Have a language expert improve your writing
Run a free plagiarism check in 10 minutes, generate accurate citations for free.
- Knowledge Base
Methodology
- How to Write a Literature Review | Guide, Examples, & Templates

How to Write a Literature Review | Guide, Examples, & Templates
Published on January 2, 2023 by Shona McCombes . Revised on September 11, 2023.
What is a literature review? A literature review is a survey of scholarly sources on a specific topic. It provides an overview of current knowledge, allowing you to identify relevant theories, methods, and gaps in the existing research that you can later apply to your paper, thesis, or dissertation topic .
There are five key steps to writing a literature review:
- Search for relevant literature
- Evaluate sources
- Identify themes, debates, and gaps
- Outline the structure
- Write your literature review
A good literature review doesn’t just summarize sources—it analyzes, synthesizes , and critically evaluates to give a clear picture of the state of knowledge on the subject.
Instantly correct all language mistakes in your text
Upload your document to correct all your mistakes in minutes
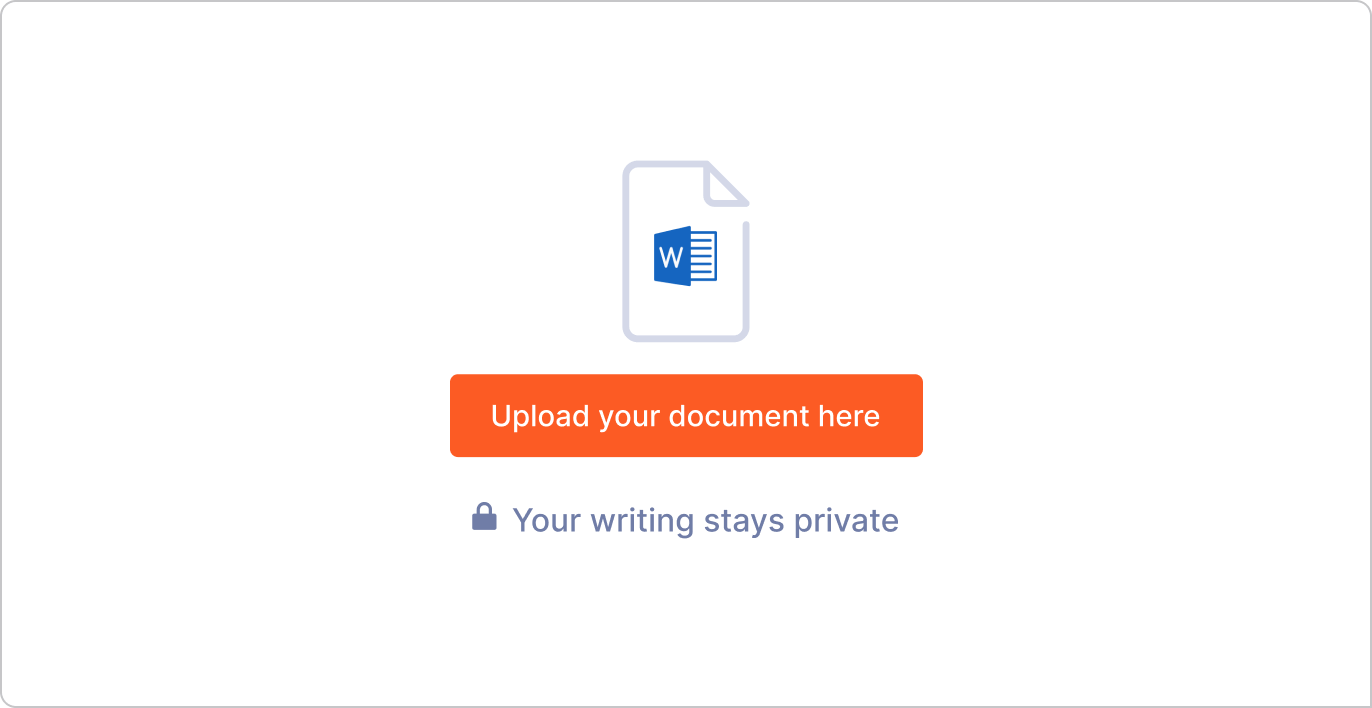
Table of contents
What is the purpose of a literature review, examples of literature reviews, step 1 – search for relevant literature, step 2 – evaluate and select sources, step 3 – identify themes, debates, and gaps, step 4 – outline your literature review’s structure, step 5 – write your literature review, free lecture slides, other interesting articles, frequently asked questions, introduction.
- Quick Run-through
- Step 1 & 2
When you write a thesis , dissertation , or research paper , you will likely have to conduct a literature review to situate your research within existing knowledge. The literature review gives you a chance to:
- Demonstrate your familiarity with the topic and its scholarly context
- Develop a theoretical framework and methodology for your research
- Position your work in relation to other researchers and theorists
- Show how your research addresses a gap or contributes to a debate
- Evaluate the current state of research and demonstrate your knowledge of the scholarly debates around your topic.
Writing literature reviews is a particularly important skill if you want to apply for graduate school or pursue a career in research. We’ve written a step-by-step guide that you can follow below.
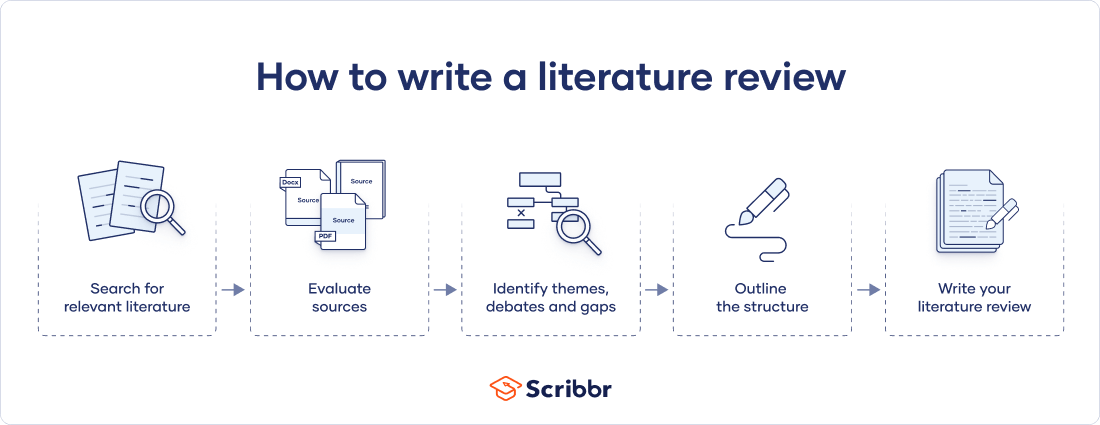
Receive feedback on language, structure, and formatting
Professional editors proofread and edit your paper by focusing on:
- Academic style
- Vague sentences
- Style consistency
See an example
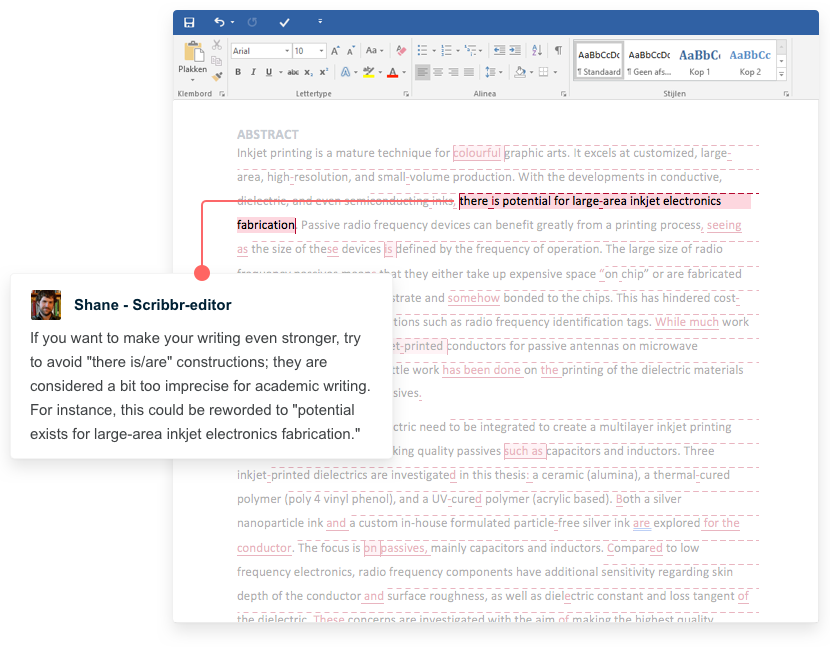
Writing literature reviews can be quite challenging! A good starting point could be to look at some examples, depending on what kind of literature review you’d like to write.
- Example literature review #1: “Why Do People Migrate? A Review of the Theoretical Literature” ( Theoretical literature review about the development of economic migration theory from the 1950s to today.)
- Example literature review #2: “Literature review as a research methodology: An overview and guidelines” ( Methodological literature review about interdisciplinary knowledge acquisition and production.)
- Example literature review #3: “The Use of Technology in English Language Learning: A Literature Review” ( Thematic literature review about the effects of technology on language acquisition.)
- Example literature review #4: “Learners’ Listening Comprehension Difficulties in English Language Learning: A Literature Review” ( Chronological literature review about how the concept of listening skills has changed over time.)
You can also check out our templates with literature review examples and sample outlines at the links below.
Download Word doc Download Google doc
Before you begin searching for literature, you need a clearly defined topic .
If you are writing the literature review section of a dissertation or research paper, you will search for literature related to your research problem and questions .
Make a list of keywords
Start by creating a list of keywords related to your research question. Include each of the key concepts or variables you’re interested in, and list any synonyms and related terms. You can add to this list as you discover new keywords in the process of your literature search.
- Social media, Facebook, Instagram, Twitter, Snapchat, TikTok
- Body image, self-perception, self-esteem, mental health
- Generation Z, teenagers, adolescents, youth
Search for relevant sources
Use your keywords to begin searching for sources. Some useful databases to search for journals and articles include:
- Your university’s library catalogue
- Google Scholar
- Project Muse (humanities and social sciences)
- Medline (life sciences and biomedicine)
- EconLit (economics)
- Inspec (physics, engineering and computer science)
You can also use boolean operators to help narrow down your search.
Make sure to read the abstract to find out whether an article is relevant to your question. When you find a useful book or article, you can check the bibliography to find other relevant sources.
You likely won’t be able to read absolutely everything that has been written on your topic, so it will be necessary to evaluate which sources are most relevant to your research question.
For each publication, ask yourself:
- What question or problem is the author addressing?
- What are the key concepts and how are they defined?
- What are the key theories, models, and methods?
- Does the research use established frameworks or take an innovative approach?
- What are the results and conclusions of the study?
- How does the publication relate to other literature in the field? Does it confirm, add to, or challenge established knowledge?
- What are the strengths and weaknesses of the research?
Make sure the sources you use are credible , and make sure you read any landmark studies and major theories in your field of research.
You can use our template to summarize and evaluate sources you’re thinking about using. Click on either button below to download.
Take notes and cite your sources
As you read, you should also begin the writing process. Take notes that you can later incorporate into the text of your literature review.
It is important to keep track of your sources with citations to avoid plagiarism . It can be helpful to make an annotated bibliography , where you compile full citation information and write a paragraph of summary and analysis for each source. This helps you remember what you read and saves time later in the process.
Prevent plagiarism. Run a free check.
To begin organizing your literature review’s argument and structure, be sure you understand the connections and relationships between the sources you’ve read. Based on your reading and notes, you can look for:
- Trends and patterns (in theory, method or results): do certain approaches become more or less popular over time?
- Themes: what questions or concepts recur across the literature?
- Debates, conflicts and contradictions: where do sources disagree?
- Pivotal publications: are there any influential theories or studies that changed the direction of the field?
- Gaps: what is missing from the literature? Are there weaknesses that need to be addressed?
This step will help you work out the structure of your literature review and (if applicable) show how your own research will contribute to existing knowledge.
- Most research has focused on young women.
- There is an increasing interest in the visual aspects of social media.
- But there is still a lack of robust research on highly visual platforms like Instagram and Snapchat—this is a gap that you could address in your own research.
There are various approaches to organizing the body of a literature review. Depending on the length of your literature review, you can combine several of these strategies (for example, your overall structure might be thematic, but each theme is discussed chronologically).
Chronological
The simplest approach is to trace the development of the topic over time. However, if you choose this strategy, be careful to avoid simply listing and summarizing sources in order.
Try to analyze patterns, turning points and key debates that have shaped the direction of the field. Give your interpretation of how and why certain developments occurred.
If you have found some recurring central themes, you can organize your literature review into subsections that address different aspects of the topic.
For example, if you are reviewing literature about inequalities in migrant health outcomes, key themes might include healthcare policy, language barriers, cultural attitudes, legal status, and economic access.
Methodological
If you draw your sources from different disciplines or fields that use a variety of research methods , you might want to compare the results and conclusions that emerge from different approaches. For example:
- Look at what results have emerged in qualitative versus quantitative research
- Discuss how the topic has been approached by empirical versus theoretical scholarship
- Divide the literature into sociological, historical, and cultural sources
Theoretical
A literature review is often the foundation for a theoretical framework . You can use it to discuss various theories, models, and definitions of key concepts.
You might argue for the relevance of a specific theoretical approach, or combine various theoretical concepts to create a framework for your research.
Like any other academic text , your literature review should have an introduction , a main body, and a conclusion . What you include in each depends on the objective of your literature review.
The introduction should clearly establish the focus and purpose of the literature review.
Depending on the length of your literature review, you might want to divide the body into subsections. You can use a subheading for each theme, time period, or methodological approach.
As you write, you can follow these tips:
- Summarize and synthesize: give an overview of the main points of each source and combine them into a coherent whole
- Analyze and interpret: don’t just paraphrase other researchers — add your own interpretations where possible, discussing the significance of findings in relation to the literature as a whole
- Critically evaluate: mention the strengths and weaknesses of your sources
- Write in well-structured paragraphs: use transition words and topic sentences to draw connections, comparisons and contrasts
In the conclusion, you should summarize the key findings you have taken from the literature and emphasize their significance.
When you’ve finished writing and revising your literature review, don’t forget to proofread thoroughly before submitting. Not a language expert? Check out Scribbr’s professional proofreading services !
This article has been adapted into lecture slides that you can use to teach your students about writing a literature review.
Scribbr slides are free to use, customize, and distribute for educational purposes.
Open Google Slides Download PowerPoint
If you want to know more about the research process , methodology , research bias , or statistics , make sure to check out some of our other articles with explanations and examples.
- Sampling methods
- Simple random sampling
- Stratified sampling
- Cluster sampling
- Likert scales
- Reproducibility
Statistics
- Null hypothesis
- Statistical power
- Probability distribution
- Effect size
- Poisson distribution
Research bias
- Optimism bias
- Cognitive bias
- Implicit bias
- Hawthorne effect
- Anchoring bias
- Explicit bias
A literature review is a survey of scholarly sources (such as books, journal articles, and theses) related to a specific topic or research question .
It is often written as part of a thesis, dissertation , or research paper , in order to situate your work in relation to existing knowledge.
There are several reasons to conduct a literature review at the beginning of a research project:
- To familiarize yourself with the current state of knowledge on your topic
- To ensure that you’re not just repeating what others have already done
- To identify gaps in knowledge and unresolved problems that your research can address
- To develop your theoretical framework and methodology
- To provide an overview of the key findings and debates on the topic
Writing the literature review shows your reader how your work relates to existing research and what new insights it will contribute.
The literature review usually comes near the beginning of your thesis or dissertation . After the introduction , it grounds your research in a scholarly field and leads directly to your theoretical framework or methodology .
A literature review is a survey of credible sources on a topic, often used in dissertations , theses, and research papers . Literature reviews give an overview of knowledge on a subject, helping you identify relevant theories and methods, as well as gaps in existing research. Literature reviews are set up similarly to other academic texts , with an introduction , a main body, and a conclusion .
An annotated bibliography is a list of source references that has a short description (called an annotation ) for each of the sources. It is often assigned as part of the research process for a paper .
Cite this Scribbr article
If you want to cite this source, you can copy and paste the citation or click the “Cite this Scribbr article” button to automatically add the citation to our free Citation Generator.
McCombes, S. (2023, September 11). How to Write a Literature Review | Guide, Examples, & Templates. Scribbr. Retrieved August 26, 2024, from https://www.scribbr.com/dissertation/literature-review/
Is this article helpful?
Shona McCombes
Other students also liked, what is a theoretical framework | guide to organizing, what is a research methodology | steps & tips, how to write a research proposal | examples & templates, "i thought ai proofreading was useless but..".
I've been using Scribbr for years now and I know it's a service that won't disappoint. It does a good job spotting mistakes”
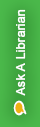
- Collections
- Research Help
- Teaching & Learning
- Library Home
Comprehensive Literature Review
- The Basic Steps
- Advanced Searching for the Literature Review
- Writing the Literature Review
- Mini-Video Demonstrations
Acknowledgements
Thank you to Jennifer Corbin for permission to use content from her research guide “Comprehensive Literature Review" ( http://libguides.tulane.edu/c.php?g=182708&p=1204573 ), Howard-TiltonLibrary, Tulane University. And thank you to the University of Santa Cruz Library for permission to incorporate content from their guide "Write a Literature Review" ( http://guides.library.ucsc.edu/write-a-literature-review).
What Is a Literature Review?
A literature review is an overview of selected articles, books and other sources about a specific subject. The purpose is to summarize the existing research that has been done on the subject in order to put your research in context and to highlight what your research will add to the existing body of knowledge. Literature reviews are typically organized in some way (chronological, thematic, methodological).
Note that your literature review is separate from your works cited page or bibliography, although sources used in your literature review will need to be cited properly in them.
Let's take a look at an example of a literature review in an article, a dissertation, and a review article.
- Article Example: Abused Drugs in Insects Oliveira, J. S.; Baia, T. C.; Gama, R. A.; Lima, K. M. G. Development of a novel non-destructive method based on spectral fingerprint for determination of abused drug in insects: An alternative entomotoxicology approach. Microchemical Journal 2014, 115, 39-46.
- Dissertation Example: Forensic Entomotoxicology Peace, Michelle Renee'. "Forensic Entomotoxicology: A Study in the Deposition and Effects of Amphetamines and Barbiturates in the Larvae of the Black Blow Fly, Phormia Regina." Order No. 3177624 Virginia Commonwealth University, 2005. Ann Arbor: ProQuest. Web. 18 Aug. 2016.
- Review Article: Determination of Post-Burial Entomology Singh R, Sharma S, Sharma A. 2016. Determination of post-burial interval using entomology: A review. J Forensic Leg Med. 42:37-40.
Purpose of a Literature Review
A literature review may constitute an essential chapter of a thesis or dissertation, or may be a self-contained review of writings on a subject. In either case, its purpose is to:
- Place each work in the context of its contribution to the understanding of the subject under review
- Describe the relationship of each work to the others under consideration
- Identify new ways to interpret, and shed light on any gaps in, previous research
- Resolve conflicts amongst seemingly contradictory previous studies
- Identify areas of prior scholarship to prevent duplication of effort
- Point the way forward for further research
- Place one's original work (in the case of theses or dissertations) in the context of existing literature
The literature review itself, however, does not present new primary scholarship.
- Next: The Basic Steps >>
- Last Updated: Oct 4, 2023 12:50 PM
- URL: https://libguides.wvu.edu/LitReview
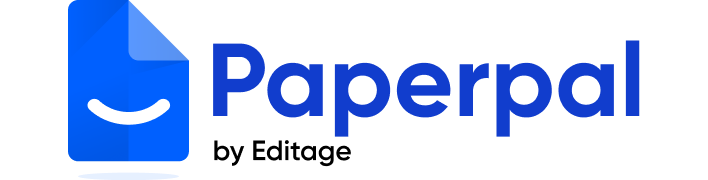
What is a Literature Review? How to Write It (with Examples)
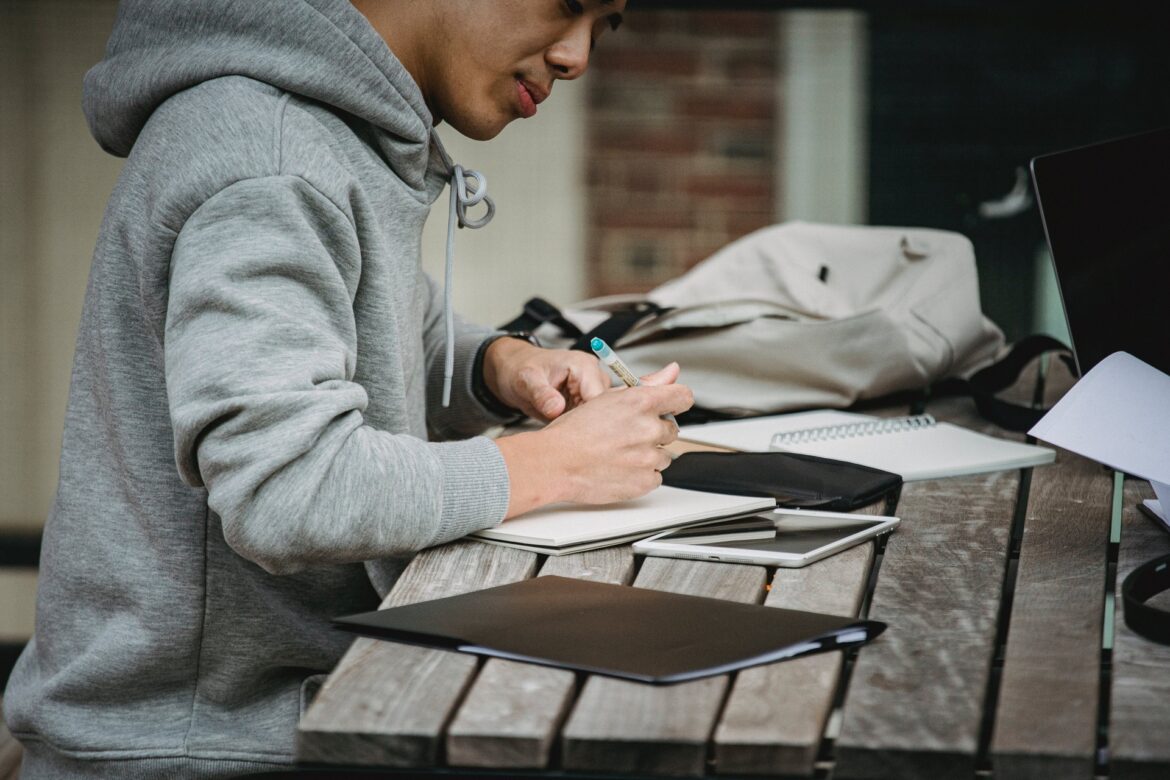
A literature review is a critical analysis and synthesis of existing research on a particular topic. It provides an overview of the current state of knowledge, identifies gaps, and highlights key findings in the literature. 1 The purpose of a literature review is to situate your own research within the context of existing scholarship, demonstrating your understanding of the topic and showing how your work contributes to the ongoing conversation in the field. Learning how to write a literature review is a critical tool for successful research. Your ability to summarize and synthesize prior research pertaining to a certain topic demonstrates your grasp on the topic of study, and assists in the learning process.
Table of Contents
- What is the purpose of literature review?
- a. Habitat Loss and Species Extinction:
- b. Range Shifts and Phenological Changes:
- c. Ocean Acidification and Coral Reefs:
- d. Adaptive Strategies and Conservation Efforts:
How to write a good literature review
- Choose a Topic and Define the Research Question:
- Decide on the Scope of Your Review:
- Select Databases for Searches:
- Conduct Searches and Keep Track:
- Review the Literature:
- Organize and Write Your Literature Review:
- How to write a literature review faster with Paperpal?
- Frequently asked questions
What is a literature review?
A well-conducted literature review demonstrates the researcher’s familiarity with the existing literature, establishes the context for their own research, and contributes to scholarly conversations on the topic. One of the purposes of a literature review is also to help researchers avoid duplicating previous work and ensure that their research is informed by and builds upon the existing body of knowledge.
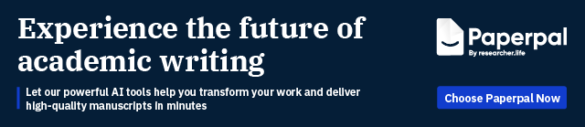
What is the purpose of literature review?
A literature review serves several important purposes within academic and research contexts. Here are some key objectives and functions of a literature review: 2
1. Contextualizing the Research Problem: The literature review provides a background and context for the research problem under investigation. It helps to situate the study within the existing body of knowledge.
2. Identifying Gaps in Knowledge: By identifying gaps, contradictions, or areas requiring further research, the researcher can shape the research question and justify the significance of the study. This is crucial for ensuring that the new research contributes something novel to the field.
Find academic papers related to your research topic faster. Try Research on Paperpal
3. Understanding Theoretical and Conceptual Frameworks: Literature reviews help researchers gain an understanding of the theoretical and conceptual frameworks used in previous studies. This aids in the development of a theoretical framework for the current research.
4. Providing Methodological Insights: Another purpose of literature reviews is that it allows researchers to learn about the methodologies employed in previous studies. This can help in choosing appropriate research methods for the current study and avoiding pitfalls that others may have encountered.
5. Establishing Credibility: A well-conducted literature review demonstrates the researcher’s familiarity with existing scholarship, establishing their credibility and expertise in the field. It also helps in building a solid foundation for the new research.
6. Informing Hypotheses or Research Questions: The literature review guides the formulation of hypotheses or research questions by highlighting relevant findings and areas of uncertainty in existing literature.
Literature review example
Let’s delve deeper with a literature review example: Let’s say your literature review is about the impact of climate change on biodiversity. You might format your literature review into sections such as the effects of climate change on habitat loss and species extinction, phenological changes, and marine biodiversity. Each section would then summarize and analyze relevant studies in those areas, highlighting key findings and identifying gaps in the research. The review would conclude by emphasizing the need for further research on specific aspects of the relationship between climate change and biodiversity. The following literature review template provides a glimpse into the recommended literature review structure and content, demonstrating how research findings are organized around specific themes within a broader topic.
Literature Review on Climate Change Impacts on Biodiversity:
Climate change is a global phenomenon with far-reaching consequences, including significant impacts on biodiversity. This literature review synthesizes key findings from various studies:
a. Habitat Loss and Species Extinction:
Climate change-induced alterations in temperature and precipitation patterns contribute to habitat loss, affecting numerous species (Thomas et al., 2004). The review discusses how these changes increase the risk of extinction, particularly for species with specific habitat requirements.
b. Range Shifts and Phenological Changes:
Observations of range shifts and changes in the timing of biological events (phenology) are documented in response to changing climatic conditions (Parmesan & Yohe, 2003). These shifts affect ecosystems and may lead to mismatches between species and their resources.
c. Ocean Acidification and Coral Reefs:
The review explores the impact of climate change on marine biodiversity, emphasizing ocean acidification’s threat to coral reefs (Hoegh-Guldberg et al., 2007). Changes in pH levels negatively affect coral calcification, disrupting the delicate balance of marine ecosystems.
d. Adaptive Strategies and Conservation Efforts:
Recognizing the urgency of the situation, the literature review discusses various adaptive strategies adopted by species and conservation efforts aimed at mitigating the impacts of climate change on biodiversity (Hannah et al., 2007). It emphasizes the importance of interdisciplinary approaches for effective conservation planning.
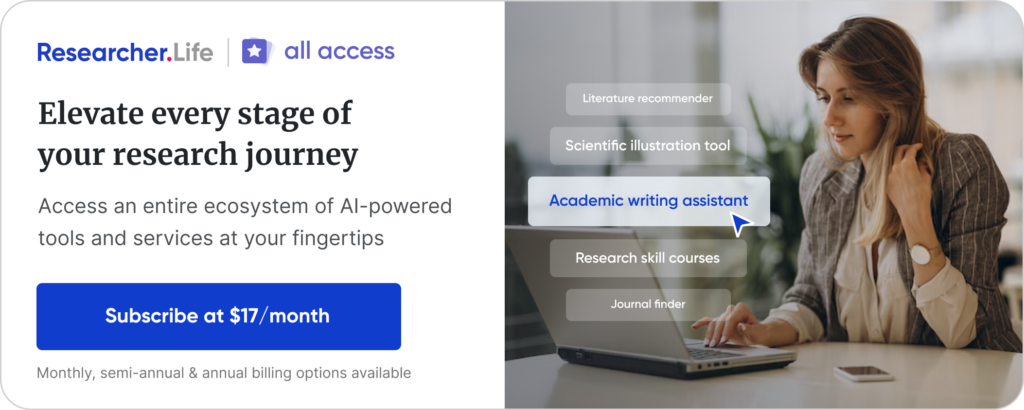
Strengthen your literature review with factual insights. Try Research on Paperpal for free!
Writing a literature review involves summarizing and synthesizing existing research on a particular topic. A good literature review format should include the following elements.
Introduction: The introduction sets the stage for your literature review, providing context and introducing the main focus of your review.
- Opening Statement: Begin with a general statement about the broader topic and its significance in the field.
- Scope and Purpose: Clearly define the scope of your literature review. Explain the specific research question or objective you aim to address.
- Organizational Framework: Briefly outline the structure of your literature review, indicating how you will categorize and discuss the existing research.
- Significance of the Study: Highlight why your literature review is important and how it contributes to the understanding of the chosen topic.
- Thesis Statement: Conclude the introduction with a concise thesis statement that outlines the main argument or perspective you will develop in the body of the literature review.
Body: The body of the literature review is where you provide a comprehensive analysis of existing literature, grouping studies based on themes, methodologies, or other relevant criteria.
- Organize by Theme or Concept: Group studies that share common themes, concepts, or methodologies. Discuss each theme or concept in detail, summarizing key findings and identifying gaps or areas of disagreement.
- Critical Analysis: Evaluate the strengths and weaknesses of each study. Discuss the methodologies used, the quality of evidence, and the overall contribution of each work to the understanding of the topic.
- Synthesis of Findings: Synthesize the information from different studies to highlight trends, patterns, or areas of consensus in the literature.
- Identification of Gaps: Discuss any gaps or limitations in the existing research and explain how your review contributes to filling these gaps.
- Transition between Sections: Provide smooth transitions between different themes or concepts to maintain the flow of your literature review.
Write and Cite as you go with Paperpal Research. Start now for free.
Conclusion: The conclusion of your literature review should summarize the main findings, highlight the contributions of the review, and suggest avenues for future research.
- Summary of Key Findings: Recap the main findings from the literature and restate how they contribute to your research question or objective.
- Contributions to the Field: Discuss the overall contribution of your literature review to the existing knowledge in the field.
- Implications and Applications: Explore the practical implications of the findings and suggest how they might impact future research or practice.
- Recommendations for Future Research: Identify areas that require further investigation and propose potential directions for future research in the field.
- Final Thoughts: Conclude with a final reflection on the importance of your literature review and its relevance to the broader academic community.
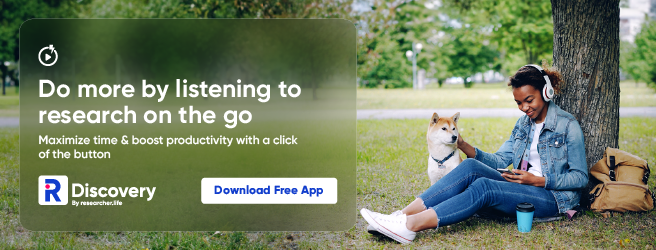
Conducting a literature review
Conducting a literature review is an essential step in research that involves reviewing and analyzing existing literature on a specific topic. It’s important to know how to do a literature review effectively, so here are the steps to follow: 1
Choose a Topic and Define the Research Question:
- Select a topic that is relevant to your field of study.
- Clearly define your research question or objective. Determine what specific aspect of the topic do you want to explore?
Decide on the Scope of Your Review:
- Determine the timeframe for your literature review. Are you focusing on recent developments, or do you want a historical overview?
- Consider the geographical scope. Is your review global, or are you focusing on a specific region?
- Define the inclusion and exclusion criteria. What types of sources will you include? Are there specific types of studies or publications you will exclude?
Select Databases for Searches:
- Identify relevant databases for your field. Examples include PubMed, IEEE Xplore, Scopus, Web of Science, and Google Scholar.
- Consider searching in library catalogs, institutional repositories, and specialized databases related to your topic.
Conduct Searches and Keep Track:
- Develop a systematic search strategy using keywords, Boolean operators (AND, OR, NOT), and other search techniques.
- Record and document your search strategy for transparency and replicability.
- Keep track of the articles, including publication details, abstracts, and links. Use citation management tools like EndNote, Zotero, or Mendeley to organize your references.
Review the Literature:
- Evaluate the relevance and quality of each source. Consider the methodology, sample size, and results of studies.
- Organize the literature by themes or key concepts. Identify patterns, trends, and gaps in the existing research.
- Summarize key findings and arguments from each source. Compare and contrast different perspectives.
- Identify areas where there is a consensus in the literature and where there are conflicting opinions.
- Provide critical analysis and synthesis of the literature. What are the strengths and weaknesses of existing research?
Organize and Write Your Literature Review:
- Literature review outline should be based on themes, chronological order, or methodological approaches.
- Write a clear and coherent narrative that synthesizes the information gathered.
- Use proper citations for each source and ensure consistency in your citation style (APA, MLA, Chicago, etc.).
- Conclude your literature review by summarizing key findings, identifying gaps, and suggesting areas for future research.
Whether you’re exploring a new research field or finding new angles to develop an existing topic, sifting through hundreds of papers can take more time than you have to spare. But what if you could find science-backed insights with verified citations in seconds? That’s the power of Paperpal’s new Research feature!
How to write a literature review faster with Paperpal?
Paperpal, an AI writing assistant, integrates powerful academic search capabilities within its writing platform. With the Research feature, you get 100% factual insights, with citations backed by 250M+ verified research articles, directly within your writing interface with the option to save relevant references in your Citation Library. By eliminating the need to switch tabs to find answers to all your research questions, Paperpal saves time and helps you stay focused on your writing.
Here’s how to use the Research feature:
- Ask a question: Get started with a new document on paperpal.com. Click on the “Research” feature and type your question in plain English. Paperpal will scour over 250 million research articles, including conference papers and preprints, to provide you with accurate insights and citations.
- Review and Save: Paperpal summarizes the information, while citing sources and listing relevant reads. You can quickly scan the results to identify relevant references and save these directly to your built-in citations library for later access.
- Cite with Confidence: Paperpal makes it easy to incorporate relevant citations and references into your writing, ensuring your arguments are well-supported by credible sources. This translates to a polished, well-researched literature review.
The literature review sample and detailed advice on writing and conducting a review will help you produce a well-structured report. But remember that a good literature review is an ongoing process, and it may be necessary to revisit and update it as your research progresses. By combining effortless research with an easy citation process, Paperpal Research streamlines the literature review process and empowers you to write faster and with more confidence. Try Paperpal Research now and see for yourself.
Frequently asked questions
A literature review is a critical and comprehensive analysis of existing literature (published and unpublished works) on a specific topic or research question and provides a synthesis of the current state of knowledge in a particular field. A well-conducted literature review is crucial for researchers to build upon existing knowledge, avoid duplication of efforts, and contribute to the advancement of their field. It also helps researchers situate their work within a broader context and facilitates the development of a sound theoretical and conceptual framework for their studies.
Literature review is a crucial component of research writing, providing a solid background for a research paper’s investigation. The aim is to keep professionals up to date by providing an understanding of ongoing developments within a specific field, including research methods, and experimental techniques used in that field, and present that knowledge in the form of a written report. Also, the depth and breadth of the literature review emphasizes the credibility of the scholar in his or her field.
Before writing a literature review, it’s essential to undertake several preparatory steps to ensure that your review is well-researched, organized, and focused. This includes choosing a topic of general interest to you and doing exploratory research on that topic, writing an annotated bibliography, and noting major points, especially those that relate to the position you have taken on the topic.
Literature reviews and academic research papers are essential components of scholarly work but serve different purposes within the academic realm. 3 A literature review aims to provide a foundation for understanding the current state of research on a particular topic, identify gaps or controversies, and lay the groundwork for future research. Therefore, it draws heavily from existing academic sources, including books, journal articles, and other scholarly publications. In contrast, an academic research paper aims to present new knowledge, contribute to the academic discourse, and advance the understanding of a specific research question. Therefore, it involves a mix of existing literature (in the introduction and literature review sections) and original data or findings obtained through research methods.
Literature reviews are essential components of academic and research papers, and various strategies can be employed to conduct them effectively. If you want to know how to write a literature review for a research paper, here are four common approaches that are often used by researchers. Chronological Review: This strategy involves organizing the literature based on the chronological order of publication. It helps to trace the development of a topic over time, showing how ideas, theories, and research have evolved. Thematic Review: Thematic reviews focus on identifying and analyzing themes or topics that cut across different studies. Instead of organizing the literature chronologically, it is grouped by key themes or concepts, allowing for a comprehensive exploration of various aspects of the topic. Methodological Review: This strategy involves organizing the literature based on the research methods employed in different studies. It helps to highlight the strengths and weaknesses of various methodologies and allows the reader to evaluate the reliability and validity of the research findings. Theoretical Review: A theoretical review examines the literature based on the theoretical frameworks used in different studies. This approach helps to identify the key theories that have been applied to the topic and assess their contributions to the understanding of the subject. It’s important to note that these strategies are not mutually exclusive, and a literature review may combine elements of more than one approach. The choice of strategy depends on the research question, the nature of the literature available, and the goals of the review. Additionally, other strategies, such as integrative reviews or systematic reviews, may be employed depending on the specific requirements of the research.
The literature review format can vary depending on the specific publication guidelines. However, there are some common elements and structures that are often followed. Here is a general guideline for the format of a literature review: Introduction: Provide an overview of the topic. Define the scope and purpose of the literature review. State the research question or objective. Body: Organize the literature by themes, concepts, or chronology. Critically analyze and evaluate each source. Discuss the strengths and weaknesses of the studies. Highlight any methodological limitations or biases. Identify patterns, connections, or contradictions in the existing research. Conclusion: Summarize the key points discussed in the literature review. Highlight the research gap. Address the research question or objective stated in the introduction. Highlight the contributions of the review and suggest directions for future research.
Both annotated bibliographies and literature reviews involve the examination of scholarly sources. While annotated bibliographies focus on individual sources with brief annotations, literature reviews provide a more in-depth, integrated, and comprehensive analysis of existing literature on a specific topic. The key differences are as follows:
Annotated Bibliography | Literature Review | |
Purpose | List of citations of books, articles, and other sources with a brief description (annotation) of each source. | Comprehensive and critical analysis of existing literature on a specific topic. |
Focus | Summary and evaluation of each source, including its relevance, methodology, and key findings. | Provides an overview of the current state of knowledge on a particular subject and identifies gaps, trends, and patterns in existing literature. |
Structure | Each citation is followed by a concise paragraph (annotation) that describes the source’s content, methodology, and its contribution to the topic. | The literature review is organized thematically or chronologically and involves a synthesis of the findings from different sources to build a narrative or argument. |
Length | Typically 100-200 words | Length of literature review ranges from a few pages to several chapters |
Independence | Each source is treated separately, with less emphasis on synthesizing the information across sources. | The writer synthesizes information from multiple sources to present a cohesive overview of the topic. |
References
- Denney, A. S., & Tewksbury, R. (2013). How to write a literature review. Journal of criminal justice education , 24 (2), 218-234.
- Pan, M. L. (2016). Preparing literature reviews: Qualitative and quantitative approaches . Taylor & Francis.
- Cantero, C. (2019). How to write a literature review. San José State University Writing Center .
Paperpal is an AI writing assistant that help academics write better, faster with real-time suggestions for in-depth language and grammar correction. Trained on millions of research manuscripts enhanced by professional academic editors, Paperpal delivers human precision at machine speed.
Try it for free or upgrade to Paperpal Prime , which unlocks unlimited access to premium features like academic translation, paraphrasing, contextual synonyms, consistency checks and more. It’s like always having a professional academic editor by your side! Go beyond limitations and experience the future of academic writing. Get Paperpal Prime now at just US$19 a month!
Related Reads:
- Empirical Research: A Comprehensive Guide for Academics
- How to Write a Scientific Paper in 10 Steps
- How Long Should a Chapter Be?
- How to Use Paperpal to Generate Emails & Cover Letters?
6 Tips for Post-Doc Researchers to Take Their Career to the Next Level
Self-plagiarism in research: what it is and how to avoid it, you may also like, academic integrity vs academic dishonesty: types & examples, dissertation printing and binding | types & comparison , what is a dissertation preface definition and examples , the ai revolution: authors’ role in upholding academic..., the future of academia: how ai tools are..., how to write a research proposal: (with examples..., how to write your research paper in apa..., how to choose a dissertation topic, how to write a phd research proposal, how to write an academic paragraph (step-by-step guide).
University Libraries
Literature review.
- What is a Literature Review?
- What is Its Purpose?
- 1. Select a Topic
- 2. Set the Topic in Context
- 3. Types of Information Sources
- 4. Use Information Sources
- 5. Get the Information
- 6. Organize / Manage the Information
- 7. Position the Literature Review
- 8. Write the Literature Review

A literature review is a comprehensive summary of previous research on a topic. The literature review surveys scholarly articles, books, and other sources relevant to a particular area of research. The review should enumerate, describe, summarize, objectively evaluate and clarify this previous research. It should give a theoretical base for the research and help you (the author) determine the nature of your research. The literature review acknowledges the work of previous researchers, and in so doing, assures the reader that your work has been well conceived. It is assumed that by mentioning a previous work in the field of study, that the author has read, evaluated, and assimiliated that work into the work at hand.
A literature review creates a "landscape" for the reader, giving her or him a full understanding of the developments in the field. This landscape informs the reader that the author has indeed assimilated all (or the vast majority of) previous, significant works in the field into her or his research.
"In writing the literature review, the purpose is to convey to the reader what knowledge and ideas have been established on a topic, and what their strengths and weaknesses are. The literature review must be defined by a guiding concept (eg. your research objective, the problem or issue you are discussing, or your argumentative thesis). It is not just a descriptive list of the material available, or a set of summaries.( http://www.writing.utoronto.ca/advice/specific-types-of-writing/literature-review )
Recommended Reading
- Next: What is Its Purpose? >>
- Last Updated: Oct 2, 2023 12:34 PM
Research Methods
- Getting Started
- Literature Review Research
- Research Design
- Research Design By Discipline
- SAGE Research Methods
- Teaching with SAGE Research Methods
Literature Review
- What is a Literature Review?
- What is NOT a Literature Review?
- Purposes of a Literature Review
- Types of Literature Reviews
- Literature Reviews vs. Systematic Reviews
- Systematic vs. Meta-Analysis
Literature Review is a comprehensive survey of the works published in a particular field of study or line of research, usually over a specific period of time, in the form of an in-depth, critical bibliographic essay or annotated list in which attention is drawn to the most significant works.
Also, we can define a literature review as the collected body of scholarly works related to a topic:
- Summarizes and analyzes previous research relevant to a topic
- Includes scholarly books and articles published in academic journals
- Can be an specific scholarly paper or a section in a research paper
The objective of a Literature Review is to find previous published scholarly works relevant to an specific topic
- Help gather ideas or information
- Keep up to date in current trends and findings
- Help develop new questions
A literature review is important because it:
- Explains the background of research on a topic.
- Demonstrates why a topic is significant to a subject area.
- Helps focus your own research questions or problems
- Discovers relationships between research studies/ideas.
- Suggests unexplored ideas or populations
- Identifies major themes, concepts, and researchers on a topic.
- Tests assumptions; may help counter preconceived ideas and remove unconscious bias.
- Identifies critical gaps, points of disagreement, or potentially flawed methodology or theoretical approaches.
- Indicates potential directions for future research.
All content in this section is from Literature Review Research from Old Dominion University
Keep in mind the following, a literature review is NOT:
Not an essay
Not an annotated bibliography in which you summarize each article that you have reviewed. A literature review goes beyond basic summarizing to focus on the critical analysis of the reviewed works and their relationship to your research question.
Not a research paper where you select resources to support one side of an issue versus another. A lit review should explain and consider all sides of an argument in order to avoid bias, and areas of agreement and disagreement should be highlighted.
A literature review serves several purposes. For example, it
- provides thorough knowledge of previous studies; introduces seminal works.
- helps focus one’s own research topic.
- identifies a conceptual framework for one’s own research questions or problems; indicates potential directions for future research.
- suggests previously unused or underused methodologies, designs, quantitative and qualitative strategies.
- identifies gaps in previous studies; identifies flawed methodologies and/or theoretical approaches; avoids replication of mistakes.
- helps the researcher avoid repetition of earlier research.
- suggests unexplored populations.
- determines whether past studies agree or disagree; identifies controversy in the literature.
- tests assumptions; may help counter preconceived ideas and remove unconscious bias.
As Kennedy (2007) notes*, it is important to think of knowledge in a given field as consisting of three layers. First, there are the primary studies that researchers conduct and publish. Second are the reviews of those studies that summarize and offer new interpretations built from and often extending beyond the original studies. Third, there are the perceptions, conclusions, opinion, and interpretations that are shared informally that become part of the lore of field. In composing a literature review, it is important to note that it is often this third layer of knowledge that is cited as "true" even though it often has only a loose relationship to the primary studies and secondary literature reviews.
Given this, while literature reviews are designed to provide an overview and synthesis of pertinent sources you have explored, there are several approaches to how they can be done, depending upon the type of analysis underpinning your study. Listed below are definitions of types of literature reviews:
Argumentative Review This form examines literature selectively in order to support or refute an argument, deeply imbedded assumption, or philosophical problem already established in the literature. The purpose is to develop a body of literature that establishes a contrarian viewpoint. Given the value-laden nature of some social science research [e.g., educational reform; immigration control], argumentative approaches to analyzing the literature can be a legitimate and important form of discourse. However, note that they can also introduce problems of bias when they are used to to make summary claims of the sort found in systematic reviews.
Integrative Review Considered a form of research that reviews, critiques, and synthesizes representative literature on a topic in an integrated way such that new frameworks and perspectives on the topic are generated. The body of literature includes all studies that address related or identical hypotheses. A well-done integrative review meets the same standards as primary research in regard to clarity, rigor, and replication.
Historical Review Few things rest in isolation from historical precedent. Historical reviews are focused on examining research throughout a period of time, often starting with the first time an issue, concept, theory, phenomena emerged in the literature, then tracing its evolution within the scholarship of a discipline. The purpose is to place research in a historical context to show familiarity with state-of-the-art developments and to identify the likely directions for future research.
Methodological Review A review does not always focus on what someone said [content], but how they said it [method of analysis]. This approach provides a framework of understanding at different levels (i.e. those of theory, substantive fields, research approaches and data collection and analysis techniques), enables researchers to draw on a wide variety of knowledge ranging from the conceptual level to practical documents for use in fieldwork in the areas of ontological and epistemological consideration, quantitative and qualitative integration, sampling, interviewing, data collection and data analysis, and helps highlight many ethical issues which we should be aware of and consider as we go through our study.
Systematic Review This form consists of an overview of existing evidence pertinent to a clearly formulated research question, which uses pre-specified and standardized methods to identify and critically appraise relevant research, and to collect, report, and analyse data from the studies that are included in the review. Typically it focuses on a very specific empirical question, often posed in a cause-and-effect form, such as "To what extent does A contribute to B?"
Theoretical Review The purpose of this form is to concretely examine the corpus of theory that has accumulated in regard to an issue, concept, theory, phenomena. The theoretical literature review help establish what theories already exist, the relationships between them, to what degree the existing theories have been investigated, and to develop new hypotheses to be tested. Often this form is used to help establish a lack of appropriate theories or reveal that current theories are inadequate for explaining new or emerging research problems. The unit of analysis can focus on a theoretical concept or a whole theory or framework.
* Kennedy, Mary M. "Defining a Literature." Educational Researcher 36 (April 2007): 139-147.
All content in this section is from The Literature Review created by Dr. Robert Larabee USC
Robinson, P. and Lowe, J. (2015), Literature reviews vs systematic reviews. Australian and New Zealand Journal of Public Health, 39: 103-103. doi: 10.1111/1753-6405.12393
What's in the name? The difference between a Systematic Review and a Literature Review, and why it matters . By Lynn Kysh from University of Southern California
Systematic review or meta-analysis?
A systematic review answers a defined research question by collecting and summarizing all empirical evidence that fits pre-specified eligibility criteria.
A meta-analysis is the use of statistical methods to summarize the results of these studies.
Systematic reviews, just like other research articles, can be of varying quality. They are a significant piece of work (the Centre for Reviews and Dissemination at York estimates that a team will take 9-24 months), and to be useful to other researchers and practitioners they should have:
- clearly stated objectives with pre-defined eligibility criteria for studies
- explicit, reproducible methodology
- a systematic search that attempts to identify all studies
- assessment of the validity of the findings of the included studies (e.g. risk of bias)
- systematic presentation, and synthesis, of the characteristics and findings of the included studies
Not all systematic reviews contain meta-analysis.
Meta-analysis is the use of statistical methods to summarize the results of independent studies. By combining information from all relevant studies, meta-analysis can provide more precise estimates of the effects of health care than those derived from the individual studies included within a review. More information on meta-analyses can be found in Cochrane Handbook, Chapter 9 .
A meta-analysis goes beyond critique and integration and conducts secondary statistical analysis on the outcomes of similar studies. It is a systematic review that uses quantitative methods to synthesize and summarize the results.
An advantage of a meta-analysis is the ability to be completely objective in evaluating research findings. Not all topics, however, have sufficient research evidence to allow a meta-analysis to be conducted. In that case, an integrative review is an appropriate strategy.
Some of the content in this section is from Systematic reviews and meta-analyses: step by step guide created by Kate McAllister.
- << Previous: Getting Started
- Next: Research Design >>
- Last Updated: Jul 15, 2024 10:34 AM
- URL: https://guides.lib.udel.edu/researchmethods
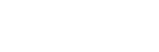
Literature Reviews
- Getting started
What is a literature review?
Why conduct a literature review, stages of a literature review, lit reviews: an overview (video), check out these books.
- Types of reviews
- 1. Define your research question
- 2. Plan your search
- 3. Search the literature
- 4. Organize your results
- 5. Synthesize your findings
- 6. Write the review
- Artificial intelligence (AI) tools
- Thompson Writing Studio This link opens in a new window
- Need to write a systematic review? This link opens in a new window
Guide Owner

Contact a Librarian
Ask a Librarian
Definition: A literature review is a systematic examination and synthesis of existing scholarly research on a specific topic or subject.
Purpose: It serves to provide a comprehensive overview of the current state of knowledge within a particular field.
Analysis: Involves critically evaluating and summarizing key findings, methodologies, and debates found in academic literature.
Identifying Gaps: Aims to pinpoint areas where there is a lack of research or unresolved questions, highlighting opportunities for further investigation.
Contextualization: Enables researchers to understand how their work fits into the broader academic conversation and contributes to the existing body of knowledge.

tl;dr A literature review critically examines and synthesizes existing scholarly research and publications on a specific topic to provide a comprehensive understanding of the current state of knowledge in the field.
What is a literature review NOT?
❌ An annotated bibliography
❌ Original research
❌ A summary
❌ Something to be conducted at the end of your research
❌ An opinion piece
❌ A chronological compilation of studies
The reason for conducting a literature review is to:
What has been written about your topic? What is the evidence for your topic? What methods, key concepts, and theories relate to your topic? Are there current gaps in knowledge or new questions to be asked? | |
Bring your reader up to date Further your reader's understanding of the topic | |
Provide evidence of... - your knowledge on the topic's theory - your understanding of the research process - your ability to critically evaluate and analyze information - that you're up to date on the literature |

Literature Reviews: An Overview for Graduate Students
While this 9-minute video from NCSU is geared toward graduate students, it is useful for anyone conducting a literature review.

Writing the literature review: A practical guide
Available 3rd floor of Perkins

Writing literature reviews: A guide for students of the social and behavioral sciences
Available online!

So, you have to write a literature review: A guided workbook for engineers

Telling a research story: Writing a literature review

The literature review: Six steps to success

Systematic approaches to a successful literature review
Request from Duke Medical Center Library

Doing a systematic review: A student's guide
- Next: Types of reviews >>
- Last Updated: Aug 20, 2024 3:37 PM
- URL: https://guides.library.duke.edu/litreviews

Services for...
- Faculty & Instructors
- Graduate Students
- Undergraduate Students
- International Students
- Patrons with Disabilities

- Harmful Language Statement
- Re-use & Attribution / Privacy
- Support the Libraries

- Find My Rep
You are here
Seven Steps to a Comprehensive Literature Review A Multimodal and Cultural Approach
- Anthony J. Onwuegbuzie
- Rebecca Frels - Lamar University, USA
- Description
What makes this book unique:
- Focuses on multimodal texts and settings such as observations, documents, social media, experts in the field and secondary data so that your review covers the full research environment
- Puts mixed methods at the centre of the process
- Shows you how to synthesize information thematically, rather than merely summarize the existing literature and findings
- Brings culture into the process to help you address bias and understand the role of knowledge interpretation, guiding you through
- Teaches the CORE of the literature review – Critical thinking, Organization, Reflections and Evaluation – and provides a guide for reflexivity at the end of each of the seven steps
- Visualizes the steps with roadmaps so you can track progress and self-evaluate as you learn the steps
This book is the essential best practices guide for students and researchers, providing the understanding and tools to approach both the ‘how’ and ‘why’ of a rigorous, comprehensive, literature review.
This is by far the most comprehensive text on how to do comprehensive literature reviews! Onwuegbuzie and Frels skilfully demonstrate that review has a methodology of its own. Both novice and experienced scholars will benefit from detailed examples and step-by-step demonstrations of ways to maximize the effectiveness of literature reviews to build new theories and develop better explanations of behaviours and outcomes.
This is the most comprehensive and user-friendly book I’ve seen on how to conduct a literature review. The authors take the distinction of qualitative, quantitative, and mixed methods research seriously, showing how each adds something important and how being open-minded results in the use of literature based on all three approaches. Overall, the book provides a process theory of literature review, that is done before, during, and after each research study. It is a must read for both PhD students and research faculty.
With noteworthy scope of content, this book is a must-have resource for beginning and experienced researchers alike. In addition to its effective pedagogical features such as visuals and end of chapter questions, this resource enables researchers to make informed decisions about the purposes of and procedures for undertaking a literature review. In so doing, the authors innovate and advance our understandings of the processes and products involved in a comprehensive literature review and provide practical guidance for each of the steps. I have been seeking such a book and plan to make this required reading for the graduate students I instruct, mentor, and supervise.
Seven Steps to a Comprehensive Literature Review is a comprehensive text book written to instruct master’s-level students, doctoral-level students, and new and experienced researchers in the process of writing a comprehensive literature review... Hopefully, this book will become an important text used by instructors as they guide college students into the writing of the literature review.
Sadly this book never arrived despite me being very interested to adopt for my MSc students dissertation stage.
The literature review is one of the toughest parts of any proposal (or postgraduate piece of work) for students to complete successfully because it asks the student to engage with the theory they will be using from the perspective of ideas alone. IT also asks the student to investigate other academics' work in a manner that they haven't really experienced before. All these "firsts" make the literature review a very confusing and oftentimes daunting process. Fortunately, "Seven Steps" provides the specific guidance that so many students need to navigate this difficult process. The systematic way in which the book approaches a topic that can be said to change with each application (e.g. How do you go about it? What to include? What to leave out? and most importantly, Why?) is indispensable for anyone teaching students new to postgraduate work, or for researchers looking for an alternative approach to a process they are otherwise well-acquainted with.
Very accessible book for students who wish to increase their capabilites in working at the front end of their papers.
Comprehensive, well structured book, which will be very useful to students planning a literature review.
this book is more relevant for the MSc students. it will be a good supplement for the student who wants to go a little further
it was actually a little more complex than I was hoping for. the text is dense and it is big book. for my BSc students it is jut a little too much
Preview this book
Sample materials & chapters.
Chapter 1: Foundations of the Literature Review
For instructors
Select a purchasing option.
- Electronic Order Options VitalSource Amazon Kindle Google Play eBooks.com Kobo
Related Products


- University of Texas Libraries
Literature Reviews
Steps in the literature review process.
- What is a literature review?
- Define your research question
- Determine inclusion and exclusion criteria
- Choose databases and search
- Review Results
- Synthesize Results
- Analyze Results
- Librarian Support
- Artificial Intelligence (AI) Tools
- You may need to some exploratory searching of the literature to get a sense of scope, to determine whether you need to narrow or broaden your focus
- Identify databases that provide the most relevant sources, and identify relevant terms (controlled vocabularies) to add to your search strategy
- Finalize your research question
- Think about relevant dates, geographies (and languages), methods, and conflicting points of view
- Conduct searches in the published literature via the identified databases
- Check to see if this topic has been covered in other discipline's databases
- Examine the citations of on-point articles for keywords, authors, and previous research (via references) and cited reference searching.
- Save your search results in a citation management tool (such as Zotero, Mendeley or EndNote)
- De-duplicate your search results
- Make sure that you've found the seminal pieces -- they have been cited many times, and their work is considered foundational
- Check with your professor or a librarian to make sure your search has been comprehensive
- Evaluate the strengths and weaknesses of individual sources and evaluate for bias, methodologies, and thoroughness
- Group your results in to an organizational structure that will support why your research needs to be done, or that provides the answer to your research question
- Develop your conclusions
- Are there gaps in the literature?
- Where has significant research taken place, and who has done it?
- Is there consensus or debate on this topic?
- Which methodological approaches work best?
- For example: Background, Current Practices, Critics and Proponents, Where/How this study will fit in
- Organize your citations and focus on your research question and pertinent studies
- Compile your bibliography
Note: The first four steps are the best points at which to contact a librarian. Your librarian can help you determine the best databases to use for your topic, assess scope, and formulate a search strategy.
Videos Tutorials about Literature Reviews
This 4.5 minute video from Academic Education Materials has a Creative Commons License and a British narrator.
Recommended Reading
- Last Updated: Aug 26, 2024 5:59 AM
- URL: https://guides.lib.utexas.edu/literaturereviews
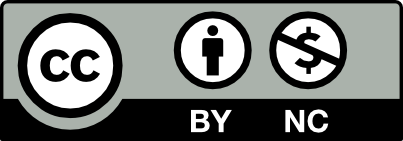
Ohio State nav bar
The Ohio State University
- BuckeyeLink
- Find People
- Search Ohio State
Literature Review
What exactly is a literature review.
- Critical Exploration and Synthesis: It involves a thorough and critical examination of existing research, going beyond simple summaries to synthesize information.
- Reorganizing Key Information: Involves structuring and categorizing the main ideas and findings from various sources.
- Offering Fresh Interpretations: Provides new perspectives or insights into the research topic.
- Merging New and Established Insights: Integrates both recent findings and well-established knowledge in the field.
- Analyzing Intellectual Trajectories: Examines the evolution and debates within a specific field over time.
- Contextualizing Current Research: Places recent research within the broader academic landscape, showing its relevance and relation to existing knowledge.
- Detailed Overview of Sources: Gives a comprehensive summary of relevant books, articles, and other scholarly materials.
- Highlighting Significance: Emphasizes the importance of various research works to the specific topic of study.
How do Literature Reviews Differ from Academic Research Papers?
- Focus on Existing Arguments: Literature reviews summarize and synthesize existing research, unlike research papers that present new arguments.
- Secondary vs. Primary Research: Literature reviews are based on secondary sources, while research papers often include primary research.
- Foundational Element vs. Main Content: In research papers, literature reviews are usually a part of the background, not the main focus.
- Lack of Original Contributions: Literature reviews do not introduce new theories or findings, which is a key component of research papers.
Purpose of Literature Reviews
- Drawing from Diverse Fields: Literature reviews incorporate findings from various fields like health, education, psychology, business, and more.
- Prioritizing High-Quality Studies: They emphasize original, high-quality research for accuracy and objectivity.
- Serving as Comprehensive Guides: Offer quick, in-depth insights for understanding a subject thoroughly.
- Foundational Steps in Research: Act as a crucial first step in conducting new research by summarizing existing knowledge.
- Providing Current Knowledge for Professionals: Keep professionals updated with the latest findings in their fields.
- Demonstrating Academic Expertise: In academia, they showcase the writer’s deep understanding and contribute to the background of research papers.
- Essential for Scholarly Research: A deep understanding of literature is vital for conducting and contextualizing scholarly research.
A Literature Review is Not About:
- Merely Summarizing Sources: It’s not just a compilation of summaries of various research works.
- Ignoring Contradictions: It does not overlook conflicting evidence or viewpoints in the literature.
- Being Unstructured: It’s not a random collection of information without a clear organizing principle.
- Avoiding Critical Analysis: It doesn’t merely present information without critically evaluating its relevance and credibility.
- Focusing Solely on Older Research: It’s not limited to outdated or historical literature, ignoring recent developments.
- Isolating Research: It doesn’t treat each source in isolation but integrates them into a cohesive narrative.
Steps Involved in Conducting a Research Literature Review (Fink, 2019)
1. choose a clear research question., 2. use online databases and other resources to find articles and books relevant to your question..
- Google Scholar
- OSU Library
- ERIC. Index to journal articles on educational research and practice.
- PsycINFO . Citations and abstracts for articles in 1,300 professional journals, conference proceedings, books, reports, and dissertations in psychology and related disciplines.
- PubMed . This search system provides access to the PubMed database of bibliographic information, which is drawn primarily from MEDLINE, which indexes articles from about 3,900 journals in the life sciences (e.g., health, medicine, biology).
- Social Sciences Citation Index . A multidisciplinary database covering the journal literature of the social sciences, indexing more than 1,725 journals across 50 social sciences disciplines.
3. Decide on Search Terms.
- Pick words and phrases based on your research question to find suitable materials
- You can start by finding models for your literature review, and search for existing reviews in your field, using “review” and your keywords. This helps identify themes and organizational methods.
- Narrowing your topic is crucial due to the vast amount of literature available. Focusing on a specific aspect makes it easier to manage the number of sources you need to review, as it’s unlikely you’ll need to cover everything in the field.
- Use AND to retrieve a set of citations in which each citation contains all search terms.
- Use OR to retrieve citations that contain one of the specified terms.
- Use NOT to exclude terms from your search.
- Be careful when using NOT because you may inadvertently eliminate important articles. In Example 3, articles about preschoolers and academic achievement are eliminated, but so are studies that include preschoolers as part of a discussion of academic achievement and all age groups.
4. Filter out articles that don’t meet criteria like language, type, publication date, and funding source.
- Publication language Example. Include only studies in English.
- Journal Example. Include all education journals. Exclude all medical journals.
- Author Example. Include all articles by Andrew Hayes.
- Setting Example. Include all studies that take place in family settings. Exclude all studies that take place in the school setting.
- Participants or subjects Example. Include children that are younger than 6 years old.
- Program/intervention Example. Include all programs that are teacher-led. Exclude all programs that are learner-initiated.
- Research design Example. Include only longitudinal studies. Exclude cross-sectional studies.
- Sampling Example. Include only studies that rely on randomly selected participants.
- Date of publication Example. Include only studies published from January 1, 2010, to December 31, 2023.
- Date of data collection Example. Include only studies that collected data from 2010 through 2023. Exclude studies that do not give dates of data collection.
- Duration of data collection Example. Include only studies that collect data for 12 months or longer.
5. Evaluate the methodological quality of the articles, including research design, sampling, data collection, interventions, data analysis, results, and conclusions.
- Maturation: Changes in individuals due to natural development may impact study results, such as intellectual or emotional growth in long-term studies.
- Selection: The method of choosing and assigning participants to groups can introduce bias; random selection minimizes this.
- History: External historical events occurring simultaneously with the study can bias results, making it hard to isolate the study’s effects.
- Instrumentation: Reliable data collection tools are essential to ensure accurate findings, especially in pretest-posttest designs.
- Statistical Regression: Selection based on extreme initial measures can lead to misleading results due to regression towards the mean.
- Attrition: Loss of participants during a study can bias results if those remaining differ significantly from those who dropped out.
- Reactive Effects of Testing: Pre-intervention measures can sensitize participants to the study’s aims, affecting outcomes.
- Interactive Effects of Selection: Unique combinations of intervention programs and participants can limit the generalizability of findings.
- Reactive Effects of Innovation: Artificial experimental environments can lead to uncharacteristic behavior among participants.
- Multiple-Program Interference: Difficulty in isolating an intervention’s effects due to participants’ involvement in other activities or programs.
- Simple Random Sampling : Every individual has an equal chance of being selected, making this method relatively unbiased.
- Systematic Sampling : Selection is made at regular intervals from a list, such as every sixth name from a list of 3,000 to obtain a sample of 500.
- Stratified Sampling : The population is divided into subgroups, and random samples are then taken from each subgroup.
- Cluster Sampling : Natural groups (like schools or cities) are used as batches for random selection, both at the group and individual levels.
- Convenience Samples : Selection probability is unknown; these samples are easy to obtain but may not be representative unless statistically validated.
- Study Power: The ability of a study to detect an effect, if present, is known as its power. Power analysis helps identify a sample size large enough to detect this effect.
- Test-Retest Reliability: High correlation between scores obtained at different times, indicating consistency over time.
- Equivalence/Alternate-Form Reliability: The degree to which two different assessments measure the same concept at the same difficulty level.
- Homogeneity: The extent to which all items or questions in a measure assess the same skill, characteristic, or quality.
- Interrater Reliability: Degree of agreement among different individuals assessing the same item or concept.
- Content Validity: Measures how thoroughly and appropriately a tool assesses the skills or characteristics it’s supposed to measure. Face Validity: Assesses whether a measure appears effective at first glance in terms of language use and comprehensiveness. Criterion Validity: Includes predictive validity (forecasting future performance) and concurrent validity (agreement with already valid measures). Construct Validity: Experimentally established to show that a measure effectively differentiates between people with and without certain characteristics.
- Relies on factors like the scale (categorical, ordinal, numerical) of independent and dependent variables, the count of these variables, and whether the data’s quality and characteristics align with the chosen statistical method’s assumptions.
6. Use a standard form for data extraction, train reviewers if needed, and ensure quality.
7. interpret the results, using your experience and the literature’s quality and content. for a more detailed analysis, a meta-analysis can be conducted using statistical methods to combine study results., 8. produce a descriptive review or perform a meta-analysis..
- Example: Bryman, A. (2007). Effective leadership in higher education: A literature review. Studies in higher education, 32(6), 693-710.
- Clarify the objectives of the analysis.
- Set explicit criteria for including and excluding studies.
- Describe in detail the methods used to search the literature.
- Search the literature using a standardized protocol for including and excluding studies.
- Use a standardized protocol to collect (“abstract”) data from each study regarding study purposes, methods, and effects (outcomes).
- Describe in detail the statistical method for pooling results.
- Report results, conclusions, and limitations.
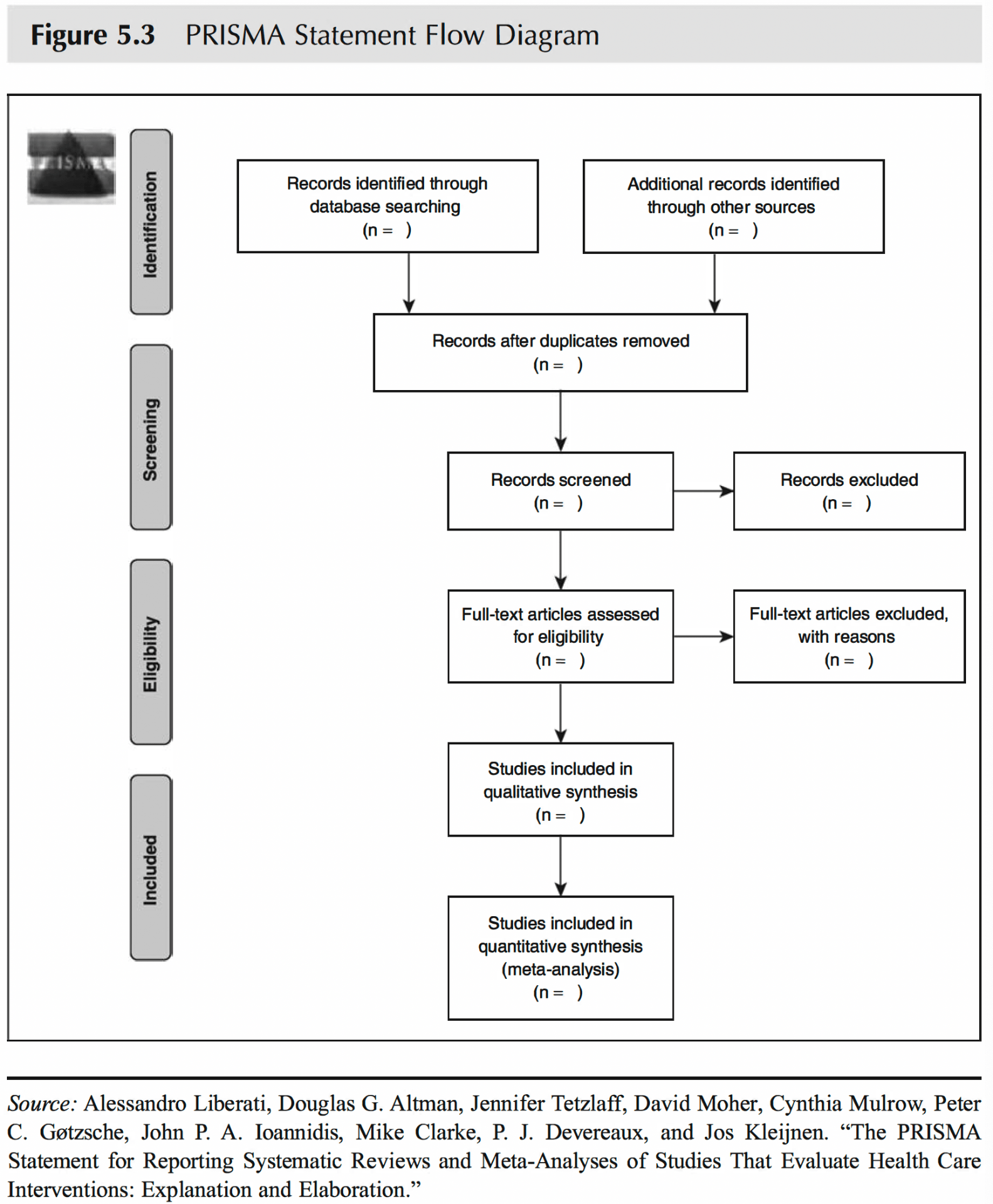
- Example: Yu, Z. (2023). A meta-analysis of the effect of virtual reality technology use in education. Interactive Learning Environments, 31 (8), 4956-4976.
- Essential and Multifunctional Bibliographic Software: Tools like EndNote, ProCite, BibTex, Bookeeper, Zotero, and Mendeley offer more than just digital storage for references; they enable saving and sharing search strategies, directly inserting references into reports and scholarly articles, and analyzing references by thematic content.
- Comprehensive Literature Reviews: Involve supplementing electronic searches with a review of references in identified literature, manual searches of references and journals, and consulting experts for both unpublished and published studies and reports.
- One of the most famous reporting checklists is the Consolidated Standards of Reporting Trials ( CONSORT ). CONSORT consists of a checklist and flow diagram. The checklist includes items that need to be addressed in the report.
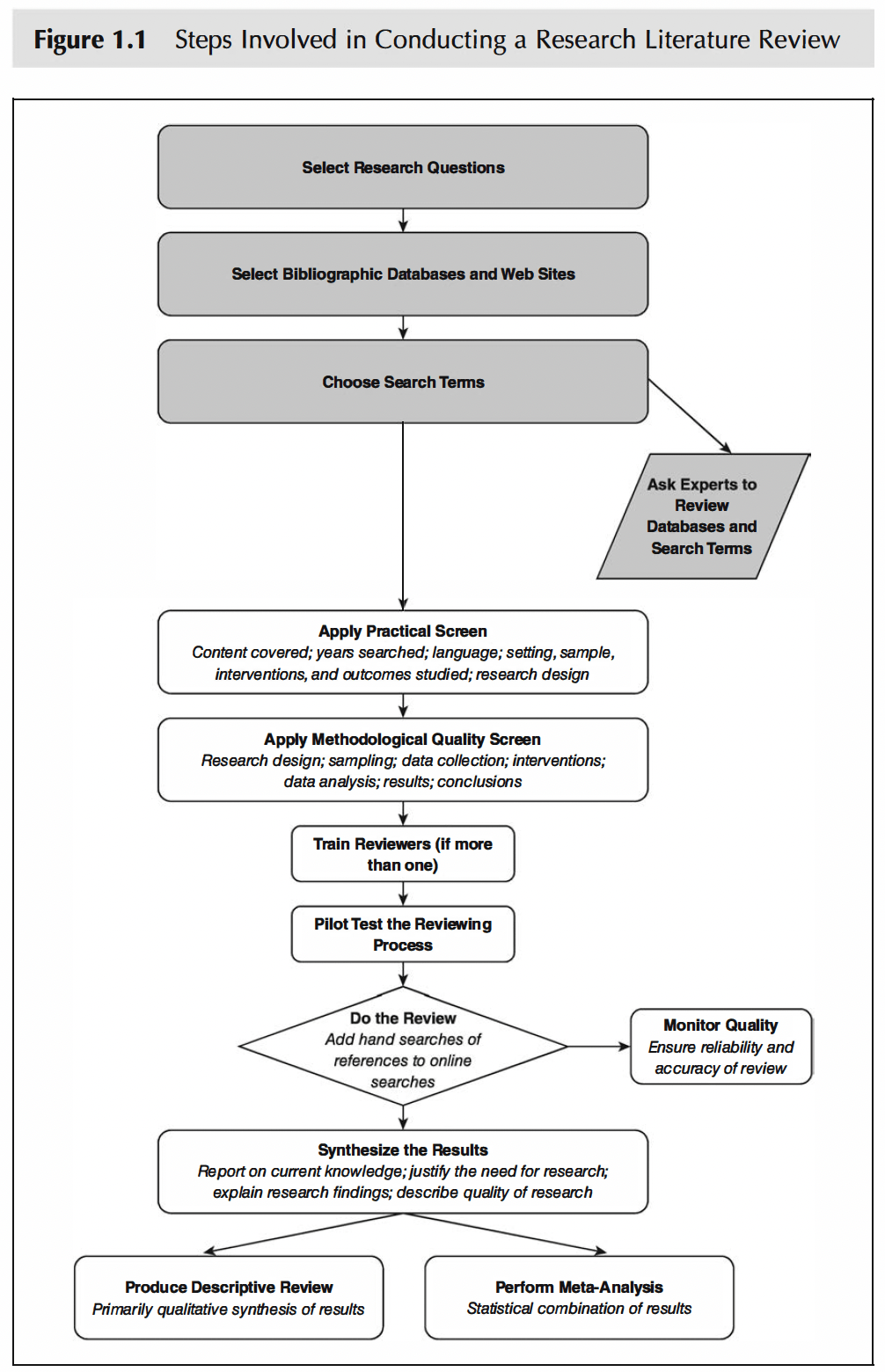
References:
Bryman, A. (2007). Effective leadership in higher education: A literature review. Studies in higher education , 32 (6), 693-710.
Fink, A. (2019). Conducting research literature reviews: From the internet to paper . Sage publications.
Yu, Z. (2023). A meta-analysis of the effect of virtual reality technology use in education. Interactive Learning Environments, 31 (8), 4956-4976.

An official website of the United States government
The .gov means it’s official. Federal government websites often end in .gov or .mil. Before sharing sensitive information, make sure you’re on a federal government site.
The site is secure. The https:// ensures that you are connecting to the official website and that any information you provide is encrypted and transmitted securely.
- Publications
- Account settings
Preview improvements coming to the PMC website in October 2024. Learn More or Try it out now .
- Advanced Search
- Journal List
- v.15(11); 2023 Nov
- PMC10748445

A Comprehensive Literature Review of Borderline Personality Disorder: Unraveling Complexity From Diagnosis to Treatment
Sanskar mishra.
1 Medicine, Jawaharlal Nehru Medical College, Datta Meghe Institute of Higher Education and Research, Wardha, IND
Alka Rawekar
2 Physiology, Jawaharlal Nehru Medical College, Datta Meghe Institute of Higher Education and Research, Wardha, IND
Bhagyesh Sapkale
Borderline personality disorder (BPD) is a severe mental illness marked by unpredictable feelings, behaviors, and relationships. Symptoms like emotional instability, impulsivity, and poor social connections are the basis for diagnostic criteria. A noteworthy discovery highlights the clinical overlap between BPD and several psychotic disorders by arguing that BPD and psychotic symptoms raise the risk of psychopathology. According to neuroimaging evidence, structural and functional brain changes, notably in regions controlling affective regulation and impulse control, are seen in BPD patients. Adolf Stern, a psychoanalyst, used the word "borderline" in 1938 to describe patients who exhibited increased symptoms during therapy and displayed masochistic tendencies. Modern BPD research has highlighted the complexity of symptoms like boredom, a former diagnostic criterion associated with feelings of emptiness.
Though there are still unanswered problems regarding its precise, practical components, the treatment technique known as Schema therapy (ST) has shown promise in treating BPD. It's interesting to note that BPD displays complex relationships with other illnesses; for instance, some neurochemical pathways coincide with those in bulimia nervosa, pointing to a deeper level of interconnection. Concerning diagnosis, BPD's defining symptoms include, among others, the fear of abandonment, identity disruption, and recurrent suicidal conduct. The range of treatment options includes pharmacological interventions and psychotherapies like dialectical behavior therapy (DBT). Even though antidepressants like selective serotonin reuptake inhibitors (SSRIs) are routinely prescribed, research on their efficacy is ongoing, underlining the significance of thorough treatment planning. In conclusion, BPD continues to be a complex condition that calls for early detection, especially considering that it usually manifests in adolescence. While many patients report symptom relief, lingering problems still exist, emphasizing the value of comprehensive and personalized treatment strategies.
Introduction and background
A psychiatric disease known as borderline personality disorder (BPD) is characterized by unpredictable mood, conduct, and interpersonal interactions [ 1 ]. There is uncertainty about BPD's origin. Based on the symptoms, clinicians form a diagnosis. Emotional instability, worthlessness, insecurity, impulsivity, and deteriorated social interactions are symptoms. According to studies, 10% of BPD patients also had bipolar I disease, and 10% had bipolar II disorder. About 10% of individuals with bipolar I had the condition, and about 20% of individuals with bipolar II had it [ 2 ]. BPD is a long-term mental health disorder characterized by suicidal conduct, unstable mood and relationships, and extreme impulsivity [ 3 ]. Typically, BPD patients attempt suicide three times in their lifetimes, most frequently by overdose; non-suicidal self-injury (NSSI) is another self-harm activity prevalent in BPD [ 4 ]. The Diagnostic and Statistical Manual of Mental Disorders (DSM), third edition's classification of BPD as an illness of the mind in 1980 brought about clinical and academic attention [ 5 ]. NSSI typically manifests as little wounds on the arms and wrists. NSSI, however, does not have a suicide motive; instead, BPD patients cut themselves compulsively to cope with uncomfortable inner states. Cutting is a way to release emotional stress, not a sign of a death wish [ 4 , 5 ].
The availability of specific efficient psychotherapies, the potential over-prescription of drugs with minimal benefits, and the danger of medically significant adverse effects make identifying BPD clinically relevant. BPD's three core symptom domains are impulsivity, affective dysregulation, and cognitive-perceptual symptoms (paranoia and dissociation). In Western nations, 0.4-3.9% of people suffer from a crippling psychiatric disease called borderline personality disorder (BPD) [ 6 ]. Regulatory bodies have not authorized any drugs to treat BPD. Despite this, up to 96% of BPD patients take at least one psychotropic drug [ 6 ]. Antipsychotics do not significantly affect mood instability, cognitive-perceptual signs and symptoms, or overall functioning [ 7 ]. They also had a minor to moderate impact on rage. The findings could not be used to evaluate individual antipsychotics because they were combined. People with borderline personality disorder are typically treated with psychotherapy [ 8 ]. According to the Diagnostic and Statistical Manual of Mental Disorders, Fifth Edition (DSM-5) model for personality disorders, high levels of disinhibition are a common feature of both borderline personality disorder and antisocial personality disorder; high levels of negative affectivity and elevated levels of antagonism are also associated with BPD and antisocial personality disorder, respectively [ 9 ]. BPD sufferers do engage in self-harming behavior [ 10 ]. Among adults, 2.7% have BPD. Addiction or substance use issues are experienced once in their lifetime by 78% of people with BPD [ 9 ]. Those with BPD who use drugs have increased levels of impulsivity and clinical instability. They exhibit more suicidal behavior, frequently leave treatment, and have shorter abstinence periods [ 11 ]. A unique therapeutic approach is necessary when borderline personality disorder and addiction are present.
Search methodology
The study aimed to conduct an exhaustive literature review on borderline personality disorder (BPD). The focus was placed on the disease's onset, diagnostic standards, signs, symptoms, treatment, and other aspects, focusing on its history, neurological foundations, and related comorbidities. Major databases like PubMed, PsycINFO, Google Scholar, Cochrane Library, and Web of Science were used. Searches included a variety of terms, including "borderline personality disorder" and "diagnosis,", as well as more specific terms like "neurological basis" and "adolescent onset". We set the study period between 1990 and 2022 to thoroughly synthesize historical and modern findings. The inclusion criteria were strict; only research focusing on BPD symptoms, treatments, historical background, neurological alterations, and comorbidities was considered. After careful removal of duplicates, non-English entries, opinion-based articles, and research with hazy procedures from an initial discovery of 1,500 articles, we ultimately selected 400 studies. The selected papers provide details about the authors, the study's goals, demographic information from the sample, and the key findings. However, there were certain restrictions. Due to database indexing limitations, not all pertinent papers may have been included, and there may be publication bias in favor of research with notable findings. Yet this exacting process resulted in a significant synthesis of BPD. The condensed information provided a more transparent comprehension of the complexity of BPD, illuminating various treatment options and pointing out areas that need further research. The Prisma flow diagram is shown in Figure Figure1 1 .

PRISMA: Preferred Reporting Items for Systematic Reviews and Meta-Analyses
Borderline personality disorder
A mental illness is marked by unpredictability in mood, behavior, and relationships. Uncertainty surrounds the origin of BPD. Symptoms are used to form a diagnosis. Emotional instability, worthlessness, insecurity, impulsivity, and deteriorated social interactions are symptoms [ 12 ]. The presence of both BPD and psychotic signs is an indication of a serious psychopath and a risk for adverse outcomes (such as suicidality), as there are more similarities than differences between the symptoms in those suffering from psychotic disorders and auditory verbal illusions, especially in BPD sufferers [ 13 ]. BPD patients have structural and functional brain changes, especially in brain areas related to impulse control and emotional and cognitive regulation [ 14 ]. Specialized psychotherapies have focused on beliefs concerning the causes and variables that maintain BPD, and they have published thorough protocols on how to treat BPD, use therapeutic methods, and manage the therapeutic alliance [ 15 ]. One of the most common DSM-5 illnesses is post-traumatic stress disorder (PTSD), with a lifetime prevalence of 10% [ 16 ].
Psychoanalyst Adolf Stern used the term "borderline" for the first time in 1938. The word was used to characterize a group of individuals whose problems became worse while they were receiving therapy, exhibiting a rigid mentality and masochistic behavior, suggesting an attempt to defend against any imagined changes in the outside world or within the person [ 17 ]. BPD discusses boredom reactivity and its relationship with emptiness. DSM previously linked boredom reactivity to BPD but later removed it [ 18 ]. Research has discovered that Schema therapy (ST) successfully treats BPD [ 19 ]. However, very little is known about how treatment works for people with BPD, mainly which specific ST components are effective or ineffective in their eyes. Adolescents with BPD do not always recover, even if they frequently shed certain BPD-related features with time. Any personality disorder with high levels of adolescent symptoms will negatively impact functioning over the course of the next ten to twenty years; these consequences are frequently more pronounced or persistent than those associated with disorders associated with Axis I [ 20 ]. A spectrum connection between borderline personality disorder and schizophrenia was implausible. Except for the periodic lapses in reality testing, borderline patients were intensely emotional and interpersonally needy [ 21 ]. Compared to women with borderline personality disorder (BPD) or bulimia nervosa/bulimia spectrum disorder (BN/BSD-BPD), women with BN/BSD-BPD showed significantly lower levels of serotonin and monoamine oxidase activity (HC). When compared to women with BN/BSD and HC, women with BN/BSD-BPD also showed higher levels of brain-derived neurotrophic factor, alterations in the methylation of the glucocorticoid receptor gene promoter (NR3C1), and dopamine receptor gene promoter methylation [ 22 ]. Both complex post-traumatic stress disorder (cPTSD) and perinatal BPD are linked to severe impairments in interpersonal functioning and an increased likelihood of psychopathology being passed down through generations [ 23 ].
Diagnosis of borderline personality disorder
BPD, a mental health disease that begins in early adulthood, is characterized by chronic instability in relationships, one's self-image, emotions, and impulsive conduct. At least five of the given nine signs must be present for a person to be diagnosed with the condition: ongoing empty feelings, intense or inappropriate anger, fear of abandonment, unstable interpersonal relationships, identity confusion, impulsivity, recurrent suicidal thoughts, emotional instability, and occasionally psychotic-like thinking or dissociation [ 24 ]. BPD covers a wide range of emotional and social difficulties. The dread of abandonment, unstable interpersonal connections that oscillate between idealization and devaluation, a shaky self-image, impulsive behaviors, and frequent suicidal thoughts are among its main symptoms. Rapid mood swings, emptiness, unrestrained wrath, and occasionally skewed perceptions or dissociation are additional symptoms that BPD sufferers may encounter [ 25 ]. These symptoms highlight the difficulties BPD sufferers have. Symptoms of BPD are shown in Table Table1 1 .
BPD: borderline personality disorder
Symptom number | Description of symptom |
i | Fear of abandonment |
ii | Unstable intimate relationships |
iii | Identification disorder |
iv | Impulsivity |
v | Repeated suicide attempts |
vi | Emotional instability |
vii | Feelings of desolation that persist |
viii | Severe, unreasonable rage |
ix | Extreme dissociation or quasi-psychotic thoughts |
Symptoms of BPD
Treatment of borderline personality disorder
The most commonly given drugs for BPD are fluoxetine, selective serotonin reuptake inhibitors (SSRIs), and citalopram, despite a shortage of research to support their usage [ 26 ]. It was shown that the most common correlation between giving antidepressants to individuals with BPD is comorbidity for affective disorders [ 26 ]. Early childhood impacts on the development of BPD's psychopathology include parenthood-related issues such as dysfunctional parenting, parenting philosophies, and parenting psychopathology [ 27 ]. Staged therapy designs, additional treatments, and technology-based interventions could be beneficial in cases where developing cost-effective interventions is necessary when people are newly diagnosed or are awaiting full-package therapy (or both) or when a particular deficit needs to be addressed in the context of ongoing treatment [ 28 ]. Furthermore, considering the growing body of research indicating that BPD is a diagnosable disorder in teenagers, treatments aimed at younger demographics constitute an important and required step. Table Table2 2 displays the treatments available for BPD.
DBT: dialectical behavior therapy; MBT: mentalization-based therapy; TFP: transference-focused psychotherapy; ST: Schema therapy
Type of treatment | Description | Purpose/Outcome |
Psychotherapy | ||
Dialectical behavior therapy (DBT) | A type of cognitive-behavioral therapy explicitly developed for BPD. It incorporates interdependence, emotion control, awareness, and distress tolerance. | Addresses self-harm behaviours, improves emotional regulation and enhances interpersonal relationships. |
Mentalization-based therapy (MBT) | It focuses on recognizing and understanding the feelings and thoughts in oneself and others. | It helps patients understand their own emotions and the emotions of others, improving interpersonal relationships. |
Transference-focused psychotherapy (TFP) | Focuses on understanding and resolving emotions and interpersonal issues through the patient-therapist connection. | It helps individuals understand their emotions and change problematic patterns of interaction. |
Schema therapy (ST) | Combines elements of cognitive, behavioural, and psychodynamic therapies. Focuses on changing negative life patterns. | It aims to identify and change maladaptive life patterns. |
Medications | ||
Antidepressants | Includes selective serotonin reuptake inhibitors (SSRIs) and others. | It can help with mood swings, irritability, and feelings of emptiness. |
Antipsychotics | They were often used in low doses. | It can reduce symptoms of anger, impulsivity, and brief psychotic episodes. |
Mood stabilizers | Such as lithium or certain anticonvulsant medications. | It can help stabilize mood swings and reduce impulsivity. |
Other treatments | ||
Hospitalization | They were often used in severe symptoms or if there's a risk of self-harm. | Provides a safe environment for stabilization and intensive treatment. |
Group therapy | It provides a place to share experiences and coping techniques. | Encourages understanding and support among peers with similar challenges. |
Psychotherapy, such as dialectical behavior therapy (DBT) for emotional regulation and relationship improvement and mentalization-based therapy (MBT) to understand one's own feelings as well as those of others, is the mainstay of treatment for BPD [ 29 ]. While Schema therapy targets dysfunctional life patterns, transference-focused psychotherapy (TFP) uses therapist-patient interaction to gain emotional insight [ 8 ]. Antipsychotic drugs control rage and impulsivity; mood stabilizers target mood swings; and antidepressants handle mood and irritability [ 8 ]. Hospitalization guarantees safety and intensive care in severe conditions. In group therapy, participants foster peer support and develop common coping mechanisms. Before beginning any treatment, it is essential to seek professional guidance. Borderline personality disorder (BPD) often manifests throughout adolescence; early identification and treatment are crucial [ 8 , 29 ]. While many BPD sufferers notice considerable improvements with time, it's essential to remember that a sizable percentage may continue to struggle with lingering symptoms as they age [ 30 ]. For prognosis, treatment planning, and patient counseling, being aware of these little differences is crucial from a professional standpoint. The analysis of the studies included is shown in Table Table3 3 .
EMDR: eye movement desensitization and reprocessing; DBT: dialectical behaviour therapy; PTSD: post-traumatic stress disorder
Author(s) | Year | Main characteristics |
Mezei et al. [ ] | 2020 | 1. Examines BPD through a developmental psychopathology lens. |
Zimmerman et al. [ ] | 2013 | 2. Investigates the connection and distinctions between BPD and bipolar disorder. |
Paris et al. [ ] | 2005 | 3. General overview, diagnosis, and management of BPD. |
Paris et al. [ ] | 2019 | 4. Probes into suicidality and its links with BPD. |
Videler et al. [ ] | 2019 | 5. Discusses BPD's manifestations across various life stages. |
Gartlehner et al. [ ] | 2021 | 6. Comprehensive analysis of pharmaceutical interventions for BPD. |
Parker et al. [ ] | 2019 | 7. Overview of pharmacological strategies for treating BPD. |
Stoffers et al. [ ] | 2012 | 8. Evaluates the effectiveness of psychological therapies for BPD. |
Helle et al. [ ] | 2019 | 9. Examines how antisocial and borderline personality disorders can coexist with alcohol use disorder. |
Reichl et al. [ ] | 2021 | 10. Focuses on self-harm behaviours within the BPD context. |
Kienast et al. [ ] | 2014 | 11. Delves into the epidemiology and treatment of BPD coexisting with addiction. |
Cremers et al. [ ] | 2021 | 12. Uses brain network measures to classify BPD during emotion regulation tasks. |
Cavelti et al. [ ] | 2021 | 13. Looks at the emergence of psychotic symptoms in BPD from a developmental perspective. |
Guendelman et al. [ ] | 2014 | 14. Investigates the neurobiological underpinnings of BPD. |
Oud et al. [ ] | 2018 | 15. Systematic review of specialized psychotherapies for adult BPD patients. |
Snoek et al. [ ] | 2020 | 16. A study comparing the costs and benefits of integrated EMDR-DBT with EMDR for PTSD patients who exhibit characteristics of BPD. |
Biskin et al. [ ] | 2012 | 17. Discusses the diagnostic criteria and methods for BPD. |
Masland et al. [ ] | 2020 | 18. Reconsiders the significance of boredom as a potential diagnostic criterion for BPD. |
Tan et al. [ ] | 2018 | 19. Uses a qualitative approach to understand patients’ perceptions of schema therapy for BPD. |
Larrivée et al. [ ] | 2013 | 20. Discusses challenges and peculiarities of diagnosing BPD in adolescents. |
Gunderson et al. [ ] | 2009 | 21. Chronicles the development and changes in the diagnosis of BPD over time. |
McDonald et al. [ ] | 2019 | 22. A thorough analysis of the genetics, epigenetics and comorbidity of BPD and bulimia nervosa. |
May et al. [ ] | 2023 | 23. Reviews interventions for borderline personality disorder and complex trauma in the perinatal period. |
Lekgabe et al. [ ] | 2021 | 24. Examines traits of BPD in adolescents suffering from anorexia nervosa. |
Symptoms - borderline personality disorder [ ] | 25. An online resource detailing the symptoms of BPD. | |
Pascual et al. [ ] | 2023 | 26. Discusses pharmacological approaches to BPD and its frequently co-occurring disorders. |
Kaur et al. [ ] | 2023 | 27. Investigates the influence of parenting in the onset and development of BPD. |
Temes et al. [ ] | 2019 | 28. Offers insights into recent advances in psychosocial interventions for BPD. |
Mayo Clinic [ ] | 2022 | 29. A resource from the Mayo Clinic detailing diagnosis and treatment modalities for BPD. |
Biskin et al. [ ] | 2015 | 30. Explores the progression and lifetime trajectory of BPD. |
Conclusions
Understanding and managing BPD remains one of the most intricate and challenging mental health issues. Due to its unpredictable moods, behaviors, and interpersonal ties, diagnosis and treatment must take a multidimensional approach. The interconnectedness of BPD with other diseases, like bipolar disorder and psychotic symptoms, emphasizes its complexity and necessitates a comprehensive approach to therapy. Although its origins are unclear, the focus on symptoms provides professionals with a clear path for diagnosis. Recent developments in psychotherapy approaches, particularly Schema therapy and dialectical behavior therapy (DBT), have given individuals suffering new hope by uncovering viable routes to recovery. Although experts frequently recommend some pharmacological therapies, ongoing controversy about their effectiveness emphasizes the need for further study in this field.
The high rate of self-harming behaviors and suicidal thoughts among BPD patients is an important cause for concern, underscoring the importance of early detection, particularly in younger groups. Early intervention can significantly change the trajectory of the condition, which is why the urgency is increased by the fact that BPD frequently manifests during adolescence. In addition, the historical context, from Stern's 1938 coining of the word “borderline” to the complexity of today's diagnosis and treatment of an illness, has drawn growing attention over the years. Significant obstacles still exist. A more complex knowledge of BPD is crucial as we go. To guarantee people afflicted by BPD receive the comprehensive support they require, ongoing research, interdisciplinary collaboration, and patient-centered care are essential.
The authors have declared that no competing interests exist.
Facial Forensics Detection Based on Deep Learning Approaches: Comprehensive Literature Review
33 Pages Posted: 20 Aug 2024
Nibras Abdullah
affiliation not provided to SSRN
Israa Mishkhal
Hassan salah.
University of Diyala
AMAN JANTAN
Fadratul hafinaz hassan.
Universiti Sains Malaysia (USM)
Facial Forensics (FF) is one of the most active research problems in computer vision and digital image forensics, with a wide variety of practical and commercial applications, including identification, access control, and interaction between people with smart devices. However, identifying a face raises serious questions about individual liberties and raises ethical issues. In recent years, important methods, algorithms, approaches, and databases have been proposed for research Facial Forensics without constraints. There are two approaches namely: the 2D approach has reached a certain level of maturity and has reported very high recognition rates; the 3D approach has been helping to reduce such ambient conditions. It has been proposed as an alternative to the problems mentioned above. The advantage of the 3D dataset is that it is invariant to the pose and lighting conditions, which improves the efficiency of the detection systems. However, the 3D dataset is a bit sensitive to changes in facial expressions. This paper presents facial forensics technologies, currently advanced methods, and future directions. It focuses specifically on the latest database of 2D and 3D facial forensics that utilized from 2018 to 2022. Furthermore, it focuses on deep learning (DL) approaches due to excellent performances. Also, potential directions for research in facial forensics are presented to provide the reader with a point of reference for topics worthy of consideration in the facial forensic field.
Keywords: Facial Forensics (FF), Artificial intelligence (AI), deep learning (DL), face dataset digital forensics.
Suggested Citation: Suggested Citation
Nibras Abdullah (Contact Author)
Affiliation not provided to ssrn ( email ).
No Address Available

University of Diyala ( email )
College of Engineering-Department of Mechanical Iraq-Diyala Baqubia, 00964 Iraq
Universiti Sains Malaysia (USM) ( email )
Minden, 11800 Malaysia
Do you have a job opening that you would like to promote on SSRN?
Paper statistics, related ejournals, artificial intelligence ejournal.
Subscribe to this fee journal for more curated articles on this topic
Digital Forensics, Instruments & Software eJournal
Forensic medicine & human identification ejournal, forensic science education ejournal.
Information
- Author Services
Initiatives
You are accessing a machine-readable page. In order to be human-readable, please install an RSS reader.
All articles published by MDPI are made immediately available worldwide under an open access license. No special permission is required to reuse all or part of the article published by MDPI, including figures and tables. For articles published under an open access Creative Common CC BY license, any part of the article may be reused without permission provided that the original article is clearly cited. For more information, please refer to https://www.mdpi.com/openaccess .
Feature papers represent the most advanced research with significant potential for high impact in the field. A Feature Paper should be a substantial original Article that involves several techniques or approaches, provides an outlook for future research directions and describes possible research applications.
Feature papers are submitted upon individual invitation or recommendation by the scientific editors and must receive positive feedback from the reviewers.
Editor’s Choice articles are based on recommendations by the scientific editors of MDPI journals from around the world. Editors select a small number of articles recently published in the journal that they believe will be particularly interesting to readers, or important in the respective research area. The aim is to provide a snapshot of some of the most exciting work published in the various research areas of the journal.
Original Submission Date Received: .
- Active Journals
- Find a Journal
- Proceedings Series
- For Authors
- For Reviewers
- For Editors
- For Librarians
- For Publishers
- For Societies
- For Conference Organizers
- Open Access Policy
- Institutional Open Access Program
- Special Issues Guidelines
- Editorial Process
- Research and Publication Ethics
- Article Processing Charges
- Testimonials
- Preprints.org
- SciProfiles
- Encyclopedia
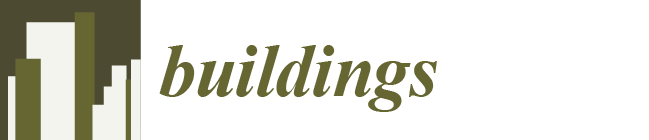
Article Menu
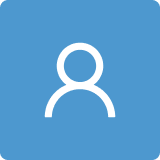
- Subscribe SciFeed
- Google Scholar
- on Google Scholar
- Table of Contents
Find support for a specific problem in the support section of our website.
Please let us know what you think of our products and services.
Visit our dedicated information section to learn more about MDPI.
JSmol Viewer
A systematic review of the evolution of the concept of resilience in the construction industry.
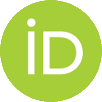
1. Introduction
2. methodology, 2.1. search strategy, 2.2. selection, 2.3. extraction, 2.4. assessment, 3.1. the subject of resilience, 3.1.1. specific class, 3.1.2. general class, 3.1.3. temporal distribution of resilience subjects, 3.2. the impact factors, 3.2.1. natural and environmental factors, 3.2.2. human and external factors, 3.2.3. temporal distribution of impact factors, 3.3. capabilities of the subject, 3.3.1. management and operations, 3.3.2. performance and development, 3.3.3. temporal distribution of subject capabilities, 4. discussion, 4.1. the evolution and ambiguity of the early concept of resilience, 4.2. complexity of impact types and functional failures, 4.3. redefining the concept of resilience in a vuca environment, 4.4. future directions and suggestions, 5. conclusions, data availability statement, acknowledgments, conflicts of interest.
- Zhang, B.; Mei, Y.; Xiong, Y.; Liu, Y. Can Digital Transformation Promote Service Innovation Performance of Construction Enterprises? The Mediating Role of Dual Innovation. Sustainability 2024 , 16 , 1176. [ Google Scholar ] [ CrossRef ]
- Murtagh, N.; Scott, L.; Fan, J. Sustainable and Resilient Construction: Current Status and Future Challenges. J. Clean. Prod. 2020 , 268 , 122264. [ Google Scholar ] [ CrossRef ]
- Cambraia, F.B.; Saurin, T.A.; Bulhões, I.R.; Formoso, C.T. A Knowledge Framework of Participation Supportive of Resilient and Safe Construction Projects: A Systematic Review. Saf. Sci. 2024 , 175 , 106494. [ Google Scholar ] [ CrossRef ]
- Ranasinghe, U.; Jefferies, M.; Davis, P.; Pillay, M. Resilience Engineering Indicators and Safety Management: A Systematic Review. Saf. Health Work 2020 , 11 , 127–135. [ Google Scholar ] [ CrossRef ] [ PubMed ]
- Robbins, J.D.; Wang, J.; Esmaeili, B. Review of Safety Resilience in Construction: Current Research Status, Challenges, and Future Needs. In Proceedings of the Construction Research Congress 2022: Health and Safety, Workforce, and Education, Arlington, VA, USA, 9–12 March 2022; Jazizadeh, F., Shealy, T., Garvin, M.J., Eds.; Amer Soc Civil Engineers: New York, NY, USA, 2022; pp. 393–402. [ Google Scholar ]
- Bhamra, R.; Dani, S.; Burnard, K. Resilience: The Concept, a Literature Review and Future Directions. Int. J. Prod. Res. 2011 , 49 , 5375–5393. [ Google Scholar ] [ CrossRef ]
- Bocchini, P.; Frangopol, D.M.; Ummenhofer, T.; Zinke, T. Resilience and Sustainability of Civil Infrastructure: Toward a Unified Approach. J. Infrastruct. Syst. 2014 , 20 , 04014004. [ Google Scholar ] [ CrossRef ]
- Holling, C.S. Resilience and Stability of Ecological Systems. Annu. Rev. Ecol. Evol. Syst. 1973 , 4 , 1–23. [ Google Scholar ] [ CrossRef ]
- Annarelli, A.; Nonino, F. Strategic and Operational Management of Organizational Resilience: Current State of Research and Future Directions. Omega 2016 , 62 , 1–18. [ Google Scholar ] [ CrossRef ]
- Pires Ribeiro, J.; Barbosa-Povoa, A. Supply Chain Resilience: Definitions and Quantitative Modelling Approaches—A Literature Review. Comput. Ind. Eng. 2018 , 115 , 109–122. [ Google Scholar ] [ CrossRef ]
- Conz, E.; Magnani, G. A Dynamic Perspective on the Resilience of Firms: A Systematic Literature Review and a Framework for Future Research. Eur. Manag. J. 2020 , 38 , 400–412. [ Google Scholar ] [ CrossRef ]
- Zhou, Y.; Wang, J.; Yang, H. Resilience of Transportation Systems: Concepts and Comprehensive Review. IEEE Trans. Intell. Transp. Syst. 2019 , 20 , 4262–4276. [ Google Scholar ] [ CrossRef ]
- Liu, W.; Song, Z. Review of Studies on the Resilience of Urban Critical Infrastructure Networks. Reliab. Eng. Syst. Saf. 2020 , 193 , 106617. [ Google Scholar ] [ CrossRef ]
- Foster, K.; Roche, M.; Delgado, C.; Cuzzillo, C.; Giandinoto, J.-A.; Furness, T. Resilience and Mental Health Nursing: An Integrative Review of International Literature. Int. J. Ment. Health Nurs. 2019 , 28 , 71–85. [ Google Scholar ] [ CrossRef ]
- Quitana, G.; Molinos-Senante, M.; Chamorro, A. Resilience of Critical Infrastructure to Natural Hazards: A Review Focused on Drinking Water Systems. Int. J. Disaster Risk Reduct. 2020 , 48 , 101575. [ Google Scholar ] [ CrossRef ]
- Page, M.J.; McKenzie, J.E.; Bossuyt, P.M.; Boutron, I.; Hoffmann, T.C.; Mulrow, C.D.; Shamseer, L.; Tetzlaff, J.M.; Akl, E.A.; Brennan, S.E.; et al. The PRISMA 2020 Statement: An Updated Guideline for Reporting Systematic Reviews. BMJ 2021 , 372 , n71. [ Google Scholar ] [ CrossRef ] [ PubMed ]
- Afzal, M.; Li, R.Y.M.; Shoaib, M.; Ayyub, M.F.; Tagliabue, L.C.; Bilal, M.; Ghafoor, H.; Manta, O. Delving into the Digital Twin Developments and Applications in the Construction Industry: A PRISMA Approach. Sustainability 2023 , 15 , 16436. [ Google Scholar ] [ CrossRef ]
- Li, R.Y.M.; Li, Y.L.; Crabbe, M.J.C.; Manta, O.; Shoaib, M. The Impact of Sustainability Awareness and Moral Values on Environmental Laws. Sustainability 2021 , 13 , 5882. [ Google Scholar ] [ CrossRef ]
- Liao, L.; Yang, C.; Quan, L. Construction Supply Chain Management: A Systematic Literature Review and Future Development. J. Clean. Prod. 2023 , 382 , 135230. [ Google Scholar ] [ CrossRef ]
- Ning, Y.; Gao, S. A Resilience Framework to Explorative Quality Management in Innovative Building Projects. J. Eng. Technol. Manag. 2021 , 62 , 101654. [ Google Scholar ] [ CrossRef ]
- Wedawatta, G.; Ingirige, B.; Amaratunga, D. Building up resilienc e of construction sector smes and their supply chains to extreme weather events. Int. J. Strateg. Prop. Manag. 2010 , 14 , 362–375. [ Google Scholar ] [ CrossRef ]
- Ranasinghe, U.; Jefferies, M.; Davis, P.; Pillay, M. Enabling a Resilient Work Environment: An Analysis of Causal Relationships between Resilience Engineering Factors in Construction Refurbishment Projects. J. Constr. Eng. Manage. 2023 , 149 , 04023078. [ Google Scholar ] [ CrossRef ]
- Zhang, W.; Dong, H.; Wen, J.; Han, Q. A Resilience-Based Decision Framework for Post-Earthquake Restoration of Bridge Networks under Uncertainty. Struct. Infrastruct. Eng. 2023 , 1–16. [ Google Scholar ] [ CrossRef ]
- Li, Y.; Dong, Y.; Frangopol, D.M.; Gautam, D. Long-Term Resilience and Loss Assessment of Highway Bridges under Multiple Natural Hazards. Struct. Infrastruct. Eng. 2020 , 16 , 626–641. [ Google Scholar ] [ CrossRef ]
- Dong, Y.; Frangopol, D.M. Probabilistic Time-Dependent Multihazard Life-Cycle Assessment and Resilience of Bridges Considering Climate Change. J. Perform. Constr. Facil. 2016 , 30 , 04016034. [ Google Scholar ] [ CrossRef ]
- Akiyama, M.; Frangopol, D.M.; Ishibashi, H. Toward Life-Cycle Reliability-, Risk- and Resilience-Based Design and Assessment of Bridges and Bridge Networks under Independent and Interacting Hazards: Emphasis on Earthquake, Tsunami and Corrosion. Struct. Infrastruct. Eng. 2020 , 16 , 26–50. [ Google Scholar ] [ CrossRef ]
- Shafieezadeh, A.; Ivey Burden, L. Scenario-Based Resilience Assessment Framework for Critical Infrastructure Systems: Case Study for Seismic Resilience of Seaports. Reliab. Eng. Syst. Saf. 2014 , 132 , 207–219. [ Google Scholar ] [ CrossRef ]
- Verschuur, J.; Pant, R.; Koks, E.; Hall, J. A Systemic Risk Framework to Improve the Resilience of Port and Supply-Chain Networks to Natural Hazards. Marit. Econ. Logist. 2022 , 24 , 489–506. [ Google Scholar ] [ CrossRef ]
- John, A.; Yang, Z.; Riahi, R.; Wang, J. A Risk Assessment Approach to Improve the Resilience of a Seaport System Using Bayesian Networks. Ocean Eng. 2016 , 111 , 136–147. [ Google Scholar ] [ CrossRef ]
- Azadeh, A.; Salehi, V.; Arvan, M.; Dolatkhah, M. Assessment of Resilience Engineering Factors in High-Risk Environments by Fuzzy Cognitive Maps: A Petrochemical Plant. Saf. Sci. 2014 , 68 , 99–107. [ Google Scholar ] [ CrossRef ]
- Xiong, C.; Huang, J.; Lu, X. Framework for City-scale Building Seismic Resilience Simulation and Repair Scheduling with Labor Constraints Driven by Time–History Analysis. Comput. Aided Civ. Eng 2020 , 35 , 322–341. [ Google Scholar ] [ CrossRef ]
- Katal, A.; Mortezazadeh, M.; Wang, L. (Leon) Modeling Building Resilience against Extreme Weather by Integrated CityFFD and CityBEM Simulations. Appl. Energy 2019 , 250 , 1402–1417. [ Google Scholar ] [ CrossRef ]
- Bruneau, M.; Reinhorn, A. Exploring the Concept of Seismic Resilience for Acute Care Facilities. Earthq. Spectra 2007 , 23 , 41–62. [ Google Scholar ] [ CrossRef ]
- Osman, M.M.; Sevinc, H. Adaptation of Climate-Responsive Building Design Strategies and Resilience to Climate Change in the Hot/Arid Region of Khartoum, Sudan. Sustain. Cities Soc. 2019 , 47 , 101429. [ Google Scholar ] [ CrossRef ]
- Feng, X.; Xiu, C.; Bai, L.; Zhong, Y.; Wei, Y. Comprehensive Evaluation of Urban Resilience Based on the Perspective of Landscape Pattern: A Case Study of Shenyang City. Cities 2020 , 104 , 102722. [ Google Scholar ] [ CrossRef ]
- Angulo, A.M.; Mur, J.; Trívez, F.J. Measuring Resilience to Economic Shocks: An Application to Spain. Ann. Reg. Sci. 2018 , 60 , 349–373. [ Google Scholar ] [ CrossRef ]
- Molina Hutt, C.; Almufti, I.; Willford, M.; Deierlein, G. Seismic Loss and Downtime Assessment of Existing Tall Steel-Framed Buildings and Strategies for Increased Resilience. J. Struct. Eng. 2016 , 142 , C4015005. [ Google Scholar ] [ CrossRef ]
- Azmi, N.A.; Sweis, G.; Sweis, R.; Sammour, F. Exploring Implementation of Blockchain for the Supply Chain Resilience and Sustainability of the Construction Industry in Saudi Arabia. Sustainability 2022 , 14 , 6427. [ Google Scholar ] [ CrossRef ]
- Yao, H.; Wang, K. Concentrated or Dispersed: The Effects of Subcontracting Organizational Arrangements on Construction Project Resilience. J. Manag. Eng. 2024 , 40 , 04024017. [ Google Scholar ] [ CrossRef ]
- Gerami Seresht, N. Enhancing Resilience in Construction against Infectious Diseases Using Stochastic Multi-Agent Approach. Autom. Constr. 2022 , 140 , 104315. [ Google Scholar ] [ CrossRef ]
- Trinh, M.T.; Feng, Y.; Mohamed, S. Framework for Measuring Resilient Safety Culture in Vietnam’s Construction Environment. J. Constr. Eng. Manag. 2019 , 145 , 04018127. [ Google Scholar ] [ CrossRef ]
- Wang, D.; Wu, Y.; Zhang, K. Interplay of Resources and Institutions in Improving Organizational Resilience of Construction Projects: A Dynamic Perspective. EMJ-Eng. Manag. J. 2023 , 35 , 346–357. [ Google Scholar ] [ CrossRef ]
- He, Z.; Wang, G.; Chen, H.; Zou, Z.; Yan, H.; Liu, L. Measuring the Construction Project Resilience from the Perspective of Employee Behaviors. Buildings 2022 , 12 , 56. [ Google Scholar ] [ CrossRef ]
- Peñaloza, G.A.; Saurin, T.A.; Formoso, C.T. Monitoring Complexity and Resilience in Construction Projects: The Contribution of Safety Performance Measurement Systems. Appl. Ergon. 2020 , 82 , 102978. [ Google Scholar ] [ CrossRef ]
- Hilu, K.A.; Hiyassat, M.A. Qualitative Assessment of Resilience in Construction Projects. Constr. Innov. 2023 , 24 , 1297–1319. [ Google Scholar ] [ CrossRef ]
- Milat, M.; Knezić, S.; Sedlar, J. Resilient Scheduling as a Response to Uncertainty in Construction Projects. Appl. Sci. 2021 , 11 , 6493. [ Google Scholar ] [ CrossRef ]
- Hamerski, D.C.; Saurin, T.A.; Formoso, C.T.; Isatto, E.L. The Contributions of the Last Planner System to Resilient Performance in Construction Projects. Constr. Manag. Econ. 2024 , 42 , 328–345. [ Google Scholar ] [ CrossRef ]
- Wang, D.; Wang, P.; Liu, Y. The Emergence Process of Construction Project Resilience: A Social Network Analysis Approach. Buildings 2022 , 12 , 822. [ Google Scholar ] [ CrossRef ]
- Trinh, M.T.; Feng, Y. A Maturity Model for Resilient Safety Culture Development in Construction Companies. Buildings 2022 , 12 , 733. [ Google Scholar ] [ CrossRef ]
- Feng, Y.; Trinh, M.T. Developing Resilient Safety Culture for Construction Projects. J. Constr. Eng. Manag. 2019 , 145 , 04019069. [ Google Scholar ] [ CrossRef ]
- Saldanha, M.C.W.; Araújo, L.L.F.; Arcuri, R.; Vidal, M.C.R.; De Carvalho, P.V.R.; De Carvalho, R.J.M. Identifying Routes and Organizational Practices for Resilient Performance: A Study in the Construction Industry. Cogn. Technol. Work 2022 , 24 , 521–535. [ Google Scholar ] [ CrossRef ]
- Trinh, M.T.; Feng, Y. Impact of Project Complexity on Construction Safety Performance: Moderating Role of Resilient Safety Culture. J. Constr. Eng. Manag. 2020 , 146 , 04019103. [ Google Scholar ] [ CrossRef ]
- Chih, Y.-Y.; Hsiao, C.Y.-L.; Zolghadr, A.; Naderpajouh, N. Resilience of Organizations in the Construction Industry in the Face of COVID-19 Disturbances: Dynamic Capabilities Perspective. J. Manag. Eng. 2022 , 38 , 04022002. [ Google Scholar ] [ CrossRef ]
- Malik, A.; Khan, K.I.A.; Qayyum, S.; Ullah, F.; Maqsoom, A. Resilient Capabilities to Tackle Supply Chain Risks: Managing Integration Complexities in Construction Projects. Buildings 2022 , 12 , 1322. [ Google Scholar ] [ CrossRef ]
- Nassereddine, H.; Seo, K.W.; Rybkowski, Z.K.; Schranz, C.; Urban, H. Propositions for a Resilient, Post-COVID-19 Future for the AEC Industry. Front. Built Environ. 2021 , 7 , 687021. [ Google Scholar ] [ CrossRef ]
- Chen, Y.; McCabe, B.; Hyatt, D. A Resilience Safety Climate Model Predicting Construction Safety Performance. Saf. Sci. 2018 , 109 , 434–445. [ Google Scholar ] [ CrossRef ]
- Gao, L.; Luo, X.; Wang, Y.; Zhang, N.; Deng, X. Retention in Challenging International Construction Assignments: Role of Expatriate Resilience. J. Constr. Eng. Manag. 2024 , 150 , 04023158. [ Google Scholar ] [ CrossRef ]
- Liu, K.; Liu, Y.; Kou, Y. Study on Construction Safety Management in Megaprojects from the Perspective of Resilient Governance. Saf. Sci. 2024 , 173 , 106442. [ Google Scholar ] [ CrossRef ]
- Xu, S.; Lin, B.; Zou, P.X.W. Examining Construction Group’s Safety Attitude Resilience under Major Disruptions: An Agent-Based Modelling Approach. Saf. Sci. 2023 , 161 , 106071. [ Google Scholar ] [ CrossRef ]
- Cai, B.; Xie, M.; Liu, Y.; Liu, Y.; Feng, Q. Availability-Based Engineering Resilience Metric and Its Corresponding Evaluation Methodology. Reliab. Eng. Syst. Saf. 2018 , 172 , 216–224. [ Google Scholar ] [ CrossRef ]
- Kilanitis, I.; Sextos, A. Integrated Seismic Risk and Resilience Assessment of Roadway Networks in Earthquake Prone Areas. Bull. Earthq. Eng. 2019 , 17 , 181–210. [ Google Scholar ] [ CrossRef ]
- Wang, H.; Zhou, J.; Dun, Z.; Cheng, J.; Li, H.; Dun, Z. Resilience Evaluation of High-Speed Railway Subgrade Construction Systems in Goaf Sites. Sustainability 2022 , 14 , 7806. [ Google Scholar ] [ CrossRef ]
- Zhang, W.; Wang, N.; Nicholson, C. Resilience-Based Post-Disaster Recovery Strategies for Road-Bridge Networks. Struct. Infrastruct. Eng. 2017 , 13 , 1404–1413. [ Google Scholar ] [ CrossRef ]
- Zhang, W.; Wang, N. Resilience-Based Risk Mitigation for Road Networks. Struct. Saf. 2016 , 62 , 57–65. [ Google Scholar ] [ CrossRef ]
- Dong, Y.; Frangopol, D.M. Risk and Resilience Assessment of Bridges under Mainshock and Aftershocks Incorporating Uncertainties. Eng. Struct. 2015 , 83 , 198–208. [ Google Scholar ] [ CrossRef ]
- Alipour, A.; Shafei, B. Seismic Resilience of Transportation Networks with Deteriorating Components. J. Struct. Eng. 2016 , 142 , C4015015. [ Google Scholar ] [ CrossRef ]
- Chen, Z.; Hammad, A.W.A.; Alyami, M. Building Construction Supply Chain Resilience under Supply and Demand Uncertainties. Autom. Constr. 2024 , 158 , 105190. [ Google Scholar ] [ CrossRef ]
- Xie, D.; Xin, J.; Wang, H.; Xiao, L. Identifying Critical Factors Affecting the Resilience of Additive Manufacturing Architecture Supply Chain. Buildings 2023 , 13 , 997. [ Google Scholar ] [ CrossRef ]
- Cherian, T.M.; Mathivathanan, D.; Arun SJ, C.J.; Ramasubramaniam, M.; Alathur, S. Influence of Supply Chain Resilience, Information Technology Capabilities and Agility on Cost and Delivery Performance in Construction Supply Chains: An Indian Perspective. Int. J. Logist. Manag. 2022 , 34 , 1050–1076. [ Google Scholar ] [ CrossRef ]
- Ekanayake, E.M.A.C.; Shen, G.Q.P.; Kumaraswamy, M.M.; Owusu, E.K.; Saka, A.B. Modeling Supply Chain Resilience in Industrialized Construction: A Hong Kong Case. J. Constr. Eng. Manag. 2021 , 147 , 05021009. [ Google Scholar ] [ CrossRef ]
- Cheng, S.; Zhou, X.; Zhang, Y.; Duan, M.; Gao, J. Study on Resilience Factors and Enhancement Strategies in Prefabricated Building Supply Chains. Buildings 2024 , 14 , 195. [ Google Scholar ] [ CrossRef ]
- Wehbe, F.; Hattab, M.A.; Hamzeh, F. Exploring Associations between Resilience and Construction Safety Performance in Safety Networks. Saf. Sci. 2016 , 82 , 338–351. [ Google Scholar ] [ CrossRef ]
- Guo, Q.; Amin, S.; Hao, Q.; Haas, O. Resilience Assessment of Safety System at Subway Construction Sites Applying Analytic Network Process and Extension Cloud Models. Reliab. Eng. Syst. Saf. 2020 , 201 , 106956. [ Google Scholar ] [ CrossRef ]
- Argyroudis, S.A.; Mitoulis, S.A.; Chatzi, E.; Baker, J.W.; Brilakis, I.; Gkoumas, K.; Vousdoukas, M.; Hynes, W.; Carluccio, S.; Keou, O.; et al. Digital Technologies Can Enhance Climate Resilience of Critical Infrastructure. Clim. Risk Manag. 2022 , 35 , 100387. [ Google Scholar ] [ CrossRef ]
- Iannacone, L.; Sharma, N.; Tabandeh, A.; Gardoni, P. Modeling Time-Varying Reliability and Resilience of Deteriorating Infrastructure. Reliab. Eng. Syst. Saf. 2022 , 217 , 108074. [ Google Scholar ] [ CrossRef ]
- Lounis, Z.; McAllister, T.P. Risk-Based Decision Making for Sustainable and Resilient Infrastructure Systems. J. Struct. Eng. 2016 , 142 , F4016005. [ Google Scholar ] [ CrossRef ]
- Dao, J.; Ng, S.T.; Yang, Y.; Zhou, S.; Xu, F.J.; Skitmore, M. Semantic Framework for Interdependent Infrastructure Resilience Decision Support. Autom. Constr. 2021 , 130 , 103852. [ Google Scholar ] [ CrossRef ]
- Burton, H.V.; Deierlein, G.; Lallemant, D.; Lin, T. Framework for Incorporating Probabilistic Building Performance in the Assessment of Community Seismic Resilience. J. Struct. Eng. 2016 , 142 , C4015007. [ Google Scholar ] [ CrossRef ]
- Franchin, P.; Cavalieri, F. Probabilistic Assessment of Civil Infrastructure Resilience to Earthquakes. Comput. Aided Civ. Eng 2015 , 30 , 583–600. [ Google Scholar ] [ CrossRef ]
- Wei, W.; Mojtahedi, M.; Yazdani, M.; Kabirifar, K. The Alignment of Australia’s National Construction Code and the Sendai Framework for Disaster Risk Reduction in Achieving Resilient Buildings and Communities. Buildings 2021 , 11 , 429. [ Google Scholar ] [ CrossRef ]
- Yang, Y.; Ng, S.T.; Xu, F.J.; Skitmore, M. Towards Sustainable and Resilient High Density Cities through Better Integration of Infrastructure Networks. Sustain. Cities Soc. 2018 , 42 , 407–422. [ Google Scholar ] [ CrossRef ]
- Jiang, S.; Ling, F.Y.Y.; Ma, G. Fostering Resilience in Project Teams: Adaptive Structuration Perspective. J. Manag. Eng. 2024 , 40 , 04023047. [ Google Scholar ] [ CrossRef ]
- Lu, C.; Yu, D.; Luo, Q.; Xu, C. A Study of the Effects of Job Stress on the Psychosocial Safety Behavior of Construction Workers: The Mediating Role of Psychological Resilience. Buildings 2023 , 13 , 1930. [ Google Scholar ] [ CrossRef ]
- Aasen, A.F.; Klakegg, O.J. Human Resilience and Cultural Change in the Construction Industry: Communication and Relationships in a Time of Enforced Adaptation. Front. Built Environ. 2023 , 9 , 1287483. [ Google Scholar ] [ CrossRef ]
- Chen, Y.; McCabe, B.; Hyatt, D. Impact of Individual Resilience and Safety Climate on Safety Performance and Psychological Stress of Construction Workers: A Case Study of the Ontario Construction Industry. J. Saf. Res. 2017 , 61 , 167–176. [ Google Scholar ] [ CrossRef ] [ PubMed ]
- Nwaogu, J.M.; Chan, A.P.C. The Impact of Coping Strategies and Individual Resilience on Anxiety and Depression among Construction Supervisors. Buildings 2022 , 12 , 2148. [ Google Scholar ] [ CrossRef ]
- Wang, T.-K.; Zhang, Q.; Chong, H.-Y.; Wang, X. Integrated Supplier Selection Framework in a Resilient Construction Supply Chain: An Approach via Analytic Hierarchy Process (AHP) and Grey Relational Analysis (GRA). Sustainability 2017 , 9 , 289. [ Google Scholar ] [ CrossRef ]
- Shishodia, A.; Verma, P.; Dixit, V. Supplier Evaluation for Resilient Project Driven Supply Chain. Comput. Ind. Eng. 2019 , 129 , 465–478. [ Google Scholar ] [ CrossRef ]
- Thurber, C. An Introduction to Seismology, Earthquakes, and Earth Structure. EoS Trans. 2003 , 84 , 209–210. [ Google Scholar ] [ CrossRef ]
- Morse, S.S.; Mazet, J.A.; Woolhouse, M.; Parrish, C.R.; Carroll, D.; Karesh, W.B.; Zambrana-Torrelio, C.; Lipkin, W.I.; Daszak, P. Prediction and Prevention of the next Pandemic Zoonosis. Lancet 2012 , 380 , 1956–1965. [ Google Scholar ] [ CrossRef ]
- Cutter, S.L.; Boruff, B.J.; Shirley, W.L. Social Vulnerability to Environmental Hazards*. Soc. Sci. Q. 2003 , 84 , 242–261. [ Google Scholar ] [ CrossRef ]
- Sauter, S.L.; Murphy, L.R.; Hurrell, J.J. Prevention of Work-Related Psychological Disorders. A National Strategy Proposed by the National Institute for Occupational Safety and Health (NIOSH). Am. Psychol. 1990 , 45 , 1146–1158. [ Google Scholar ] [ CrossRef ] [ PubMed ]
- Daboun, O.; Md Yusof, A.; Khoso, A.R. Relationship Management in Construction Projects: Systematic Literature Review. Eng. Manag. J. 2023 , 35 , 120–143. [ Google Scholar ] [ CrossRef ]
- Karuppiah, K.; Sankaranarayanan, B.; Ali, S.M. A Systematic Review of Sustainable Business Models: Opportunities, Challenges, and Future Research Directions. Decis. Anal. J. 2023 , 8 , 100272. [ Google Scholar ] [ CrossRef ]
- Grzybowska, K.; Tubis, A.A. Supply Chain Resilience in Reality VUCA-An International Delphi Study. Sustainability 2022 , 14 , 10711. [ Google Scholar ] [ CrossRef ]
- Li, K.; Ma, J.; Gao, J.; Xu, C.; Li, W.; Mao, Y.; Jiang, S. Resilience Assessment of Urban Distribution Network Under Heavy Rain: A Knowledge- Informed Data-Driven Approach. IEEE Access 2023 , 11 , 63741–63750. [ Google Scholar ] [ CrossRef ]
- Yang, Y.; Ng, S.T.; Zhou, S.; Xu, F.J.; Li, D.; Li, H. A Federated Pre-Event Community Resilience Approach for Assessing Physical and Social Sub-Systems: An Extreme Rainfall Case in Hong Kong. Sustain. Cities Soc. 2020 , 52 , 101859. [ Google Scholar ] [ CrossRef ]
- Bozza, A.; Asprone, D.; Fabbrocino, F. Urban Resilience: A Civil Engineering Perspective. Sustainability 2017 , 9 , 103. [ Google Scholar ] [ CrossRef ]
- Hao, H.; Bi, K.; Chen, W.; Pham, T.M.; Li, J. Towards next Generation Design of Sustainable, Durable, Multi-Hazard Resistant, Resilient, and Smart Civil Engineering Structures. Eng. Struct. 2023 , 277 , 115477. [ Google Scholar ] [ CrossRef ]
- Herrera, H. Resilience for Whom? The Problem Structuring Process of the Resilience Analysis. Sustainability 2017 , 9 , 1196. [ Google Scholar ] [ CrossRef ]
- Juncos, A.E. Resilience in Peacebuilding: Contesting Uncertainty, Ambiguity, and Complexity. Contemp. Secur. Policy 2018 , 39 , 559–574. [ Google Scholar ] [ CrossRef ]
- Li, Y.; Lei, S.; Sun, W.; Hu, C.; Hou, Y. A Distributionally Robust Resilience Enhancement Strategy for Distribution Networks Considering Decision-Dependent Contingencies. IEEE Trans. Smart Grid 2024 , 15 , 1450–1465. [ Google Scholar ] [ CrossRef ]
- Dolla, T.; Jain, K.; Delhi, V.S.K. Strategies for Digital Transformation in Construction Projects: Stakeholders’ Perceptions and Actor Dynamics for Industry 4.0. J. Inf. Technol. Constr. 2023 , 28 , 151–175. [ Google Scholar ] [ CrossRef ]
- Browder, R.E.; Dwyer, S.M.; Koch, H. Upgrading Adaptation: How Digital Transformation Promotes Organizational Resilience. Strateg. Entrep. J. 2024 , 18 , 128–164. [ Google Scholar ] [ CrossRef ]
- Yang, Y.; Ng, S.T.; Li, N.; Xu, X.; Xu, P.; Xu, F.J. Adapting HLA-Based Co-Simulation for Interdependent Infrastructure Resilience Management. Autom. Constr. 2023 , 150 , 104860. [ Google Scholar ] [ CrossRef ]
- Rathnasiri, P.; Adeniyi, O.; Thurairajah, N. Data-Driven Approaches to Built Environment Flood Resilience: A Scientometric and Critical Review. Adv. Eng. Inform. 2023 , 57 , 102085. [ Google Scholar ] [ CrossRef ]
- Heidari, A.; Peyvastehgar, Y.; Amanzadegan, M. A Systematic Review of the BIM in Construction: From Smart Building Management to Interoperability of BIM & AI. Archit. Sci. Rev. 2024 , 67 , 237–254. [ Google Scholar ] [ CrossRef ]
Click here to enlarge figure
Database | Search Strings | Number |
---|---|---|
Scopus | (TITLE-ABS-KEY(“construction industry” OR “civil engineering” OR “construction engineering” OR “architecture engineering” OR “construction sector” OR “building industry” OR “construction project”) AND TITLE-ABS-KEY(“resilience” OR “adaptive capacity” OR “robustness” OR “recoverability” OR “durability”) AND TITLE (“resilience” OR “resilient”)) | 451 |
Web of Science | (TS = (“construction industry” OR “civil engineering” OR “construction engineering” OR “architecture engineering” OR “construction sector” OR “building industry” OR “construction project”) AND TS = (“resilience” OR “adaptive capacity” OR “robustness” OR “recoverability” OR “durability”) AND TI = (“resilience” OR “resilient”) ) | 763 |
Main Categories | Subcategories | Number of Samples | Total |
---|---|---|---|
Specific Class | Specific Construction Projects | 3 | |
Concrete Facilities and Systems | 4 | 18 | |
Definite Geographic Locations | 4 | ||
Specific Infrastructure Systems | 7 | ||
General Class | Industries and Fields | 18 | |
Concepts and Methods | 4 | 52 | |
Extensive Networks and Systems | 19 | ||
Social and Community Impact | 11 |
Main Categories | Subcategories | Number of Samples | Total |
---|---|---|---|
Natural and environmental factors | Geological events | 16 | |
Climate and weather events | 8 | 27 | |
Biological events | 3 | ||
Human and external factors | Economic and supply chain risks | 3 | |
Job and workplace-related risks | 6 | 49 | |
Security and safety risks | 10 | ||
System and infrastructure risks | 11 | ||
Uncertainties | 19 |
Main Categories | Subcategories | Number of Samples | Total |
---|---|---|---|
Management and Operations | Safety and Risk Management | 32 | 60 |
Operational Management | 21 | ||
Human and Community Management | 5 | ||
Resource and Inventory Management | 2 | ||
Performance and Development | Structural and System Performance | 7 | |
Network Performance | 2 | 17 | |
Sustainability and Environmental Management | 6 | ||
Quality and Improvement Management | 2 |
The statements, opinions and data contained in all publications are solely those of the individual author(s) and contributor(s) and not of MDPI and/or the editor(s). MDPI and/or the editor(s) disclaim responsibility for any injury to people or property resulting from any ideas, methods, instructions or products referred to in the content. |
Share and Cite
Li, J.; Yu, H.; Deng, X. A Systematic Review of the Evolution of the Concept of Resilience in the Construction Industry. Buildings 2024 , 14 , 2643. https://doi.org/10.3390/buildings14092643
Li J, Yu H, Deng X. A Systematic Review of the Evolution of the Concept of Resilience in the Construction Industry. Buildings . 2024; 14(9):2643. https://doi.org/10.3390/buildings14092643
Li, Jinjing, Haizhe Yu, and Xiaopeng Deng. 2024. "A Systematic Review of the Evolution of the Concept of Resilience in the Construction Industry" Buildings 14, no. 9: 2643. https://doi.org/10.3390/buildings14092643
Article Metrics
Article access statistics, further information, mdpi initiatives, follow mdpi.
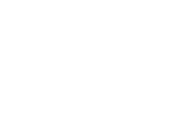
Subscribe to receive issue release notifications and newsletters from MDPI journals
- Alzheimer's disease & dementia
- Arthritis & Rheumatism
- Attention deficit disorders
- Autism spectrum disorders
- Biomedical technology
- Diseases, Conditions, Syndromes
- Endocrinology & Metabolism
- Gastroenterology
- Gerontology & Geriatrics
- Health informatics
- Inflammatory disorders
- Medical economics
- Medical research
- Medications
- Neuroscience
- Obstetrics & gynaecology
- Oncology & Cancer
- Ophthalmology
- Overweight & Obesity
- Parkinson's & Movement disorders
- Psychology & Psychiatry
- Radiology & Imaging
- Sleep disorders
- Sports medicine & Kinesiology
- Vaccination
- Breast cancer
- Cardiovascular disease
- Chronic obstructive pulmonary disease
- Colon cancer
- Coronary artery disease
- Heart attack
- Heart disease
- High blood pressure
- Kidney disease
- Lung cancer
- Multiple sclerosis
- Myocardial infarction
- Ovarian cancer
- Post traumatic stress disorder
- Rheumatoid arthritis
- Schizophrenia
- Skin cancer
- Type 2 diabetes
- Full List »
share this!
August 26, 2024
This article has been reviewed according to Science X's editorial process and policies . Editors have highlighted the following attributes while ensuring the content's credibility:
fact-checked
trusted source
Comprehensive review identifies three key concepts for recovery from substance use disorders
by Research Society on Alcoholism
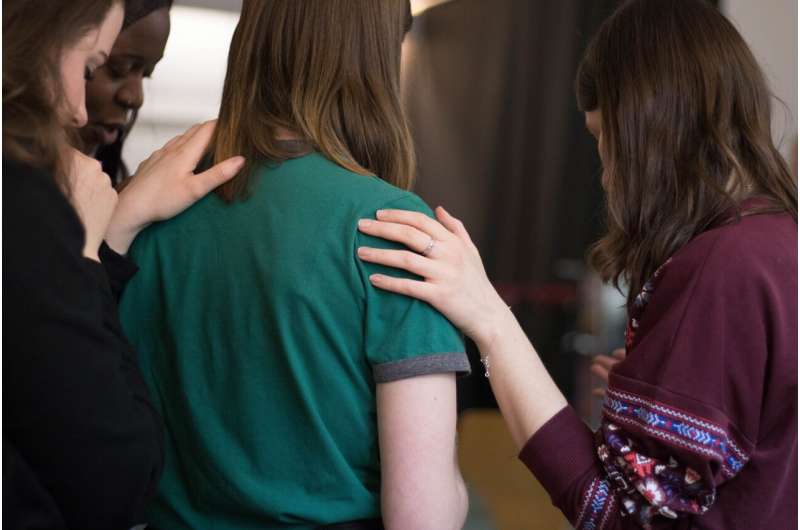
Certain concepts have a demonstrated basis for aiding recovery from dangerous alcohol or substance use, according to an analysis of scientific literature since 1990. Self-efficacy (a belief in one's ability to achieve a goal), social support, and managing cravings are among the treatment elements best supported by evidence.
Effective treatment for alcohol use disorder (AUD) and other substance use disorders (SUDs) depends on understanding how human behaviors change and incorporating that knowledge into clinical practice . An ongoing research effort continues to investigate varying treatment approaches and how they relate to recovery outcomes, but those findings have not been well synthesized into a useful format.
For the new review published in Alcohol: Clinical & Experimental Research , researchers from around the U.S. reviewed published studies, identified the treatment elements best supported by data, and evaluated their potential as key factors in behavior change. The researchers drew on existing study design criteria for validating conclusions about treatment elements.
The researchers explored reviews of studies published between 2008 and 2023 involving AUD and SUD treatments and the effects on substance use and related outcomes in adults. Three constructs involved in treatment were the most well-supported by data from 11 studies: self-efficacy, social support, and craving (coping skills, also well-supported, did not suit the current review process).
They then reviewed 48 studies published between 1990 and 2023 that focused on one or more of these three concepts in adults' recovery, and that met rigorous methodology standards. The 48 studies used varied research designs, participant samples, and contexts.
The analyzed studies provided support for self-efficacy, social support, and craving as factors that likely influence people's behaviors in treatment or recovery. The researchers called for these three constructs to be incorporated into AUD and SUD treatment and clinical training.
Such an approach could improve recovery interventions, inform new treatments and clinical training , help clinicians align patients with approaches likely to work for them, and hone community-based recovery programs.
The researchers called for additional research on how these three concepts drive behavior change and for mining existing science to identify other evidence-based approaches. They recommended several directions for future research. These included expanding the examined outcomes to other manifestations of mental and physical health and experimenting with key elements of treatment to generate direct evidence of associations between those constructs and outcomes.
Investigating the roles of context (such as policies, incentives, social norms , and settings) and combinations of influences could improve outcomes across varied real-world situations. Specifying how behavioral change occurs—such as the relevant neurological and biological pathways—is a critical gap that needs to be addressed.
Explore further
Feedback to editors

Multipurpose vaccine shows new promise in the presence of pre-existing immunity
10 hours ago

Researchers develop affordable, rapid blood test for brain cancer

Discovery gives answers to parents of children with rare neurological gene mutation
11 hours ago

Human-centered AI tool to improve sepsis management can identify missing information

AI-powered, big data research enhances understanding of systemic vasculitis

Team develops injectable heart stimulator for emergency situations

Finding epilepsy hotspots before surgery: A faster, non-invasive approach
13 hours ago

Personalized brain stimulation significantly decreases depression symptoms in pilot study

An ancient signaling pathway and 20 years of research offer hope for rare cancer

How mindset could affect the body's response to vaccination
Related stories.

Reduced drug use is a meaningful treatment outcome for people with stimulant use disorders, data show
Jan 10, 2024

Researchers investigate non-abstinent recovery for people with cocaine use disorder
May 23, 2024

Study reviews approaches to cocaine treatment for Black Americans
Apr 26, 2024

From opioid overdose to treatment initiation: Outcomes associated with peer support in emergency departments
Apr 16, 2024

Holistic approach best for tackling nonmedical drug use, study finds
Apr 24, 2020

Among young people, being around peers may elicit greater drinking cravings than the presence of alcohol
Jun 5, 2023
Recommended for you

Researchers find e-cigarette use disrupts the nasal microbiome
Aug 26, 2024

Frequent cannabis users miss more workdays, research shows

A compound in rosemary extract can reduce cocaine sensitivity
Aug 20, 2024

An implantable sensor could reverse opioid overdoses
Aug 14, 2024

New insights into brain's reward circuitry could aid addiction treatment

Hooked on a feeling: Opioids evoke positive feelings through a newly identified brain region
Aug 12, 2024
Let us know if there is a problem with our content
Use this form if you have come across a typo, inaccuracy or would like to send an edit request for the content on this page. For general inquiries, please use our contact form . For general feedback, use the public comments section below (please adhere to guidelines ).
Please select the most appropriate category to facilitate processing of your request
Thank you for taking time to provide your feedback to the editors.
Your feedback is important to us. However, we do not guarantee individual replies due to the high volume of messages.
E-mail the story
Your email address is used only to let the recipient know who sent the email. Neither your address nor the recipient's address will be used for any other purpose. The information you enter will appear in your e-mail message and is not retained by Medical Xpress in any form.
Newsletter sign up
Get weekly and/or daily updates delivered to your inbox. You can unsubscribe at any time and we'll never share your details to third parties.
More information Privacy policy
Donate and enjoy an ad-free experience
We keep our content available to everyone. Consider supporting Science X's mission by getting a premium account.
E-mail newsletter
Integrating deep learning for accurate gastrointestinal cancer classification: a comprehensive analysis of MSI and MSS patterns using histopathology data
- Original Article
- Open access
- Published: 26 August 2024
Cite this article
You have full access to this open access article
- Abeer A. Wafa 1 ,
- Reham M. Essa 2 ,
- Amr A. Abohany ORCID: orcid.org/0000-0002-7408-5073 3 &
- Hanan E. Abdelkader 4
Early detection of microsatellite instability (MSI) and microsatellite stability (MSS) is crucial in the fight against gastrointestinal (GI) cancer. MSI is a sign of genetic instability often associated with DNA repair mechanism deficiencies, which can cause (GI) cancers. On the other hand, MSS signifies genomic stability in microsatellite regions. Differentiating between these two states is pivotal in clinical decision-making as it provides prognostic and predictive information and treatment strategies. Rapid identification of MSI and MSS enables oncologists to tailor therapies more accurately, potentially saving patients from unnecessary treatments and guiding them toward regimens with the highest likelihood of success. Detecting these microsatellite status markers at an initial stage can improve patient outcomes and quality of life in GI cancer management. Our research paper introduces a cutting-edge method for detecting early GI cancer using deep learning (DL). Our goal is to identify the optimal model for GI cancer detection that surpasses previous works. Our proposed model comprises four stages: data acquisition, image processing, feature extraction, and classification. We use histopathology images from the Cancer Genome Atlas (TCGA) and Kaggle website with some modifications for data acquisition. In the image processing stage, we apply various operations such as color transformation, resizing, normalization, and labeling to prepare the input image for enrollment in our DL models. We present five different DL models, including convolutional neural networks (CNNs), a hybrid of CNNs-simple RNN (recurrent neural network), a hybrid of CNNs with long short-term memory (LSTM) (CNNs-LSTM), a hybrid of CNNs with gated recurrent unit (GRU) (CNNs-GRU), and a hybrid of CNNs-SimpleRNN-LSTM-GRU. Our empirical results demonstrate that CNNs-SimpleRNN-LSTM-GRU outperforms other models in accuracy, specificity, recall, precision, AUC, and F1, achieving an accuracy of 99.90%. Our proposed methodology offers significant improvements in GI cancer detection compared to recent techniques, highlighting the potential of DL-based approaches for histopathology data. We expect our findings to inspire future research in DL-based GI cancer detection.
Similar content being viewed by others
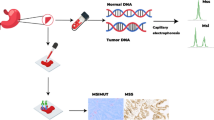
Automatic extraction of lightweight and efficient neural network architecture of heavy convolutional architectures to predict microsatellite instability from hematoxylin and eosin histology in gastric cancer
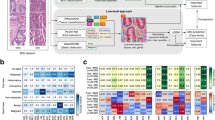
Comparative analysis of high- and low-level deep learning approaches in microsatellite instability prediction
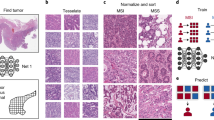
Deep learning can predict microsatellite instability directly from histology in gastrointestinal cancer
Explore related subjects.
- Artificial Intelligence
- Medical Imaging
Avoid common mistakes on your manuscript.
1 Introduction
Cancer has become one of the main causes of premature death in most countries. One of the most prevalent types of cancer is gastrointestinal (GI) cancer; esophageal, gastric, colonic, and rectal tumors are all examples of GI cancers. These tumors have a variety of clinical symptoms but some common traits despite having unique but related origins [ 1 ]. GI cancers account for about 20% of all cancer diagnoses and 22.5% of cancer deaths worldwide. Gastric cancer (GC) is the third most common cause of cancer death and the fifth most common type of cancer, but esophageal cancer has a very low prevalence. After lung and breast cancer, colorectal cancer (CRC) is the third most prevalent cancer in the world, but it also ranks second in terms of cancer-related fatalities [ 2 ].
GI cancer, a collective term for a range of malignancies affecting the digestive tract, including the esophagus, stomach, liver, pancreas, colon, and rectum, remains a significant global health challenge. Among these, CRC stands as a prevalent and often lethal manifestation. The intricacies and heterogeneity of GI cancers necessitate innovative approaches for early diagnosis and tailored treatment. In recent years, the role of microsatellite instability (MSI) and microsatellite stability (MSS) [ 3 ] in these malignancies has come into focus, and the integration of artificial intelligence (AI), particularly deep learning (DL), has emerged as a promising avenue for enhancing early detection and diagnostic precision.
MSI and MSS [ 4 , 5 , 6 ] represent distinct genetic phenotypes with profound clinical implications in managing GI cancer. Microsatellites are short, repetitive DNA sequences dispersed throughout the genome, and MSI arises when these sequences exhibit instability due to defects in DNA mismatch repair (MMR) mechanisms. This instability increases the mutation burden, leading to tumors characterized by unique genetic profiles. Conversely, MSS tumors exhibit genetic stability within their microsatellite regions but may harbor distinct genetic alterations.
The differentiation between MSI and MSS in GI cancer patients [ 7 , 8 ] is paramount, as it significantly informs clinical decision-making. MSI tumors are often associated with a more favorable prognosis and heightened sensitivity to immunotherapies, while MSS tumors may necessitate distinct therapeutic strategies. The accurate and timely identification of MSI or MSS status is crucial for optimizing treatment plans and ultimately improving patient outcomes.
Despite the abundance of prognostic and predictive biomarkers, high mortality rates for GI cancer patients indicate that there is still room for improvement in prognosis, opening the door to more individualized treatment approaches that could improve prognosis and/or reduce side effects. Oncology defines biomarkers as markers that also reveal the existence or absence of cancer or tumor behavior, such as therapy response or likelihood of disease recurrence. At least for the more prevalent cancer types, developing several molecular biomarkers in oncology has made it possible to treat tumors more precisely [ 9 , 10 ].
Early diagnosis of GI diseases is crucial to prevent their progression into malignant diseases. There are vital indicators that aid in diagnosing GI disorders, which, if identified early, can lead to prompt treatment and a reduction in mortality rates. However, despite the availability of these diagnostic tools, the death rate due to GI diseases remains high, signifying a failure in early diagnosis and management. It is imperative to improve our diagnostic approach to ensure timely and accurate identification of GI diseases to provide appropriate care and reduce mortality rates [ 11 ]. Cutting-edge medical technologies are now employed to identify cancer at its nascent stage, with radical resection being the go-to treatment option for nearly half of all cases. Thanks to the ever-increasing number and importance of molecular biomarkers in standard clinical practice, cancer treatments are now more accurately customized to the genetic profile of a specific tumor. However, the workflow’s expenses, processing time, and tissue prerequisites are also on the rise [ 12 , 13 ].
Preliminary identification of diseases like polyps and tumors through endoscopy is crucial for timely and effective treatment. However, manual diagnosis can be time-consuming and challenging, requiring extensive experience and clinical knowledge to track all video frames [ 14 ]. To overcome these difficulties, computer-aided diagnostic systems based on DL and hybrid techniques can be developed to assist doctors in making appropriate diagnoses during the early stages of a disease. By leveraging AI in the medical field, it may be possible to improve medical performance, reduce costs, and enhance the satisfaction of both patients and medical staff [ 15 ].
Biomarkers often necessitate tumor tissue in addition to standard diagnostic materials. Yet, the wealth of valuable clinical information within available tumor tissue remains largely untapped. Fortunately, modern advances in DL, an AI technology, have unlocked the ability to extract previously elusive information directly from typical cancer histology images, potentially providing valuable clinical insights [ 10 ]. With the help of artificial neural networks (ANNs), DL techniques can identify recurring patterns within complex datasets. Given the high information density of image data, it is an ideal candidate for analysis with DL techniques [ 16 ].
The growing use of AI, particularly DL-based AI, for tumor pathology is due to the development of digital pathology and advanced computer vision algorithms [ 17 ]. These DL-based algorithms can perform various tasks in tumor pathology, such as tumor diagnosis, subtyping, grading, staging, prognostic prediction, and identifying pathological features, biomarkers, and genetic changes. The use of AI in pathology improves diagnostic accuracy, reduces the workload of pathologists, and allows them to focus more on high-level decision-making tasks. Furthermore, AI is beneficial for pathologists to meet the demands of precision oncology [ 18 , 19 ].
Medical computer vision has shown great promise with AI-based diagnostic support systems, specifically through convolutional neural network (CNN)-based image analysis tools. CNN-based automatic diagnosis of endoscopic findings is becoming a mainstream DL technology. This technology can help endoscopists provide more accurate diagnoses by automatically detecting and classifying endoscopic lesions [ 20 ]. However, the success of DL for endoscopy relies heavily on the availability of high-quality endoscopic images and the endoscopist’s increased understanding of the technology. DL-based image analysis has the potential to benefit numerous medical specialties that use image data, including the accurate detection of tumors and segmental organs on computer tomography (CT) images, surpassing human capabilities [ 21 ].
1.1 Motivations
Gastrointestinal (GI) cancer is a leading cause of cancer-related deaths, often going undetected in its early stages due to the limitations of traditional diagnostic methods. These methods are invasive and subjective and can lead to inconsistent diagnoses and delays in treatment. There is a pressing need for advanced diagnostic tools that enhance accuracy and speed to address these challenges.
Deep learning (DL) models present a transformative solution. By automating the analysis of complex histopathological images, DL can significantly improve the accuracy and consistency of GI cancer diagnoses. This research aims to leverage DL, particularly hybrid models combining convolutional neural networks (CNNs) and recurrent neural networks (RNNs), to capture intricate spatial and temporal patterns in histopathology images. This innovative approach aims to facilitate early detection, thereby improving patient outcomes and advancing the field of medical diagnostics.
The importance of this research lies in its potential to revolutionize GI cancer diagnosis. Early detection is crucial for effective treatment and improved survival rates. By developing a system that can accurately and quickly identify GI cancer early, we can significantly reduce the burden on healthcare systems, lower treatment costs, and, most importantly, save lives. This study not only contributes to the body of knowledge in medical AI but also has the potential for real-world impact, offering a reliable tool for clinicians in the fight against cancer.
1.2 Objectives
The primary objective of this research is to revolutionize early gastrointestinal (GI) cancer detection using advanced deep learning (DL) models. This proposed approach not only aims to provide accurate diagnoses but also to enhance patient outcomes through timely intervention. The specific goals are as follows:
Comprehensive preprocessing pipeline: Establish a robust preprocessing pipeline for histopathology images, integrating essential steps such as resizing, labeling, normalization, and color transformation. This ensures standardized and high-quality data preparation for subsequent analysis.
Efficient data handling techniques: Implement advanced data processing strategies to effectively manage and optimize large-scale histopathology image datasets. These techniques enhance computational efficiency and facilitate swift and accurate analysis.
Hybrid DL model design and evaluation: Develop and rigorously evaluate hybrid deep learning models that combine convolutional neural networks (CNNs) with various recurrent neural networks (RNNs) like SimpleRNN, GRU, and LSTM. These models excel in capturing nuanced spatial and temporal patterns, enhancing feature extraction and classification capabilities.
Robust performance metrics: Employ a comprehensive suite of performance metrics including accuracy, precision (PR), recall (RC), F1 score (F1), specificity (SP), area under the curve (AUC), and processing time. This thorough evaluation validates the effectiveness and reliability of the DL models.
Clinical application integration: Identify and recommend the most effective DL model for seamless integration into clinical workflows, ensuring practical applicability and enhancing diagnostic decision-making in real-world settings.
Proposed system overview: Provide a detailed breakdown of the proposed system, encompassing preprocessing, feature extraction, classification, and evaluation stages. Describe the architecture of each model component, elucidating their roles within the overall framework.
1.3 Contributions
This paper introduces several contributions, which are listed as follows:
Innovative system design: Proposes a comprehensive system for early GI cancer detection, integrating specific DL models tailored to histopathology image analysis, effectively managing a large dataset of 192,312 images.
Advanced preprocessing pipeline: Develops a sophisticated pipeline including data resizing, labeling, normalization, and color transformation, optimized for histopathology images. It ensures scalability and efficiency while maintaining high-quality data input for the DL models.
Handling large datasets: Utilizes CNNs integrated with various RNN architectures (SimpleRNN, GRU, LSTM) to address the challenge of large dataset size, enhancing processing speed and accuracy.
Experimental model integration: Introduces a novel integration of CNNs with RNNs for GI cancer detection. This experiment-driven approach, including custom hybrid models like CNN-SimpleRNN-LSTM-GRU, combines strengths of different networks for better accuracy and efficiency.
Interpretable AI: Incorporates methods such as attention mechanisms or saliency mapping to enhance model interpretability, facilitating clinical understanding and trust in the diagnostic decisions made by the models.
Performance optimization: Highlights hybrid models’ effectiveness in automated feature extraction and classification, focusing on improved accuracy, validation loss, and real-time processing capabilities.
Clinical relevance: Demonstrates practical application of hybrid DL models, supporting clinicians with accurate, timely diagnostic decisions, adaptable to different clinical settings.
Comprehensive evaluation and metrics: Provides a thorough evaluation using metrics like accuracy, precision(PR), recall(RC), F1 score (F1), specificity (SP), AUC, loss, and processing time, validating the approach’s effectiveness.
Resource management and computational efficiency: Employs strategies for optimizing computational resources and ensuring scalability, facilitating application to larger datasets or other medical image data types.
Future research directions: Suggests avenues for exploring molecular signatures of MSI and MSS, improving model interpretability, and testing transferability across diverse patient cohorts and datasets.
1.4 Structure
The following structure is adopted in this paper: We begin by reviewing prior research in the field in Sect. 2 . Section 3 outlines different techniques for analyzing and diagnosing GI cancer. The results of experimental evaluations of the proposed systems on the dataset are presented in Sect. 4 . Sections 4.4.6 and 4.5 summarize the discussion and comparison of the performance of the proposed approaches. Finally, Sect. 5 concludes the paper with recommendations for future research.
2 Literature review
This section will review several prior investigations into identifying GI diseases, especially GI cancer. We’ll also go over the techniques used to make this diagnosis and how AI and DL may aid in making the earliest and most accurate diagnoses of these disorders and assist doctors in making accurate diagnostic decisions as the following subsection:
2.1 Deep learning and neural network approaches in GI cancer diagnosis
Kather et al. [ 22 ] identified ResNet18 as the optimal neural network architecture for tumor detection in the Cancer Genome Atlas (TCGA) dataset. Their study revealed that ResNet18 exhibited several advantages, including shorter training times, superior classification performance, and a reduced risk of overfitting due to its fewer parameters. Achieving an impressive AUC score of 0.84, this study underscores the importance of selecting appropriate neural network architectures to enhance diagnostic accuracy and efficiency.
Chen et al. [ 23 ] demonstrated the efficacy of support vector machines (SVM) in automatic microsatellite instability (MSI) classification using data derived from TCGA’s stomach adenocarcinoma dataset. Their model displayed remarkable performance, with a training AUC of 0.976 and a validation AUC of 0.95. This highlights the promise of traditional machine learning (ML) techniques in MSI classification, maintaining their relevance alongside newer AI methods.
Yamashita et al. [ 24 ] introduced MSINet, a DL model for MSI detection. Evaluated on external datasets with 100 H &E-stained whole slide images (WSIs), the model achieved a net present value (NPV) of 93.7%, RC of 76.0%, SP of 66.6%, and an AUC of 0.779. Compared to expert GI pathologists, MSINet demonstrates how DL models can complement human expertise in pathology, enhancing diagnostic consistency and accuracy.
Cao et al. [ 25 ] performed a comprehensive study involving two patient cohorts from TCGA-COAD and an Asian-CRC cohort. Their innovative pathomics model, EPLA, demonstrated remarkable performance, achieving an AUC of 0.8848 in the TCGA-COAD cohort and 0.8504 in the Asian-CRC cohort. These outcomes underscore the significant potential of EPLA in clinical settings for CRC diagnosis and treatment, emphasizing the need for robust model validation across diverse populations.
Zhang et al. [ 26 ] developed a deep learning model based on preoperative CT that can predict the prognosis of patients with advanced gastric cancer. Machine learning features were extracted from the maximal tumor layer of portal vein CT images. The LASSOCox regression method was used to select features and generate labels. Then, a Cox regression model was used to integrate markers and clinicopathological information to build prediction models. Tumor segmentation was also performed to provide a more detailed analysis. It was recommended to study the possibility of verifying the results of this study retrospectively through tumor segmentation and image classification.
Padmavathi et al. [ 27 ] proposed a novel deep learning (DL) technique for classifying Gastrointestinal Disease categories from wireless capsule endoscopy (WCE) images using the mean filter to remove noise from given input images. The DenseNet-121 technique is then used to extract features such as shape and position from wireless capsule endoscopy images. The enhanced whale optimization algorithm (EWOA) is used to select the features. The experiments used the Kvasir v2 dataset and performed well regarding RC, PR, accuracy, and F1.
2.2 Incorporating transfer learning and advanced techniques
Khan et al. [ 28 ] proposed a transfer learning strategy using DL techniques and the Xception network to distinguish between MSI and MSS cancers based on histological images from formalin-fixed paraffin-embedded (FFPE) materials sourced from TCGA. Their approach yielded a notable accuracy rate of 90.17% and a test AUC of 0.932, highlighting its potential in advancing the diagnosis and treatment of these cancers. This showcases the effectiveness of transfer learning in adapting pre-trained models to new medical imaging tasks with high accuracy.
Lee et al. [ 29 ] automated MSI status classification in GC tissue slides using frozen and FFPE samples from TCGA. Their model achieved impressive AUCs of 0.893 and 0.902 for the respective sample types and an AUC of 0.874 in an external validation Asian FFPE cohort. This underscores the potential for automated MSI classification in clinical practice, highlighting the versatility and robustness of their approach.
Zhu et al. [ 30 ] developed an effective tool for predicting MSI status and assessing tumor recurrence risk in GC using preoperative Dual-Layer Computed Tomography (DLCT) scans. The study achieved excellent predictive accuracy, with an AUC of 0.879, high sensitivity, and specificity. This enhances preoperative evaluations of GC patients, demonstrating the utility of integrating advanced imaging techniques with AI for comprehensive cancer assessment.
Saldanha et al. [ 31 ] executed a retrospective study on Swarm Learning (SL) and its effectiveness in predicting molecular biomarkers in GC. Their SL-based classifier demonstrated robust performance, achieving AUCs of 0.8092 for MSI precision and 0.8372 for Epstein–Barr virus (EBV) prediction in external validation. Notably, the centralized model, trained on all datasets on a single computer, displayed comparable performance.
Qiu et al. [ 32 ] explored the relationship between MSI status and various molecular markers in CRC, developing a DL framework that predicted MSI status based solely on H &E staining images. Their framework yielded promising results, achieving an acceptable AUC of 0.809 in fivefold cross-validation for predicting MSI status using H &E images and a significantly improved AUC of 0.952 when combined with DNA methylation data. This study emphasizes the potential of DL and H &E images for MSI status prediction in CRC.
Yu et al. [ 33 ] contributed to the field by developing an innovative Deep Neural Network (DNN)-based approach to identify MSI in gastric whole slide images. Their model incorporated non-local and visual context fusion modules, resulting in an impressive accuracy rate of 96.53% and an AUC of 0.99 on the TCGA-STAD public dataset. These findings hold significant promise for advancing the diagnosis and treatment of MSI in GC.
2.3 Exploring newer AI techniques: vision transformers
Recent advancements in computer vision have seen the rise of vision transformers (ViTs) [ 34 ], which have shown significant promise in various image classification tasks [ 35 , 36 ]. Here, we discuss notable applications of ViTs in medical imaging and GI cancer diagnosis:
Adaptive vision transformers (Adavit): Adavit offers an adaptive mechanism for Vision Transformers [ 37 , 38 ], enhancing image recognition efficiency. This approach is particularly useful in medical imaging, where high accuracy and efficient computation are paramount.
Cross-attention multi-scale vision transformer (CrossViT): CrossViT [ 39 , 40 ] improves image classification by leveraging cross-attention mechanisms and multi-scale feature extraction. This method’s ability to integrate information from different scales can be critical in identifying and classifying subtle features in medical images.
Evaluation of vision transformers for traffic sign classification: Although focused on traffic signs [ 41 ], this study’s principles of evaluating and optimizing Vision Transformers can be applied to medical imaging, particularly for recognizing complex patterns in histopathological slides.
Classification of brain tumor from magnetic resonance imaging using vision transformers ensembling: This study [ 42 ] demonstrates ViTs’ potential in brain tumor classification, indicating their applicability to other cancers, including GI cancer, by combining multiple transformer models for improved accuracy.
Therefore, integrating vision transformers into GI cancer diagnosis could potentially enhance performance due to their ability to capture long-range dependencies and intricate patterns in medical images, making them a promising direction for future research.
2.4 Concluding remarks
From the above, we can conclude that the clinical application of digital biomarkers faces significant obstacles. However, implementing DL concepts offers considerable advantages for diagnostic and therapeutic decision-making. Despite this, there are still substantial hurdles to overcome with AI integration. These include algorithm validation and interpretability, computing systems, and skepticism from pathologists, clinicians, patients, regulators, and reimbursements. Our presentation provides an overview of how AI-based approaches can be incorporated into pathologists’ workflows. We discuss the challenges and possibilities of AI implementation in tumor pathology. Recent investigations into CNN-based image analysis in GI cancer pathology have presented encouraging findings. However, the studies were conducted in observational and retrospective contexts. Large-scale trials are necessary to evaluate performance and predict clinical usefulness. Extensive validation through large-scale trials is required before CNN-based prediction models can be authorized as medical devices.
3 The suggested approach
In this paper, a system is proposed to detect GI cancer at an early stage by utilizing specific DL models. The system comprises several key steps. Initially, the histopathology images undergo a preprocessing pipeline incorporating several techniques, including data resizing, labeling, normalization, and color transformation. The preprocessed dataset is subsequently used to develop training and testing datasets on which the recommended DL models are constructed and trained. Attention mechanisms or saliency mapping were integrated to enhance the interpretability of the models, facilitating clinical understanding and trust in their diagnostic decisions. During this study’s experiment, a significant challenge was encountered due to the large size of the data. As a result, only CNNs were employed. However, time posed another obstacle, which was overcome by combining CNNs with each type of RNN family, namely SimpleRNN, gated recurrent unit (GRU), and long short-term memory (LSTM), followed by their amalgamation. The subsequent sections detail how these DL models operate and perform automated feature extraction and classification tasks. The training accuracy and validation loss values were assessed at each period. Then, the effectiveness of the DL models was evaluated using assessment measures such as accuracy, precision(PR), recall(RC), F1 score(F1), and processing time. Figure 1 shows the general design of the proposed system for early detection of GI cancer.
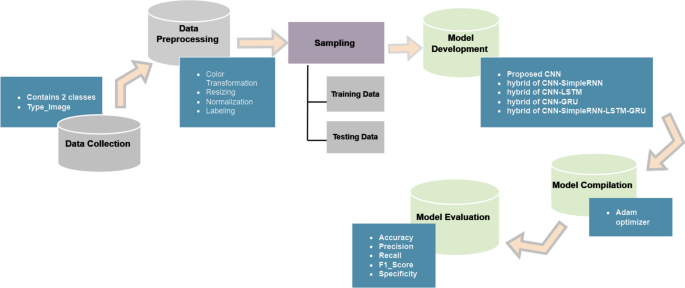
Overall design of the proposed system for early detection of GI cancer
3.1 The suggested neural network architecture based
3.1.1 convolutional neural networks.
Convolutional neural networks (CNNs) [ 43 , 44 , 45 , 46 ] represent a powerful class of DL models that have revolutionized the field of computer vision and expanded their reach into other domains. These networks are designed to process grid-like data, particularly images, with remarkable efficiency and accuracy. The fundamental concept underlying CNNs is the convolution operation, which mimics how the human visual system recognizes patterns and features. In a CNN, multiple layers of convolution, pooling, and nonlinear activation functions work together to automatically extract hierarchical features from input data, gradually learning to recognize complex patterns. This hierarchical feature extraction makes CNNs exceptionally well suited for tasks like image classification, object detection, and image segmentation. Their success has extended to diverse applications, including medical image analysis, natural language processing, and playing strategic games. CNNs continue to be a fundamental tool in AI, shaping how we process and understand visual information in the digital age. Its fundamental components and mathematical operations can be described as follows:
Input data: In a CNN, the input data are represented as a 3D tensor, where the dimensions are (height, width, and channels). Each dimension corresponds to the image’s height, width, and the number of color channels (e.g., red, green, blue for a color image). Mathematically, this input can be denoted as I(x, y, c), where (x, y) are the spatial coordinates, and c represents the channel.
Convolution operation: The core operation of a CNN is the convolution. It involves applying a set of learnable filters (kernels) to the input data to extract features. Each filter is a small grid of weights. The convolution operation at a specific location ( x , y ) in the feature map can be mathematically defined as follows:
where ( C * F )( x , y ) is the result of the convolution at location ( x , y ), the double summation is over the spatial dimensions i and j, and the triple summation is over the input data channels c.
Activation function: After the convolution operation, an activation function, often ReLU (Rectified Linear Unit), is applied element-wise to introduce non-linearity. The ReLU activation function is defined as:
where x is the result of the convolution.
Pooling layer: Pooling layers are used to downsample the feature maps, reducing their spatial dimensions. Max-pooling is a common pooling operation where the maximum value within a small window is retained. Mathematically, for a pooling window size W and a feature map F, the pooling operation can be defined as:
where ( P * F )( x ’, y ’) is the result of pooling at location ( x ’, y ’) and the summation is over the pooling window dimensions i and j.
Fully connected layers: The last layers of a CNN are typically fully connected layers. These layers flatten the feature maps and connect every neuron to the previous layer’s neurons. Mathematically, if N is the number of neurons in the fully connected layer, and x is a vector representing the flattened feature maps, the operation is given by:
where FC( x ) represents the output of the fully connected layer, W is a weight matrix, and b is a bias vector.
Output layer: The final layer of the CNN, known as the output layer, produces the network’s prediction or classification. Depending on the task, it might use different activation functions. For instance, image classification could employ the softmax function to assign probabilities to different classes. The softmax operation ensures that the resulting probabilities sum up to 1. Its operation is given by:
where \(P(y_i)\) is the probability of the input belonging to class i, \(z_i\) is the unnormalized score (logit) associated with class i, and \(\sum\) represents the summation over all classes from 1 to n.
By stacking multiple convolutional layers, activation functions, pooling layers, and fully connected layers, a CNN, as in Fig. 2 , learns to extract and represent features automatically at various abstraction levels. This mathematical framework allows CNNs to excel in various computer vision tasks, from image classification to object detection and segmentation.
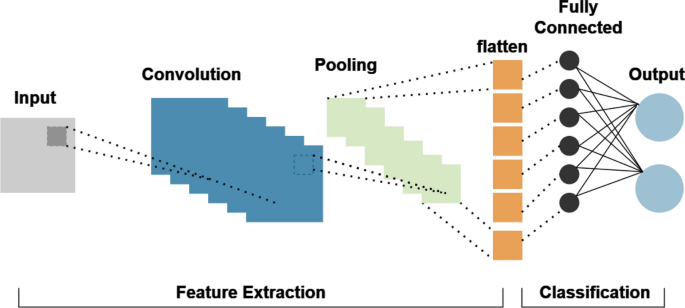
CNN architecture
3.1.2 Recurrent neural networks
Recurrent neural networks (RNNs) [ 47 , 48 , 49 , 50 ] are a class of ANNs designed to process sequences of data while maintaining the memory of past information. Scientifically, RNNs are characterized by their unique architecture featuring recurrent connections, which allow information to flow through loops within the network. This distinctive property makes RNNs particularly suitable for tasks involving sequential data, where the order of elements matters, such as time-series analysis, natural language processing, and speech recognition.
The fundamental component of an RNN is its hidden state, which evolves as the network processes input sequences. This hidden state acts as a form of memory, capturing and storing relevant information from previous time steps and influencing the network’s predictions at the current time step. Mathematically, RNNs are described by equations that update the hidden state and produce output predictions based on the current input and the previous hidden state.
However, traditional RNNs suffer from issues such as the vanishing gradient problem, which limits their ability to capture long-range dependencies in sequences. Advanced RNN variants, including LSTM and GRU networks, have been developed to overcome these limitations. These variants introduce gating mechanisms that regulate the flow of information within the network, allowing them to capture and retain important information over longer sequences.
The RNN family encompasses a range of architectures and variations, each with specific advantages and use cases. For instance, one-to-one RNNs handle fixed-size inputs and outputs, while one-to-many RNNs generate sequences from a single input, e.g., image captioning. Many-to-one RNNs, on the other hand, process sequences and produce a single output, e.g., sentiment analysis, and many-to-many RNNs handle sequential inputs and outputs e.g., machine translation. Understanding these variations and choosing the appropriate RNN type is crucial for tackling diverse sequence-related tasks in scientific research and practical applications. Despite their usefulness, RNNs have some limitations, such as sensitivity to hyperparameters and difficulties in parallelization. These have led to the development of alternative architectures like Transformers, which have shown exceptional performance in handling sequential data across various domains. RNNs come in various types, each tailored to specific tasks and requirements. Here are some common types of RNNs:
SimpleRNN: A vanilla recurrent neural network (RNN), also known as a Simple RNN [ 44 , 51 , 52 ], is a type of ANN designed for processing data sequences. It is widely used in natural language processing, speech recognition, and time-series analysis due to its ability to capture sequential dependencies. In this explanation, we will delve into the scientific details of how a Vanilla RNN works. At its core, a Vanilla RNN, as in Fig. 3 , consists of a network of interconnected neurons, with each neuron representing a hidden state at a particular time step. Let’s denote the hidden state at time step t as \(h{_t}\) . The fundamental operation of a Vanilla RNN can be expressed mathematically as follows:
where \(X{_t}\) represents the input at time step t, W is the weight matrix connecting the input to the hidden state, U is the weight matrix connecting the previous hidden state to the current hidden state, and f is an activation function that introduces non-linearity into the network. Common choices for the activation function include the hyperbolic tangent \(\tanh\) or the \(\sigma\) . The above equation shows how the current hidden state \(h{_t}\) is computed based on the input \(X{_t}\) and the previous hidden state \(h{_{t-1}}\) ." This recurrent connection allows the network to maintain a memory of past information and use it to influence the current state. It is a form of weighted summation of the input and the previous hidden state, where the weights are determined by the matrices W and U . Training a Vanilla RNN involves optimizing the weights W and U to minimize a loss function that measures the difference between the predicted and target outputs. This is typically done using a technique called backpropagation through time (BPTT), which is an extension of the standard backpropagation algorithm for feedforward neural networks. BPTT computes gradients for each time step and adjusts the weights accordingly. However, Vanilla RNNs suffer from several limitations. One significant issue is the vanishing gradient problem, where gradients can become extremely small as they are propagated back during training. This can lead to difficulties in learning long-range dependencies. To address this problem, more advanced RNN variants like LSTM and Gated GRU have been developed. In conclusion, a Vanilla RNN is a fundamental type of RNN used for sequence modeling. It operates by updating a hidden state at each time step based on the input and the previous hidden state, using weighted connections. While it has been instrumental in various applications, it has limitations related to the vanishing gradient problem mitigated by more advanced RNN architectures. Understanding the mathematical operations behind Vanilla RNNs is essential for grasping the fundamentals of sequence modeling in neural networks.
LSTM: Long short-term memory (LSTM) [ 53 , 54 , 55 , 56 ], a specialized RNN architecture known for its ability to capture and manage long-range dependencies in sequential data. LSTM is an advanced variant of RNNS designed to address the limitations of traditional RNNs, particularly the vanishing gradient problem, which hampers their ability to capture relationships between distant time steps in a sequence. LSTM, as in Fig. 4 , achieves this by introducing a complex but effective gating mechanism composed of three key gates: the input gate ( i ), the forget gate ( f ), and the output gate ( o ).
Forget gate (f): The forget gate is crucial in deciding what information to retain or forget from the previous cell state \(C_{t-1}\) . It takes as input the previous hidden state \(h_{t-1}\) and the current input \(X_t\) . Through a sigmoid activation function \(\sigma\) , it outputs values between 0 and 1 for each element in a vector. These values determine what parts of the previous cell state should be retained values close to 1 and what should be discarded values close to 0 as in the following equation:
where \(W{_f}\) represents the weights associated with the forget gate and \(b{_f}\) is the bias term.
Input gate (i) and candidate cell state update ( \(\tilde{C{_t}})\) : The input gate, similar to the forget gate, utilizes the previous hidden state \(h_{t-1}\) and the current input \(X{_t}\) . It employs two parts: the input gate \(i{_t}\) and the candidate cell state update \(\tilde{C{_t}}\) . The input gate \(i_t\) also uses the sigmoid activation to control what new information should be added to the cell state. Simultaneously, the candidate cell state update \(\tilde{C{_t}}\) computes a new candidate cell state with a hyperbolic tangent \(\tanh\) activation function, providing a range of possible values for the new cell state as in the following equations:
Cell state update ( \(C_{t}\) ): The cell state \(C_{t}\) is updated by combining information from the previous cell state that was determined to be relevant by the forget gate \(f_{t}\) and the new candidate values \(\tilde{C_{t}}\) determined by the input gate \(i_{t}\) as in the following equation:
Output gate (o) and hidden state (h): The output gate (o) decides which parts of the cell state should be exposed as the hidden state \(h_{t}\) and propagated to the next time step. Like the input and forget gates, it uses the previous hidden state \(h_{t-1}\) and the current input \(X_{t}\) , employing a sigmoid activation for gating and a tanh activation to compute the new hidden state as in the following equations:
The LSTM architecture incorporates these gates and states to selectively retain, update, and expose information over time. It is exceptionally suited for modeling complex sequential data with long-term dependencies. LSTMs have proved highly effective in various applications, including natural language processing, speech recognition, and time-series forecasting, where understanding intricate temporal relationships is paramount. The gating mechanism of LSTMs addresses the vanishing gradient problem, enabling the network to learn and remember important information over extended sequences, making them a powerful tool in deep learning.
GRU: Gated recurrent unit (GRU) [ 57 , 58 , 59 ], another popular RNN architecture that shares similarities with LSTM but has a simpler structure while retaining the ability to capture long-range dependencies in sequential data. GRU, like LSTM, is designed to mitigate the vanishing gradient problem and improve the modeling of temporal relationships. It is also effective at preventing exploding gradients to some extent. Exploding gradients occur when the gradients become exceedingly large during training, which can lead to numerical instability and slow convergence. GRUs, as in Fig. 5 , through their gating mechanism, help stabilize the gradients and prevent them from becoming excessively large. It achieves this through two gates: the update gate ( z ) and the reset gate ( r ).
Update gate (z) and reset gate (r): At the heart of the GRU architecture are the update and reset gates. These gates are responsible for controlling the flow of information within the unit. The update gate determines what portion of the previous hidden state \(h_{t-1}\) should be preserved. In contrast, the reset gate decides what information from the previous hidden state and current input \(X_{t}\) should be forgotten. Both gates are computed using sigmoid activation functions as in the following equations:
where \(W_{z}\) and \(W_{r}\) represent the weight matrices associated with the update and reset gates.
Candidate hidden state \(\tilde{h_{t}}\) : The candidate hidden state \(\tilde{h_{t}}\) is a temporary representation of the information that might be included in the current hidden state. It is computed by applying the reset gate \(r_{t}\) to the previous hidden state \(h{t-1}\) and combining it with the current input \(x_{t}\) through a hyperbolic tangent tanh activation as in the following equation:
where \(W_h\) represents the weight matrix associated with the candidate’s hidden state.
Update of the hidden state \(h_t\) : The final step in the GRU’s operations involves updating the hidden state \(h_t\) by taking into account the update gate \(z_t\) and the candidate hidden state \(\tilde{h_t}\) as in the following equation:
This equation combines the previous hidden state \(h_{t-1}\) with the candidate hidden state \(\tilde{h_t}\) based on the values of the update gate \(z_t\) . If the update gate is close to 1, it means that most of the previous hidden state will be preserved, and if it is close to 0, it means that most of the new information will be retained.
In summary, the GRU is an RNN architecture that utilizes update and reset gates to regulate the flow of information within the network. While simpler than LSTM’s, its design still enables it to capture long-range dependencies in sequential data effectively. GRUs have gained popularity due to their computational efficiency and competitive performance in various applications, including natural language processing, speech recognition, and time-series forecasting. The mathematical operations described here are the key components of the GRU’s functionality, allowing it to model complex temporal relationships in sequential data.
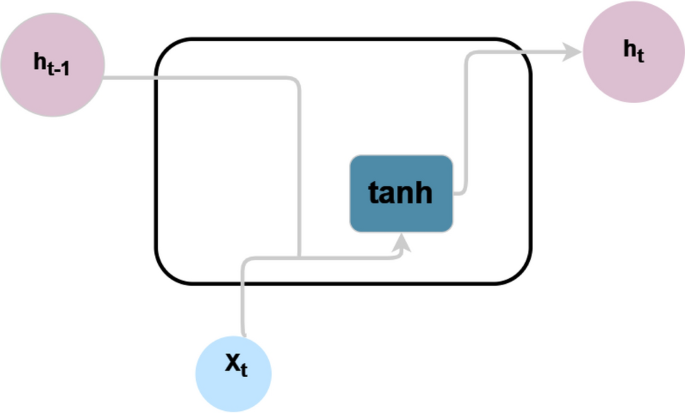
Vanilla RNN architecture
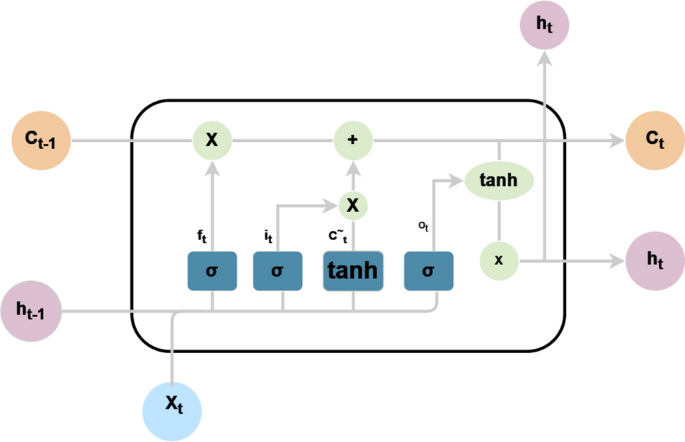
LSTM architecture
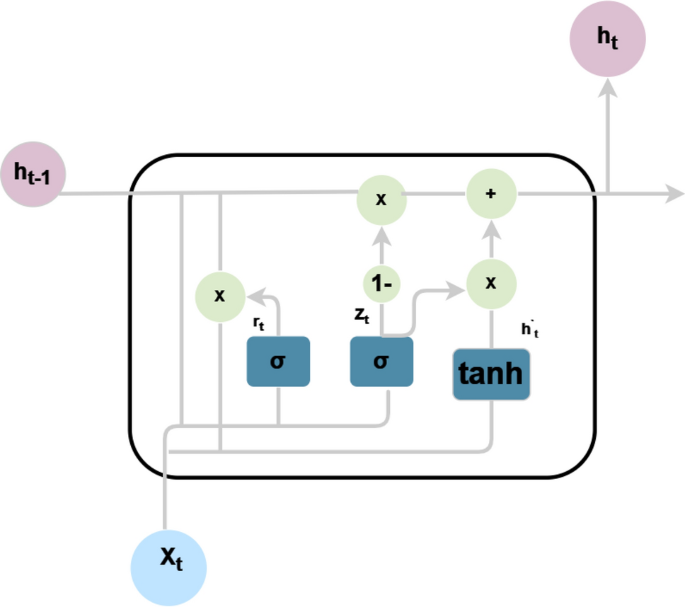
GRU architecture
3.2 The suggested DL models
3.2.1 the suggested cnn model.
In this paper, the suggested CNN model in Fig. 6 involves 11 layers. It consists of several convolutional and pooling layers to discover the preprocessed images’ features and perform the classification task. The model is created sequentially, meaning you can add layers sequentially, one after the other. The following layers make up the proposed CNNs model:
Convolutional layers:
First convolutional layer: Let the input image be \(\textbf{I} \in \mathbb {R}^{65 \times 65 \times 3}\) . The first convolutional layer applies 16 filters \(\textbf{W}_1 \in \mathbb {R}^{3 \times 3 \times 3 \times 16}\) with ReLU activation. The output \(\textbf{O}_1\) is given by:
where \(*\) denotes the convolution operation, and \(\textbf{b}_1 \in \mathbb {R}^{16}\) is the bias term.
Subsequent convolutional layers: For the \(i\) -th convolutional layer ( \(i=2,3,4\) ), each with \(f_i\) filters of size \(3 \times 3\) , the output \(\textbf{O}_i\) is:
where \(\textbf{W}_i \in \mathbb {R}^{3 \times 3 \times f_{i-1} \times f_i}\) and \(\textbf{b}_i \in \mathbb {R}^{f_i}\) . The number of filters \(f_i\) increases as follows: \(f_2 = 32\) , \(f_3 = 64\) , and \(f_4 = 128\) .
Max-pooling layers: Each max-pooling layer reduces the spatial dimensions of the feature maps. If \(\textbf{P}_i\) denotes the output of the max-pooling operation after the \(i\) -th convolutional layer, it is given by:
where \(\text {MaxPool}\) represents the max-pooling operation with a pool size of \(2 \times 2\) .
Flatten layer: The output of the final max-pooling layer \(\textbf{P}_4\) is flattened to form a 1D vector \(\textbf{F}\) :
Fully connected (dense) layers:
First dense layer: The first dense layer with 512 units applies the ReLU activation function. The output \(\textbf{D}_1\) is:
where \(\textbf{W}_5 \in \mathbb {R}^{n \times 512}\) (with \(n\) being the length of the flattened vector) and \(\textbf{b}_5 \in \mathbb {R}^{512}\) .
Second dense layer: The second dense layer, with 2 units and softmax activation, produces the final class probabilities \(\textbf{D}_2\) :
where \(\textbf{W}_6 \in \mathbb {R}^{512 \times 2}\) and \(\textbf{b}_6 \in \mathbb {R}^{2}\) .
The architecture described ensures the effective extraction of spatial features through convolutional layers, dimensionality reduction via max-pooling layers, and final classification using fully connected layers.
The reason for selecting CNNs stems from their well-established efficacy in handling image processing tasks. Their ability to capture spatial hierarchies in images through convolutional and pooling layers provides an effective means to discern complex textures and patterns. When considering histopathology data, these images often contain intricate spatial structures and features that require sophisticated feature extraction. CNNs excel in extracting these relevant spatial features, making them highly suitable for initial processing and feature extraction in GI cancer classification.
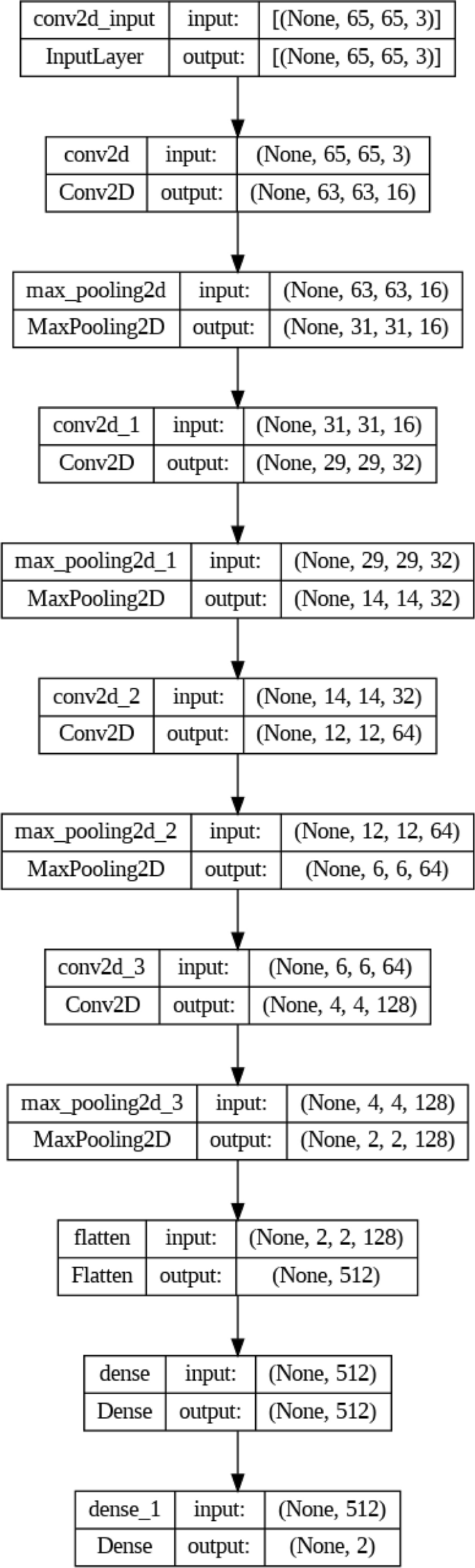
Proposed CNN model
3.2.2 The hybrid CNN-SimpleRNN model
In this paper, a hybrid model that combines CNNs with SimpleRNN, as in Fig. 7 , can be a valuable tool in image classification, even when the data are not inherently sequential. While CNNs are typically used for processing spatial information in images, and SimpleRNN is known for handling sequential data, this combination introduces a unique capability to capture non-sequential patterns and relationships. When applied to image classification with non-sequential data, this hybrid model can have a profound impact on both accuracy and training time.
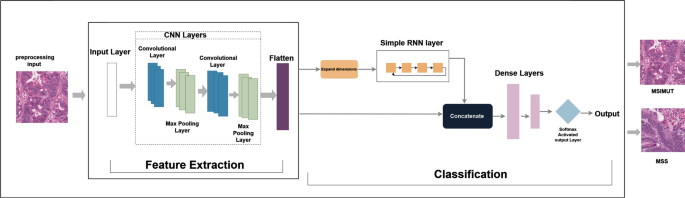
Hybrid CNN-SimpleRNN architecture
The description of this hybrid model is presented in Fig. 8 and in the following points:
Input layer: The input image \(\textbf{I} \in \mathbb {R}^{50 \times 50 \times 3}\) represents a 50x50 pixel image with three color channels (RGB).
CNN layers:
First CNN layer:
where \(\textbf{W}_1 \in \mathbb {R}^{3 \times 3 \times 3 \times 64}\) are the filters and \(\textbf{b}_1 \in \mathbb {R}^{64}\) is the bias.
First max-pooling layer:
Second CNN layer:
where \(\textbf{W}_2 \in \mathbb {R}^{3 \times 3 \times 64 \times 128}\) and \(\textbf{b}_2 \in \mathbb {R}^{128}\) .
Second max-pooling layer:
Third CNN layer:
where \(\textbf{W}_3 \in \mathbb {R}^{3 \times 3 \times 128 \times 512}\) and \(\textbf{b}_3 \in \mathbb {R}^{512}\) .
Third max-pooling layer:
Flatten layer: The output of the final max-pooling layer is flattened into a 1D vector \(\textbf{F}\) :
SimpleRNN integration:
The flattened vector \(\textbf{F}\) is reshaped for RNN processing. Let \(\textbf{F}_{\text {exp}}\) be the expanded vector along the time dimension.
The SimpleRNN layer processes the expanded vector:
where \(\textbf{W}_R\) and \(\textbf{b}_R\) are the weights and biases of the SimpleRNN layer, respectively.
Combination layer: The outputs of the CNN and SimpleRNN layers are concatenated:
Output layer: The final dense layer with 2 units and softmax activation for classification:
where \(\textbf{W}_O \in \mathbb {R}^{d \times 2}\) and \(\textbf{b}_O \in \mathbb {R}^{2}\) .
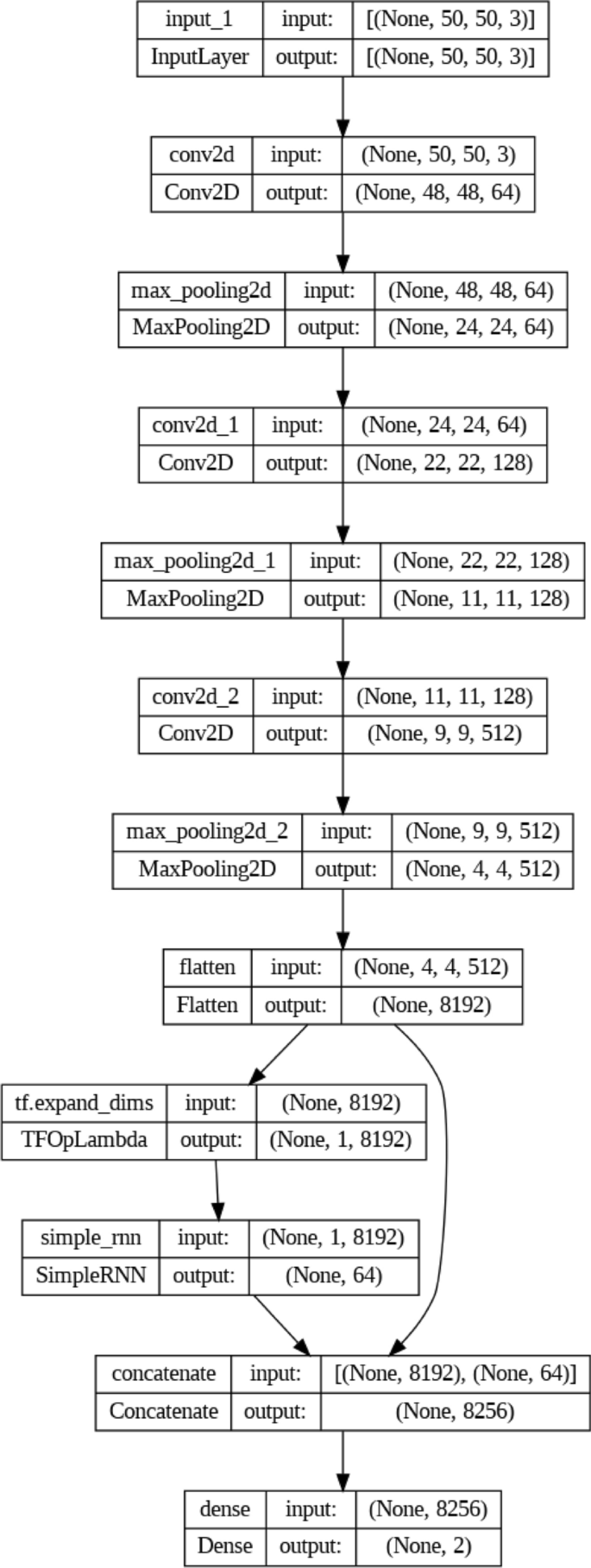
Hybrid CNN-SimpleRNN model
Finally, the use of this hybrid architecture not only improves the model’s classification capabilities in the presence of non-sequential data patterns but can also have an impact on reducing the time required for both training and testing, as it is explained in Sect. 4.4 .
The rationale for choosing SimpleRNNs in this hybrid model lies in their ability to capture temporal dependencies in data. This integration provides temporal context when combined with CNNs, even in non-sequential histopathology data. In alignment with histopathology data, the hybrid model’s approach delivers a nuanced understanding of both spatial and temporal patterns. This enables a more precise and adaptable classification of gastrointestinal (GI) cancer, contributing to a more comprehensive interpretation of complex histopathological images.
3.2.3 The hybrid CNN-LSTM model
A hybrid model that combines CNNs with LSTM networks, as in Fig. 9 provides a robust solution for image classification, particularly when dealing with non-sequential data. While CNNs are traditionally employed for image feature extraction, LSTMs are well suited for capturing sequential dependencies and patterns, such as those in image data. This hybrid approach enhances the model’s ability to recognize non-sequential features and relationships within the data while also introducing the potential to reduce the time required for both training and testing.
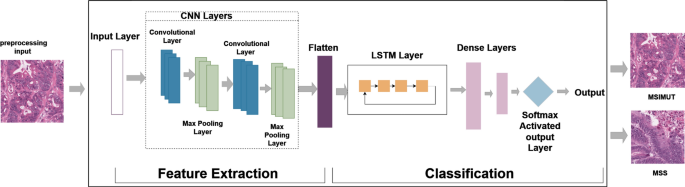
Hybrid CNN-LSTM architecture
The description of this hybrid model is presented in Fig. 10 and as in the following points:
Time-distributed CNN layers:
First time-distributed CNN layer:
where \(\textbf{W}_1 \in \mathbb {R}^{3 \times 3 \times 3 \times 64}\) are the filters, \(\textbf{b}_1 \in \mathbb {R}^{64}\) is the bias, and \(t\) represents the time step.
First time-distributed max-pooling layer:
Second time-distributed CNN layer:
Second time-distributed max-pooling layer:
Third time-distributed CNN layer:
where \(\textbf{W}_3 \in \mathbb {R}^{3 \times 3 \times 128 \times 128}\) and \(\textbf{b}_3 \in \mathbb {R}^{128}\) .
Third time-distributed max-pooling Layer:
Time-distributed flatten layer: The output of the final max-pooling layer is flattened into a 1D vector for each time step \(t\) :
LSTM layer: The sequence of flattened vectors \(\{\textbf{F}^{(t)}\}\) is processed by the LSTM layer:
where \(\textbf{H}_t\) and \(\textbf{C}_t\) are the hidden and cell states at time step \(t\) , and \(\textbf{W}_L\) and \(\textbf{b}_L\) are the weights and biases of the LSTM layer.
where \(\textbf{W}_O \in \mathbb {R}^{256 \times 2}\) and \(\textbf{b}_O \in \mathbb {R}^{2}\) .
Integrating an LSTM network into the traditional CNN architecture offers the model a better understanding of non-sequential data patterns and relationships. While the precise reduction in training and testing time can vary based on factors like dataset size, complexity, and hardware resources, this hybrid approach can potentially enhance the efficiency and accuracy of the image classification process. Combining the strengths of CNNs for spatial feature extraction and LSTMs for sequential dependencies, this model provides a robust solution for image classification tasks with non-sequential data.
The choice of LSTMs in this hybrid model is based on their advanced capability to capture long-term dependencies and address the vanishing gradient problem. LSTMs are well suited for complex temporal feature extraction. When combined with CNNs, LSTMs can detect intricate spatial and temporal relationships in histopathology images, contributing to the precise classification of GI cancer.
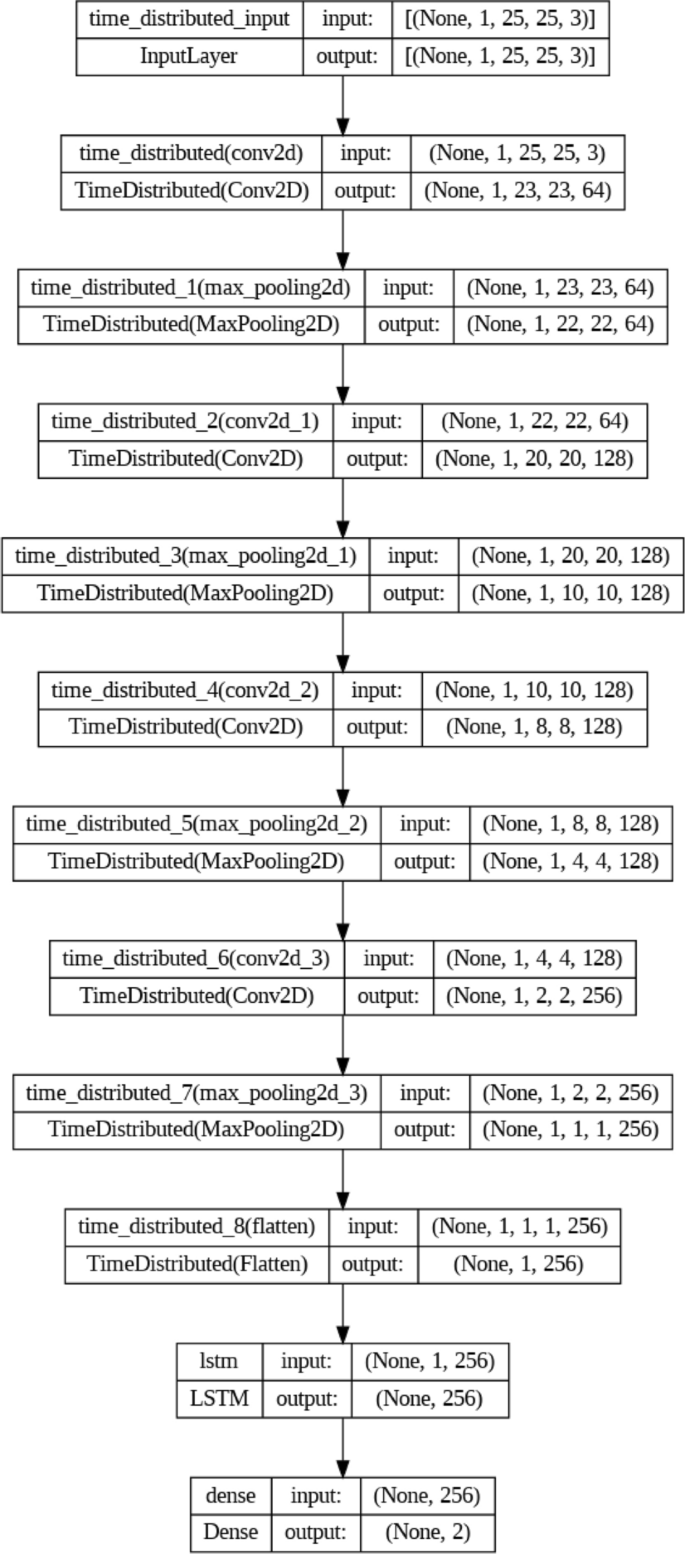
Hybrid CNN-LSTM model
3.2.4 The hybrid CNN-GRU model
A hybrid model that combines CNNs with GRU, as in Fig. 11 , presents a powerful approach to image classification, particularly when dealing with non-sequential data. CNNs excel at extracting spatial features from images, while GRUs, a type of RNN, can capture temporal dependencies in data. This hybrid model not only enhances the recognition of non-sequential patterns and relationships within the data but also can potentially reduce the time required for training and testing.
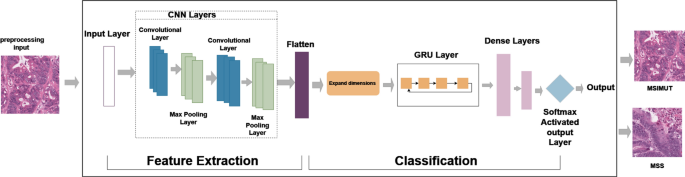
Hybrid CNN-GRU architecture
The description of this hybrid model is presented in Fig. 12 and in the following points:
Input layer: The model processes input images of dimensions (50, 50, 3), representing a \(50 \times 50\) pixel image with three color channels (RGB).
where \(\textbf{W}_1 \in \mathbb {R}^{3 \times 3 \times 3 \times 16}\) are the filters, and \(\textbf{b}_1 \in \mathbb {R}^{16}\) is the bias.
where \(\textbf{W}_2 \in \mathbb {R}^{3 \times 3 \times 16 \times 32}\) and \(\textbf{b}_2 \in \mathbb {R}^{32}\) .
where \(\textbf{W}_3 \in \mathbb {R}^{3 \times 3 \times 32 \times 64}\) and \(\textbf{b}_3 \in \mathbb {R}^{64}\) .
Fourth CNN layer:
where \(\textbf{W}_4 \in \mathbb {R}^{3 \times 3 \times 64 \times 128}\) and \(\textbf{b}_4 \in \mathbb {R}^{128}\) .
Fourth max-pooling layer:
Flatten layer: The output of the final max-pooling layer is flattened into a 1D vector:
GRU integration:
Expanding time dimension: The flattened vector \(\textbf{F}\) is expanded along the time dimension (axis=1) to prepare the data for the GRU layer:
GRU layer: A GRU layer with 64 units processes the expanded vector:
where \(\textbf{H}_t\) is the hidden state at time step \(t\) , and \(\textbf{W}_G\) and \(\textbf{b}_G\) are the weights and biases of the GRU layer.
where \(\textbf{W}_O \in \mathbb {R}^{64 \times 2}\) and \(\textbf{b}_O \in \mathbb {R}^{2}\) .
Integrating a GRU network into the traditional CNN architecture gives the model a broader understanding of non-sequential data patterns and relationships. While the exact reduction in training and testing time will depend on factors like dataset size, complexity, and available hardware resources, this hybrid approach can potentially enhance the efficiency and accuracy of the image classification process. Combining the strengths of CNNs for spatial feature extraction and GRUs for temporal dependencies, this model offers a robust solution for image classification tasks with non-sequential data.
The reason for selecting GRUs in this model is their computational efficiency and ability to detect temporal dependencies within data. Compared to LSTMs, GRUs offer a more streamlined approach while still maintaining strong performance. In alignment with histopathology data, GRUs can work in conjunction with CNNs to recognize temporal patterns alongside spatial features. This makes them suitable for identifying complex relationships in histopathology images that require a nuanced understanding of temporal context.
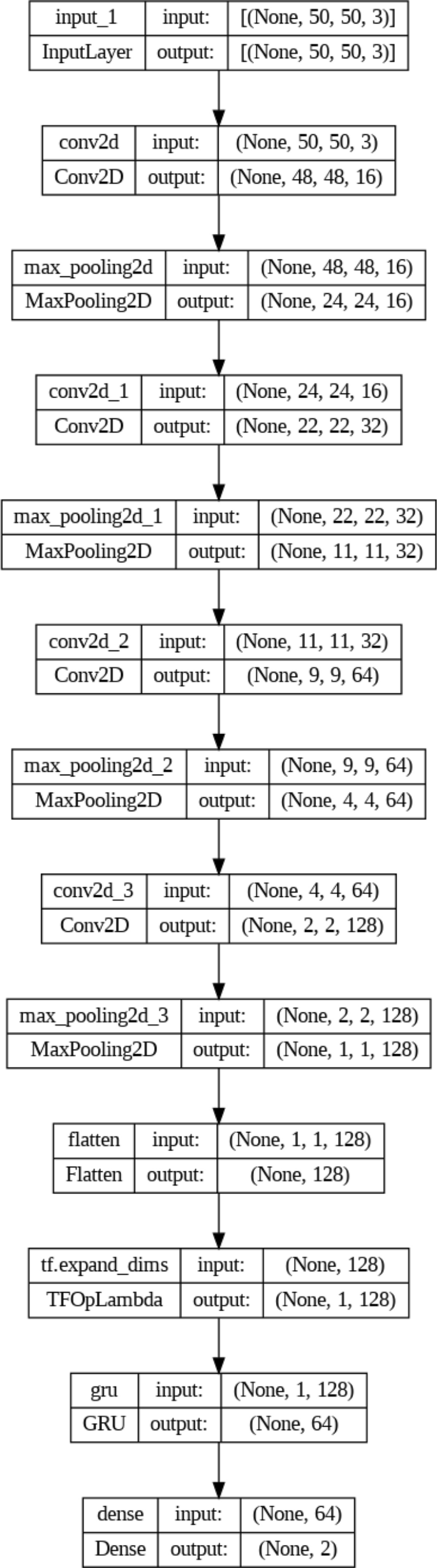
Hybrid CNN-GRU model
3.2.5 The hybrid CNN-SimpleRNN-LSTM-GRU model:
A hybrid model that combines CNNs with multiple RNN layers as in Fig. 13 , including SimpleRNN, LSTM, and GRU, presents a comprehensive and versatile approach to image classification, particularly when dealing with non-sequential data. While CNNs excel at extracting spatial features from images, RNNs are well suited to capture temporal and sequential dependencies within data. This hybrid model offers an extensive understanding of non-sequential data patterns and relationships, and it can significantly affect the efficiency of both training and testing processes.
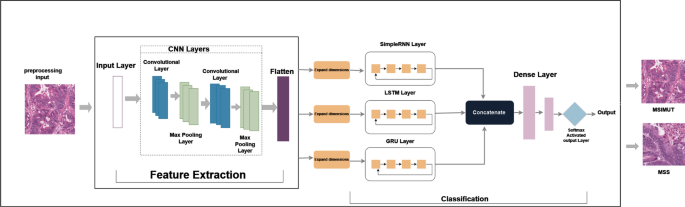
Hybrid CNN-SimpleRNN-LSTM-GRU architecture
The description of the hybrid model is presented in Fig. 14 and in the following points:
Input layer: The model processes input images with dimensions of (50, 50, 3), representing a \(50 \times 50\) pixel image with three color channels (RGB).
CNN layers: The model begins with four CNN layers. Each layer applies a 2D convolution operation followed by a max-pooling operation:
where \(\textbf{W}_1 \in \mathbb {R}^{3 \times 3 \times 3 \times 16}\) are the filters and \(\textbf{b}_1 \in \mathbb {R}^{16}\) is the bias.
Subsequent CNN and max-pooling layers follow a similar structure with increased filter numbers (32, 64, and 128).
Expanding time dimension: The flattened vector \(\textbf{F}\) is expanded along the time dimension (axis=1) to prepare the data for the SimpleRNN layer:
SimpleRNN layer: A SimpleRNN layer with 64 units processes the expanded vector:
where \(\textbf{H}^{\text {SimpleRNN}}_t\) is the hidden state at time step \(t\) , and \(\textbf{W}_{SR}\) and \(\textbf{b}_{SR}\) are the weights and biases of the SimpleRNN layer.
LSTM integration:
A separate sequence of the expanded data is input to an LSTM layer with 64 units:
where \(\textbf{H}^{\text {LSTM}}_t\) is the hidden state, \(\textbf{C}_{t-1}\) is the cell state at time step \(t-1\) , and \(\textbf{W}_{LSTM}\) and \(\textbf{b}_{LSTM}\) are the weights and biases of the LSTM layer.
Another sequence of the expanded data is input to a GRU layer with 64 units:
where \(\textbf{H}^{\text {GRU}}_t\) is the hidden state at time step \(t\) , and \(\textbf{W}_{GRU}\) and \(\textbf{b}_{GRU}\) are the weights and biases of the GRU layer.
Concatenation layer: The outputs of the SimpleRNN, LSTM, and GRU layers are concatenated:
Output layer: A dense output layer with 2 units and softmax activation for classification:
where \(\textbf{W}_O \in \mathbb {R}^{192 \times 2}\) and \(\textbf{b}_O \in \mathbb {R}^{2}\) .
Integrating SimpleRNN, LSTM, and GRU networks alongside the traditional CNN architecture allows the model to analyze non-sequential data patterns and relationships comprehensively. While the exact reduction in training and testing time will depend on factors like dataset size, complexity, and available hardware resources, this hybrid approach can significantly improve the efficiency and accuracy of the image classification process. Combining the strengths of CNNs for spatial feature extraction with multiple RNN architectures for temporal dependencies, this model offers a powerful solution for image classification tasks with non-sequential data.
The rationale for choosing this hybrid model stems from its capacity to blend the spatial feature extraction prowess of convolutional neural networks (CNNs) with the temporal feature extraction capabilities of SimpleRNN, LSTM, and GRU. This integration provides a robust framework that can accommodate diverse temporal and spatial features found in histopathology images, thereby enhancing the precision of the classification process. In terms of alignment with histopathology data, the hybrid model’s combined approach offers a comprehensive insight into both spatial and temporal patterns. Such a dual understanding facilitates a more accurate and adaptable classification of gastrointestinal (GI) cancer in histopathological images, offering a deeper and more nuanced interpretation of complex biological features.
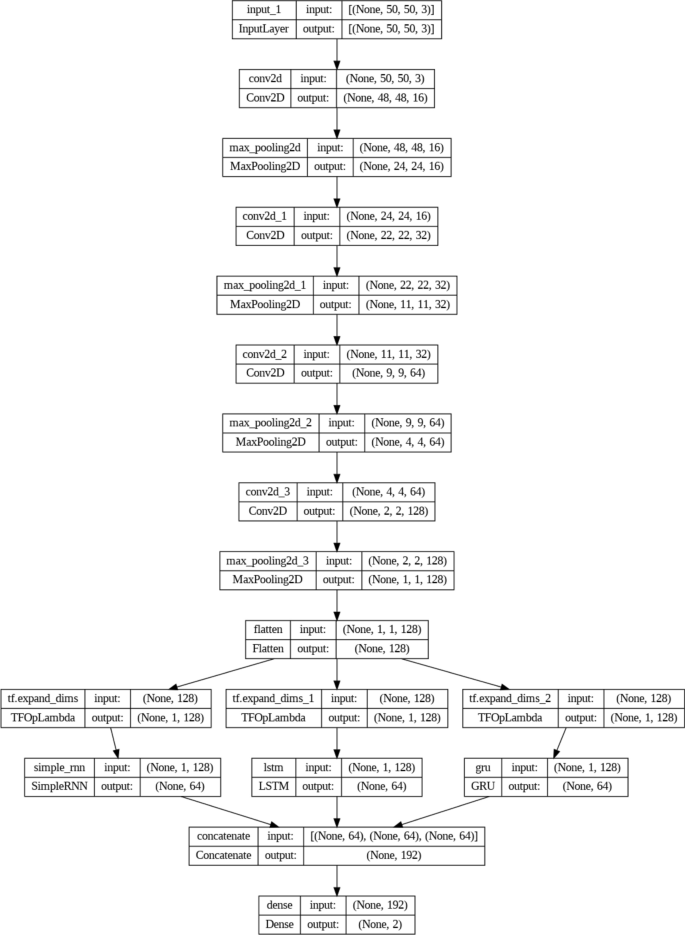
Hybrid CNN-SimpleRNN-LSTM-GRU model
4 Exploratory outcomes and analysis
This section presents the results of our proposed models and an evaluation of the datasets used for training and testing. The final results were obtained by averaging all the evaluation metrics, and we have also recorded the time taken for training and testing. To assess the performance of our models, we have included the datasets used in the Sect. 4.1 . We have also provided workplace characteristics in Sect. 4.2 and performance indicators in Sect. 4.3 . Finally, Sect. 4.4 presents a comparative analysis.
4.1 Dataset characterization
The proposed and state-of-the-art models are assessed in this paper using histopathology data. All slides of this data are available at. Footnote 1 It is accessible through Zenodo at Footnote 2 and also through Kaggle at. Footnote 3 The dataset, which includes 192312 histopathology images, is divided into two classes: 75039 MSIMUT images and 117273 MSS images, as demonstrated in Figs. 15 and 16 .
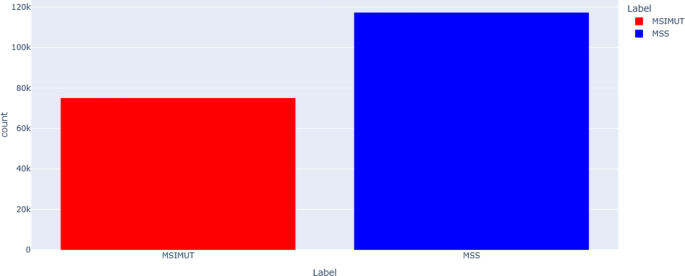
Dataset visualization
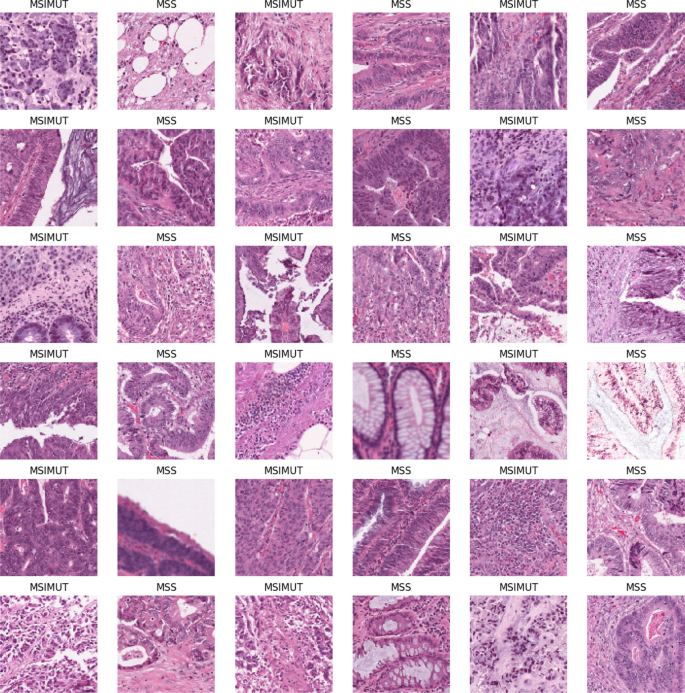
Visualization sample of the data
4.1.1 Data preprocessing
The data undergo a series of preprocessing steps, which include resizing, color transformation, and normalization, followed by a labeling process. These measures ensure that the data are in a suitable format for analysis or modeling.
Image preprocessing [ 60 , 61 , 61 , 62 ] refers to a set of techniques and operations that are applied to digital images to prepare them for further analysis, interpretation, or improvement in computer vision, image processing, and related fields. It is a crucial step that involves manipulating the image data to enhance its quality, extract relevant information, or make it more suitable for our problem. These preprocessing steps as the following:
The initial step in preparing images for machine learning is resizing [ 63 , 64 , 65 ]. This process involves adjusting the image dimensions to a standardized size, promoting consistency throughout the dataset. By doing so, machine learning models make the images more easily processed. Additionally, resizing facilitates a reduction in computational complexity and memory requirements. However, selecting a size that optimizes efficiency while preserving essential image details is crucial.
The second step, called Color Transformation [ 66 , 67 ], enables the conversion of images into a standardized color space, such as grayscale or RGB. This crucial step ensures consistency in color information across the dataset, facilitating models’ extraction of relevant features. Additionally, color transformation can enhance or simplify the visual information contained in the images.
In the third step, known as normalization [ 68 , 69 ], pixel values are scaled to a standardized range, usually between 0 and 1. This process effectively eliminates any variations in intensity and contrast between images. By doing so, the model becomes less susceptible to discrepancies in illumination and color, ultimately enhancing its ability to generalize across varying lighting conditions.
During the final phase of image processing, labeling is performed to allocate categorical or numerical labels to every image, as demonstrated by [ 70 , 71 ]. This step is pivotal for supervised machine learning endeavors since it facilitates the model’s ability to identify and associate visual characteristics with specific categories or results. Hence, precise labeling is essential for successful model training and assessment. In this paper, the data are labeled as 0 for MSIMUT and 1 for MSS and then shuffled and split as 80% training and 20% testing to be as in Table 1 .
Quality Assessment Measures:
Image quality: We conducted a rigorous evaluation of the quality of histopathology images before preprocessing [ 72 ]. This assessment included checks for factors such as image resolution, clarity, and the presence of artifacts or distortions that might affect model performance.
Consistency check: The consistency of the dataset was evaluated by ensuring uniform labeling and normalization across all images [ 73 , 74 ]. This step helps reduce bias and ensure the data’s robustness for deep learning analysis.
4.2 Working circumstances
The simulation has generated its results by utilizing powerful hardware, specifically an Intel Core i7 CPU, 64 GB of RAM, and an NVIDIA GTX 1050i GPU. Additionally, Python, Keras, Tensorflow, and Sklearn were utilized as programming tools to carry out the necessary programming tasks. Table 2 presents the recommended hyperparameters for the proposed models, while other standard parameter options, including the loss function and maximum number of epochs, are also available. The chosen optimizer for this task is Adam, and the loss function that was applied is shown in Table 2 .
4.3 Evaluation metrics
In the field of predictive modeling, it is essential to assess the effectiveness of the presented models. Assessment measures such as recall (RC), precision (PR), accuracy, and F1 score (F1) are commonly used to evaluate the performance of these models. These metrics are determined by the assessment parameters known as true positive (TP), true negative (TN), false positive (FP), and false negative (FN). Accurate assessment measures are vital in determining the effectiveness of predictive models, and they can help businesses and academics make important decisions based on these models. Therefore, it is crucial to understand the significance of these metrics and how they relate to the assessment of predictive models.
The mathematical definition of recall (RC), as outlined in [ 75 ], is established based on the ratio of true positives (TP) to the product of TP and false negatives (FN). This relationship is depicted in Eq. 17 .
To determine precision (PR), as described in [ 76 ], Eq. 18 is utilized. PR is calculated as TP divided by the product of TP and FP. This formula provides a quantitative measure of the accuracy of the results and helps assess the model’s effectiveness.
Equation 19 describes accuracy as follows:
The F1 score(F1) [ 77 ] measures a model’s accuracy by combining its PR and RC. It is calculated by multiplying the two values and dividing the result by their sum, and then multiplying by two. This formula is represented in Eq. 20 .
Specificity (SP) is expressed as in Eq.( 21 ):
Area under the curve (AUC) [ 78 , 79 ] is a widely used metric in the field of ML for evaluating the performance of binary classification models. It is derived from the ROC (Receiver Operating Characteristic) curve, which is a graphical representation of a model’s ability to distinguish between two classes as the classification threshold varies. The AUC value ranges from 0 to 1, with higher values indicating better model performance. An AUC of 0.5 suggests random guessing, while an AUC of 1 signifies a perfect model.
4.4 Comparative analysis
This paper proposes a framework for the early detection of GI cancer, which faces the challenge of processing a large amount of data. Various DL models were employed to achieve high accuracy and efficiency in the shortest possible time to address this issue. A CNN-based model was proposed, followed by a hybrid model that combined CNNs with each type of RNN (SimpleRNN, LSTM, and GRU). A fully integrated model, CNN-SimpleRNN-LSTM-GRU, was developed to meet the objective.
4.4.1 The findings of the suggested CNNs
This section presents the simulation results of our suggested CNNs. Our models underwent 100 epochs with a batch size of 300, as outlined in Table 2 . Figure 17 showcases the accuracy, loss curves, and confusion matrix of the CNNs. During the training phase, our suggested CNNs achieved an impressive accuracy of 98.53%. For the testing phase, we provide the evaluation metrics of the model in Table 3 , which includes accuracy, PR, RC, SP, F1, and AUC, all measured at 97.10%, 95.6%, 92.4%, 98.6%, 94.0%, and 0.955, respectively.
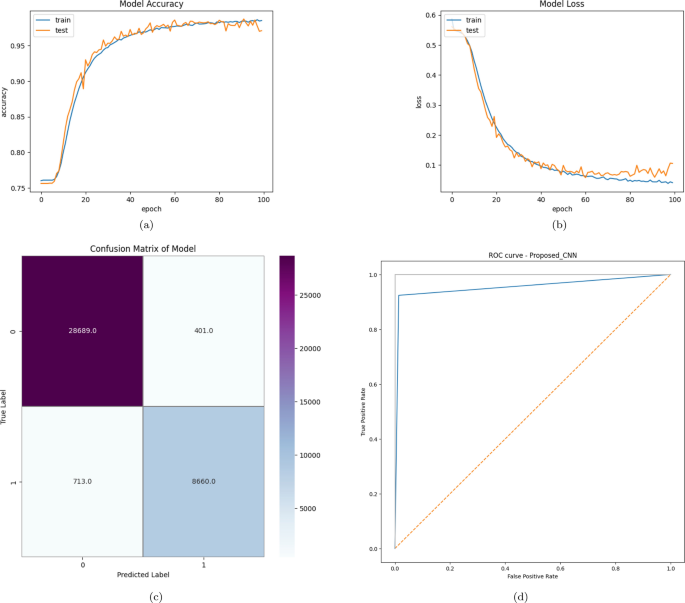
Graphical outputs of the suggested CNNs (a) accuracy curve of the suggested CNNs; (b) loss curve of the suggested CNNs model; (c) confusion matrix of the suggested CNNs model; and (d) ROC curve of the suggested CNNs model
4.4.2 The findings of the hybrid CNN-SimpleRNN model:
This section showcases the simulation results of the hybrid CNN-SimpleRNN model. Our model underwent training for 25 epochs with a batch size of 300, as outlined in Table 2 . In Fig. 18 , we present the model’s accuracy, loss curves, and confusion matrix. We are thrilled to announce that our hybrid model achieved a perfect 100.00% accuracy during training. Moving on to the testing phase, we evaluated the metrics of the CNN-SimpleRNN model, which we present in Table 4 . Our model delivered impressive results, with accuracy, PR, RC, SP, F1, and AUC of 99.74%, 99.9%, 99.0%, 100.0%, 99.5%, and 0.995, respectively.
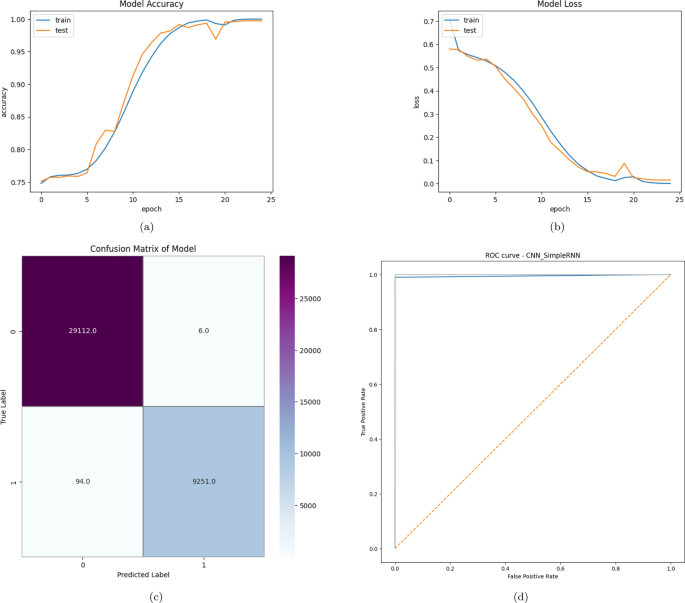
Graphical outputs of the hybrid CNN-SimpleRNN model (a) accuracy curve of CNN-SimpleRNN model; (b) loss curve of CNN-SimpleRNN model; (c) confusion matrix of CNN-SimpleRNN model; and (d) ROC curve of CNN-SimpleRNN
4.4.3 The findings of the hybrid CNN-LSTM model:
This section showcases the simulation results of the hybrid CNN-LSTM model. The model was trained using 65 epochs with a batch size of 350, as outlined in Table 2 . Figure 19 illustrates the hybrid’s accuracy, loss curves, and confusion matrix. During training, the CNN-LSTM model achieved an accuracy of 100.00%. In the testing stage, the evaluation metrics are presented in Table 5 , where the model achieved an accuracy, PR, RC, SP, F1, and AUC of 99.81%, 100.00%, 99.3%, 100.00%, 99.6%, and 0.996, respectively.
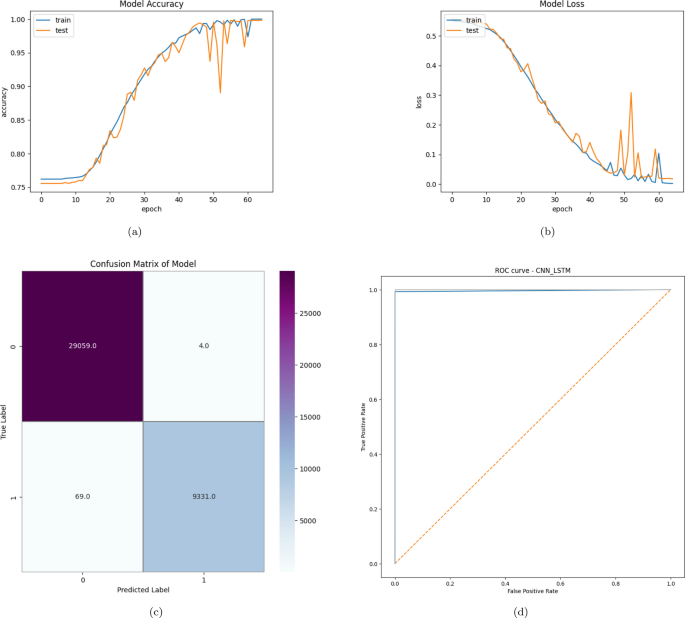
Graphical outputs of the hybrid CNN-LSTM model (a) accuracy curve of CNN-LSTM model; (b) loss curve of CNN-LSTM model; (c) confusion matrix of CNN-LSTM model, and (d) ROC curve of CNN-LSTM
4.4.4 The findings of the hybrid CNN-GRU model:
Here, we present the simulation results of the hybrid CNN-GRU model. The model was trained with 80 epochs using a batch size of 300, as detailed in Table 2 . Figure 20 showcases the hybrid model’s accuracy, loss curves, and confusion matrix. Notably, during training, the hybrid CNN-GRU model achieved an impressive accuracy of 99.75%. Moving onto the testing stage, we showcase the evaluation metrics of the hybrid CNN-GRU model in Table 6 . Impressively, the model achieved exceptional accuracy, PR, RC, SP, F1, and AUC of 99.70%, 99.8%, 99.0%, 99.9%, 99.4%, and 0.995, respectively.

Graphical outputs of the hybrid CNN-GRU model (a) accuracy curve of CNN-GRU model; (b) loss curve of CNN-GRU model; (c) confusion matrix of CNN-GRU model; and (d) ROC curve of CNN-GRU
4.4.5 The findings of the hybrid CNN-SimpleRNN-LSTM-GRU model
In this section, we present the simulation results of the hybrid CNN-SimpleRNN-LSTM-GRU model. Our model underwent 100 training epochs with a batch size of 350, as outlined in Table 2 . As depicted in Fig. 21 , the accuracy, loss curves, and confusion matrix are displayed for our hybrid model. During training, our hybrid model achieved a perfect accuracy of 100.00%. We evaluated our hybrid model using various metrics in Table 7 in the testing phase. Our model achieved impressive scores for accuracy, PR, RC, SP, F1, and AUC, with results of 99.90%, 99.9%, 99.6%, 100.00%, 99.8%, and 1.00, respectively.
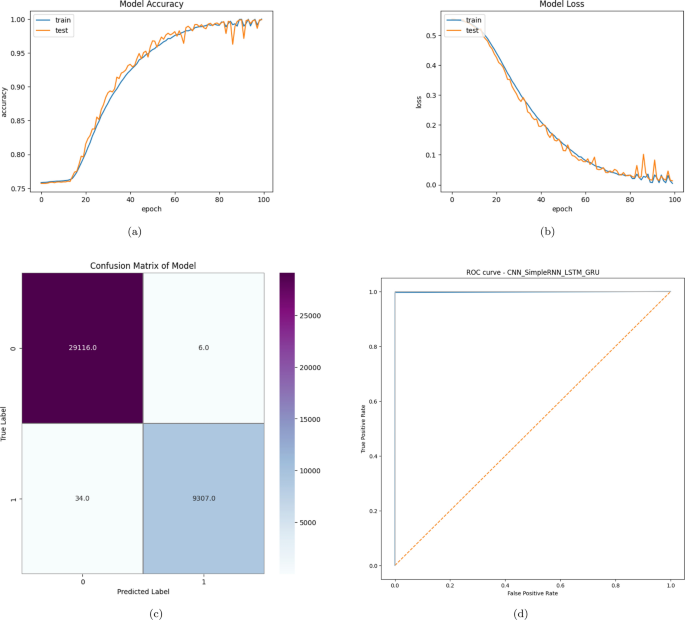
Graphical outputs of the hybrid CNN-SimpleRNN-LSTM-GRU model (a) accuracy curve of CNN-SimpleRNN-LSTM-GRU model; (b) loss curve of CNN-SimpleRNN-LSTM-GRU model; (c) confusion matrix of CNN-SimpleRNN-LSTM-GRU model; and (d) ROC curve of CNN-SimpleRNN-LSTM-GRU model
4.4.6 Results discussion
After careful analysis, it is observed that the CNNS-SimpleRNN-LSTM-GRU hybrid model is the top performer. It boasts outstanding real-time performance and outperforms all other models in nearly every metric. This model is a true all-rounder, ranking first in accuracy, RC, F1, SP, AUC, loss, and processing time, with a respectable second-place ranking in PR. The CNNs-LSTM hybrid is also a strong contender, taking first place in PR and SP and second place in accuracy, RC, F1, AUC, and processing time, with a third-place ranking in loss. The CNNs-SimpleRNN hybrid is a close third, ranking first in SP, second in PR and loss, and third in accuracy, RC, F1, and AUC, with a fourth-place ranking in processing time. The CNNs-GRU hybrid is also a worthy competitor, securing second place in SP and third place in PR, RC, AUC, and processing time, with a fourth-place ranking in accuracy, F1, and loss. Regrettably, the Suggested CNNs model did not meet the standard and ranked last in all measures (Table 8 ).
It has also been proved that the combination of CNN and RNN families has shown promising results, especially when considering time as a significant factor. Overcoming the challenge posed by time was a critical aspect of our approach, as we aimed to achieve the most optimal outcomes. The results obtained demonstrated the remarkable success of our methodology. It is confirmed more in Sect. 4.5 .
These findings have significant clinical implications. High accuracy and recall are crucial for correctly identifying cancerous patterns, reducing false negatives, and ensuring timely intervention. Specificity ensures that healthy tissues are not misclassified, minimizing unnecessary treatments. The high AUC values indicate robust model performance across different thresholds, enhancing reliability in clinical settings as the following:
High specificity and sensitivity: The model’s high specificity (SP of 100.0) ensures that it correctly identifies patients without the disease, reducing false positives. This minimizes unnecessary treatments and anxiety for patients. The high sensitivity (RC of 99.6) ensures that most cases of the disease are correctly identified, which is crucial for early detection and treatment.
Early detection: Due to its high accuracy and swift processing times, this hybrid model can be seamlessly integrated into clinical workflows to support the early detection of gastrointestinal cancer. Early diagnosis significantly improves patient outcomes by enabling timely intervention.
Reduction in diagnostic time: The model’s efficient processing times (training time of 8 min and testing time of 3 s) enables rapid analysis of patient data, offering quick diagnostic results. This efficiency is particularly valuable in clinical settings where prompt decision-making is essential.
Potential for personalized medicine: The model’s precise classification capabilities can aid in the development of personalized treatment plans. By accurately identifying MSI and MSS patterns, clinicians can customize treatment strategies based on the specific genetic and molecular characteristics of the tumor.
4.5 Comparison with the state-of-the-art models
According to the findings in Table 9 , the hybrid of CNNs-LSTM effectively improves precision measures. However, the hybrid of CNNs-SimpleRNN-LSTM-GRU surpasses the other proposed models in almost all measures, including processing time. Notably, this hybrid model also outperforms similar models using the same dataset. This can be attributed to its various advantages, which include:
Spatial and temporal features integration: A hybrid model incorporating CNN for spatial feature extraction and RNN layers (SimpleRNN, LSTM, and GRU) for sequential or temporal information processing allows for the integration of both spatial and temporal features in a single model. This can be crucial in tasks where both types of information are relevant, such as video analysis.
Efficient information capture: CNN layers excel at capturing spatial information from images, while RNN layers are designed to capture sequential patterns and dependencies. This combination enables the model to efficiently capture and exploit information across different dimensions in the data.
Sequential context handling: RNN layers (SimpleRNN, LSTM, and GRU) are well suited for tasks that require an understanding of sequential context, like recognizing patterns or temporal dependencies within image sequences, making them beneficial for various scientific applications.
Reduced overfitting: Including RNN layers can help mitigate overfitting, as they can capture long-range dependencies and smooth out noise in the data. This can result in more robust and generalized models, especially in cases where data are limited.
Adaptive model learning: The dynamic nature of RNN layers, such as LSTM and GRU, allows the model to adapt to varying sequences or temporal patterns, enhancing the model’s ability to handle different types of input data.
Contextual image understanding: A hybrid model can better understand the contextual relationships within images for scientific image analysis. It can capture not only individual object features but also how they relate to each other over time or across different image regions.
Complex pattern recognition: The combination of CNN for feature extraction and RNN for sequential analysis is powerful for recognizing complex image patterns. This can be valuable in scientific domains where identifying intricate structures or phenomena is crucial.
Interpretability and explainability: Hybrid models are often more interpretable as they allow you to analyze spatial and temporal features separately. This can be advantageous in scientific research, where understanding the model’s decision-making process is essential.
Customization for specific tasks: The hybrid architecture can be customized to suit the unique requirements of your image classification task, making it highly adaptable and efficient for scientific applications that may have specialized needs.
Scientific insights: By combining these neural network components in a hybrid model, you may gain scientific insights into the interactions between spatial and temporal information in your data, contributing to a deeper understanding of the underlying phenomena.
In addition to the advantages mentioned earlier, a hybrid CNN-SimpleRNN-LSTM-GRU model proved its efficiency in decreasing processing time because of the following:
Parallel processing: CNN layers are well suited for parallel processing, as they can simultaneously analyze different regions of an image. This parallelism can lead to faster feature extraction compared to sequential processing.
Reduced computation: RNN layers, especially LSTM and GRU, are computationally more efficient than fully connected layers. By incorporating these layers, you can reduce the overall computational burden, leading to faster inference times.
Hierarchical feature extraction: The combination of CNN for spatial feature extraction and RNN for temporal processing enables a hierarchical approach, where spatial features are extracted first, and then, the sequential analysis is performed. This can lead to more efficient utilization of computational resources.
Early exit mechanisms: Hybrid models can be designed with early exit mechanisms, allowing the model to make predictions at intermediate stages. This can be useful in real-time applications, where quick decisions based on partial information may be needed, saving processing time.
Reduced model complexity: A well-designed hybrid model can be more compact than a standalone deep CNN or RNN. Reduced model complexity leads to faster training and inference times, making it suitable for applications with strict time constraints.
Efficient memory usage: RNN layers, especially GRU, have a more efficient memory management mechanism than standard RNNs. This can help reduce memory consumption, which is crucial for processing large images or long sequences.
Optimized hyperparameters: The flexibility of the hybrid architecture allows you to optimize hyperparameters for faster training and inference. To balance accuracy and speed, you can experiment with layer sizes, batch sizes, and learning rates.
Effective utilization of hardware: Modern hardware, such as GPUs and TPUs, is designed to handle various operations efficiently. By combining CNN and RNN layers, you can better utilize the capabilities of these hardware accelerators for faster computations.
Model pruning: Hybrid models can be pruned to remove unnecessary connections or layers, resulting in a more streamlined model. Pruned models are faster to execute, which is essential for real-time applications.
Streaming data processing: In scenarios where you need to process streaming or real-time data, hybrid models can be more responsive due to their efficient spatial and temporal processing combination, enabling faster decision-making.
The findings in Table 9 also corroborate the clinical relevance and effectiveness of hybrid deep learning models, emphasizing their significant role in medical diagnostics as the following:
Integration of spatial and temporal features: Studies by Yamashita et al. [ 24 ] and Kather et al. [ 22 ] demonstrate the enhanced model performance when combining spatial features captured by CNNs with temporal features processed by RNNs, consistent with our results.
Improved performance metrics: Our high-performance metrics align with the results reported by Chen et al. [ 23 ] and Qiu et al. [ 32 ], indicating that hybrid models surpass traditional methods in accuracy and diagnostic capability.
Clinical relevance: Research conducted by Khan et al. [ 28 ] and Lee et al. [ 29 ] underscores the clinical importance of deep learning models in cancer diagnosis. Our study reinforces these findings, highlighting the practical utility of a hybrid CNN-SimpleRNN-LSTM-GRU model in clinical environments.
Efficiency and processing time: The efficient processing times we observe align with findings from Zhu et al. [ 30 ].
5 Conclusion
Our investigation into the early detection of GI cancer through The integration of histopathology data, coupled with The application of five distinct DL models, has yielded valuable insights. Notably, our findings emphasize the pivotal role of MSI and MSS in refining diagnostic accuracy. Among the models evaluated, the CNN-SimpleRNN-LSTM-GRU architecture emerged as the frontrunner, excelling across critical metrics such as accuracy, RC, SP, F1, and AUC. This model’s nuanced integration of recurrent layers showcases its efficacy in capturing temporal dependencies within the histopathology data, thus enhancing its discriminatory power in distinguishing between MSI and MSS cases. However, it is imperative to recognize each model’s inherent advantages and limitations. While the CNN-LSTM demonstrated commendable PR, the computational demands of more intricate architectures warrant careful consideration, especially in resource-constrained settings. Furthermore, the varying performance across models underscores the importance of tailoring diagnostic approaches to specific clinical requirements, considering the distinct characteristics of MSI and MSS patterns. Future research endeavors could delve deeper into unraveling the intricate molecular signatures associated with MSI and MSS, refining the specificity of diagnostic models. Exploring the transferability of our findings across diverse patient cohorts and datasets would contribute to the robustness of the proposed diagnostic framework. Additionally, investigations into the interpretability of these DL models concerning the nuanced histopathological features linked to MSI and MSS could facilitate greater clinical acceptance. In conclusion, our study not only underscores the promising potential of DL in early GI cancer detection but also highlights the critical role of MSI and MSS stratification in enhancing diagnostic precision. The ongoing pursuit of optimized models, informed by a comprehensive understanding of molecular intricacies, is essential for advancing the field and realizing the full clinical impact of AI-driven diagnostics in oncology.
Data availability
Data are available on request from the authors.
https://portal.gdc.cancer.gov .
http://doi.org/10.5281/zenodo.2530835 .
https://www.kaggle.com/datasets/joangibert/tcga_coad_msi_mss_jpg .
Abbreviations
Deep learning
Recurrent neural networks
Long short-term memory
Microsatellite instability
The Cancer Genome Atlas
Gastric cancer
Whole slide images
Artificial neural networks
Support vector machines
Dual-layer computed tomography
Deep neural network
True positive
False positive
Backpropagation through time
Specificity
Machine learning
Convolutional neural networks
Gated recurrent unit
Microsatellite stability
Gastrointestinal
Colorectal cancer
Mismatch repair
Computer tomography
Net present value
Swarm learning
Rectified linear unit
True negative
False negative
Area under the curve
Xie Y, Shi L, He X, Luo Y (2021) Gastrointestinal cancers in China, the USA, and Europe. Gastroenterol Rep 9(2):91–104
Article Google Scholar
Ağagündüz D, Cocozza E, Cemali Ö, Bayazıt AD, Nanì MF, Cerqua I, Morgillo F, Saygılı SK, Berni Canani R, Amero P et al (2023) Understanding the role of the gut microbiome in gastrointestinal cancer: a review. Front Pharmacol 14:1130562
Wang J, Xiu J, Farrell A, Baca Y, Arai H, Battaglin F, Kawanishi N, Soni S, Zhang W, Millstein J et al (2023) Mutational analysis of microsatellite-stable gastrointestinal cancer with high tumour mutational burden: a retrospective cohort study. Lancet Oncol 24(2):151–161
Yamashita K, Arimura Y, Kurokawa S, Itoh F, Endo T, Hirata K, Imamura A, Kondo M, Sato T, Imai K (2000) Microsatellite instability in patients with multiple primary cancers of the gastrointestinal tract. Gut 46(6):790–794
Yuza K, Nagahashi M, Watanabe S, Takabe K, Wakai T (2017) Hypermutation and microsatellite instability in gastrointestinal cancers. Oncotarget 8(67):112103
Shinozaki-Ushiku A, Kunita A, Iwasaki A, Kato M, Yamazawa S, Abe H, Ushiku T (2023) Microsatellite instability profiles of gastrointestinal cancers: comparison between non-colorectal and colorectal origin. Histopathology 82(3):466–477
Marrelli D, Polom K, Pascale V, Vindigni C, Piagnerelli R, De Franco L, Ferrara F, Roviello G, Garosi L, Petrioli R et al (2016) Strong prognostic value of microsatellite instability in intestinal type non-cardia gastric cancer. Ann Surg Oncol 23:943–950
Zito Marino F, Amato M, Ronchi A, Panarese I, Ferraraccio F, De Vita F, Tirino G, Martinelli E, Troiani T, Facchini G et al (2022) Microsatellite status detection in gastrointestinal cancers: PCR/NGS is mandatory in negative/patchy MMR immunohistochemistry. Cancers 14(9):2204
Villalobos P, Wistuba II (2017) Lung cancer biomarkers, hematology/oncology. Clinics 31(1):13–29
Google Scholar
Echle A, Rindtorff NT, Brinker TJ, Luedde T, Pearson AT, Kather JN (2021) Deep learning in cancer pathology: a new generation of clinical biomarkers. Br J Cancer 124(4):686–696
Khan MA, Sahar N, Khan WZ, Alhaisoni M, Tariq U, Zayyan MH, Kim YJ, Chang B (2022) Gestronet: A framework of saliency estimation and optimal deep learning features based gastrointestinal diseases detection and classification. Diagnostics 12(11):2718
Kim SY, Kim TW (2020) Current challenges in the implementation of precision oncology for the management of metastatic colorectal cancer. ESMO Open 5(2):e000634
Magalhães G, Calisto R, Freire C, Silva R, Montezuma D, Canberk S, Schmitt F (2024) Invisible for a few but essential for many: the role of histotechnologists in the establishment of digital pathology. J Histotechnol 47(1):39–52
Ben-Aharon I, van Laarhoven HW, Fontana E, Obermannova R, Nilsson M, Lordick F (2023) Early-onset cancer in the gastrointestinal tract is on the rise-evidence and implications. Cancer Discov 13(3):538–551
Ahmed IA, Senan EM, Shatnawi HSA (2023) Hybrid models for endoscopy image analysis for early detection of gastrointestinal diseases based on fused features. Diagnostics 13(10):1758
Sahiner B, Pezeshk A, Hadjiiski LM, Wang X, Drukker K, Cha KH, Summers RM, Giger ML (2019) Deep learning in medical imaging and radiation therapy. Med Phys 46(1):e1–e36
Shafi S, Parwani AV (2023) Artificial intelligence in diagnostic pathology. Diagn Pathol 18(1):109
Jiang Y, Yang M, Wang S, Li X, Sun Y (2020) Emerging role of deep learning-based artificial intelligence in tumor pathology. Cancer Commun 40(4):154–166
Huss R, Raffler J, Märkl B (2023) Artificial intelligence and digital biomarker in precision pathology guiding immune therapy selection and precision oncology. Cancer Rep 6:e1796
Min JK, Kwak MS, Cha JM (2019) Overview of deep learning in gastrointestinal endoscopy. Gut Liver 13(4):388
Jaspers TJ, Boers, TG, Kusters CH, Jong MR, Jukema JB, de Groof AJ, Bergman JJ, de With PH, van der Sommen F (2023) Investigating the impact of image quality on endoscopic ai model performance. In: International workshop on applications of medical AI, Springer, pp 32–41
Kather JN, Pearson AT, Halama N, Jäger D, Krause J, Loosen SH, Marx A, Boor P, Tacke F, Neumann UP et al (2019) Deep learning can predict microsatellite instability directly from histology in gastrointestinal cancer. Nat Med 25(7):1054–1056
Chen T, Zhang C, Liu Y, Zhao Y, Lin D, Hu Y, Yu J, Li G (2019) A gastric cancer LncRNAs model for MSI and survival prediction based on support vector machine. BMC Genomics 20(1):1–7
Yamashita R, Long J, Longacre T, Peng L, Berry G, Martin B, Higgins J, Rubin DL, Shen J (2021) Deep learning model for the prediction of microsatellite instability in colorectal cancer: a diagnostic study. Lancet Oncol 22(1):132–141
Cao R, Yang F, Ma S-C, Liu L, Zhao Y, Li Y, Wu D-H, Wang T, Lu W-J, Cai W-J et al (2020) Development and interpretation of a pathomics-based model for the prediction of microsatellite instability in colorectal cancer. Theranostics 10(24):11080
Zhang Z, Peng J (2023) Clinical nursing and postoperative prediction of gastrointestinal cancer based on CT deep learning model. J Radiat Res Appl Sci 16(2):100561
Padmavathi P, Harikiran J (2023) Se-resnet: A novel method for gastrointestinal (GI) diseases classification from wireless capsule endoscopy (WCE) images. Traitement du Signal 40(4)
Khan Z, Loganathan R (2022) Transfer Learning Based Classification of MSI and MSS Gastrointestinal Cancer. EasyChair
Lee SH, Lee Y, Jang H-J (2023) Deep learning captures selective features for discrimination of microsatellite instability from pathologic tissue slides of gastric cancer. Int J Cancer 152(2):298–307
Zhu Y, Wang P, Wang B, Jiang Z, Li Y, Jiang J, Zhong Y, Xue L, Jiang L (2023) Dual-layer spectral-detector CT for predicting microsatellite instability status and prognosis in locally advanced gastric cancer. Insights Imaging 14(1):151
Saldanha OL, Muti HS, Grabsch HI, Langer R, Dislich B, Kohlruss M, Keller G, van Treeck M, Hewitt KJ, Kolbinger FR et al (2023) Direct prediction of genetic aberrations from pathology images in gastric cancer with swarm learning. Gastric Cancer 26(2):264–274
Qiu W, Yang J, Wang B, Yang M, Tian G, Wang P, Yang J (2022) Evaluating the microsatellite instability of colorectal cancer based on multimodal deep learning integrating histopathological and molecular data. Front Oncol 12:925079
Yu S-N, Huang S-C, Wang W-C, Chang YP, Chen K-H, Chen T-C (2023) Prediction of microsatellite instability from gastric histological images based on residual attention networks with non-local modules, IEEE Access
Yin H, Vahdat A, Alvarez JM, Mallya A, Kautz J, Molchanov P (2022) A-vit: adaptive tokens for efficient vision transformer. In: Proceedings of the IEEE/CVF conference on computer vision and pattern recognition, pp 10809–10818
Ren K, Hong G, Chen X, Wang Z (2023) A covid-19 medical image classification algorithm based on transformer. Sci Rep 13(1):5359
Azad R, Kazerouni A, Heidari M, Aghdam EK, Molaei A, Jia Y, Jose A, Roy R, Merhof D (2023) Advances in medical image analysis with vision transformers: a comprehensive review. Med Image Anal 91:103000
Meng L, Li H, Chen B-C, Lan S, Wu Z, Jiang Y-G, Lim S-N (2022) Adavit: adaptive vision transformers for efficient image recognition. In: Proceedings of the IEEE/CVF conference on computer vision and pattern recognition, pp 12309–12318
Yin H, Vahdat A, Alvarez J, Mallya A, Kautz J, Molchanov P (2021) Adavit: adaptive tokens for efficient vision transformer, arXiv preprint arXiv:2112.07658
Chen C-FR, Fan Q, Panda R (2021) CrossViT: Cross-attention multi-scale vision transformer for image classification, in: Proceedings of the IEEE/CVF international conference on computer vision, pp 357–366
Xu H, Zheng T, Liu Y, Zhang Z, Xue C, Li J (2024) A joint convolutional cross vit network for hyperspectral and light detection and ranging fusion classification. Remote Sens 16(3):489
Zheng Y, Jiang W (2022) Evaluation of vision transformers for traffic sign classification. Wirel Commun Mob Comput 2022(1):3041117
Tummala S, Kadry S, Bukhari SAC, Rauf HT (2022) Classification of brain tumor from magnetic resonance imaging using vision transformers ensembling. Curr Oncol 29(10):7498–7511
Chauhan R, Ghanshala KK, Joshi R (2018) Convolutional neural network (CNN) for image detection and recognition. In: First international conference on secure cyber computing and communication (ICSCCC). IEEE, pp 278–282
Albawi S, Mohammed TA, Al-Zawi S (2017) Understanding of a convolutional neural network. In: International conference on engineering and technology (ICET). IEEE, pp 1–6
Yamashita R, Nishio M, Do RKG, Togashi K (2018) Convolutional neural networks: an overview and application in radiology. Insights Imaging 9:611–629
O’Shea K, Nash R (2015) An introduction to convolutional neural networks, arXiv preprint arXiv:1511.08458
Dhruv P, Naskar S (2019) Image classification using convolutional neural network (CNN) and recurrent neural network (RNN): a review. Machine Learning Inf Processing Proc ICMLIP 2020:367–381
Grossberg S (2013) Recurrent neural networks. Scholarpedia 8(2):1888
Medsker LR, Jain L (2001) Recurrent neural networks. Design Appl 5(64–67):2
Salehinejad H, Sankar S, Barfett J, Colak E, Valaee S (2017) Recent advances in recurrent neural networks, arXiv preprint arXiv:1801.01078
Ma Y, Principe JC (2019) A taxonomy for neural memory networks. IEEE Trans Neural Netw Learn Syst 31(6):1780–1793
Article MathSciNet Google Scholar
Kag A, Saligrama V (2021) Time adaptive recurrent neural network. In: Proceedings of the IEEE/CVF conference on computer vision and pattern recognition, pp 15149–15158
Yu Y, Si X, Hu C, Zhang J (2019) A review of recurrent neural networks: LSTM cells and network architectures. Neural Comput 31(7):1235–1270
Staudemeyer RC, Morris ER (2019) Understanding LST–a tutorial into long short-term memory recurrent neural networks, arXiv preprint arXiv:1909.09586
Smagulova K, James AP (2019) A survey on lSTM memristive neural network architectures and applications. Eur Phy J Spec Top 228(10):2313–2324
Sherstinsky A (2020) Fundamentals of recurrent neural network (RNN) and long short-term memory (ISTM) network. Phys D 404:132306
Dey R, Salem FM (2017) Gate-variants of gated recurrent unit (GRU) neural networks. In: IEEE 60th international Midwest symposium on circuits and systems (MWSCAS). IEEE, pp 1597–1600
Shewalkar A, Nyavanandi D, Ludwig SA (2019) Performance evaluation of deep neural networks applied to speech recognition: RNN, LSTM and GRU. J Artif Intell Soft Comput Res 9(4):235–245
Fu R, Zhang Z, Li L (2016) Using LSTM and GRU neural network methods for traffic flow prediction. In: 31st Youth academic annual conference of Chinese association of automation (YAC). IEEE, pp 324–328
Awcock GJ, Thomas R (1995) Applied image processing. Springer
Petrou MM, Petrou C (2010) Image processing: the fundamentals. John Wiley & Sons
Huang TS, Schreiber WF, Tretiak OJ (1971) Image processing. Proc IEEE 59(11):1586–1609
Sullivan BJ, Ansari R, Giger ML, MacMahon H (1995) Effects of image preprocessing/resizing on diagnostic quality of compressed medical images [chest radiographs application]. In: Proceedings., international conference on image processing, Vol 2, IEEE, pp 13–16
Perumal S, Velmurugan T (2018) Preprocessing by contrast enhancement techniques for medical images. Int J Pure Appl Math 118(18):3681–3688
Brisinello M, Grbić R, Pul M, Anđelić T (2017) Improving optical character recognition performance for low quality images. In: International symposium ELMAR. IEEE, pp 167–171
Förstner W (2003) Image preprocessing for feature extraction in digital intensity, color and range images, in: Geomatic method for the analysis of data in the earth sciences, Springer, pp 165–189
Khirade SD, Patil A (2015) Plant disease detection using image processing. In: International conference on computing communication control and automation. IEEE, pp 768–771
Sharif M, Mohsin S, Jamal MJ, Raza M (2010) Illumination normalization preprocessing for face recognition. In: The 2nd conference on environmental science and information application technology, vol 2. IEEE, pp 44–47
Meier J, Bock R, Michelson G, Nyúl LG, Hornegger J (2007) Effects of preprocessing eye fundus images on appearance based glaucoma classification. In: Computer analysis of images and patterns: 12th international conference, CAIP 2007, Vienna, Austria, August 27–29, 2007. Proceedings 12, Springer, pp 165–172
Caseneuve G, Valova I, LeBlanc N, Thibodeau M (2021) Chest X-ray image preprocessing for disease classification. Procedia Comput Sci 192:658–665
Pérez-García F, Sparks R, Ourselin S (2021) Torchio: a python library for efficient loading, preprocessing, augmentation and patch-based sampling of medical images in deep learning. Comput Methods Programs Biomed 208:106236
Shiranita K, Hayashi K, Otsubo A, Miyajima T, Takiyama R (2000) Grading meat quality by image processing. Pattern Recogn 33(1):97–104
Amato G, Falchi F, Gennaro C (2011) Geometric consistency checks for kNN based image classification relying on local features. In: Proceedings of the fourth international conference on similarity search and applications, pp 81–88
S. Sergyán, L. Csink (2005) Consistency check of image databases. In: Proceedings of 2nd Romanian-Hungarian joint symposium on applied computational intelligence, Timisoara, Romania, Citeseer, pp 201–206
Amigó E, Gonzalo J, Artiles J, Verdejo F (2009) A comparison of extrinsic clustering evaluation metrics based on formal constraints. Inf Retr 12:461–486
De Medeiros AKA, Guzzo A, Greco G, Van der Aalst WM, Weijters A, Van Dongen BF, Saccà D (2008) Process mining based on clustering: a quest for precision. In: Business process management workshops: BPM 2007 international workshops, BPI, BPD, CBP, ProHealth, RefMod, semantics4ws, Brisbane, Australia, September 24, 2007, Revised Selected Papers 5, Springer, pp 17–29
Amigó E, Gonzalo J, Artiles J, Verdejo F (2011) Combining evaluation metrics via the unanimous improvement ratio and its application to clustering tasks. J Artif Intell Res 42:689–718
MathSciNet Google Scholar
Lobo JM, Jiménez-Valverde A, Real R (2008) AUC: a misleading measure of the performance of predictive distribution models. Glob Ecol Biogeogr 17(2):145–151
Huang J, Ling CX (2005) Using AUC and accuracy in evaluating learning algorithms. IEEE Trans Knowl Data Eng 17(3):299–310
Download references
Open access funding provided by The Science, Technology & Innovation Funding Authority (STDF) in cooperation with The Egyptian Knowledge Bank (EKB).
Author information
Authors and affiliations.
Faculty of Computers and Artificial Intelligence, Helwan University, Helwan, Egypt
Abeer A. Wafa
Department of Information System, Higher Institute for Management and Information Technology, Kafr El-Sheikh, Egypt
Reham M. Essa
Faculty of Computers and Information, Kafrelsheikh University, Kafr El-Sheikh, Egypt
Amr A. Abohany
Faculty of Specific Education, Mansoura University, Mansoura, Egypt
Hanan E. Abdelkader
You can also search for this author in PubMed Google Scholar
Corresponding author
Correspondence to Amr A. Abohany .
Ethics declarations
Conflict of interest.
All the authors declare that they have no conflict of interest.
Additional information
Publisher's note.
Springer Nature remains neutral with regard to jurisdictional claims in published maps and institutional affiliations.
Rights and permissions
Open Access This article is licensed under a Creative Commons Attribution 4.0 International License, which permits use, sharing, adaptation, distribution and reproduction in any medium or format, as long as you give appropriate credit to the original author(s) and the source, provide a link to the Creative Commons licence, and indicate if changes were made. The images or other third party material in this article are included in the article's Creative Commons licence, unless indicated otherwise in a credit line to the material. If material is not included in the article's Creative Commons licence and your intended use is not permitted by statutory regulation or exceeds the permitted use, you will need to obtain permission directly from the copyright holder. To view a copy of this licence, visit http://creativecommons.org/licenses/by/4.0/ .
Reprints and permissions
About this article
Wafa, A.A., Essa, R.M., Abohany, A.A. et al. Integrating deep learning for accurate gastrointestinal cancer classification: a comprehensive analysis of MSI and MSS patterns using histopathology data. Neural Comput & Applic (2024). https://doi.org/10.1007/s00521-024-10287-y
Download citation
Received : 21 March 2024
Accepted : 29 July 2024
Published : 26 August 2024
DOI : https://doi.org/10.1007/s00521-024-10287-y
Share this article
Anyone you share the following link with will be able to read this content:
Sorry, a shareable link is not currently available for this article.
Provided by the Springer Nature SharedIt content-sharing initiative
- Gastrointestinal (GI)
- Convolutional neural networks (CNNs)
- CNNs-SimpleRNN
- CNNs-SimpleRNN-LSTM-GRU
- Find a journal
- Publish with us
- Track your research

IMAGES
COMMENTS
Literature Reviews that are organized methodologically consist of paragraphs/sections that are based on the methods used in the literature found.This approach is most appropriate when you are using new methods on a research question that has already been explored.Since literature review structures are not mutually exclusive, you can organize the use of these methods in chronological order.
Examples of literature reviews. Step 1 - Search for relevant literature. Step 2 - Evaluate and select sources. Step 3 - Identify themes, debates, and gaps. Step 4 - Outline your literature review's structure. Step 5 - Write your literature review.
A literature review is an overview of selected articles, books and other sources about a specific subject. The purpose is to summarize the existing research that has been done on the subject in order to put your research in context and to highlight what your research will add to the existing body of knowledge.
A literature review is a critical analysis and synthesis of existing research on a particular topic. It provides an overview of the current state of knowledge, identifies gaps, and highlights key findings in the literature. 1 The purpose of a literature review is to situate your own research within the context of existing scholarship ...
As mentioned previously, there are a number of existing guidelines for literature reviews. Depending on the methodology needed to achieve the purpose of the review, all types can be helpful and appropriate to reach a specific goal (for examples, please see Table 1).These approaches can be qualitative, quantitative, or have a mixed design depending on the phase of the review.
A literature review is a comprehensive summary of previous research on a topic. The literature review surveys scholarly articles, books, and other sources relevant to a particular area of research. The review should enumerate, describe, summarize, objectively evaluate and clarify this previous research. It should give a theoretical base for the ...
Literature Review is a comprehensive survey of the works published in a particular field of study or line of research, usually over a specific period of time, in the form of an in-depth, critical bibliographic essay or annotated list in which attention is drawn to the most significant works.. Also, we can define a literature review as the collected body of scholarly works related to a topic:
The first element we discuss is a review of research (literature reviews), which highlights the need for a specific research question, study problem, or topic of investigation. ... comprehensive, and critical literature review reveals the understanding of the topic that the researcher brings to the study. Literature reviews should not be so big ...
What is a literature review? Definition: A literature review is a systematic examination and synthesis of existing scholarly research on a specific topic or subject. Purpose: It serves to provide a comprehensive overview of the current state of knowledge within a particular field. Analysis: Involves critically evaluating and summarizing key findings, methodologies, and debates found in ...
This is the most comprehensive and user-friendly book I've seen on how to conduct a literature review. The authors take the distinction of qualitative, quantitative, and mixed methods research seriously, showing how each adds something important and how being open-minded results in the use of literature based on all three approaches.
A comprehensive and a critical literature review can create a solid foundation for advancing knowledge by identifying the current status of the research, existing research gaps and where future ...
Maria Watson is a PhD candidate in the Urban and Regional Science program at Texas A&M University. Her research interests include disaster recovery, public policy, and economic development. Literature reviews establish the foundation of academic inquires. However, in the planning field, we lack rigorous systematic reviews.
Writing a literature review requires a range of skills to gather, sort, evaluate and summarise peer-reviewed published data into a relevant and informative unbiased narrative. Digital access to research papers, academic texts, review articles, reference databases and public data sets are all sources of information that are available to enrich ...
Types of Literature Review are as follows: Narrative literature review: This type of review involves a comprehensive summary and critical analysis of the available literature on a particular topic or research question. It is often used as an introductory section of a research paper. Systematic literature review: This is a rigorous and ...
A literature review is probably the most common academic writing activity that is performed by scholars and graduate students. Imel [] identified a literature review as being either part of a larger study or as a research effort on its own.As a part of a larger study, Imel [] identified the literature is "the foundation for the study."It has been suggested that the literature review for a ...
A literature review provides a comprehensive overview of existing knowledge on a particular topic, which is quintessential to any research project. Researchers employ various literature reviews based on their research goals and methodologies. ... Literature review in research serves several key purposes, including: Background of the study ...
Literature Review and Research Design by Dave Harris This book looks at literature review in the process of research design, and how to develop a research practice that will build skills in reading and writing about research literature--skills that remain valuable in both academic and professional careers. Literature review is approached as a process of engaging with the discourse of scholarly ...
Comprehensive Literature Reviews: Involve supplementing electronic searches with a review of references in identified literature, manual searches of references and journals, and consulting experts for both unpublished and published studies and reports. Reporting Standards: Checking for Research Writing and Reviewing.
A literature review is an assessment of a body of research that addresses a particular topic or research question. It aims to review the critical points of current knowledge, as well as theoretical and methodological contributions to a particular topic. Purpose of a Literature Review: it is a building block for your thesis or dissertation
Researchers approach literature review themes by monitoring the development of research interests, identifying ongoing changes resulting from significant field advances, and investigating new ...
For this review, a comprehensive literature search was performed from many databases using generic search terms such as Qualitative Research, Criteria, etc. The following databases were chosen for the literature search based on the high number of results: IEEE Explore, ScienceDirect, PubMed, Google Scholar, and Web of Science.
A Comprehensive Literature Review of Borderline Personality Disorder: Unraveling Complexity From Diagnosis to Treatment. Monitoring Editor: Alexander Muacevic and John R Adler. ... Research has discovered that Schema therapy (ST) successfully treats BPD . However, very little is known about how treatment works for people with BPD, mainly which ...
Literature reviews provide readers with syntheses and analyses of research in specific subject areas (Cooper, 1998).In this study, a selection and analysis of papers with the topic of trust in megaprojects was conducted in four phases (Fig. 1.), following the established PRISMA Statement methodology (Moher et al., 2010).PRISMA is an abbreviation for "preferred reporting items for systematic ...
In a literature review of the research works from 2007 to 2018, Gawke et al. (2019) highlighted three approaches to the conceptualization of intrapreneurship. For Gawke, three types of definition of intrapreneurship can be distinguished. The first way refers to entrepreneurial orientation.
Originality/Value: Therefore, this paper has reviewed and presented an updated literature review of microservices andmicrofrontends integration, together with the current trends for future studies.
We uncover the key aspects and activities that underpin sustainable energy development through a meticulous and systematic review of existing literature. Our research journey begins with analysing publication trends and tracing the chronological trajectory of scholarly output on sustainable energy development.
In recent years, important methods, algorithms, approaches, and databases have been proposed for research Facial Forensics without constraints. There are two approaches namely: the 2D approach has reached a certain level of maturity and has reported very high recognition rates; the 3D approach has been helping to reduce such ambient conditions.
Despite the growing importance of resilience in the construction sector, the existing literature often focuses on specific systems or functions without integrating a holistic view of resilience. Hence, there is a pressing need for comprehensive research that synthesizes these dimensions to provide a clearer framework for resilience in the construction industry. To find the inherent patterns ...
Certain concepts have a demonstrated basis for aiding recovery from dangerous alcohol or substance use, according to an analysis of scientific literature since 1990. Self-efficacy (a belief in one ...
Early detection of microsatellite instability (MSI) and microsatellite stability (MSS) is crucial in the fight against gastrointestinal (GI) cancer. MSI is a sign of genetic instability often associated with DNA repair mechanism deficiencies, which can cause (GI) cancers. On the other hand, MSS signifies genomic stability in microsatellite regions. Differentiating between these two states is ...