- See us on facebook
- See us on twitter
- See us on youtube
- See us on linkedin
- See us on instagram

Experimental depression treatment is nearly 80% effective in controlled study
In a double-blind controlled study, high doses of magnetic brain stimulation, given on an accelerated timeline and individually targeted, caused remission in 79% of trial participants with severe depression.
October 28, 2021 - By Mandy Erickson

Since receiving an experimental depression treatment at Stanford, Tommy Van Brocklin has been walking Scout for "the sheer joy of it." Nellie Van Brocklin
A new type of magnetic brain stimulation brought rapid remission to almost 80% of participants with severe depression in a study conducted at the Stanford University School of Medicine .
The treatment, known as Stanford accelerated intelligent neuromodulation therapy (SAINT) or simply Stanford neuromodulation therapy, is an intensive, individualized form of transcranial magnetic stimulation. In the study, remission typically occurred within days and lasted months. The only side effects were temporary fatigue and headaches.
“It works well, it works quickly and it’s noninvasive,” said Nolan Williams , MD, an assistant professor of psychiatry and behavioral sciences. “It could be a game changer.” Williams is the senior author of the study, which was published Oct. 29 in the American Journal of Psychiatry .
Twenty-nine people with treatment-resistant depression participated in the study: About half received SAINT, and the rest underwent a placebo procedure that mimicked the real treatment. After five days of treatment, 78.6% of the participants in the treatment group were no longer depressed, according to several standard methods of evaluation. “It’s quite a dramatic effect, and it’s quite sustained,” said Alan Schatzberg , MD, the Kenneth T. Norris, Jr. Professor in Psychiatry and Behavioral Sciences, who was a co-author of the study.
A lifetime of depression
Tommy Van Brocklin, 60, has suffered from depression since he was 15. “In 1975, they didn’t have the medication and understanding they do now,” he said. “I was told I wasn’t trying hard enough.”
“I’ve functioned all these years, but it’s been very difficult at times,” the civil engineer added. Talk therapy helped “for about half a day after an appointment.” When selective serotonin reuptake inhibitors became available in the 1990s, he started on paroxetine, commonly sold under the brand name Paxil.
“It worked like a miracle drug,” he said, but after 10 or 15 years it started to lose its effect. After 25 years, it stopped working entirely. He tried other medications, but none helped; one even made him suicidal.
His sister, who lives near Stanford, connected him with the researchers studying SAINT. He flew from his home in Memphis, Tennessee, and underwent the treatment in September. He felt nothing the first day; on day two, he began feeling emotional — “I felt the struggle of what I’d been through all these years.”
“The next day, all of a sudden, it broke through,” he said. “I felt so much better, and it’s stuck with me.”
Specialized magnetic stimulation
The transcranial magnetic stimulation treatment currently approved by the Food and Drug Administration requires six weeks of once-daily sessions. Only about half of patients who undergo the treatment improve, and only about a third experience remission from depression.
SAINT advances that treatment by targeting the magnetic pulses according to each patient’s neurocircuitry and providing a greater number of pulses at a faster pace.
In the study, the researchers first used MRI to locate the best location to target within each participant’s dorsolateral prefrontal cortex, which regulates executive functions, such as problem solving and inhibiting unwanted responses. They applied the stimulation in a subregion that has the strongest relationship with the subgenual cingulate, a part of the brain that is overactive in people experiencing depression. The transcranial magnetic stimulation strengthens the connection between the two regions, facilitating dorsolateral prefrontal cortex control of the activity in the subgenual cingulate.
The researchers also used 1,800 pulses per session instead of 600. (The larger amount has been used safely in other forms of brain stimulation for neurological disorders such as Parkinson’s disease.) And instead of providing one treatment a day, they gave participants 10 10-minute treatments, with 50-minute breaks in between.
For the control group, the researchers disguised the treatment with a magnetic coil that mimicked the experience of the magnetic pulse; both the control and active treatment groups wore noise-canceling earphones and received a topical ointment to dull sensation. Neither the researcher administering the procedure nor the participant knew whether the participant was receiving real treatment.
A hard-to-treat group
The trial participants ranged in age from 22 to 80; on average, they had suffered depression for nine years. They had tried medications, but either they had had no effect or they had stopped working. During the trial, participants who were on medication maintained their regular dosage; participants who weren’t taking medications did not start any.

Nolan Williams demonstrates SAINT, the magnetic brain stimulation therapy he and his colleagues developed, on Deirdre Lehman, a participant in a previous study of the treatment. Steve Fisch
Within four weeks after treatment, 12 of the 14 participants who had received the treatment improved, and 11 of them met FDA criteria for remission. In contrast, only two of the 15 participants who had received the placebo met the criteria for remission.
Because the study participants typically felt better within days of starting SAINT, the researchers are hoping it can be used to quickly treat patients who are at a crisis point. Patients who start taking medication for depression typically don’t experience any reduction of symptoms for a month.
“We want to get this into emergency departments and psychiatric wards where we can treat people who are in a psychiatric emergency,” Williams said. “The period right after hospitalization is when there’s the highest risk of suicide.”
Van Brocklin said that since he returned home following treatment, he’s made some radical changes. “I have a really strong desire to get my life together,” he said.
“I don’t procrastinate anymore,” he added. “I’m sleeping better. I completely quit alcohol. I’m walking my dog and playing the guitar again, for nothing more than the sheer joy of it.”
Most importantly, he said, “I’m remaining positive and being respectful of others. These are big changes in my life.”
Other Stanford scientists who contributed to the study are former postdoctoral scholars Eleanor Cole, PhD, and Angela Phillips, PhD; Brandon Bentzley, MD, PhD, David Carreon, MD, Jennifer Keller, PhD, Kristin Raj, MD, and Flint Espil, PhD, all clinical assistant professors of psychiatry and behavioral sciences; clinical research coordinators Katy Stimpson, Romina Nejad, Clive Veerapal, Nicole Odenwald and Maureen Chang; former clinical research coordinators Fahim Barmak, MD, Naushaba Khan and Rachel Rapier; postdoctoral scholars Kirsten Cherian, PhD, James Bishop, PhD, Azeezat Azeez, PhD, and John Coetzee, PhD; life science research professional Heather Pankow; clinical research manager Jessica Hawkins; Charles DeBattista, MD, professor of psychiatry and behavioral sciences; and Booil Jo, PhD, associate professor of psychiatry and behavioral sciences.
Scientists from the U.S. Department of Veterans Affairs; Palo Alto University; the Centre for Neuroimaging and Cognitive Genomics at the National University of Ireland; and the School of Medicine at Southern Illinois University, Carbondale, contributed to the research.
The research was funded by a Brain and Behavior Research Foundation Young Investigator Award, Charles R. Schwab, the David and Amanda Chao Fund II, the Amy Roth PhD Fund, the Neuromodulation Research Fund, the Lehman Family, the Still Charitable Trust, the Marshall and Dee Ann Payne Fund, and the Gordie Brookstone Fund.
Stanford’s Department of Psychiatry and Behavioral Sciences also contributed to the work.
If you're interested in participating in a study, please email [email protected] .
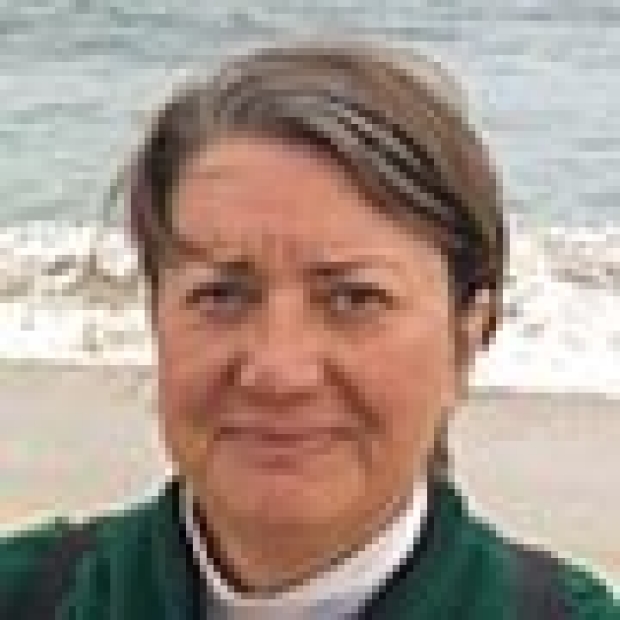
About Stanford Medicine
Stanford Medicine is an integrated academic health system comprising the Stanford School of Medicine and adult and pediatric health care delivery systems. Together, they harness the full potential of biomedicine through collaborative research, education and clinical care for patients. For more information, please visit med.stanford.edu .
Hope amid crisis
Psychiatry’s new frontiers
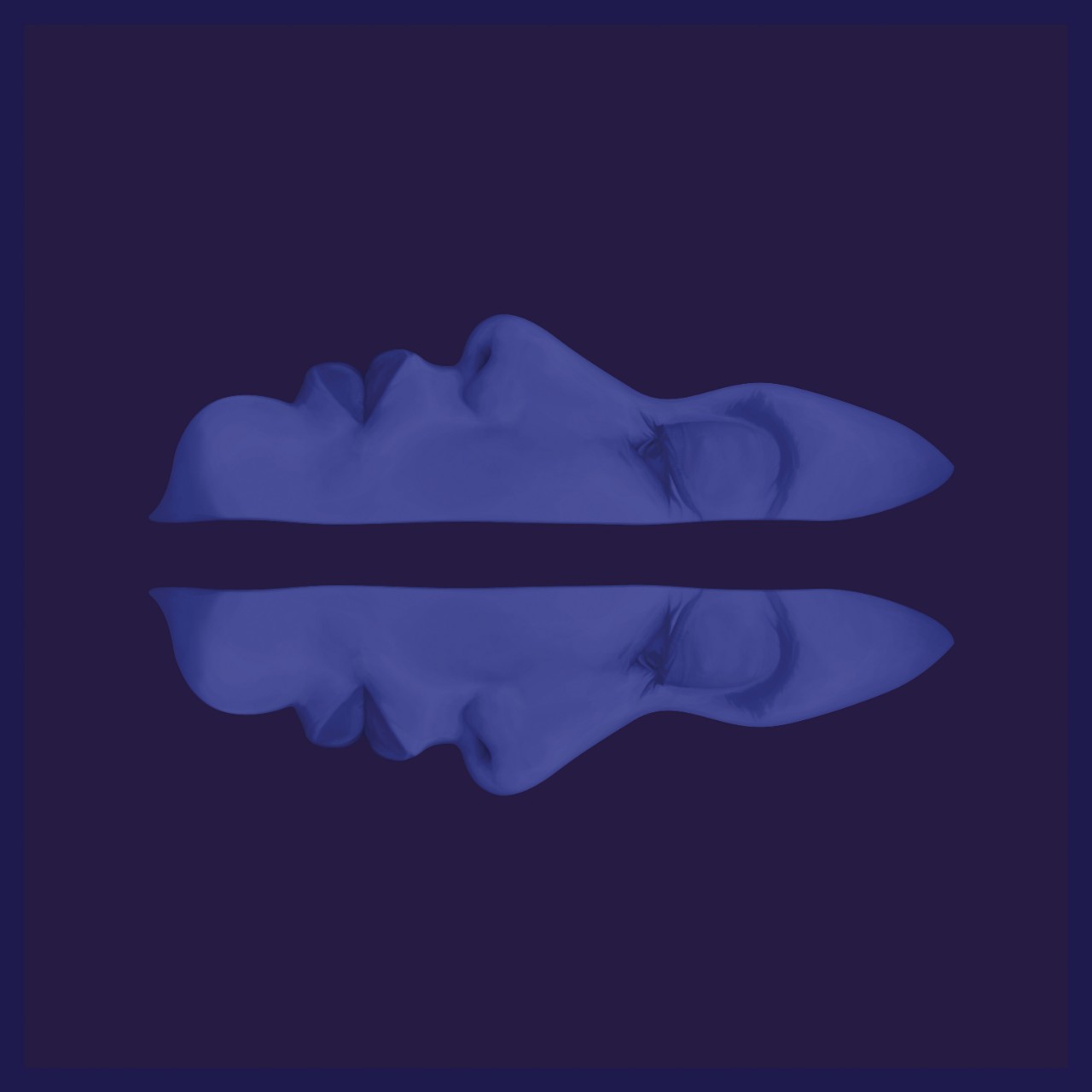
Click through the PLOS taxonomy to find articles in your field.
For more information about PLOS Subject Areas, click here .
Loading metrics
Open Access
Peer-reviewed
Research Article
Recent quantitative research on determinants of health in high income countries: A scoping review
Roles Conceptualization, Data curation, Formal analysis, Investigation, Methodology, Project administration, Software, Visualization, Writing – original draft, Writing – review & editing
* E-mail: [email protected]
Affiliation Centre for Health Economics Research and Modelling Infectious Diseases, Vaccine and Infectious Disease Institute, University of Antwerp, Antwerp, Belgium

Roles Conceptualization, Data curation, Funding acquisition, Project administration, Resources, Supervision, Validation, Visualization, Writing – review & editing
- Vladimira Varbanova,
- Philippe Beutels
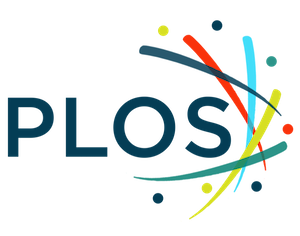
- Published: September 17, 2020
- https://doi.org/10.1371/journal.pone.0239031
- Peer Review
- Reader Comments
Identifying determinants of health and understanding their role in health production constitutes an important research theme. We aimed to document the state of recent multi-country research on this theme in the literature.
We followed the PRISMA-ScR guidelines to systematically identify, triage and review literature (January 2013—July 2019). We searched for studies that performed cross-national statistical analyses aiming to evaluate the impact of one or more aggregate level determinants on one or more general population health outcomes in high-income countries. To assess in which combinations and to what extent individual (or thematically linked) determinants had been studied together, we performed multidimensional scaling and cluster analysis.
Sixty studies were selected, out of an original yield of 3686. Life-expectancy and overall mortality were the most widely used population health indicators, while determinants came from the areas of healthcare, culture, politics, socio-economics, environment, labor, fertility, demographics, life-style, and psychology. The family of regression models was the predominant statistical approach. Results from our multidimensional scaling showed that a relatively tight core of determinants have received much attention, as main covariates of interest or controls, whereas the majority of other determinants were studied in very limited contexts. We consider findings from these studies regarding the importance of any given health determinant inconclusive at present. Across a multitude of model specifications, different country samples, and varying time periods, effects fluctuated between statistically significant and not significant, and between beneficial and detrimental to health.
Conclusions
We conclude that efforts to understand the underlying mechanisms of population health are far from settled, and the present state of research on the topic leaves much to be desired. It is essential that future research considers multiple factors simultaneously and takes advantage of more sophisticated methodology with regards to quantifying health as well as analyzing determinants’ influence.
Citation: Varbanova V, Beutels P (2020) Recent quantitative research on determinants of health in high income countries: A scoping review. PLoS ONE 15(9): e0239031. https://doi.org/10.1371/journal.pone.0239031
Editor: Amir Radfar, University of Central Florida, UNITED STATES
Received: November 14, 2019; Accepted: August 28, 2020; Published: September 17, 2020
Copyright: © 2020 Varbanova, Beutels. This is an open access article distributed under the terms of the Creative Commons Attribution License , which permits unrestricted use, distribution, and reproduction in any medium, provided the original author and source are credited.
Data Availability: All relevant data are within the manuscript and its Supporting Information files.
Funding: This study (and VV) is funded by the Research Foundation Flanders ( https://www.fwo.be/en/ ), FWO project number G0D5917N, award obtained by PB. The funder had no role in study design, data collection and analysis, decision to publish, or preparation of the manuscript.
Competing interests: The authors have declared that no competing interests exist.
Introduction
Identifying the key drivers of population health is a core subject in public health and health economics research. Between-country comparative research on the topic is challenging. In order to be relevant for policy, it requires disentangling different interrelated drivers of “good health”, each having different degrees of importance in different contexts.
“Good health”–physical and psychological, subjective and objective–can be defined and measured using a variety of approaches, depending on which aspect of health is the focus. A major distinction can be made between health measurements at the individual level or some aggregate level, such as a neighborhood, a region or a country. In view of this, a great diversity of specific research topics exists on the drivers of what constitutes individual or aggregate “good health”, including those focusing on health inequalities, the gender gap in longevity, and regional mortality and longevity differences.
The current scoping review focuses on determinants of population health. Stated as such, this topic is quite broad. Indeed, we are interested in the very general question of what methods have been used to make the most of increasingly available region or country-specific databases to understand the drivers of population health through inter-country comparisons. Existing reviews indicate that researchers thus far tend to adopt a narrower focus. Usually, attention is given to only one health outcome at a time, with further geographical and/or population [ 1 , 2 ] restrictions. In some cases, the impact of one or more interventions is at the core of the review [ 3 – 7 ], while in others it is the relationship between health and just one particular predictor, e.g., income inequality, access to healthcare, government mechanisms [ 8 – 13 ]. Some relatively recent reviews on the subject of social determinants of health [ 4 – 6 , 14 – 17 ] have considered a number of indicators potentially influencing health as opposed to a single one. One review defines “social determinants” as “the social, economic, and political conditions that influence the health of individuals and populations” [ 17 ] while another refers even more broadly to “the factors apart from medical care” [ 15 ].
In the present work, we aimed to be more inclusive, setting no limitations on the nature of possible health correlates, as well as making use of a multitude of commonly accepted measures of general population health. The goal of this scoping review was to document the state of the art in the recent published literature on determinants of population health, with a particular focus on the types of determinants selected and the methodology used. In doing so, we also report the main characteristics of the results these studies found. The materials collected in this review are intended to inform our (and potentially other researchers’) future analyses on this topic. Since the production of health is subject to the law of diminishing marginal returns, we focused our review on those studies that included countries where a high standard of wealth has been achieved for some time, i.e., high-income countries belonging to the Organisation for Economic Co-operation and Development (OECD) or Europe. Adding similar reviews for other country income groups is of limited interest to the research we plan to do in this area.
In view of its focus on data and methods, rather than results, a formal protocol was not registered prior to undertaking this review, but the procedure followed the guidelines of the PRISMA statement for scoping reviews [ 18 ].
We focused on multi-country studies investigating the potential associations between any aggregate level (region/city/country) determinant and general measures of population health (e.g., life expectancy, mortality rate).
Within the query itself, we listed well-established population health indicators as well as the six world regions, as defined by the World Health Organization (WHO). We searched only in the publications’ titles in order to keep the number of hits manageable, and the ratio of broadly relevant abstracts over all abstracts in the order of magnitude of 10% (based on a series of time-focused trial runs). The search strategy was developed iteratively between the two authors and is presented in S1 Appendix . The search was performed by VV in PubMed and Web of Science on the 16 th of July, 2019, without any language restrictions, and with a start date set to the 1 st of January, 2013, as we were interested in the latest developments in this area of research.
Eligibility criteria
Records obtained via the search methods described above were screened independently by the two authors. Consistency between inclusion/exclusion decisions was approximately 90% and the 43 instances where uncertainty existed were judged through discussion. Articles were included subject to meeting the following requirements: (a) the paper was a full published report of an original empirical study investigating the impact of at least one aggregate level (city/region/country) factor on at least one health indicator (or self-reported health) of the general population (the only admissible “sub-populations” were those based on gender and/or age); (b) the study employed statistical techniques (calculating correlations, at the very least) and was not purely descriptive or theoretical in nature; (c) the analysis involved at least two countries or at least two regions or cities (or another aggregate level) in at least two different countries; (d) the health outcome was not differentiated according to some socio-economic factor and thus studied in terms of inequality (with the exception of gender and age differentiations); (e) mortality, in case it was one of the health indicators under investigation, was strictly “total” or “all-cause” (no cause-specific or determinant-attributable mortality).
Data extraction
The following pieces of information were extracted in an Excel table from the full text of each eligible study (primarily by VV, consulting with PB in case of doubt): health outcome(s), determinants, statistical methodology, level of analysis, results, type of data, data sources, time period, countries. The evidence is synthesized according to these extracted data (often directly reflected in the section headings), using a narrative form accompanied by a “summary-of-findings” table and a graph.
Search and selection
The initial yield contained 4583 records, reduced to 3686 after removal of duplicates ( Fig 1 ). Based on title and abstract screening, 3271 records were excluded because they focused on specific medical condition(s) or specific populations (based on morbidity or some other factor), dealt with intervention effectiveness, with theoretical or non-health related issues, or with animals or plants. Of the remaining 415 papers, roughly half were disqualified upon full-text consideration, mostly due to using an outcome not of interest to us (e.g., health inequality), measuring and analyzing determinants and outcomes exclusively at the individual level, performing analyses one country at a time, employing indices that are a mixture of both health indicators and health determinants, or not utilizing potential health determinants at all. After this second stage of the screening process, 202 papers were deemed eligible for inclusion. This group was further dichotomized according to level of economic development of the countries or regions under study, using membership of the OECD or Europe as a reference “cut-off” point. Sixty papers were judged to include high-income countries, and the remaining 142 included either low- or middle-income countries or a mix of both these levels of development. The rest of this report outlines findings in relation to high-income countries only, reflecting our own primary research interests. Nonetheless, we chose to report our search yield for the other income groups for two reasons. First, to gauge the relative interest in applied published research for these different income levels; and second, to enable other researchers with a focus on determinants of health in other countries to use the extraction we made here.
- PPT PowerPoint slide
- PNG larger image
- TIFF original image
https://doi.org/10.1371/journal.pone.0239031.g001
Health outcomes
The most frequent population health indicator, life expectancy (LE), was present in 24 of the 60 studies. Apart from “life expectancy at birth” (representing the average life-span a newborn is expected to have if current mortality rates remain constant), also called “period LE” by some [ 19 , 20 ], we encountered as well LE at 40 years of age [ 21 ], at 60 [ 22 ], and at 65 [ 21 , 23 , 24 ]. In two papers, the age-specificity of life expectancy (be it at birth or another age) was not stated [ 25 , 26 ].
Some studies considered male and female LE separately [ 21 , 24 , 25 , 27 – 33 ]. This consideration was also often observed with the second most commonly used health index [ 28 – 30 , 34 – 38 ]–termed “total”, or “overall”, or “all-cause”, mortality rate (MR)–included in 22 of the 60 studies. In addition to gender, this index was also sometimes broken down according to age group [ 30 , 39 , 40 ], as well as gender-age group [ 38 ].
While the majority of studies under review here focused on a single health indicator, 23 out of the 60 studies made use of multiple outcomes, although these outcomes were always considered one at a time, and sometimes not all of them fell within the scope of our review. An easily discernable group of indices that typically went together [ 25 , 37 , 41 ] was that of neonatal (deaths occurring within 28 days postpartum), perinatal (fetal or early neonatal / first-7-days deaths), and post-neonatal (deaths between the 29 th day and completion of one year of life) mortality. More often than not, these indices were also accompanied by “stand-alone” indicators, such as infant mortality (deaths within the first year of life; our third most common index found in 16 of the 60 studies), maternal mortality (deaths during pregnancy or within 42 days of termination of pregnancy), and child mortality rates. Child mortality has conventionally been defined as mortality within the first 5 years of life, thus often also called “under-5 mortality”. Nonetheless, Pritchard & Wallace used the term “child mortality” to denote deaths of children younger than 14 years [ 42 ].
As previously stated, inclusion criteria did allow for self-reported health status to be used as a general measure of population health. Within our final selection of studies, seven utilized some form of subjective health as an outcome variable [ 25 , 43 – 48 ]. Additionally, the Health Human Development Index [ 49 ], healthy life expectancy [ 50 ], old-age survival [ 51 ], potential years of life lost [ 52 ], and disability-adjusted life expectancy [ 25 ] were also used.
We note that while in most cases the indicators mentioned above (and/or the covariates considered, see below) were taken in their absolute or logarithmic form, as a—typically annual—number, sometimes they were used in the form of differences, change rates, averages over a given time period, or even z-scores of rankings [ 19 , 22 , 40 , 42 , 44 , 53 – 57 ].
Regions, countries, and populations
Despite our decision to confine this review to high-income countries, some variation in the countries and regions studied was still present. Selection seemed to be most often conditioned on the European Union, or the European continent more generally, and the Organisation of Economic Co-operation and Development (OECD), though, typically, not all member nations–based on the instances where these were also explicitly listed—were included in a given study. Some of the stated reasons for omitting certain nations included data unavailability [ 30 , 45 , 54 ] or inconsistency [ 20 , 58 ], Gross Domestic Product (GDP) too low [ 40 ], differences in economic development and political stability with the rest of the sampled countries [ 59 ], and national population too small [ 24 , 40 ]. On the other hand, the rationales for selecting a group of countries included having similar above-average infant mortality [ 60 ], similar healthcare systems [ 23 ], and being randomly drawn from a social spending category [ 61 ]. Some researchers were interested explicitly in a specific geographical region, such as Eastern Europe [ 50 ], Central and Eastern Europe [ 48 , 60 ], the Visegrad (V4) group [ 62 ], or the Asia/Pacific area [ 32 ]. In certain instances, national regions or cities, rather than countries, constituted the units of investigation instead [ 31 , 51 , 56 , 62 – 66 ]. In two particular cases, a mix of countries and cities was used [ 35 , 57 ]. In another two [ 28 , 29 ], due to the long time periods under study, some of the included countries no longer exist. Finally, besides “European” and “OECD”, the terms “developed”, “Western”, and “industrialized” were also used to describe the group of selected nations [ 30 , 42 , 52 , 53 , 67 ].
As stated above, it was the health status of the general population that we were interested in, and during screening we made a concerted effort to exclude research using data based on a more narrowly defined group of individuals. All studies included in this review adhere to this general rule, albeit with two caveats. First, as cities (even neighborhoods) were the unit of analysis in three of the studies that made the selection [ 56 , 64 , 65 ], the populations under investigation there can be more accurately described as general urban , instead of just general. Second, oftentimes health indicators were stratified based on gender and/or age, therefore we also admitted one study that, due to its specific research question, focused on men and women of early retirement age [ 35 ] and another that considered adult males only [ 68 ].
Data types and sources
A great diversity of sources was utilized for data collection purposes. The accessible reference databases of the OECD ( https://www.oecd.org/ ), WHO ( https://www.who.int/ ), World Bank ( https://www.worldbank.org/ ), United Nations ( https://www.un.org/en/ ), and Eurostat ( https://ec.europa.eu/eurostat ) were among the top choices. The other international databases included Human Mortality [ 30 , 39 , 50 ], Transparency International [ 40 , 48 , 50 ], Quality of Government [ 28 , 69 ], World Income Inequality [ 30 ], International Labor Organization [ 41 ], International Monetary Fund [ 70 ]. A number of national databases were referred to as well, for example the US Bureau of Statistics [ 42 , 53 ], Korean Statistical Information Services [ 67 ], Statistics Canada [ 67 ], Australian Bureau of Statistics [ 67 ], and Health New Zealand Tobacco control and Health New Zealand Food and Nutrition [ 19 ]. Well-known surveys, such as the World Values Survey [ 25 , 55 ], the European Social Survey [ 25 , 39 , 44 ], the Eurobarometer [ 46 , 56 ], the European Value Survey [ 25 ], and the European Statistics of Income and Living Condition Survey [ 43 , 47 , 70 ] were used as data sources, too. Finally, in some cases [ 25 , 28 , 29 , 35 , 36 , 41 , 69 ], built-for-purpose datasets from previous studies were re-used.
In most of the studies, the level of the data (and analysis) was national. The exceptions were six papers that dealt with Nomenclature of Territorial Units of Statistics (NUTS2) regions [ 31 , 62 , 63 , 66 ], otherwise defined areas [ 51 ] or cities [ 56 ], and seven others that were multilevel designs and utilized both country- and region-level data [ 57 ], individual- and city- or country-level [ 35 ], individual- and country-level [ 44 , 45 , 48 ], individual- and neighborhood-level [ 64 ], and city-region- (NUTS3) and country-level data [ 65 ]. Parallel to that, the data type was predominantly longitudinal, with only a few studies using purely cross-sectional data [ 25 , 33 , 43 , 45 – 48 , 50 , 62 , 67 , 68 , 71 , 72 ], albeit in four of those [ 43 , 48 , 68 , 72 ] two separate points in time were taken (thus resulting in a kind of “double cross-section”), while in another the averages across survey waves were used [ 56 ].
In studies using longitudinal data, the length of the covered time periods varied greatly. Although this was almost always less than 40 years, in one study it covered the entire 20 th century [ 29 ]. Longitudinal data, typically in the form of annual records, was sometimes transformed before usage. For example, some researchers considered data points at 5- [ 34 , 36 , 49 ] or 10-year [ 27 , 29 , 35 ] intervals instead of the traditional 1, or took averages over 3-year periods [ 42 , 53 , 73 ]. In one study concerned with the effect of the Great Recession all data were in a “recession minus expansion change in trends”-form [ 57 ]. Furthermore, there were a few instances where two different time periods were compared to each other [ 42 , 53 ] or when data was divided into 2 to 4 (possibly overlapping) periods which were then analyzed separately [ 24 , 26 , 28 , 29 , 31 , 65 ]. Lastly, owing to data availability issues, discrepancies between the time points or periods of data on the different variables were occasionally observed [ 22 , 35 , 42 , 53 – 55 , 63 ].
Health determinants
Together with other essential details, Table 1 lists the health correlates considered in the selected studies. Several general categories for these correlates can be discerned, including health care, political stability, socio-economics, demographics, psychology, environment, fertility, life-style, culture, labor. All of these, directly or implicitly, have been recognized as holding importance for population health by existing theoretical models of (social) determinants of health [ 74 – 77 ].
https://doi.org/10.1371/journal.pone.0239031.t001
It is worth noting that in a few studies there was just a single aggregate-level covariate investigated in relation to a health outcome of interest to us. In one instance, this was life satisfaction [ 44 ], in another–welfare system typology [ 45 ], but also gender inequality [ 33 ], austerity level [ 70 , 78 ], and deprivation [ 51 ]. Most often though, attention went exclusively to GDP [ 27 , 29 , 46 , 57 , 65 , 71 ]. It was often the case that research had a more particular focus. Among others, minimum wages [ 79 ], hospital payment schemes [ 23 ], cigarette prices [ 63 ], social expenditure [ 20 ], residents’ dissatisfaction [ 56 ], income inequality [ 30 , 69 ], and work leave [ 41 , 58 ] took center stage. Whenever variables outside of these specific areas were also included, they were usually identified as confounders or controls, moderators or mediators.
We visualized the combinations in which the different determinants have been studied in Fig 2 , which was obtained via multidimensional scaling and a subsequent cluster analysis (details outlined in S2 Appendix ). It depicts the spatial positioning of each determinant relative to all others, based on the number of times the effects of each pair of determinants have been studied simultaneously. When interpreting Fig 2 , one should keep in mind that determinants marked with an asterisk represent, in fact, collectives of variables.
Groups of determinants are marked by asterisks (see S1 Table in S1 Appendix ). Diminishing color intensity reflects a decrease in the total number of “connections” for a given determinant. Noteworthy pairwise “connections” are emphasized via lines (solid-dashed-dotted indicates decreasing frequency). Grey contour lines encircle groups of variables that were identified via cluster analysis. Abbreviations: age = population age distribution, associations = membership in associations, AT-index = atherogenic-thrombogenic index, BR = birth rate, CAPB = Cyclically Adjusted Primary Balance, civilian-labor = civilian labor force, C-section = Cesarean delivery rate, credit-info = depth of credit information, dissatisf = residents’ dissatisfaction, distrib.orient = distributional orientation, EDU = education, eHealth = eHealth index at GP-level, exch.rate = exchange rate, fat = fat consumption, GDP = gross domestic product, GFCF = Gross Fixed Capital Formation/Creation, GH-gas = greenhouse gas, GII = gender inequality index, gov = governance index, gov.revenue = government revenues, HC-coverage = healthcare coverage, HE = health(care) expenditure, HHconsump = household consumption, hosp.beds = hospital beds, hosp.payment = hospital payment scheme, hosp.stay = length of hospital stay, IDI = ICT development index, inc.ineq = income inequality, industry-labor = industrial labor force, infant-sex = infant sex ratio, labor-product = labor production, LBW = low birth weight, leave = work leave, life-satisf = life satisfaction, M-age = maternal age, marginal-tax = marginal tax rate, MDs = physicians, mult.preg = multiple pregnancy, NHS = Nation Health System, NO = nitrous oxide emissions, PM10 = particulate matter (PM10) emissions, pop = population size, pop.density = population density, pre-term = pre-term birth rate, prison = prison population, researchE = research&development expenditure, school.ref = compulsory schooling reform, smoke-free = smoke-free places, SO = sulfur oxide emissions, soc.E = social expenditure, soc.workers = social workers, sugar = sugar consumption, terror = terrorism, union = union density, UR = unemployment rate, urban = urbanization, veg-fr = vegetable-and-fruit consumption, welfare = welfare regime, Wwater = wastewater treatment.
https://doi.org/10.1371/journal.pone.0239031.g002
Distances between determinants in Fig 2 are indicative of determinants’ “connectedness” with each other. While the statistical procedure called for higher dimensionality of the model, for demonstration purposes we show here a two-dimensional solution. This simplification unfortunately comes with a caveat. To use the factor smoking as an example, it would appear it stands at a much greater distance from GDP than it does from alcohol. In reality however, smoking was considered together with alcohol consumption [ 21 , 25 , 26 , 52 , 68 ] in just as many studies as it was with GDP [ 21 , 25 , 26 , 52 , 59 ], five. To aid with respect to this apparent shortcoming, we have emphasized the strongest pairwise links. Solid lines connect GDP with health expenditure (HE), unemployment rate (UR), and education (EDU), indicating that the effect of GDP on health, taking into account the effects of the other three determinants as well, was evaluated in between 12 to 16 studies of the 60 included in this review. Tracing the dashed lines, we can also tell that GDP appeared jointly with income inequality, and HE together with either EDU or UR, in anywhere between 8 to 10 of our selected studies. Finally, some weaker but still worth-mentioning “connections” between variables are displayed as well via the dotted lines.
The fact that all notable pairwise “connections” are concentrated within a relatively small region of the plot may be interpreted as low overall “connectedness” among the health indicators studied. GDP is the most widely investigated determinant in relation to general population health. Its total number of “connections” is disproportionately high (159) compared to its runner-up–HE (with 113 “connections”), and then subsequently EDU (with 90) and UR (with 86). In fact, all of these determinants could be thought of as outliers, given that none of the remaining factors have a total count of pairings above 52. This decrease in individual determinants’ overall “connectedness” can be tracked on the graph via the change of color intensity as we move outwards from the symbolic center of GDP and its closest “co-determinants”, to finally reach the other extreme of the ten indicators (welfare regime, household consumption, compulsory school reform, life satisfaction, government revenues, literacy, research expenditure, multiple pregnancy, Cyclically Adjusted Primary Balance, and residents’ dissatisfaction; in white) the effects on health of which were only studied in isolation.
Lastly, we point to the few small but stable clusters of covariates encircled by the grey bubbles on Fig 2 . These groups of determinants were identified as “close” by both statistical procedures used for the production of the graph (see details in S2 Appendix ).
Statistical methodology
There was great variation in the level of statistical detail reported. Some authors provided too vague a description of their analytical approach, necessitating some inference in this section.
The issue of missing data is a challenging reality in this field of research, but few of the studies under review (12/60) explain how they dealt with it. Among the ones that do, three general approaches to handling missingness can be identified, listed in increasing level of sophistication: case-wise deletion, i.e., removal of countries from the sample [ 20 , 45 , 48 , 58 , 59 ], (linear) interpolation [ 28 , 30 , 34 , 58 , 59 , 63 ], and multiple imputation [ 26 , 41 , 52 ].
Correlations, Pearson, Spearman, or unspecified, were the only technique applied with respect to the health outcomes of interest in eight analyses [ 33 , 42 – 44 , 46 , 53 , 57 , 61 ]. Among the more advanced statistical methods, the family of regression models proved to be, by and large, predominant. Before examining this closer, we note the techniques that were, in a way, “unique” within this selection of studies: meta-analyses were performed (random and fixed effects, respectively) on the reduced form and 2-sample two stage least squares (2SLS) estimations done within countries [ 39 ]; difference-in-difference (DiD) analysis was applied in one case [ 23 ]; dynamic time-series methods, among which co-integration, impulse-response function (IRF), and panel vector autoregressive (VAR) modeling, were utilized in one study [ 80 ]; longitudinal generalized estimating equation (GEE) models were developed on two occasions [ 70 , 78 ]; hierarchical Bayesian spatial models [ 51 ] and special autoregressive regression [ 62 ] were also implemented.
Purely cross-sectional data analyses were performed in eight studies [ 25 , 45 , 47 , 50 , 55 , 56 , 67 , 71 ]. These consisted of linear regression (assumed ordinary least squares (OLS)), generalized least squares (GLS) regression, and multilevel analyses. However, six other studies that used longitudinal data in fact had a cross-sectional design, through which they applied regression at multiple time-points separately [ 27 , 29 , 36 , 48 , 68 , 72 ].
Apart from these “multi-point cross-sectional studies”, some other simplistic approaches to longitudinal data analysis were found, involving calculating and regressing 3-year averages of both the response and the predictor variables [ 54 ], taking the average of a few data-points (i.e., survey waves) [ 56 ] or using difference scores over 10-year [ 19 , 29 ] or unspecified time intervals [ 40 , 55 ].
Moving further in the direction of more sensible longitudinal data usage, we turn to the methods widely known among (health) economists as “panel data analysis” or “panel regression”. Most often seen were models with fixed effects for country/region and sometimes also time-point (occasionally including a country-specific trend as well), with robust standard errors for the parameter estimates to take into account correlations among clustered observations [ 20 , 21 , 24 , 28 , 30 , 32 , 34 , 37 , 38 , 41 , 52 , 59 , 60 , 63 , 66 , 69 , 73 , 79 , 81 , 82 ]. The Hausman test [ 83 ] was sometimes mentioned as the tool used to decide between fixed and random effects [ 26 , 49 , 63 , 66 , 73 , 82 ]. A few studies considered the latter more appropriate for their particular analyses, with some further specifying that (feasible) GLS estimation was employed [ 26 , 34 , 49 , 58 , 60 , 73 ]. Apart from these two types of models, the first differences method was encountered once as well [ 31 ]. Across all, the error terms were sometimes assumed to come from a first-order autoregressive process (AR(1)), i.e., they were allowed to be serially correlated [ 20 , 30 , 38 , 58 – 60 , 73 ], and lags of (typically) predictor variables were included in the model specification, too [ 20 , 21 , 37 , 38 , 48 , 69 , 81 ]. Lastly, a somewhat different approach to longitudinal data analysis was undertaken in four studies [ 22 , 35 , 48 , 65 ] in which multilevel–linear or Poisson–models were developed.
Regardless of the exact techniques used, most studies included in this review presented multiple model applications within their main analysis. None attempted to formally compare models in order to identify the “best”, even if goodness-of-fit statistics were occasionally reported. As indicated above, many studies investigated women’s and men’s health separately [ 19 , 21 , 22 , 27 – 29 , 31 , 33 , 35 , 36 , 38 , 39 , 45 , 50 , 51 , 64 , 65 , 69 , 82 ], and covariates were often tested one at a time, including other covariates only incrementally [ 20 , 25 , 28 , 36 , 40 , 50 , 55 , 67 , 73 ]. Furthermore, there were a few instances where analyses within countries were performed as well [ 32 , 39 , 51 ] or where the full time period of interest was divided into a few sub-periods [ 24 , 26 , 28 , 31 ]. There were also cases where different statistical techniques were applied in parallel [ 29 , 55 , 60 , 66 , 69 , 73 , 82 ], sometimes as a form of sensitivity analysis [ 24 , 26 , 30 , 58 , 73 ]. However, the most common approach to sensitivity analysis was to re-run models with somewhat different samples [ 39 , 50 , 59 , 67 , 69 , 80 , 82 ]. Other strategies included different categorization of variables or adding (more/other) controls [ 21 , 23 , 25 , 28 , 37 , 50 , 63 , 69 ], using an alternative main covariate measure [ 59 , 82 ], including lags for predictors or outcomes [ 28 , 30 , 58 , 63 , 65 , 79 ], using weights [ 24 , 67 ] or alternative data sources [ 37 , 69 ], or using non-imputed data [ 41 ].
As the methods and not the findings are the main focus of the current review, and because generic checklists cannot discern the underlying quality in this application field (see also below), we opted to pool all reported findings together, regardless of individual study characteristics or particular outcome(s) used, and speak generally of positive and negative effects on health. For this summary we have adopted the 0.05-significance level and only considered results from multivariate analyses. Strictly birth-related factors are omitted since these potentially only relate to the group of infant mortality indicators and not to any of the other general population health measures.
Starting with the determinants most often studied, higher GDP levels [ 21 , 26 , 27 , 29 , 30 , 32 , 43 , 48 , 52 , 58 , 60 , 66 , 67 , 73 , 79 , 81 , 82 ], higher health [ 21 , 37 , 47 , 49 , 52 , 58 , 59 , 68 , 72 , 82 ] and social [ 20 , 21 , 26 , 38 , 79 ] expenditures, higher education [ 26 , 39 , 52 , 62 , 72 , 73 ], lower unemployment [ 60 , 61 , 66 ], and lower income inequality [ 30 , 42 , 53 , 55 , 73 ] were found to be significantly associated with better population health on a number of occasions. In addition to that, there was also some evidence that democracy [ 36 ] and freedom [ 50 ], higher work compensation [ 43 , 79 ], distributional orientation [ 54 ], cigarette prices [ 63 ], gross national income [ 22 , 72 ], labor productivity [ 26 ], exchange rates [ 32 ], marginal tax rates [ 79 ], vaccination rates [ 52 ], total fertility [ 59 , 66 ], fruit and vegetable [ 68 ], fat [ 52 ] and sugar consumption [ 52 ], as well as bigger depth of credit information [ 22 ] and percentage of civilian labor force [ 79 ], longer work leaves [ 41 , 58 ], more physicians [ 37 , 52 , 72 ], nurses [ 72 ], and hospital beds [ 79 , 82 ], and also membership in associations, perceived corruption and societal trust [ 48 ] were beneficial to health. Higher nitrous oxide (NO) levels [ 52 ], longer average hospital stay [ 48 ], deprivation [ 51 ], dissatisfaction with healthcare and the social environment [ 56 ], corruption [ 40 , 50 ], smoking [ 19 , 26 , 52 , 68 ], alcohol consumption [ 26 , 52 , 68 ] and illegal drug use [ 68 ], poverty [ 64 ], higher percentage of industrial workers [ 26 ], Gross Fixed Capital creation [ 66 ] and older population [ 38 , 66 , 79 ], gender inequality [ 22 ], and fertility [ 26 , 66 ] were detrimental.
It is important to point out that the above-mentioned effects could not be considered stable either across or within studies. Very often, statistical significance of a given covariate fluctuated between the different model specifications tried out within the same study [ 20 , 49 , 59 , 66 , 68 , 69 , 73 , 80 , 82 ], testifying to the importance of control variables and multivariate research (i.e., analyzing multiple independent variables simultaneously) in general. Furthermore, conflicting results were observed even with regards to the “core” determinants given special attention, so to speak, throughout this text. Thus, some studies reported negative effects of health expenditure [ 32 , 82 ], social expenditure [ 58 ], GDP [ 49 , 66 ], and education [ 82 ], and positive effects of income inequality [ 82 ] and unemployment [ 24 , 31 , 32 , 52 , 66 , 68 ]. Interestingly, one study [ 34 ] differentiated between temporary and long-term effects of GDP and unemployment, alluding to possibly much greater complexity of the association with health. It is also worth noting that some gender differences were found, with determinants being more influential for males than for females, or only having statistically significant effects for male health [ 19 , 21 , 28 , 34 , 36 , 37 , 39 , 64 , 65 , 69 ].
The purpose of this scoping review was to examine recent quantitative work on the topic of multi-country analyses of determinants of population health in high-income countries.
Measuring population health via relatively simple mortality-based indicators still seems to be the state of the art. What is more, these indicators are routinely considered one at a time, instead of, for example, employing existing statistical procedures to devise a more general, composite, index of population health, or using some of the established indices, such as disability-adjusted life expectancy (DALE) or quality-adjusted life expectancy (QALE). Although strong arguments for their wider use were already voiced decades ago [ 84 ], such summary measures surface only rarely in this research field.
On a related note, the greater data availability and accessibility that we enjoy today does not automatically equate to data quality. Nonetheless, this is routinely assumed in aggregate level studies. We almost never encountered a discussion on the topic. The non-mundane issue of data missingness, too, goes largely underappreciated. With all recent methodological advancements in this area [ 85 – 88 ], there is no excuse for ignorance; and still, too few of the reviewed studies tackled the matter in any adequate fashion.
Much optimism can be gained considering the abundance of different determinants that have attracted researchers’ attention in relation to population health. We took on a visual approach with regards to these determinants and presented a graph that links spatial distances between determinants with frequencies of being studies together. To facilitate interpretation, we grouped some variables, which resulted in some loss of finer detail. Nevertheless, the graph is helpful in exemplifying how many effects continue to be studied in a very limited context, if any. Since in reality no factor acts in isolation, this oversimplification practice threatens to render the whole exercise meaningless from the outset. The importance of multivariate analysis cannot be stressed enough. While there is no “best method” to be recommended and appropriate techniques vary according to the specifics of the research question and the characteristics of the data at hand [ 89 – 93 ], in the future, in addition to abandoning simplistic univariate approaches, we hope to see a shift from the currently dominating fixed effects to the more flexible random/mixed effects models [ 94 ], as well as wider application of more sophisticated methods, such as principle component regression, partial least squares, covariance structure models (e.g., structural equations), canonical correlations, time-series, and generalized estimating equations.
Finally, there are some limitations of the current scoping review. We searched the two main databases for published research in medical and non-medical sciences (PubMed and Web of Science) since 2013, thus potentially excluding publications and reports that are not indexed in these databases, as well as older indexed publications. These choices were guided by our interest in the most recent (i.e., the current state-of-the-art) and arguably the highest-quality research (i.e., peer-reviewed articles, primarily in indexed non-predatory journals). Furthermore, despite holding a critical stance with regards to some aspects of how determinants-of-health research is currently conducted, we opted out of formally assessing the quality of the individual studies included. The reason for that is two-fold. On the one hand, we are unaware of the existence of a formal and standard tool for quality assessment of ecological designs. And on the other, we consider trying to score the quality of these diverse studies (in terms of regional setting, specific topic, outcome indices, and methodology) undesirable and misleading, particularly since we would sometimes have been rating the quality of only a (small) part of the original studies—the part that was relevant to our review’s goal.
Our aim was to investigate the current state of research on the very broad and general topic of population health, specifically, the way it has been examined in a multi-country context. We learned that data treatment and analytical approach were, in the majority of these recent studies, ill-equipped or insufficiently transparent to provide clarity regarding the underlying mechanisms of population health in high-income countries. Whether due to methodological shortcomings or the inherent complexity of the topic, research so far fails to provide any definitive answers. It is our sincere belief that with the application of more advanced analytical techniques this continuous quest could come to fruition sooner.
Supporting information
S1 checklist. preferred reporting items for systematic reviews and meta-analyses extension for scoping reviews (prisma-scr) checklist..
https://doi.org/10.1371/journal.pone.0239031.s001
S1 Appendix.
https://doi.org/10.1371/journal.pone.0239031.s002
S2 Appendix.
https://doi.org/10.1371/journal.pone.0239031.s003
- View Article
- Google Scholar
- PubMed/NCBI
- 75. Dahlgren G, Whitehead M. Policies and Strategies to Promote Equity in Health. Stockholm, Sweden: Institute for Future Studies; 1991.
- 76. Brunner E, Marmot M. Social Organization, Stress, and Health. In: Marmot M, Wilkinson RG, editors. Social Determinants of Health. Oxford, England: Oxford University Press; 1999.
- 77. Najman JM. A General Model of the Social Origins of Health and Well-being. In: Eckersley R, Dixon J, Douglas B, editors. The Social Origins of Health and Well-being. Cambridge, England: Cambridge University Press; 2001.
- 85. Carpenter JR, Kenward MG. Multiple Imputation and its Application. New York: John Wiley & Sons; 2013.
- 86. Molenberghs G, Fitzmaurice G, Kenward MG, Verbeke G, Tsiatis AA. Handbook of Missing Data Methodology. Boca Raton: Chapman & Hall/CRC; 2014.
- 87. van Buuren S. Flexible Imputation of Missing Data. 2nd ed. Boca Raton: Chapman & Hall/CRC; 2018.
- 88. Enders CK. Applied Missing Data Analysis. New York: Guilford; 2010.
- 89. Shayle R. Searle GC, Charles E. McCulloch. Variance Components: John Wiley & Sons, Inc.; 1992.
- 90. Agresti A. Foundations of Linear and Generalized Linear Models. Hoboken, New Jersey: John Wiley & Sons Inc.; 2015.
- 91. Leyland A. H. (Editor) HGE. Multilevel Modelling of Health Statistics: John Wiley & Sons Inc; 2001.
- 92. Garrett Fitzmaurice MD, Geert Verbeke, Geert Molenberghs. Longitudinal Data Analysis. New York: Chapman and Hall/CRC; 2008.
- 93. Wolfgang Karl Härdle LS. Applied Multivariate Statistical Analysis. Berlin, Heidelberg: Springer; 2015.
The impact of loneliness on depression during the COVID-19 pandemic in China: a two-wave follow-up study
- Open access
- Published: 03 May 2024
Cite this article
You have full access to this open access article
- Linlin Yan ORCID: orcid.org/0000-0001-7192-8074 1 ,
- Xu Ding 1 ,
- Yiqun Gan 2 ,
- Naiyi Wang 3 , 4 ,
- Jianhui Wu 1 , 5 &
- Hongxia Duan 1 , 5 , 6
102 Accesses
Explore all metrics
During the COVID-19 pandemic, many regions and countries implemented lockdowns and isolation to curb the virus’s spread, which might increase loneliness and lead to a series of psychological distress. This study aims to investigate the association between loneliness and depression during the COVID-19 pandemic and examine whether perceived social support and perceived internal control mediate the loneliness-depression relationship in China. Self-report questionnaires were distributed online in two waves during the pandemic in 2020. At Wave 1, demographics and loneliness were reported when the lockdown was initially implemented in China, and at Wave 2, as the pandemic came under control and the epicenter lifted its lockdown. Depression, perceived social support, and perceived internal control were measured at both two waves. Higher levels of loneliness at Wave 1 were associated with more depression at Wave 2 after controlling for baseline depression and demographic variables. Simple mediation models showed that both perceived social support and internal control at two waves independently mediated the relationship between Wave 1 loneliness and Wave 2 depression. Additionally, the serial multiple mediation model indicated that perceived social support and perceived internal control sequentially mediated the path from loneliness to subsequent depression. A higher level of loneliness during the initial lockdown was linked with more severe depression with the development of the COVID-19 pandemic. Both perceived social support and perceived internal control acted as essential protective factors against depression from loneliness. Meanwhile, social support might protect mental health by enhancing the sense of self-control when facing loneliness.
Similar content being viewed by others
Loneliness and psychotic symptoms: the mediating role of depression.
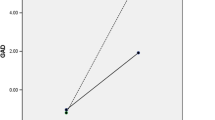
Effects of biopsychosocial factors on the association between loneliness and mental health risks during the COVID-19 lockdown
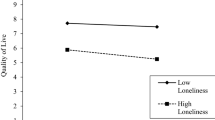
Moderation effects of loneliness between fatalism and wellbeing during the COVID-19 pandemic
Avoid common mistakes on your manuscript.
Introduction
The coronavirus disease (COVID-19) developed into a global pandemic since December 2019. Since then, governments announced and reinforced quarantine or lockdowns in many regions. The COVID-19 pandemic caused a threat to the physical and mental health of the general population (Duan et al., 2020 ; Yan et al., 2021 ). The increased level of loneliness was widespread during the lockdown (Beam & Kim, 2020 ), which in turn was associated with worse physical and psychological well-being (Maes et al., 2019 ). Additionally, depression has emerged as a public health issue before and during the pandemic (Park et al., 2019 ). This study aims to investigate the association between loneliness and depression during the COVID-19 pandemic and examine whether perceived social support and perceived internal control mediate the loneliness-depression relationship.
Loneliness and mental health
Loneliness is defined as an unpleasant experience perceived by an individual due to the insufficient quality and quantity of social relationship networks (Perlman & Peplau, 1981 ). Loneliness has become a growing problem in modern society (Cacioppo & Cacioppo, 2018 ). For instance, the prevalence of loneliness among the elderly in China has shown a dramatically rising trend (Luo & Waite, 2014 ). A national survey in Switzerland has also found that about 40% of the 20,007 respondents have felt lonely (Richard et al., 2017 ). After the outbreak of the COVID-19 pandemic, 43% of participants reported moderate to severe levels of loneliness, and more than 60% frequently felt “being separated from society” (Killgore et al., 2020 ).
Loneliness has shown an adverse influence on human health, both mentally and physically. For example, lonely individuals exhibited a reduction in prosocial behavior (Twenge et al., 2007 ), more symptoms of social anxiety (Maes et al., 2019 ), and a higher prevalence of suicide ideation (Stravynski & Boyer, 2001 ). Furthermore, feeling lonely is one of the typical features of depressive symptoms (Erzen & Çikrikci, 2018 ). Extensive studies have shown that loneliness is an essential predictor of depression, such that a higher score of loneliness is associated with more severe depressive symptoms in older people (Liu et al., 2016 ), adolescents (Ladd & Ettekal, 2013 ) as well as among the general population (Rossi et al., 2020 ). An 8-year follow-up study has demonstrated that chronic loneliness in childhood increased individuals’ susceptibility to depression in adolescence (Qualter et al., 2010 ). A longitudinal study revealed that the elderly who feel lonelier are more likely to develop major depression disorders and general anxiety disorders in two years (Domènech-Abella et al., 2019 ). Specifically, loneliness positively predicted depression among the general population in Israel during the COVID-19 pandemic (Palgi et al., 2020 ) and older adults in the United States (Krendl & Perry, 2020 ). However, few studies have investigated the potential risk or protective factors in the loneliness-depression correlation during the COVID-19 pandemic.
In stress and adaptation literature, the impact of people’s resources plays a vital role in stress resistance and resiliency (Hobfoll, 1989 , 2002 ). Psychological resources are described as entities or means to obtain valued ends and include social and personal aspects. i.e., the external social environment and the self (Hobfoll, 2002 ). Caplan ( 1974 ) pointed out that social support and a sense of mastery were two critical elements for maintaining well-being. As most frequently discussed, social support and perceived control appear to be the central elements of social and personal resources, respectively (Taylor & Stanton, 2007 ). Social support and perceived control have consistently shown adaptive values in dealing with stressful situations (e.g., violence or cancer) (Bebanic et al., 2017 ; Helgeson et al., 2004 ). Here, this study aims to examine the role of psychological resources, including perceived social support and perceived control, in the loneliness-depression relationship during the COVID-19 pandemic.
Social resource: role of perceived social support
Social support refers to an essential coping resource or assistance from friends, family, or significant others under stressful situations (e.g., the COVID-19 outbreak) (Sarason et al., 1983 ; Zimet et al., 1988 ). There are two potential dimensions of social support: the actual size of one’s social support system and the subjective assessment of social support provided by the system (Sarason et al., 1983 ). Studies have provided consistent evidence that perceived social support is a solid and positive indicator of individuals’ well-being (Karademas, 2006 ) and life satisfaction (Siedlecki et al., 2014 ).
As a crucial coping mechanism under challenges, social support has also been demonstrated to protect psychological health during the COVID-19 pandemic (Saltzman et al., 2020 ). The value of social support seemed particularly relevant to loneliness due to the lockdown and related measures such as quarantine and social distancing during the COVID-19 pandemic. First, perceived social support was well-documented as one of the most crucial protective factors against loneliness (Lasgaard et al., 2010 ). Second, numerous studies have also revealed the buffering role of social support on symptoms of depression (Duan et al., 2020 ) and anxiety (Yue et al., 2020 ). Therefore, perceived social support, as a core element of social resources, might alleviate depression resulting from loneliness during the COVID-19 pandemic.
Personal resource: role of perceived internal control
Perceived control is frequently discussed in conjunction with stressful life events, in which events perceived as uncontrollable are more distressing than those perceived as controllable (Foa et al., 1992 ). According to Skinner ( 1995 ), experiencing control is an innate and universal need for individuals to facilitate interactions with their environment. A lack of perceived control can result in learned helplessness, which is considered one of the main characteristics of depression (Rubinstein, 2004 ). More perceptions of control might help individuals regulate their emotions and behaviors, especially under challenges or threats (Skinner, 1995 ), manifesting in lower levels of loneliness (Andrew & Meeks, 2018 ), depression (Gallagher & McKinley, 2009 ), and anxiety (Gallagher et al., 2014 ).
It is worthwhile to mention that external control of events or outcomes might not be feasible for many situations like the current public health emergency (i.e., the COVID-19 pandemic), while perceived control over internal personal states like emotions and thoughts appears to be more critical and practical for adaptation under adverse or threatening circumstances (Pallant, 2000 ). For instance, participants showed decreased external control over the infection with the development of the COVID-19 pandemic, which further resulted in irrational behaviors like stocking up on food (Goodwin et al., 2021 ). By contrast, an earlier study with cancer patients found that perceived control over internal states, such as emotional and physical responses to disease, instead of control over the disease course per se, predicted better overall psychological adjustments, including fewer symptoms of depression and anxiety (Thompson et al., 1993 ). Hence, under the background of the COVID-19 pandemic, higher perceived internal control as an essential personal resource may reduce loneliness-related depression, i.e., more perceptions of internal control might buffer the association between loneliness and depression.
A serial multiple mediation model
Based on the brief literature review above, this study aims to investigate the mediating effects of perceived social support and perceived internal control in the association between loneliness and depression during the COVID-19 pandemic. Notably, perceived social support and perceived internal control might not act independently, as social resources are reciprocally interrelated to personal resources (Hobfoll et al., 1990 ). For instance, a nine-year longitudinal study found that perceived control acted as both an antecedent and an outcome of social support (Gerstorf et al., 2011 ). This finding aligns with earlier propositions that social support can exert positive effects by promoting a sense of self-control in the face of adverse events (Cohen & Wills, 1985 ; Hobfoll, 1989 ). Therefore, a serial multiple mediation model was proposed in our study to explore whether perceived social support and perceived internal control work together in the loneliness-depression relation during the COVID-19 pandemic.
The present study
We aimed to examine the following hypotheses:
Loneliness at Wave 1 would be positively associated with depression at Wave 2.
Perceived social support and perceived internal control would mediate the relationship between loneliness at Wave 1 and depression at Wave 2, respectively.
Perceived social support and perceived internal control would sequentially mediate the path from Wave 1 loneliness to Wave 2 depression. Specifically, in a serial way, higher levels of loneliness at Wave 1 would be linked to lower levels of perceived social support and perceived internal control at Wave 1, which would be related to greater depression at Wave 2.
Participants and procedures
The present study was part of a big project (Duan et al., 2020 ; Yan et al., 2021 ), and included data collection in two waves: from January 31st to February 9th (Wave 1) and from March 15th to March 28th (Wave 2) in 2020, covering the period from the initial lockdown to when the restrictions were lifted in China (Ren, 2020 ). Questionnaires were administered using WenJuanXing (an online survey tool developed by Changsha Ranxing Information Technology Co., Ltd., Hunan, China). We commissioned WenJuanXing to recruit participants randomly from their user database in China, and participants were compensated separately with monetary incentives for their participation in the two waves of the survey. Ethical approval was provided by the ethics committee at the local university.
Six infrequent items (e.g., “I do not remember my name”) were used to identify invalid respondents for the attention checks for online research (Newman et al., 2021 ). Participants with at least four correct answers to attention checks were included in the final analyses. At the first wave, 5,019 respondents were contacted and 3,206 met the inclusion criteria above, resulting in a compliance rate of 63.88%. Among these 3,206 participants, 1,390 completed all questionnaires in Wave 2, yielding a compliance rate of 43.36% Footnote 1 . Therefore, data from these 1,390 individuals were analyzed in the current study and the demographic characteristics are shown in Table 1 . 403 participants in the final sample were from Hubei Province, and others were from the 29 provinces and regions in mainland China.
The UCLA (University of California, Los Angeles) Loneliness Scale (ULS), developed by Russell et al. ( 1980 ), was used to measure loneliness levels for its wide application in other studies under the COVID-19 pandemic (e.g., Alheneidi et al., 2021 ). Zhou and colleagues ( 2012 ) translated these items into Chinese and demonstrated good psychometric properties in the revised 6-item version (i.e., ULS-6). ULS-6 is a unidimensional scale rated from 1 (never) to 5 (all the time), and an exemplary item is “There is no one I can turn to”. Participants rated their loneliness levels at Wave 1. Cronbach’s alpha of ULS-6 for the present study was 0.745.
The Psychological Questionnaire for Emergent Events of Public Health was used to measure depression in two waves, and this scale has been commonly applied to assess psychological states within the context of the COVID-19 pandemic recently (e.g., Zhou et al., 2021 ). This scale was revised from the SARS Psychological Behaviour Questionnaire (Gao et al., 2004 ) and its dimension of depression includes six items. Each item was scored on a 4-point Likert scale, such as “My appetite was poor” or “I have no energy for things”. Cronbach’s alpha coefficients were 0.809 and 0.803 at Wave 1 and Wave 2, respectively.
- Perceived social support
The Perceived Social Support Scale (PSSS) measured respondents’ subjective understanding and feeling of social support (Zimet et al., 1988 ) in Wave 1 and Wave 2. The Chinese version of the PSSS was translated and validated by Jiang ( 2001 ). Participants responded via a 7-point Likert self-report scale (1 = strongly disagree; 7 = strongly agree), with higher scores indicating a higher degree of perceived social support. For instance, “I can talk about my problems with my friends”. The internal consistency coefficients were 0.812 and 0.828 at Wave 1 and 2, respectively.
- Perceived internal control
Perceived internal control was measured using the Perceived Control of Internal States Scale (PCISS; Pallant, 2000 ). There are 18 items, and each item is rated from 1 (strongly disagree) to 5 (strongly agree). An exemplary item is “I am usually able to keep my thoughts under control”. Higher scores were indicative of more perceptions of control over internal states. Respondents rated according to their current and actual situations. Cronbach alpha coefficients for PCISS were 0.915 and 0.925 at Wave 1 and Wave 2.
Statistical analysis
The statistical analyses were conducted in IBM SPSS Statistics 24.0 (IBM Corp., Armonk, NY, USA). Each scale was generated from the average of total scores. Age was coded as continuous variables, gender was coded as the binary variable, and education and monthly income were coded as ordinal variables (see Supplemental Table S1 for variables’ codes and response categories). All the continuous variables were standardized for the subsequent analyses. All analyses regarded a two-tailed significance level < 0.05 as statistically significant.
First, the paired t -test was used to compare depression, perceived social support, and perceived internal control across the two surveys (Wave 1 vs. Wave 2). Meanwhile, correlation analyses were conducted to explore the potential relationships among variables. Then, simple mediation models using Model 4 from PROCESS macro (Hayes, 2017 ) were built to test the mediating effects of perceived social support or perceived internal control on the association between Wave 1 loneliness and Wave 2 depression. Finally, a multiple mediation model was conducted using Model 6 to examine whether perceived social support and perceived internal control mediate the loneliness-depression relation sequentially. The indirect effect was produced using the percentile bootstrap with 5,000 samples to generate 95% confidence intervals for the mediated paths (Hayes & Scharkow, 2013 ). Confidence intervals that do not include zero indicate significant effects. The effect size for the mediation analysis was quantified as the relative mediation effect ( P M = a*b/c) for the indirect effect (Preacher & Kelley, 2011 ). The standard error for linear regression models was estimated using HC3 (Davidson & MacKinnon, 1993 ) to keep the test size at the nominal level regardless of heteroscedasticity (Long & Ervin, 2000 ).
Comparison analyses
Perceived social support showed a statistically significant increase from Wave 1 to Wave 2 ( t = -8.607, p < .001; Wave 1: 5.54 ± 0.73; Wave 2: 5.69 ± 0.68), while the levels of perceived internal control did not show statistically significant changes between the two waves ( t = -1.510, p = .131; Wave 1: 3.61 ± 0.61; Wave 2: 3.63 ± 0.63). Furthermore, there was a statistically significant increase ( t = -3.362, p = .001) in depression levels from Wave 1 (1.56 ± 0.51) to Wave 2 (1.60 ± 0.52).
Correlation analyses
Loneliness at Wave 1 was positively associated with depression both at wave 1 ( r = .356, p < .001) and Wave 2 ( r = 320, p < .001) (see Supplemental Table S2 ). Perceived social support (Wave 1: r = − .210, p < .001; Wave 2: r = − .240, p < .001) and perceived internal control at two waves (Wave 1: r = − .323, p < .001; Wave 2: r = − .418, p < .001) were negatively related to depression at Wave 2 (see Supplemental Table S2 ).
Mediation analyses
The mediating role of perceived social support.
Perceived social support at Wave 1 was treated as a mediator between Wave 1 loneliness and Wave 2 depression 2 , controlling for Wave 1 depression and demographic variables (gender, age, education, and monthly income). Results showed that there was a statistically significant direct effect between loneliness and depression (Path c : β = .132, 95% CI = [.075,.189], p < .001). This association kept significant after taking Wave 1 perceived social support into the model (Path c’ : β = 0.105, 95% CI = [0.045, 0.165], p < .001). The indirect effect of loneliness on depression through perceived social support was statistically significant (a*b = 0.026, 95% CI = [0.007, 046]) and accounted for 19.70% of the total effect.
The mediating role of perceived internal control
Similarly, when Wave 1 perceived internal control served as the mediator Footnote 2 , there was still a statistically significant direct effect of loneliness on depression (Path c’ : β = 0.092, 95% CI= [0.033, 0.151], p = .002) and the indirect path was significant (a*b = 0.040, 95% CI = [0.017, 064]). Additionally, perceived internal control explained 30.30% of the total effect of loneliness on depression.
The serial multiple mediation model
As shown in Table 2 ; Fig. 1 , the multiple mediations showed three indirect paths from Wave 1 loneliness to Wave 2 depression. First, Path 1 ( loneliness → perceived social support → depression ) was not statistically significant (a*b = 0.016, 95% CI = [-0.005, 0.037]). Second, Path 2 ( loneliness → perceived internal control → depression ) was statistically significant, and the indirect effect explained for 16.67% of the total effect (a*b = 0.022, 95% CI = [0.006, 0.041]). Third, Path 3 ( loneliness → perceived social support → perceived internal control → depression ) was also statistically significant (indirect effect = 0.011, 95% CI = = [0.003, 0.019]) and the proportion of this indirect path was 8.33%. Therefore, perceived social support and perceived internal control at Wave 1 sequentially mediated the relationship between loneliness and depression Footnote 3 .
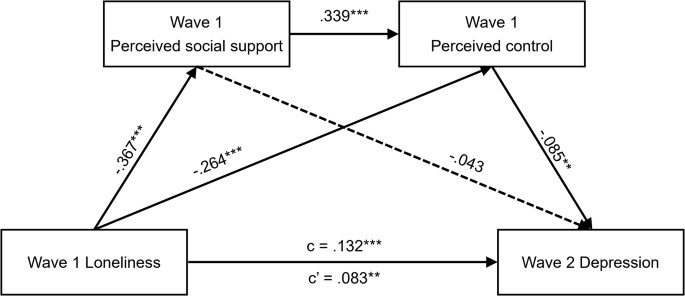
The serial multiple mediation model of the relationship between Wave 1 loneliness and Wave 2 depression through Wave 1 perceived social support and perceived internal control sequentially. Standardized regression coefficients are shown along the paths (*** p < .001; ** p < .01; * p < .05). Solid lines are statistically significant, and the dotted line is not significant
This study aims to explore the impact of loneliness on depression and the potential mediating roles of perceived social support and perceived internal control in the loneliness-depression link during the COVID-19 pandemic. As hypothesized, higher levels of loneliness at Wave 1 were associated with increased depression at Wave 2 after adjusting for baseline depression and demographic variables. Simple mediation models showed significant and independent indirect effects of perceived social support and perceived internal control (both in Wave 1 and Wave 2) on the loneliness-depression association. Furthermore, the serial multiple mediation model indicated that perceived social support and perceived internal control sequentially mediated the relationship between loneliness and depression.
When comparing Wave 1 and Wave 2, we found that perceived social support significantly increased, which might come from the increasing social support from neighborhoods and satisfaction with the performance of the central government (e.g., quick medical supplies and policy responses) (Chen et al., 2020 ). In contrast, depression deteriorated from the initial lockdown (Wave 1) to the relative remission phase (Wave 2), suggesting a lasting and severe psychological burden of the pandemic (Duan et al., 2020 ; Meaklim et al., 2021 ). Additionally, the perceived internal control did not change significantly across the two surveys, confirming the relative stability of perceived control (e.g., You et al., 2011 ).
We found that the higher level of loneliness during the initial lockdown phase was related to higher levels of depression during the relative remission phase, even after controlling for the initial depression symptoms. This result was further consistent with previous literature conducted in a large community-based cohort study (Beutel et al., 2017 ) or among the general population from different countries during the COVID-19 pandemic (Okruszek et al., 2020 ; Palgi et al., 2020 ). Insufficient social connections during the lockdown and social distancing as a preventive measurement for infection might be the main contributors to loneliness during the pandemic (Beam & Kim, 2020 ; Miller, 2020 ). In turn, these measurements might result in the generation of social anhedonia (Barkus & Badcock, 2019 ). Furthermore, studies also found that loneliness is closely linked to the inflammatory reactivity of stress, a crucial marker for future depressive symptoms (Aschbacher et al., 2012 ; Brown et al., 2018 ).
Subjective perception of social support as an essential social resource could account for the relationship between loneliness and subsequent depression. Specifically, higher levels of perceived social support can buffer the adverse effects of loneliness on depression. As a critical psychological resource, perceived social support has been proven effective in buffering against loneliness (Lee & Goldstein, 2016 ). According to the theory of the self-reinforcing loneliness loop, lonely individuals tend to exhibit more negative social expectations and further distance themselves from social partners to avoid possible social threats like social rejection (Cacioppo & Hawkley, 2009 ). Therefore, this study revealed that higher levels of perceived social support could protect mental health in stressful or threatening situations (e.g., the COVID-19 pandemic) by breaking or weakening the self-reinforcing loneliness loop. Conversely, the lack of social support may increase hopelessness and eventually lead to depression (Abramson et al., 1989 ), i.e., enhancing the self-reinforcing loneliness loop.
Perceived internal control was another significant mediator in the indirect path of loneliness to depression during the lockdown. This might indicate that lonely individuals report less emotional control over their emotions and behaviors during the pandemic, and lower internal control ultimately aggravates depression. This result was consistent with Newall and colleagues’ findings ( 2014 ) that persistent lonely people tend to experience lower perceptions of control than the rarely lonely group, and the decline of perceived control is a risk factor for increased loneliness in the future. First, consistent with the previous finding (Zhao & Shi, 2020 ), the current study supported that higher levels of loneliness were associated with fewer perceptions of internal control in the face of the uncontrollable pandemic. The loneliness model also proposed that loneliness may elicit implicit vigilance for social threats and thereby dampen self-regulation over one’s thoughts, feelings, and behavior (Hawkley & Cacioppo, 2010 ). Second, we found that lower perceived internal control is linked with subsequent more severe depression. A similar result was also revealed in the prospective study in maltreated children that adequate perceived internal control predicted decreased internalizing problems like anxiety and depression (Bolger & Patterson, 2001 ). When exposed to uncontrollable circumstances (e.g., pandemic or cancer diagnosis), individuals with better control over internal states (emotions, thoughts, etc.) may exhibit fewer negative appraisals of the adverse situations and take more adaptive coping strategies like social interactions, further leading to better psychological adjustment later (Henselmans, 2009 ).
Based on the association between perceived social support and perceived internal control, the current study also tested the serial multiple mediation model and found that perceived social support and perceived internal control mediated the relationship between loneliness and depression in a sequential way. Specifically, participants with lower levels of loneliness might be inclined to perceive more social support, and high perceived social support further enhances the perception of internal control, thereby decreasing depression levels during the pandemic. According to the support-efficacy model, received and perceived support can stimulate the generation of self-efficacy (a related concept with perceived control) by developing a positive sense of self, consequently leading to better well-being (Antonucci & Jackson, 1987 ). Therefore, social resources (i.e., social support) can be translated into personal resources (i.e., self-control or efficacy). For example, Gerstorf et al. ( 2011 ) found that a supportive relationship with family contributed to higher subjective control and increased perceived control. Furthermore, in addition to the positive effect of perceived social support on perceived internal control, our finding further highlighted this synergy in reducing loneliness-related depression. This echoed the integrated resources model that social and personal resources must work together to help individuals cope with threats or challenges (Holahan et al., 1999 ). Nonetheless, the multiple mediation model showed that perceived internal control, but not social support could independently mediate the path from loneliness to depression (path 1 and path 2). Altogether, these results suggested that the effect of subjective social support, to some extent, might be limited when facing adverse situations but instead could serve as a more robust buffer when these social resources are internalized into personal resources.
The current study had several limitations. First, the survey used online convenience sampling in China, which limited its ecological validity in methodology. There were significant demographic differences between respondents who completed both two waves and respondents who dropped out at Wave 2, suggesting that these demographic variables might bias our results. Second, all questionnaires were self-reported, which participants might underreport, or overestimate based on their current state. However, it is worthwhile to mention that self-report is the most effective method within public mental health services and research (Bentley et al., 2019 ), and all the questionnaires we used are standard and valid. Third, this longitudinal study collected data only at two waves and might not be able to sketch the dynamic mental health trajectories with the evolution of the pandemic. Nonetheless, this study captured the critical moments, covering the period from the initial lockdown to when the epicenter lifted its lockdown. Last, the current study is implemented within the background of the COVID-19 pandemic outbreak, and future studies need to explore whether these findings are repeatable during a “normal” time.
Despite the limitations above, this research also provided some implications. Depression demonstrated a slight increase even when the COVID-19 pandemic was under a certain degree of control, indicating the importance of mental health services in the general population when facing emergent public events. Considering that higher levels of loneliness may increase the risk of future depression, it might be crucial to screen the population susceptible to psychopathology at the early stage of such pandemics. Perceived social support and perceived control are essential protective factors against depression from loneliness. Some potential interventions might be practical to alleviate the feeling of loneliness and loneliness-derived mental health problems. For instance, cognitive intervention research demonstrated that social network enhancement could mitigate depression and loneliness and improve life quality (Winningham & Pike, 2007 ). Mindfulness has also been shown to be effective in helping individuals experience more control and well-being by boosting flexible responses to the environment’s demands (Smith & Neupert, 2020 ). Additionally, external social support may be a potential intervention target for enhancing personal resources such as perceived internal control.
Conclusions
Higher loneliness was associated with more depression symptoms. Both perceived internal control and perceived social support acted as essential protective factors against depression from loneliness during the COVID-19 pandemic. Furthermore, social support can exert positive effects when facing loneliness by enhancing the sense of self-control.
Data availability
The data that support the findings of this study are not openly available due to reasons of sensitivity and are available from the corresponding author upon reasonable request.
We divided the Wave 1 valid participants ( n = 3,206) into respondents ( n = 1,390) and non-respondents ( n = 1,816) in terms of whether they participated in Wave 2, and we further compared the difference of demographic variables (age, gender, education, and monthly income) between the two groups. Results showed significant demographic differences including age, gender, education, and monthly income between the two groups (see Supplemental Table S1 for details).
We also conducted two simple mediation models between loneliness and depression using Wave 2 perceived social support and perceived control as mediators, respectively. Results showed significant indirect paths of both perceived social support and perceived control at Wave 2 on the association between Wave 1 loneliness and Wave 2 depression (see Supplemental Table S3 for details).
We also conducted Furthermore, we also tested the serial multiple mediation model in the relationship between loneliness and depression when Wave 2 perceived social support and perceived control served as the mediators successively. Similarly, results suggested that Path 1 (loneliness → perceived social support → depression) was not significant. There were two significant indirect paths from loneliness to depression: Path 2 (loneliness → perceived internal control → depression) and Path 3 (loneliness → perceived social support → perceived internal control → depression) (see Supplemental Table S4 and Figure S1 for details).
Abramson, L. Y., Metalsky, G. I., & Alloy, L. B. (1989). Hopelessness depression: A theory-based subtype of depression. Psychological Review , 96 (2), 358.
Article Google Scholar
Alheneidi, H., AlSumait, L., AlSumait, D., & Smith, A. P. (2021). Loneliness and problematic internet use during COVID-19 lock-down. Behavioral Sciences , 11 (1), 5.
Andrew, N., & Meeks, S. (2018). Fulfilled preferences, perceived control, life satisfaction, and loneliness in elderly long-term care residents. Aging & Mental Health , 22 (2), 183–189.
Antonucci, T. C., & Jackson, J. S. (1987). Social support, interpersonal efficacy, and health: A life course perspective.
Aschbacher, K., Epel, E., Wolkowitz, O. M., Prather, A. A., Puterman, E., & Dhabhar, F. S. (2012). Maintenance of a positive outlook during acute stress protects against pro-inflammatory reactivity and future depressive symptoms. Brain Behavior and Immunity , 26 (2), 346–352.
Article PubMed Google Scholar
Barkus, E., & Badcock, J. C. (2019). A transdiagnostic perspective on social anhedonia. Frontiers in Psychiatry , 10 , 216.
Article PubMed PubMed Central Google Scholar
Beam, C. R., & Kim, A. J. (2020). Psychological sequelae of social isolation and loneliness might be a larger problem in young adults than older adults. Psychological Trauma: Theory Research Practice and Policy , 12 (S1), S58.
Bebanic, V., Clench-Aas, J., Raanaas, R. K., & Nes, B., R (2017). The relationship between violence and psychological distress among men and women: Do sense of mastery and social support matter? Journal of Interpersonal Violence , 32 (16), 2371–2395.
Bentley, N., Hartley, S., & Bucci, S. (2019). Systematic review of self-report measures of general mental health and wellbeing in adolescent mental health. Clinical Child and Family Psychology Review , 22 (2), 225–252.
Beutel, M. E., Klein, E. M., Brähler, E., Reiner, I., Jünger, C., Michal, M., & Tibubos, A. N. (2017). Loneliness in the general population: Prevalence, determinants and relations to mental health. Bmc Psychiatry , 17 (1), 97.
Bolger, K. E., & Patterson, C. J. (2001). Pathways from child maltreatment to internalizing problems: Perceptions of control as mediators and moderators. Development and Psychopathology , 13 (4), 913–940.
Brown, E. G., Gallagher, S., & Creaven, A. M. (2018). Loneliness and acute stress reactivity: A systematic review of psychophysiological studies. Psychophysiology , 55(5), e13031.
Cacioppo, J. T., & Cacioppo, S. (2018). The growing problem of loneliness. The Lancet , 391 (10119), 426.
Cacioppo, J. T., & Hawkley, L. C. (2009). Perceived social isolation and cognition. Trends in Cognitive Sciences , 13 (10), 447–454.
Caplan, G. (1974). Support systems and community mental health: Lectures on concept development . behavioral.
Chen, X., Gao, H., Zou, Y., & Lin, F. (2020). Changes in psychological wellbeing, attitude and information-seeking behaviour among people at the epicentre of the COVID-19 pandemic: A panel survey of residents in Hubei Province. China Epidemiology & Infection , 148.
Cohen, S., & Wills, T. A. (1985). Stress, social support, and the buffering hypothesis. Psychological Bulletin , 98 (2), 310.
Davidson, R., & MacKinnon, J. G. (1993). Estimation and inference in econometrics. OUP Catalogue .
Domènech-Abella, J., Mundó, J., Haro, J. M., & Rubio-Valera, M. (2019). Anxiety, depression, loneliness and social network in the elderly: Longitudinal associations from the Irish longitudinal study on Ageing (TILDA). Journal of Affective Disorders , 246 , 82–88.
Duan, H., Yan, L., Ding, X., Gan, Y., Kohn, N., & Wu, J. (2020). Impact of the COVID-19 pandemic on mental health in the general Chinese population: Changes, predictors and psychosocial correlates. Psychiatry Research , 293 , 113396.
Erzen, E., & Çikrikci, Ö. (2018). The effect of loneliness on depression: A meta-analysis. International Journal of Social Psychiatry , 64 (5), 427–435.
Foa, E. B., Zinbarg, R., & Rothbaum, B. O. (1992). Uncontrollability and unpredictability in post-traumatic stress disorder: An animal model. Psychological Bulletin , 112 (2), 218.
Gallagher, M. W., Bentley, K. H., & Barlow, D. H. (2014). Perceived control and vulnerability to anxiety disorders: A meta-analytic review. Cognitive Therapy and Research , 38 (6), 571–584.
Gallagher, R., & McKinley, S. (2009). Anxiety, depression and perceived control in patients having coronary artery bypass grafts. Journal of Advanced Nursing , 65 (11), 2386–2396.
Gao, Y., Xu, M., Yang, Y., & Yao, K. (2004). Discussion on the coping style of undergraduates and the correlative factors during the epidemic period of SARS. Chinese Medical Ethics , 17 (2), 60–63.
Google Scholar
Gerstorf, D., Röcke, C., & Lachman, M. E. (2011). Antecedent–consequent relations of perceived control to health and social support: Longitudinal evidence for between-domain associations across adulthood. Journals of Gerontology Series B: Psychological Sciences and Social Sciences , 66 (1), 61–71.
Goodwin, R., Wiwattanapantuwong, J., Tuicomepee, A., Suttiwan, P., Watakakosol, R., & Ben-Ezra, M. (2021). Anxiety, perceived control and pandemic behaviour in Thailand during COVID-19: Results from a national survey. Journal of Psychiatric Research , 135 , 212–217.
Hawkley, L. C., & Cacioppo, J. T. (2010). Loneliness matters: A theoretical and empirical review of consequences and mechanisms. Annals of Behavioral Mmedicine , 40 (2), 218–227.
Hayes, A. F. (2017). Introduction to mediation, moderation, and conditional process analysis: A regression-based approach . Guilford.
Hayes, A. F., & Scharkow, M. (2013). The relative trustworthiness of inferential tests of the indirect effect in statistical mediation analysis: Does method really matter? Psychological Science , 24 (10), 1918–1927.
Helgeson, V. S., Snyder, P., & Seltman, H. (2004). Psychological and physical adjustment to breast cancer over 4 years: Identifying distinct trajectories of change. Health Psychology , 23 (1), 3.
Henselmans, I. (2009). Psychological Well-being and Perceived Control after a Brest Cancer diagnosis . University Library Groningen.
Hobfoll, S. E. (1989). Conservation of resources: A new attempt at conceptualizing stress. American Psychologist , 44 (3), 513.
Hobfoll, S. E. (2002). Social and psychological resources and adaptation. Review of General Psychology , 6 (4), 307–324.
Hobfoll, S. E., Freedy, J., Lane, C., & Geller, P. (1990). Conservation of social resources: Social support resource theory. Journal of Social and Personal Relationships , 7 (4), 465–478.
Holahan, C. J., Moos, R. H., Holahan, C. K., & Cronkite, R. C. (1999). Resource loss, resource gain, and depressive symptoms: A 10-year model. Journal of Personality and Social Psychology , 77 (3), 620.
Jiang, Q. (2001). Perceived social support scale. Chinese Journal of Behavioral Medicine and Brain Science , 10 (10), 41–43.
Karademas, E. C. (2006). Self-efficacy, social support and well-being: The mediating role of optimism. Personality and Individual Differences , 40 (6), 1281–1290.
Killgore, W. D., Cloonen, S. A., Taylor, E. C., & Dailey, N. S. (2020). Loneliness: A signature mental health concern in the era of COVID-19. Psychiatry Research , 113117.
Krendl, A. C., & Perry, B. L. (2020). The impact of sheltering in place during the COVID-19 pandemic on older adults’ social and mental well-being. The Journals of Gerontology Series B: Psychological Sciences and Social Sciences .
Ladd, G. W., & Ettekal, I. (2013). Peer-related loneliness across early to late adolescence: Normative trends, intra-individual trajectories, and links with depressive symptoms. Journal of Adolescence , 36 (6), 1269–1282.
Lasgaard, M., Nielsen, A., Eriksen, M. E., & Goossens, L. (2010). Loneliness and social support in adolescent boys with autism spectrum disorders. Journal of Autism and Developmental Disorders , 40 (2), 218–226.
Lee, C. Y. S., & Goldstein, S. E. (2016). Loneliness, stress, and social support in young adulthood: Does the source of support matter? Journal of Youth and Adolescence , 45 (3), 568–580.
Liu, L., Gou, Z., & Zuo, J. (2016). Social support mediates loneliness and depression in elderly people. Journal of Health Psychology , 21 (5), 750–758.
Long, J. S., & Ervin, L. H. (2000). Using heteroscedasticity consistent standard errors in the linear regression model. The American Statistician , 54 (3), 217–224.
Luo, Y., & Waite, L. J. (2014). Loneliness and mortality among older adults in China. Journals of Gerontology Series B: Psychological Sciences and Social Sciences , 69 (4), 633–645.
Maes, M., Nelemans, S. A., Danneel, S., Fernández-Castilla, B., Van den Noortgate, W., Goossens, L., & Vanhalst, J. (2019). Loneliness and social anxiety across childhood and adolescence: Multilevel meta-analyses of cross-sectional and longitudinal associations. Developmental Psychology , 55 (7), 1548.
Meaklim, H., Junge, M. F., Varma, P., Finck, W. A., & Jackson, M. L. (2021). Pre-existing and post-pandemic insomnia symptoms are associated with high levels of stress, anxiety and depression globally during the COVID-19 pandemic. Journal of Clinical Sleep Medicine , jcsm–9354.
Miller, G. (2020). Social distancing prevents infections, but it can have unintended consequences. Science .
Newall, N. E., Chipperfield, J. G., & Bailis, D. S. (2014). Predicting stability and change in loneliness in later life. Journal of Social and Personal Relationships , 31 (3), 335–351.
Newman, A., Bavik, Y. L., Mount, M., & Shao, B. (2021). Data collection via online platforms: Challenges and recommendations for future research. Applied Psychology , 70 (3), 1380–1402.
Okruszek, L., Aniszewska-Stańczuk, A., Piejka, A., Wiśniewska, M., & Żurek, K. (2020). Safe but lonely? Loneliness, mental health symptoms and COVID-19.
Palgi, Y., Shrira, A., Ring, L., Bodner, E., Avidor, S., Bergman, Y., & Hoffman, Y. (2020). The loneliness pandemic: Loneliness and other concomitants of depression, anxiety and their comorbidity during the COVID-19 outbreak. Journal of Affective disorders .
Pallant, J. F. (2000). Development and validation of a scale to measure perceived control of internal states. Journal of Personality Assessment , 75 (2), 308–337.
Park, C., Rosenblat, J. D., Brietzke, E., Pan, Z., Lee, Y., Cao, B., & McIntyre, R. S. (2019). Stress, epigenetics and depression: A systematic review. Neuroscience & Biobehavioral Reviews , 102 , 139–152.
Perlman, D., & Peplau, L. A. (1981). Toward a social psychology of loneliness. Personal Relationships , 3 , 31–56.
Preacher, K. J., & Kelley, K. (2011). Effect size measures for mediation models: Quantitative strategies for communicating indirect effects. Psychological Methods , 16 (2), 93.
Qualter, P., Brown, S. L., Munn, P., & Rotenberg, K. J. (2010). Childhood loneliness as a predictor of adolescent depressive symptoms: An 8-year longitudinal study. European Child & Adolescent Psychiatry , 19 (6), 493–501.
Ren, X. (2020). Pandemic and lockdown: A territorial approach to COVID-19 in China, Italy and the United States. Eurasian Geography and Economics , 61 (4–5), 423–434.
Richard, A., Rohrmann, S., Vandeleur, C. L., Schmid, M., Barth, J., & Eichholzer, M. (2017). Loneliness is adversely associated with physical and mental health and lifestyle factors: Results from a Swiss national survey. PloS One , 12(7), e0181442.
Rossi, R., Socci, V., Talevi, D., Mensi, S., Niolu, C., Pacitti, F., & Di Lorenzo, G. (2020). COVID-19 pandemic and lockdown measures impact on mental health among the general population in Italy. Frontiers in Psychiatry , 11 , 790.
Rubinstein, G. (2004). Locus of control and helplessness: Gender differences among bereaved parents. Death Studies , 28 (3), 211–223.
Russell, D., Peplau, L. A., & Cutrona, C. E. (1980). The revised UCLA Loneliness Scale: Concurrent and discriminant validity evidence. Journal of Personality and Social Psychology , 39 (3), 472.
Saltzman, L. Y., Hansel, T. C., & Bordnick, P. S. (2020). Loneliness, isolation, and social support factors in post-COVID-19 mental health. Psychological Trauma: Theory Research Practice and Policy , 12 (S1), S55.
Sarason, I. G., Levine, H. M., Basham, R. B., & Sarason, B. R. (1983). Assessing social support: The social support questionnaire. Journal of Personality and Social Psychology , 44 (1), 127.
Siedlecki, K. L., Salthouse, T. A., Oishi, S., & Jeswani, S. (2014). The relationship between social support and subjective well-being across age. Social Indicators Research , 117 (2), 561–576.
Skinner, E. A. (1995). Perceived control, motivation, & coping (Vol. 8). Sage.
Smith, E. L., & Neupert, S. D. (2020). Daily Stressor exposure: Examining interactions of delinquent networks, Daily Mindfulness and Control among emerging adults. Current Psychology , 1–9.
Stravynski, A., & Boyer, R. (2001). Loneliness in relation to suicide ideation and parasuicide: A population-wide study. Suicide and Life-threatening Behavior , 31 (1), 32–40.
Taylor, S. E., & Stanton, A. L. (2007). Coping resources, coping processes, and mental health. Annu Rev Clin Psychol , 3 , 377–401.
Thompson, S. C., Sobolew-Shubin, A., Galbraith, M. E., Schwankovsky, L., & Cruzen, D. (1993). Maintaining perceptions of control: Finding perceived control in low-control circumstances. Journal of Personality and Social Psychology , 64 (2), 293.
Twenge, J. M., Baumeister, R. F., DeWall, C. N., Ciarocco, N. J., & Bartels, J. M. (2007). Social exclusion decreases prosocial behavior. Journal of Personality and Social Psychology , 92 (1), 56.
Winningham, R. G., & Pike, N. L. (2007). A cognitive intervention to enhance institutionalized older adults’ social support networks and decrease loneliness. Aging & Mental Health , 11 (6), 716–721.
Yan, L., Gan, Y., Ding, X., Wu, J., & Duan, H. (2021). The relationship between perceived stress and emotional distress during the COVID-19 outbreak: Effects of boredom proneness and coping style. Journal of Anxiety Disorders , 77 , 102328.
You, S., Hong, S., & Ho, H. Z. (2011). Longitudinal effects of perceived control on academic achievement. The Journal of Educational Research , 104 (4), 253–266.
Yue, C., Liu, C., Wang, J., Zhang, M., Wu, H., Li, C., & Yang, X. (2020). Association between social support and anxiety among pregnant women in the third trimester during the coronavirus disease 2019 (COVID-19) epidemic in Qingdao, China: The mediating effect of risk perception. The International Journal of Social Psychiatry .
Zhao, X., & Shi, C. (2020). Loneliness of adult and juvenile prisoner influences on psychological affect: Mediation role of control source. Journal of Investigative Psychology and Offender Profiling , 17 (2), 93–100.
Zhou, L., Li, Z., Hu, M., & Xiao, S. (2012). Reliability and validity of ULS-8 loneliness scale in elderly samples in a rural community. Zhong Nan Da Xue Xue bao Yi xue ban = Journal of Central South University Medical Sciences , 37 (11), 1124–1128.
PubMed Google Scholar
Zhou, S. J., Wang, L. L., Qi, M., Yang, X. J., Gao, L., Zhang, S. Y.,... & Chen, J. X. (2021). Depression, anxiety, and suicidal ideation in Chinese university students during the COVID-19 pandemic. F rontiers in Psychology , 12 , 669833.
Zimet, G. D., Dahlem, N. W., Zimet, S. G., & Farley, G. K. (1988). The multidimensional scale of perceived social support. Journal of Personality Assessment , 52 (1), 30–41.
Download references
Acknowledgements
This work was supported by the [Shenzhen-Hong Kong Institute of Brain Science-Shenzhen Fundamental Research Institutions] under Grant [2019SHIBS0003]; [Natural Science Foundation of Guangdong Province] under Grant [2022A151501097]; [National Natural Science Foundation of China] under Grant [31920103009].
Author information
Authors and affiliations.
Center for Brain Disorder and Cognitive Science, Shenzhen University, 3688#, Nanhai Avenue, Nanshan District, Shenzhen, 518060, China
Linlin Yan, Xu Ding, Jianhui Wu & Hongxia Duan
School of Psychological and Cognitive Sciences, Beijing Key Laboratory of Behavior and Mental Health, Peking University, Beijing, China
Faculty of Education, Beijing Normal University, Beijing, China
Lab for Educational Neuroscience, Center for Educational Science and Technology, Beijing Normal University, Beijing, China
Shenzhen Institute of Neuroscience, Shenzhen, China
Jianhui Wu & Hongxia Duan
Donders Institute for Brain, Cognition and Behavior, Radboud University Medical Center, Nijmegen, The Netherlands
Hongxia Duan
You can also search for this author in PubMed Google Scholar
Corresponding author
Correspondence to Hongxia Duan .
Ethics declarations
Interest statement.
On behalf of all authors, the corresponding author states that there is no conflict of interest.
Additional information
Publisher’s note.
Springer Nature remains neutral with regard to jurisdictional claims in published maps and institutional affiliations.

Electronic supplementary material
Below is the link to the electronic supplementary material.
Supplementary Material 1
Rights and permissions.
Open Access This article is licensed under a Creative Commons Attribution 4.0 International License, which permits use, sharing, adaptation, distribution and reproduction in any medium or format, as long as you give appropriate credit to the original author(s) and the source, provide a link to the Creative Commons licence, and indicate if changes were made. The images or other third party material in this article are included in the article’s Creative Commons licence, unless indicated otherwise in a credit line to the material. If material is not included in the article’s Creative Commons licence and your intended use is not permitted by statutory regulation or exceeds the permitted use, you will need to obtain permission directly from the copyright holder. To view a copy of this licence, visit http://creativecommons.org/licenses/by/4.0/ .
Reprints and permissions
About this article
Yan, L., Ding, X., Gan, Y. et al. The impact of loneliness on depression during the COVID-19 pandemic in China: a two-wave follow-up study. Curr Psychol (2024). https://doi.org/10.1007/s12144-024-05898-6
Download citation
Accepted : 17 March 2024
Published : 03 May 2024
DOI : https://doi.org/10.1007/s12144-024-05898-6
Share this article
Anyone you share the following link with will be able to read this content:
Sorry, a shareable link is not currently available for this article.
Provided by the Springer Nature SharedIt content-sharing initiative
Advertisement
- Find a journal
- Publish with us
- Track your research
- Open access
- Published: 11 October 2019
Depression and anxiety among people living with and beyond cancer: a growing clinical and research priority
- Claire L. Niedzwiedz ORCID: orcid.org/0000-0001-6133-4168 1 ,
- Lee Knifton 2 , 3 ,
- Kathryn A. Robb 1 ,
- Srinivasa Vittal Katikireddi 4 &
- Daniel J. Smith 1
BMC Cancer volume 19 , Article number: 943 ( 2019 ) Cite this article
85k Accesses
321 Citations
39 Altmetric
Metrics details
A cancer diagnosis can have a substantial impact on mental health and wellbeing. Depression and anxiety may hinder cancer treatment and recovery, as well as quality of life and survival. We argue that more research is needed to prevent and treat co-morbid depression and anxiety among people with cancer and that it requires greater clinical priority. For background and to support our argument, we synthesise existing systematic reviews relating to cancer and common mental disorders, focusing on depression and anxiety.
We searched several electronic databases for relevant reviews on cancer, depression and anxiety from 2012 to 2019. Several areas are covered: factors that may contribute to the development of common mental disorders among people with cancer; the prevalence of depression and anxiety; and potential care and treatment options. We also make several recommendations for future research. Numerous individual, psychological, social and contextual factors potentially contribute to the development of depression and anxiety among people with cancer, as well as characteristics related to the cancer and treatment received. Compared to the general population, the prevalence of depression and anxiety is often found to be higher among people with cancer, but estimates vary due to several factors, such as the treatment setting, type of cancer and time since diagnosis. Overall, there are a lack of high-quality studies into the mental health of people with cancer following treatment and among long-term survivors, particularly for the less prevalent cancer types and younger people. Studies that focus on prevention are minimal and research covering low- and middle-income populations is limited.
Research is urgently needed into the possible impacts of long-term and late effects of cancer treatment on mental health and how these may be prevented, as increasing numbers of people live with and beyond cancer.
Peer Review reports
A cancer diagnosis can have a wide-ranging impact on mental health and the prevalence of depression and anxiety among people with cancer is high [ 1 , 2 ]. Among those with no previous psychiatric history, a diagnosis of cancer is associated with heightened risk of common mental disorders, which may adversely affect cancer treatment and recovery, as well as quality of life and survival [ 3 ]. People who have previously used psychiatric services may be particularly vulnerable and at greater risk of mortality following a cancer diagnosis [ 4 ]. However, the mental health needs of people with cancer, with or without a prior psychiatric history, are often given little attention during and after cancer treatment, which is primarily focused on monitoring physical health symptoms and side effects. Advances in the earlier detection of cancer and improved cancer treatments means that people are now living longer with cancer, presenting a significant global challenge. The total number of people who are alive within 5 years of a cancer diagnosis was estimated to be 43.8 million in 2018 for 36 cancers across 185 countries [ 5 ], and in the United States alone, the number of cancer survivors is projected to rise exponentially from 15.5 million in 2016 to 26.1 million in 2040 [ 6 ].
The main objective of this article is to argue that more research is needed into the prevention, care and treatment of co-morbid depression and anxiety among people with cancer and highlight it as a growing clinical and policy priority. For background and to support our argument, we provide a current evidence review of systematic reviews relating to common mental disorders amongst people living with and beyond cancer. We cover the factors that may increase the risk of experiencing co-morbid depression and anxiety, epidemiology, and potential care and treatment options.
We searched three key electronic databases: Medline, PsycINFO and CINAHL (Cumulative Index to Nursing and Allied Health Literature) for relevant reviews (favouring those using systematic methods) using the following search terms: (neoplasm OR carcinoma OR tumo*r OR cancer) AND (depression OR anxiety) AND review. Only English language articles were considered and searches were limited to the years 2012 to 2017 and updated during February 2019. These years were considered adequate to capture the main themes relating to cancer and common mental disorders in the current literature. The references of highly relevant articles were scrutinised for additional papers and a Google search for important grey literature was also conducted. A minority of significant research articles known to the authors were also consulted.
Factors influencing the development of depression and anxiety among people with cancer
A variety of factors are likely to interact to influence the development of depression and anxiety among people with cancer (summarised in Fig. 1 ), but these are not well understood [ 1 ], and require further research. Individual risk factors that may increase the risk of depression, similar to the general population, include demographic factors, such as age and gender, and social and economic factors such as unemployment, fewer educational qualifications and a lack of social support [ 7 ]. The development of depression and anxiety among people with cancer is also likely to depend on factors at the structural level, including healthcare costs and access, as well as access to welfare support, such as disability benefits, as cancer can have a significant financial impact [ 8 , 9 ]. Several psychological factors are also important. A key factor is the presence of pre-existing mental health problems and their severity. Research has demonstrated that individuals who have previously accessed mental health services before a cancer diagnosis experience excess mortality due to certain cancers, which may reflect late diagnosis, inadequate treatment and a higher rate of adverse health behaviours [ 4 , 10 ]. Personality factors, such as neuroticism, and existing coping skills may also contribute [ 11 ]. The risk of suicide among people with cancer is higher than the general population for certain diagnoses that tend to have poorer prognoses, such as mesothelioma and lung cancer, especially in the first 6 months after diagnosis [ 12 , 13 ]. Individuals who have previously engaged in suicidal behaviour are likely to be particularly vulnerable.
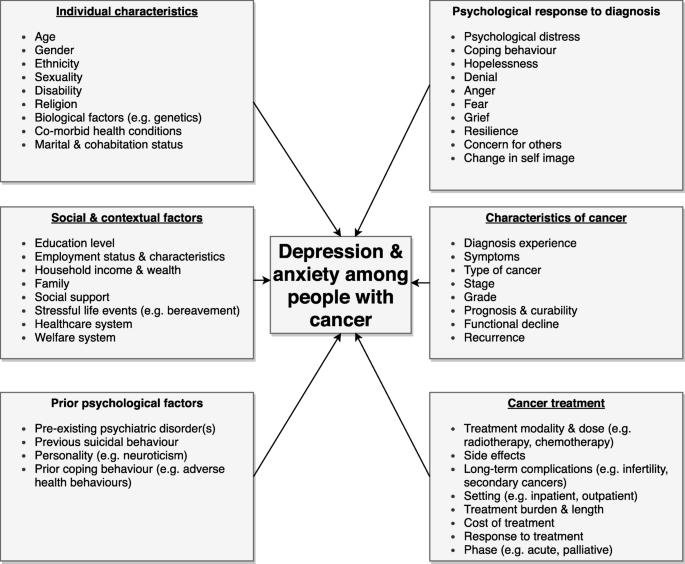
Factors that may contribute to depression and anxiety among people living with and beyond cancer
The individual psychological response to a cancer diagnosis is also likely to be an important component. The experience of being diagnosed, particularly if the diagnosis has been delayed, can be a significant source of distress and can impact on illness acceptance [ 14 ]. Feelings of hopelessness, loss of control and uncertainty around survival and death can also have a detrimental impact, particularly in patients with a poor prognosis. Anxiety around a cancer diagnosis can also lead to sleep disturbance, which may increase the risk of depression [ 15 ]. The stigma surrounding both mental illness and certain types of cancer, such as lung cancer, can lead to feelings of guilt and shame, which could contribute to the onset of depression. For example, the link between smoking and lung cancer can lead to some patients blaming themselves for their illness and experiencing stigma if they have engaged in smoking [ 14 ].
A variety of factors related to the cancer and its treatment are likely to impact on the development of depression and anxiety, including the type of cancer, stage and prognosis. Cancer treatments including immunotherapy and chemotherapy may induce depression through particular biological mechanisms, such as inflammatory pathways, and some medications used to treat chemotherapy-induced nausea can reduce dopaminergic transmission, which is implicated in the development of depressive symptoms [ 16 ]. The use of steroids in cancer treatment can induce depression [ 17 ], and androgen deprivation therapy in the treatment of prostate cancer is also associated with increased risk [ 18 ]. The physical symptoms of specific cancers can also contribute to depression (e.g. incontinence and sexual dysfunction associated with prostate cancer) [ 19 ]. Iatrogenic distress is also commonly reported amongst patients, which could increase the risk of experiencing later problems with depression and anxiety, including post-traumatic stress disorder [ 20 ]. This is often related to a combination of poor communication, a lack of consideration of psychological concerns and disjointed care [ 14 , 20 ].
Prevalence of depression and anxiety among people with cancer
The prevalence of common mental disorders among people with cancer varies widely in the published literature. The mean prevalence of depression using diagnostic interviews is around 13% and using all assessment methods it varies from approximately 4 to 49% [ 2 , 21 ]. This wide variation is due to several factors including the treatment setting, type of cancer included and method used to screen for symptoms (e.g. interview by trained psychiatrist or self-report instrument). The estimated prevalence of depression was found to be 3% in patients with lung cancer, compared to 31% in patients with cancer of the digestive tract, when diagnostic interviews were used [ 21 ]. A meta-analyses of 15 studies meeting a number of quality criteria, including the use of diagnostic interviews, found that the estimated prevalence of depression varied across treatment settings (5 to 16% in outpatients, 4 to 14% in inpatients, 4 to 11% in mixed outpatient and inpatient samples, and 7 to 49% in palliative care) [ 2 ]. There is no universal standardised tool which is recommended for depression screening in patients with cancer and the method used is likely to differ depending on the treatment setting. A meta-analysis of screening and case finding tools for depression in cancer settings identified 63 studies that used 19 different screening tools for depression [ 22 ]. Common screening methods for depression include semi-structured diagnostic interviews, the Hospital Anxiety and Depression Scale - depression subscale (HADS-D) and Center for Epidemiologic Studies Depression Scale (CESD), which are designed to measure the severity of depressive symptoms.
An important aspect that needs to be considered is the timing of increased psychiatric risk. Studies demonstrate that depression tends to be highest during the acute phase and decreases following treatment, but again this likely differs depending on the type of cancer and prognosis [ 21 ]. Using diagnostic interviews, the prevalence of depression during treatment was found to be 14%, 9% in the first year after diagnosis and 8% a year or more after treatment in a meta-analysis of 211 studies [ 21 ]. Of the 238 cohorts included, around 30% included only breast cancer patients and there is a need for research including rarer types of cancer.
As well as the type of cancer, the type of mental health outcome considered is also important and fewer studies have examined anxiety. A systematic review and meta-analysis study focusing on patients with ovarian cancer found that anxiety tended to be higher following treatment (27%) and during treatment (26%), and was lowest pre-treatment (19%) [ 23 ]. The heightened anxiety observed post-treatment may be due to reduced clinical consultations and support following treatment, potential transfer to a palliative setting, and fear of recurrence. Fear of recurrence is one of the most commonly reported issues and an important area of unmet need for cancer survivors [ 24 ]. A lack of outward physical symptoms in ovarian cancer also means that self-monitoring is difficult [ 23 ]. In the same study of ovarian cancer patients, depression was highest before treatment (25%) and during treatment (23%), and reduced following treatment (13%). This is in the context of a lifetime prevalence for clinical depression and anxiety of around 10 and 8%, respectively, amongst women in the UK [ 23 , 25 ].
A similar systematic review of depression and anxiety among patients with prostate cancer found that anxiety tended to be highest pre-treatment (27%) and lowered during treatment (15%) and post-treatment (18%) [ 26 ]. Rates of depression were relatively similar following treatment (18%), during treatment (15%) and pre-treatment (17%), with the 95% confidence intervals for these estimates largely overlapping. For reference, the prevalence of clinical depression and anxiety in men aged over 65 years is less than 9 and 6%, respectively [ 26 ]. A systematic review on the prevalence of psychological distress among testicular cancer survivors demonstrated that around one in five experienced clinically significant anxiety, compared to one in eight among general population controls, with fear of recurrence again being one of the key issues reported [ 27 ]. However, depression was no more prevalent amongst those surviving testicular cancer compared to the general population. In Scotland, the prevalence of depression was found to be highest in patients with lung cancer (13%), followed by gynaecological cancer (11%), breast cancer (9%), colorectal cancer (7%), and genitourinary cancer (6%) [ 28 ]. The authors found depression to be more likely among younger and more socially disadvantaged individuals. In addition, 73% of the patients with depression were not receiving treatment for their mental health. Further research is needed to ascertain the factors which contribute to the uptake and efficacy of treatment for depression. This study also only considered people with cancer who had attended specialist cancer clinics within a defined time period, which likely excluded people who were diagnosed many years ago.
The longer-term psychological impact of cancer has received comparatively little research. The few studies in this area have mainly focused on women with breast cancer and demonstrate that depressive symptoms can persist for over 5 years after diagnosis, though the prevalence of anxiety was not elevated compared to the general population [ 29 ]. A systematic review of the prevalence of depression and anxiety among long-term cancer survivors, including all types, found that anxiety was more prevalent among cancer survivors, compared to healthy controls [ 30 ]. Few studies have focused specifically on younger cancer survivors and more research is needed in this area. A representative study of young adult cancer survivors aged 15 to 39 years in the United States demonstrated that moderate (23% vs 17%) and severe (8% vs 3%) mental distress were significantly higher in those living with cancer for at least 5 years after diagnosis, compared to controls [ 31 ]. 75 and 52% of people with cancer with moderate and severe distress, respectively, had not talked to a mental health professional, with the cost of treatment a potential barrier. Limitations of this study included the focus on self-reported mental distress and not clinical depression or anxiety, as well as the relatively small sample size.
Many studies in this area have a poor response rate, lack representativeness, are based on a small sample of patients (often with the most common types of cancer), which often exclude those with cognitive impairment and patients who are too physically or mentally unwell to take part [ 32 ]. Future studies would benefit from using administrative health data [ 33 ], for example, linking together cancer registries, inpatient and outpatient records and prescribing data. There are also a lack of studies covering populations from low- and middle-income countries [ 34 ]. The estimated prevalence of comorbid common mental disorders is likely to vary depending on the country studied, due to factors such as the health and welfare system. These factors may influence mental health inequalities among people with cancer, which has received little research focus. In a Scottish study, depression was found to be higher in the least advantaged groups (19%), compared to the most advantaged (10%) [ 35 ]. Cancer and comorbid anxiety was also unequally distributed; in the least advantaged groups around 12% had both conditions, compared to 7% among the most advantaged [ 35 ]. Further research is needed in this area to quantify, monitor and prevent inequalities among people with cancer.
It should also be highlighted that the psychological impact of cancer may not always be negative and many people will not experience problems with depression and anxiety. Experiencing temporary distress related to a cancer diagnosis may lead to positive psychological changes in the long-term whereby individuals feel a greater appreciation of life and are able to re-evaluate their priorities [ 36 ]. The factors that protect against the development of common mental disorders and contribute to positive mental health among people living with and beyond cancer merits further research.
Treatment and management of depression and anxiety among people with cancer
To effectively manage and treat depression and anxiety among people with cancer, symptoms must first be identified. However, several social and clinical barriers have been reported. A key issue is the lack of physician time for assessing symptoms. There can also be a normalisation of distress and attribution of the somatic symptoms of depression and anxiety to the cancer. Patients may not disclose psychiatric symptoms because of the stigma surrounding mental health conditions [ 37 ]. Screening for depression and anxiety among patients with cancer is also only of value if it leads to effective treatment and support that is able to improve patient outcomes. Patients may be more reluctant to discuss their mental health needs if they perceive a lack of effective treatment options.
The existing evidence for treating anxiety and depression among patients with cancer is limited and of varying quality [ 38 ]. Studies with small sample sizes are common; this mitigates against the detection of meaningful changes in patient outcomes and these studies often suffer from a high rate of attrition, which likely reflects the high symptom burden and reduced survival in this patient population [ 39 ]. Systematic reviews demonstrate there is a preponderance of studies from the United States, which include a high number of studies focusing on female patients with breast cancer [ 40 ]. However, these studies demonstrate that psychotherapy, psychoeducation and relaxation training may have small to medium short-term effects on relieving emotional distress and reducing symptoms of anxiety and depression, as well as improving health-related quality of life. The evidence for pharmacological treatment of depression with antidepressants is mixed - there are very few studies in this area and those that exist are of low quality [ 41 ]. There is also concern around potential side effects of antidepressants and drug interactions that may affect the efficacy of cancer treatments [ 42 ].
A systematic review and meta-analysis focusing on cognitive behavioural therapy (CBT) found that it may be effective in reducing depression and anxiety and improving quality of life in patients with cancer in the short-term, but potential long-term effects were only sustained for quality of life [ 43 ]. However, in this meta-analysis the included participants were primarily women with breast cancer and there are a lack of studies covering other cancer types. It is likely that collaborative care interventions which involve partnership between psychiatry, clinical psychology and primary care, overseen by a care manager are likely to be most effective in the management and treatment of depression amongst people with cancer [ 44 ]. Treatment should be based on patient preference and also take into account potential adverse side effects [ 44 ]. In a UK-based study it was found that only a third of patients with cancer and related psychological or emotional distress were willing to be referred for support [ 45 ]. Qualitative studies also demonstrate that patients often do not want to discuss their feelings with nurses during cancer treatment [ 46 ]. However, patients valued having the option to talk about their emotions, but they preferred to choose with whom and when. There is therefore a need for further research into some of the barriers to obtaining mental health support among those affected by cancer and experiencing distress to prevent future problems.
The self-management of psychological distress among people with cancer may be beneficial and could help prevent distress becoming clinical depression or anxiety. Self-management can be defined as: “The individual’s ability to manage the symptoms, treatment, physical and psychosocial consequences and lifestyle changes inherent in living with a chronic condition. Efficacious self-management encompasses the ability to monitor one’s condition and to affect the cognitive, behavioural and emotional responses necessary to maintain a satisfactory quality of life. Thus, a dynamic and continuous process of self-regulation is established.” [ 47 ] . Studies on self-management, cancer and psychological distress have focused on the treatment phase, with fewer investigating interventions following treatment or at the end of life [ 48 ]. There is evidence to suggest that self-management of psychological distress in cancer can help to empower patients and families to care for themselves in a way which is preferable for them. Self-management interventions that have shown promise include education, monitoring, teaching and counselling to help patients manage the short- and long-term physical and psychosocial effects of cancer [ 48 ]. However, a recent systematic review examining the impact of self-management interventions on outcomes including quality of life, self-efficacy and symptom management (such as psychological distress) amongst cancer survivors demonstrated a lack of evidence to support any specific intervention and found that the six included interventions lacked sustainability, bringing into question their long-term effectiveness and value for money [ 49 ]. Again, the included studies were dominated by women with breast cancer, with only two covering other cancers.
Effective treatment and management strategies may also differ according to the demographic group affected. In a report by CLIC (Cancer and Leukaemia in Childhood) Sargent which surveyed 146 young people with cancer, keeping in touch with friends and family, talking to others with similar experiences and access to the internet in hospital were reported to help maintain mental health during cancer treatment [ 50 ]. Of the young people who mentioned they would find it helpful to talk to other people with similar experiences, 60% said they would prefer to do this online. Young people also reported that the available services were not tailored to deal with those aged under 18 or the emotional impact of cancer. In addition, those who accessed services mentioned that there is a lack of suitable long-term emotional support. Just over 40% of the young people who took part did not access support for their mental health needs.
It is clear that a more personalised approach to supporting the psychological health of people with cancer is needed [ 51 ]. Some people may not want or require support or treatment, others will be able to self-manage, and some may have more complex needs that require more intensive follow-up and support. At diagnosis, the psychological health of patients should be considered alongside their physical health and sources of support offered. Needs and symptoms may also change over time. Evaluation of more recent personalised approaches to follow-up care that have been adopted in several areas including England and Northern Ireland [ 51 ] are needed to understand the role they may have in preventing longer term depression and anxiety amongst cancer survivors.
A key barrier affecting research progress in this area is funding [ 52 ]. In the UK, money spent on research into the biology of cancer was more than five times than that spent on ‘Cancer Control, Survivorship and Outcomes’ during 2017/18 [ 53 ]. Research into the mental health and wellbeing of people living with and beyond cancer is likely to only be a small part of this. Research is urgently needed in this area as more people survive cancer and for some cancers, such as multiple myeloma and colorectal cancer, risk is increasing in younger cohorts [ 54 ]. The long-term (those that begin during treatment and continue afterwards) and late effects of cancer treatment (those that begin after treatment is completed), such as secondary cancers, infertility, chronic pain and insomnia, are likely to affect the mental wellbeing of cancer survivors, potentially contributing to depression and anxiety [ 6 ]. The National Cancer Research Institute (NCRI) in the UK have also recently highlighted research into the short-term and long-term psychological impacts of cancer and its treatment as a key priority, following surveys of over 3500 patients, carers, and health and social care professionals [ 55 ].
The mental health of people living with and beyond cancer in its various types and stages is an important and growing research and clinical priority. Compared to the general population, the prevalence of anxiety and depression is often higher among people with cancer, but estimates vary due to a number of factors, such as the type and stage of cancer. Patients often do not obtain psychological support or treatment. This is likely due to several factors, including lack of awareness and identification of psychiatric symptoms, an absence of support available or offered, lack of evidence around effective treatments, stigma, and patient preference. In particular, we highlight the lack of high-quality research into the mental health of long-term cancer survivors, the potential impact of long-term and late effects of cancer treatment, and the few studies focused on prevention. Further research that includes the less common types of cancer is required, as well as the inclusion of younger people and populations from low- and middle-income countries. Given the increasing numbers of people living with and beyond cancer, this research is of timely importance.
Availability of data and materials
All data generated or analysed during this study are included in this published article.
Abbreviations
Cognitive behavioural therapy
Center for Epidemiologic Studies Depression Scale
Cumulative Index to Nursing and Allied Health Literature
Cancer and Leukaemia in Childhood
Hospital Anxiety and Depression Scale - depression subscale
National Cancer Research Institute
Pitman A, Suleman S, Hyde N, Hodgkiss A. Depression and anxiety in patients with cancer. BMJ. 2018;361:k1415.
Article PubMed Google Scholar
Walker J, Hansen CH, Martin P, Sawhney A, Thekkumpurath P, Beale C, Symeonides S, Wall L, Murray G, Sharpe M. Prevalence of depression in adults with cancer: a systematic review. Ann Oncol. 2013;24(4):895–900.
Article CAS PubMed Google Scholar
Zhu J, Fang F, Sjölander A, Fall K, Adami HO, Valdimarsdóttir U. First-onset mental disorders after cancer diagnosis and cancer-specific mortality: a nationwide cohort study. Ann Oncol. 2017;28(8):1964–9.
Klaassen Z, Wallis CJD, Goldberg H, Chandrasekar T, Sayyid RK, Williams SB, Moses KA, Terris MK, Nam RK, Urbach D, et al. The impact of psychiatric utilisation prior to cancer diagnosis on survival of solid organ malignancies. Br J Cancer. 2019;120:840–7.
Article PubMed PubMed Central Google Scholar
Bray F, Ferlay J, Soerjomataram I, Siegel RL, Torre LA, Jemal A. Global cancer statistics 2018: GLOBOCAN estimates of incidence and mortality worldwide for 36 cancers in 185 countries. CA Cancer J Clin. 2018;68(6):394–424.
Shapiro CL. Cancer survivorship. N Engl J Med. 2018;379(25):2438–50.
Wen S, Xiao H, Yang Y. The risk factors for depression in cancer patients undergoing chemotherapy: a systematic review. Support Care Cancer. 2019;27(1):57–67.
Gilligan AM, Alberts DS, Roe DJ, Skrepnek GH. Death or Debt? National Estimates of Financial Toxicity in Persons with Newly-Diagnosed Cancer. Am J Med. 2018;131(10):1187–99 e1185.
Lu L, O'Sullivan E, Sharp L. Cancer-related financial hardship among head and neck cancer survivors: Risk factors and associations with health-related quality of life. Psycho-Oncology. 2019;28(4):863–71.
Musuuza JS, Sherman ME, Knudsen KJ, Sweeney HA, Tyler CV, Koroukian SM. Analyzing excess mortality from cancer among individuals with mental illness. Cancer. 2013;119(13):2469–76.
Cook SA, Salmon P, Hayes G, Byrne A, Fisher PL. Predictors of emotional distress a year or more after diagnosis of cancer: a systematic review of the literature. Psycho-oncology. 2018;27(3):791–801.
Henson KE, Brock R, Charnock J, Wickramasinghe B, Will O, Pitman A. Risk of suicide after cancer diagnosis in England. JAMA Psychiatry. 2019;76(1):51–60.
Wang SM, Chang JC, Weng SC, Yeh MK, Lee CS. Risk of suicide within 1 year of cancer diagnosis. Int J Cancer. 2018;142(10):1986–93.
Ball H, Moore S, Leary A. A systematic literature review comparing the psychological care needs of patients with mesothelioma and advanced lung cancer. Eur J Oncol Nurs. 2016;25:62–7.
Howell D, Harris C, Aubin M, Olson K, Sussman J, MacFarlane J, Taylor C, Oliver TK, Keller-Olaman S, Davidson JR, et al. Sleep disturbance in adults with cancer: a systematic review of evidence for best practices in assessment and management for clinical practice. Ann Oncol. 2013;25(4):791–800.
Smith HR. Depression in cancer patients: pathogenesis, implications and treatment (review). Oncol Lett. 2015;9(4):1509–14.
Article CAS PubMed PubMed Central Google Scholar
Ismail MF, Lavelle C, Cassidy EM. Steroid-induced mental disorders in cancer patients: a systematic review. Future Oncol. 2017;13(29):2719–31.
Nead KT, Sinha S, Yang DD, Nguyen PL. Association of androgen deprivation therapy and depression in the treatment of prostate cancer: A systematic review and meta-analysis. Urologic Oncol. 2017;35(11):664 e661–664.e669.
CAS Google Scholar
De Sousa A, Sonavane S, Mehta J. Psychological aspects of prostate cancer: a clinical review. Prostate Cancer Prostatic Dis. 2012;15(2):120–7.
Cordova MJ, Riba MB, Spiegel D. Post-traumatic stress disorder and cancer. Lancet Psychiatry. 2017;4(4):330–8.
Krebber AMH, Buffart LM, Kleijn G, Riepma IC, de Bree R, Leemans CR, Becker A, Brug J, van Straten A, Cuijpers P, et al. Prevalence of depression in cancer patients: a meta-analysis of diagnostic interviews and self-report instruments. Psycho-Oncology. 2014;23(2):121–30.
Mitchell AJ, Meader N, Davies E, Clover K, Carter GL, Loscalzo MJ, Linden W, Grassi L, Johansen C, Carlson LE, et al. Meta-analysis of screening and case finding tools for depression in cancer: evidence based recommendations for clinical practice on behalf of the depression in Cancer care consensus group. J Affect Disord. 2012;140(2):149–60.
Watts S, Prescott P, Mason J, McLeod N, Lewith G. Depression and anxiety in ovarian cancer: a systematic review and meta-analysis of prevalence rates. BMJ Open. 2015;5(11):e007618.
Simard S, Thewes B, Humphris G, Dixon M, Hayden C, Mireskandari S, Ozakinci G. Fear of cancer recurrence in adult cancer survivors: a systematic review of quantitative studies. J Cancer Surviv. 2013;7(3):300–22.
Halliwell E, Main L, Richardson C. The fundamental facts: the latest facts and figures on mental health: mental Health Foundation; 2007.
Google Scholar
Watts S, Leydon G, Birch B, Prescott P, Lai L, Eardley S, Lewith G. Depression and anxiety in prostate cancer: a systematic review and meta-analysis of prevalence rates. BMJ Open. 2014;4(3):e003901.
Smith AB, Rutherford C, Butow P, Olver I, Luckett T, Grimison P, Toner G, Stockler M, King M. A systematic review of quantitative observational studies investigating psychological distress in testicular cancer survivors. Psycho-Oncology. 2018;27(4):1129–37.
Walker J, Hansen CH, Martin P, Symeonides S, Ramessur R, Murray G, Sharpe M. Prevalence, associations, and adequacy of treatment of major depression in patients with cancer: a cross-sectional analysis of routinely collected clinical data. Lancet Psychiatry. 2014;1(5):343–50.
Maass SW, Roorda C, Berendsen AJ, Verhaak PF, de Bock GH. The prevalence of long-term symptoms of depression and anxiety after breast cancer treatment: a systematic review. Maturitas. 2015;82(1):100–8.
Mitchell AJ, Ferguson DW, Gill J, Paul J, Symonds P. Depression and anxiety in long-term cancer survivors compared with spouses and healthy controls: a systematic review and meta-analysis. Lancet Oncol. 2013;14(8):721–32.
Kaul S, Avila JC, Mutambudzi M, Russell H, Kirchhoff AC, Schwartz CL. Mental distress and health care use among survivors of adolescent and young adult cancer: a cross-sectional analysis of the National Health Interview Survey. Cancer. 2017;123(5):869–78.
Ryan D, Gallagher P, Wright S, Cassidy E. Methodological challenges in researching psychological distress and psychiatric morbidity among patients with advanced cancer: what does the literature (not) tell us? Palliat Med. 2012;26(2):162–77.
Lu L, Deane J, Sharp L. Understanding survivors’ needs and outcomes: the role of routinely collected data. Curr Opinion Supportive Palliative Care. 2018;12(3):254–60.
Article Google Scholar
Walker ZJ, Jones MP, Ravindran AV. Psychiatric disorders among people with cancer in low- and lower-middle-income countries: study protocol for a systematic review and meta-analysis. BMJ Open. 2017;7(8):e017043.
Barnett K, Mercer SW, Norbury M, Watt G, Wyke S, Guthrie B. Epidemiology of multimorbidity and implications for health care, research, and medical education: a cross-sectional study. Lancet. 2012;380(9836):37–43.
Casellas-Grau A, Ochoa C, Ruini C. Psychological and clinical correlates of posttraumatic growth in cancer: a systematic and critical review. Psycho-Oncology. 2017;26(12):2007–18.
Kissane DW. Unrecognised and untreated depression in cancer care. Lancet Psychiatry. 2014;1(5):320–1.
Rodin G, Lloyd N, Katz M, Green E, Mackay JA, Wong RK. Supportive care guidelines Group of Cancer Care Ontario Program in evidence-based care: the treatment of depression in cancer patients: a systematic review. Support Care Cancer. 2007;15(2):123–36.
Hui D, Glitza I, Chisholm G, Yennu S, Bruera E. Attrition rates, reasons, and predictive factors in supportive care and palliative oncology clinical trials. Cancer. 2013;119(5):1098–105.
Faller H, Schuler M, Richard M, Heckl U, Weis J, Küffner R. Effects of psycho-oncologic interventions on emotional distress and quality of life in adult patients with cancer: systematic review and meta-analysis. J Clin Oncol. 2013;31(6):782–93.
Ostuzzi G, Matcham F, Dauchy S, Barbui C, Hotopf M. Antidepressants for the treatment of depression in people with cancer. Cochrane Database Syst Rev. 2018;4(4):CD011006.
Ostuzzi G, Benda L, Costa E, Barbui C. Efficacy and acceptability of antidepressants on the continuum of depressive experiences in patients with cancer: systematic review and meta-analysis. Cancer Treat Rev. 2015;41(8):714–24.
Osborn RL, Demoncada AC, Feuerstein M. Psychosocial interventions for depression, anxiety, and quality of life in cancer survivors: meta-analyses. Int J Psychiatry Med. 2006;36(1):13–34.
Li M, Kennedy EB, Byrne N, Gérin-Lajoie C, Katz MR, Keshavarz H, Sellick S, Green E. Systematic review and meta-analysis of collaborative care interventions for depression in patients with cancer. Psycho-Oncol. 2017;26(5):573–87.
Baker-Glenn EA, Park B, Granger L, Symonds P, Mitchell AJ. Desire for psychological support in cancer patients with depression or distress: validation of a simple help question. Psycho-Oncol. 2011;20(5):525–31.
Kvåle K. Do cancer patients always want to talk about difficult emotions? A qualitative study of cancer inpatients communication needs. Eur J Oncol Nurs. 2007;11(4):320–7.
Barlow J, Wright C, Sheasby J, Turner A, Hainsworth J. Self-management approaches for people with chronic conditions: a review. Patient Educ Couns. 2002;48(2):177–87.
McCorkle R, Ercolano E, Lazenby M, Schulman-Green D, Schilling LS, Lorig K, Wagner EH. Self-management: enabling and empowering patients living with cancer as a chronic illness. CA Cancer J Clin. 2011;61(1):50–62.
Boland L, Bennett K, Connolly D. Self-management interventions for cancer survivors: a systematic review. Support Care Cancer. 2018;26(5):1585–95.
PubMed Google Scholar
Sargent CLIC. Hidden costs: the mental health impact of a cancer diagnosis on young people; 2017.
Alfano CM, Mayer DK, Bhatia S, Maher J, Scott JM, Nekhlyudov L, Merrill JK, Henderson TO. Implementing personalized pathways for cancer follow-up care in the United States: proceedings from an American Cancer Society–American Society of Clinical Oncology summit. CA Cancer J Clin. 2019;69(3):234–47.
Foster C, Calman L, Richardson A, Pimperton H, Nash R. Improving the lives of people living with and beyond cancer: generating the evidence needed to inform policy and practice. J Cancer Policy. 2018;15:92–5.
National Cancer Research Institute. Spend by Research Category and Disease Site. [ https://www.ncri.org.uk/ncri-cancer-research-database-old/spend-by-research-category-and-disease-site/ ]. Accessed 27 June 2019.
Sung H, Siegel RL, Rosenberg PS, Jemal A. Emerging cancer trends among young adults in the USA: analysis of a population-based cancer registry. Lancet Public Health. 2019;4(3):e137–47.
National Cancer Research Institute. The UK Top living with and beyond cancer research priorities. [ https://www.ncri.org.uk/lwbc/#lwbc_questions ]. Accessed 27 June 2019.
Download references
Acknowledgements
This article is built on a literature review conducted by CLN and LK for a project on ‘Supporting the mental and emotional health of people with cancer’ funded by the Big Lottery Fund when CLN was an employee of the Mental Health Foundation in Scotland during 2017.
CLN is currently supported by the Medical Research Council (grant number MR/R024774/1). SVK is funded by a NHS Research Scotland (NRS) Senior Clinical Fellowship (SCAF/15/02), the Medical Research Council (MC_UU_12017/13 & MC_UU_12017/15) and Scottish Government Chief Scientist Office (SPHSU13 & SPHSU15). The funders had no role in the study design; collection, analysis and interpretation of data; the writing of the article; and in the decision to submit it for publication.
Author information
Authors and affiliations.
Institute of Health & Wellbeing, University of Glasgow, Glasgow, Scotland, UK
Claire L. Niedzwiedz, Kathryn A. Robb & Daniel J. Smith
University of Strathclyde, Centre for Health Policy, Glasgow, Scotland, UK
Lee Knifton
Mental Health Foundation, Glasgow, Scotland, UK
MRC/CSO Social and Public Health Sciences Unit, University of Glasgow, Glasgow, Scotland, UK
Srinivasa Vittal Katikireddi
You can also search for this author in PubMed Google Scholar
Contributions
CLN and LK conceived the article. CLN conducted the searches and drafted the manuscript. CLN, LK, SVK, KAR and DJS interpreted the findings. All authors critically revised the manuscript, read and approved the final version.
Corresponding author
Correspondence to Claire L. Niedzwiedz .
Ethics declarations
Ethics approval and consent to participate.
Not applicable
Consent for publication
Competing interests.
The authors declare that they have no competing interests except for the funding acknowledged.
Additional information
Publisher’s note.
Springer Nature remains neutral with regard to jurisdictional claims in published maps and institutional affiliations.
Rights and permissions
Open Access This article is distributed under the terms of the Creative Commons Attribution 4.0 International License ( http://creativecommons.org/licenses/by/4.0/ ), which permits unrestricted use, distribution, and reproduction in any medium, provided you give appropriate credit to the original author(s) and the source, provide a link to the Creative Commons license, and indicate if changes were made. The Creative Commons Public Domain Dedication waiver ( http://creativecommons.org/publicdomain/zero/1.0/ ) applies to the data made available in this article, unless otherwise stated.
Reprints and permissions
About this article
Cite this article.
Niedzwiedz, C.L., Knifton, L., Robb, K.A. et al. Depression and anxiety among people living with and beyond cancer: a growing clinical and research priority. BMC Cancer 19 , 943 (2019). https://doi.org/10.1186/s12885-019-6181-4
Download citation
Received : 19 March 2019
Accepted : 20 September 2019
Published : 11 October 2019
DOI : https://doi.org/10.1186/s12885-019-6181-4
Share this article
Anyone you share the following link with will be able to read this content:
Sorry, a shareable link is not currently available for this article.
Provided by the Springer Nature SharedIt content-sharing initiative
- Mental health
- Multimorbidity
- Survivorship
ISSN: 1471-2407
- Submission enquiries: [email protected]
- General enquiries: [email protected]
Psychotherapy for the treatment of depression: a comprehensive review of controlled outcome research
Affiliation.
- 1 Department of Psychology, Memphis State University, Tennessee 38152.
- PMID: 2200072
- DOI: 10.1037/0033-2909.108.1.30
Previous quantitative reviews of research on the efficacy of psychotherapy for depression have included only a subset of the available research or limited their focus to a single outcome measure. The present review offers a more comprehensive quantitative integration of this literature. Using studies that compared psychotherapy with either no treatment or another form of treatment, this article assesses (a) the overall effectiveness of psychotherapy for depressed clients, (b) its effectiveness relative to pharmacotherapy, and (c) the clinical significance of treatment outcomes. Findings from the review confirm that depressed clients benefit substantially from psychotherapy, and these gains appear comparable to those observed with pharmacotherapy. Initial analysis suggested some differences in the efficacy of various types of treatment; however, once the influence of investigator allegiance was removed, there remained no evidence for the relative superiority of any 1 approach. In view of these results, the focus of future research should be less on differentiating among psychotherapies for depression than on identifying the factors responsible for improvement.
Publication types
- Research Support, Non-U.S. Gov't
- Depressive Disorder / psychology
- Depressive Disorder / therapy*
- Follow-Up Studies
- Psychotherapy / methods*
- U.S. Department of Health & Human Services

- Virtual Tour
- Staff Directory
- En Español
You are here
Nih research matters.
January 15, 2019
Physical activity may reduce depression symptoms
At a glance.
- Researchers found that sleep problems, a lack of energy, and physical inactivity may lead to a depressed mood and mood changes.
- The findings reverse conventional wisdom that depression leads to physical inactivity and show that the opposite may be true.
- Physical activity could be an effective target for strategies to change mood states.

Physical activity can help improve your health and quality of life. Not getting enough physical activity can increase your risk for diabetes, heart disease, cancers, and mental health disorders.
Current theories about depression suggest that sleep problems, a lack of energy, and physical inactivity can result from a depressed mood. To investigate the relationship between mood disorders and these factors, a team led by Dr. Kathleen Merikangas at NIH’s National Institute of Mental Health (NIMH) and Dr. Vadim Zipunnikov at John Hopkins University collected real-time measures of physical activity and sleep.
The scientists enrolled 54 adults with bipolar disorder, which involves episodes of depression; 91 with major depressive disorder; and 97 with no history of mood disorders. The study was supported in part by NIMH. Results were published online on December 12, 2018, in JAMA Psychiatry .
For two weeks, the researchers monitored participants’ physical activity using mobile monitoring devices worn around the wrist. They collected minute-by-minute physical activity data and used this information to estimate how long participants slept. Participants also completed diary entries of their mood and energy levels four times a day for two weeks. At each assessment, participants were asked to rate the degree to which they felt “very happy” to “very sad” and “very tired” to “very energetic.”
The researchers found that physical activity affected the participants’ mood afterward, but mood didn’t affect the amount of physical activity they engaged in later. Physical activity also affected how energetic participants felt and how long they slept. These relationships went both ways: energy levels and sleep also affected how much physical activity participants later engaged in.
Among those with bipolar disorder, 25 had a more severe form called bipolar I. The relationships among physical activity, sleep, mood, and energy were substantially stronger in people with this disorder than in other participants, suggesting that people with bipolar-I disorder may react more strongly to changes in these areas of day-to-day life.
The results suggest that physical activity may play a central role in mood regulation, and thus might be an effective target for interventions to change mood states.
“The research team and I are currently conducting additional studies to understand these complex interactions better—repeating the assessments to study the generalizability of these findings over longer periods of time,” Merikangas says.
Related Links
- Mental Disorders May Share Molecular Origins
- Depression Screening and Treatment in Adults
- Placebo Effect in Depression Treatment
- Brain Scan May Predict Best Depression Treatment
- Bipolar Disorder
- Exercise and Physical Activity
- Benefits of Physical Activity
References: Real-time Mobile Monitoring of the Dynamic Associations Among Motor Activity, Energy, Mood, and Sleep in Adults With Bipolar Disorder . Merikangas KR, Swendsen J, Hickie IB, Cui L, Shou H, Merikangas AK, Zhang J, Lamers F, Crainiceanu C, Volkow ND, Zipunnikov V. JAMA Psychiatry . 2018 Dec 12. doi: 10.1001/jamapsychiatry.2018.3546. [Epub ahead of print]. PMID: 30540352.
Funding: NIH’s National Institute of Mental Health (NIMH); Johns Hopkins University; Netherlands Organization for Scientific Research (NWO); and European Union Seventh Framework Programme.
Connect with Us
- More Social Media from NIH
ORIGINAL RESEARCH article
A qualitative investigation of the montgomery–åsberg depression rating scale: discrepancies in rater perceptions and data trends in remote assessments of rapid-acting antidepressants in treatment resistant depression.

- 1 WCG Clinical Endpoint Solutions, Princeton, NJ, United States
- 2 Seton Hall University, South Orange, NJ, United States
- 3 Department of Psychiatry, Whanganui District Health Board, Whanganui, New Zealand
- 4 Chelsea and Westminster Hospital NHS Foundation Trust, London, United Kingdom
- 5 The PANSS Institute, New York, NY, United States
Introduction: Despite the development of many successful pharmaceutical interventions, a significant subset of patients experience treatment-resistant depression (TRD). Ketamine and its derivatives constitute a novel therapeutic approach to treat TRD; however, standard tools, such as the Montgomery–Åsberg Depression Rating Scale (MADRS) are still being used to measure symptoms and track changes.
Methods: The aim of this study was to review item-level differences between rate of data change (MADRS score) and rater-weighted perception of the most useful items for assessing change in symptoms while remotely conducting the 10-item version of the MADRS in TRD in a clinical trial of rapid-acting antidepressants. Two studies of rapid-acting antidepressants in the treatment of TRD were used to identify item-scoring trends when MADRS is administered remotely and repeatedly (733 subjects across 10 visits). Scoring trends were evaluated in tandem to a rater survey completed by 75 raters. This was completed to gain insight on MADRS items’ perceived level of helpfulness when assessing change of symptoms in rapid-acting antidepressant trials.
Results: MADRS items ‘Reduced sleep’, ‘Apparent sadness’, and ‘Pessimistic thoughts’ were found to have the greatest average data change by visit, while raters ranked ‘Reported sadness’, ‘Lassitude’ and ‘Apparent sadness’ as the most helpful items when assessing symptom change.
Discussion: The diversion between rate of data-change ranking and rater perception of helpfulness could be related to difficulty in assessing specific items, to the novel treatment itself, and/or to the sensitivity to symptom change to which raters are accustomed in traditional antidepressant treatments.
1 Introduction
In addressing the challenge of treatment-resistant depression (TRD), this manuscript focuses on the evolving landscape of depression treatment and the role of rapid-acting antidepressants (RAAD), such as ketamine and its derivative, esketamine. These novel treatments, approved by the FDA in 2019 for TRD ( 1 ), constitute a significant advance in the pharmacotherapy of depression, but pose new questions about the effectiveness of traditional assessment tools like the Montgomery–Åsberg Depression Rating Scale (MADRS) in evaluating rapid symptom changes. The 10-item MADRS, a standard in clinical research and practice, is used to measure symptom severity and changes in depressive syndromes, yet its suitability for novel, rapid-acting treatments remains under-explored, particularly in the context of remote administration ( 2 , 3 ).
Depression, a leading contributor to global disability, affects about 322 million people worldwide, with an 18.4% increase in prevalence between 2005 and 2015 ( 4 ). Traditional treatments have evolved significantly since the 1950s, from tricyclic antidepressants and monoamine oxidase inhibitors to second-generation antidepressants like SSRIs and SNRIs. However, for a substantial subset of patients, these treatments fail to provide full or partial remission, leading to the classification of TRD ( 5 – 7 ). The complexity of defining and measuring treatment resistance, as highlighted by studies like the Sequenced Treatment Alternatives to Relieve Depression (STAR*D) trial, underscores the need for effective treatment options and reliable assessment methods ( 8 – 10 ). Rapid-acting antidepressants, a departure from the monoaminergic focus of traditional treatments, offer a promising avenue for treating TRD. Ketamine, antagonizing the NMDA receptor, induces a glutamate surge, fostering brain adaptability and pathway creation ( 11 , 12 ). Its rapid symptom relief, observed within 24 to 72 hours post-administration, marks a stark contrast to the gradual effects of conventional antidepressants ( 13 – 15 ). However, the long-term effects and optimal dosing of ketamine remain areas for further research ( 16 – 18 ).
The MADRS, designed to sensitively capture treatment-induced symptom changes in depression, has historically been administered in-person but is increasingly used in remote settings. This shift raises concerns, particularly for items like ‘Apparent sadness,’ which rely on observational assessment ( 19 – 24 ). Despite its widespread use and proven interrater reliability across various languages, the appropriateness of MADRS for rapid-acting antidepressants, especially in remote settings, warrants examination ( 25 – 35 ). In addition to that RAADs can generate responses within hours or days, rather than weeks or months, while our rating instruments were designed to assess mood symptoms over a 7-day time frame, typically. Consequently, adaptative approaches are required for existing scales to meet the need of adjustment. Yavorsky et al. provide an excellent summary on adaptations to the standard rating instruments allowing to reflect short-term changes in which RAADs act, as well as implementing novel rating measures. Critically, they also discuss limitations and challenges to the currently used rating measures including any conceptual biases of raters ( 36 ).
This publication, therefore, seeks to analyze the item-level differences in MADRS scores and the raters’ perception of their helpfulness in determining symptom severity change in TRD during clinical trials of rapid-acting antidepressants. The study hypothesizes that while some MADRS items may align with rater perceptions, discrepancies are expected due to the unique nature of rapid-acting antidepressant treatment and the challenges of remote assessment. This investigation is crucial for ensuring that depression assessment tools remain relevant and effective in the rapidly evolving landscape of antidepressant therapy.
2 Materials and methods
2.1 data change on madrs items in two protocols of a phase 3 study.
We have established the rate of change of all items of MADRS for the entirety of two separate protocols of a Phase 3 study of rapid-acting antidepressants using a similar design, but varying in terms visit frequency (between 24 hours and 7 days). We used deidentified datasets only containing the visit date, but no other information that would be considered protected health information, only to perform a qualitative, exploratory analysis looking at individual MADRS items and changes in their score across visits. Rate of change was calculated by first determining between-visit MADRS data change by item; we then divided the per-item change by the number of days that had passed between an individual visit and the previous visit to establish change per day. We rank-ordered the per-visit change for a given item. The data was solely used to create the rankings discussed, and no other quantitative analysis has been completed for the purpose of this study.
2.2 Remote rater-experience survey
We conducted a survey of clinical research professionals who have participated in the above-mentioned Phase 3 programs of rapid-acting antidepressants utilizing new modalities for the assessment of depressive symptoms, including remote evaluation technologies (telepsychiatry) and versions of MADRS that have been adapted for use over shorter recall periods, e.g. last 24 hours. A total of 75 experienced raters from 13 countries were recruited for this survey study, all of whom participated in at least one TRD clinical trial for rapid-acting antidepressants. All survey participants were polled about which MADRS items they considered most and least helpful for assessing changes in symptoms in two protocols of a Phase 3 study with the same rapid-acting agent to treat TRD. Many raters participated in these studies on almost a daily basis, with a rotation of subjects. Items considered as most useful as endorsed by raters for assessing TRD related symptom changes were ranked as most important, second most important, and so on.
We compared the data change on MADRS items and the raters’ rankings to gain additional insight into a.) the rater experience of each item’s helpfulness in determining depression severity and b.) the rate of change in MADRS scores in a clinical trial for rapid-acting antidepressants in TRD.
Surveyed raters conducted assessments via telephone to ensure the integrity of blinding in these trials. Raters were selected from studies with more than 700 subjects combined (N = 733) across 20 countries and 16 different languages. In the two examined protocols of a Phase 3 study with the same rapid-acting agent to treat TRD, 733 subjects were included in the dataset across 10 visits (excluding early termination; 12 in visits in total). MADRS total scores ranged from 0 to 53 across visits for each protocol. Mean total MADRS score was 30.39. Individual item scores ranged from 0 to 6 for each item. Ranking of items was determined by rater perception of helpfulness in assessing symptom change, and by average MADRS score change in between-visit data. Rater ranking order (from 10 to 1) correlates with an increasing level of perceived helpfulness in determining symptom change, with 1 being the most helpful. Data ranking of MADRS items based on the deidentified dataset (from 10 to 1) correlates with a greater average rate of MADRS item-level score change between visits, with 1 representing the item with the greatest average between-visit MADRS item score change. Helpfulness of MADRS items in assessing symptom change, ranked according to average rate of data change and by rater perception of helpfulness is shown in Figure 1 , with a ranking in the first position representing the MADRS item with either the highest rate of symptom change according to greatest average between-visit MADRS data change (‘By data change’) or as being perceived as the most helpful by raters (‘By rater perception’) when assessing symptoms.
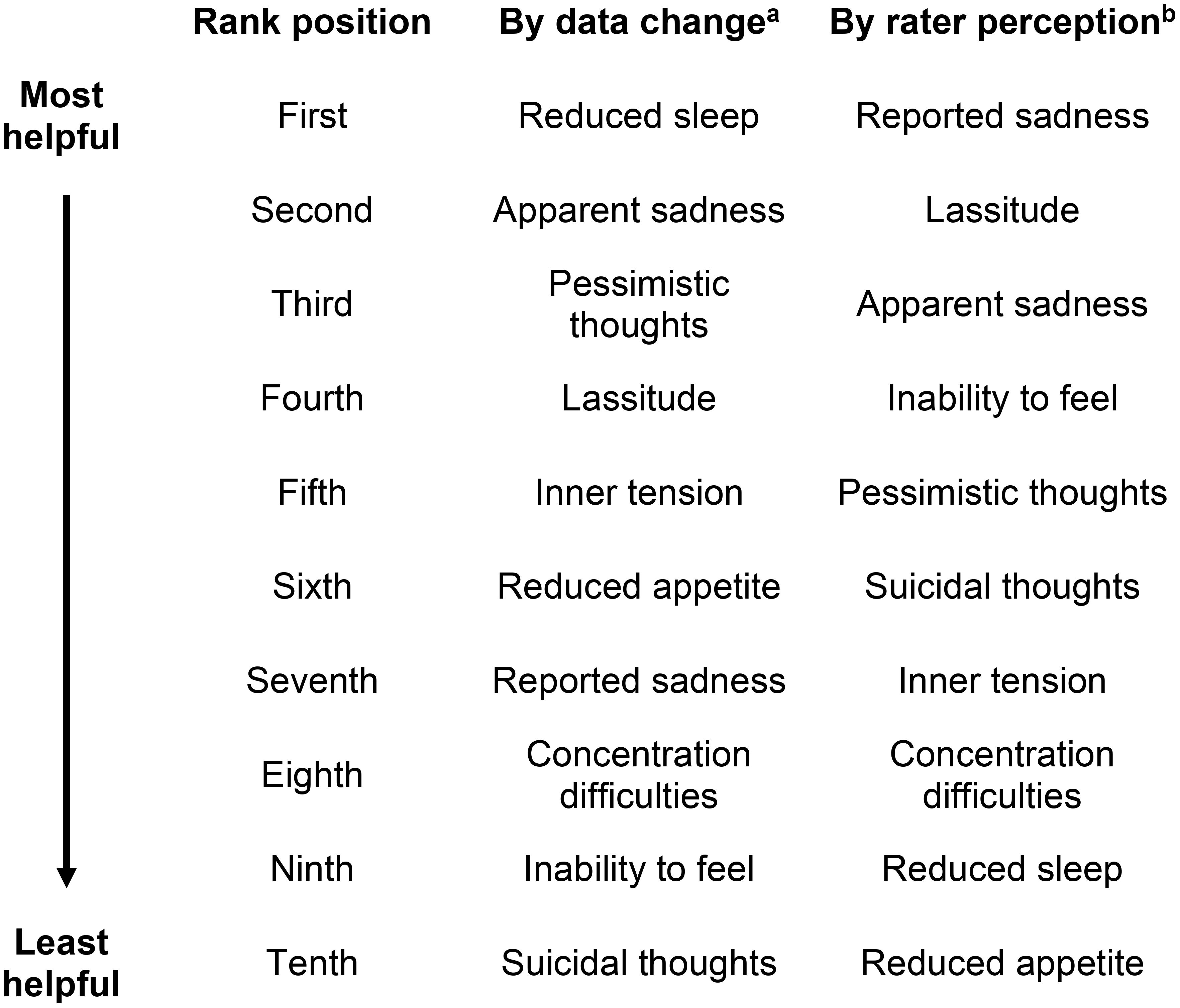
Figure 1 Ranking of MADRS items according to rater perception and data change. a Ranking by data change: ranking from tenth position to first position correlates with the average rate of data change between visits, with first position representing the MADRS item with the highest rate of symptom change according to data. b Ranking by rater perception: ranking from tenth position to first position correlates with the degree of helpfulness in assessing symptoms of depression, with first position representing the MADRS item perceived as the most helpful when assessing symptom change.
‘Reduced sleep’, ‘Apparent sadness’, and ‘Pessimistic thoughts’ were found to have the highest rate of data change by visit, however raters ranked ‘Reduced sleep’ in the ninth position of helpfulness, with ‘Apparent sadness’ and ‘Pessimistic thoughts’ placed third and fifth in terms of perceived helpfulness. In contrast, ‘Reported sadness’ was perceived as being the most helpful item for raters in assessing symptoms of depression but ranked seventh according to rate of data change. ‘Reduced appetite’ was ranked as the least useful item by rater perception, while rate of data change places this in the sixth position of helpfulness. ‘Suicidal thoughts’ was ranked sixth according to rater perception but placed tenth by rate of data change. The low ranking according to data change was caused by the smallest average change between visits for this item. ‘Inability to feel’ is ranked as the fourth most helpful item in terms of rater perception and shows the second lowest average change between visits in the data, ranking ninth according to data change.
4 Discussion
When examining the first item of the MADRS, ‘Reported sadness’, the account of symptoms and depression by subjects is the most important evidence one can receive. However, several factors including age of subject, age at onset of first depressive episode, education, length of illness, etc. could influence how sadness is being reported by subjects ( 37 ), and these may be due to differences in how depression has been conceptualized ( 38 ). Therefore, relying heavily on clinical background can be imperative for the clinician when administering and scoring the MADRS. ‘Apparent sadness’ also appears to be of critical interest, as it would have been presumed as a difficult item to assess in remote assessments. Nevertheless, both earlier studies by Kobak et al. ( 32 ) and a recent study by Sumiyoshi et al. conducted in Japan ( 39 ) found excellent reliability for the MADRS in remotely interviewing patients with MDD showing high consistency between remote and in-person interviews. These studies also emphasize the key importance of well-trained raters, which might be critical for accurately assigning ratings for more challenging items such as ‘apparent sadness’.
Appetite changes are not shared symptoms across all subjects with depression, and there can be marked increases or decreases in appetite in patients diagnosed with depression. Simmons et al. ( 40 ) report that only 48% of subjects experience reduced appetite, leaving 52% unaccounted for. Raters may be noticing this disconnect, with the focus of appetite in MADRS relying on reduction as opposed to bidirectional change and weighting the importance of this item accordingly.
We believe the discrepancy between rater-perceived ranking and ranking based on rate of data change for ‘Reduced sleep’ is due to the variability in the data itself. Sleep as a construct may be moving too quickly to be meaningful to raters more used to conducting traditional assessments. Additionally, sleep can move in more than one direction (i.e., it can both increase and decrease), and can be attributed to multiple factors not including symptoms of depression or drug treatment effects (environmental, pain, change in caffeine consumption, etc.), potentially deprioritizing sleep to raters and encouraging them to rely more heavily on other items.
Ranking for raters on ‘Suicidal thoughts’ (6 th on perceived helpfulness, but last on data change order) could demonstrate the importance of this item when it is reported, although the frequency of report may be low, and overall severity in most cases may also be low. When patients report suicidal thoughts or behaviors during MADRS interviews, the weight of this item may increase for raters, although the frequency with which it is seen in our dataset was also low.
In assessing depression, ‘Inability to feel’, involving changes in emotions, may become one of the most useful items to assess overall depression when reported, however, it may again be an item that infrequently shows large variability over shorter assessment periods.
The performance of traditional assessments to obtain ratings/scores for studies of novel treatments for depression is a critically important matter. It is imperative to reliably measure changes in symptoms—particularly for treatments that may carry unique side-effect profiles and safety risks. Researchers have used assessments on the widely agreed upon core symptoms of depression for decades. MADRS, though widely accepted by regulatory agencies and used by clinicians and researchers, may have a different value and clinical significance in the context of rapid-acting antidepressants. Using MADRS in short-interval, remote evaluations, with repeated assessments performed within 24 hours, might present challenges in accurately capturing symptom change. Certainly, not all depressed individuals have the same depressive symptoms at baseline and the dynamic nature of a therapeutic response to RAADs could potentially result in a rapid alleviation of certain symptoms, e.g. improvements in subjective mood, while leaving some other functional aspects less improved, resulting in a heterogeneity of symptom resolution and a sense of uncertainty in raters ( 36 ).
As research increasingly supports the use of rapid-acting antidepressants, and as their market approval increases, it is incumbent on clinicians to review and refine assessment processes. The rapid change in symptoms presents a challenge for clinicians, especially if the assessment is administered remotely. This study has compared how the rate of between-visit data changes for MADRS items relate to those that raters have identified as being more helpful or more challenging. The diversion between rate of data-change and rater perception of helpfulness could be related to several factors, one being difficulty in assessing specific items. A depression rater training study by Sajatovic et al. ( 41 ) showed no significant difference between raters based on country, level of experience with diagnosis, or previous training in terms of the items they identified as the most difficult to rate, namely ‘Apparent sadness’, ‘Inner tension’, ‘Concentration difficulties’, ‘Lassitude’, and ‘Inability to feel’. Similar results have been shown when comparing rater training using MADRS to other mood rating scales, such as the Hamilton Depression Rating Scale (HAM-D) and Young Mania Rating Scale (YMRS) in a bipolar disorder trial ( 42 ). We see the same MADRS items (listed above) identified as most difficult to rate with no significant difference for raters based on country, experience, diagnosis, or previous training, suggesting the items themselves present difficulty to raters ( 43 ). MADRS has also been noted as a more difficult scale to utilize when compared to other commonly used depressive symptom rating scales (HAM-D and YMRS), thus it could benefit from further insight and qualitative analysis ( 41 ).
A recent factor analysis conducted on two esketamine trials has taken an interesting approach to explore potential symptomatic clusters grouped around the rate of symptom change as detected by MADRS items ( 44 ). Three factors were identified labelled as affective/anhedonic symptoms (apparent sadness, reported sadness, lassitude, inability to feel); anxiety and vegetative symptoms (inner tension, reduced sleep, reduced appetite, concentration difficulties); and hopelessness (pessimistic thoughts, suicidal thoughts). Strikingly, our results on rater perception of items follows exactly these clusters with the affective/anhedonic factor listed as the most helpful for raters, followed by the hopelessness factor, and lastly the anxiety and vegetative factor symptom group, which is probably prone to the highest variability and heterogeneity overall.
Thus, another aspect potentially explaining the diversion between MADRS score changes and raters’ perceptions could be the novel treatment itself or the sensitivity to the consequent symptom change to which raters are accustomed. An example for this is the fluctuation of sleep on a day-to-day basis that can affect daily ratings when assessing change in symptoms with the MADRS. Research indicates that MADRS item responses - those related to sleep, most specifically - change in relation to patient experience and when compared to the prior administered assessment. Sleep, however, also influences memory and emotional memory in subjects with depression ( 45 ) and this can present additional challenges when ratings are conducted based on subject’s report every day or every other day, as is common in clinical trials of rapid-acting antidepressants.
There are inherent complications in the assessment of rapid change in TRD over short periods of time and rapid (i.e., between-visit) symptom changes at the item level can pose a challenge to ranking and assessing for severity. Singh et al. ( 14 ) examined the efficacy of both full and abbreviated MADRS scores in evaluating the response to intravenous esketamine. They conducted assessments of depressive symptoms at 2-, 4-, and 24-hours post-infusion, opting to omit the sleep and appetite items for the shorter 2 and 4-hour assessments. Johnson and colleagues also investigated the MADRS’s suitability within a 24-hour recall period, finding comparable content validity and high internal consistency and test-retest reliability ( 46 ). While most participants deemed a 24-hour recall period sufficient for assessing meaningful changes in depression symptoms, reduced sleep and appetite were noted exceptions, echoing Singh et al.’s decision to exclude these items. Yavorsky et al. analyzed esketamine trial data, revealing the MADRS’s sensitivity to change over short periods, albeit with limited responsiveness in the sleep and suicide items ( 47 ). These findings underscore the challenge of effectively capturing clinical change within a 24-hour timeframe, particularly for traditional depressive symptoms like mood, appetite, and sleep disturbances. Novel treatments for TRD have yet to yield novel assessments that are sensitive to change over such short periods of time.
Each item discussed and present in the MADRS is important in assessing overall depression and symptomology of the diagnoses, and clinical experience brings clarity to differences in items and scores, such as the importance of suicidal thoughts and sleep deprivation.
5 Conclusion
Rapid-acting antidepressants, such as ketamine and its derivatives, appear to induce more rapid changes in depression symptoms, which presents a challenge in the accurate capture of symptom change when using conventional rating scales. Our findings indicate a diversion between the rate of data change as measured by MADRS scores vs the raters’ perception of helpfulness of specific MADRS items in determining clinical improvement and depression severity change. While each MADRS item remains important in an assessment of depression symptomatology, it may be beneficial to refine raters’ sensitivity to changes in depressive symptoms over short periods of time, and to the specific side effects associated with novel treatment approaches. Further to this, gaining experience in the use of rapid-acting antidepressants, and in the ability to measure short and long-term effects of these novel treatments, might influence our notion of defining criteria for treatment resistance in depression.
Data availability statement
The raw data supporting the conclusions of this article will be made available by the authors, without undue reservation.
Ethics statement
Ethical review and approval was not required for the study of human participants in accordance with the local legislation and institutional requirements. Written informed consent from the participants was not required to participate in this study in accordance with the national legislation and the institutional requirements.
Author contributions
GC: Writing – review & editing, Writing – original draft. RB: Writing – review & editing, Writing – original draft. AM: Writing – review & editing, Writing – original draft. SN: Writing – review & editing, Writing – original draft. MO: Writing – review & editing, Writing – original draft.
The author(s) declare that no financial support was received for the research, authorship, and/or publication of this article.
Conflict of interest
GC, RB and SN are employed by WCG Clinical Endpoint Solutions. AM is a contractor of WCG Clinical Endpoint Solutions and a part-time employee of Whanganui District Health Board, Whanganui, NZ. MO is employed by WCG Clinical Endpoint Solutions; has received royalties from Pearson Inc. from sales of the PANSS Manual; and has received past grant funding from NIH, Brain & Behavior Foundation, the Stanley Medical Research Institute, and the Qatar National Research Fund.
The reviewer WL declared a past co-authorship with the author MO to the handling editor.
Publisher’s note
All claims expressed in this article are solely those of the authors and do not necessarily represent those of their affiliated organizations, or those of the publisher, the editors and the reviewers. Any product that may be evaluated in this article, or claim that may be made by its manufacturer, is not guaranteed or endorsed by the publisher.
1. Food & Drug Administration. FDA approves new nasal spray medication for treatment-resistant depression; available only at a certified doctor’s office or clinic. (2019).
Google Scholar
2. Montgomery SA, Asberg M. A new depression scale designed to be sensitive to change. Br J Psychiatry . (1979) 134:382–29. doi: 10.1192/bjp.134.4.382
PubMed Abstract | CrossRef Full Text | Google Scholar
3. First MB. Diagnostic and statistical manual of mental disorders, 5th edition, and clinical utility. J Nerv Ment Dis . (2013) 201(9):727–9. doi: 10.1097/NMD.0b013e3182a2168a
4. World Health Organization. Depression and other common mental disorders: global health estimates (No. WHO/MSD/MER/2017.2) (2017). Available online at: https://apps.who.int/iris/handle/10665/254610 (Accessed September 5, 2023).
5. Nestler EJ, Barrot M, DiLeone RJ, Eisch AJ, Gold SJ, Monteggia LM. Neurobiology of depression. Neuron . (2002) 34:13–25. doi: 10.1016/S0896-6273(02)00653-0
6. Fava M, Davidson KG. Definition and epidemiology of treatment-resistant depression. Psychiatr Clin North Am . (1996) 19:179–200. doi: 10.1016/S0193-953X(05)70283-5
7. Sackeim HA. The definition and meaning of treatment-resistant depression. J Clin Psychiatry . (2001) 62 Suppl 16:10–7.
PubMed Abstract | Google Scholar
8. Souery D, Papakostas GI, Trivedi MH. Treatment-resistant depression. J Clin Psychiatry . (2006) 67 Suppl 6:16–22.
9. Rush AJ. STAR*D: what have we learned? Am J Psychiatry . (2007) 164:201–4. doi: 10.1176/ajp.2007.164.2.201
10. Rush AJ, Trivedi MH, Wisniewski SR, Nierenberg AA, Stewart JW, Warden D, et al. Acute and longer-term outcomes in depressed outpatients requiring one or several treatment steps: a STAR*D report. Am J Psychiatry . (2006) 163:1905–17. doi: 10.1176/ajp.2006.163.11.1905
11. Abdallah CG, Adams TG, Kelmendi B, Esterlis I, Sanacora G, Krystal JH. Ketamine’s mechanism of action: a path to rapid-acting antidepressants. Depress Anxiety . (2016) 33:689–97. doi: 10.1002/da.22501
12. Jelen LA, Young AH, Stone JM. Ketamine: A tale of two enantiomers. J Psychopharmacol . (2021) 35:109–23. doi: 10.1177/0269881120959644
13. Aan Het Rot M, Zarate CA Jr, Charney DS, Mathew SJ. Ketamine for depression: where do we go from here? Biol Psychiatry . (2012) 72:537–47. doi: 10.1016/j.biopsych.2012.05.003
14. Singh JB, Fedgchin M, Daly EJ, De Boer P, Cooper K, Lim P, et al. A double-blind, randomized, placebo-controlled, dose-frequency study of intravenous ketamine in patients with treatment-resistant depression. Am J Psychiatry . (2016) 173:816–26. doi: 10.1176/appi.ajp.2016.16010037
15. Murrough JW, Iosifescu DV, Chang LC, Al Jurdi RK, Green CE, Perez AM, et al. Antidepressant efficacy of ketamine in treatment-resistant major depression: a two-site randomized controlled trial. Am J Psychiatry . (2013) 170:1134–42. doi: 10.1176/appi.ajp.2013.13030392
16. Lent JK, Arredondo A, Pugh MA, Austin PN. Ketamine and treatment-resistant depression. AANA J . (2019) 87:411–9.
17. Katalinic N, Lai R, Somogyi A, Mitchell PB, Glue P, Loo CK. Ketamine as a new treatment for depression: a review of its efficacy and adverse effects. Aust N Z J Psychiatry . (2013) 47:710–27. doi: 10.1177/0004867413486842
18. Short B, Fong J, Galvez V, Shelker W, Loo CK. Side-effects associated with ketamine use in depression: a systematic review. Lancet Psychiatry . (2018) 5:65–78. doi: 10.1016/S2215-0366(17)30272-9
19. Asberg M, Schalling D. Construction of a new psychiatric rating instrument, the Comprehensive Psychopathological Rating Scale (CPRS). Prog Neuropsychopharmacol . (1979) 3:405–12. doi: 10.1016/0364-7722(79)90055-9
20. Iannuzzo RW, Jaeger J, Goldberg JF, Kafantaris V, Sublette ME. Development and reliability of the HAM-D/MADRS interview: an integrated depression symptom rating scale. Psychiatry Res . (2006) 145:21–37. doi: 10.1016/j.psychres.2005.10.009
21. Hermens ML, Adèr HJ, van Hout HP, Terluin B, van Dyck R, de Haan M. Administering the MADRS by telephone or face-to-face: a validity study. Ann Gen Psychiatry . (2006) 5:3. doi: 10.1186/1744-859X-5-3
22. Maier W, Philipp M, Heuser I, Schlegel S, Buller R, Wetzel H. Improving depression severity assessment–I. Reliability, internal validity and sensitivity to change of three observer depression scales. J Psychiatr Res . (1988) 22:3–12. doi: 10.1016/0022-3956(88)90022-2
23. Maust D, Cristancho M, Gray L, Rushing S, Tjoa C, Thase ME. Psychiatric rating scales. In: Handbook of Clinical Neurology , vol. 106. Elsevier (2012). p. 227–37.
24. Svanborg P, Asberg M. A comparison between the Beck Depression Inventory (BDI) and the self-rating version of the Montgomery Asberg Depression Rating Scale (MADRS). J Affect Disord . (2001) 64:203–16. doi: 10.1016/S0165-0327(00)00242-1
25. Schmidtke A, Fleckenstein P, Moises W, Beckmann H. Studies of the reliability and validity of the German version of the Montgomery-Asberg Depression Rating Scale (MADRS). Schweiz Arch Neurol Psychiatr . (1988) 139:51–65.
26. Corruble E, Purper D, Payan C, Guelfi J. Inter-rater reliability of two depression rating scales, MADRS and DRRS, based on videotape records of structured interviews. Eur Psychiatry . (1998) 13:264–6. doi: 10.1016/S0924-9338(98)80032-1
27. Ozer SK, Demir B, Tugal O, et al. Montgomery-Asberg depression rating scale: inter-rater reliability and validity study. Turk Psikiyatri Derg . (2022) 33(3):187–95. doi: 10.5080/u25844
28. Takahashi N, Tomita K, Higuchi T, Inada T. The inter-rater reliability of the Japanese version of the Montgomery-Asberg depression rating scale (MADRS) using a structured interview guide for MADRS (SIGMA). Hum Psychopharmacol . (2004) 19:187–92. doi: 10.1002/hup.576
29. Satthapisit S, Posayaanuwat N, Sasaluksananont C, Kaewpornsawan T, Singhakun S. The comparison of Montgomery and Asberg Depression Rating Scale (MADRS thai) to diagnostic and statistical manual of mental disorders (DSM) and to Hamilton Rating Scale for Depression (HRSD): validity and reliability. J Med Assoc Thai . (2007) 90(3):524–31.
30. Zhong B, Wang Y, Chen H, et al. Reliability, validity and sensitivity of Montgomery-Asberg depression rating scale for patients with current major depression disorder. Chin J Behav Med Brain Sci . (2011) 20:85–7.
31. Ahmadpanah M, Sheikhbabaei M, Haghighi M, Roham F, Jahangard L, Akhondi A, et al. Validity and test-retest reliability of the Persian version of the Montgomery-Asberg Depression Rating Scale. Neuropsychiatr Dis Treat . (2016) 12:603–7. doi: 10.2147/NDT.S103869
32. Kobak KA, Williams JB, Jeglic E, Salvucci D, Sharp IR. Face-to-face versus remote administration of the Montgomery-Asberg Depression Rating Scale using videoconference and telephone. Depress Anxiety . (2008) 25(11):913–9. doi: 10.1002/da.v25:11
33. Holländare F, Andersson G, Engström I. A comparison of psychometric properties between internet and paper versions of two depression instruments (BDI-II and MADRS-S) administered to clinic patients. J Med Internet Res . (2010) 12:e49. doi: 10.2196/jmir.1392
34. Targum SD, Daly E, Fedgchin M, Cooper K, Singh JB. Comparability of blinded remote and site-based assessments of response to adjunctive esketamine or placebo nasal spray in patients with treatment resistant depression. J Psychiatr Res . (2019) 111:68–73. doi: 10.1016/j.jpsychires.2019.01.017
35. Targum SD, Catania CJ. Audio-digital recordings for surveillance in clinical trials of major depressive disorder. Contemp Clin Trials Commun . (2019) 14:100317. doi: 10.1016/j.conctc.2019.100317
36. Yavorsky C, Ballard E, Opler M, Sedway J, Targum SD, Lenderking W. Recommendations for selection and adaptation of rating scales for clinical studies of rapid-acting antidepressants. Front Psychiatry . (2023) 14:1135828. doi: 10.3389/fpsyt.2023.1135828
37. Rush AJ, Carmody TJ, Ibrahim HM, Trivedi MH, Biggs MM, Shores-Wilson K, et al. Comparison of self-report and clinician ratings on two inventories of depressive symptomatology. Psychiatr Serv . (2006) 57:829–37. doi: 10.1176/ps.2006.57.6.829
38. Carter JD, Frampton CM, Mulder RT, Luty SE, Joyce PR. The relationship of demographic, clinical, cognitive and personality variables to the discrepancy between self and clinician rated depression. J Affect Disord . (2010) 124:202–6. doi: 10.1016/j.jad.2009.11.011
39. Sumiyoshi T, Morio Y, Kawashima T, Tachimori H, Hongo S, Kishimoto T, et al. Feasibility of remote interviews in assessing disease severity in patients with major depressive disorder: A pilot study. Neuropsychopharmacol Rep . (2024) 44(1):149–57. doi: 10.1002/npr2.12411
40. Simmons WK, Burrows K, Avery JA, Kerr KL, Bodurka J, Savage CR, et al. Depression-related increases and decreases in appetite: dissociable patterns of aberrant activity in reward and interoceptive neurocircuitry. Am J Psychiatry . (2016) 173:418–28. doi: 10.1176/appi.ajp.2015.15020162
41. Sajatovic M, Gaur R, Tatsuoka C, De Santi S, Lee N, Laredo J, et al. Rater training for a multi-site, international clinical trial: what mood symptoms may be most difficult to rate? Psychopharmacol Bull . (2011) 44:5–14.
42. Hamilton M. A rating scale for depression. J Neurol Neurosurg Psychiatry . (1960) 23:56–62. doi: 10.1136/jnnp.23.1.56
43. Gaur R, Sajatovic M, Lee N, Nath G, Ramirez L, Kaviani H. , in: Rater Training on HAMD, MADRS and YMRS – what were the difficult items to rate? Poster session presented at New Clinical Drug Evaluation Unit (NCDEU) 50th Anniversary Meeting, Boca Raton, Florida, June 14–17, 2010.
44. Borentain S, Gogate J, Williamson D, Carmody T, Trivedi M, Jamieson C, et al. Montgomery-Åsberg Depression Rating Scale factors in treatment-resistant depression at onset of treatment: Derivation, replication, and change over time during treatment with esketamine. Int J Methods Psychiatr Res . (2022) 31:e1927. doi: 10.1002/mpr.1927
45. Harrington MO, Nedberge KM, Durrant SJ. The effect of sleep deprivation on emotional memory consolidation in participants reporting depressive symptoms. Neurobiol Learn Mem . (2018) 152:10–9. doi: 10.1016/j.nlm.2018.04.013
46. Johnson K, Devine J, Ho K, Howard K, Saretsky T, Jamieson C, et al. Evidence to support Montgomery-Åsberg depression rating scale administration every 24 hours to assess rapid onsetof treatment response. J Clin Psychiatry . (2016) 77:1681–6. doi: 10.4088/JCP.15m10253
47. Yavorsky C, Singh J, Engelhardt N. Can the MADRS measure rapid changes in depressive symptoms in response to esketamine treatment? Neuropsychopharmacol . (2017) 42:S476–652. doi: 10.3389/fpsyt.2023.1135828
CrossRef Full Text | Google Scholar
Keywords: depression, MADRS = Montgomery-Asberg depression rating scale, rater perception, rapid acting antidepressants, structured interview guide for the MADRS (SIGMA)
Citation: Capodilupo G, Blattner R, Must A, Navarro SG and Opler M (2024) A qualitative investigation of the Montgomery–Åsberg depression rating scale: discrepancies in rater perceptions and data trends in remote assessments of rapid-acting antidepressants in treatment resistant depression. Front. Psychiatry 15:1289630. doi: 10.3389/fpsyt.2024.1289630
Received: 06 September 2023; Accepted: 16 April 2024; Published: 01 May 2024.
Reviewed by:
Copyright © 2024 Capodilupo, Blattner, Must, Navarro and Opler. This is an open-access article distributed under the terms of the Creative Commons Attribution License (CC BY) . The use, distribution or reproduction in other forums is permitted, provided the original author(s) and the copyright owner(s) are credited and that the original publication in this journal is cited, in accordance with accepted academic practice. No use, distribution or reproduction is permitted which does not comply with these terms.
*Correspondence: Mark Opler, [email protected]
Disclaimer: All claims expressed in this article are solely those of the authors and do not necessarily represent those of their affiliated organizations, or those of the publisher, the editors and the reviewers. Any product that may be evaluated in this article or claim that may be made by its manufacturer is not guaranteed or endorsed by the publisher.
We couldn’t find any results matching your search.
Please try using other words for your search or explore other sections of the website for relevant information.
We’re sorry, we are currently experiencing some issues, please try again later.
Our team is working diligently to resolve the issue. Thank you for your patience and understanding.
News & Insights

RIVN Quantitative Stock Analysis - Benjamin Graham
May 04, 2024 — 01:07 pm EDT
Written by John Reese for Validea ->
Below is Validea's guru fundamental report for RIVIAN AUTOMOTIVE INC ( RIVN ) . Of the 22 guru strategies we follow, RIVN rates highest using our Value Investor model based on the published strategy of Benjamin Graham . This deep value methodology screens for stocks that have low P/B and P/E ratios, along with low debt and solid long-term earnings growth.
RIVIAN AUTOMOTIVE INC ( RIVN ) is a large-cap growth stock in the Auto & Truck Manufacturers industry. The rating using this strategy is 57% based on the firm’s underlying fundamentals and the stock’s valuation. A score of 80% or above typically indicates that the strategy has some interest in the stock and a score above 90% typically indicates strong interest.
The following table summarizes whether the stock meets each of this strategy's tests. Not all criteria in the below table receive equal weighting or are independent, but the table provides a brief overview of the strong and weak points of the security in the context of the strategy's criteria.
Detailed Analysis of RIVIAN AUTOMOTIVE INC
RIVN Guru Analysis
RIVN Fundamental Analysis
More Information on Benjamin Graham
Benjamin Graham Portfolio
Top Benjamin Graham Stocks
About Benjamin Graham : The late Benjamin Graham may be the oldest of the gurus we follow, but his impact on the investing world has lasted for decades after his death in 1976. Known as both the "Father of Value Investing" and the founder of the entire field of security analysis, Graham mentored several of history's greatest investors -- including Warren Buffett -- and inspired a slew of others, including John Templeton, Mario Gabelli, and another of Validea's gurus, John Neff. Graham built his fortune and reputation after living through some extremely difficult times, including both the Great Depression and his own family's financial woes following his father's death when Benjamin was a young man. His investment firm posted per annum returns of about 20 percent from 1936 to 1956, far outpacing the 12.2 percent average return for the market during that time.
Additional Research Links
Top NASDAQ 100 Stocks
Factor-Based ETF Portfolios
Harry Browne Permanent Portfolio
Ray Dalio All Weather Portfolio
High Shareholder Yield Stocks
About Validea : Validea is an investment research service that follows the published strategies of investment legends. Validea offers both stock analysis and model portfolios based on gurus who have outperformed the market over the long-term, including Warren Buffett, Benjamin Graham, Peter Lynch and Martin Zweig. For more information about Validea, click here
The views and opinions expressed herein are the views and opinions of the author and do not necessarily reflect those of Nasdaq, Inc.

Stocks mentioned
More related articles.
This data feed is not available at this time.
Sign up for the TradeTalks newsletter to receive your weekly dose of trading news, trends and education. Delivered Wednesdays.
To add symbols:
- Type a symbol or company name. When the symbol you want to add appears, add it to My Quotes by selecting it and pressing Enter/Return.
- Copy and paste multiple symbols separated by spaces.
These symbols will be available throughout the site during your session.
Your symbols have been updated
Edit watchlist.
- Type a symbol or company name. When the symbol you want to add appears, add it to Watchlist by selecting it and pressing Enter/Return.
Opt in to Smart Portfolio
Smart Portfolio is supported by our partner TipRanks. By connecting my portfolio to TipRanks Smart Portfolio I agree to their Terms of Use .

IMAGES
VIDEO
COMMENTS
A relationship network of depression research was established, highlighting the highly influential countries, journals, categories, authors, institutions, cited articles, and keywords in this research field. The study identifies great research potential in the field of depression, provides scientific guidance for researchers to find potential ...
A new type of magnetic brain stimulation brought rapid remission to almost 80% of participants with severe depression in a study conducted at the Stanford University School of Medicine. The treatment, known as Stanford accelerated intelligent neuromodulation therapy (SAINT) or simply Stanford neuromodulation therapy, is an intensive, individualized form of transcranial magnetic stimulation.
Background Identifying determinants of health and understanding their role in health production constitutes an important research theme. We aimed to document the state of recent multi-country research on this theme in the literature. Methods We followed the PRISMA-ScR guidelines to systematically identify, triage and review literature (January 2013—July 2019). We searched for studies that ...
During the COVID-19 pandemic, many regions and countries implemented lockdowns and isolation to curb the virus's spread, which might increase loneliness and lead to a series of psychological distress. This study aims to investigate the association between loneliness and depression during the COVID-19 pandemic and examine whether perceived social support and perceived internal control mediate ...
Background A cancer diagnosis can have a substantial impact on mental health and wellbeing. Depression and anxiety may hinder cancer treatment and recovery, as well as quality of life and survival. We argue that more research is needed to prevent and treat co-morbid depression and anxiety among people with cancer and that it requires greater clinical priority. For background and to support our ...
Fatigue is a frequently reported symptom in major depressive disorder, occurring in over 90% of patients. Clinical presentations of fatigue within major depressive disorder encompass overlapping physical, cognitive and emotional aspects. While this review addresses the epidemiology, burden, function …
Abstract. Previous quantitative reviews of research on the efficacy of psychotherapy for depression have included only a subset of the available research or limited their focus to a single outcome measure. The present review offers a more comprehensive quantitative integration of this literature. Using studies that compared psychotherapy with ...
The current review included studies published between January 1990 and October 2010 and reporting on depression among undergraduate university students including medical students. According to this current review the average depression prevalence is 30.6%, a higher rate than the 9% found in the general population rates of the US (range 6-12% ...
Physical activity can help improve your health and quality of life. Not getting enough physical activity can increase your risk for diabetes, heart disease, cancers, and mental health disorders. Current theories about depression suggest that sleep problems, a lack of energy, and physical inactivity can result from a depressed mood.
The percent of U.S. college students who self-r eported a. diagnosis with depression was recently estimated to be about 25% (American College. Health Association, 2019). However, higher rates have ...
A qualitative investigation of the Montgomery-Åsberg depression rating scale: discrepancies in rater perceptions and data trends in remote assessments of rapid-acting antidepressants in treatment resistant depression ... and no other quantitative analysis has been completed for the purpose of this study. ... Research indicates that MADRS ...
1. Introduction. Tinnitus is defined as a sound perceived without stimulus from an external acoustic source [].The prevalence ranges from 5 to 43% worldwide and from 9 to 28% in Europe [2,3].This prevalence appears to increase with age, and males are more commonly affected [2,4].The wide range of prevalence data may be due to the lack of a common definition of tinnitus in research [].
The findings indicated that social media addiction has a positive correlation with depression and depression significantly predicts social media addiction. Summary of Factor analysis between the ...
Vagal Nerve Stimulation-an implantable option. Vagal Nerve Stimulation (VNS) is an FDA-approved, non- medication treatment option for severe or bipolar depression. Like ketamine, it has a 70% ...
Research Crisis Services Methodology A quantitative cross-sectional design was used to collect data through an online survey platform between September 13 and December 16, 2023. A sample of individuals ages 13 to 24 who resided in the United States, including its territories, was recruited via targeted ads on social media.
This deep value methodology screens for stocks that have low P/B and P/E ratios, along with low debt and solid long-term earnings growth. ALBEMARLE CORPORATION ( ALB) is a large-cap growth stock ...
RIVN Quantitative Stock Analysis - Benjamin Graham ... including both the Great Depression and his own family's financial woes following his father's death when Benjamin was a young man ...