publication date: 10 October 2020
Educational data mining (EDM) and learning analytics, which are highly related subjects but have different definitions and focuses, have enabled instructors to obtain a holistic view of student progress and trigger corresponding decision-making. Furthermore, the automation part of EDM is closer to the concept of artificial intelligence. Due to the wide applications of artificial intelligence in assorted fields, the authors are curious about the state-of-art of related applications in Education.
Design/methodology/approach
This study focused on systematically reviewing 1,219 EDM studies that were searched from five digital databases based on a strict search procedure. Although 33 reviews were attempted to synthesize research literature, several research gaps were identified. A comprehensive and systematic review report is needed to show us: what research trends can be revealed and what major research topics and open issues are existed in EDM research.
Results show that the EDM research has moved toward the early majority stage; EDM publications are mainly contributed by “actual analysis” category; machine learning or even deep learning algorithms have been widely adopted, but collecting actual larger data sets for EDM research is rare, especially in K-12. Four major research topics, including prediction of performance, decision support for teachers and learners, detection of behaviors and learner modeling and comparison or optimization of algorithms, have been identified. Some open issues and future research directions in EDM field are also put forward.
Research limitations/implications
Limitations for this search method include the likelihood of missing EDM research that was not captured through these portals.
Originality/value
This systematic review has not only reported the research trends of EDM but also discussed open issues to direct future research. Finally, it is concluded that the state-of-art of EDM research is far from the ideal of artificial intelligence and the automatic support part for teaching and learning in EDM may need improvement in the future work.
- Educational data mining
- Learning analytics
- Systematic review
- Prediction of performance
- Decision support
- Artificial intelligence
Acknowledgements
Conflict of interest: The authors have declared no conflicts of interest for this article.
This study was supported by National Natural Science Foundation of China Under Grant No. 61877027.
Du, X. , Yang, J. , Hung, J.-L. and Shelton, B. (2020), "Educational data mining: a systematic review of research and emerging trends", Information Discovery and Delivery , Vol. 48 No. 4, pp. 225-236. https://doi.org/10.1108/IDD-09-2019-0070
Emerald Publishing Limited
Copyright © 2020, Emerald Publishing Limited
Related articles
All feedback is valuable.
Please share your general feedback
Report an issue or find answers to frequently asked questions
Contact Customer Support
A Survey on Tools and Techniques of Classification in Educational Data Mining
- Conference paper
- First Online: 20 August 2024
- Cite this conference paper
- D. I. George Amalarethinam 8 &
- A. Emima 8
Part of the book series: Communications in Computer and Information Science ((CCIS,volume 2065))
Included in the following conference series:
- International Conference on Applied Intelligence and Informatics
23 Accesses
Educational Data Mining (EDM) is one of the newest topics to emerge in recent years, and it focuses on developing strategies for analyzing various forms of data gathered from the academic circle. EDM fosters collaboration among educators, data scientists, and machine learning specialists. The interdisciplinary character of EDM creates an atmosphere in which educators and data scientists collaborate to develop and apply efficient approaches for extracting insights from educational data. EDM methods and techniques with Machine learning techniques are utilized to extract meaningful and useful information from large dataset. EDM strives to develop intelligent systems that personalize educational experiences to individual individuals by understanding their unique learning styles and problems. For scientists and researchers, realistic applications of Machine Learning in the EDM sectors offer new frontiers and present new problems. This transition to individualized approaches represents a radical shift in educational practices, stressing a student-centered and successful learning environment. One of the most important research areas in EDM is predicting student success. The prediction algorithms and techniques must be developed, to forecast students’ performance, which aids the tutor, institution to boost the level of students’ performance. Beyond forecasting student achievement, EDM is increasingly focusing on the creation of personalized learning systems and adaptive educational technology. EDM’s goal is to construct intelligent systems that personalize educational experiences to individual students by utilizing classification algorithms and data mining tools. This paper examines various classification techniques in prediction methods and data mining tools used in EDM.
This is a preview of subscription content, log in via an institution to check access.
Access this chapter
Subscribe and save.
- Get 10 units per month
- Download Article/Chapter or eBook
- 1 Unit = 1 Article or 1 Chapter
- Cancel anytime
- Available as PDF
- Read on any device
- Instant download
- Own it forever
- Available as EPUB and PDF
- Compact, lightweight edition
- Dispatched in 3 to 5 business days
- Free shipping worldwide - see info
Tax calculation will be finalised at checkout
Purchases are for personal use only
Institutional subscriptions
Al-Barrak, M.A., Al-Razgan, M.: Predicting students final GPA using decision trees: a case study. Int. J. Inf. Educ. Technol. 6 (7), 528 (2016)
Google Scholar
Costa, E.B., Fonseca, B., Santana, M.A., de Araújo, F.F., Rego, J.: Evaluating the effectiveness of educational data mining techniques for early prediction of students’ academic failure in introductory programming courses. Comput. Hum. Behav. 73 , 247–256 (2017)
Article Google Scholar
Roy, S., Garg, A.: Predicting academic performance of student using classification techniques. In: 4th IEEE Uttar Pradesh Section International Conference on Electrical, Computer and Electronics (UPCON) University, Mathura, 26–28 October (2017)
Aleem, A., Gore, M.M.: Educational data mining methods: a survey. In: 9th IEEE International Conference on Communication Systems and Network Technologies (2020)
Hussain, S., Dahan, N.A., Ba-Alwi, F.M., Ribata, N.: Educational data mining and analysis of students’ academic performance using WEKA. Indonesian J. Electr. Eng. Comput. Sci. 9 (2), 447 (2018). https://doi.org/10.11591/ijeecs.v9.i2.pp447-459 . ISSN 2502-4752
Anoopkumar, M., Md. Zubair Rahman, A.M.J.: Model of tuned J48 classification and analysis of performance prediction in educational data mining. Int. J. Appl. Eng. Res. 13 (20), 14717–14727 (2018). ISSN 0973-4562
Fernandes, E., Holanda, M., Victorino, M., Borges, V., Carvalho, R., Van Erven, G.: Educational data mining: predictive analysis of academic performance of public school students in the capital of Brazil. J. Bus. Res. 94 , 335–343 (2019). https://doi.org/10.1016/j.jbusres.2018.02.012
Ashrafa, M., Zamanb, M., Ahmed, M.: An intelligent prediction system for educational data mining based on ensemble and filtering approaches. In: International Conference on Computational Intelligence and Data Science - ICCIDS (2019)
Salal, Y.K., Abdullaev, S.M., Kumar, M.: Educational data mining: student performance prediction in academic. Int. J. Eng. Adv. Technol. (IJEAT) 8 (4C) (2019). ISSN 2249-8958
Jalota, C., Agrawal, R.: Analysis of educational data mining using classification. In: International Conference on Machine Learning, Big Data, Cloud and Parallel Computing (COMITCon). IEEE (2019)
Francis, B.K., Babu, S.S.: Predicting Academic Performance of Students Using a Hybrid Data Mining Approach. Springer, Heidelberg (2019)
Book Google Scholar
Sawant, T.U., Pol, U.R., Patankar, P.S.: Student placement prediction model using gradient boosted tree algorithm. JETIR 6 (5), 499 (2019)
Adekitan, A.I., Salau, O.: The impact of engineering students’ performance in the first three years on their graduation result using educational data mining. Heliyon 5 (2), e01250 (2019). https://doi.org/10.1016/j.heliyon.2019.e01250
Ganesh Karthikeyan, V., Thangaraj, P., Karthik, S.: Towards developing hybrid educational data mining model (HEDM) for efficient and accurate student performance evaluation. Soft. Comput. 24 (24), 18477–18487 (2020). https://doi.org/10.1007/s00500-020-05075-4
Sokkhey, P., Okazaki, T.: Developing web-based support systems for predicting poor-performing students using educational data mining techniques. (IJACSA) Int. J. Adv. Comput. Sci. Appl. 11 (7) (2020)
Injadat, M.N., Moubayed, A., Nassif, A.B., Shami, A.: Multi-split optimized bagging ensemble model selection for multi-class educational data mining. Appl. Intell. 50 (12), 4506–4528 (2020). https://doi.org/10.1007/s10489-020-01776-3
Tsiakmaki, M., Kostopoulos, G., Kotsiantis, S., Ragos, O.: Implementing AutoML in educational data mining for prediction tasks. Appl. Sci. 10 (1), 90 (2019). https://doi.org/10.3390/app10010090
El Aouifi, H., El Hajji, M., Es-Saady, Y., Douzi, H.: Predicting learner’s performance through video sequences viewing behavior analysis using educational data-mining. Educ. Inf. Technol. 26 , 5799–5814 (2021)
Patil, S., Chaudhari, U., Kangane, S., Shelar, R., Mahajan, S.: Predicting student’s performance using machine learning algorithm. Int. J. Res. Publ. Rev. 2 (7), 495–499 (2021)
Salih, N.Z., Khalaf, W.: Prediction of student’s performance through educational data mining techniques. Indonesian J. Electr. Eng. Comput. Sci. 22 (3), 1708 (2021). https://doi.org/10.11591/ijeecs.v22.i3.pp1708-1715
López-Zambrano, J., Lara, J.A., Romero, C.: Improving the portability of predicting students’ performance models by using ontologies. J. Comput. High. Educ. 34 (1), 1–19 (2021). https://doi.org/10.1007/s12528-021-09273-3
Hasan, H., Yulastri, A., Ganefri, G., Putri, T.T.A., Marta, R.: Prediction of student entrepreneurship future work based on entrepreneurship course using the naïve Bayes classifier model. Sinkron 9 (1), 525–532 (2024). https://doi.org/10.33395/sinkron.v9i1.13293
Widarta, A.E.W., Luthfi, A., Dewa, C.K.: Prediction of student performance based on behavior using e-learning during the Covid-19 pandemic using support vector Machine. Sinkron 9 (1), 332–345 (2024). https://doi.org/10.33395/sinkron.v9i1.12857
Priyambudi, Z.S., Nugroho, Y.S.: Which algorithm is better? An implementation of normalization to predict student performance. In: AIP Conference Proceedings, vol. 2926, no. 1. AIP Publishing (2024)
Batool, S., Rashid, J., Nisar, M.W., Kim, J., Kwon, H.-Y., Hussain, A.: Educational data mining to predict students’ academic performance: a survey study. Educ. Inf. Technol. 28 (1), 905–971 (2023). https://doi.org/10.1007/s10639-022-11152-y
Selvakumari, S.: Design of a prediction model to predict students’ performance using educational data mining and machine learning. Eng. Proc. 59 (1) (2023)
Baek, C., Doleck, T.: Educational data mining versus learning analytics: a review of publications from 2015 to 2019. Interact. Learn. Environ. 31 (6), 3828–3850 (2023). https://doi.org/10.1080/10494820.2021.1943689
Dol, S.M., Jawandhiya, P.M.: Classification technique and its combination with clustering and association rule mining in educational data mining—a survey. Eng. Appl. Artif. Intell. 122 , 106071 (2023). https://doi.org/10.1016/j.engappai.2023.106071
AL-Mashanji, A.K., Hamza, A.H., Alhasnawy, L.H.: Computational prediction algorithms and tools used in educational data mining: a review. J. Univ. Babylon Pure Appl. Sci. (2023)
Alamgir, Z., Akram, H., Karim, S., Wali, A.: Enhancing student performance prediction via educational data mining on academic data. Inform. Educ. 23 , 1–24 (2023). https://doi.org/10.15388/infedu.2024.04
Marjan, M.A., Uddin, M.P., Afjal, M.I.: An educational data mining system for predicting and enhancing tertiary students’ programming skill. Comput. J. 66 (5), 1083–1101 (2023). https://doi.org/10.1093/comjnl/bxab214
Feng, G., Fan, M.: Research on learning behavior patterns from the perspective of educational data mining: evaluation, prediction and visualization. Expert Syst. Appl. 23 (2024)
Le Quy, T.: Fairness-aware Machine Learning in Educational Data Mining (2024)
Ouahi, M., Khoulji, S., Kerkeb, M.L.: Advancing sustainable learning environments: a literature review on data encoding techniques for student performance prediction using deep learning models in education. In: E3S Web of Conferences, vol. 477, p. 00074. EDP Sciences (2024)
Jhody, J.R.: Penerapan Teknik Data Mining terhadap Prediksi Pemilihan Jurusan IPA/IPS Siswa Menggunakan Algoritma C4. 5. Jurnal Media Teknologi dan Informasi 1 (1) (2024)
Download references
Author information
Authors and affiliations.
Department of Computer Science, Jamal Mohamed College (Autonomous), Tiruchirappalli, 620 020, Tamil Nadu, India
D. I. George Amalarethinam & A. Emima
You can also search for this author in PubMed Google Scholar
Corresponding author
Correspondence to A. Emima .
Editor information
Editors and affiliations.
Nottingham Trent University, Nottingham, UK
Mufti Mahmud
Higher Colleges of Technology, Dubai, United Arab Emirates
Hanene Ben-Abdallah
Jahangirnagar University, Dhaka, Bangladesh
M. Shamim Kaiser
Military Technological College, Muscat, Oman
Muhammad Raisuddin Ahmed
Maebashi Institute of Technology, Gunma, Japan
Rights and permissions
Reprints and permissions
Copyright information
© 2024 The Author(s), under exclusive license to Springer Nature Switzerland AG
About this paper
Cite this paper.
George Amalarethinam, D.I., Emima, A. (2024). A Survey on Tools and Techniques of Classification in Educational Data Mining. In: Mahmud, M., Ben-Abdallah, H., Kaiser, M.S., Ahmed, M.R., Zhong, N. (eds) Applied Intelligence and Informatics. AII 2023. Communications in Computer and Information Science, vol 2065. Springer, Cham. https://doi.org/10.1007/978-3-031-68639-9_7
Download citation
DOI : https://doi.org/10.1007/978-3-031-68639-9_7
Published : 20 August 2024
Publisher Name : Springer, Cham
Print ISBN : 978-3-031-68638-2
Online ISBN : 978-3-031-68639-9
eBook Packages : Computer Science Computer Science (R0)
Share this paper
Anyone you share the following link with will be able to read this content:
Sorry, a shareable link is not currently available for this article.
Provided by the Springer Nature SharedIt content-sharing initiative
- Publish with us
Policies and ethics
- Find a journal
- Track your research
- Open access
- Published: 03 March 2022
Educational data mining: prediction of students' academic performance using machine learning algorithms
- Mustafa Yağcı ORCID: orcid.org/0000-0003-2911-3909 1
Smart Learning Environments volume 9 , Article number: 11 ( 2022 ) Cite this article
62k Accesses
159 Citations
38 Altmetric
Metrics details
Educational data mining has become an effective tool for exploring the hidden relationships in educational data and predicting students' academic achievements. This study proposes a new model based on machine learning algorithms to predict the final exam grades of undergraduate students, taking their midterm exam grades as the source data. The performances of the random forests, nearest neighbour, support vector machines, logistic regression, Naïve Bayes, and k-nearest neighbour algorithms, which are among the machine learning algorithms, were calculated and compared to predict the final exam grades of the students. The dataset consisted of the academic achievement grades of 1854 students who took the Turkish Language-I course in a state University in Turkey during the fall semester of 2019–2020. The results show that the proposed model achieved a classification accuracy of 70–75%. The predictions were made using only three types of parameters; midterm exam grades, Department data and Faculty data. Such data-driven studies are very important in terms of establishing a learning analysis framework in higher education and contributing to the decision-making processes. Finally, this study presents a contribution to the early prediction of students at high risk of failure and determines the most effective machine learning methods.

Introduction
The application of data mining methods in the field of education has attracted great attention in recent years. Data Mining (DM) is the discovery of data. It is the field of discovering new and potentially useful information or meaningful results from big data (Witten et al., 2011 ). It also aims to obtain new trends and new patterns from large datasets by using different classification algorithms (Baker & Inventado, 2014 ).
Educational data mining (EDM) is the use of traditional DM methods to solve problems related to education (Baker & Yacef, 2009 ; cited in Fernandes et al., 2019 ). EDM is the use of DM methods on educational data such as student information, educational records, exam results, student participation in class, and the frequency of students' asking questions. In recent years, EDM has become an effective tool used to identify hidden patterns in educational data, predict academic achievement, and improve the learning/teaching environment.
Learning analytics has gained a new dimension through the use of EDM (Waheed et al., 2020 ). Learning analytics covers the various aspects of collecting student information together, better understanding the learning environment by examining and analysing it, and revealing the best student/teacher performance (Long & Siemens, 2011 ). Learning analytics is the compilation, measurement and reporting of data about students and their contexts in order to understand and optimize learning and the environments in which it takes place. It also deals with the institutions developing new strategies.
Another dimension of learning analytics is predicting student academic performance, uncovering patterns of system access and navigational actions, and determining students who are potentially at risk of failing (Waheed et al., 2020 ). Learning management systems (LMS), student information systems (SIS), intelligent teaching systems (ITS), MOOCs, and other web-based education systems leave digital data that can be examined to evaluate students' possible behavior. Using EDM method, these data can be employed to analyse the activities of successful students and those who are at risk of failure, to develop corrective strategies based on student academic performance, and therefore to assist educators in the development of pedagogical methods (Casquero et al., 2016 ; Fidalgo-Blanco et al., 2015 ).
The data collected on educational processes offer new opportunities to improve the learning experience and to optimize users' interaction with technological platforms (Shorfuzzaman et al., 2019 ). The processing of educational data yields improvements in many areas such as predicting student behaviour, analytical learning, and new approaches to education policies (Capuano & Toti, 2019 ; Viberg et al., 2018 ). This comprehensive collection of data will not only allow education authorities to make data-based policies, but also form the basis of software to be developed with artificial intelligence on the learning process.
EDM enables educators to predict situations such as dropping out of school or less interest in the course, analyse internal factors affecting their performance, and make statistical techniques to predict students' academic performance. A variety of DM methods are employed to predict student performance, identify slow learners, and dropouts (Hardman et al., 2013 ; Kaur et al., 2015 ). Early prediction is a new phenomenon that includes assessment methods to support students by proposing appropriate corrective strategies and policies in this field (Waheed et al., 2020 ).
Especially during the pandemic period, learning management systems, quickly put into practice, have become an indispensable part of higher education. While students use these systems, the log records produced have become ever more accessible. (Macfadyen & Dawson, 2010 ; Kotsiantis et al., 2013 ; Saqr et al., 2017 ). Universities now should improve the capacity of using these data to predict academic success and ensure student progress (Bernacki et al., 2020 ).
As a result, EDM provides the educators with new information by discovering hidden patterns in educational data. Using this model, some aspects of the education system can be evaluated and improved to ensure the quality of education.
In various studies on EDM, e-learning systems have been successfully analysed (Lara et al., 2014 ). Some studies have also classified educational data (Chakraborty et al., 2016 ), while some have tried to predict student performance (Fernandes et al., 2019 ).
Asif et al. ( 2017 ) focused on two aspects of the performance of undergraduate students using DM methods. The first aspect is to predict the academic achievements of students at the end of a four-year study program. The second one is to examine the development of students and combine them with predictive results. He divided the students into two parts as low achievement and high achievement groups. He have found that it is important for the educators to focus on a small number of courses indicating particularly good or poor performance in order to offer timely warnings, support underperforming students and offer advice and opportunities to high-performing students. Cruz-Jesus et al. ( 2020 ) predicted student academic performance with 16 demographics such as age, gender, class attendance, internet access, computer possession, and the number of courses taken. Random forest, logistic regression, k-nearest neighbours and support vector machines, which are among the machine learning methods, were able to predict students’ performance with accuracy ranging from 50 to 81%.
Fernandes et al. ( 2019 ) developed a model with the demographic characteristics of the students and the achievement grades obtained from the in-term activities. In that study, students' academic achievement was predicted with classification models based on Gradient Boosting Machine (GBM). The results showed that the best qualities for estimating achievement scores were the previous year's achievement scores and unattendance. The authors found that demographic characteristics such as neighbourhood, school and age information were also potential indicators of success or failure. In addition, he argued that this model could guide the development of new policies to prevent failure. Similarly, by using the student data requested during registration and environmental factors, Hoffait and Schyns ( 2017 ) determined the students with the potential to fail. He found that students with potential difficulties could be classified more precisely by using DM methods. Moreover, their approach makes it possible to rank the students by levels of risk. Rebai et al. ( 2020 ) proposed a machine learning-based model to identify the key factors affecting academic performance of schools and to determine the relationship between these factors. He concluded that the regression trees showed that the most important factors associated with higher performance were school size, competition, class size, parental pressure, and gender proportions. In addition, according to the random forest algorithm results, the school size and the percentage of girls had a powerful impact on the predictive accuracy of the model.
Ahmad and Shahzadi, ( 2018 ) proposed a machine learning-based model to find an answer to the question whether students were at risk regarding their academic performance. Using the students' learning skills, study habits, and academic interaction features, they made a prediction with a classification accuracy of 85%. The researchers concluded that the model they proposed could be used to determine academically unsuccessful student. Musso et al., ( 2020 ) proposed a machine learning model based on learning strategies, perception of social support, motivation, socio-demographics, health condition, and academic performance characteristics. With this model, he predicted the academic performance and dropouts. He concluded that the predictive variable with the highest effect on predicting GPA was learning strategies while the variable with the greatest effect on determining dropouts was background information.
Waheed et al., ( 2020 ) designed a model with artificial neural networks on students' records related to their navigation through the LMS. The results showed that demographics and student clickstream activities had a significant impact on student performance. Students who navigated through courses performed higher. Students' participation in the learning environment had nothing to do with their performance. However, he concluded that the deep learning model could be an important tool in the early prediction of student performance. Xu et al. ( 2019 ) determined the relationship between the internet usage behaviors of university students and their academic performance and he predicted students’ performance with machine learning methods. The model he proposed predicted students' academic performance at a high level of accuracy. The results suggested that Internet connection frequency features were positively correlated with academic performance, whereas Internet traffic volume features were negatively correlated with academic performance. In addition, he concluded that internet usage features had an important role on students' academic performance. Bernacki et al. ( 2020 ) tried to find out whether the log records in the learning management system alone would be sufficient to predict achievement. He concluded that the behaviour-based prediction model successfully predicted 75% of those who would need to repeat a course. He also stated that, with this model, students who might be unsuccessful in the subsequent semesters could be identified and supported. Burgos et al. ( 2018 ) predicted the achievement grades that the students might get in the subsequent semesters and designed a tool for students who were likely to fail. He found that the number of unsuccessful students decreased by 14% compared to previous years. A comparative analysis of studies predicting the academic achievement grades using machine learning methods is given in Table 1 .
A review of previous research that aimed to predict academic achievement indicates that researchers have applied a range of machine learning algorithms, including multiple, probit and logistic regression, neural networks, and C4.5 and J48 decision trees. However, random forests (Zabriskie et al., 2019 ), genetic programming (Xing et al., 2015 ), and Naive Bayes algorithms (Ornelas & Ordonez, 2017 ) were used in recent studies. The prediction accuracy of these models reaches very high levels.
Prediction accuracy of student academic performance requires an deep understanding of the factors and features that impact student results and the achievement of student (Alshanqiti & Namoun, 2020 ). For this purpose, Hellas et al. ( 2018 ) reviewed 357 articles on student performance detailing the impact of 29 features. These features were mainly related to psychomotor skills such as course and pre-course performance, student participation, student demographics such as gender, high school performance, and self-regulation. However, the dropout rates were mainly influenced by student motivation, habits, social and financial issues, lack of progress, and career transitions.
The literature review suggests that, it is a necessity to improve the quality of education by predicting the academic performance of the students and supporting those who are in the risk group. In the literature, the prediction of academic performance was made with many and various variables, various digital traces left by students on the internet (browsing, lesson time, percentage of participation) (Fernandes et al., 2019 ; Rubin et al., 2010 ; Waheed et al., 2020 ; Xu et al., 2019 ) and students demographic characteristics (gender, age, economic status, number of courses attended, internet access, etc.) (Bernacki et al., 2020 ; Rizvi et al., 2019 ; García-González & Skrita, 2019 ; Rebai et al., 2020 ; Cruz-Jesus et al., 2020 ; Aydemir, 2017 ), learning skills, study approaches, study habits (Ahmad & Shahzadi, 2018 ), learning strategies, social support perception, motivation, socio-demography, health form, academic performance characteristics (Costa-Mendes et al., 2020 ; Gök, 2017 ; Kılınç, 2015 ; Musso et al., 2020 ), homework, projects, quizzes (Kardaş & Güvenir, 2020 ), etc. In almost all models developed in such studies, prediction accuracy is ranging from 70 to 95%. Hovewer, collecting and processing such a variety of data both takes a lot of time and requires expert knowledge. Similarly, Hoffait and Schyns ( 2017 ) suggested that collecting so many data is difficult and socio-economic data are unnecessary. Moreover, these demographic or socio-economic data may not always give the right idea of preventing failure (Bernacki et al., 2020 ).
The study concerns predicting students’ academic achievement using grades only, no demographic characteristics and no socio-economic data. This study aimed to develop a new model based on machine learning algorithms to predict the final exam grades of undergraduate students taking their midterm exam grades, Faculty and Department of the students.
For this purpose, classification algorithms with the highest performance in predicting students’ academic achievement were determined by using machine learning classification algorithms. The reason for choosing the Turkish Language-I course was that it is a compulsory course that all students enrolled in the university must take. Using this model, students’ final exam grades were predicted. These models will enable the development of pedagogical interventions and new policies to improve students' academic performance. In this way, the number of potentially unsuccessful students can be reduced following the assessments made after each midterm.
This section describes the details of the dataset, pre-processing techniques, and machine learning algorithms employed in this study.
Educational institutions regularly store all data that are available about students in electronic medium. Data are stored in databases for processing. These data can be of many types and volumes, from students’ demographics to their academic achievements. In this study, the data were taken from the Student Information System (SIS), where all student records are stored at a State University in Turkey. In these records, the midterm exam grades, final exam grades, Faculty, and Department of 1854 students who have taken the Turkish Language-I course in the 2019–2020 fall semester were selected as the dataset. Table 2 shows the distribution of students according to the academic unit. Moreover, as a additional file 1 the dataset are presented.
Midterm and final exam grades are ranging from 0 to 100. In this system, the end-of-semester achievement grade is calculated by taking 40% of the midterm exam and 60% of the final exam. Students with achievement grade below 60 are unsuccessful and those above 60 are successful. The midterm exam is usually held in the middle of the academic semester and the final exam is held at the end of the semester. There are approximately 9 weeks (2.5 months) from the midterm exam to the final exam. In other words, there is a two and a half month period for corrective actions for students who are at risk of failing thanks to the final exam predictions made. In other words, the answer to the question of how effective the student's performance in the middle of the semester is on his performance at the end of the semester was investigated.
Data identification and collection
At this phase, it is determined from which source the data will be stored, which features of the data will be used, and whether the collected data is suitable for the purpose. Feature selection involves decreasing the number of variables used to predict a particular outcome. The goal; to facilitate the interpretability of the model, reduce complexity, increase the computational efficiency of algorithms, and avoid overfitting.
Establishing DM model and implementation of algorithm
RF, NN, LR, SVM, NB and kNN were employed to predict students' academic performance. The prediction accuracy was evaluated using tenfold cross validation. The DM process serves two main purposes. The first purpose is to make predictions by analyzing the data in the database (predictive model). The second one is to describe behaviors (descriptive model). In predictive models, a model is created by using data with known results. Then, using this model, the result values are predicted for datasets whose results are unknown. In descriptive models, the patterns in the existing data are defined to make decisions.
When the focus is on analysing the causes of success or failure, statistical methods such as logistic regression and time series can be employed (Ortiz & Dehon, 2008 ; Arias Ortiz & Dehon, 2013 ). However, when the focus is on forecasting, neural networks (Delen, 2010 ; Vandamme et al., 2007 ), support vector machines (Huang & Fang, 2013 ), decision trees (Delen, 2011 ; Nandeshwar et al., 2011 ) and random forests (Delen, 2010 ; Vandamme et al., 2007 ) is more efficient and give more accurate results. Statistical techniques are to create a model that can successfully predict output values based on available input data. On the other hand, machine learning methods automatically create a model that matches the input data with the expected target values when a supervised optimization problem is given.
The performance of the model was measured by confusion matrix indicators. It is understood from the literature that there is no single classifier that works best for prediction results. Therefore, it is necessary to investigate which classifiers are more studied for the analysed data (Asif et al., 2017 ).
Experiments and results
The entire experimental phase was performed with Orange machine learning software. Orange is a powerful and easy-to-use component-based DM programming tool for expert data scientists as well as for data science beginners. In Orange, data analysis is done by stacking widgets into workflows. Each widget includes some data retrieval, data pre-processing, visualization, modelling, or evaluation task. A workflow is a series of actions or actions that will be performed on the platform to perform a specific task. Comprehensive data analysis charts can be created by combining different components in a workflow. Figure 1 shows the workflow diagram designed.
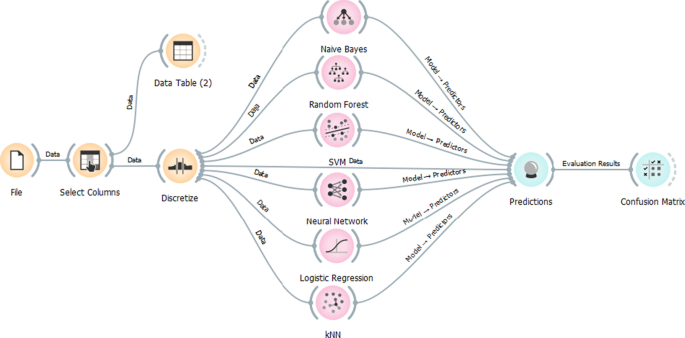
The workflow of the designed model
The dataset included midterm exam grades, final exam grades, Faculty, and Department of 1854 students taking the Turkish Language-I course in the 2019–2020 Fall Semester. The entire dataset is provided as Additional file 1 . Table 3 shows part of the dataset.
In the dataset, students' midterm exam grades, final exam grades, faculty, and department information were determined as features. Each measure contains data associated with a student. Midterm exam and final exam grade variables were explained under the heading "dataset". The faculty variable represents Faculties in Kırşehir Ahi Evran University and the department variable represents departments in faculties. In the development of the model, the midterm, the faculty, and the department information were determined as the independent variable and the final was determined as the dependent variable. Table 4 shows the variable model.
After the variable model was determined, the midterm exam grades and final exam grades were categorized according to the equal-width discretization model. Table 5 shows the criteria used in converting midterm exam grades and final exam grades into the categorical format.
In Table 6 , the values in the final column are the actual values. The values in the RF, SVM, LR, KNN, NB, and NN columns are the values predicted by the proposed model. For example, according to Table 5 , std1’s actual final grade was in the range 55 to 77. While the predicted value of the RF, SVM, LR, NB, and NN models were in the range of, the predicted value of the kNN model was greater than 77.
Evaluation of the model performance
The performance of model was evaluated with confusion matrix, classification accuracy (CA), precision, recall, f-score (F1), and area under roc curve (AUC) metrics.
Confusion matrix
The confusion matrix shows the current situation in the dataset and the number of correct/incorrect predictions of the model. Table 7 shows the confusion matrix. The performance of the model is calculated by the number of correctly classified instances and incorrectly classified instances. The rows show the real numbers of the samples in the test set, and the columns represent the estimation of the model.
In Table 6 , true positive (TP) and true negative (TN) show the number of correctly classified instances. False positive (FP) shows the number of instances predicted as 1 (positive) while it should be in the 0 (negative) class. False negative (FN) shows the number of instances predicted as 0 (negative) while it should be in class 1 (positive).
Table 8 shows the confusion matrix for the RF algorithm. In the confusion matrix of 4 × 4 dimensions, the main diagonal shows the percentage of correctly predicted instances, and the matrix elements other than the main diagonal shows the percentage of errors predicted.
Table 8 shows that 84.9% of those with the actual final grade greater than 77.5, 71.2% of those with range 55–77.5, 65.4% of those with range 32.5–55, and 60% of those with less than 32.5 were predicted correctly. Confusion matrixs of other algorithms are shown in Tables 9 , 10 , 11 , 12 , and 13 .
Classification accuracy: CA is the ratio of the correct predictions (TP + TN) to the total number of instances (TP + TN + FP + FN).
Precision: Precision is the ratio of the number of positive instances that are correctly classified to the total number of instances that are predicted positive. Gets a value in the range [0.1].
Recall: Recall i s the ratio of the correctly classified number of positive instances to the number of all instances whose actual class is positive. The Recall is also called the true positive rate. Gets a value in the range [0.1].
F-Criterion (F1): There is an opposite relationship between precision and recall. Therefore, the harmonic mean of both criteria is calculated for more accurate and sensitive results. This is called the F-criterion.
Receiver operating characteristics (ROC) curve
The AUC-ROC curve is used to evaluate the performance of a classification problem. AUC-ROC is a widely used metric to evaluate the performance of machine learning algorithms, especially in cases where there are unbalanced datasets, and explains how well the model is at predicting.
AUC: Area under the ROC curve
The larger the area covered, the better the machine learning algorithms at distinguishing given classes. AUC for the ideal value is 1. The AUC, Classification Accuracy (CA), F-Criterion (F1), precision, and recall values of the models are shown in Table 14 .
The AUC value of RF, NN, SVM, LR, NB, and kNN algorithms were 0.860, 0.863, 0.804, 0.826, 0.810, and 0.810 respectively. The classification accuracy of the RF, NN, SVM, LR, NB, and kNN algorithms were also 0.746, 0.746, 0.735, 0.717, 0.713, and 0,699 respectively. According to these findings, for example, the RF algorithm was able to achieve 74.6% accuracy. In other words, there was a very high-level correlation between the data predicted and the actual data. As a result, 74.6% of the samples were been classified correctly.
Discussion and conclusion
This study proposes a new model based on machine learning algorithms to predict the final exam grades of undergraduate students, taking their midterm exam grades as the source data. The performances of the Random Forests, nearest neighbour, support vector machines, Logistic Regression, Naïve Bayes, and k-nearest neighbour algorithms, which are among the machine learning algorithms, were calculated and compared to predict the final exam grades of the students. This study focused on two parameters. The first parameter was the prediction of academic performance based on previous achievement grades. The second one was the comparison of performance indicators of machine learning algorithms.
The results show that the proposed model achieved a classification accuracy of 70–75%. According to this result, it can be said that students' midterm exam grades are an important predictor to be used in predicting their final exam grades. RF, NN, SVM, LR, NB, and kNN are algorithms with a very high accuracy rate that can be used to predict students' final exam grades. Furthermore, the predictions were made using only three types of parameters; midterm exam grades, Department data and Faculty data. The results of this study were compared with the studies that predicted the academic achievement grades of the students with various demographic and socio-economic variables. Hoffait and Schyns ( 2017 ) proposed a model that uses the academic achievement of students in previous years. With this model, they predicted students' performance to be successful in the courses they will take in the new semester. They found that 12.2% of the students had a very high risk of failure, with a 90% confidence rate. Waheed et al. ( 2020 ) predicted the achievement of the students with demographic and geographic characteristics. He found that it has a significant effect on students' academic performance. He predicted the failure or success of the students by 85% accuracy. Xu et al. ( 2019 ) found that internet usage data can distinguish and predict students' academic performance. Costa-Mendes et al. ( 2020 ), Cruz-Jesus et al. ( 2020 ), Costa-Mendes et al. ( 2020 ) predicted the academic achievement of students in the light of income, age, employment, cultural level indicators, place of residence, and socio-economic information. Similarly, Babić ( 2017 ) predicted students’ performance with an accuracy of 65% to 100% with artificial neural networks, classification tree, and support vector machines methods.
Another result of this study was RF, NN and SVM algorithms have the highest classification accuracy, while kNN has the lowest classification accuracy. According to this result, it can be said that RF, NN and SVM algorithms perform with more accurate results in predicting the academic achievement grades of students with machine learning algorithms. The results were compared with the results of the research in which machine learning algorithms were employed to predict academic performance according to various variables. For example, Hoffait and Schyns ( 2017 ) compared the performances of LR, ANN and RF algorithms to identify students at high risk of academic failure on their various demographic characteristics. They ranked the algorithms from those with the highest accuracy to the ones with the lowest accuracy as LR, ANN, and RF. On the other hand, Waheed et al. ( 2020 ) found that the SVM algorithm performed higher than the LR algorithm. According to Xu et al. ( 2019 ), the algorithm with the highest performance is SVM, followed by the NN algorithm, and the decision tree is the algorithm with the lowest performance.
The proposed model predicted the final exam grades of students with 73% accuracy. According to this result, it can be said that academic achievement can be predicted with this model in the future. By predicting students' achievement grades in future, students can be allowed to review their working methods and improve their performance. The importance of the proposed method can be better understood, considering that there is approximately 2.5 months between the midterm exams and the final exams in higher education. Similarly, Bernacki et al. ( 2020 ) work on the early warning model. He proposed a model to predict the academic achievements of students using their behavior data in the learning management system before the first exam. His algorithm correctly identified 75% of students who failed to earn the grade of B or better needed to advance to the next course. Ahmad and Shahzadi ( 2018 ) predicted students at risk for academic performance with 85% accuracy evaluating their study habits, learning skills, and academic interaction features. Cruz-Jesus et al. ( 2020 ) predicted students' end-of-semester grades with 16 independent variables. He concluded that students could be given the opportunity of early intervention.
As a result, students' academic performances were predicted using different predictors, different algorithms and different approaches. The results confirm that machine learning algorithms can be used to predict students’ academic performance. More importantly, the prediction was made only with the parameters of midterm grade, faculty and department. Teaching staff can benefit from the results of this research in the early recognition of students who have below or above average academic motivation. Later, for example, as Babić ( 2017 ) points out, they can match students with below-average academic motivation by students with above-average academic motivation and encourage them to work in groups or project work. In this way, the students' motivation can be improved, and their active participation in learning can be ensured. In addition, such data-driven studies should assist higher education in establishing a learning analytics framework and contribute to decision-making processes.
Future research can be conducted by including other parameters as input variables and adding other machine learning algorithms to the modelling process. In addition, it is necessary to harness the effectiveness of DM methods to investigate students' learning behaviors, address their problems, optimize the educational environment, and enable data-driven decision making.
Availability of data and materials
The datasets used and/or analyzed during the current study are available from the corresponding author on reasonable request.
Abbreviations
- Educational data mining
Random forests
Neural networks
Support vector machines
Logistic regression
Naïve Bayes
K-nearest neighbour
Decision trees
Artificial neural networks
Extremely randomized trees
Regression trees
Multilayer perceptron neural network
Feed-forward neural network
Adaptive resonance theory mapping
Learning management systems
Student information systems
Intelligent teaching systems
Classification accuracy
Area under roc curve
True positive
True negative
False positive
False negative
Receiver operating characteristics
Ahmad, Z., & Shahzadi, E. (2018). Prediction of students’ academic performance using artificial neural network. Bulletin of Education and Research, 40 (3), 157–164.
Google Scholar
Alshanqiti, A., & Namoun, A. (2020). Predicting student performance and its influential factors using hybrid regression and multi-label classification. IEEE Access, 8 , 203827–203844. https://doi.org/10.1109/access.2020.3036572
Article Google Scholar
Arias Ortiz, E., & Dehon, C. (2013). Roads to success in the Belgian French Community’s higher education system: predictors of dropout and degree completion at the Université Libre de Bruxelles. Research in Higher Education, 54 (6), 693–723. https://doi.org/10.1007/s11162-013-9290-y
Asif, R., Merceron, A., Ali, S. A., & Haider, N. G. (2017). Analyzing undergraduate students’ performance using educational data mining. Computers and Education, 113 , 177–194. https://doi.org/10.1016/j.compedu.2017.05.007
Aydemir, B. (2017). Predicting academic success of vocational high school students using data mining methods graduate . [Unpublished master’s thesis]. Pamukkale University Institute of Science.
Babić, I. D. (2017). Machine learning methods in predicting the student academic motivation. Croatian Operational Research Review, 8 (2), 443–461. https://doi.org/10.17535/crorr.2017.0028
Baker, R. S., & Inventado, P. S. (2014). Educational data mining and learning analytics. Learning analytics (pp. 61–75). Springer.
Chapter Google Scholar
Baker, R. S., & Yacef, K. (2009). The state of educational data mining in 2009: A review and future visions. Journal of Educational Data Mining, 1 (1), 3–17.
Bernacki, M. L., Chavez, M. M., & Uesbeck, P. M. (2020). Predicting achievement and providing support before STEM majors begin to fail. Computers & Education, 158 (August), 103999. https://doi.org/10.1016/j.compedu.2020.103999
Burgos, C., Campanario, M. L., De, D., Lara, J. A., Lizcano, D., & Martínez, M. A. (2018). Data mining for modeling students’ performance: A tutoring action plan to prevent academic dropout. Computers and Electrical Engineering, 66 (2018), 541–556. https://doi.org/10.1016/j.compeleceng.2017.03.005
Capuano, N., & Toti, D. (2019). Experimentation of a smart learning system for law based on knowledge discovery and cognitive computing. Computers in Human Behavior, 92 , 459–467. https://doi.org/10.1016/j.chb.2018.03.034
Casquero, O., Ovelar, R., Romo, J., Benito, M., & Alberdi, M. (2016). Students’ personal networks in virtual and personal learning environments: A case study in higher education using learning analytics approach. Interactive Learning Environments, 24 (1), 49–67. https://doi.org/10.1080/10494820.2013.817441
Chakraborty, B., Chakma, K., & Mukherjee, A. (2016). A density-based clustering algorithm and experiments on student dataset with noises using Rough set theory. In Proceedings of 2nd IEEE international conference on engineering and technology, ICETECH 2016 , March (pp. 431–436). https://doi.org/10.1109/ICETECH.2016.7569290
Costa-Mendes, R., Oliveira, T., Castelli, M., & Cruz-Jesus, F. (2020). A machine learning approximation of the 2015 Portuguese high school student grades: A hybrid approach. Education and Information Technologies, 26 , 1527–1547. https://doi.org/10.1007/s10639-020-10316-y
Cruz-Jesus, F., Castelli, M., Oliveira, T., Mendes, R., Nunes, C., Sa-Velho, M., & Rosa-Louro, A. (2020). Using artificial intelligence methods to assess academic achievement in public high schools of a European Union country. Heliyon . https://doi.org/10.1016/j.heliyon.2020.e04081
Delen, D. (2010). A comparative analysis of machine learning techniques for student retention management. Decision Support Systems, 49 (4), 498–506. https://doi.org/10.1016/j.dss.2010.06.003
Delen, D. (2011). Predicting student attrition with data mining methods. Journal of College Student Retention: Research, Theory and Practice, 13 (1), 17–35. https://doi.org/10.2190/CS.13.1.b
Fernandes, E., Holanda, M., Victorino, M., Borges, V., Carvalho, R., & Van Erven, G. (2019). Educational data mining : Predictive analysis of academic performance of public school students in the capital of Brazil. Journal of Business Research, 94 (February 2018), 335–343. https://doi.org/10.1016/j.jbusres.2018.02.012
Fidalgo-Blanco, Á., Sein-Echaluce, M. L., García-Peñalvo, F. J., & Conde, M. Á. (2015). Using Learning Analytics to improve teamwork assessment. Computers in Human Behavior, 47 , 149–156. https://doi.org/10.1016/j.chb.2014.11.050
García-González, J. D., & Skrita, A. (2019). Predicting academic performance based on students’ family environment: Evidence for Colombia using classification trees. Psychology, Society and Education, 11 (3), 299–311. https://doi.org/10.25115/psye.v11i3.2056
Gök, M. (2017). Predicting academic achievement with machine learning methods. Gazi University Journal of Science Part c: Design and Technology, 5 (3), 139–148.
Hardman, J., Paucar-Caceres, A., & Fielding, A. (2013). Predicting students’ progression in higher education by using the random forest algorithm. Systems Research and Behavioral Science, 30 (2), 194–203. https://doi.org/10.1002/sres.2130
Hellas, A., Ihantola, P., Petersen, A., Ajanovski, V.V., Gutica, M., Hynninen, T., Knutas, A., Leinonen, J., Messom, C., & Liao, S.N. (2018). Predicting academic performance: a systematic literature review. In Proceedings companion of the 23rd annual ACM conference on innovation and technology in computer science education (pp. 175–199).
Hoffait, A., & Schyns, M. (2017). Early detection of university students with potential difficulties. Decision Support Systems, 101 (2017), 1–11. https://doi.org/10.1016/j.dss.2017.05.003
Huang, S., & Fang, N. (2013). Predicting student academic performance in an engineering dynamics course: A comparison of four types of predictive mathematical models. Computers and Education, 61 (1), 133–145. https://doi.org/10.1016/j.compedu.2012.08.015
Kardaş, K., & Güvenir, A. (2020). Analysis of the effects of Quizzes, homeworks and projects on final exam with different machine learning techniques. EMO Journal of Scientific, 10 (1), 22–29.
Kaur, P., Singh, M., & Josan, G. S. (2015). Classification and prediction based data mining algorithms to predict slow learners in education sector. Procedia Computer Science, 57 , 500–508. https://doi.org/10.1016/j.procs.2015.07.372
Kılınç, Ç. (2015). Examining the effects on university student success by data mining techniques. [Unpublished master’s thesis]. Eskişehir Osmangazi University Institute of Science.
Kotsiantis, S., Tselios, N., Filippidi, A., & Komis, V. (2013). Using learning analytics to identify successful learners in a blended learning course. International Journal of Technology Enhanced Learning, 5 (2), 133–150. https://doi.org/10.1504/IJTEL.2013.059088
Lara, J. A., Lizcano, D., Martínez, M. A., Pazos, J., & Riera, T. (2014). A system for knowledge discovery in e-learning environments within the European Higher Education Area—Application to student data from Open University of Madrid, UDIMA. Computers and Education, 72 , 23–36. https://doi.org/10.1016/j.compedu.2013.10.009
Long, P., & Siemens, G. (2011). Penetrating the fog: Analytics in learning and education. Educause Review, 46 (5), 31–40.
Macfadyen, L. P., & Dawson, S. (2010). Mining LMS data to develop an “early warning system” for educators: A proof of concept. Computers & Education, 54 (2), 588–599. https://doi.org/10.1016/j.compedu.2009.09.008
Musso, M. F., Hernández, C. F. R., & Cascallar, E. C. (2020). Predicting key educational outcomes in academic trajectories: A machine-learning approach. Higher Education, 80 (5), 875–894. https://doi.org/10.1007/s10734-020-00520-7
Nandeshwar, A., Menzies, T., & Nelson, A. (2011). Learning patterns of university student retention. Expert Systems with Applications, 38 (12), 14984–14996. https://doi.org/10.1016/j.eswa.2011.05.048
Ornelas, F., & Ordonez, C. (2017). Predicting student success: A naïve bayesian application to community college data. Technology, Knowledge and Learning, 22 (3), 299–315. https://doi.org/10.1007/s10758-017-9334-z
Ortiz, E. A., & Dehon, C. (2008). What are the factors of success at University? A case study in Belgium. Cesifo Economic Studies, 54 (2), 121–148. https://doi.org/10.1093/cesifo/ifn012
Rebai, S., Ben Yahia, F., & Essid, H. (2020). A graphically based machine learning approach to predict secondary schools performance in Tunisia. Socio-Economic Planning Sciences, 70 (August 2018), 100724. https://doi.org/10.1016/j.seps.2019.06.009
Rizvi, S., Rienties, B., & Ahmed, S. (2019). The role of demographics in online learning; A decision tree based approach. Computers & Education, 137 (August 2018), 32–47. https://doi.org/10.1016/j.compedu.2019.04.001
Rubin, B., Fernandes, R., Avgerinou, M. D., & Moore, J. (2010). The effect of learning management systems on student and faculty outcomes. The Internet and Higher Education, 13 (1–2), 82–83. https://doi.org/10.1016/j.iheduc.2009.10.008
Saqr, M., Fors, U., & Tedre, M. (2017). How learning analytics can early predict under-achieving students in a blended medical education course. Medical Teacher, 39 (7), 757–767. https://doi.org/10.1080/0142159X.2017.1309376
Shorfuzzaman, M., Hossain, M. S., Nazir, A., Muhammad, G., & Alamri, A. (2019). Harnessing the power of big data analytics in the cloud to support learning analytics in mobile learning environment. Computers in Human Behavior, 92 (February 2017), 578–588. https://doi.org/10.1016/j.chb.2018.07.002
Vandamme, J.-P., Meskens, N., & Superby, J.-F. (2007). Predicting academic performance by data mining methods. Education Economics, 15 (4), 405–419. https://doi.org/10.1080/09645290701409939
Viberg, O., Hatakka, M., Bälter, O., & Mavroudi, A. (2018). The current landscape of learning analytics in higher education. Computers in Human Behavior, 89 (July), 98–110. https://doi.org/10.1016/j.chb.2018.07.027
Waheed, H., Hassan, S. U., Aljohani, N. R., Hardman, J., Alelyani, S., & Nawaz, R. (2020). Predicting academic performance of students from VLE big data using deep learning models. Computers in Human Behavior, 104 (October 2019), 106189. https://doi.org/10.1016/j.chb.2019.106189
Witten, I. H., Frank, E., & Hall, M. A. (2011). Data mining practical machine learning tools and techniques (3rd ed.). Morgan Kaufmann.
Xing, W., Guo, R., Petakovic, E., & Goggins, S. (2015). Participation-based student final performance prediction model through interpretable Genetic Programming: Integrating learning analytics, educational data mining and theory. Computers in Human Behavior, 47 , 168–181.
Xu, X., Wang, J., Peng, H., & Wu, R. (2019). Prediction of academic performance associated with internet usage behaviors using machine learning algorithms. Computers in Human Behavior, 98 (January), 166–173. https://doi.org/10.1016/j.chb.2019.04.015
Zabriskie, C., Yang, J., DeVore, S., & Stewart, J. (2019). Using machine learning to predict physics course outcomes. Physical Review Physics Education Research, 15 (2), 020120. https://doi.org/10.1103/PhysRevPhysEducRes.15.020120
Download references
Acknowledgements
Not applicable.
Author information
Authors and affiliations.
Kırşehir Ahi Evran University, Faculty of Engineering and Architecture, 40100, Kırşehir, Turkey
Mustafa Yağcı
You can also search for this author in PubMed Google Scholar
Contributions
All authors read and approved the final manuscript.
Corresponding author
Correspondence to Mustafa Yağcı .
Ethics declarations
Competing interests.
The authors declare that they have no competing interests.
Additional information
Publisher's note.
Springer Nature remains neutral with regard to jurisdictional claims in published maps and institutional affiliations.
Supplementary Information
Additional file 1:, rights and permissions.
Open Access This article is licensed under a Creative Commons Attribution 4.0 International License, which permits use, sharing, adaptation, distribution and reproduction in any medium or format, as long as you give appropriate credit to the original author(s) and the source, provide a link to the Creative Commons licence, and indicate if changes were made. The images or other third party material in this article are included in the article's Creative Commons licence, unless indicated otherwise in a credit line to the material. If material is not included in the article's Creative Commons licence and your intended use is not permitted by statutory regulation or exceeds the permitted use, you will need to obtain permission directly from the copyright holder. To view a copy of this licence, visit http://creativecommons.org/licenses/by/4.0/ .
Reprints and permissions
About this article
Cite this article.
Yağcı, M. Educational data mining: prediction of students' academic performance using machine learning algorithms. Smart Learn. Environ. 9 , 11 (2022). https://doi.org/10.1186/s40561-022-00192-z
Download citation
Received : 15 November 2021
Accepted : 15 February 2022
Published : 03 March 2022
DOI : https://doi.org/10.1186/s40561-022-00192-z
Share this article
Anyone you share the following link with will be able to read this content:
Sorry, a shareable link is not currently available for this article.
Provided by the Springer Nature SharedIt content-sharing initiative
- Machine learning
- Predicting achievement
- Learning analytics
- Early warning systems
- All topics »
- Fact sheets
- Feature stories
- Publications
- Questions & answers
- Tools and toolkits
- Coronavirus disease (COVID-19) pandemic
- Ukraine emergency
- Environment and health
Mpox (monkeypox)
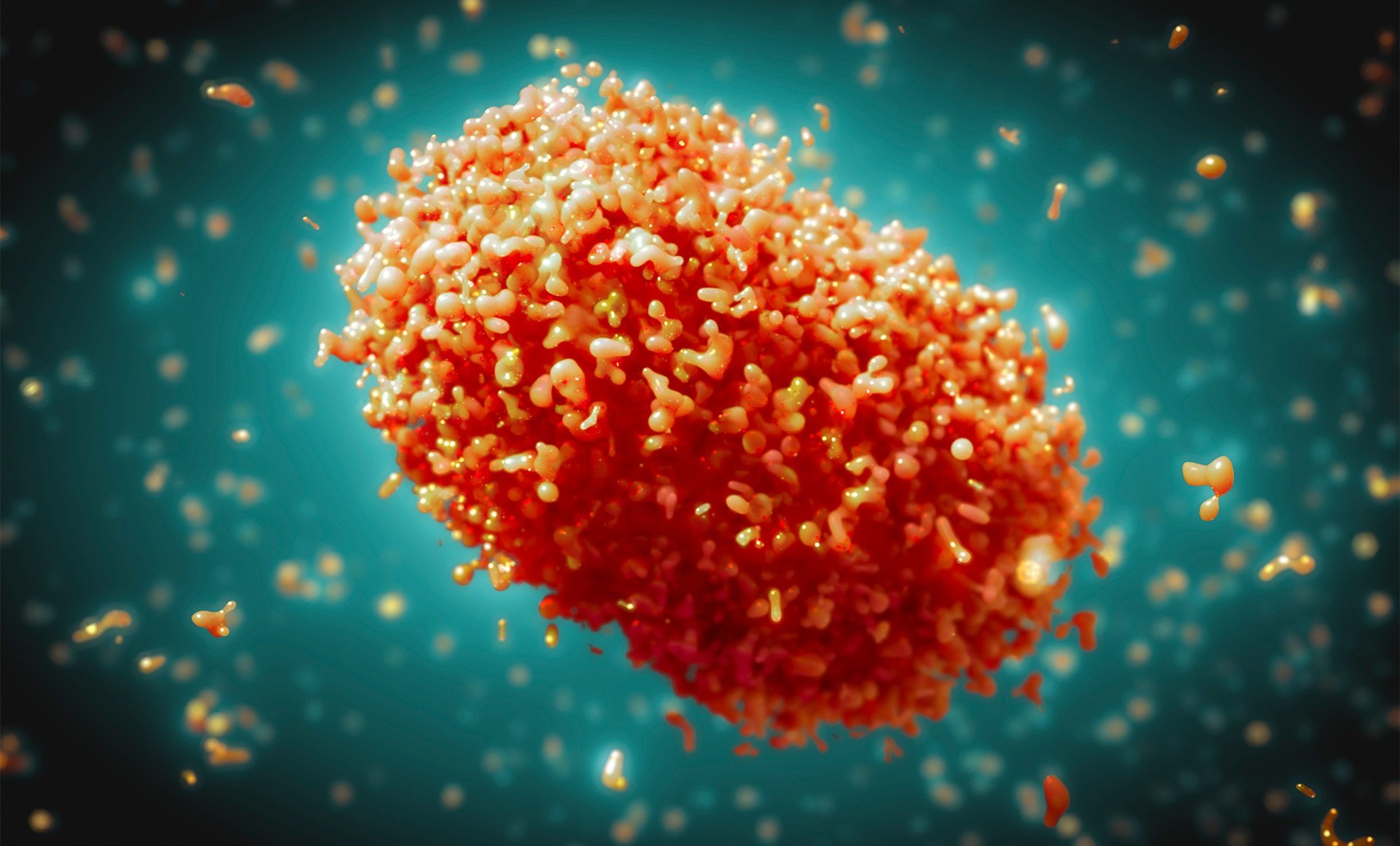
- Calls for experts
- Initiatives
- European Programme of Work
- Sustainable Development Goals
- The Pan-European Mental Health Coalition
- Empowerment through Digital Health
- The European Immunization Agenda 2030
- Healthier behaviours: incorporating behavioural and cultural insights
- Moving towards UHC
- Protecting against health emergencies
- Promoting health and well-being
- News stories
- Media releases
- Photo stories
- Questions and answers
Media Contacts
Newsletters
- European Health Information Gateway
- European health report
- Core health indicators
- WHO Immunization Data portal
- Noncommunicable diseases (NCD) dashboard
- Events
- Teams »
- Data and digital health
- Policy & Governance f. Health through the Life Course
- Groups and networks »
- Health Evidence Network (HEN)
The European Health Report 2021 »
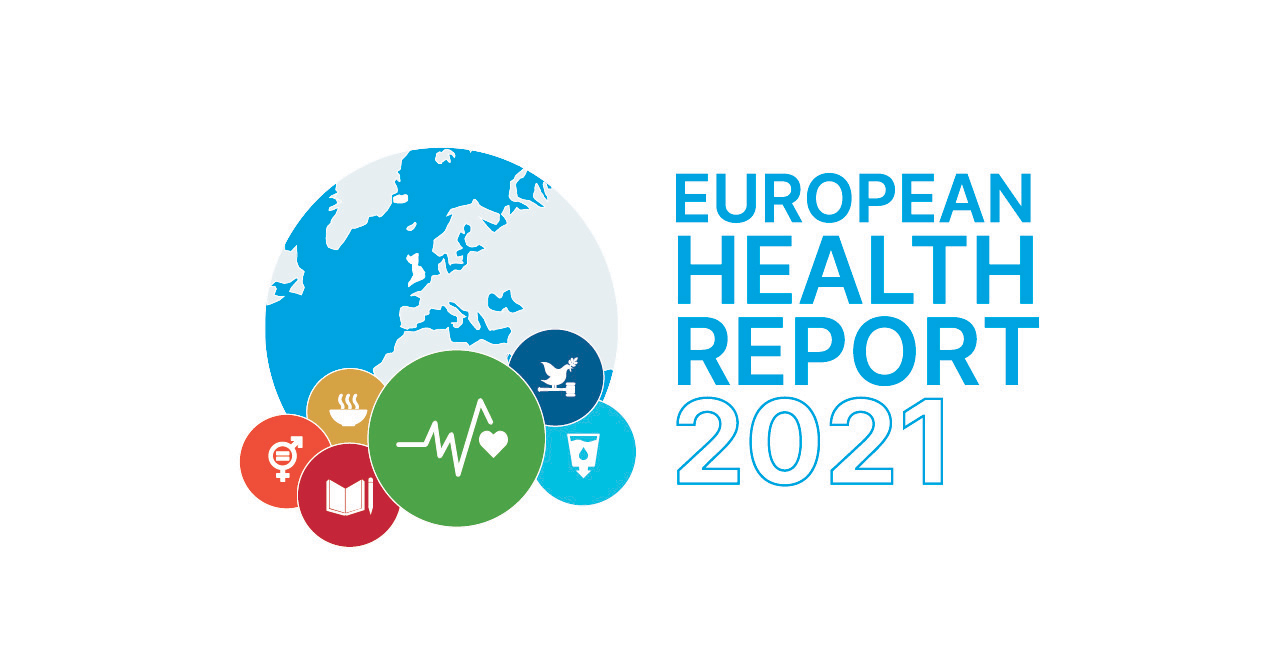
- Conflict in Israel and the occupied Palestinian territory
- Armenian refugee health response
- Climate crisis: extreme weather
- Türkiye and Syria earthquakes
- About health emergencies
- Health emergencies newsletter
- Health emergencies list
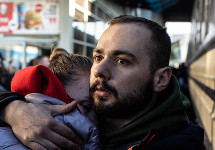
- Regional Director
- Executive Council
- Technical centres
- Faces of WHO
- Regional Committee for Europe
- Standing Committee
- Partners
- Groups and networks
- WHO collaborating centres
74th session of the WHO Regional Committee for Europe
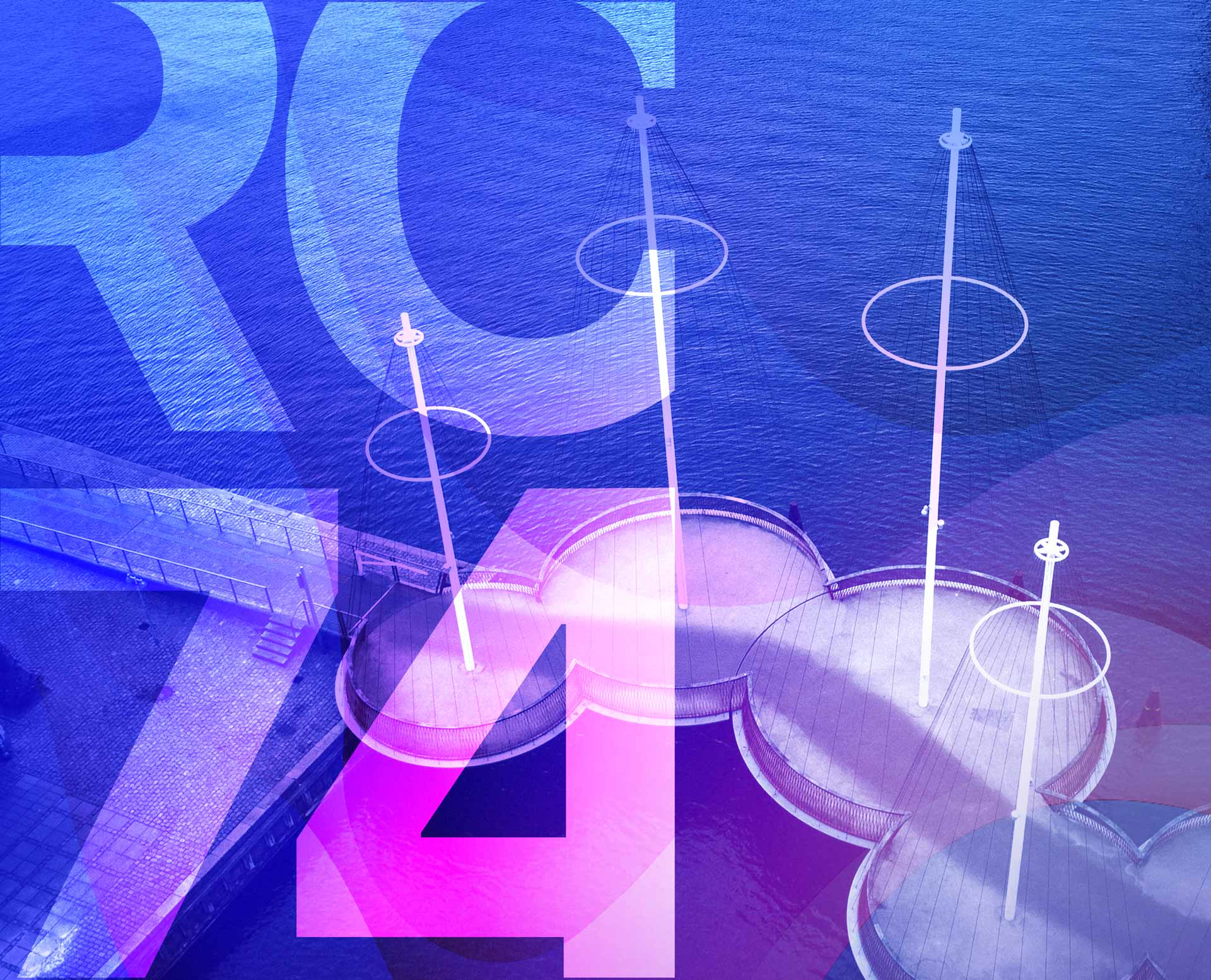
Alarming decline in adolescent condom use, increased risk of sexually transmitted infections and unintended pregnancies, reveals new WHO report
Copenhagen, 29 August 2024
New report reveals high rates of unprotected sex among adolescents across Europe, with significant implications for health and safety
An urgent report from the WHO Regional Office for Europe reveals that condom use among sexually active adolescents has declined significantly since 2014, with rates of unprotected sex worryingly high. This is putting young people at significant risk of sexually transmitted infections (STIs) and unplanned pregnancies. The new data were published as part of the multi-part Health Behaviour in School-aged Children (HBSC) study, which surveyed over 242 000 15-year-olds across 42 countries and regions in 2014–2022.
Far-reaching consequences of unprotected sex
Overall, the report highlights that a substantial proportion of sexually active 15-year-olds are engaging in unprotected sexual intercourse, which WHO warns can have far-reaching consequences for young people, including unintended pregnancies, unsafe abortions and an increased risk of contracting STIs. The high prevalence of unprotected sex indicates significant gaps in age-appropriate comprehensive sexuality education, including sexual health education, and access to contraceptive methods.
Worrying decline in condom use
Compared to 2014 levels, the new data show a significant decline in the number of adolescents reporting condom use during last sexual intercourse. From the data, it is clear that the decrease in condom use is pervasive, spanning multiple countries and regions, with some experiencing more dramatic reductions than others.
The report underscores the urgent need for targeted interventions to address these concerning trends and promote safer sexual practices among young people within the wider context of equipping them with the foundation they need for optimal health and well-being.
“While the report’s findings are dismaying, they are not surprising,” noted Dr Hans Henri P. Kluge, WHO Regional Director for Europe. “Age-appropriate comprehensive sexuality education remains neglected in many countries, and where it is available, it has increasingly come under attack in recent years on the false premise that it encourages sexual behaviour, when the truth is that equipping young persons with the right knowledge at the right time leads to optimal health outcomes linked to responsible behaviour and choices. We are reaping the bitter fruit of these reactionary efforts, with worse to come, unless governments, health authorities, the education sector and other essential stakeholders truly recognize the root causes of the current situation and take steps to rectify it. We need immediate and sustained action, underpinned by data and evidence, to halt this cascade of negative outcomes, including the likelihood of higher STI rates, increased health-care costs, and – not least – disrupted education and career paths for young persons who do not receive the timely information and support they need.”
Key findings from the report
- Decline in condom use: the proportion of sexually active adolescents who used a condom at last intercourse fell from 70% to 61% among boys and 63% to 57% among girls between 2014 and 2022.
- High rates of unprotected sex: almost a third of adolescents (30%) reported using neither a condom nor the contraceptive pill at last intercourse, a figure that has barely changed since 2018.
- Socioeconomic differences: adolescents from low-affluence families were more likely to report not using a condom or the contraceptive pill at last sexual intercourse than their peers from more affluent families (33% compared with 25%).
- Contraceptive pill use: the report indicates that contraceptive pill use during last sexual intercourse remained relatively stable between 2014 and 2022, with 26% of 15-year-olds reporting that they or their partners used the contraceptive pill at their last sexual intercourse.
Need for comprehensive sexuality education
The findings underscore the importance of providing comprehensive sexual health education and resources for young people. “As teenagers, having access to accurate information about sexual health is vital,” said Éabha, a 16-year-old from Ireland. “We need education that covers everything from consent to contraception, so we can make informed decisions and protect ourselves.”
“Comprehensive sexuality education is key to closing these gaps and empowering all young people to make informed decisions about sex at a particularly vulnerable moment in their lives, as they transition from adolescence to adulthood,” said Dr András Költő of the University of Galway, the lead author of the report. “But education must go beyond just providing information. Young people need safe spaces to discuss issues like consent, intimate relationships, gender identity and sexual orientation, and we – governments, health and education authorities, and civil society organizations – should help them develop crucial life skills including transparent, non-judgmental communication and decision-making.”
Roadmap for action, despite worrying trends
While the findings are sobering, they also offer a roadmap for the way ahead.
The report calls for sustainable investments in age-appropriate comprehensive sexuality education, youth-friendly sexual and reproductive health services, and enabling policies and environments that support adolescent health and rights.
“The findings of this report should serve as a catalyst for action. Adolescents deserve the knowledge and resources to make informed decisions about their sexual health. We have the evidence, the tools and the strategies to improve adolescent sexual health outcomes. What we need, though, is the political will and the resources to make it happen,” said Dr Margreet de Looze of Utrecht University, one of the report’s co-authors.
Call to action for policy-makers and educators
The WHO Regional Office for Europe calls upon policy-makers, educators and health-care providers to prioritize adolescent sexual health by:
- Investing in comprehensive sexuality education: implementing and funding evidence-based sexuality education programmes in schools that cover a wide range of topics, including contraception, STIs, consent, healthy relationships, gender equality and LGBTQIA+ (lesbian, gay, bisexual, transgender, queer, questioning, intersex, asexual, plus) issues. In this, the International Technical Guidance on Sexuality Education, produced by a consortium of United Nations agencies and partners, is key.
- Enhancing access to youth-friendly sexual health services: ensuring that adolescents everywhere have access to confidential, non-judgmental and affordable sexual health services that meet their specific needs and preferences.
- Promoting open dialogue: encouraging open and honest conversations about sexual health within families, schools and communities to reduce stigma and increase awareness.
- Training educators: providing specialized training for teachers and health-care providers to deliver effective and inclusive sex education. Such resources should be made available in both school and out-of-school settings.
- Conducting further research: investigating the underlying reasons for the decline in condom use and the variations in sexual health behaviours across different populations to inform targeted interventions. This includes analysing messages and other content adolescents are exposed to across social media and online platforms, given their reach and impact.
“Ultimately, what we are seeking to achieve for young persons is a solid foundation for life and love,” said Dr Kluge. “Sexual and reproductive health and rights, informed by the right knowledge at the right time along with the right health and well-being services, is critical. By empowering adolescents to make informed decisions about their sexual health, we ultimately safeguard and improve their overall well-being. This is what all parents and families should want for their children, everywhere.”
Communications officer
Bhanu Bhatnagar
Press & Media Relations Officer WHO Regional Office for Europe
Joseph Hancock
Communications Officer for the HBSC study
WHO/Europe Press Office
A focus on adolescent sexual health in Europe, central Asia and Canada: Health Behaviour in School-aged Children international report from the 2021/2022 survey
Health Behaviour in School-aged Children (HBSC) study

- Vision and Mission
- Diversity, Equity, Inclusion, and Belonging (DEIB)
- Volunteer Opportunities
- Parents and Caregivers
- Press Releases
- In Memoriam
- Annual Reports
- Data and Workforce
- MOCA-Peds: Research and Evaluation
- Milestones and EPAs Research
- Publications
- Data Requests
- About the Foundation
- Funding Information
- Behavioral and Mental Health
- Child and Family Health
- Roadmap Project
- Global Health
- Foundation Board of Directors
- Contact the Foundation
- Admission Requirements
- Absences from Training
- Waiver of Accredited Training
- Other Training Irregularities
- Pediatrics-Neurology
- Pediatrics-Neurodevelopmental Disabilities
- Allergy and Immunology
- Accelerated Research Pathway (ARP) Details
- Integrated Research Pathway (IRP)
- Alternative Pathway for Academic Faculty
- Residents & Fellows Evaluation & Tracking
- Program Directors Training Verification
- Time-Limited Eligibility FAQs
- Plan for Supervised Practice in General Pediatrics
- General Criteria for Subspecialty Certification
- Adolescent Medicine
- Pediatric Cardiology
- Child Abuse Pediatrics
- Developmental-Behavioral Pediatrics
- Neonatal-Perinatal Medicine
- Pediatric Critical Care Medicine
- Pediatric Emergency Medicine
- Pediatric Endocrinology
- Pediatric Gastroenterology
- Pediatric Hematology-Oncology
- Pediatric Hospital Medicine
- Pediatric Infectious Diseases
- Pediatric Nephrology
- Pediatric Pulmonology
- Pediatric Rheumatology
- Hospice and Palliative Medicine
- Medical Toxicology
- Sleep Medicine
- Sports Medicine
- Pediatric Transplant Hepatology
- Absences from Subspecialty Training
- Other Subspecialty Training Irregularities
- Accelerated Research Pathway (ARP)
- Subspecialty Fast-Tracking
- Dual Subspecialty Training
- Combined Training in Adult and Pediatric Subspecialties
- Scholarly Activity
- Training Pathways for J-1 Visas
- Fellowship Evaluations
- Physician Competencies
- Plan for Supervised Practice in a Pediatric Subspecialty
- Exam Development
- General Pediatrics Content Outline
- Content Outlines for Subspecialties
- Scoring FAQs
- Exam Security
- Score Reporting
- Exam Pass Rates
- Medicine-Pediatrics Program
- Pediatrics-Anesthesiology Program
- Pediatrics Emergency Medicine Program
- Pediatrics-Medical Genetics Program
- Pediatrics-Physical Medicine and Rehabilitation Program
- Pediatrics-Child and Adolescent Psychiatry Program
- Initial Certification FAQs
- Eligibility
- Enrollment and Fees
- American Board of Internal Medicine
- Other ABMS Specialty Boards
- RCPSC Reciprocal MOC Credit
- Pediatric Training Programs
- Non-Pediatric Training Programs
- CME Credit for MOC
- Multiple Certificates
- Expired Certificates
- Revocation of Certificates
- Core Competencies
- Medical Professionalism
- Self-Assessment Activities
- Question of the Week (QOW)
- CME Activities
- Internet Search and Learning (e.g., UpToDate)
- Life Support Certifications
- Diversity, Equity, and Inclusion Education
- Substance Use Disorder Training
- Exam Dates and Locations
- Apply and Schedule
- Test Accommodations
- Exam Day: What to Expect
- ABP Online Tutorials
- Content and Preparation
- Content Outline for General Pediatrics
- MOC Score Reporting
- Videos: Tips, Strategies, and Tutorials
- 2024 Dates and Deadlines
- 2024 Exam Content
- 2025 Exam Content (Preliminary)
- MOCA-Peds FAQs
- MOCA-Peds Scoring
- Participant Agreement
- MOCA-Peds Mobile App
- Local and National Projects
- Your Own QI Project
- Patient-Centered Medical Home (PCMH)
- Pediatric Community Health Initiative (PCHI)
- Leading Improvements in Child Health and Health Care
- Medical Education Program Improvements
- Reciprocal MOC Credit
- Online Modules (PIMs)
- Not clinically active?
- Activities for MOC Part 2
- Large Quality Improvement Project
- Becoming a Pediatric Portfolio Sponsor
- Quality Improvement Resources
- Publicity Resources
- Web-Based Part 4 Activities
- Completion Data Portal
- Info For Program Directors
- Info for Residents and Fellows
- Admission Requirements for General Pediatrics
- Exam Dates and Fees
- Scheduling and Rescheduling Exams
- Computer-Based Administration Policy
- Subspecialty Certification and Admission Requirements
- Subspecialty Content Outlines
- Exam Dates and Fees for Subspecialties
- How to Apply
- How to Apply for ITE
- ITE Exam Dates and Fees
- ITE Proctoring Materials
- How to Apply for SITE
- Dates and Fees for SITE
- SITE Proctoring Materials
- Maintenance of Certification
- For Parents and Caregivers
- ABP Portfolio
- Maintenance of Certification (MOC)
- Lifelong Learning (MOC Part 2)
- Quality Improvement (MOC Part 4)
- Contact the ABP
- ABP Volunteer Opportunities
- Guide to Clinical Competence
- How Does the ABP Follow My Progress?
- General Pediatrics Exam Dates and Fees
- Subspecialties Exam Dates and Fees
- Non-Standard Pathways for General Pediatrics
- Non-Standard Pathways for Subspecialists
- Become Certified
- MOC for Residents and Fellows
- Newsletters
- Presentations
- Entrustable Professional Activities (EPAs)
- EPAs for General Pediatrics
- EPAs for Subspecialties
- Milestones, Competencies, and EPAs
- EPA-Milestone Navigator
- Next Steps for Training Programs
- Table of Contents
- Chapter 1: Promoting Professionalism: An Overview for Medical Educators
- Chapter 2: Professionalism in Patient Care
- Chapter 3: Professionalism with Physician Colleagues and Other Health Professionals
- Chapter 4: Wellness and Its Impact on Professionalism
- Chapter 5: Society and Professionalism
- Chapter 6: Professionalism After Training
- Chapter 7: Electronic Professionalism
- Chapter 8: Humanism Within Pediatrics
- Chapter 9: When a Learner Is Not Meeting Expectations Relating to Professionalism
- Chapter 10: Identity Formation and Trustworthiness
- Home: Global Health Guide
- Acknowledgments
- Appendices and Templates
- Introduction
- Chapter 1: Global Health Education in Pediatric Training Programs: Core Considerations
- Chapter 2: Global Health Training at Home: Competencies and Implementation
- Chapter 3: Local and Global Health
- Chapter 4: Going Global: Training Program Preparation
- Chapter 5: Going Global: Trainee Preparation
- Chapter 6: Evaluation and Assessment: Who, What, Where, Why, and How
- Chapter 7: Accreditation and Certification Considerations
- Chapter 8: Fellowship Opportunities in Global Health
- Chapter 9: Post-Graduate Fellowship and Work Opportunities in Global Health
- Chapter 10: Partnership and Bidirectional Trainee Exchanges
- Abbreviations and References
- MOC for Your Residents
- Adolescent Medicine Survey Results
- Child Abuse Pediatrics Survey Results
- Developmental-Behavioral Pediatrics Survey Results
- Neonatal-Perinatal Medicine Survey Results
- Pediatric Cardiology Survey Results
- Pediatric Critical Care Medicine Survey Results
- Pediatric Emergency Medicine Survey Results
- Pediatric Endocrinology Survey Results
- Pediatric Gastroenterology Survey Results
- Pediatric Hematology-Oncology Survey Results
- Pediatric Infectious Diseases Survey Results
- Pediatric Nephrology Survey Results
- Pediatric Pulmonology Survey Results
- Pediatric Rheumatology Survey Results
- What is Board Certification?
- Our History
- Senior Leadership Team
- Board of Directors
- Current Committees
- Current Subboards
- Pediatrics and Medical Organizations
- Frequently Asked Questions (FAQs)
- Analysis of All ABP Certifications since 1934
- General Pediatrics Since 1934
- Pediatric Subspecialties Since 1961
- Pediatricians Certified at Other Boards
- Pediatricians with Multiple ABP Certifications
- Race and Ethnicity Data
- Future U.S. Pediatric Subspecialty Workforce
- General Pediatricians U.S. State and County Maps
- Pediatric Subspecialty U.S. State and County Maps
- Ratio of Pediatric Subspecialists to Children by Hospital Referral Region
- Driving Distance to Visit a Pediatric Subspecialists
- Yearly Growth in General Pediatrics Residents
- Yearly Growth in Pediatric Fellows
- ABP Data Compared to NRMP Data
- Pediatric Program Map and Listing
- Pediatric Practice Data: 2018 to 2022
- Workforce Data Initiative
- MOCA-Peds: A Review of Participation Since 2019
- Milestone and EPA Research
- Future of Testing Conference
- Name Changes
Pediatric Practice Data for 2019 to 2023
This multi-page dashboard includes survey data from more than 42,000 physicians certified in General Pediatrics and the pediatric subspecialties and reviews topics related to:
- Work settings;
- Hours worked;
- Percent time in clinical, research, administration, and teaching;
- Clinical practice areas;
- Educational debt; and
These data were collected via the survey offered after each pediatrician re-enrolls in Maintenance of Certification (MOC).
View 2018-2022 data .
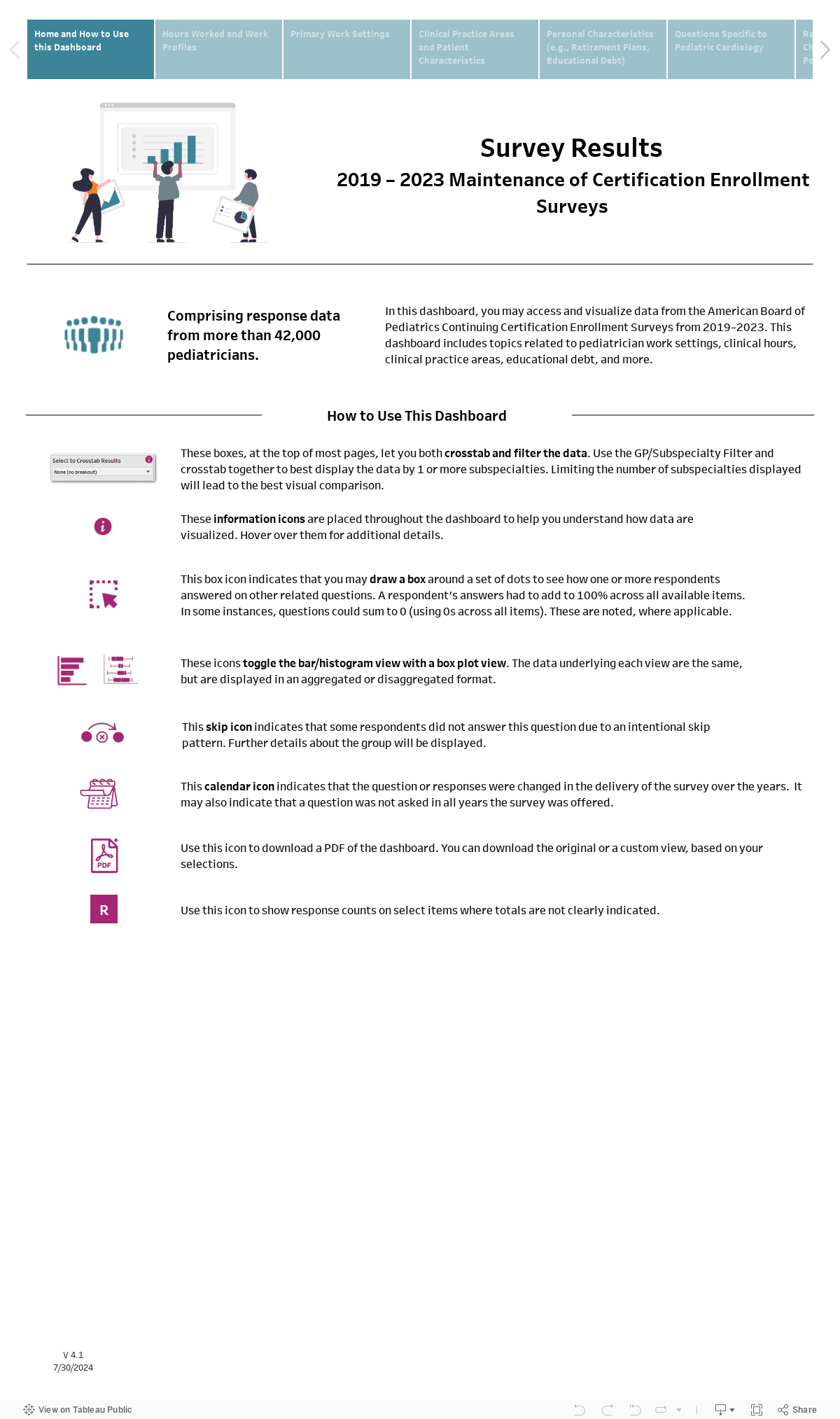

IMAGES
VIDEO
COMMENTS
About the Journal. The Journal of Educational Data Mining (JEDM; ISSN: 2157-2100; see indexing) is published by the International Educational Data Mining Society (IEDMS). It is an international and interdisciplinary forum of research on computational approaches for analyzing electronic repositories of student data to answer educational questions.
Educational Data Mining, Learning Analytics, Data Mining on Education, Data-Driven Decision ... Both communities share a common interest in data-intensive approaches to educational research, ... The first book about EDM/LA topics was published on 2006 and it was entitled Data Mining in E-Learning (Romero and Ventura, 2006). ...
Educational data mining (EDM) and learning analytics, which are highly related subjects but have different definitions and focuses, have enabled instructors to obtain a holistic view of student progress and trigger corresponding decision-making. ... Four major research topics, including prediction of performance, decision support for teachers ...
Educational data mining is becoming a more and more popular research field in recent years, mainly with the help of cross research conducted by various disciplines (such as computer science ...
It reviews in a comprehensible and very general way how Educational Data Mining and Learning Analytics have been applied over educational data. In the last decade, this research area has evolved enormously and a wide range of related terms are now used in the bibliography such as Academic Analytics, Institutional Analytics, Teaching Analytics ...
Paper — Educational Data Mining for Student Per formance Prediction: A Systematic Literature Revie w … Conference on Teaching, Assessment, and Learning for Engineerin g (TALE 2018) , 651-656.
To improve and facilitate the acquisition of learning outcomes, teachers often use innovative teaching methods such as gamification to keep students' attention and increase their motivation. In recent years, the use of educational data mining (EDM) methods to explore academic topics has increased. With the expansion of EDM, a gap in the literature and the need for a literature review to ...
Editors: Alejandro Peña-Ayala. Provides an updated view of the application of Data Mining to the educational arena. Copes two key targets: applications and trends. Focuses on the Data Mining logistics: models, tasks, methods, algorithms. Part of the book series: Studies in Computational Intelligence (SCI, volume 524) 62k Accesses. 200 Citations.
Educational data mining assists educational institutions in measuring the teaching and learning process and improving their student recruitment and retention policies. Hussain et al. (2022) proposed a decision support system based on a multi-layered Aspect2Labels (A2L) approach. It is a three-layered topic modelling approach, the first layer ...
Education is one of the most important aspects of a society's development. Educational methods that provide a slow or insufficient education have an influence on society and the nation's development [].In recent years, Educational Data Mining (EDM) has emerged as a distinct analysis field that examines and analyses data contained in student databases in order to better understand and ...
Based on fully mining educational big data, this study developed an early alert dynamic model of academically at-risk students to confirm and extend this proposition. ... Her research interests focus on teaching and learning practices from an interdisciplinary perspective and higher education development. She has published more than 20 research ...
Topics of interest to the conference include but are not limited to: Developing new techniques for mining educational data. Closing the loop between EDM research and learning sciences Informing data mining research with educational and/or motivational theories; Actionable advice rooted in educational data mining research, experiments, and outcomes
Many domain models have previously been improved by using optimal learning and instructional sequences. This article examines a variety of educational data mining strategies in great detail. Data mining in education also faces a number of concerns and obstacles, which are explored in this article. Export citation and abstract BibTeX RIS.
A broad range of data mining techniques can be utilized for big data in education, which Baker and Siemens (2014) broadly categorize into prediction methods, including inferential methods that model knowledge as it changes; structure discovery algorithms, with emphasis on discovering the structures of content and skills in an educational domain and the structures of social networks of learners ...
Educational data mining (EDM) is the analysis of huge sets of learner-related (Barneveld, Arnold, and Campbell Citation 2012; Siemens et al. Citation 2011) with the aid of methods like KDD, business intelligence, educational data mining, social network analysis, operational research, machine learning, and information visualization with the aim ...
Review of Education is an official BERA journal publishing educational research from throughout the world, and papers on topics of international interest. ... which combines quantitative and qualitative analysis. For quantitative analysis, advancements in data mining, ... hence revealing emerging themes within a given research topic (e.g ...
It reviews in a comprehensible and very general way how Educational Data Mining and Learning Analytics. ... (EDM/LA) research community Topics of interest Description Reference Analyzing educational theories To analyze how learning theories and learning analytics could be integrated in educational research. (Wong et al., 2019) Analyzing ...
The AERA Open special topic on education data science reveals the perspectives and concerns held by a subset of scholars genuinely interested in direct engagement with education research, and it offers an overview of the wider suite of data and methods beyond AERA Open that constitute EDS. While we have some degree of optimism about the ...
Educational data mining has become an effective tool for exploring the hidden relationships in educational data and predicting students' academic achievements. This study proposes a new model based on machine learning algorithms to predict the final exam grades of undergraduate students, taking their midterm exam grades as the source data. The performances of the random forests, nearest ...
Topic modeling is a valuable and appropriate tool to uncover meaningful topics from large quantities of textual data and yet largely unexplored by education related research (Chen et al., 2020a).There have been a few examples of educational technology related literature reviews successfully incorporating a topic modeling approach to analyze abstracts and keywords of articles to uncover ...
Topics of interest to the conference include but are not limited to: Developing new techniques for mining educational data. Closing the loop between EDM research and learning sciences. Informing data mining research with educational and/or motivational theories; Actionable advice rooted in educational data mining research, experiments, and outcomes
1.1 Data Mining, a concept and a cha llenge. Educational data mining can be defined as " An emerging discipline concerned with. developing methods for exploring the unique types of data that c ...
Educational data mining (EDM) is a research field concerned with the application of data mining, machine learning and statistics to information generated from educational settings (e.g., universities and intelligent tutoring systems).At a high level, the field seeks to develop and improve methods for exploring this data, which often has multiple levels of meaningful hierarchy, in order to ...
Published Date: 7/1/2020 Page.311-321 Vol 8 No 07 2020. Abstract. Educational Data Mining techniques have been widely used in MOOC environments to perform different educational. analyzes. In this ...
Currently, data centers account for about 1%-1.3% of global electricity consumption, versus crypto mining's roughly 0.4%, according to the International Energy Agency. That disparity is expected ...
Copenhagen, 29 August 2024New report reveals high rates of unprotected sex among adolescents across Europe, with significant implications for health and safety An urgent report from the WHO Regional Office for Europe reveals that condom use among sexually active adolescents has declined significantly since 2014, with rates of unprotected sex worryingly high. This is putting young people at ...
The potential influence of data mining analytics in higher education is a novel emerging field of research. Data from various educational organizations is explored and made operational, for ...
This multi-page dashboard includes survey data from more than 42,000 physicians certified in General Pediatrics and the pediatric subspecialties and reviews topics related to:Work settings;Hours worked;Percent time in clinical, research, administration, and teaching;Clinical practice areas;Educational debt; andMore.These data were collected via the survey offered after each pediatrician re ...
In educational data mining, the topics you may choose as. 1) Effect of online education in a Pandemic situation. 2) Student enrollment in an educational organization. 3) Finding the interest of ...