Qualitative Secondary Analysis: A Case Exemplar
- PMID: 29254902
- PMCID: PMC5911239
- DOI: 10.1016/j.pedhc.2017.09.007
Qualitative secondary analysis (QSA) is the use of qualitative data that was collected by someone else or was collected to answer a different research question. Secondary analysis of qualitative data provides an opportunity to maximize data utility, particularly with difficult-to-reach patient populations. However, qualitative secondary analysis methods require careful consideration and explicit description to best understand, contextualize, and evaluate the research results. In this article, we describe methodologic considerations using a case exemplar to illustrate challenges specific to qualitative secondary analysis and strategies to overcome them.
Keywords: Critical illness; ICU; qualitative research; secondary analysis.
Copyright © 2017 National Association of Pediatric Nurse Practitioners. Published by Elsevier Inc. All rights reserved.

Publication types
- Research Support, N.I.H., Extramural
- Research Support, Non-U.S. Gov't
- Data Interpretation, Statistical*
- Information Dissemination
- Informed Consent
- Qualitative Research*
- Ventilator Weaning
Grants and funding
- R01 NR007973/NR/NINR NIH HHS/United States
- R03 AG063276/AG/NIA NIH HHS/United States
- Find My Rep
You are here
Qualitative Secondary Research A Step-By-Step Guide
- Claire Largan
- Theresa Morris - University College Birmingham, UK
- Description
Perfect for those doing dissertations and research projects, it provides an accessible introduction to the theory of secondary research and sets out the advantages and limitations of using this kind of research. Drawing on years of teaching and research experience, the authors
· Offer step-by-step advice on how to use qualitative secondary data · Walk you through each stage of the research process · Provide practical, ethical tools to help you with your project · Show you how to avoid the potential pitfalls of using secondary data.
Clear and easy to understand, this book is a ready-made toolkit for successfully using qualitative secondary data. From beginner level and beyond, this no-nonsense guide takes the confusion and worry out of doing a secondary research project.
I recommend this book to students and more experienced researchers who want to conduct qualitative secondary research. It is a timely and accessible guide.
Overall, the authors have written a well thought out, accessible and comprehensive book, which is a welcome addition to a relatively small literature on secondary data and documentary analysis.
I will definitely be using this in my own research, to ensure that I have not forgotten important elements in my research design and analysis, and will be recommending chapter 5 (ethics in qualitative secondary research) as additional reading in the ethics chapter of my forthcoming book aimed at undergraduate student
Concise and coherent text on QSR. Have been searching for a text that is accessible for students that will allow them to reflect on their progress as researchers while also equipping them with a voice to justify their choices. This meets those parameters.
This is an excellent introductory text for a methodology that has become accepted practice and increasingly expected by research funding bodies. Making full use of collected data is an ethical principle and will prepare students well for future practice.
Very good resource for students and graduates alike. Definitely a must-read and should-work-with book :-)
The contents of the book allows students to carry out research with ease, the book has an easy flow and many useful areas for undergraduates to follow and complete any research work
This book should be an essential companion for anyone undertaking a research project. This underrepresented topic area is broken down into comprehensive chapters that provide a practical approach whilst prompting critical reflection also. Highly recommended.
a well-crafted and accessibly-written textbook which willl be very useful to students at several levels
This is an essential and accessible book for all undergraduate and postgraduate students wishing to carry out secondary research. This book offers a step-by-step guide into the processes of qualitative research, whilst allowing readers to develop their own critical thinking skills.
Miss Novlett Mitchell University College Birmingham
This book provides insights about qualititavie research and it is very useful for every dissertation module. I am so glad I had the opportunity to include it in my module
Preview this book
For instructors.
Please select a format:
Select a Purchasing Option
- Electronic Order Options VitalSource Amazon Kindle Google Play eBooks.com Kobo
Related Products

SAGE Research Methods is a research methods tool created to help researchers, faculty and students with their research projects. SAGE Research Methods links over 175,000 pages of SAGE’s renowned book, journal and reference content with truly advanced search and discovery tools. Researchers can explore methods concepts to help them design research projects, understand particular methods or identify a new method, conduct their research, and write up their findings. Since SAGE Research Methods focuses on methodology rather than disciplines, it can be used across the social sciences, health sciences, and more.
With SAGE Research Methods, researchers can explore their chosen method across the depth and breadth of content, expanding or refining their search as needed; read online, print, or email full-text content; utilize suggested related methods and links to related authors from SAGE Research Methods' robust library and unique features; and even share their own collections of content through Methods Lists. SAGE Research Methods contains content from over 720 books, dictionaries, encyclopedias, and handbooks, the entire “Little Green Book,” and "Little Blue Book” series, two Major Works collating a selection of journal articles, and specially commissioned videos.
Root out friction in every digital experience, super-charge conversion rates, and optimize digital self-service
Uncover insights from any interaction, deliver AI-powered agent coaching, and reduce cost to serve
Increase revenue and loyalty with real-time insights and recommendations delivered to teams on the ground
Know how your people feel and empower managers to improve employee engagement, productivity, and retention
Take action in the moments that matter most along the employee journey and drive bottom line growth
Whatever they’re are saying, wherever they’re saying it, know exactly what’s going on with your people
Get faster, richer insights with qual and quant tools that make powerful market research available to everyone
Run concept tests, pricing studies, prototyping + more with fast, powerful studies designed by UX research experts
Track your brand performance 24/7 and act quickly to respond to opportunities and challenges in your market
Explore the platform powering Experience Management
- Free Account
- For Digital
- For Customer Care
- For Human Resources
- For Researchers
- Financial Services
- All Industries
Popular Use Cases
- Customer Experience
- Employee Experience
- Net Promoter Score
- Voice of Customer
- Customer Success Hub
- Product Documentation
- Training & Certification
- XM Institute
- Popular Resources
- Customer Stories
- Artificial Intelligence
Market Research
- Partnerships
- Marketplace
The annual gathering of the experience leaders at the world’s iconic brands building breakthrough business results, live in Salt Lake City.
- English/AU & NZ
- Español/Europa
- Español/América Latina
- Português Brasileiro
- REQUEST DEMO
- Experience Management
- Secondary Research
Try Qualtrics for free
Secondary research: definition, methods, & examples.
19 min read This ultimate guide to secondary research helps you understand changes in market trends, customers buying patterns and your competition using existing data sources.
In situations where you’re not involved in the data gathering process ( primary research ), you have to rely on existing information and data to arrive at specific research conclusions or outcomes. This approach is known as secondary research.
In this article, we’re going to explain what secondary research is, how it works, and share some examples of it in practice.
Free eBook: The ultimate guide to conducting market research
What is secondary research?
Secondary research, also known as desk research, is a research method that involves compiling existing data sourced from a variety of channels . This includes internal sources (e.g.in-house research) or, more commonly, external sources (such as government statistics, organizational bodies, and the internet).
Secondary research comes in several formats, such as published datasets, reports, and survey responses , and can also be sourced from websites, libraries, and museums.
The information is usually free — or available at a limited access cost — and gathered using surveys , telephone interviews, observation, face-to-face interviews, and more.
When using secondary research, researchers collect, verify, analyze and incorporate it to help them confirm research goals for the research period.
As well as the above, it can be used to review previous research into an area of interest. Researchers can look for patterns across data spanning several years and identify trends — or use it to verify early hypothesis statements and establish whether it’s worth continuing research into a prospective area.
How to conduct secondary research
There are five key steps to conducting secondary research effectively and efficiently:
1. Identify and define the research topic
First, understand what you will be researching and define the topic by thinking about the research questions you want to be answered.
Ask yourself: What is the point of conducting this research? Then, ask: What do we want to achieve?
This may indicate an exploratory reason (why something happened) or confirm a hypothesis. The answers may indicate ideas that need primary or secondary research (or a combination) to investigate them.
2. Find research and existing data sources
If secondary research is needed, think about where you might find the information. This helps you narrow down your secondary sources to those that help you answer your questions. What keywords do you need to use?
Which organizations are closely working on this topic already? Are there any competitors that you need to be aware of?
Create a list of the data sources, information, and people that could help you with your work.
3. Begin searching and collecting the existing data
Now that you have the list of data sources, start accessing the data and collect the information into an organized system. This may mean you start setting up research journal accounts or making telephone calls to book meetings with third-party research teams to verify the details around data results.
As you search and access information, remember to check the data’s date, the credibility of the source, the relevance of the material to your research topic, and the methodology used by the third-party researchers. Start small and as you gain results, investigate further in the areas that help your research’s aims.
4. Combine the data and compare the results
When you have your data in one place, you need to understand, filter, order, and combine it intelligently. Data may come in different formats where some data could be unusable, while other information may need to be deleted.
After this, you can start to look at different data sets to see what they tell you. You may find that you need to compare the same datasets over different periods for changes over time or compare different datasets to notice overlaps or trends. Ask yourself: What does this data mean to my research? Does it help or hinder my research?
5. Analyze your data and explore further
In this last stage of the process, look at the information you have and ask yourself if this answers your original questions for your research. Are there any gaps? Do you understand the information you’ve found? If you feel there is more to cover, repeat the steps and delve deeper into the topic so that you can get all the information you need.
If secondary research can’t provide these answers, consider supplementing your results with data gained from primary research. As you explore further, add to your knowledge and update your findings. This will help you present clear, credible information.
Primary vs secondary research
Unlike secondary research, primary research involves creating data first-hand by directly working with interviewees, target users, or a target market. Primary research focuses on the method for carrying out research, asking questions, and collecting data using approaches such as:
- Interviews (panel, face-to-face or over the phone)
- Questionnaires or surveys
- Focus groups
Using these methods, researchers can get in-depth, targeted responses to questions, making results more accurate and specific to their research goals. However, it does take time to do and administer.
Unlike primary research, secondary research uses existing data, which also includes published results from primary research. Researchers summarize the existing research and use the results to support their research goals.
Both primary and secondary research have their places. Primary research can support the findings found through secondary research (and fill knowledge gaps), while secondary research can be a starting point for further primary research. Because of this, these research methods are often combined for optimal research results that are accurate at both the micro and macro level.
Sources of Secondary Research
There are two types of secondary research sources: internal and external. Internal data refers to in-house data that can be gathered from the researcher’s organization. External data refers to data published outside of and not owned by the researcher’s organization.
Internal data
Internal data is a good first port of call for insights and knowledge, as you may already have relevant information stored in your systems. Because you own this information — and it won’t be available to other researchers — it can give you a competitive edge . Examples of internal data include:
- Database information on sales history and business goal conversions
- Information from website applications and mobile site data
- Customer-generated data on product and service efficiency and use
- Previous research results or supplemental research areas
- Previous campaign results
External data
External data is useful when you: 1) need information on a new topic, 2) want to fill in gaps in your knowledge, or 3) want data that breaks down a population or market for trend and pattern analysis. Examples of external data include:
- Government, non-government agencies, and trade body statistics
- Company reports and research
- Competitor research
- Public library collections
- Textbooks and research journals
- Media stories in newspapers
- Online journals and research sites
Three examples of secondary research methods in action
How and why might you conduct secondary research? Let’s look at a few examples:
1. Collecting factual information from the internet on a specific topic or market
There are plenty of sites that hold data for people to view and use in their research. For example, Google Scholar, ResearchGate, or Wiley Online Library all provide previous research on a particular topic. Researchers can create free accounts and use the search facilities to look into a topic by keyword, before following the instructions to download or export results for further analysis.
This can be useful for exploring a new market that your organization wants to consider entering. For instance, by viewing the U.S Census Bureau demographic data for that area, you can see what the demographics of your target audience are , and create compelling marketing campaigns accordingly.
2. Finding out the views of your target audience on a particular topic
If you’re interested in seeing the historical views on a particular topic, for example, attitudes to women’s rights in the US, you can turn to secondary sources.
Textbooks, news articles, reviews, and journal entries can all provide qualitative reports and interviews covering how people discussed women’s rights. There may be multimedia elements like video or documented posters of propaganda showing biased language usage.
By gathering this information, synthesizing it, and evaluating the language, who created it and when it was shared, you can create a timeline of how a topic was discussed over time.
3. When you want to know the latest thinking on a topic
Educational institutions, such as schools and colleges, create a lot of research-based reports on younger audiences or their academic specialisms. Dissertations from students also can be submitted to research journals, making these places useful places to see the latest insights from a new generation of academics.
Information can be requested — and sometimes academic institutions may want to collaborate and conduct research on your behalf. This can provide key primary data in areas that you want to research, as well as secondary data sources for your research.
Advantages of secondary research
There are several benefits of using secondary research, which we’ve outlined below:
- Easily and readily available data – There is an abundance of readily accessible data sources that have been pre-collected for use, in person at local libraries and online using the internet. This data is usually sorted by filters or can be exported into spreadsheet format, meaning that little technical expertise is needed to access and use the data.
- Faster research speeds – Since the data is already published and in the public arena, you don’t need to collect this information through primary research. This can make the research easier to do and faster, as you can get started with the data quickly.
- Low financial and time costs – Most secondary data sources can be accessed for free or at a small cost to the researcher, so the overall research costs are kept low. In addition, by saving on preliminary research, the time costs for the researcher are kept down as well.
- Secondary data can drive additional research actions – The insights gained can support future research activities (like conducting a follow-up survey or specifying future detailed research topics) or help add value to these activities.
- Secondary data can be useful pre-research insights – Secondary source data can provide pre-research insights and information on effects that can help resolve whether research should be conducted. It can also help highlight knowledge gaps, so subsequent research can consider this.
- Ability to scale up results – Secondary sources can include large datasets (like Census data results across several states) so research results can be scaled up quickly using large secondary data sources.
Disadvantages of secondary research
The disadvantages of secondary research are worth considering in advance of conducting research :
- Secondary research data can be out of date – Secondary sources can be updated regularly, but if you’re exploring the data between two updates, the data can be out of date. Researchers will need to consider whether the data available provides the right research coverage dates, so that insights are accurate and timely, or if the data needs to be updated. Also, fast-moving markets may find secondary data expires very quickly.
- Secondary research needs to be verified and interpreted – Where there’s a lot of data from one source, a researcher needs to review and analyze it. The data may need to be verified against other data sets or your hypotheses for accuracy and to ensure you’re using the right data for your research.
- The researcher has had no control over the secondary research – As the researcher has not been involved in the secondary research, invalid data can affect the results. It’s therefore vital that the methodology and controls are closely reviewed so that the data is collected in a systematic and error-free way.
- Secondary research data is not exclusive – As data sets are commonly available, there is no exclusivity and many researchers can use the same data. This can be problematic where researchers want to have exclusive rights over the research results and risk duplication of research in the future.
When do we conduct secondary research?
Now that you know the basics of secondary research, when do researchers normally conduct secondary research?
It’s often used at the beginning of research, when the researcher is trying to understand the current landscape . In addition, if the research area is new to the researcher, it can form crucial background context to help them understand what information exists already. This can plug knowledge gaps, supplement the researcher’s own learning or add to the research.
Secondary research can also be used in conjunction with primary research. Secondary research can become the formative research that helps pinpoint where further primary research is needed to find out specific information. It can also support or verify the findings from primary research.
You can use secondary research where high levels of control aren’t needed by the researcher, but a lot of knowledge on a topic is required from different angles.
Secondary research should not be used in place of primary research as both are very different and are used for various circumstances.
Questions to ask before conducting secondary research
Before you start your secondary research, ask yourself these questions:
- Is there similar internal data that we have created for a similar area in the past?
If your organization has past research, it’s best to review this work before starting a new project. The older work may provide you with the answers, and give you a starting dataset and context of how your organization approached the research before. However, be mindful that the work is probably out of date and view it with that note in mind. Read through and look for where this helps your research goals or where more work is needed.
- What am I trying to achieve with this research?
When you have clear goals, and understand what you need to achieve, you can look for the perfect type of secondary or primary research to support the aims. Different secondary research data will provide you with different information – for example, looking at news stories to tell you a breakdown of your market’s buying patterns won’t be as useful as internal or external data e-commerce and sales data sources.
- How credible will my research be?
If you are looking for credibility, you want to consider how accurate the research results will need to be, and if you can sacrifice credibility for speed by using secondary sources to get you started. Bear in mind which sources you choose — low-credibility data sites, like political party websites that are highly biased to favor their own party, would skew your results.
- What is the date of the secondary research?
When you’re looking to conduct research, you want the results to be as useful as possible , so using data that is 10 years old won’t be as accurate as using data that was created a year ago. Since a lot can change in a few years, note the date of your research and look for earlier data sets that can tell you a more recent picture of results. One caveat to this is using data collected over a long-term period for comparisons with earlier periods, which can tell you about the rate and direction of change.
- Can the data sources be verified? Does the information you have check out?
If you can’t verify the data by looking at the research methodology, speaking to the original team or cross-checking the facts with other research, it could be hard to be sure that the data is accurate. Think about whether you can use another source, or if it’s worth doing some supplementary primary research to replicate and verify results to help with this issue.
We created a front-to-back guide on conducting market research, The ultimate guide to conducting market research , so you can understand the research journey with confidence.
In it, you’ll learn more about:
- What effective market research looks like
- The use cases for market research
- The most important steps to conducting market research
- And how to take action on your research findings
Download the free guide for a clearer view on secondary research and other key research types for your business.
Related resources
Market intelligence 10 min read, marketing insights 11 min read, ethnographic research 11 min read, qualitative vs quantitative research 13 min read, qualitative research questions 11 min read, qualitative research design 12 min read, primary vs secondary research 14 min read, request demo.
Ready to learn more about Qualtrics?
- Privacy Policy

Home » Secondary Data – Types, Methods and Examples
Secondary Data – Types, Methods and Examples
Table of Contents

Secondary Data
Definition:
Secondary data refers to information that has been collected, processed, and published by someone else, rather than the researcher gathering the data firsthand. This can include data from sources such as government publications, academic journals, market research reports, and other existing datasets.
Secondary Data Types
Types of secondary data are as follows:
- Published data: Published data refers to data that has been published in books, magazines, newspapers, and other print media. Examples include statistical reports, market research reports, and scholarly articles.
- Government data: Government data refers to data collected by government agencies and departments. This can include data on demographics, economic trends, crime rates, and health statistics.
- Commercial data: Commercial data is data collected by businesses for their own purposes. This can include sales data, customer feedback, and market research data.
- Academic data: Academic data refers to data collected by researchers for academic purposes. This can include data from experiments, surveys, and observational studies.
- Online data: Online data refers to data that is available on the internet. This can include social media posts, website analytics, and online customer reviews.
- Organizational data: Organizational data is data collected by businesses or organizations for their own purposes. This can include data on employee performance, financial records, and customer satisfaction.
- Historical data : Historical data refers to data that was collected in the past and is still available for research purposes. This can include census data, historical documents, and archival records.
- International data: International data refers to data collected from other countries for research purposes. This can include data on international trade, health statistics, and demographic trends.
- Public data : Public data refers to data that is available to the general public. This can include data from government agencies, non-profit organizations, and other sources.
- Private data: Private data refers to data that is not available to the general public. This can include confidential business data, personal medical records, and financial data.
- Big data: Big data refers to large, complex datasets that are difficult to manage and analyze using traditional data processing methods. This can include social media data, sensor data, and other types of data generated by digital devices.
Secondary Data Collection Methods
Secondary Data Collection Methods are as follows:
- Published sources: Researchers can gather secondary data from published sources such as books, journals, reports, and newspapers. These sources often provide comprehensive information on a variety of topics.
- Online sources: With the growth of the internet, researchers can now access a vast amount of secondary data online. This includes websites, databases, and online archives.
- Government sources : Government agencies often collect and publish a wide range of secondary data on topics such as demographics, crime rates, and health statistics. Researchers can obtain this data through government websites, publications, or data portals.
- Commercial sources: Businesses often collect and analyze data for marketing research or customer profiling. Researchers can obtain this data through commercial data providers or by purchasing market research reports.
- Academic sources: Researchers can also obtain secondary data from academic sources such as published research studies, academic journals, and dissertations.
- Personal contacts: Researchers can also obtain secondary data from personal contacts, such as experts in a particular field or individuals with specialized knowledge.
Secondary Data Formats
Secondary data can come in various formats depending on the source from which it is obtained. Here are some common formats of secondary data:
- Numeric Data: Numeric data is often in the form of statistics and numerical figures that have been compiled and reported by organizations such as government agencies, research institutions, and commercial enterprises. This can include data such as population figures, GDP, sales figures, and market share.
- Textual Data: Textual data is often in the form of written documents, such as reports, articles, and books. This can include qualitative data such as descriptions, opinions, and narratives.
- Audiovisual Data : Audiovisual data is often in the form of recordings, videos, and photographs. This can include data such as interviews, focus group discussions, and other types of qualitative data.
- Geospatial Data: Geospatial data is often in the form of maps, satellite images, and geographic information systems (GIS) data. This can include data such as demographic information, land use patterns, and transportation networks.
- Transactional Data : Transactional data is often in the form of digital records of financial and business transactions. This can include data such as purchase histories, customer behavior, and financial transactions.
- Social Media Data: Social media data is often in the form of user-generated content from social media platforms such as Facebook, Twitter, and Instagram. This can include data such as user demographics, content trends, and sentiment analysis.
Secondary Data Analysis Methods
Secondary data analysis involves the use of pre-existing data for research purposes. Here are some common methods of secondary data analysis:
- Descriptive Analysis: This method involves describing the characteristics of a dataset, such as the mean, standard deviation, and range of the data. Descriptive analysis can be used to summarize data and provide an overview of trends.
- Inferential Analysis: This method involves making inferences and drawing conclusions about a population based on a sample of data. Inferential analysis can be used to test hypotheses and determine the statistical significance of relationships between variables.
- Content Analysis: This method involves analyzing textual or visual data to identify patterns and themes. Content analysis can be used to study the content of documents, media coverage, and social media posts.
- Time-Series Analysis : This method involves analyzing data over time to identify trends and patterns. Time-series analysis can be used to study economic trends, climate change, and other phenomena that change over time.
- Spatial Analysis : This method involves analyzing data in relation to geographic location. Spatial analysis can be used to study patterns of disease spread, land use patterns, and the effects of environmental factors on health outcomes.
- Meta-Analysis: This method involves combining data from multiple studies to draw conclusions about a particular phenomenon. Meta-analysis can be used to synthesize the results of previous research and provide a more comprehensive understanding of a particular topic.
Secondary Data Gathering Guide
Here are some steps to follow when gathering secondary data:
- Define your research question: Start by defining your research question and identifying the specific information you need to answer it. This will help you identify the type of secondary data you need and where to find it.
- Identify relevant sources: Identify potential sources of secondary data, including published sources, online databases, government sources, and commercial data providers. Consider the reliability and validity of each source.
- Evaluate the quality of the data: Evaluate the quality and reliability of the data you plan to use. Consider the data collection methods, sample size, and potential biases. Make sure the data is relevant to your research question and is suitable for the type of analysis you plan to conduct.
- Collect the data: Collect the relevant data from the identified sources. Use a consistent method to record and organize the data to make analysis easier.
- Validate the data: Validate the data to ensure that it is accurate and reliable. Check for inconsistencies, missing data, and errors. Address any issues before analyzing the data.
- Analyze the data: Analyze the data using appropriate statistical and analytical methods. Use descriptive and inferential statistics to summarize and draw conclusions from the data.
- Interpret the results: Interpret the results of your analysis and draw conclusions based on the data. Make sure your conclusions are supported by the data and are relevant to your research question.
- Communicate the findings : Communicate your findings clearly and concisely. Use appropriate visual aids such as graphs and charts to help explain your results.
Examples of Secondary Data
Here are some examples of secondary data from different fields:
- Healthcare : Hospital records, medical journals, clinical trial data, and disease registries are examples of secondary data sources in healthcare. These sources can provide researchers with information on patient demographics, disease prevalence, and treatment outcomes.
- Marketing : Market research reports, customer surveys, and sales data are examples of secondary data sources in marketing. These sources can provide marketers with information on consumer preferences, market trends, and competitor activity.
- Education : Student test scores, graduation rates, and enrollment statistics are examples of secondary data sources in education. These sources can provide researchers with information on student achievement, teacher effectiveness, and educational disparities.
- Finance : Stock market data, financial statements, and credit reports are examples of secondary data sources in finance. These sources can provide investors with information on market trends, company performance, and creditworthiness.
- Social Science : Government statistics, census data, and survey data are examples of secondary data sources in social science. These sources can provide researchers with information on population demographics, social trends, and political attitudes.
- Environmental Science : Climate data, remote sensing data, and ecological monitoring data are examples of secondary data sources in environmental science. These sources can provide researchers with information on weather patterns, land use, and biodiversity.
Purpose of Secondary Data
The purpose of secondary data is to provide researchers with information that has already been collected by others for other purposes. Secondary data can be used to support research questions, test hypotheses, and answer research objectives. Some of the key purposes of secondary data are:
- To gain a better understanding of the research topic : Secondary data can be used to provide context and background information on a research topic. This can help researchers understand the historical and social context of their research and gain insights into relevant variables and relationships.
- To save time and resources: Collecting new primary data can be time-consuming and expensive. Using existing secondary data sources can save researchers time and resources by providing access to pre-existing data that has already been collected and organized.
- To provide comparative data : Secondary data can be used to compare and contrast findings across different studies or datasets. This can help researchers identify trends, patterns, and relationships that may not have been apparent from individual studies.
- To support triangulation: Triangulation is the process of using multiple sources of data to confirm or refute research findings. Secondary data can be used to support triangulation by providing additional sources of data to support or refute primary research findings.
- To supplement primary data : Secondary data can be used to supplement primary data by providing additional information or insights that were not captured by the primary research. This can help researchers gain a more complete understanding of the research topic and draw more robust conclusions.
When to use Secondary Data
Secondary data can be useful in a variety of research contexts, and there are several situations in which it may be appropriate to use secondary data. Some common situations in which secondary data may be used include:
- When primary data collection is not feasible : Collecting primary data can be time-consuming and expensive, and in some cases, it may not be feasible to collect primary data. In these situations, secondary data can provide valuable insights and information.
- When exploring a new research area : Secondary data can be a useful starting point for researchers who are exploring a new research area. Secondary data can provide context and background information on a research topic, and can help researchers identify key variables and relationships to explore further.
- When comparing and contrasting research findings: Secondary data can be used to compare and contrast findings across different studies or datasets. This can help researchers identify trends, patterns, and relationships that may not have been apparent from individual studies.
- When triangulating research findings: Triangulation is the process of using multiple sources of data to confirm or refute research findings. Secondary data can be used to support triangulation by providing additional sources of data to support or refute primary research findings.
- When validating research findings : Secondary data can be used to validate primary research findings by providing additional sources of data that support or refute the primary findings.
Characteristics of Secondary Data
Secondary data have several characteristics that distinguish them from primary data. Here are some of the key characteristics of secondary data:
- Non-reactive: Secondary data are non-reactive, meaning that they are not collected for the specific purpose of the research study. This means that the researcher has no control over the data collection process, and cannot influence how the data were collected.
- Time-saving: Secondary data are pre-existing, meaning that they have already been collected and organized by someone else. This can save the researcher time and resources, as they do not need to collect the data themselves.
- Wide-ranging : Secondary data sources can provide a wide range of information on a variety of topics. This can be useful for researchers who are exploring a new research area or seeking to compare and contrast research findings.
- Less expensive: Secondary data are generally less expensive than primary data, as they do not require the researcher to incur the costs associated with data collection.
- Potential for bias : Secondary data may be subject to biases that were present in the original data collection process. For example, data may have been collected using a biased sampling method or the data may be incomplete or inaccurate.
- Lack of control: The researcher has no control over the data collection process and cannot ensure that the data were collected using appropriate methods or measures.
- Requires careful evaluation : Secondary data sources must be evaluated carefully to ensure that they are appropriate for the research question and analysis. This includes assessing the quality, reliability, and validity of the data sources.
Advantages of Secondary Data
There are several advantages to using secondary data in research, including:
- Time-saving : Collecting primary data can be time-consuming and expensive. Secondary data can be accessed quickly and easily, which can save researchers time and resources.
- Cost-effective: Secondary data are generally less expensive than primary data, as they do not require the researcher to incur the costs associated with data collection.
- Large sample size : Secondary data sources often have larger sample sizes than primary data sources, which can increase the statistical power of the research.
- Access to historical data : Secondary data sources can provide access to historical data, which can be useful for researchers who are studying trends over time.
- No ethical concerns: Secondary data are already in existence, so there are no ethical concerns related to collecting data from human subjects.
- May be more objective : Secondary data may be more objective than primary data, as the data were not collected for the specific purpose of the research study.
Limitations of Secondary Data
While there are many advantages to using secondary data in research, there are also some limitations that should be considered. Some of the main limitations of secondary data include:
- Lack of control over data quality : Researchers do not have control over the data collection process, which means they cannot ensure the accuracy or completeness of the data.
- Limited availability: Secondary data may not be available for the specific research question or study design.
- Lack of information on sampling and data collection methods: Researchers may not have access to information on the sampling and data collection methods used to gather the secondary data. This can make it difficult to evaluate the quality of the data.
- Data may not be up-to-date: Secondary data may not be up-to-date or relevant to the current research question.
- Data may be incomplete or inaccurate : Secondary data may be incomplete or inaccurate due to missing or incorrect data points, data entry errors, or other factors.
- Biases in data collection: The data may have been collected using biased sampling or data collection methods, which can limit the validity of the data.
- Lack of control over variables: Researchers have limited control over the variables that were measured in the original data collection process, which can limit the ability to draw conclusions about causality.
About the author
Muhammad Hassan
Researcher, Academic Writer, Web developer
You may also like

Primary Data – Types, Methods and Examples

Qualitative Data – Types, Methods and Examples

Research Data – Types Methods and Examples

Quantitative Data – Types, Methods and Examples

Information in Research – Types and Examples
- Open access
- Published: 23 May 2024
Older people’s perception of being frail – a qualitative exploration
- Abigail J. Hall 1 ,
- Silviya Nikolova 2 ,
- Matthew Prescott 3 &
- Victoria A. Goodwin 1
BMC Geriatrics volume 24 , Article number: 453 ( 2024 ) Cite this article
Metrics details
Frailty is a suggested consequence of ageing, but with a variety of different definitions the understanding of what it means to be frail is challenging. There is a common belief that frailty results in a reduction of physical functioning and ability and therefore is likely to significantly affect a person’s quality of life. The aim of this study was to explore the understanding of older people about the meaning of frailty and the potential consequences of being classified as frail.
This paper forms a secondary analysis of a process evaluation of a complex intervention that was embedded within the individually randomised Home-based Extended Rehabilitation of Older people (HERO) trial. A maximum variation, purposive sampling strategy sought to recruit participants with a wide range of characteristics. Data collection included observations of the delivery of the intervention, documentary analysis and semi-structured interviews with participants. Thematic analysis was used to make sense of the observational and interview data, adopting both inductive and deductive approaches.
Ninety three HERO trial participants were sampled for the process evaluation with a total of 60 observational home visits and 35 interviews were undertaken. There was a wide range in perceptions about what it meant to be classified as frail with no clear understanding from our participants. However, there was a negative attitude towards frailty with it being considered something that needed to be avoided where possible. Frailty was seen as part of a negative decline that people struggled to associate with. There was discussion about frailty being temporary and that it could be reduced or avoided with sufficient physical exercise and activity.
Our study provides insight into how older people perceive and understand the concept of frailty. Frailty is a concept that is difficult for patients to understand, with most associating the term with an extreme degree of physical and cognitive decline. Having a label of being “frail” was deemed to be negative and something to be avoided, suggesting the term needs to be used cautiously.
Trial registration
ISRCTN 13927531. Registered on April 19, 2017.
Peer Review reports
Frailty is a clinical condition suggested to be an inevitable consequence of ageing [ 1 ] and despite no universally accepted definition, there is consensus that it involves susceptibility to external stressors such as physical, psychological and social factors [ 2 ], alongside a loss of biological reserve [ 3 ]. As such, even minor stressors can result in significant changes in health status [ 4 ]. A global ageing population, with an expected 2 billion of the world’s population predicted to be over 60 years of age by 2050 [ 5 ], will only exacerbate the challenges of conditions, such as frailty, with an increased prevalence in older populations. Therefore, understanding the challenges and consequences of frailty could be considered vital to improve healthy ageing and health and social care outcomes at an individual and societal level.
The multifaceted concept of frailty emphasises impacts on a person’s physical and psychological health and therefore the potential influence this has on social functioning – which are all factors reported to affect a person’s quality of life (QoL). Indeed, those living with frailty themselves have highlighted the importance in maintaining QoL rather than a focus on biomedical measures of outcomes relating to disease [ 6 ]. Therefore, the importance of understanding a person’s perception of their level of frailty and their resultant QoL is vital to understand how to target interventions to manage frailty alongside the medical management.
Existing literature focuses on older adults’ perceptions of frailty rather than the perceptions of those who are classified as being frail [ 7 , 8 , 9 , 10 , 11 ] as well as the difference between actually “being” frail and “feeling” frail [ 12 ]. There is a plethora of qualitative literature exploring perceptions of ageing in general, but with little focus specifically on frailty [ 13 ]. Similarly, there is literature exploring the perceptions of frailty from healthcare professionals [ 14 , 15 ].
This paper forms a secondary analysis of a process evaluation (PE) of a complex intervention that was embedded within the Home-based Extended Rehabilitation of Older people (HERO) randomised controlled trial involving 742 participants living with frailty following a hospital admission for an acute illness or injury [ 16 ]. The process evaluation explored the community delivery of a complex intervention and involved a variety of different interacting components. This individualised, graded, and progressive 24-week exercise programme was delivered by National Health Service (NHS) physiotherapy teams to people aged 65 and older living with frailty. Frailty as an inclusion criteria for the HERO trial was identified using the Clinical Frailty Scale (CFS) [ 17 ] following discharge from hospital (+/- rehabilitation) after an acute illness or injury. The intervention incorporated behaviour change techniques based on social cognitive theory, including providing information on benefits of exercise; setting graded tasks; goal setting, problem solving, reorganising the physical environment to facilitate exercise, encouragement, feedback, prompting self-monitoring and rewards.
Evidence from the HERO trial suggests that there is incongruity between older people “being frail” and “feeling frail” [ 9 , 12 ], and that feeling frail can have a large detrimental effect on the person’s wellbeing [ 18 ]. Thus, the person’s perception of their level of frailty has the potential to directly impact on their physical and psychological wellbeing. The aim of this paper was to explore the perceptions of frailty for those who were assessed to be frail. It will also consider how these perceptions of frailty affect an individual’s everyday functioning and QoL.
The qualitative PE for the HERO trial employed a qualitative mixed methods approach [ 19 ] including a variety of data collection methods such as non-participant observations, semi-structured interviews, and documentary analysis. The data for this paper was obtained from the interviews with participants as the focus is on individual’s own perception of their frailty. Carers were often present at these interviews but contributed little to the data and the non-participant observations which allowed the researcher to observe the delivery of the intervention.
Social Cognitive Theory (SCT) [ 18 ] was used to explore the intervention and perceptions of frailty from the perspective of the participant and guided the development of the topic guides and subsequent analysis. SCT is extensively used in health and social care research and explains how individuals within social systems enact multiple human processes, including the acquisition and adoption of information and knowledge. Its focus was the interplay between personal factors, their behaviour, and their environments. The COREQ checklist [ 20 ] was used to report the study.
Research ethics committee approval was obtained from the Health Research Authority Yorkshire & The Humber – Bradford Leeds Research Ethics Committee – reference 17/YH/0097. All participants gave written informed consent to take part in the study.
Recruitment
Inclusion criteria.
HERO trial inclusion criteria are detailed in the trial protocol [ 16 ], but broadly included individuals who were:
Age ≥ 65 years of age
Community dwelling
Acutely admitted to hospital with acute illness or injury then discharged home from hospital or associated rehabilitation
Classified as mild, moderate, or severe frailty, defined as a score of 5–7 on the 9-item (CFS)
Able to complete the Timed Up and Go test independently (i.e. stand from a chair and three meters before turning to return and sit in the chair)
HERO trial participants were asked to optionally consent to the trial PE. Those people consenting to PE activity were then purposively sampled to ensure all types of data collection included participants with a wide variety of experiences, demographics, and contexts to represent a wider perspective of the population under study. The researchers had no prior relationship with the participants. Participants were aware of the purpose of the research and the aims of the interviews. Participants were contacted via letter to request their involvement in the interviews. A maximum variation, purposive sampling strategy was used for the following characteristics:
levels of frailty (Clinical Frailty Scale (CFS) levels 5–7)
level of intervention (Home-based Older People’s Exercise (HOPE) programme levels 1–3)
intervention delivery site (NHS trust)
Recruitment to the process evaluation continued until it was felt no new themes were emerging and thus, we had sufficient information power [ 21 ] to answer our overall research questions and objectives.
HERO trial participants consenting to PE activity and randomised to receive the trial intervention were sampled and approached to participate in intervention delivery. These observations were scheduled to observe a variety of different participants at various stages of the intervention (from the first face to face home visit to the final face to face visit). While trial participants sampled for the PE had already consented to their involvement, permission to observe a therapy session was sought ahead of every observation from both the therapist and the participant.
Participant and carer interviews included trial participants in both the intervention and usual care arms of the trial. These interviews were scheduled approximately six months after an individual entered the HERO trial, and were timed to correspond with the intervention finishing for those randomised to receive it. Due to the lapse of time between consenting to participate in the HERO trial, and the scheduling of the process evaluation interviews, additional process evaluation participant information was provided and participants were asked to confirm consent prior to interview. Sampled participants were approached via a letter of invitation which was then followed up by a telephone call to discuss their potential involvement in a face-to-face interview. The interviews were all conducted in the participant’s own home and lasted for an average of 60 min. Two of the sampled participants refused an interview at this point – both reporting that they were unwell. Several of the participants we interviewed had carers or relatives present for the interview – the majority did not contribute to the interview and simply listened to the conversation.
Data collection
Topic guides were developed by the research team and used when undertaking interviews and used SCT to structure the lines of enquiry. All questions on the topic guide were used in every interview to ensure breadth of discussion. The two interviewers (AH and FZ) were both post-doctoral academics with extensive experience of qualitative research, including multiple publications and have both undertaken extensive training in qualitative research. The topic guides were developed to ensure that interviewees’ experiences were explored in relation to the underpinning theory relating to behaviour change. The topic guides were developed to explore many components of the intervention, but there were specific questions which related to frailty and the participants’ perception of what this meant to them. The topic guide and questions were initially piloted with several participants and after making small typographic changes, were then used for all other participants. Interviews were audio recorded, encrypted and later transcribed. Field notes were taken where appropriate. At the end of the interview, the participant was asked if they were happy for all their data to be included in the analysis. All data were pseudo-anonymised, and unique identification numbers associated with participants removed. All data were stored electronically on password protected secure servers. In order to ensure that each non-participation observation was explored consistently, a checklist and template was developed. This included observations of the delivery of the intervention, the environment as well as the interaction and relationship between the therapist(s) and the participant and carers.
Types of data collected
Figure 1 depicts the data that were collected for the whole of the process evaluation, with the red boxes demonstrating the sources of data for this study. Data were collected as per the description in the trial protocol [ 16 ].
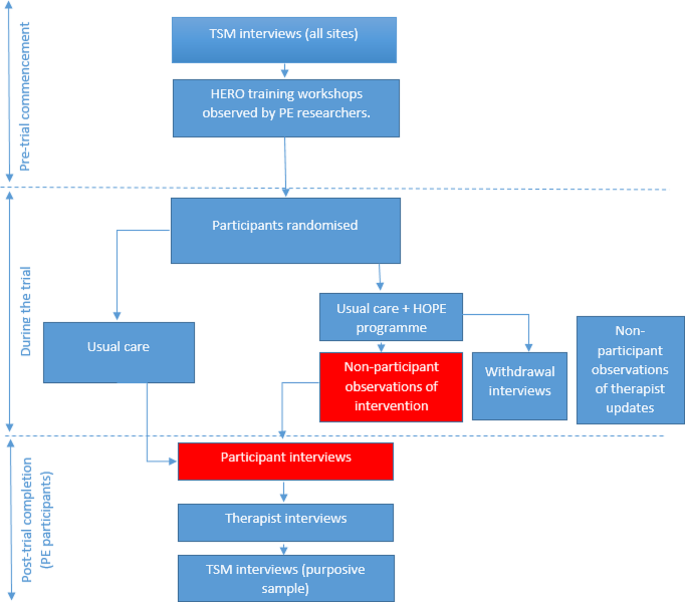
HERO trial Process evaluation activities (PE = process evaluation, TSM = therapy service manager, HOPE programme = The Home-based Older People’s Exercise programme)
An approach based on thematic analysis [ 22 ] was used to interpret the observational and interview data, adopting both inductive and deductive approaches. The first stage of data analysis involved the two researchers (AH and FZ) collecting the raw data from interview transcripts, field notes and patient observations. NVivo 11 (QSR International) was used to organise and store the data with all transcripts, observational frameworks and field notes uploaded to the software. Following completion of data collection, a first stage of coding was undertaken independently by each researcher. This resulted in preliminary codes for each set of participant data being generated and was reviewed jointly by the researchers and discussed with a third reviewer (DJC). This was part of a process of consensus building around the generation of themes. The themes that were being developed were continually reviewed in the context of the intervention logic model and used to develop a framework. Data were triangulated from all data sources to gain a clear understanding about any conflicting themes – for example, the observational data was compared to the data from the interviews and therapy record to determine if there was any conflicting data in what was observed and what was reported.
In total, 93 HERO trial participants were sampled for the process evaluation, and their characteristics are detailed in Table 1 . 38 intervention participants had their intervention delivery observed with a total of 60 observational home visits (the majority of participants had two observations) and 35 interviews were undertaken.
One of the main criteria for inclusion in the HERO trial was an individual being classified as frail, however the main trial did not specifically inform people that they had been classified as ‘frail’ or discuss the CFS classification in such terms. As part of the PE data collection, we sought to determine general attitudes towards frailty as a term and concept, and determine whether those interviewed considered themselves as being frail. While some participants realised that they could be characterised as being frail and saw it as an inevitable consequence of ageing, others disputed it, feeling that it was a negative characteristic they didn’t wish to be associated with. There was also a feeling that frailty was a state that could fluctuate, it was a fluid state that could improve or worsen. Some participants felt they could control their level of frailty, others felt overwhelmed by it and daunted by the prospect of becoming frailer.
Perception of frailty
Despite all our older main trial participants being classified as frail, there were very different attitudes towards frailty and perceptions of what being frail meant to them and to others. When asked to define what they understood “frail” to mean, the vast majority related frailty to a person’s physical abilities, thus if they felt they were physically able they would not perceive themselves to be frail.
Others related frailty to an image of what a frail individual might look like, often describing the image to be a thin, “fragile”, person who might look malnourished and be described to be “cognitively poor”. None of our participants described themselves as looking frail, indeed frailty wasn’t a concept that most found easy to identify with.
While participants often related frailty to physical difficulties, there was a belief expressed by a few of our participants that cognitive deficits (or lack of) contributed to their perception of frailty. Often, where an interviewee had some physically difficulties, if they perceived themselves to be cognitively sound, they didn’t feel they were frail – thus for some there is an element of needing both physical and cognitive deficits in determining whether somebody feels frail.
Your abilities. Things that you can’t do that you used to do. I’m not frail in my mind….But when you know the things that, up to possibly a year ago, you could do and suddenly you’re unable to do them, and I had a dramatic weight loss. I mean, I’ve lost three stone. – (156, mild frailty)
Pre-existing disabilities appeared to play a role in considering whether somebody was frail or not. Functional limitations relating to long standing disability were seen as separate when considering frailty, and thus did not predispose a person to being considered frail.
While some participants reported that their carers or family supported them to do functional tasks to aid building confidence, others were cautious about doing so and were fearful that enabling the person in this way may lead to over-confidence, which could pose a risk to their safety.
There was a common belief that frailty related to a limitation in being able to do the tasks or activities that they wanted to be able to do. These tasks varied from simple activities of daily living that had become harder to more vigorous activities such as running. This inability to undertake a task provided very clear evidence that a person was physically deteriorating and often people related to a reduction in QoL and thus to an onset of frailty.
You know when you want to run and you can’t run, and I think, I’m getting very frail now. (283 moderate frailty) Just not being able to do things. Not being able to get on the bus and go to the supermarket, and being tired halfway around, and falling asleep all the time, I fall asleep through the day, I’ve… I’ll be watching something or doing something and my eyes just go, and I’m asleep for about an hour, which is a long time, and then when I go to bed I’m wide awake. – (18 mild frailty)
Challenges undertaking functional tasks led people to seeking or accepting help. While some people felt that this inability to undertake functional activities classified them as being frail, others simply found the challenges associated with it frustrating, but didn’t feel this classified them as frail.
Yes, well I mean I have needed help, I’ve got things that help in your kitchen and things like that, you know, to do different jobs that you can’t do anymore, you know, there’s certain things I can’t do, like even the things like the bleach bottles, you know where they have those locks on, I’m up a gum tree with them, I can’t press and do, my hands just aren’t doing it, so but I don’t think, I’ve never thought of that as frail, I just thought of that as a blooming nuisance, I have to get [son] to come, or somebody to come and do it you see (368 moderate frailty)
Identifying as ’frail’ was problematic for many of our participants, however, there was evidence to suggest that their relatives and carers often told them that they were frail – frequently relating this to things that they were unable to do.
P : He’s always saying “I can’t do this, I can’t do that”. (283 moderate frailty)
Despite our participants all being classified as being “frail”, none of them overtly recognised themselves as being frail.
Denial / avoidance
Our data indicated multiple negative connotations towards the term “frailty”. It was something that people perceived to be a negative characteristic and was something that they wanted to avoid, despite an acceptance that with increased age, came increased risk of frailty. The majority of participants we sampled in the process evaluation were classified as mild to moderately frail (CFS level 5 and 6), however, even the participants with severe frailty wished to avoid considering themselves as being frail.
I know I must be getting frailer but I try not to think about it to be quite honest. I think, well if I can still do it I can’t be that frail can I. And that’s what I say to myself you see, I can’t be so bad if I can still do it so I’m going to try. – (154, severe frailty)
There was an element of comparing themselves to other people who were less functionally able, and this appeared to give them comfort that they weren’t as frail as somebody else, or frail at all. If they knew people who were less physically able, this gave them confidence that they were doing well.
I don’t think that frailty is, as such is an issue, and you know, see elderly people who are much less able than I am. – (321 mild frailty) .
Participants related confidence to feelings of frailty. This confidence was important to enable them to undertake daily activities or things they enjoyed. Being unable to do these things was found to be associated with a reduction in QoL. Where they had lost confidence to undertake a task they previously could do, or participate in certain activities, they related this to a feeling of being frail. This loss of confidence was reported to have been as a result of injury or illness, or in some cases just as a result of general decline.
I suppose I did, but then it comes back to confidence, if you’re not confident in doing something, whether that is a sign of frailty I don’t know, - (321 mild frailty)
The participant often pointed out their cognitive abilities as a means to indicate they were not frail. The participant, in some cases, didn’t realise how many physical tasks they struggled to achieve until they were discussed and led them to reconsider whether they were indeed frail.
Many participants felt shame associated with classification of frailty and tried to mentally justify to themselves the reasons why they were not frail. It was perceived to be something to try and avoid as much as possible as it had significant negative connotations for a person’s QoL. The concept of QoL was raised by several participants and it was felt that frailty had a direct relationship with QoL – thus as a person got frailer, their QoL reduced.
Well they wouldn’t be able to do anything at all would they, that’s how I feel, if you’re so frail. I mean, life’s not worth living if you get that frail is it. – (154 severe frailty)
Reversibility
While participants frequently struggled to accept being classified as frail, they could often relate to feelings of frailty at different stages of their life and how these were temporary. Several participants reflected on a specific period of ill-health or an injury and a perception of frailty, but as a transient problem. During this time they accepted that their functional ability and QoL would temporarily reduce, but this would only be short lived. Thus, the transient nature of frailty meant that they felt they had a level of control over it.
I have been [frail] this last few months, because I’ve been in and out of the hospital, but normally I’m not a frail person, I’m not… (18 mild frailty)
Other participants, despite being classified as frail, did not feel that such events should result in a classification of frailty and the temporal nature of deterioration didn’t relate to being frail and to be classified as frail required a long period of time.
There was a general belief that there were positive actions that could be taken to reduce frailty which most commonly was reported to be the use of exercise. Within the intervention participants, perceived improvements in physical ability relating to undertaking the HOPE programme led some people to believe that their level of frailty was being reduced.
Um, well before I started doing the programme I felt really frail and then once I actually got into the programme itself I could feel meself getting stronger and stronger each time I were doing it and, but personally I really enjoyed doing it. – (235 mild frailty)
Where people engaged with exercise, they noted improvements in functional ability which allowed them to do more activities that they enjoyed and thus resulted in an improvement in their QoL. Examples given included being able to engage more with their grandchildren or going out to cafes with friends and families. While exercise was seen to reduce frailty, there was a common theme that participants wanted to delay the onset or progression of frailty as much as possible. The negative connotations that people described related to ‘frail older people’ invoked fear about a reduction in their QoL and functional abilities and was something they wanted to avoid for themselves, thus there was a difficulty accepting a classification of frailty.
Well I think when you can’t do what you used to do, I do think now, sometimes, I must admit sometimes I do feel a bit frail because I can’t do what I used to do … but it don’t go, but I do try. And I make meself go, I don’t sit and feel sorry for meself, I’ve never been that type, so I do try and make meself go as much as I can. – (33 mild frailty)
The aim of this paper was to explore the perception of frailty from those who are classified as being frail. It also considered how individuals’ perception of frailty, may affect their everyday functioning and QoL. Our participants were all recruited to a large randomised controlled trial, with inclusion requiring classification as frail (score of 5–7 on the 9-item (CFS) [ 17 ]. Thus all our trial participants had at least a degree of functional dependence due to physical or cognitive deficits, yet their perceptions of what it meant to be frail varied and the effect this had on their attitudes to their QoL and physical abilities was also inconsistent. Most participants sampled in the process evaluation were either mild or moderately frail, however, those that were severely frail appeared to have greatest disconnect from considering themselves frail than those with lesser levels of frailty. While being frail reduces the ability to undertake functional tasks, participants reported getting help to undertake activities which allowed them to maintain their QoL.
One of the main findings of our study was a noted disconnect that people felt between being classified as frail and identifying as frail. The majority did not realise that they were classified as frail and reasoned why they should not be. Unanimously, our participants described frailty as being a negative state from both a physical and a psychological aspect, which is consistent with existing literature [ 7 , 8 , 9 , 12 , 23 ]. However, most participants did not have a clear idea what it meant to be frail, but still felt it was something they wanted to avoid.
In their study, Warmoth and colleagues [ 9 ] reported that participants felt that their frailty was “beyond their control”, however, many of our participants felt that frailty was actually transient in its nature and there were measures that could be undertaken to reduce the likelihood of becoming frail or to reverse it. These mainly revolved around undertaking exercise, or from ensuring that they continue to do activities – regardless of how difficult they found them. It is conceivable that our trial participants allocated to receive the HOPE programme had positive experiences of exercise impacting upon their feelings of control relating to frailty levels. Indeed, out trial population were rather self-selecting in that they had volunteered to participate in an RCT involving an exercise programme as extended rehabilitation. One might assume therefore that the participants valued rehabilitation and exercise as a component there of. Literature supports the notion of reversible frailty. A systematic review [ 24 ] of 46 studies with an included 15,690 participants suggests that frailty is reversible with a combination of muscle strength training and protein supplementation. A further systematic review and network meta- analysis [ 25 ] including 66 RCTs concluded that physical activity interventions, when compared to placebo and standard care, were associated with reductions in frailty.
Despite our participants reporting that physical frailty was potentially reversible, there was a belief that cognitive frailty was not. Cognitive frailty was believed to be a strong indicator as to classifying somebody as frail. Indeed, despite recognising their own physical difficulties, some of our participants relied on their cognitive abilities as a reason to not self-identify as frail. Wang and colleagues [ 26 ] explored the interdependency between cognitive frailty and physical frailty and suggested that early identification of cognitive frailty could facilitate specific interventions which could increase (or delay decline of) independence in older adults. The importance of maintaining independence was key to our participants. A person being classified as frail on the CFS, and them identifying as frail were often not consistent. The maintenance of physical abilities – and thus independence - appeared to reduce their feelings of being frail, participants had a tendency to focus on the preservation of abilities in reasoning why they we not frail, rather than recognising lost abilities. In instances where carers highlighted areas of dependence, some participants began to recognise a state of frailty.
Our participants were classified as being frail according to the CFS [ 17 ]. Although an interplay between physical frailty and dementia/cognitive decline is well recognised [ 27 ], the CFS focuses on function, an individual’s limitation of functions and dependence on others without differentiation between specific physical and cognitive deficits impacting the functional status. It is beyond the scope of this paper to compare measured cognitive and physical abilities of the HERO trial participants. Accepting that the status of individuals may have changed through the first 6 months involved in the trial pre-interview, all trial participants were sufficiently cognisant to provide informed consent to trial participation, and all scored ≥ 20 on the Montreal Cognitive Assessment as an eligibility criterion. Exercise has been suggested to be beneficial to improve both physical and cognitive frailty [ 28 ]. Furthermore, frailty is defined as a vulnerability to external stressors [ 3 ], however, there was no indication from our data that people perceived it to be about vulnerability, suggesting further disconnect between health care professionals’ classification of frailty and older people’s beliefs around a state of frailty.
Implications
This study highlights several important factors for frailty research and engaging frail older people in healthcare services. Firstly, our data has highlighted how people find it hard to relate to the terminology around frailty, with many perceiving frailty to be an extreme near end of life state. However, in the UK, many services are termed “frailty” services, thus if people do not relate themselves to this term, it may result in a failure to engage with services that could be of benefit to them. Furthermore, our participants believed that frailty could be reversed or delayed with targeted interventions such as exercise. This has important implications for describing the benefits of exercise to this population.
Frailty is a term used by healthcare professionals to describe a state of physical and mental vulnerability, however, there is a disconnect with how older people and health care professionals understand the term. Frailty as a concept used in healthcare, is difficult for older people to understand and identify with, with most frail older adults associating the term with an extreme degree of physical and cognitive decline. Having a label of being “frail” was something that was deemed to be negative and something to be avoided, suggesting the use of the term needs to be used cautiously. Some frail older people could recognise transient periods where they would identify themselves as frail, but felt able to control their level of frailty to some extent (particularly via exercise). A strong desire to avoid frailty was driven by negative attitudes towards their perceptions of frailty and the association with lower QoL.
Data availability
The datasets used and/or analysed during the current study are available from the corresponding author on reasonable request.
Abbreviations
Clinical frailty scale
Consolidated criteria for reporting qualitative research
Home-based Extended Rehabilitation for Older people
Home-based Older People’s Exercise
General Practitioner
National Health Service
Normalisation Process Theory
Process evaluation
Quality of life
Randomised controlled trial
Social cognitive theory
Therapy service manager
Society BG. Frailty- What’s it all about 2018 [Available from: www.bgs.org.uk/resources/frailty-what%E2%80%99s-it-all-about .
Mulla E, Montgomery U. Frailty: an overview. InnovAiT. 2020;13(2):71–9.
Article Google Scholar
Clegg A, Young J, Iliffe S, Rikkert MO, Rockwood K. Frailty in elderly people. Lancet. 2013;381(9868):752–62.
Article PubMed Google Scholar
Hale M, Shah S, Clegg A. Frailty, inequality and resilience. Clin Med. 2019;19(3):219.
World Health Organisation. Ageing and health 2018 [ https://www.who.int/news-room/fact-sheets/detail/ageing-and-health#:~:text=People%20worldwide%20are%20living%20longer.%20Today%2C%20for%20the,million%20people%20are%20aged%2080%20years%20or%20older .
Costanza R, Fisher B, Ali S, Beer C, Bond L, Boumans R, et al. Quality of life: an approach integrating opportunities, human needs, and subjective well-being. Ecol Econ. 2007;61(2–3):267–76.
Grenier AM. The contextual and social locations of older women’s experiences of disability and decline. J Aging Stud. 2005;19(2):131–46.
Grenier A. Constructions of frailty in the English language, care practice and the lived experience. Ageing Soc. 2007;27(3):425–45.
Warmoth K, Lang IA, Phoenix C, Abraham C, Andrew MK, Hubbard RE, et al. Thinking you’re old and frail’: a qualitative study of frailty in older adults. Ageing Soc. 2016;36(7):1483–500.
Nicholson C, Gordon AL, Tinker A. Changing the way we. view talk about frailty… Age Ageing. 2017;46(3):349–51.
Schoenborn NL, Rasmussen SEVP, Xue Q-L, Walston JD, McAdams-Demarco MA, Segev DL, et al. Older adults’ perceptions and informational needs regarding frailty. BMC Geriatr. 2018;18(1):1–7.
Grenier A. The distinction between being and feeling frail: exploring emotional experiences in health and social care. J Social Work Pract. 2006;20(3):299–313.
Cotter VT, Gonzalez EW. Self-concept in older adults: an integrative review of empirical literature. Holist Nurs Pract. 2009;23(6):335–48.
Ambagtsheer RC, Archibald MM, Lawless M, Mills D, Yu S, Beilby JJ. General practitioners’ perceptions, attitudes and experiences of frailty and frailty screening. Australian J Gen Pract. 2019;48(7):426–33.
Archibald MM, Lawless M, Gill TK, Chehade MJ. Orthopaedic surgeons’ perceptions of frailty and frailty screening. BMC Geriatr. 2020;20(1):1–11.
Prescott M, Lilley-Kelly A, Cundill B, Clarke D, Drake S, Farrin AJ, et al. Home-based Extended Rehabilitation for older people (HERO): study protocol for an individually randomised controlled multi-centre trial to determine the clinical and cost-effectiveness of a home-based exercise intervention for older people with frailty as extended rehabilitation following acute illness or injury, including embedded process evaluation. Trials. 2021;22(1):1–17.
Rockwood K, Song X, MacKnight C, Bergman H, Hogan DB, McDowell I, et al. A global clinical measure of fitness and frailty in elderly people. CMAJ. 2005;173(5):489–95.
Article PubMed PubMed Central Google Scholar
Andrew MK, Fisk JD, Rockwood K. Psychological well-being in relation to frailty: a frailty identity crisis? Int Psychogeriatr. 2012;24(8):1347–53.
Plano Clark VL. Mixed methods research. J Posit Psychol. 2017;12(3):305–6.
Tong A, Sainsbury P, Craig J. Consolidated criteria for reporting qualitative research (COREQ): a 32-item checklist for interviews and focus groups. Int J Qual Health Care. 2007;19(6):349–57.
Malterud K, Siersma VD, Guassora AD. Sample size in qualitative interview studies: guided by information power. Qual Health Res. 2016;26(13):1753–60.
Clarke V, Braun V, Hayfield N. Thematic analysis. Qualitative Psychology: Practical Guide Res Methods. 2015;3:222–48.
Google Scholar
Puts MT, Shekary N, Widdershoven G, Heldens J, Deeg DJ. The meaning of frailty according to Dutch older frail and non-frail persons. J Aging Stud. 2009;23(4):258–66.
Travers J, Romero-Ortuno R, Bailey J, Cooney M-T. Delaying and reversing frailty: a systematic review of primary care interventions. Br J Gen Pract. 2019;69(678):e61–9.
Negm AM, Kennedy CC, Thabane L, Veroniki A-A, Adachi JD, Richardson J, et al. Management of frailty: a systematic review and network meta-analysis of randomized controlled trials. J Am Med Dir Assoc. 2019;20(10):1190–8.
Wang W, Si H, Yu R, Qiao X, Jin Y, Ji L et al. Effects of reversible cognitive frailty on disability, quality of life, depression, and hospitalization: a prospective cohort study. Aging Ment Health. 2021:1–8.
Robertson DA, Savva GM, Kenny RA. Frailty and cognitive impairment—a review of the evidence and causal mechanisms. Ageing Res Rev. 2013;12(4):840–51.
Dulac M, Aubertin-Leheudre M. Exercise: an important key to prevent physical and cognitive frailty. Frailty. 2016:107.
Download references
Acknowledgements
The authors would like to thank all participants who took part in this study.
The study is funded by the National Institute for Health Research - Health Technology Assessment programme (15/47/07). The study funder is not involved nor has any responsibility in the collection, management, analysis, and interpretation of data; writing of the report; and decision to submit the report for publication. The views expressed are those of the author(s) and not necessarily those of the NHS, the NIHR or the Department of Health and Social Care.
Author information
Authors and affiliations.
Public Health and Sports Science Department, University of Exeter, Heavitree Road, Exeter, EX1 2LU, UK
Abigail J. Hall & Victoria A. Goodwin
Real World Methods & Evidence Generation, IQVIA, Reading, UK
Silviya Nikolova
Bradford Teaching Hospitals NHS Foundation Trust, Bristol, UK
Matthew Prescott
You can also search for this author in PubMed Google Scholar
Contributions
AH wrote the main manuscript text. All authors contributed to the analysis. All authors contributed to the reviewing and drafting of the paper. All authors reviewed the manuscript.
Corresponding author
Correspondence to Abigail J. Hall .
Ethics declarations
Ethics approval and consent to participate.
Research ethics committee approval was obtained from HRA Yorkshire & The Humber – Bradford Leeds Research Ethics Committee – reference 17/YH/0097. All participants gave written informed consent to take part in the study.
Consent for publication
Not applicable.
Competing interests
The authors declare no competing interests.

Additional information
Publisher’s note.
Springer Nature remains neutral with regard to jurisdictional claims in published maps and institutional affiliations.
Rights and permissions
Open Access This article is licensed under a Creative Commons Attribution 4.0 International License, which permits use, sharing, adaptation, distribution and reproduction in any medium or format, as long as you give appropriate credit to the original author(s) and the source, provide a link to the Creative Commons licence, and indicate if changes were made. The images or other third party material in this article are included in the article’s Creative Commons licence, unless indicated otherwise in a credit line to the material. If material is not included in the article’s Creative Commons licence and your intended use is not permitted by statutory regulation or exceeds the permitted use, you will need to obtain permission directly from the copyright holder. To view a copy of this licence, visit http://creativecommons.org/licenses/by/4.0/ . The Creative Commons Public Domain Dedication waiver ( http://creativecommons.org/publicdomain/zero/1.0/ ) applies to the data made available in this article, unless otherwise stated in a credit line to the data.
Reprints and permissions
About this article
Cite this article.
Hall, A.J., Nikolova, S., Prescott, M. et al. Older people’s perception of being frail – a qualitative exploration. BMC Geriatr 24 , 453 (2024). https://doi.org/10.1186/s12877-024-05079-x
Download citation
Received : 20 July 2023
Accepted : 14 May 2024
Published : 23 May 2024
DOI : https://doi.org/10.1186/s12877-024-05079-x
Share this article
Anyone you share the following link with will be able to read this content:
Sorry, a shareable link is not currently available for this article.
Provided by the Springer Nature SharedIt content-sharing initiative
- Older people
- Qualitative
BMC Geriatrics
ISSN: 1471-2318
- Submission enquiries: [email protected]
- General enquiries: [email protected]
- Search Menu
- Sign in through your institution
- Advance articles
- Editor's Choice
- Focus Issue Archive
- Open Access Articles
- JAMIA Journal Club
- Author Guidelines
- Submission Site
- Open Access
- Call for Papers
- About Journal of the American Medical Informatics Association
- About the American Medical Informatics Association
- Journals Career Network
- Editorial Board
- Advertising and Corporate Services
- Self-Archiving Policy
- Dispatch Dates
- For Reviewers
- Journals on Oxford Academic
- Books on Oxford Academic
Article Contents
Background and significance, materials and methods, author contributions, supplementary material, conflict of interest, data availability, understanding enterprise data warehouses to support clinical and translational research: impact, sustainability, demand management, and accessibility.

- Article contents
- Figures & tables
- Supplementary Data
Thomas R Campion, Catherine K Craven, David A Dorr, Elmer V Bernstam, Boyd M Knosp, Understanding enterprise data warehouses to support clinical and translational research: impact, sustainability, demand management, and accessibility, Journal of the American Medical Informatics Association , 2024;, ocae111, https://doi.org/10.1093/jamia/ocae111
- Permissions Icon Permissions
Healthcare organizations, including Clinical and Translational Science Awards (CTSA) hubs funded by the National Institutes of Health, seek to enable secondary use of electronic health record (EHR) data through an enterprise data warehouse for research (EDW4R), but optimal approaches are unknown. In this qualitative study, our goal was to understand EDW4R impact, sustainability, demand management, and accessibility.
We engaged a convenience sample of informatics leaders from CTSA hubs ( n = 21) for semi-structured interviews and completed a directed content analysis of interview transcripts.
EDW4R have created institutional capacity for single- and multi-center studies, democratized access to EHR data for investigators from multiple disciplines, and enabled the learning health system. Bibliometrics have been challenging due to investigator non-compliance, but one hub’s requirement to link all study protocols with funding records enabled quantifying an EDW4R’s multi-million dollar impact. Sustainability of EDW4R has relied on multiple funding sources with a general shift away from the CTSA grant toward institutional and industry support. To address EDW4R demand, institutions have expanded staff, used different governance approaches, and provided investigator self-service tools. EDW4R accessibility can benefit from improved tools incorporating user-centered design, increased data literacy among scientists, expansion of informaticians in the workforce, and growth of team science.
As investigator demand for EDW4R has increased, approaches to tracking impact, ensuring sustainability, and improving accessibility of EDW4R resources have varied.
This study adds to understanding of how informatics leaders seek to support investigators using EDW4R across the CTSA consortium and potentially elsewhere.
Healthcare organizations increasingly seek to enable secondary use of electronic health record (EHR) data for analytics and research. Toward this goal, many institutions have deployed a combination of people, process, and technology referred to as an enterprise data warehouse for research (EDW4R). 1 Through funding from the National Institutes of Health (NIH) National Center for Advancing Translational Science (NCATS), a group of 60 HCOs, or Clinical and Translational Science Awards (CTSA) program “hubs,” have almost all implemented an EDW4R. 2 , 3 However, optimal approaches to build and support EDW4R remain unknown. In prior studies, we found variation in EDW4R operations in CTSA hubs with respect to organizational and technical architecture, processes for access, and service management 4 as well as enterprise information technology (IT) relationships, data governance, workforce, and cloud computing. 5 The goal of this study was to address gaps in understanding EDW4R impact, sustainability, demand management, and accessibility.
With widespread EDW4R adoption among CTSA hubs, multiple stakeholders have pursued ever-increasing use of EDW4R resources to accelerate science. As investigators have demonstrated value of using EHR data for phenotyping patients, 6 determining cancer response, 7 and automating clinical documentation, 8 they have also characterized limitations of EHR data and developed strategies for improving data quality through imputation. 9–11 Moreover, secondary use of EHR data is challenging 12 due to cross-system record linkage, 13 standardization gaps, 14 analytical assumptions, 15 and collider bias, 16 among other factors. To support a greater number and variety of studies, including investigations using artificial intelligence and multimodal data, 17 institutions have integrated EHR data with imaging, 18 , 19 biospecimen, 20 insurance claim, 21 and genomic 22 sources. Additionally, scholars have noted EDW4R as essential for data-driven learning health systems. 23–25 To participate in multi-institutional consortia, EDW4R teams have adopted 1 or more common data models (CDMs) to represent clinical information. For example, using the PCORnet ® CDM, PCORnet ® has determined disease prevalence when combining EHR and claims data for 17 to 170 million patients. 26–28 Additionally, the NIH All of Us Research Program, which has enrolled more than 500 000 participants to provide data from EHR as well as genomic and lifestyle sources, 29 has enabled large-scale phenome-wide association studies. 30 Efforts in the Observational Health Data Sciences and Informatics (OHDSI) consortium as well as the NIH National COVID Cohort Collaborative (N3C) have continued to advance standardized vocabularies 31 and data quality, 32 respectively, while the NIH Researching COVID to Enhance Recovery (RECOVER) initiative has demonstrated benefits of an open science approach for machine learning with EHR data. 33 In industry, at least 1 drug company has described efforts to address challenges in generating real world evidence from EHR data, 34 and the TriNetX network connecting healthcare organizations’ EHR data with pharmaceutical sponsors has continued to grow in size of participants and scope of activities. 35 Despite widespread EDW4R adoption among CTSA hubs and expanded use of EDW4R data by multiple stakeholders, optimal approaches for EDW4R operation remain unknown, which hinders resource allocation within individual hubs and across the CTSA consortium.
In prior work, we identified areas that needed further study including EDW4R impact, sustainability, demand management, and accessibility. Impact describes how investigators apply EDW4R to scientific processes, how institutions measure EDW4R, 36 and how investigator use of EDW4R affects institutional activities. 37 Impact includes types of research benefitting from EDW4R as well as metrics of interest to IT and research leadership.
Sustainability refers to how institutions support EDW4R activities over time. Long a topic of discussion among CTSA hubs, 38 , 39 funding for research informatics, 40 and EDW4R especially, 3 continues to evolve through combinations of financial sources, collaborations with enterprise IT organizations, and other mechanisms. 5
Demand management addresses how EDW4R teams respond to requests for patient data and usage of resources. Delivery of data occurs through multiple modalities, including analyst-mediated custom reports and investigator self-service of tools like i2b2. 4 In addition to technical solutions, demand management addresses allocation of resources through governance, prioritization, and education. 41 , 42
Accessibility encompasses ideal EDW4R user experience and opportunities to make tools easier for informaticians and non-informaticians to use EDW4R resources. In addition to technology elements such as modern query tools 43 and user interface design, 44 accessibility includes social elements such as service delivery 45 and data literacy. 46
To foster development of best practices for secondary use of data from EHR and other sources for research, the CTSA consortium’s Informatics Enterprise Committee (iEC) formed the Enterprise Data Warehouse for Research Working Group (EDW4R WG) with leadership from 2 co-authors (B.M.K. and T.R.C.). The EDW4R WG collaborated with the NCATS National Center for Data to Health (CD2H), and 1 co-author (D.A.D.) functioned as a liaison between the groups. The University of Iowa Institutional Review Board (IRB) determined this study to be non-human subject research.
Data collection
We engaged a convenience sample of informatics leaders ( n = 23) from CTSA hubs ( n = 21) for semi-structured interviews conducted via Zoom between January and November 2022. Informed by previous work, EDW4R WG discussion, and recommendations from the CTSA Steering Committee, a guide developed by 2 co-authors (B.M.K. and T.R.C.) facilitated interviews ( Appendix S1 ). Notably, the CTSA Steering Committee defined interview questions about EDW4R accessibility, specifically ideal user experience and opportunities for new tools. During sessions with participants, 2 interviewers (B.M.K. and T.R.C.) recorded notes electronically or on paper with subsequent transcription into electronic form.
Data analysis
The study team completed a directed content analysis 47 of interview transcripts. After 2 co-authors (B.M.K. and T.R.C.) labeled concepts and relationships, peer debriefing 48 occurred with 3 co-authors (C.K.C., D.A.D., and E.V.B.), who were not involved in conducting the interviews but were experts in EDW4R, reviewing initial findings. Feedback from peer debriefing refined analysis of concepts and relationships further; 2 co-authors (B.M.K. and T.R.C.) then presented the refined analysis to EDW4R WG monthly meeting attendees, which included some interview participants, as part of member checking. Feedback from member checking further refined and validated findings.
We transcribed over 50 pages of text from interviews with 23 participants from 21 CTSA hubs conducted across almost 30 hours. Two interviews included 2 respondents from the same hub to clarify and expand comments as necessary; responses from the same hub accorded rather than conflicted with each other. Table 1 describes characteristics of participants.
Characteristics of participants.
In characterizing EDW4R impact, 7 hubs described using EHR data to support 2 primary scientific workflows: identification of potential participants for prospective studies and data extraction for retrospective observational studies; 2 other hubs stated investigators used EDW4R only to support participant identification for prospective studies. Additionally, 5 hubs indicated EDW4R enabled institutional participation in national data consortia, such as PCORnet, OHDSI, and the National COVID Cohort Collaborative (N3C) among others. Four hubs indicated EDW4R “democratized” or enabled easier EHR data access for researchers from multiple medical school departments as well as across other colleges, including business, engineering, and arts and sciences. Hubs also indicated that EDW4R supported major institutional programs, including COVID response ( n = 3) and National Cancer Institute Cancer Center designation ( n = 2). Two hubs highlighted learning health system projects with data-driven patient care workflows as evidence of EDW4R impact.
For measuring EDW4R impact, participants noted challenges with tracking publications that used EDW4R ( n = 7), especially due to investigator non-compliance citing CTSA grant support ( n = 4). At least 3 hubs described indirect impact where investigators “just disappear” following, for example, initial consultation with EDW4R staff for clinical trial eligibility determination. In contrast to EDW4R, 1 participant noted that measurement of high-performance computing usage and impact was straightforward, as study teams worked directly with informatics staff on projects. One hub described an institutional requirement to associate each IRB-approved study protocol with 1 or more grant records, which, because the intake process required specification of a study protocol, enabled quantifying the EDW4R’s multi-million dollar impact on the biomedical research enterprise.
Regarding measures of EDW4R impact, participants described research leadership as focused on traditional metrics of academic productivity such as grants and papers ( n = 7) and salary support of faculty and staff ( n = 2). Although at least 3 hubs conducted annual surveys of faculty to understand EDW4R impact, 1 hub indicated the success rate of investigators pursuing grant funding using EDW4R resources was unknown and likely low. Of interest to IT leadership were few complaints from faculty ( n = 2) as well as “burger-flipping metrics” such as number of data deliveries, users, and queries ( n = 5). Notably, 1 hub described IT leadership finding value in EDW4R removing research requests from the workload of clinical IT, thus freeing capacity to support clinical operations and quality reporting. At least 3 hubs described EDW4R team growth as a measure of impact, and only 1 hub used a validated framework 49 to measure impact.
Four hubs indicated institutional leaders and investigators recognized that EDW4R achieved economies of scale. Three hubs described a measure of EDW4R success as effectively incentivizing investigators to use centralized resources rather than invest in separate analytics infrastructure. As 1 respondent noted, a hub must “[push] researchers to spend money in the right way rather than in a free-for-all fashion” so that, as another respondent indicated, “…people use the centralized [EDW4R] tools and don’t go wandering off on their own,” which increases inefficiency and risk of privacy breaches. For 1 hub, a small EDW4R team that “punch[ed] above [its] weight” and demonstrated “pragmatic excellence” justified an institutional request for additional resources.
Two hubs also described a potential shift in approach by institutional leadership in measuring EDW4R impact. A respondent from an exceptionally mature hub as noted in EDW4R WG discussions stated, “The assumption at the institution is that researchers will demonstrate value through outcomes, which leads to lowering of interest in monitoring activities. Instead of process we’re focusing on [measuring grants and high-impact publications enabled by the EDW4R].” Another respondent indicated, “Trying to measure impact is a little like measuring impact of HVAC (heating, ventilation, and air conditioning) on research,” suggesting measurement of EDW4R is challenging and unnecessary while benefit is understood and a taken-for-granted assumption of organizational life.
Sustainability
Respondents described a mix of funding from CTSA hub, medical school, health system, investigator grant, and industry sources. Participants noted a shift away from CTSA grant funding as the main source with 4 hubs describing increased dependence on institutional support. Two hubs independently described a funding structure consisting of 40% health system, 40% grants, and 20% industry. Notably, the hubs described industry funding as addressing technology infrastructure costs but cautioned against higher levels of commercial funding to ensure EDW4R activities remained aligned with the CTSA hub mission. An example of an industry-funded project described by a respondent involved using the EDW4R to generate real world evidence from EHR data. Two hubs described institutional investment for EDW4R with the expectation of cost recovery from grants.
Chargebacks were common cost recovery approaches for EDW4R teams with most hubs ( n = 15) indicating investigators were receptive to paying them. As 1 respondent indicated, “Nothing is free [to investigators]; some things are subsidized.” At least 2 hubs reported investigators being more willing to allocate grant funding to CTSA informatics faculty rather than staff despite faculty being more expensive. One hub indicated that request delivery time was a greater concern among investigators than cost. At a hub recognized as an exemplar in EDW4R WG discussions, a respondent noted that increasing staff was not a scalable solution, as faculty absorbed all staff capacity. Instead, the hub posited long-term sustainability as requiring improved informatics tools to empower researchers.
Regarding perceptions of costs from leadership, participant responses ranged from supportive ( n = 2) and acceptable for now ( n = 2) to actively seeking cost reductions ( n = 3) to “no news is good news” ( n = 6). A major cost was staffing with 1 hub noting, “Money is not the problem; hiring people is the problem,” and another hub employing undergraduate students to deliver EDW4R data for investigator requests. In 1 hub, finance leadership sought for EDW4R staff to track time in 20-minute increments to enable more granular cost accounting, which caused EDW4R leadership to push back against the proposal to not burden staff and cause them to seek employment elsewhere.
Demand management
Nearly all hubs ( n = 19) reported an increase in demand for EDW4R services over time, especially during the COVID-19 pandemic. Over 15 years, 1 hub reported scaling support from 150 to 6000 data requests per year. To address increased demand, multiple hubs ( n = 6) reported hiring additional staff. However, as 1 respondent indicated, “Our users doubled and tickets tripled, but our budget and team have not tripled, and our institution is not twice as successful in getting grants.” Hubs ( n = 6) also reported increasingly supporting investigators with self-service tools, such as TriNetX, i2b2, ATLAS, Epic SlicerDicer, and homegrown solutions. However, 3 hubs noted a need to increase data literacy among investigators using self-service tools, including how data in query tools relate to front-end EHR displays. As 1 respondent indicated, “[Investigators] don’t want data; they want answers.”
Prioritization of data requests proved challenging with teams employing a modified first-in-first-out (FIFO) approach with grant funding, alignment with clinical objectives, and investigator relationships expediting particular activities at the expense of others ( n = 7); 2 of the approaches assigned points to requests for ranking and fulfillment. Two respondents expressed concern that prioritization approaches blocked unfunded junior faculty from obtaining resources and developing as scientists.
Governance approaches varied with 5 hubs describing institution-wide oversight committees and 3 describing decision-making by EDW4R leadership. Hubs pursuing multi-stakeholder governance described benefits such as limiting investigator influence in resource allocation decisions ( n = 1) and separating EDW4R project prioritization from data request fulfillment ( n = 2) as well as challenges in aligning different interests ( n = 4). As 1 respondent indicated, “It is difficult to get consensus from researchers. They’re not thinking at a meta-level. They’re thinking about, ‘What’s my project and how do I get it done?’ That’s why they’re great at science. But you can’t get them together and [have them determine how to comprehensively meet the needs of researchers across the institution].” Citing overhead of managing complex relationships, 3 hubs relied on an EDW4R executive, such as a chief research information officer (CRIO), for governance decisions.
Accessibility: ideal user experience
Regarding the ideal user experience for EDW4R, respondents described multiple investigator personas requiring different solutions. One respondent distinguished between 2 types of investigators, “those who can do statistics and code versus those who cannot,” while others characterized distinct needs of clinical trialists, health services researchers, and data scientists. As 2 respondents indicated, EDW4R platforms need to clearly state to investigators the skills required for use.
For clinical trialists and other less technically facile researchers, 2 hubs described the ideal experience as being similar to a Google search, whereby an investigator can provide natural language terms for clinical data without needing programming skills or controlled vocabulary knowledge. Four hubs described a user experience wherein features of tools help improve data literacy for investigators. As 1 respondent indicated, systems should “…present not only data but context of data, exposing quality of data and true meaning of the vocabularies and models” to scientists.
Several hubs ( n = 9) described TriNetX as valuable for self-service access for investigators new to using EHR data for research. Respondents characterized TriNetX’s user interface as “clean, modern, and intuitive,” “the best example of how to make [self-service EHR data query] platforms easier” to use, and helpful for improving investigator data literacy. One hub indicated that the TriNetX experience could improve through implementation of alerts to guide investigator behavior in building queries (eg, use temporal relationships). While describing value of TriNetX, 1 respondent also expressed concern about the tradeoff between ease of use of the platform and reliance on a private company.
For technically facile investigators such as health services researchers and data scientists, respondents ( n = 4) characterized ideal user experience as involving access to computational resources and documentation about data, including source system lineage, mappings to common data models (eg, OMOP), and pre-configured sets of clinical concepts (eg, diabetes diagnosis codes and laboratory results). Four hubs described investigators interacting with EDW4R data through a secure institutional enclave with compute capacity, analytical software (ie, R, SAS, Python), and approved datasets ( n = 3), thereby obviating need for EDW4R teams to distribute spreadsheets containing EHR data extracts via email. Two hubs described institution-specific EDW4R infrastructure as limiting science, with 1 hub advocating for a centralized EDW4R shared across the CTSA consortium similar to N3C and another hub suggesting adoption of common infrastructure and containerized workflows to enable collaboration without transferring data across institutional boundaries. To foster development of technical skills necessary to use sophisticated data resources, 2 hubs highlighted value of clinician investigators conveying to other physician scientists success stories of learning and applying statistics and programming “in their own words” rather than as shared by informaticians.
Regardless of an investigator’s technical skills, several hubs ( n = 9) characterized ideal EDW4R user experience as involving team science or engagement between investigator, informatician, and biostatistician. Emphasizing value of multidisciplinary collaboration, 1 respondent indicated, “Data scientists don’t have all the answers. There is a gap between data insights and clinical insights. Converging these two is key.” As 1 respondent indicated, “Investigators think about a spreadsheet with 400 columns [representing different clinical variables], but the EDW4R is different” and requires developing common understanding between data requesters and providers. Multiple respondents stated that informaticians are well-positioned as the bridge between researcher and EDW4R for thoughtful interrogation of data. One participant described value of an informatics professional “[who] is available quickly, understands the problem quickly, and is able to deliver data in a timely and affordable fashion” to enable a study team to generate a grant proposal or publication. As another respondent indicated, informatics professionals help investigators “understand what one can request, feel respected and empowered, and learn bad news and good news, including things [informatics professionals] can help with that they didn’t know.” Another hub described a goal of having biostatistics and epidemiology staff extract data from an EDW4R so informatics staff can focus on more complex activities, such as deepening expertise of source system data.
Accessibility: opportunities for tools
To improve EDW4R accessibility for informaticians and non-informaticians, respondents described opportunities to incorporate user-centered design into platforms ( n = 4). One respondent noted for-profit companies such as TriNetX have invested in user interface and user experience while CTSA hubs have not. Another respondent characterized EDW4R tooling as “functionally motivated, not focused on human experience…built by engineers for engineers.” Two respondents described human–computer interaction as a scientific discipline that the EDW4R community should embrace to improve user experience.
Regarding whether improved EDW4R tools represent the largest need for non-informaticians, respondents almost equally agreed ( n = 5), partially agreed ( n = 6), and disagreed ( n = 6). Respondents agreeing indicated new tools were necessary to overcome human–computer interaction barriers, unsolved problems of clinical information extraction and representation, and a lack of informaticians to engage with study teams. As 1 respondent stated, “We are completely slammed with non-informatics community users. It’s amazing how much time is spent trying to help people help themselves…Without a way to empower non-informaticians, we will not be able to scale to keep up with demand.”
Those partially agreeing that EDW4R tools are the biggest gap for non-informaticians described a need to balance new tool development with expansions in researcher training, data engineering, and financial support. As 1 respondent indicated, “There are a lot of unsexy things in data work that are expensive that no one talks about” critical to EDW4R success. Participants disagreeing that EDW4R tools are the greatest barrier for non-informaticians described greater needs for thoughtful interrogation of EHR data, data literacy among non-informaticians, skilled informaticians, team science, and grant support for informatics effort. As 1 respondent stated, “The biggest bottleneck is getting people used to working with data and doing biostatistics and data science…Not solving [the problem] for data-savvy people and instead racing toward solving for non-informaticians is [a] huge [change in the wrong direction].” Two hubs expressed that funders should require involvement of informaticians as key personnel in grants as is customary of biostatisticians. By increasing availability of data without commensurate improvements in data understanding, 2 hubs cautioned that data interpretation and use could lead to danger. As 1 respondent indicated, “With an increase of data literacy across the enterprise, we have hope.”
In this qualitative study, informatics leaders from 21 CTSA hubs described approaches to understanding impact, ensuring sustainability, managing demand, and improving accessibility of EDW4R resources. The findings suggest emergence of markers of maturity and potential best practices as well as challenges in sustainability and opportunities for optimizing tools and teams.
Two observations of impact may be markers of maturity of EDW4R operations. 1 First, mature organizations appeared to shift measurement activities from EDW4R teams’ “burger-flipping” volume metrics 4 to researchers’ scholarly output and funding. Hubs where stakeholders recognize EDW4R as integral to scientific outcomes may be more mature. Second, investing in centralized EDW4R rather than standalone efforts, thus realizing economies of scale in EDW4R activities, may indicate maturity. EDW4R organization depends on “[t]he relationship between a CTSA hub and its [affiliated clinical enterprise(s)],” 4 and distinction between research and operational EHR data needs requires an institution to engage investigators with a more versatile workforce. 5 Centralization of EDW4R appears to yield efficiency.
For measuring impact of EDW4R, 1 hub’s institutional requirement for investigators to link all study protocols with funding records (ie, grants, contracts, institutional support) may represent a best practice for other institutions to adopt. Widespread implementation may enable EDW4R teams and the CTSA consortium to track funding of activities supported rather than rely on bibliometrics, which remains a challenge for hubs. As observed in nearly 2 decades of scholarship 3 , 39 , 40 and the current study, EDW4R sustainability remains a major barrier for CTSA hubs, and institutional support appears critical. 4 , 5 Notably, 2 hubs independently reported a goal of achieving support through 40% institutional, 40% extramural award, and 20% industry sources, an approach that other hubs may wish to pursue and could become a best practice.
Access to EDW4R services tailored to different types of investigators emerged as a common goal among hubs, all of which have observed demand for EDW4R services outpace capacity. To support non-informaticians, some hubs characterized a “Google-like search” of EHR data, which large language models (LLMs) with prompts may help address. 50 Respondents also recommended providing guidance to investigators within tools regarding data context and query strategies, which a solution based on InfoButtons could address. 51 Ultimately, however, workforce expansion 5 and team science appear crucial for success. Bringing together clinicians, biostatisticians, and informaticians who understand the strengths and limitations of EHR data and research design along with better tools may represent a tripartite solution for the CTSA consortium to achieve.
Limitations of this study require consideration. First, the convenience sample of 21 CTSA hubs represents neither all CTSA hubs nor all healthcare organizations. However, prior qualitative EDW4R studies 4 , 5 informed quantitative survey activities of CTSA hubs, 1 which future work can similarly address. Second, to protect privacy of participants, we omitted details related to respondents’ role seniority and presented context from interviews, verified through the EDW4R WG meeting series, using relative terms like “exceptionally mature hub,” which future qualitative and quantitative studies may address. Third, we conducted interviews prior to widespread adoption of LLM-based approaches that may affect user experience for engaging with EDW4R resources. Future studies can investigate EDW4R user-experience through use of emerging technologies.
Clinical and translational scientists increasingly rely on EDW4R services, and CTSA hubs need to responsibly allocate resources in a changing landscape. Although non-academic healthcare organizations differ in focus from CTSA hubs and other academic settings, all healthcare organizations consist of stakeholders from clinical, legal, privacy, IT, analytics, and other units that may seek to engage in secondary use of EHR data. Understanding how institutions across the CTSA consortium operate EDW4R can inform how CTSA hubs and other entities deliver critical data infrastructure.
This qualitative study of EDW4R operations adds to understanding of how informatics leaders seek to meet investigator needs for EHR data across the CTSA consortium. Scholars and practitioners may find findings valuable for measurement and operation of EDW4R infrastructure.
Boyd M. Knosp and Thomas R. Campion conceptualized the study and interview guide, conducted interviews, transcribed notes, and performed analysis. CTSA Steering Committee members provided guidance on accessibility questions in the interview guide. Catherine K. Craven, David A. Dorr, and Elmer V. Bernstam provided iterative interview guide and analytical feedback. Thomas R. Campion wrote the manuscript with contributions from Boyd M. Knosp. Catherine K. Craven, David A. Dorr, and Elmer V. Bernstam edited the manuscript. Thomas R. Campion and Boyd M. Knosp revised the manuscript.
Supplementary material is available at Journal of the American Medical Informatics Association online.
This study received support from the National Institutes of Health National Center for Advancing Translational Sciences through grant numbers UL1TR 002537 (Iowa), 000457 (Weill Cornell), 002369 (OHSU), and 003167 (UTHSCH).
The authors have no competing interests to report.
The data underlying this article will be shared on reasonable request to the corresponding author.
Knosp BM , Dorr DA , Campion TR. Maturity in enterprise data warehouses for research operations: analysis of a pilot study . J Clin Transl Sci . 2023 ; 7 ( 1 ): e70 .
Google Scholar
Obeid JS , Beskow LM , Rape M , et al. A survey of practices for the use of electronic health records to support research recruitment . J Clin Transl Sci . 2017 ; 1 ( 4 ): 246 - 252 .
MacKenzie SL , Wyatt MC , Schuff R , Tenenbaum JD , Anderson N. Practices and perspectives on building integrated data repositories: results from a 2010 CTSA survey . J Am Med Inform Assoc . 2012 ; 19 ( e1 ): e119 – e124 .
Campion TR , Craven CK , Dorr DA , Knosp BM. Understanding enterprise data warehouses to support clinical and translational research . J Am Med Inform Assoc . 2020 ; 27 ( 9 ): 1352 - 1358 .
Knosp BM , Craven CK , Dorr DA , Bernstam EV , Campion TR. Understanding enterprise data warehouses to support clinical and translational research: enterprise information technology relationships, data governance, workforce, and cloud computing . J Am Med Inform Assoc . 2022 ; 29 ( 4 ): 671 - 676 .
Carroll RJ , Eyler AE , Denny JC. Naïve Electronic Health Record phenotype identification for rheumatoid arthritis . AMIA Annu Symp Proc . 2011 ; 2011 : 189 - 196 .
Tan RSYC , Lin Q , Low GH , et al. Inferring cancer disease response from radiology reports using large language models with data augmentation and prompting . J Am Med Inform Assoc . 2023 ; 30 ( 10 ): 1657 - 1664 .
Hartman VC , Bapat SS , Weiner MG , Navi BB , Sholle ET , Campion TR. A method to automate the discharge summary hospital course for neurology patients . J Am Med Inform Assoc . 2023 ; 30 ( 12 ): 1995 - 2003 .
Khan MS , Carroll RJ. Inference-based correction of multi-site height and weight measurement data in the All of Us research program . J Am Med Inform Assoc . 2022 ; 29 ( 4 ): 626 - 630 .
Foer D , Rubins DM , Nguyen V , et al. Utilization of electronic health record sex and gender demographic fields: a metadata and mixed methods analysis . J Am Med Inform Assoc . 2024 ;31(4): 910 - 918 .
Cusick MM , Sholle ET , Davila MA , Kabariti J , Cole CL , Campion TR. A method to improve availability and quality of patient race data in an electronic health record system . Appl Clin Inform . 2020 ; 11 ( 5 ): 785 - 791 .
Hersh WR , Weiner MG , Embi PJ , et al. Caveats for the use of operational electronic health record data in comparative effectiveness research . Med Care . 2013 ; 51 ( 8 Suppl 3 ): S30 -S3 7 .
Joffe E , Byrne MJ , Reeder P , et al. A benchmark comparison of deterministic and probabilistic methods for defining manual review datasets in duplicate records reconciliation . J Am Med Inform Assoc . 2014 ; 21 ( 1 ): 97 - 104 .
Bernstam EV , Warner JL , Krauss JC , et al. Quantitating and assessing interoperability between electronic health records . J Am Med Inform Assoc . 2022 ; 29 ( 5 ): 753 - 760 .
Diaz-Garelli F , Johnson TR , Rahbar MH , Bernstam EV. Exploring the hazards of scaling up clinical data analyses: a drug side effect discovery case report . AMIA Jt Summits Transl Sci Proc . 2021 ; 2021 : 180 - 189 .
Weiskopf NG , Dorr DA , Jackson C , Lehmann HP , Thompson CA. Healthcare utilization is a collider: an introduction to collider bias in EHR data reuse . J Am Med Inform Assoc . 2023 ; 30 ( 5 ): 971 - 977 .
Wang M , Sushil M , Miao BY , Butte AJ. Bottom-up and top-down paradigms of artificial intelligence research approaches to healthcare data science using growing real-world big data . J Am Med Inform Assoc . 2023 ; 30 ( 7 ): 1323 - 1332 .
Schüffler PJ , Geneslaw L , Yarlagadda DVK , et al. Integrated digital pathology at scale: a solution for clinical diagnostics and cancer research at a large academic medical center . J Am Med Inform Assoc . 2021 ; 28 ( 9 ): 1874 - 1884 .
Hsu W , Markey MK , Wang MD. Biomedical imaging informatics in the era of precision medicine: progress, challenges, and opportunities . J Am Med Inform Assoc . 2013 ; 20 ( 6 ): 1010 - 1013 .
Castro VM , Gainer V , Wattanasin N , et al. The Mass General Brigham Biobank Portal: an i2b2-based data repository linking disparate and high-dimensional patient data to support multimodal analytics . J Am Med Inform Assoc . 2022 ; 29 ( 4 ): 643 - 651 .
Visweswaran S , McLay B , Cappella N , et al. An atomic approach to the design and implementation of a research data warehouse . J Am Med Inform Assoc . 2022 ; 29 ( 4 ): 601 - 608 .
Campion TR , Sholle ET , Pathak J , Johnson SB , Leonard JP , Cole CL. An architecture for research computing in health to support clinical and translational investigators with electronic patient data . J Am Med Inform Assoc . 2022 ; 29 ( 4 ): 677 - 685 .
Embi PJ , Payne PRO , Friedman CP. Learning from data: a recurring feature on the science and practice of data-driven learning health systems . Learn Health Syst . 2022 ; 6 ( 1 ): e10302 .
Grumbach K , Lucey CR , Johnston SC. Transforming from centers of learning to learning health systems: the challenge for academic health centers . JAMA . 2014 ; 311 ( 11 ): 1109 - 1110 .
Starren JB , Winter AQ , Lloyd-Jones DM. Enabling a learning health system through a unified enterprise data warehouse: the experience of the Northwestern University Clinical and Translational Sciences (NUCATS) Institute . Clin Transl Sci . 2015 ; 8 ( 4 ): 269 - 271 .
Marsolo K , Kiernan D , Toh S , et al. Assessing the impact of privacy-preserving record linkage on record overlap and patient demographic and clinical characteristics in PCORnet ® , the National Patient-Centered Clinical Research Network . J Am Med Inform Assoc . 2023 ; 30 ( 3 ): 447 - 455 .
Hogan WR , Shenkman EA , Robinson T , et al. The OneFlorida Data Trust: a centralized, translational research data infrastructure of statewide scope . J Am Med Inform Assoc . 2022 ; 29 ( 4 ): 686 - 693 .
Waitman LR , Song X , Walpitage DL , et al. Enhancing PCORnet Clinical Research Network data completeness by integrating multistate insurance claims with electronic health records in a cloud environment aligned with CMS security and privacy requirements . J Am Med Inform Assoc . 2022 ; 29 ( 4 ): 660 - 670 .
Mayo KR , Basford MA , Carroll RJ , et al. The All of Us data and research center: creating a secure, scalable, and sustainable ecosystem for biomedical research . Annu Rev Biomed Data Sci . 2023 ; 6 : 443 - 464 .
Zeng C , Schlueter DJ , Tran TC , et al. Comparison of phenomic profiles in the All of Us Research Program against the US general population and the UK Biobank . J Am Med Inform Assoc . 2024 ; 31 ( 4 ): 846 - 854 .
Reich C , Ostropolets A , Ryan P , et al. OHDSI Standardized Vocabularies—a large-scale centralized reference ontology for international data harmonization . J Am Med Inform Assoc . 2024 ; 31 ( 3 ): 583 - 590 .
Pfaff ER , Girvin AT , Gabriel DL , N3C Consortium , et al. Synergies between centralized and federated approaches to data quality: a report from the national COVID cohort collaborative . J Am Med Inform Assoc . 2022 ; 29 ( 4 ): 609 - 618 .
Pfaff ER , Girvin AT , Crosskey M , N3C and RECOVER Consortia , et al. De-black-boxing health AI: demonstrating reproducible machine learning computable phenotypes using the N3C-RECOVER Long COVID model in the All of Us data repository . J Am Med Inform Assoc . 2023 ; 30 ( 7 ): 1305 - 1312 .
Baron JM , Paranjape K , Love T , Sharma V , Heaney D , Prime M. Development of a “meta-model” to address missing data, predict patient-specific cancer survival and provide a foundation for clinical decision support . J Am Med Inform Assoc . 2021 ; 28 ( 3 ): 605 - 615 .
Palchuk MB , London JW , Perez-Rey D , et al. A global federated real-world data and analytics platform for research . JAMIA Open . 2023 ; 6 ( 2 ): ooad035 .
Wood EA , Campion TR. Design and implementation of an integrated data model to support clinical and translational research administration . J Am Med Inform Assoc . 2022 ; 29 ( 9 ): 1559 - 1566 .
Luke DA , Sarli CC , Suiter AM , et al. The translational science benefits model: a new framework for assessing the health and societal benefits of clinical and translational sciences . Clin Transl Sci . 2018 ; 11 ( 1 ): 77 - 84 .
Murphy SN , Dubey A , Embi PJ , et al. Current state of information technologies for the clinical research enterprise across academic medical centers . Clin Transl Sci . 2012 ; 5 ( 3 ): 281 - 284 .
DiLaura R , Turisco F , McGrew C , Reel S , Glaser J , Crowley WF. Use of informatics and information technologies in the clinical research enterprise within US academic medical centers: progress and challenges from 2005 to 2007 . J Investig Med . 2008 ; 56 ( 5 ): 770 - 779 .
Obeid JS , Tarczy-Hornoch P , Harris PA , et al. Sustainability considerations for clinical and translational research informatics infrastructure . J Clin Transl Sci . 2018 ; 2 ( 5 ): 267 - 275 .
Meeker D , Fu P , Garcia G , et al. Establishing a research informatics program in a public healthcare system: a case report with model documents . J Am Med Inform Assoc . 2022 ; 29 ( 4 ): 694 - 700 .
Walters KM , Jojic A , Pfaff ER , et al. Supporting research, protecting data: one institution’s approach to clinical data warehouse governance . J Am Med Inform Assoc . 2022 ; 29 ( 4 ): 707 - 712 .
Dobbins NJ , Spital CH , Black RA , et al. Leaf: an open-source, model-agnostic, data-driven web application for cohort discovery and translational biomedical research . J Am Med Inform Assoc . 2020 ; 27 ( 1 ): 109 - 118 .
Bakken S. User interfaces remain an important area of study . J Am Med Inform Assoc . 2023 ; 31 ( 1 ): 13 - 14 .
Marsolo K. Informatics and operations—let’s get integrated . J Am Med Inform Assoc . 2013 ; 20 ( 1 ): 122 - 124 .
Fridsma D. Basic informatics literacy: building a health information technology workforce . J Am Med Inform Assoc . 2016 ; 23 ( 3 ): 666 .
Hsieh H-F , Shannon SE. Three approaches to qualitative content analysis . Qual Health Res . 2005 ; 15 ( 9 ): 1277 - 1288 .
Lincoln YS , Guba E. Naturalistic Inquiry . Newbury Park, CA : Sage Publications ; 1985 .
Google Preview
Holtrop JS , Estabrooks PA , Gaglio B , et al. Understanding and applying the RE-AIM framework: clarifications and resources . J Clin Transl Sci . 2021 ; 5 ( 1 ): e126 .
Chen S , Li Y , Lu S , et al. Evaluating the ChatGPT family of models for biomedical reasoning and classification . J Am Med Inform Assoc . 2024 ; 31 ( 4 ): 940 - 948 .
Kennell T Jr , Dempsey DM , Cimino JJ. i3b3: Infobuttons for i2b2 as a mechanism for investigating the information needs of clinical researchers . AMIA Annu Symp Proc . 2017 ; 2016 : 696 - 704 .
Supplementary data
Email alerts, citing articles via.
- Recommend to your Library
Affiliations
- Online ISSN 1527-974X
- Copyright © 2024 American Medical Informatics Association
- About Oxford Academic
- Publish journals with us
- University press partners
- What we publish
- New features
- Open access
- Institutional account management
- Rights and permissions
- Get help with access
- Accessibility
- Advertising
- Media enquiries
- Oxford University Press
- Oxford Languages
- University of Oxford
Oxford University Press is a department of the University of Oxford. It furthers the University's objective of excellence in research, scholarship, and education by publishing worldwide
- Copyright © 2024 Oxford University Press
- Cookie settings
- Cookie policy
- Privacy policy
- Legal notice
This Feature Is Available To Subscribers Only
Sign In or Create an Account
This PDF is available to Subscribers Only
For full access to this pdf, sign in to an existing account, or purchase an annual subscription.
- Open access
- Published: 23 May 2024
Challenges of care coordination for complex patients among family medicine residents in a community ambulatory clinic: a qualitative study
- Moses Tan Mong Heng 1 ,
- Gilbert Yeo Tian Seng 2 &
- Eng Sing Lee 3 , 4
BMC Medical Education volume 24 , Article number: 562 ( 2024 ) Cite this article
Metrics details
Care coordination has been identified as one of five focuses of HealthierSG. Family medicine residents are expected to collaborate with other healthcare professionals for complex patients by the end of residency. However, many residents felt that it was challenging to coordinate care effectively among healthcare stakeholders. However, to date, no qualitative studies have explored these challenges. Therefore, this study aimed to understand the challenges encountered by family medicine residents when coordinating care for complex patients.
This was a qualitative descriptive study in which semi structured in-depth interviews were conducted and guided by a topic guide. Total population sampling of 15 third-year family medicine residents in the National Healthcare Group Polyclinics was performed. The interviews were performed over Zoom and were transcribed. Thematic analysis was subsequently performed to analyse the transcripts. Coding was performed iteratively by two independent researchers. Disagreements were adjudicated by a third coder. A coding framework was agreed upon. Potential themes were then independently developed based on the coding framework.
Six themes emerged from the data, namely, interprofessional communications, accessibility, personal knowledge, time constraints, patient factors and caregiver dissent.
Challenges faced by family medicine residents are multifaceted. While a few are systemic and pertain to the broader healthcare framework, others, such as issues of unfamiliarity with institutional workflows, community resources, and confidentiality, pertain to the microcosm of residency itself. These are reversible areas for improvement. These challenges can be addressed during planning of residency curricula to better equip family medicine residents with coordinating care for complex patients in the future.
Peer Review reports
The Agency for Integrated Care of Singapore defines complex patients as those fulfilling at least two of the following three health domains: complex medical issues (three or more chronic conditions or advanced disease), functional impairment (requiring assistance in one or more activities of daily living or cognitive impairment) or psychosocial impairment (caregiver issues, family issues, financial issues, social isolation or psychological issues) [ 1 ]. As Singapore experienced rapid aging, the prevalence of chronic conditions has increased. Interaction between chronic conditions adds to the complexity of management. In addition, the complexity of chronic disease, coupled with psychosocial issues, reduces individuals’ ability to seek help and self-manage care while simultaneously rendering care delivery more complex [ 2 , 3 ].
Among the many strategies available for enhancing care delivery for complex patients, care coordination has been identified by the World Health Organisation (WHO) as a global priority for reorienting health services to the needs of complex patients [ 4 ]. Care coordination has become increasingly necessary as patients become more chronically ill, and our healthcare system has become more complex and fragmented [ 5 ]. When performed correctly, care coordination reduces hospital admissions; improves the quality of chronic disease management, patient satisfaction, and provides better access to specialty care [ 6 ]. The need for greater collaboration and coordination of care has also gained greater recognition in Singapore as mobilising community partnerships and constructing an integrated health and social ecosystem have been identified as key focuses of the HealthierSG initiative. Healthier SG is a multi-year initiative by Singapore’s Ministry of Health to shift the focus from reactively caring for those who are sick, to proactively preventing individuals from falling sick. This requires a systems effort to integrate care and support community partnerships, as well as a paradigm shift for the population to reshape their behaviors and lifestyles [ 7 ].
Despite the growing emphasis on care coordination, current research performed among family physicians in Singapore has revealed key areas of deficiency, such as inadequate knowledge of care prioritisation, patient characteristics such as cognitive or memory problems, poor social support, and low levels of motivation, hampering efficient care coordination between healthcare professionals for complex patients [ 8 ]. To date, no similar studies have been conducted on family medicine residents, who are expected to be proficient in this aspect after the completion of a three-year family medicine residency programme [ 9 ]. The ability to standardise the teaching of care coordination is also made even more challenging due to marked heterogeneity in the residency curriculum between institutions.
In National Healthcare Group Polyclinics (NHGP), family medicine residents are exposed to the breadth of family medicine through chronic disease care in the polyclinic interspersed with rotations to hospital outpatient clinics. At the end of residency, the Accreditation Council for Graduate Medical Education (ACGME) requires residents to perform effective coordination of patient-centered care during patient consultations with nurses, allied health, across different disciplines and specialties and to advocate for safe and effective transitions of care within and across healthcare delivery systems, including outpatient settings [ 10 ].
Effective care coordination can be understood from the conceptual framework laid out by the WHO which categorises care coordination into three major aspects, namely, sequential coordination, parallel coordination and indirect influences on provider relations [ 4 , 11 ]. Sequential care coordination entails coordinating cross-sectoral transfers of care between tertiary and primary care. Parallel coordination of care encompasses co-working within multidisciplinary healthcare teams located in a common setting. Indirect influences on provider relations refer to any intervention to encourage both internal and external coordination [ 11 ].
We aim to uncover the additional challenges, both known and unknown, that residents face in aspects of care coordination. Understanding these challenges would allow educators to facilitate this domain during residency training, so that they could be better prepared to coordinate care for complex patients when they become family physicians.
Study participants
Total population sampling was carried out to recruit all 15 third-year family medicine residents from NHGP who had experience with the care of at least three complex patients in the area of care coordination. We excluded first- and second-year family medicine residents as the majority of their time are spent at providing care in the hospital setting.
Eligible participants were identified by a name list provided by the residency administrators and consent was sought by residency administrators for the research team to contact the participants. Subsequently, we contacted all third-year family medicine residents in NHGP by telephone, provided information on the research, confirmed their eligibility and scheduled a timing for consent taking. Participation in the research was voluntary. The study team was not involved in any direct supervisory role or part of the core teaching faculty to ensure that participation was voluntary. Adequate time was given for participants to consider their decision to participate in the study. The interviews were then conducted on separate occasions after consent was obtained. Ethical approval was obtained from the National Healthcare Group Domain Specific Review Board (Ref: 2020/01377).
NHGP forms the primary healthcare arm of the National Healthcare Group (NHG). It operates eight polyclinics that serve a significant proportion of the population in the central and northern parts of Singapore. NHGP provides a comprehensive range of health services for the family, including treatment for acute medical conditions, management of chronic diseases, women & child health services, and dental care. The focus of NHGP’s care is on health promotion and disease prevention, early and accurate diagnosis, and disease management through physician-led team-based care.
NHG family medicine residents run resident care continuity (RCC) clinics once a week at their base polyclinics. There are 15 third-year family medicine residents. Some of these RCC clinics are embedded within a team consisting of a care manager and a care coordinator. In addition to the health services described above, family medicine residents also review patients discharged from inpatient settings and specialist outpatient clinics. Family medicine residents can then take over their care and continue community follow-up longitudinally.
Data collection
Data were collected from January 2021 to January 2022 through individual in-depth interviews performed via video conferencing over Zoom® in view of pandemic restrictions [ 12 ].
Socioeconomic demographic information, including age, sex, ethnicity as well as school of undergraduate medical degree, post graduate postings and number of post graduate medical degrees were sought before the start of the interview. This was followed by a semi structured interview conducted by the principal investigator.
An interview guide was designed with reference to the conceptual framework laid out by the WHO on effective care coordination, existing literature and discussion among the research team members. A sample of the main interview questions is provided in Table 1 . The interview guide consisted of questions on participants’ background and understanding of care coordination, followed by questions about the perceived challenges of care coordination for complex patients. The interviews proceeded in a way consistent with natural conversation and questions were not necessarily covered in the stated order. The participants were given the opportunity to discuss freely based on the questions asked. Probes and follow-up questions were used throughout the interview to clarify and to facilitate discussion. The interview questions were narrowed and modified over the course of the study using an iterative process based on the content of previous interviews.
The interviews were digitally audio-recorded and transcribed verbatim. The transcripts were then verified by the principal investigator via audio recordings. Relevant fieldnotes, observations and reflexive diaries were also recorded during and after the interviews.
Data analysis
A qualitative descriptive research methodology was chosen to explore the perspectives on challenges that residents face with regard to care coordination for complex patients. This methodology allows investigators to remain close to the data to obtain an accurate and detailed representation of the residents’ experiences and perceptions [ 13 ].
Using qualitative thematic analysis [ 14 ], the research team (MTMH, GYTS, LES) coded a sample of transcripts independently, before meeting to discuss and agree on a coding framework that was then applied to all transcripts. The qualitative findings are reported using the Consolidated Criteria for Reporting Qualitative Research (COREC) [ 15 ].
Participant characteristics
All 15 third-year residents from NHGP provided consent for the interviews. There were 11 female participants. The mean age of the 15 participants was 30 years (range from 29 to 32 years). Most participants obtained their undergraduate degree from Yong Loo Lin School of Medicine, Singapore, with two who obtained their degree from the University of Malaya and one from King’s College London. Two participants had postgraduate diplomas in Family Medicine (GDFM) [ 16 ]. Six participants had prior experience working in a polyclinic setting before residency, while three participants did not have any prior experience working in other postings as medical officers before residency.
Six overarching challenges faced by residents in coordinating care for complex patients in primary care were shared.
Challenges with interprofessional communications
Paucity of handovers.
Residents reported that handover notes between healthcare professionals might be absent or that they may not contain important details on areas to follow up on. This results in the omission of essential information, which affects subsequent consultations.
Sometimes when I look through my colleague’s handover notes, I do not find anything about discussion; you know, referring to specialists and all. Therefore that part I feel is very challenging because, I do not know what to tell the patient. (Resident 3)
We may discuss or have the patients share things that are more than what we handover, because we only handover a summary, rather than the details. Therefore, important details might sometimes be lost along the way. (Resident 4)
Overreliance on physical memos
Another reason behind poor interprofessional communications stems from overreliance on physical memos. Sometimes, patients forget to bring these memos for their appointments. Residents also lamented that memos that are too long would lead to information fatigue, while those that are too short lack clarity on areas for primary care to follow up on.
In addition, there are scenarios in which the patient forgets to bring the memo. (Resident 1)
When we see post discharge complex patients, we get a memo that is like a copy of the discharge summary; it is not very specific. Therefore, we must take time to read through and decipher the issues that need to be addressed in the polyclinic. (Resident 3)
However, sometimes, certain unresolved matters in the hospital are not mentioned in the memo, so we are also not sure whether we should follow up on those issues. (Resident 11)
Challenges with accessibility to care
Absence of a common platform for accessing information.
Residents highlighted that the absence of a common platform across the nation has affected their ability to access patients’ clinical progress notes from other institutions. This results in an overreliance on the patient to provide subjective information on their progress, which is often challenging in view of heterogeneity in patient health literacy.
We can see some hospitals’ clinic notes, but we do not have access to other hospitals’ clinic notes as they do not function on the same platform as us. Therefore, it is quite challenging to truly know why the patient is here and exactly what you need to do, especially when the patient himself also has no idea what needs to be reviewed. (Resident 2)
Despite having NEHR, we are still unable to see the clinical records of some hospitals, especially for their outpatient clinics. (Resident 4)
Conflicts arising from patient confidentiality
Confidentiality was mentioned by the participants as a stumbling block to care coordination in complex patients. Residents are uncertain regarding the limits of case discussion for fear of infringing the Personal Data Protection Act (PDPA) [ 17 ]. Because of the perceived issues surrounding the PDPA, they are unsure of what could or could not be shared.
For referrals to the Emergency Department, I will check the NEHR on the same day to ensure that the patient has gone. However, for referrals to outpatient clinics, I cannot check the NEHR more than one day after the consult due to PDPA. Hence, I would not know whether the patient has gone. (Resident 13)
We are not supposed to discuss with other residents or other doctors, who are not involved in the patients’ care because of PDPA. The problem lies if it is truly a complicated case, I would not know what can or cannot be shared; and whether I would be infringing on PDPA. (Resident 14)
Inaccessibility to partners
Care coordination is a challenge because specialists and community partners are not as accessible or responsive as participants would like them to be at this moment in time, when residents would like to discuss the care of complex patients with them. Logistical barriers and lack of responses from partners are some of the reasons cited by participants.
If you have proper contact for certain complex issues or conditions, then I think it will be great. However, I do not have the channel to contact these specialists. (Resident 11)
It is quite difficult to call a neurologist or even find a number because we do not have access to the directories of hospital doctors. (Resident 6)
I tried calling or emailing the specialists, but I did not receive any reply. (Resident 8)
Challenges with knowledge deficiencies
Residents also cited personal knowledge deficiencies in identifying suitable community resources for organising care. They also highlighted difficulties keeping pace with rapidly evolving institutional workflows.
I am not sure of the community partners whom we can tap on to coordinate care, such as social services, and how we go about getting these. (Resident 11)
My lack of knowledge stems more from the workflows of the institution to understand its process and how to seek help for these complex patients who need different clinical services to coordinate their care. Nobody taught me all this. (Resident 12)
Challenges with time constraints
Due to the lack of time in the polyclinic, participants feel challenged that they would usually have to prioritise the management of medical issues over social issues due to the lack of time. They would have to defer these social issues to the next appointment. Residents are also concerned that these important social issues may also not be addressed in the next appointment as the patient might be too medically complex. Residents lamented that because of a lack of time, it is difficult to perform anything additional for the patient, such as coordinating care with community partners for better health outcomes.
With the pressure of time, we would want to prioritise medical issues over social issues. Sometimes, we are also not sure whether these social issues will be addressed in the next appointment because the patient is just too complex. (Resident 5)
Because we are always very rushed, it is very difficult to do anything extra for the patient such as coordinating care with other parties, other than a typical consultation of history, physical examination and medical management. (Resident 1)
Challenges with patients
Conflicts with respecting patient autonomy.
Another major impediment to care coordination for complex patients is the patients themselves and their unwillingness to receive care. Residents sometimes feel that they are left with no choice but to respect the patients’ autonomy at the expense of optimal management for their complex medical conditions.
Sometimes we do have such patients where they have very fixed beliefs, and when you try to challenge them, they are also not keen to listen. (Resident 7)
If failing all my advice, he is still not keen; I will have to respect his autonomy to decide against the referral, which might to his health detriment. (Resident 9)
Poor health literacy
Residents also described that a patient’s poor health literacy can greatly impact his or her ability to coordinate care. As complex patients have many medical conditions, they must have clear understanding of their medications and clinical management. Poor knowledge of their medical conditions can lead to a high rate of appointment defaults and affect residents’ ability to plan shared care with other members of the healthcare team, as patients do not turn up for their appointments in the first place.
I think the classic examples will be those elderly with many conditions, yet they are unaware of their medical conditions. Sometimes, you know that if you let them out the door, they would still be lost, and they would continue to default all their subsequent appointments with the specialists or allied health. (Resident 8)
Patient health literacy is an important obstacle to coordinating care. (Resident 9)
Challenges with caregivers
Caregivers with differing priorities.
Participants cited caregivers with differing priorities as the most widely encountered and recurring reason that caregivers gave against better coordination with other healthcare professionals. They cited work exigencies and lack of suitable time to bring their relatives to polyclinics for consultations with other healthcare professionals.
The most common thing is that they tell us that they are struggling with finances, but when you offer a referral to the medical social worker, they declined because they find that it is too inconvenient to make a separate trip down to the polyclinic to see him. (Resident 13)
This is not because the patient does not need resources; rather, the problem is because the caregiver thinks that it is very troublesome. (Resident 14)
Multiple caregivers
Having multiple caregivers and spokespersons attending to the care of the patient would also impair care coordination between caregivers, patients and other healthcare professionals. Residents spoke about different caregivers having varying views on the care of their loved ones, and sometimes these views might conflict, leading to confusion between patients and healthcare teams.
If there are too many caregivers on board, this might pose an issue, especially if the patient is quite complex and a decision has to be made whether to engage the specialists or refer the patient to community resources. This prolongs the consultation. (Resident 9)
The family is also slightly lost because different children, who are in charge of different areas of the patient’s care, still have different opinions on his care. (Resident 5)
In this study, the participants provided insights into the challenges they faced as family medicine residents towards care coordination for patients with complex needs. These challenges stem from many factors, including interprofessional communication, accessibility, personal knowledge, time constraints, patient factors and caregiver dissent. Nonetheless, all participants remained committed to the concept of collaboration between themselves and other healthcare professionalisms, despite these challenges. Residents also comprehend that their practice of medicine cannot be performed in silos. Coordination and collaboration with community and tertiary partners are necessary to ensure that complex patients do not fall through the cracks, but are well supported at every level of healthcare touchpoint.
However, the implementation and administration of care coordination with partners leave much to be desired. Family medicine residents highlighted important challenges associated with sequential coordination, such as inaccessibility to specialists and overreliance on physical memos for communication, with the unfortunate part of patients serving as deliverymen to deliver letters from one doctor to the nextOn the whole, communications with specialists seemed to be either one-way or delayed. Due to the paucity of cross-talk and exchange of ideas to develop a shared care plan for complex patients, family medicine residents feel isolated and unsupported. Care fragmentation has implications for both their learning about care collaboration and patient safety. Therefore, much can be done to align the paradigms of specialists in tertiary hospitals with those of primary care providers in the overall management of complex patients.
Within the institution, inadequate handovers between healthcare professionals and time constraints are some of the parallel challenges highlighted by residents that impede their efficiency at care coordination. While time constraints are a systemic concern, ensuring proper handovers within the institution can be inculcated to improve communication and collaboration.
As learners, the lack of knowledge on workflows and community resources are important indirect influences on internal and external coordination between healthcare professionals. While community resources are taught to residents, these are taught in a bolus manner. Perhaps, one could consider adopting spaced repetitions to encourage retention, as spacing out encounters with materials over time produce superior long-term learning, compared with repetitions that are massed together [ 18 ]. As such encounters are also more often caught than taught, educators could also use opportunities during patient encounters to teach residents how care could be collaborated with the community.
Although there is a paucity of studies focusing on the challenges faced by family medicine residents in coordinating care for patients with complex needs, several studies have been performed within primary care settings; these findings resonate with our findings. Locally, a study examining the public primary care system using a public care model identified the overreliance of physical memos, absence of a common platform and inaccessibility of specialists to respond in a timely manner as the main challenges faced at care coordination [ 19 ]. Another study also described ineffective communication and handovers between staff, resulting in a lack of care coordination and threats to patient safety [ 20 ]. The ineffective communication between primary and secondary care was also supported by another study that found delayed replies in response letters and omission of important details such as current medications and changes to medications lists. This might further impede patient safety [ 21 ]. Finally, a systematic review of qualitative studies on family physicians’ care for complex patients highlighted issues related to care prioritisation and patient characteristics, such as cognitive or memory problems, poor social support, and low levels of motivation hampering care coordination for complex patients [ 22 ]. Even though participants in the cited studies were primary care physicians and not residents, the corroboration of themes from other studies with our study reflects its pervasiveness among doctors. These are therefore important areas to look into to enhance care coordination and transitions in our healthcare ecosystem.
Despite these similarities, this study contributes new perspectives on the challenges of residents face in care coordination in areas of self-recognition of workflow unfamiliarity and the double edged sword of confidentiality. Some of the findings might differ from those of studies involving family physicians. First, there is a lack of knowledge on workflows. While residents are concerned about keeping up with changing workflows, family doctors from other countries are worried about the feasibility of applying these guidelines in a patient-centered manner, instead of a disease-specific manner [ 8 ]. This difference between these two groups may be attributed to the lack of constant presence in the primary care setting during the early years of residency. The inherent concerns regarding workflows may also be related to their inability to regard patients as a whole and to prioritise their issues. Keeping abreast of workflows might be a coping mechanism to ensure that patients are at least managed safely under their care. Therefore, not only should educators highlight important workflow updates to residents when they are out-rotated to tertiary hospitals, but there is also a need to train residents to treat patients as a whole so that residents can develop a rational approach for managing complex patients within their immediate context.
Secondly, there is an issue with confidentiality. Residents viewed confidentiality as a double-edged sword. While they understood the need to maintain patient confidentiality, they were torn by the extent of confidentiality should they need to discuss with other members of healthcare teams due to their lack of knowledge. This is a new area that has not been explored in preexisting research involving family medicine doctors. Perhaps this reflects the residents’ lack of experience dealing with such issues on confidentiality and the way to coordinate care within its boundaries.
In Singapore, family medicine residency is a three-year programme aimed at training doctors to become full-fledge family physicians who are proficient at coordinating care in the community. The findings from this study revealed that the challenges that our residents faced were similar to those faced by family physicians in other countries. These findings show that such challenges transcend geographical locations and all aspects of medical care.
While some of these challenges, such as time constraints, are systemic issues, other aspects, such as documentation and handovers, personal knowledge deficiencies, confidentiality and accessibility to partners, can be improved. This can be done through frequent updates on institutional workflows and community resources during residency meetings to residents. Residents could also run through mock case studies of complex patients to train themselves on areas of improvement in terms of handovers and documentation. Multidisciplinary team discussions with allied health professionals would also facilitate better understanding of the resources available and promote the exchange of ideas. Shared care plan discussion with patients’ involvement can provide them with an opportunity to express their healthcare decisions [ 23 ]. Furthermore, facilitating electronic integration and education of healthcare professionals to enhance integration of primary and secondary care of chronic diseases can facilitate better clinical outcomes 24 . Additionally, group discussions can be conducted to discuss commonly encountered issues of confidentiality to provide a better understanding among residents. Finally, common secured specialist-specific chat groups can be established to enhance accessibility when specialist inputs are needed.
It is with this hope that these timely interventions, when implemented in a longitudinal manner over the three years of residency, can culminate in the development of a family physician who is comfortable planning and coordinating care with relevant healthcare professionals within the community and tertiary setting.
Strengths and limitations
To our knowledge, this is the first qualitative study on the challenges perceived by residents on care coordination in complex patients. The use of total population sampling with full participation of all the residents in the institution and in-depth interviews painted a complete picture by harnessing the views of all residents in the institution. This provided a bird’s eye view to their challenges in terms of coordinating care with these complex patients to inform future residency education interventions. The trustworthiness of the study was enhanced by striving for credibility, dependability, transferability and confirmability.
This study has a few limitations. This study looked at residents within an institution itself and not across the whole of Singapore; hence, it may not be transferable to the rest of the family medicine residents in other institutions. Additionally, the investigator who conducted the interviews analysed the transcripts, which may have been influenced by personal experiences with residency. To mitigate this bias, we employed reflexivity and had another two investigators (GYTS and LES) who was not involved in residency to analyse the data independently.
The findings from this study identified both gaps in learning among residents and gaps in teaching residents with respect to understanding how to coordinate care, finding resources to better coordinate care and making sense of the boundaries of confidentiality where care coordination for complex patients is concerned. It is therefore vital to address these gaps early so that residents can be comfortable with collaborating with healthcare professionals from other institutions for their patients.
Data availability
The datasets used and analysed for this study are available from the corresponding authors upon reasonable request.
Abbreviations
Agency of Integrated Care
World Health Organisation
National Healthcare Group Polyclinics
Accreditation Council for Graduate Medical Education
Personal Data Protection Act
National Electronic Health Records
Community Case Management Service (CCMS). The Agency for Integrated Care (AIC). (2019). https://fycs.org/wp-content/uploads/2023/04/CCMS-Referral-Form-V1.pdf Accessed 6 February 2021.
Zulman D. Quality of care for patients with multiple chronic conditions: the role of comorbidity interrelatedness. J Gen Intern Med. 2014;29:529–37.
Article Google Scholar
Vogeli C, Shields A. Multiple chronic conditions: prevalence, health consequences, and implications for quality, care management, and costs. J Gen Intern Med. 2007;22:391–5.
World Health Organisation. Continuity and coordination of care: a practice brief to support implementation of the WHO Framework on integrated people-centred health services. (2019). Accessed 4 March 2021.
Stille C, Bell D. Coordinating care across diseases, settings, and clinicians: a key role for the generalist in practice. Ann Intern Med. 2015;142:700–8.
Friedman A, Shaw E, et al. Facilitators and barriers to care coordination in patient-centered Medical homes (PCMHs) from coordinators’ perspectives. J Am Board Fam Med. 2016;29:90–101.
Ministry of Health, Singapore. Promoting healthier living while targeting specific subpopulations. (2022). https://www.moh.gov.sg/news-highlights/details/promoting-overall-healthier-living-while-targeting-specific-sub-populations . Accessed 15 May 2021.
Adeniji C, Kenning C, et al. What are the core predictors of ‘hassles’ among patients with multimorbidity in primary care? A cross sectional study. BMC Health Serv Res. 2015;15:255.
NHG Polyclinics. NHG Residency Family Medicine: Learning Experience. (2020). https://www.nhgeducation.nhg.com.sg/nhgresidency/programmes/training-and-residency-programmes/family-medicine/learning-experience . Accessed 8 June 2021.
International ACGME. LLC. Family Medicine Milestones for Singapore. (2017) Accessed 20 February 2021.
Øvretveit J. Does clinical coordination improve quality and save money? A summary of review and evidence. (2011) https://www.health.org.uk/sites/default/files/DoesClinicalCoordinationImproveQualityAndSaveMoneyVol1_summary.pdf Accessed 4 April 2021.
Ministry of Health, Singapore. Updates on Phase 3 (Heightened Alert) Measures. (2021) https://www.moh.gov.sg/news-highlights/details/updates-on-phase-3-(heightened-alert)-measures . Accessed 8 September 2021.
Bradshaw C, Doody O. Employing a qualitative description Approach in Health Care Research. Glob Qual Nurs Res. 2017. https://doi.org/10.1177/2333393617742282
Braun V. Using thematic analysis in psychology. Qual Res Psychol. 2006;3(2):77–101.
Tong A, Sainsbury P et al. Consolidated criteria for reporting qualitative research (COREQ): a 32–item checklist for interviews and focus groups. Int J Qual Health Care. 2007;19(6):349–57. College of Family Physicians, Singapore. Graduate Diploma in Family Medicine. (2023). https://www.cfps.org.sg/programmes/graduate-diploma-in-fm/ . Accessed 18 September 2024.
Personal Data Protection Commission, Singapore. Advisory Guidelines for the Healthcare Sector. (2017). Accessed 6 October 2021.
Kang S. Spaced Repetition promotes efficient and effective learning: policy implications for instruction. Policy Insights Behav Brain Sci. 2016;3(1):12–6.
Foo K, Sundram M, et al. Facilitators and barriers of managing patients with multiple chronic conditions in the community: a qualitative study. BMC Public Health. 2020;20:273.
Hays R, Daker-White G, et al. Threats to patient safety in primary care reported by older people with multimorbidity: baseline findings from a longitudinal qualitative study and implications for intervention. BMC Health Serv Res. 2017;17:754.
Dinsdale E, Hannigan A, et al. Communication between primary and secondary care: deficits and danger. Fam Pract. 2020;37(1):63–8.
Google Scholar
Sinnott C, McHugh S, et al. GPs’ perspectives on the management of patients with multimorbidity: systematic review and synthesis of qualitative research. BMJ Open. 2013. https://doi.org/10.1136/bmjopen-2013-003610
Bevilacqua G, Bolcato M. et.al. Shared care plan: an extraordinary tool for the personalisation of medicine and respect for self determination. Acta Biomed, 2021; 92(1).
Murtagh M, Mccombe G, et al. Integrating primary and secondary care to enhance chronic disease management: a scoping review. Int J Integr Care. 2021;21(1):4, 1–15.
Download references
Acknowledgements
The authors would like to thank all the residents who participated in this study. The authors would also like to thank the members of the National Healthcare Group Polyclinics Clinical Research Unit for their technical and administrative support.
This study was supported by the National Healthcare Group Polyclinics Research Grant (Ref: RF-2020-001).
Author information
Authors and affiliations.
National Healthcare Group Polyclinics, 21 Geylang East Central, Singapore, 389707, Singapore
Moses Tan Mong Heng
Department of Transitional Care, Woodlands Health, 17 Woodlands Dr 17, Singapore, 737628, Singapore
Gilbert Yeo Tian Seng
National Healthcare Group Polyclinics, 3 Fusionopolis Link, Nexus @ one-north, Singapore, 138543, Singapore
Eng Sing Lee
Lee Kong Chian School of Medicine, Singapore, 11 Mandalay Road, 308207, Singapore
You can also search for this author in PubMed Google Scholar
Contributions
M.T.M.H, G.Y.T.S. and L.E.S. conceived the study in discussions. M.T.M.H. conducted the interviews, observed, and made field notes, led the data analysis and drafted the manuscript. G.T.Y.S. and L.E.S. provided additional reflections on the data analysis and significant restructuring of the first draft of the manuscript. All the authors contributed to the discussion of the findings, provided critical comments and approved the final manuscript.
Corresponding author
Correspondence to Moses Tan Mong Heng .
Ethics declarations
Ethics approval and consent to participate.
Ethics approval was obtained from the National Healthcare Group Domain Specific Review Board (Ref: 2020/01377). Participants were given a study information sheet before completing the consent form. Written informed consent was obtained from all participants prior to the interviews. All study procedures were conducted in accordance with relevant ethical guidelines and regulations.
Consent for publication
All participants provided consent for the findings to be published in peer-reviewed scientific journals and presented at conferences.
Competing interests
The authors declare no competing interests.
Additional information
Publisher’s note.
Springer Nature remains neutral with regard to jurisdictional claims in published maps and institutional affiliations.
Rights and permissions
Open Access This article is licensed under a Creative Commons Attribution 4.0 International License, which permits use, sharing, adaptation, distribution and reproduction in any medium or format, as long as you give appropriate credit to the original author(s) and the source, provide a link to the Creative Commons licence, and indicate if changes were made. The images or other third party material in this article are included in the article’s Creative Commons licence, unless indicated otherwise in a credit line to the material. If material is not included in the article’s Creative Commons licence and your intended use is not permitted by statutory regulation or exceeds the permitted use, you will need to obtain permission directly from the copyright holder. To view a copy of this licence, visit http://creativecommons.org/licenses/by/4.0/ . The Creative Commons Public Domain Dedication waiver ( http://creativecommons.org/publicdomain/zero/1.0/ ) applies to the data made available in this article, unless otherwise stated in a credit line to the data.
Reprints and permissions
About this article
Cite this article.
Heng, M.T.M., Seng, G.Y.T. & Lee, E.S. Challenges of care coordination for complex patients among family medicine residents in a community ambulatory clinic: a qualitative study. BMC Med Educ 24 , 562 (2024). https://doi.org/10.1186/s12909-024-05543-7
Download citation
Received : 30 December 2023
Accepted : 08 May 2024
Published : 23 May 2024
DOI : https://doi.org/10.1186/s12909-024-05543-7
Share this article
Anyone you share the following link with will be able to read this content:
Sorry, a shareable link is not currently available for this article.
Provided by the Springer Nature SharedIt content-sharing initiative
- Primary care
- Qualitative interviews
- Care coordination
- Complex patients
BMC Medical Education
ISSN: 1472-6920
- Submission enquiries: [email protected]
- General enquiries: [email protected]

An official website of the United States government
The .gov means it’s official. Federal government websites often end in .gov or .mil. Before sharing sensitive information, make sure you’re on a federal government site.
The site is secure. The https:// ensures that you are connecting to the official website and that any information you provide is encrypted and transmitted securely.
- Publications
- Account settings
Preview improvements coming to the PMC website in October 2024. Learn More or Try it out now .
- Advanced Search
- Journal List

Barriers and facilitators to qualitative data sharing in the United States: A survey of qualitative researchers
Jessica mozersky.
1 Bioethics Research Center, Division of General Medical Sciences, Washington University School of Medicine, St. Louis, MO, United States of America
Tristan McIntosh
Heidi a. walsh, meredith v. parsons, melody goodman.
2 School of Global Public Health, New York University, New York, NY, United States of America
James M. DuBois
Associated data.
The de-identified survey data underlying the results reported here are included with this manuscript as Supporting Information .
Qualitative health data are rarely shared in the United States (U.S.). This is unfortunate because gathering qualitative data is labor and time-intensive, and data sharing enables secondary research, training, and transparency. A new U.S. federal policy mandates data sharing by 2023, and is agnostic to data type. We surveyed U.S. qualitative researchers (N = 425) on the barriers and facilitators of sharing qualitative health or sensitive research data. Most researchers (96%) have never shared qualitative data in a repository. Primary concerns were lack of participant permission to share data, data sensitivity, and breaching trust. Researcher willingness to share would increase if participants agreed and if sharing increased the societal impact of their research. Key resources to increase willingness to share were funding, guidance, and de-identification assistance. Public health and biomedical researchers were most willing to share. Qualitative researchers need to prepare for this new reality as sharing qualitative data requires unique considerations.
Introduction
Qualitative health data—such as data from interviews or focus groups—are rarely shared in the United States (U.S.) [ 1 p.161, 2 ]. This is unfortunate because qualitative data are labor and time-intensive to gather, and data sharing would enable secondary research, enhance training, and increase transparency. In contrast, qualitative data sharing is more common in places such as the UK, Finland, Germany, and Australia [ 3 , 4 ]. The UK Data Service is now a well-established archive providing infrastructure and services to facilitate qualitative data sharing with a collection of nearly 1000 data sets [ 3 ].
The National Institutes of Health (NIH), the largest federal funding body of health research in the U.S., recently updated its policy for data management and sharing to increase data sharing obligations and enforcement. The policy will take effect in 2023. NIH guidance states that, ‘data should be made as widely and freely available as possible while safeguarding the privacy of participants, and protecting confidential and proprietary data’ [ 5 ]. The policy is agnostic to data types, defining data broadly as ‘recorded factual material commonly accepted in the scientific community as necessary to validate and replicate research findings’ including unpublished data [ 5 ]. This policy is noteworthy because funded researchers frequently share quantitative data to comply with NIH policy, but the revised policy will require data sharing of all types of data, potentially leaving qualitative researchers unprepared for the coming reality.
Our prior work has identified a series of benefits and concerns regarding qualitative data sharing (QDS). It could increase the transparency of research and enable verification of findings, which can foster public trust [ 1 , 2 , 6 – 8 ]. Sharing data enables secondary users to explore new research questions, or collate findings across multiple studies, maximizing the value of data that are often costly and resource intensive to collect. QDS may reduce participant burden by allowing researchers to use existing data rather than collect new data. QDS also provides an opportunity for students to learn how to conduct data analysis, examining research questions using real data when they have no funding to gather their own [ 1 , 3 , 4 , 9 – 14 ].
However, in healthcare settings, qualitative researchers often investigate sensitive or stigmatized issues with vulnerable participants [ 10 , 15 ]. Given that qualitative data are often sensitive, qualitative researchers have expressed concerns about informed consent, protecting confidentiality, maintaining trust and relationships, and ensuring appropriate secondary uses of data, if data were shared [ 1 – 3 , 10 , 16 , 17 ]. Some argue that the information disclosed by participants is only made possible because of the trusting relationship between researcher and participant [ 1 , 18 , 19 ]. Researchers fear that sharing qualitative data could undermine this trust and that participants may be prevented from providing full and honest disclosure if they know data are going to be shared. In addition, participants may consent to have their data interpreted for one purpose, not for secondary purposes by a different researcher.
Qualitative data are non-numeric, which poses an additional de-identification challenge because identifiers may be located anywhere within long passages of narrative text [ 20 ]. Currently, researchers must manually search for and locate identifiers within qualitative data during data cleaning and analyses, but the process is labor intensive and we lack tools to support researchers in this process. Adequate de-identification of qualitative data requires balancing the protection of individual identities while ensuring adequate contextual detail remains to enable secondary use. In concurrent work, we are developing automated support software to assist researchers in the de-identification process [ 21 ].
Data repositories can also help address researcher concerns about QDS. Repositories store, preserve, and manage data in a manner that enables data sharing, discovery, and citation [ 3 , 22 , 23 ]. Repositories are staffed with experts who can help with data curation, provide guidance on preparing data for deposit, and work with researchers to determine an appropriate level of restriction for their data, including restricted access and delayed access options for secondary users [ 24 ]. Secondary users of sensitive data typically sign a data use agreement, which stipulates that they will not attempt to identify participants and they must obtain Institutional Review Board (IRB) approval prior to receiving data [ 25 ]. The data use agreement is brokered by the repository.
When adopting a new and controversial practice, it is important to engage stakeholders to understand the facilitators and barriers to uptake, and to promote stakeholder buy-in for the new practice [ 26 , 27 ]. In this article, we report findings from a survey of qualitative researchers regarding their experience and attitudes toward sharing health related or sensitive qualitative data in a repository where other researchers could access data. Qualitative data are gathered in diverse fields, including public health, social work, anthropology, medicine, occupational and physical therapy, nursing, bioethics, psychology, and clinical research [ 28 ]. Hence, we recruited broadly across health-related fields to ensure broad representation from qualitative researchers. We aimed to identify researchers’ top concerns, and factors that might increase their willingness to share.
The survey is part of a larger project to identify and overcome practical and ethical barriers to QDS in the U.S. (R01HG009351-01A1). In the next phase of our research, we will conduct a formative evaluation trial with 30 qualitative researchers that involves using our newly developed de-identification support software and guidelines prior to depositing their qualitative research data set with our partner repository, the Inter-university Consortium for Political and Social Research (ICPSR) at the University of Michigan. All survey participants were invited to take part in the formative evaluation QDS pilot at the end of the survey. The survey aimed to answer four research questions:
- RQ #1: How supportive are qualitative researchers of sharing qualitative data with a repository?
- RQ #2: What exploratory variables are associated with overall attitudes toward qualitative data sharing?
- RQ #3: What are the most common concerns that qualitative researchers have about sharing their qualitative data with a repository?
- RQ#4: What are the most common considerations and resources that would make qualitative researchers more willing to share their qualitative data with a repository?
Materials and methods
Survey development.
Prior to survey development, formative in-depth interviews were conducted with 30 qualitative researchers to inform survey content [ 2 ]. During these interviews, researchers were asked about the practical and ethical barriers and facilitators of sharing qualitative data with a repository. Transcripts from these interviews were coded for perceived barriers and concerns, as well as perceived benefits and facilitators of sharing qualitative data. Interviewees expressed a wide range of concerns and identified several benefits of sharing qualitative data with a repository. These data and prior literature on QDS guided the development of the survey items [ 1 , 14 , 19 , 29 – 31 ].
Recruitment
This study used a non-probability, criterion-based sampling approach. A non-probability approach was necessary because there is no way to identify the number nor identity of all qualitative researchers. Criterion-based sampling was used to target appropriate informants: Individuals who conduct qualitative research in the U.S. We restricted our focus to the U.S. because regulations, oversight policies, and data sharing practices might all affect attitudes, and these vary across nations.
Qualitative researchers were contacted via email through a range of recruitment mediums. We identified publicly available contact information of investigators through NIH RePORTER by using the search terms ‘qualitative,’ ‘interview,’ and ‘focus group.’ We also searched academic institution websites and recruited through the listservs of professional organizations for qualitative researchers in general (e.g., Society for Medical Anthropology). To ensure adequate representation from minority groups, we recruited through professional organizations for researchers from minority communities (e.g., Robert Wood Johnson Foundation New Connections Network, Brothers of the Academy, Sisters of the Academy, Latina Researchers Network) [ 32 ]. We also used a word of mouth approach, in which we contacted colleagues through our professional networks to request they send the recruitment email to potential qualitative researchers.
An email was distributed to qualitative researchers with a request to take the survey via an anonymous survey link. We asked professional organizations and academic institutions to send the link through their listservs and post the study information and anonymous survey link on their social media accounts (e.g., Twitter or Facebook). The survey was administered using Qualtrics survey software, which is password and firewall protected. The research was approved as exempt by the Institutional Review Board at Washington University (IRB#201811123). Participants who completed the survey were entered into a raffle to win one of ten cash prizes worth $100 for participating.
Data analysis
Data were analyzed using Stata statistical software (version 16.0); statistical significance is assessed as p<0.05. Bivariate analyses were conducted using Pearson’s chi-squared test to explore which demographic variables are associated with overall attitudes toward QDS within this sample.
Of the 676 respondents who initiated the web-based survey, 251 were excluded from analyses for not meeting study criteria (i.e., participants had not led and conducted qualitative research with human subjects, participants did not work at an institution in the U.S.) or did not complete more than 50% of the survey, which was forced-choice to avoid the problem of missing data. The final sample comprised of 425 qualitative researchers in the U.S. from a variety of academic disciplines including: public health, bioethics, and clinical fields (i.e., medicine, nursing, occupational and physical therapy) (n = 152, 38%); anthropology and sociology (n = 133, 33%); and other disciplines (n = 118, 29%). Table 1 presents the demographic information describing the sample. The majority of participants were female (n = 324, 76%), White (n = 242, 57%), and between 30–49 years old (n = 260, 61%).
*Question only applies to participants who have shared qualitative data previously ( N = 85).
Most qualitative researchers indicated they had been conducting qualitative research for more than 10 years (n = 235, 55%) and collect health information (n = 301, 71%) and other sensitive information (n = 206, 48%) in their qualitative research. Participants reported conducting research with various populations, including pregnant women (n = 85, 20%), children (n = 130, 31%), prisoners (n = 13, 3%), sexual minorities (n = 101, 24%), individuals with cognitive impairments (n = 47, 11%), those older than 65 (n = 144, 34%), patients (n = 203, 48%), racial and ethnic minorities (n = 333, 78%), economically disadvantaged people (n = 286, 67%), and individuals with sensitive diagnoses (n = 135, 32%). A variety of qualitative methods were used by respondents, the most common being interviews (n = 415, 98%) and focus groups (n = 321, 76%). Their work has been funded by government agencies (n = 276, 65%), private foundations (n = 202, 48%), corporations (n = 22, 5%), and investigator’s institutions (n = 314, 74%).
Experience and overall attitudes toward qualitative data sharing
The vast majority of researchers (n = 410, 96%) have never shared qualitative data in a repository. Qualitative researchers were asked to rate on a seven-point Likert scale the extent to which they oppose or support sharing qualitative research data (1 = strongly oppose, 7 = strongly support). Attitudes about sharing qualitative data were mixed. Specifically, 41% (n = 174) of participants oppose sharing qualitative data (including strongly oppose and slightly oppose), and 49% (n = 208) of participants support QDS (including strongly support and slightly support), indicating a bimodal distribution of attitudes toward sharing qualitative data with a repository.
Participants’ field of study was significantly related to attitudes toward QDS, such that those who conducted research in public health, clinical fields (e.g., nursing and medicine), and bioethics were more likely to support sharing qualitative data ( M = 4.52, SE = .16) than those who conducted research in disciplines of anthropology or sociology ( M = 3.58, SE = .17). Participant race was significantly associated with attitudes toward QDS. Hispanic ( M = 4.40, SE = .32) and Asian ( M = 4.48, SE = .39) qualitative researchers tended to be more supportive of sharing qualitative data compared to their White ( M = 4.16, SE = .13) and Black ( M = 3.94, SE = .22) qualitative researcher counterparts.
Age was associated with opposition to sharing qualitative data with a repository. Generally, younger qualitative researchers (e.g., age 20–29, M = 4.00, SE = .38; age 30–39, M = 4.08, SE = .16) tended to be less supportive of sharing qualitative data than qualitative researchers who were older (e.g., age 50–59, M = 4.21, SE = .22; 60+, M = 4.39, SE = .26). Finally, participant sex was associated with attitudes toward sharing qualitative data, such that males ( M = 4.81, SE = .21) were more supportive of sharing qualitative data than females ( M = 4.04, SE = .11).
Interest in qualitative data sharing pilot study
All survey participants were asked if they were interested in participating in our pilot study that involves using newly created de-identification support software on a qualitative data set prior to deposit in a data repository. Here, we treat interest in participating in the pilot as a measure of researcher willingness to share qualitative data. Out of 425 qualitative researchers, 134 (32%) expressed interest in participating in the pilot. Bivariate analyses indicate that collecting sensitive qualitative data ( p = 0.046), the sex of the researcher ( p = 0.006), and prior sharing experience ( p = 0.019) are significantly associated with interest in participating in the pilot. Of those researchers who gather sensitive information (n = 206), 36% (n = 74) expressed interest in the pilot compared to 27% (n = 60) of those who do not gather sensitive information (n = 219). Men (n = 33, 45%) were more likely to express interest in participating in the pilot study compared to women (n = 98, 30%). Participants who reported sharing qualitative research data with people outside of their research team in the past (n = 85) were more likely to be interested in participating in the pilot study (n = 36, 42%) compared to those who have not shared data previously (n = 98, 29%).
Concerns regarding qualitative data sharing
Qualitative researchers were asked how concerned they were about various factors related to sharing their qualitative data through a repository, on a scale of 1 (not at all concerned) to 5 (extremely concerned). Table 2 presents the frequencies of these concerns. Researchers’ greatest concerns (rated item a 3 or above) included that they lack participant permission (n = 370, 87%), data sensitivity (360, 85%), concerns about breaching participant trust (n = 349, 82%), IRB or institutional policies (n = 336, 79%), and inability to adequately de-identify data (n = 334, 79%).
Items were rated on a scale of 1 (not at all concerned), 2 (slightly concerned), 3 (moderately concerned), 4 (very concerned), or 5 (extremely concerned). Participants were considered to be concerned if they rated 3–5.
Facilitators to qualitative data sharing
Participants were asked how likely certain considerations would increase their willingness to share qualitative data through a repository, on a scale of 1 (not at all likely) to 5 (very likely). Table 3 presents the frequencies of these considerations (rated item a 4 or above). Researchers indicated that they would be most likely to share their qualitative data if doing so increased the societal impact of their research (n = 353, 83%), if participants agreed to have their data shared (n = 339, 80%), and if sharing led to increased future collaborations (n = 322, 76%).
The degree to which each consideration would increase willingness to share qualitative data were rated on a scale of 1 (not at all likely), 2 (somewhat unlikely), 3 (neutral), 4 (somewhat likely), or 5 (very likely). Participants were considered willing to share if they rated 4 or 5.
Resources to facilitate qualitative data sharing
Qualitative Researchers were asked to rate, on a scale of 1 (not at all) to 5 (a great deal), how much certain resources would increase their willingness to share their qualitative data through a repository. Table 4 presents the frequencies of these resources (rated item a 4 or above). Participants indicated that they would be more willing to share their data if repository costs were covered by funding agencies (n = 294, 69%), if they received clear guidance on ethics and compliance-related issues (n = 259, 61%), if repositories assisted with data anonymization (n = 243, 57%), and if repositories provided consultations on sharing qualitative data (n = 207, 49%).
The degree to which each resource would increase willingness to share qualitative data were rated on a scale of 1 (not at all), 2 (a little), 3 (a moderate amount), 4 (a lot) or 5 (a great deal). Participants were considered willing to share if they rated a 4 or 5.
Findings from the current study indicate that QDS in the U.S. remains rare, with only 4% of qualitative researchers having ever shared qualitative data in a repository. While nearly half of researchers expressed support for QDS, most researchers are not actually sharing qualitative data currently. These findings, although focused on qualitative researchers in the U.S., have implications for qualitative researchers more broadly given the international shift towards data sharing and open science, including qualitative data, which has historically not been shared.
Limitations
We used a criterion-based sampling approach which limits the generalizability of our findings. This non-probability approach was necessary because there is no way to identify all qualitative researchers, so our approach was to target appropriate informants. When individuals completed the entire survey, we had no missing data from them because we used forced choice; however, some individuals chose not to complete the survey after establishing eligibility. We do not know how those who completed the survey differ from non-responders. In addition, we restricted data collection to US qualitative researchers so our findings may not generalize to other national contexts with different legal and regulatory frameworks. Finally, we conducted analyses on the association of demographics (e.g., age, sex, and field of study) with attitudes toward data sharing and willingness to participate in our data sharing pilot project. These associations are “within sample” associations and should be interpreted as such.
Resources and guidance needed
Clear and transparent consent forms.
Researchers’ top concerns related to obtaining informed consent, ensuring participants agreed, and not breaching trust. Notably, in concurrent work, we conducted qualitative interviews with 30 qualitative research participants and found the majority supported QDS and some assumed data sharing was already happening [ 8 ]. Participants were broadly supportive of QDS so long as confidentiality was maintained and data were shared with other researchers. Going forward, qualitative researchers must ensure clear and transparent informed consent that communicates data sharing plans at the outset of a study as this could significantly facilitate QDS, and be acceptable to participants. Historically, qualitative researchers often promise in informed consent documents to destroy data when the research ends or that no one outside the research team will ever access data. These statements prohibit data sharing from the outset. While such statements may be appropriate in some cases where data are too sensitive to share or cannot be de-identified adequately, in many cases clear and transparent consent forms that obtain permission for data sharing will enable QDS going forward. We believe it is appropriate for consent forms to specifically disclose that secondary analyses may explore new research questions [ 33 ]. Importantly, such broad statements would pertain to secondary analyses conducted by third parties on shared data as well as analyses on new research questions conducted by the original investigators, which is the most common form of “secondary analyses” currently [ 33 ].
In addition, consent forms will need to specifically disclose that secondary analyses may be conducted once data is shared, and that such analyses may explore new or different research questions than originally planned [ 33 ]. A recent review of qualitative secondary analyses found that the majority were conducted by the original investigators involved in the parent study, primarily to explore new questions on a subset of existing data, and there was a lack of clarity when reporting on whether these analyses were an extension of the primary analyses or a secondary analyses [ 33 ]. Informed consent documents need to include a clearer differentiation of primary and secondary analyses, including that secondary analyses could explore topics entirely unrelated to the primary study [ 33 ].
Repositories
Qualitative researchers expressed concerns regarding losing control of who accesses data (77%), financial costs of preparing data (67%), concerns about potential repository technological failures (55%), and lack of knowledge of repositories and QDS in general (52%). At the same time, 66% of researchers indicated they would be more willing to share if repositories provided a secure infrastructure for data storage. We encourage researchers to explore available repositories, whether institutional or national, as appropriate repositories can provide the necessary tools and guidance to facilitate QDS such as archiving data securely (and in perpetuity) and restricting secondary users’ access to data. Restricted access, rather than public access, is likely appropriate for most types of sensitive qualitative health data. In the U.S., funders such as the NIH allow data sharing costs to be included in budgets, and researchers should confirm with their funders as QDS may be an allowable cost [ 5 ].
Assistance with de-identification
The majority of qualitative researchers (79%) expressed concerns that qualitative data cannot be adequately de-identified, and 59% reported that resources to assist with de-identification would enhance their willingness for QDS. Currently researchers must manually sift through data to look for and remove potential identifiers, which is labor intensive. In addition, there are no standards specific to qualitative data to determine when it is adequately de-identified. In concurrent work, we are developing automated software to assist qualitative researchers de-identifying qualitative data [ 21 ]. Such automated tools will facilitate de-identification prior to data sharing, although researcher input is still required to verify that data are adequately de-identified. It is also essential that data retain adequate contextual details to enable secondary users to interpret the data. Repositories can provide guidance on the necessary accompanying documentation and contextual data to enable secondary use.
Factors associated with willingness to share: An area for future research
Our data indicate that public health, clinical health, and bioethics researchers are more open to QDS than researchers from other fields such as anthropology and sociology. This may be partially due to the common use in anthropology and sociology of ethnographic methods such as participant observation that require detailed, and often deeply personal, field notes. These data may be especially difficult to de-identify and present greater challenges for sharing than a one-time qualitative interview conducted in a public health or medical research setting. Alternately, this may reflect the cultures in which public health, medical, and nursing researchers work and their funding sources [ 9 ]. In contrast to researchers in disciplines like anthropology who may return to field notes throughout their careers for analyses, researchers in medicine, nursing, and public health are more likely to conduct contract funded research with a clear end date [ 9 ] and funders may also require data sharing. These qualitative researchers often have data that may not have been ‘fully mined’ by the time research funding ends, creating opportunities for further analyses [ 9 ]. Biomedical and public health researchers may be prime candidates for championing and helping to normalize QDS.
Qualitative researchers’ age, sex, and race were associated with attitudes toward QDS in our sample, with men, those who are older in age, and Asians and Hispanics being more supportive of QDS compared to their counterparts. Given that our survey was not designed to determine why these factors are associated with attitudes towards sharing, future research is needed to better understand whether, how, and why individual factors may influence willingness to share. Future research should also examine what resources could help overcome barriers to sharing qualitative data with a repository outside of the U.S., as barriers will likely differ by national context.
QDS is feasible and can improve healthcare
Qualitative data are often sensitive, provide rich information, and seek to explore complex inquiries not adequately addressed using quantitative methods. Qualitative insights have changed healthcare and practice, suggesting there is much unrealized potential if more qualitative data were shared. For instance, a systematic review of 77 original qualitative studies on a form of chronic pain led to a new understanding of pain as an ‘adversarial struggle’, illustrating that a central component of therapy is that patients must feel recognized and heard by physicians [ 13 ]. At the same time, the existence of nearly 80 studies on a similar topic suggests that qualitative research may at times be wasteful or duplicative; an avoidable occurrence if qualitative data were shared more broadly [ 11 ].
QDS has the potential to improve transparency, promote secondary data analysis, and facilitate research training, but researcher attitudes and behaviors need to change. In fact, researchers cited increasing the societal impact of their work and future collaborations as key factors that would increase their willingness to share. Realizing such goals will require actually changing behavior and long-held attitudes about QDS. The UK illustrates this potential for change. There has been a slow but steady rise in qualitative data sharing as a result of the open data movement, funding policies, changing attitudes, and the availability of practical procedures and ‘mature infrastructure’ through the UK Data Archive [ 3 ]. An analysis of 267 data sets in the UK Data Archive (not necessarily health related) indicates there were 7,155 unique downloads of these data sets. Data were primarily used for learning (64%), research (15%), and teaching (13%) and demonstrate the ‘scale and significance of the reuse of data for teaching and learning’[ 3 ].
Our current project aims to provide the necessary support and resources to facilitate QDS in the U.S., including developing a software to support the de-identification of qualitative data, and a QDS Toolkit containing guidance and materials. We are engaging diverse stakeholders to identify concerns and needs, develop and evaluate the Toolkit, and will disseminate the Toolkit to strategic groups while evaluating its adoption. At the end of the project, the Toolkit—including the software—will be made available to support data sharing in an ethical manner in the U.S.
The NIH is moving ‘toward a future in which data sharing is a community norm’[ 5 ], including sharing de-identified qualitative health data, and other funding agencies may soon follow suit. It is imperative that qualitative researchers increase their knowledge of how to share their qualitative research data responsibly. Widespread, responsible sharing of qualitative data can have a lasting positive impact on health knowledge and interventions [ 9 , 11 – 13 , 34 , 35 ]. Systematic guidelines and support for responsible and ethical QDS are needed to realize the potential benefits while protecting confidentiality and maintaining trust among research participants and the research community. Some data, if released and not shared responsibly, could present real harm to participants. However, responsible sharing of qualitative health data is possible and would maximize the value and use of data for health, social science, and policymaking.
Supporting information
Acknowledgments.
The authors thank all participants who completed the survey and Ruby Varghese for her assistance with data collection.
Funding Statement
This project is funded by the National Human Genome Research Institute (NHGRI https://www.genome.gov ) R01HG009351 (PI DuBois) (JM, TM, HW, MP, MG, JD) and the National Center for Advancing Translational Sciences (UL1TR002345 https://ncats.nih.gov ) (JM, TM, MP, JD). The funders had no role in study design, data collection and analysis, decision to publish, or preparation of the manuscript.
Data Availability

IMAGES
VIDEO
COMMENTS
SDA involves investigations where data collected for a previous study is analyzed - either by the same researcher(s) or different researcher(s) - to explore new questions or use different analysis strategies that were not a part of the primary analysis (Szabo and Strang, 1997).For research involving quantitative data, SDA, and the process of sharing data for the purpose of SDA, has become ...
Concerns about secondary data analysis when using qualitative data. The primary concerns about SDA with qualitative data surround rigor and ethics from a number of stakeholder perspectives, including research participants, funders, and the researchers themselves. Heaton (2004) suggests that a strength of secondary analysis of qualitative data ...
Qualitative secondary analysis (QSA) is the use of qualitative data collected by someone else or to answer a different research question. Secondary analysis of qualitative data provides an opportunity to maximize data utility particularly with difficult to reach patient populations. However, QSA methods require careful consideration and ...
increased, little is known about the current state of qualitative secondary data analysis or how researchers are conducting secondary data analysis with qualitative data. This critical interpretive synthesis examined research articles (n¼71) published between 2006 and 2016 that involved qualitative secondary data analysis and assessed the
The researcher must have access to source data, as opposed to secondary source data (e.g., a medical record review). Original qualitative data sources could be videotaped or audiotaped interviews or transcripts, or other notes from a qualitative study (Rew, Koniak-Griffin, Lewis, Miles, & O'Sullivan, 2000). Another possible source for ...
Qualitative secondary analysis (QSA) is the use of qualitative data that was collected by someone else or was collected to answer a different research question. Secondary analysis of qualitative data provides an opportunity to maximize data utility, particularly with difficult-to-reach patient populations. However, qualitative secondary ...
2.1 Re-use of pre-existing research data. Secondary analysis involves the re-use of pre-existing qualitative data derived. from previous research studies. These data include material such as semi. structured interviews, responses to open-ended questions in questionnaires, field notes and research diaries.
Secondary data analysis is a valuable research approach that can be used to advance knowledge across many disciplines through the use of quantitative, qualitative, or mixed methods data to answer new research questions ( Polit & Beck, 2021 ). This research method dates to the 1960s and involves the utilization of existing or primary data ...
Qualitative secondary analysis (QSA) is the use of qualitative data that was collected by someone else or was collected to answer a different research question. Secondary analysis of qualitative data provides an opportunity to maximize data utility, particularly with difficult-to-reach patient populations.
1. Introduction. The analysis of qualitative data is often the most complex stage of the research process given that there is no universal procedure that applies to every situation (Turcotte, Dufour, & Saint-Jacques, Citation 2009).Depending on the context, the purpose of the study, or the researcher's epistemological stance, it can be complex to select the most appropriate approach for ...
Qualitative Research (General) | Secondary Data Analysis. April 2019 | 368 pages | SAGE Publications Ltd. Download flyer. Description. Contents. Reviews. Preview. Using secondary data offers unique opportunities and challenges. This practical book will guide you through finding, managing and analysing qualitative secondary data in an error-free ...
Qualitative Research, Secondary Analysis, Online Research Data . Introduction . Quantitative researchers regularly conduct and publish results of secondary analyses of existing datasets including the National Health and Nutrition Examination Survey (NHANES) and the Behavior Risk Factor Surveillance System (BRFSS). Reports based on results of
When to use secondary research. Secondary research is a very common research method, used in lieu of collecting your own primary data. It is often used in research designs or as a way to start your research process if you plan to conduct primary research later on.. Since it is often inexpensive or free to access, secondary research is a low-stakes way to determine if further primary research ...
School of Social Work, Wa yne State University, USA. Abstract. While secondary data analysis of quantitative data has become commonplace and encouraged. across disciplines, the practice of ...
methods and findings from existing qualitative research in an attempt to generate and synthesise meanings from multiple studies, for example, the meta-study of qualitative data (Paterson et al., 2001), meta-ethnography (Noblit and Hare, 1988), meta-sociology (Furfey, 1953) and meta-study (Zhao, 1991), as the aim of a secondary analysis is to ...
Secondary research, also known as desk research, is a research method that involves compiling existing data sourced from a variety of channels. This includes internal sources (e.g.in-house research) or, more commonly, external sources (such as government statistics, organizational bodies, and the internet).
Types of secondary data are as follows: Published data: Published data refers to data that has been published in books, magazines, newspapers, and other print media. Examples include statistical reports, market research reports, and scholarly articles. Government data: Government data refers to data collected by government agencies and departments.
The qualitative PE for the HERO trial employed a qualitative mixed methods approach [] including a variety of data collection methods such as non-participant observations, semi-structured interviews, and documentary analysis.The data for this paper was obtained from the interviews with participants as the focus is on individual's own perception of their frailty.
Secondary data analysis. Secondary analysis refers to the use of existing research data to find answer to a question that was different from the original work ( 2 ). Secondary data can be large scale surveys or data collected as part of personal research. Although there is general agreement about sharing the results of large scale surveys, but ...
To foster development of best practices for secondary use of data from EHR and other sources for research, the CTSA consortium's Informatics Enterprise Committee (iEC) formed the Enterprise Data Warehouse for Research Working Group (EDW4R WG) with leadership from 2 co-authors (B.M.K. and T.R.C.). ... In this qualitative study, informatics ...
A qualitative descriptive research methodology was chosen to explore the perspectives on challenges that residents face with regard to care coordination for complex patients. This methodology allows investigators to remain close to the data to obtain an accurate and detailed representation of the residents' experiences and perceptions [ 13 ].
Introduction. Qualitative health data—such as data from interviews or focus groups—are rarely shared in the United States (U.S.) [1 p.161, 2].This is unfortunate because qualitative data are labor and time-intensive to gather, and data sharing would enable secondary research, enhance training, and increase transparency.
This study examined secondary school science teachers' conceptions of the nature of scientific knowledge (NOSK). The participants were convenient samples consisting of 48 (M = 38, F = 10) teachers from three secondary schools. An open-ended questionnaire was administered to collect data. Qualitative analysis involved comparing teachers' responses to experts' views on NOSK.