- Dissertation Proofreading and Editing
- Dissertation Service
- Dissertation Proposal Service
- Dissertation Chapter
- Dissertation Topic and Outline
- Statistical Analysis Services
- Model Answers and Exam Notes
- Dissertation Samples
- Essay Writing Service
- Assignment Service
- Report Service
- Coursework Service
- Literature Review Service
- Reflective Report Service
- Presentation Service
- Poster Service
- Criminal Psychology Dissertation Topics | List of Trending Ideas With Research Aims
- Cognitive Psychology Dissertation Topics | 10 Top Ideas For Research in 2024
- Social Psychology Dissertation Topics | 10 Latest Research Ideas
- Top 10 Clinical Psychology Dissertation Topics with Research Aims
- Educational Psychology Dissertation Topics | 10 Interesting Ideas For Research
- Customer Service Dissertation Topics | List of Latest Ideas For Students
- 15 Interesting Music Dissertation Topics
- Business Intelligence Dissertation Topics | List of Top Ideas With Research Aims
- Physical Education Dissertation Topics | 15 Interesting Title Examples
- 15 Top Forensic Science Dissertation Topics with Research Aims
- Islamic Finance Dissertation Topics | List of 15 Top Ideas With Research Aims
- Dissertation Examples
- Dissertation Proposal Examples
- Essay Examples
- Report Examples
- Coursework Examples
- Assignment Examples
- Literature Review Examples
- Dissertation Topic and Outline Examples
- Dissertation Chapter Examples
- Dissertation Help
- Dissertation Topics
- Academic Library
- Assignment Plagiarism Checker
- Coursework Plagiarism Checke
- Dissertation Plagiarism Checker
- Thesis Plagiarism Checker
- Report Plagiarism Checke
- Plagiarism Remover Service
- Plagiarism Checker Free Service
- Turnitin Plagiarism Checker Free Service
- Free Plagiarism Checker for Students
- Difference Between Paraphrasing & Plagiarism
- Free Similarity Checker
- How Plagiarism Checkers Work?
- How to Cite Sources to Avoid Plagiarism?
- Free Topics
- Get a Free Quote
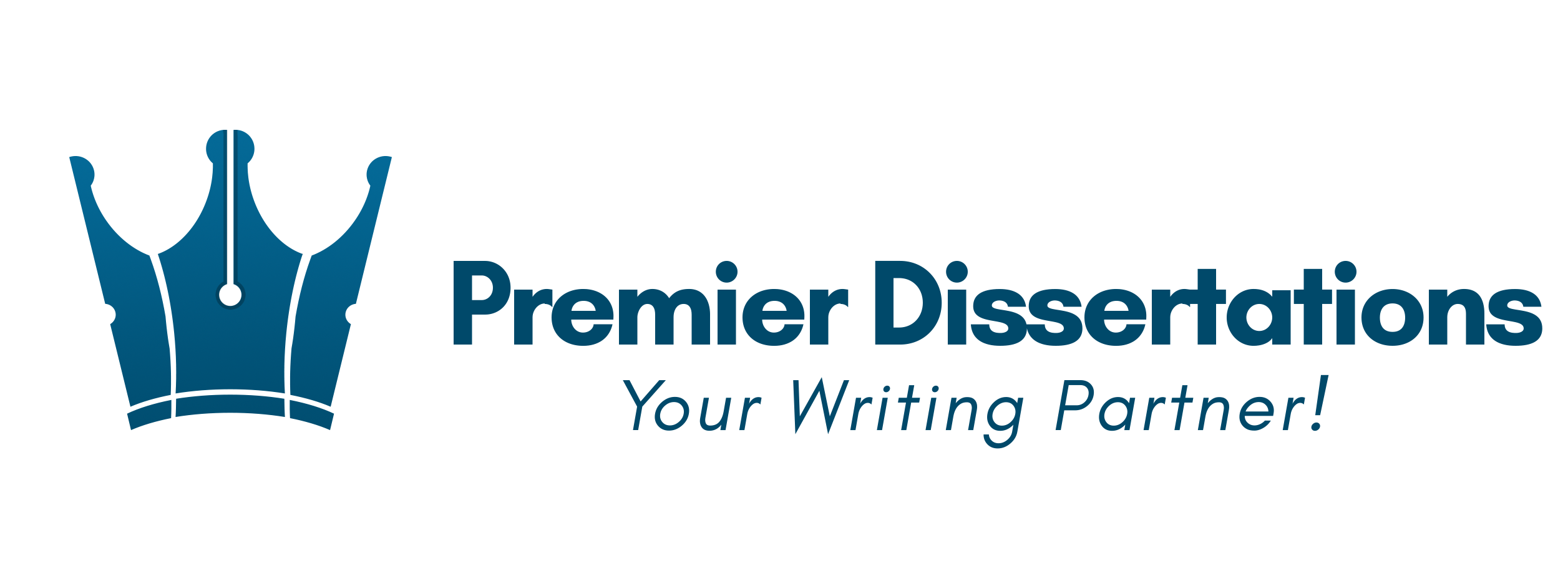
- Report Generating Service
- Model Answers and Exam Notes Writing
- Reflective or Personal Report Writing
- Poster Writing
- Literature Review Writing
- Premier Sample Dissertations
- Course Work
- Cognitive Psychology Dissertation Topics
- Physical Education Dissertation Topics
- 15 Top Forensic Science Dissertation Topics
- Top 10 Clinical Psychology Dissertation Topics
- Islamic Finance Dissertation Topics
- Social Psychology Dissertation Topics
- Educational Psychology Dissertation Topics
- Business Intelligence Dissertation Topics
- Customer Service Dissertation Topics
- Criminal Psychology Dissertation Topics

- Literature Review Example
- Report Example
- Assignment Example
- Coursework Example

- Coursework Plagiarism Checker
- Turnitin Plagiarism Checker
- Paraphrasing and Plagiarism
- Best Dissertation Plagiarism Checker
- Report Plagiarism Checker
- Similarity Checker
- Plagiarism Checker Free
- FREE Topics

Get an experienced writer start working
Review our examples before placing an order, learn how to draft academic papers, a step-by-step guide to dissertation data analysis.
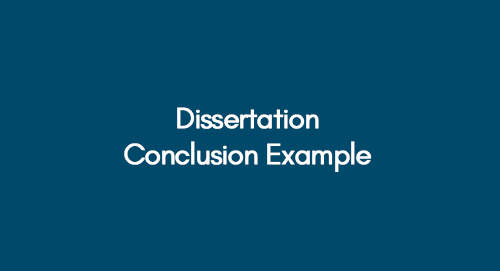
How to Write a Dissertation Conclusion? | Tips & Examples
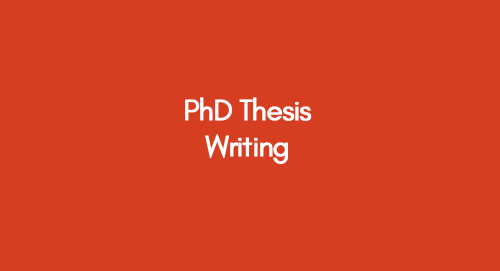
What is PhD Thesis Writing? | Beginner’s Guide
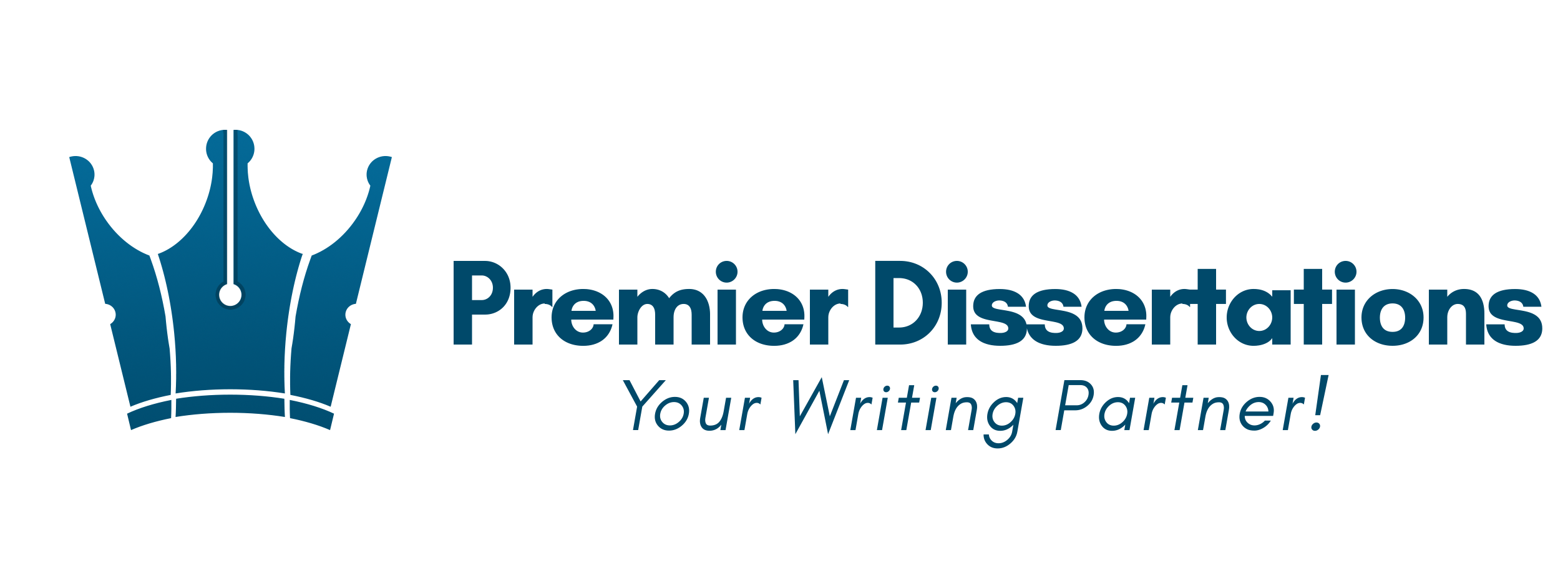
A data analysis dissertation is a complex and challenging project requiring significant time, effort, and expertise. Fortunately, it is possible to successfully complete a data analysis dissertation with careful planning and execution.
As a student, you must know how important it is to have a strong and well-written dissertation, especially regarding data analysis. Proper data analysis is crucial to the success of your research and can often make or break your dissertation.
To get a better understanding, you may review the data analysis dissertation examples listed below;
- Impact of Leadership Style on the Job Satisfaction of Nurses
- Effect of Brand Love on Consumer Buying Behaviour in Dietary Supplement Sector
- An Insight Into Alternative Dispute Resolution
- An Investigation of Cyberbullying and its Impact on Adolescent Mental Health in UK
3-Step Dissertation Process!

Get 3+ Topics

Dissertation Proposal

Get Final Dissertation
Types of data analysis for dissertation.
The various types of data Analysis in a Dissertation are as follows;
1. Qualitative Data Analysis
Qualitative data analysis is a type of data analysis that involves analyzing data that cannot be measured numerically. This data type includes interviews, focus groups, and open-ended surveys. Qualitative data analysis can be used to identify patterns and themes in the data.
2. Quantitative Data Analysis
Quantitative data analysis is a type of data analysis that involves analyzing data that can be measured numerically. This data type includes test scores, income levels, and crime rates. Quantitative data analysis can be used to test hypotheses and to look for relationships between variables.
3. Descriptive Data Analysis
Descriptive data analysis is a type of data analysis that involves describing the characteristics of a dataset. This type of data analysis summarizes the main features of a dataset.
4. Inferential Data Analysis
Inferential data analysis is a type of data analysis that involves making predictions based on a dataset. This type of data analysis can be used to test hypotheses and make predictions about future events.
5. Exploratory Data Analysis
Exploratory data analysis is a type of data analysis that involves exploring a data set to understand it better. This type of data analysis can identify patterns and relationships in the data.
Time Period to Plan and Complete a Data Analysis Dissertation?
When planning dissertation data analysis, it is important to consider the dissertation methodology structure and time series analysis as they will give you an understanding of how long each stage will take. For example, using a qualitative research method, your data analysis will involve coding and categorizing your data.
This can be time-consuming, so allowing enough time in your schedule is important. Once you have coded and categorized your data, you will need to write up your findings. Again, this can take some time, so factor this into your schedule.
Finally, you will need to proofread and edit your dissertation before submitting it. All told, a data analysis dissertation can take anywhere from several weeks to several months to complete, depending on the project’s complexity. Therefore, starting planning early and allowing enough time in your schedule to complete the task is important.
Essential Strategies for Data Analysis Dissertation
A. Planning
The first step in any dissertation is planning. You must decide what you want to write about and how you want to structure your argument. This planning will involve deciding what data you want to analyze and what methods you will use for a data analysis dissertation.
B. Prototyping
Once you have a plan for your dissertation, it’s time to start writing. However, creating a prototype is important before diving head-first into writing your dissertation. A prototype is a rough draft of your argument that allows you to get feedback from your advisor and committee members. This feedback will help you fine-tune your argument before you start writing the final version of your dissertation.
C. Executing
After you have created a plan and prototype for your data analysis dissertation, it’s time to start writing the final version. This process will involve collecting and analyzing data and writing up your results. You will also need to create a conclusion section that ties everything together.
D. Presenting
The final step in acing your data analysis dissertation is presenting it to your committee. This presentation should be well-organized and professionally presented. During the presentation, you’ll also need to be ready to respond to questions concerning your dissertation.
Data Analysis Tools
Numerous suggestive tools are employed to assess the data and deduce pertinent findings for the discussion section. The tools used to analyze data and get a scientific conclusion are as follows:
a. Excel
Excel is a spreadsheet program part of the Microsoft Office productivity software suite. Excel is a powerful tool that can be used for various data analysis tasks, such as creating charts and graphs, performing mathematical calculations, and sorting and filtering data.
b. Google Sheets
Google Sheets is a free online spreadsheet application that is part of the Google Drive suite of productivity software. Google Sheets is similar to Excel in terms of functionality, but it also has some unique features, such as the ability to collaborate with other users in real-time.
c. SPSS
SPSS is a statistical analysis software program commonly used in the social sciences. SPSS can be used for various data analysis tasks, such as hypothesis testing, factor analysis, and regression analysis.
d. STATA
STATA is a statistical analysis software program commonly used in the sciences and economics. STATA can be used for data management, statistical modelling, descriptive statistics analysis, and data visualization tasks.
SAS is a commercial statistical analysis software program used by businesses and organizations worldwide. SAS can be used for predictive modelling, market research, and fraud detection.
R is a free, open-source statistical programming language popular among statisticians and data scientists. R can be used for tasks such as data wrangling, machine learning, and creating complex visualizations.
g. Python
A variety of applications may be used using the distinctive programming language Python, including web development, scientific computing, and artificial intelligence. Python also has a number of modules and libraries that can be used for data analysis tasks, such as numerical computing, statistical modelling, and data visualization.
Testimonials
Very satisfied students
This is our reason for working. We want to make all students happy, every day. Review us on Sitejabber
Tips to Compose a Successful Data Analysis Dissertation
a. Choose a Topic You’re Passionate About
The first step to writing a successful data analysis dissertation is to choose a topic you’re passionate about. Not only will this make the research and writing process more enjoyable, but it will also ensure that you produce a high-quality paper.
Choose a topic that is particular enough to be covered in your paper’s scope but not so specific that it will be challenging to obtain enough evidence to substantiate your arguments.
b. Do Your Research
data analysis in research is an important part of academic writing. Once you’ve selected a topic, it’s time to begin your research. Be sure to consult with your advisor or supervisor frequently during this stage to ensure that you are on the right track. In addition to secondary sources such as books, journal articles, and reports, you should also consider conducting primary research through surveys or interviews. This will give you first-hand insights into your topic that can be invaluable when writing your paper.
c. Develop a Strong Thesis Statement
After you’ve done your research, it’s time to start developing your thesis statement. It is arguably the most crucial part of your entire paper, so take care to craft a clear and concise statement that encapsulates the main argument of your paper.
Remember that your thesis statement should be arguable—that is, it should be capable of being disputed by someone who disagrees with your point of view. If your thesis statement is not arguable, it will be difficult to write a convincing paper.
d. Write a Detailed Outline
Once you have developed a strong thesis statement, the next step is to write a detailed outline of your paper. This will offer you a direction to write in and guarantee that your paper makes sense from beginning to end.
Your outline should include an introduction, in which you state your thesis statement; several body paragraphs, each devoted to a different aspect of your argument; and a conclusion, in which you restate your thesis and summarize the main points of your paper.
e. Write Your First Draft
With your outline in hand, it’s finally time to start writing your first draft. At this stage, don’t worry about perfecting your grammar or making sure every sentence is exactly right—focus on getting all of your ideas down on paper (or onto the screen). Once you have completed your first draft, you can revise it for style and clarity.
And there you have it! Following these simple tips can increase your chances of success when writing your data analysis dissertation. Just remember to start early, give yourself plenty of time to research and revise, and consult with your supervisor frequently throughout the process.
How Does It Work ?
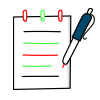
Fill the Form
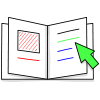
Writer Starts Working
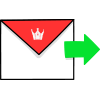
3+ Topics Emailed!
Studying the above examples gives you valuable insight into the structure and content that should be included in your own data analysis dissertation. You can also learn how to effectively analyze and present your data and make a lasting impact on your readers.
In addition to being a useful resource for completing your dissertation, these examples can also serve as a valuable reference for future academic writing projects. By following these examples and understanding their principles, you can improve your data analysis skills and increase your chances of success in your academic career.
You may also contact Premier Dissertations to develop your data analysis dissertation.
For further assistance, some other resources in the dissertation writing section are shared below;
How Do You Select the Right Data Analysis
How to Write Data Analysis For A Dissertation?
How to Develop a Conceptual Framework in Dissertation?
What is a Hypothesis in a Dissertation?
Get an Immediate Response
Discuss your requirments with our writers
WhatsApp Us Email Us Chat with Us
Get 3+ Free Dissertation Topics within 24 hours?
Your Number
Academic Level Select Academic Level Undergraduate Masters PhD
Area of Research
admin farhan
Related posts.
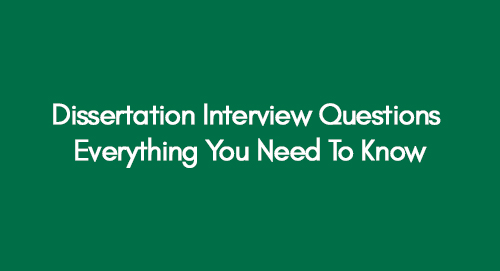
Dissertation Interview Questions | Everything You Need To Know
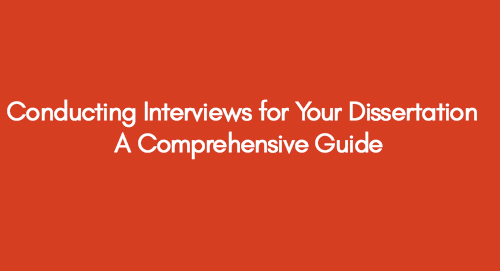
Conducting Interviews for Your Dissertation | A Comprehensive Guide
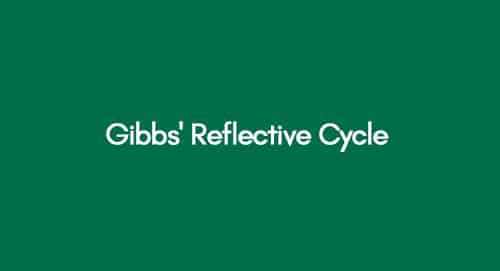
What is Gibbs’ Reflective Cycle and How Can It Benefit You? | Applications and Example
Comments are closed.
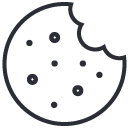

How To Write The Results/Findings Chapter
By: Derek Jansen (MBA) | Expert Reviewed By: Kerryn Warren (PhD) | July 2021
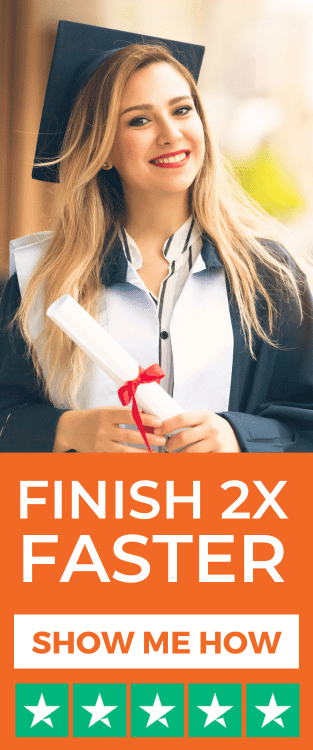
Overview: Quantitative Results Chapter
- What exactly the results chapter is
- What you need to include in your chapter
- How to structure the chapter
- Tips and tricks for writing a top-notch chapter
- Free results chapter template
What exactly is the results chapter?
The results chapter (also referred to as the findings or analysis chapter) is one of the most important chapters of your dissertation or thesis because it shows the reader what you’ve found in terms of the quantitative data you’ve collected. It presents the data using a clear text narrative, supported by tables, graphs and charts. In doing so, it also highlights any potential issues (such as outliers or unusual findings) you’ve come across.
But how’s that different from the discussion chapter?
Well, in the results chapter, you only present your statistical findings. Only the numbers, so to speak – no more, no less. Contrasted to this, in the discussion chapter , you interpret your findings and link them to prior research (i.e. your literature review), as well as your research objectives and research questions . In other words, the results chapter presents and describes the data, while the discussion chapter interprets the data.
Let’s look at an example.
In your results chapter, you may have a plot that shows how respondents to a survey responded: the numbers of respondents per category, for instance. You may also state whether this supports a hypothesis by using a p-value from a statistical test. But it is only in the discussion chapter where you will say why this is relevant or how it compares with the literature or the broader picture. So, in your results chapter, make sure that you don’t present anything other than the hard facts – this is not the place for subjectivity.
It’s worth mentioning that some universities prefer you to combine the results and discussion chapters. Even so, it is good practice to separate the results and discussion elements within the chapter, as this ensures your findings are fully described. Typically, though, the results and discussion chapters are split up in quantitative studies. If you’re unsure, chat with your research supervisor or chair to find out what their preference is.
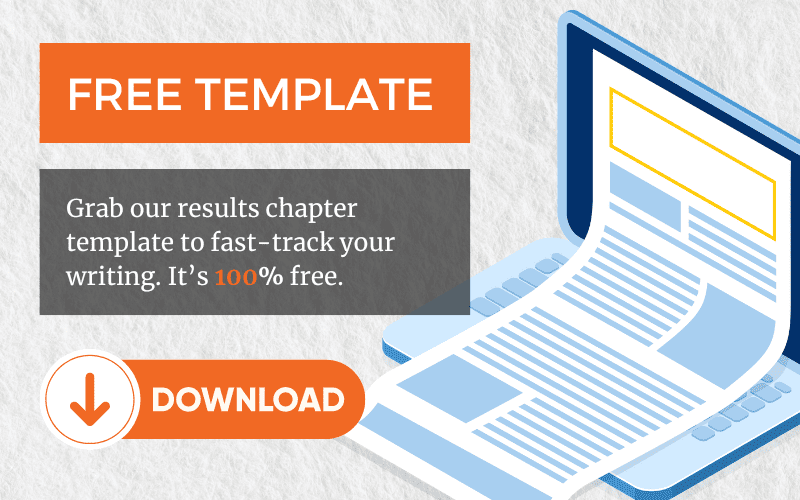
What should you include in the results chapter?
Following your analysis, it’s likely you’ll have far more data than are necessary to include in your chapter. In all likelihood, you’ll have a mountain of SPSS or R output data, and it’s your job to decide what’s most relevant. You’ll need to cut through the noise and focus on the data that matters.
This doesn’t mean that those analyses were a waste of time – on the contrary, those analyses ensure that you have a good understanding of your dataset and how to interpret it. However, that doesn’t mean your reader or examiner needs to see the 165 histograms you created! Relevance is key.
How do I decide what’s relevant?
At this point, it can be difficult to strike a balance between what is and isn’t important. But the most important thing is to ensure your results reflect and align with the purpose of your study . So, you need to revisit your research aims, objectives and research questions and use these as a litmus test for relevance. Make sure that you refer back to these constantly when writing up your chapter so that you stay on track.
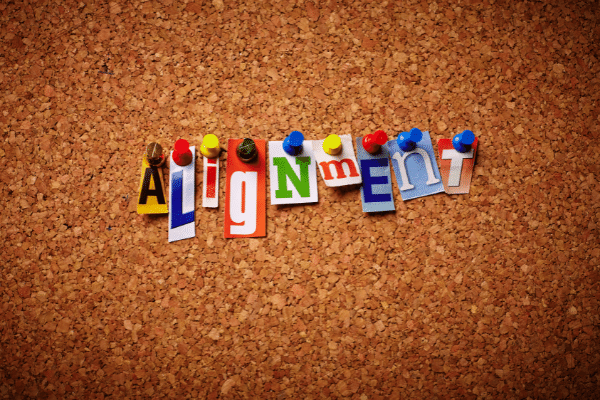
As a general guide, your results chapter will typically include the following:
- Some demographic data about your sample
- Reliability tests (if you used measurement scales)
- Descriptive statistics
- Inferential statistics (if your research objectives and questions require these)
- Hypothesis tests (again, if your research objectives and questions require these)
We’ll discuss each of these points in more detail in the next section.
Importantly, your results chapter needs to lay the foundation for your discussion chapter . This means that, in your results chapter, you need to include all the data that you will use as the basis for your interpretation in the discussion chapter.
For example, if you plan to highlight the strong relationship between Variable X and Variable Y in your discussion chapter, you need to present the respective analysis in your results chapter – perhaps a correlation or regression analysis.
Need a helping hand?
How do I write the results chapter?
There are multiple steps involved in writing up the results chapter for your quantitative research. The exact number of steps applicable to you will vary from study to study and will depend on the nature of the research aims, objectives and research questions . However, we’ll outline the generic steps below.
Step 1 – Revisit your research questions
The first step in writing your results chapter is to revisit your research objectives and research questions . These will be (or at least, should be!) the driving force behind your results and discussion chapters, so you need to review them and then ask yourself which statistical analyses and tests (from your mountain of data) would specifically help you address these . For each research objective and research question, list the specific piece (or pieces) of analysis that address it.
At this stage, it’s also useful to think about the key points that you want to raise in your discussion chapter and note these down so that you have a clear reminder of which data points and analyses you want to highlight in the results chapter. Again, list your points and then list the specific piece of analysis that addresses each point.
Next, you should draw up a rough outline of how you plan to structure your chapter . Which analyses and statistical tests will you present and in what order? We’ll discuss the “standard structure” in more detail later, but it’s worth mentioning now that it’s always useful to draw up a rough outline before you start writing (this advice applies to any chapter).
Step 2 – Craft an overview introduction
As with all chapters in your dissertation or thesis, you should start your quantitative results chapter by providing a brief overview of what you’ll do in the chapter and why . For example, you’d explain that you will start by presenting demographic data to understand the representativeness of the sample, before moving onto X, Y and Z.
This section shouldn’t be lengthy – a paragraph or two maximum. Also, it’s a good idea to weave the research questions into this section so that there’s a golden thread that runs through the document.
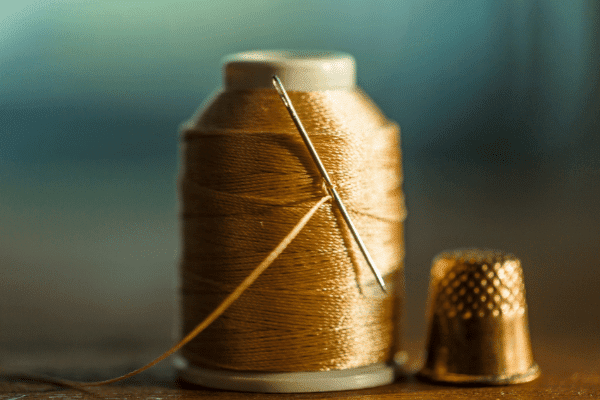
Step 3 – Present the sample demographic data
The first set of data that you’ll present is an overview of the sample demographics – in other words, the demographics of your respondents.
For example:
- What age range are they?
- How is gender distributed?
- How is ethnicity distributed?
- What areas do the participants live in?
The purpose of this is to assess how representative the sample is of the broader population. This is important for the sake of the generalisability of the results. If your sample is not representative of the population, you will not be able to generalise your findings. This is not necessarily the end of the world, but it is a limitation you’ll need to acknowledge.
Of course, to make this representativeness assessment, you’ll need to have a clear view of the demographics of the population. So, make sure that you design your survey to capture the correct demographic information that you will compare your sample to.
But what if I’m not interested in generalisability?
Well, even if your purpose is not necessarily to extrapolate your findings to the broader population, understanding your sample will allow you to interpret your findings appropriately, considering who responded. In other words, it will help you contextualise your findings . For example, if 80% of your sample was aged over 65, this may be a significant contextual factor to consider when interpreting the data. Therefore, it’s important to understand and present the demographic data.
Step 4 – Review composite measures and the data “shape”.
Before you undertake any statistical analysis, you’ll need to do some checks to ensure that your data are suitable for the analysis methods and techniques you plan to use. If you try to analyse data that doesn’t meet the assumptions of a specific statistical technique, your results will be largely meaningless. Therefore, you may need to show that the methods and techniques you’ll use are “allowed”.
Most commonly, there are two areas you need to pay attention to:
#1: Composite measures
The first is when you have multiple scale-based measures that combine to capture one construct – this is called a composite measure . For example, you may have four Likert scale-based measures that (should) all measure the same thing, but in different ways. In other words, in a survey, these four scales should all receive similar ratings. This is called “ internal consistency ”.
Internal consistency is not guaranteed though (especially if you developed the measures yourself), so you need to assess the reliability of each composite measure using a test. Typically, Cronbach’s Alpha is a common test used to assess internal consistency – i.e., to show that the items you’re combining are more or less saying the same thing. A high alpha score means that your measure is internally consistent. A low alpha score means you may need to consider scrapping one or more of the measures.
#2: Data shape
The second matter that you should address early on in your results chapter is data shape. In other words, you need to assess whether the data in your set are symmetrical (i.e. normally distributed) or not, as this will directly impact what type of analyses you can use. For many common inferential tests such as T-tests or ANOVAs (we’ll discuss these a bit later), your data needs to be normally distributed. If it’s not, you’ll need to adjust your strategy and use alternative tests.
To assess the shape of the data, you’ll usually assess a variety of descriptive statistics (such as the mean, median and skewness), which is what we’ll look at next.
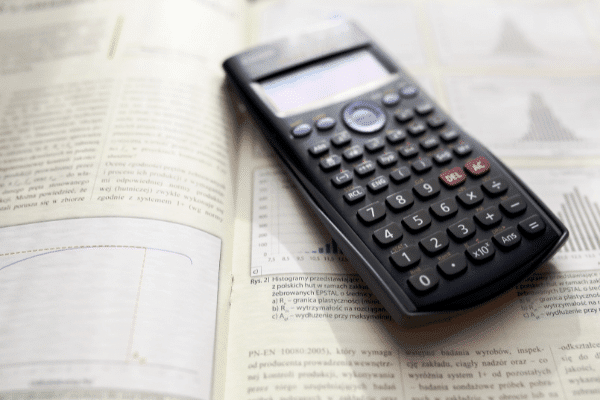

Step 5 – Present the descriptive statistics
Now that you’ve laid the foundation by discussing the representativeness of your sample, as well as the reliability of your measures and the shape of your data, you can get started with the actual statistical analysis. The first step is to present the descriptive statistics for your variables.
For scaled data, this usually includes statistics such as:
- The mean – this is simply the mathematical average of a range of numbers.
- The median – this is the midpoint in a range of numbers when the numbers are arranged in order.
- The mode – this is the most commonly repeated number in the data set.
- Standard deviation – this metric indicates how dispersed a range of numbers is. In other words, how close all the numbers are to the mean (the average).
- Skewness – this indicates how symmetrical a range of numbers is. In other words, do they tend to cluster into a smooth bell curve shape in the middle of the graph (this is called a normal or parametric distribution), or do they lean to the left or right (this is called a non-normal or non-parametric distribution).
- Kurtosis – this metric indicates whether the data are heavily or lightly-tailed, relative to the normal distribution. In other words, how peaked or flat the distribution is.
A large table that indicates all the above for multiple variables can be a very effective way to present your data economically. You can also use colour coding to help make the data more easily digestible.
For categorical data, where you show the percentage of people who chose or fit into a category, for instance, you can either just plain describe the percentages or numbers of people who responded to something or use graphs and charts (such as bar graphs and pie charts) to present your data in this section of the chapter.
When using figures, make sure that you label them simply and clearly , so that your reader can easily understand them. There’s nothing more frustrating than a graph that’s missing axis labels! Keep in mind that although you’ll be presenting charts and graphs, your text content needs to present a clear narrative that can stand on its own. In other words, don’t rely purely on your figures and tables to convey your key points: highlight the crucial trends and values in the text. Figures and tables should complement the writing, not carry it .
Depending on your research aims, objectives and research questions, you may stop your analysis at this point (i.e. descriptive statistics). However, if your study requires inferential statistics, then it’s time to deep dive into those .
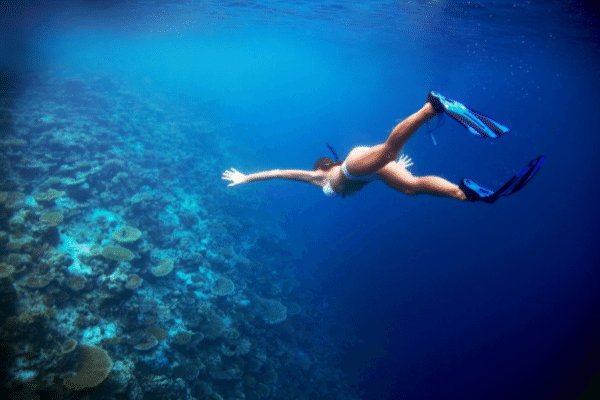
Step 6 – Present the inferential statistics
Inferential statistics are used to make generalisations about a population , whereas descriptive statistics focus purely on the sample . Inferential statistical techniques, broadly speaking, can be broken down into two groups .
First, there are those that compare measurements between groups , such as t-tests (which measure differences between two groups) and ANOVAs (which measure differences between multiple groups). Second, there are techniques that assess the relationships between variables , such as correlation analysis and regression analysis. Within each of these, some tests can be used for normally distributed (parametric) data and some tests are designed specifically for use on non-parametric data.
There are a seemingly endless number of tests that you can use to crunch your data, so it’s easy to run down a rabbit hole and end up with piles of test data. Ultimately, the most important thing is to make sure that you adopt the tests and techniques that allow you to achieve your research objectives and answer your research questions .
In this section of the results chapter, you should try to make use of figures and visual components as effectively as possible. For example, if you present a correlation table, use colour coding to highlight the significance of the correlation values, or scatterplots to visually demonstrate what the trend is. The easier you make it for your reader to digest your findings, the more effectively you’ll be able to make your arguments in the next chapter.
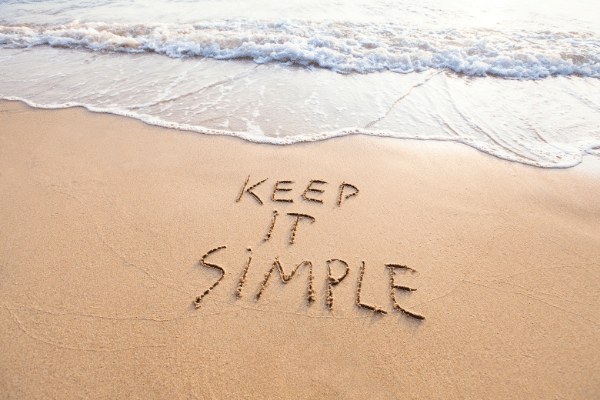
Step 7 – Test your hypotheses
If your study requires it, the next stage is hypothesis testing. A hypothesis is a statement , often indicating a difference between groups or relationship between variables, that can be supported or rejected by a statistical test. However, not all studies will involve hypotheses (again, it depends on the research objectives), so don’t feel like you “must” present and test hypotheses just because you’re undertaking quantitative research.
The basic process for hypothesis testing is as follows:
- Specify your null hypothesis (for example, “The chemical psilocybin has no effect on time perception).
- Specify your alternative hypothesis (e.g., “The chemical psilocybin has an effect on time perception)
- Set your significance level (this is usually 0.05)
- Calculate your statistics and find your p-value (e.g., p=0.01)
- Draw your conclusions (e.g., “The chemical psilocybin does have an effect on time perception”)
Finally, if the aim of your study is to develop and test a conceptual framework , this is the time to present it, following the testing of your hypotheses. While you don’t need to develop or discuss these findings further in the results chapter, indicating whether the tests (and their p-values) support or reject the hypotheses is crucial.
Step 8 – Provide a chapter summary
To wrap up your results chapter and transition to the discussion chapter, you should provide a brief summary of the key findings . “Brief” is the keyword here – much like the chapter introduction, this shouldn’t be lengthy – a paragraph or two maximum. Highlight the findings most relevant to your research objectives and research questions, and wrap it up.
Some final thoughts, tips and tricks
Now that you’ve got the essentials down, here are a few tips and tricks to make your quantitative results chapter shine:
- When writing your results chapter, report your findings in the past tense . You’re talking about what you’ve found in your data, not what you are currently looking for or trying to find.
- Structure your results chapter systematically and sequentially . If you had two experiments where findings from the one generated inputs into the other, report on them in order.
- Make your own tables and graphs rather than copying and pasting them from statistical analysis programmes like SPSS. Check out the DataIsBeautiful reddit for some inspiration.
- Once you’re done writing, review your work to make sure that you have provided enough information to answer your research questions , but also that you didn’t include superfluous information.
If you’ve got any questions about writing up the quantitative results chapter, please leave a comment below. If you’d like 1-on-1 assistance with your quantitative analysis and discussion, check out our hands-on coaching service , or book a free consultation with a friendly coach.
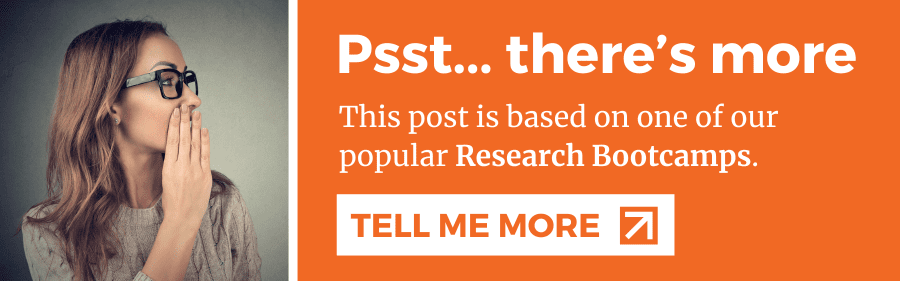
Learn More About Quantitative:
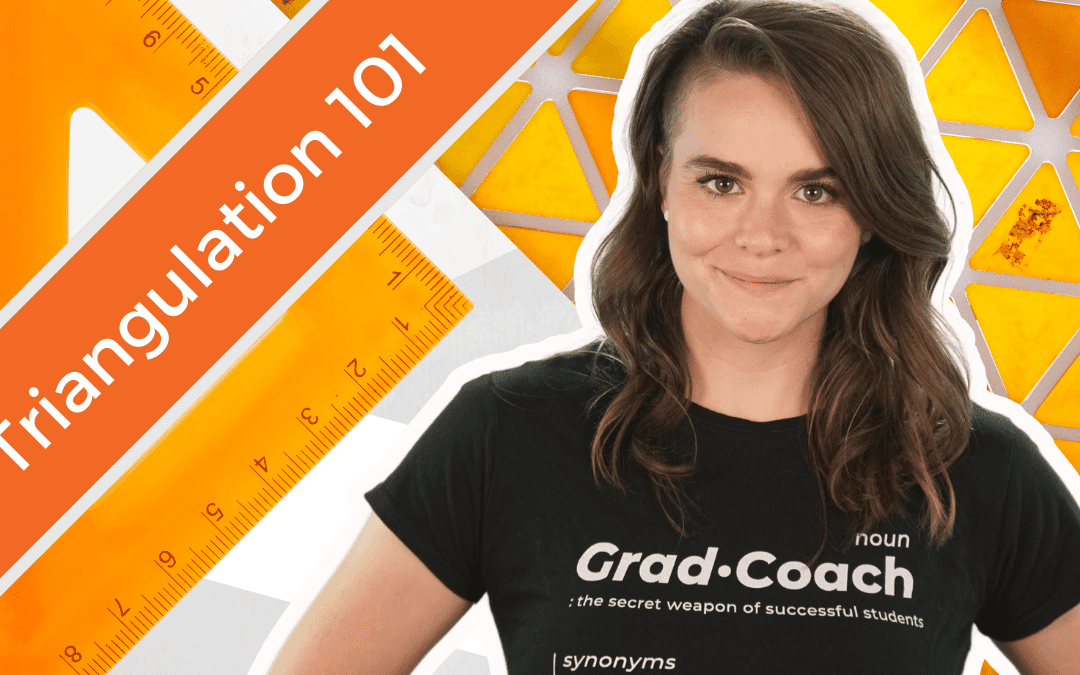
Triangulation: The Ultimate Credibility Enhancer
Triangulation is one of the best ways to enhance the credibility of your research. Learn about the different options here.
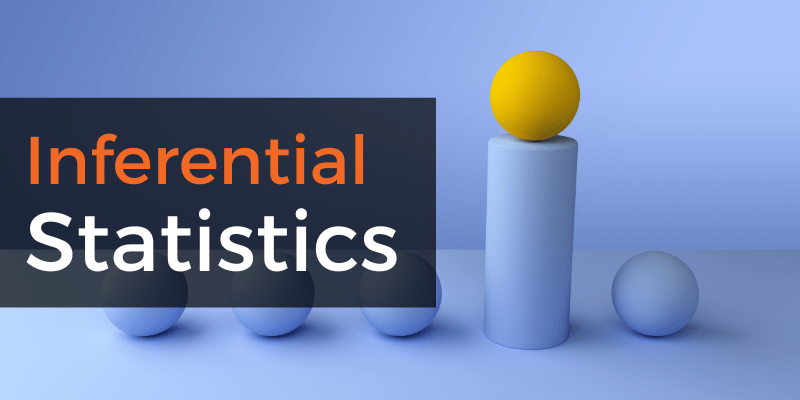
Inferential Statistics 101: Simple Explainer (With Examples)
Learn about the key concepts and tests within inferential statistics, including t-tests, ANOVA, chi-square, correlation and regression.
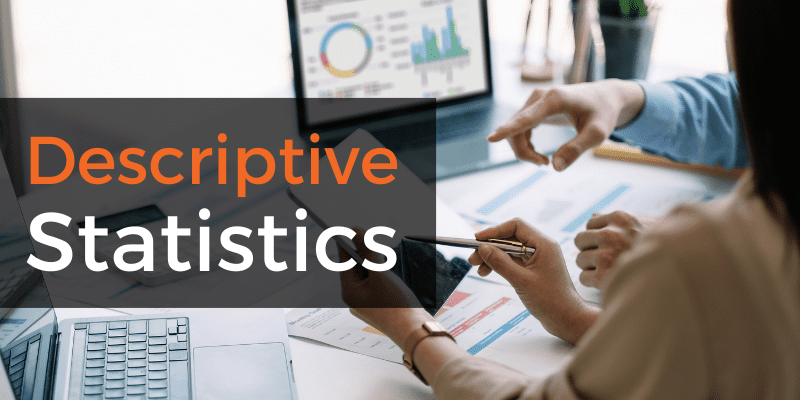
Descriptive Statistics 101: Simple Explainer (With Examples)
Learn about the key concepts and measures within descriptive statistics, including measures of central tendency and dispersion.
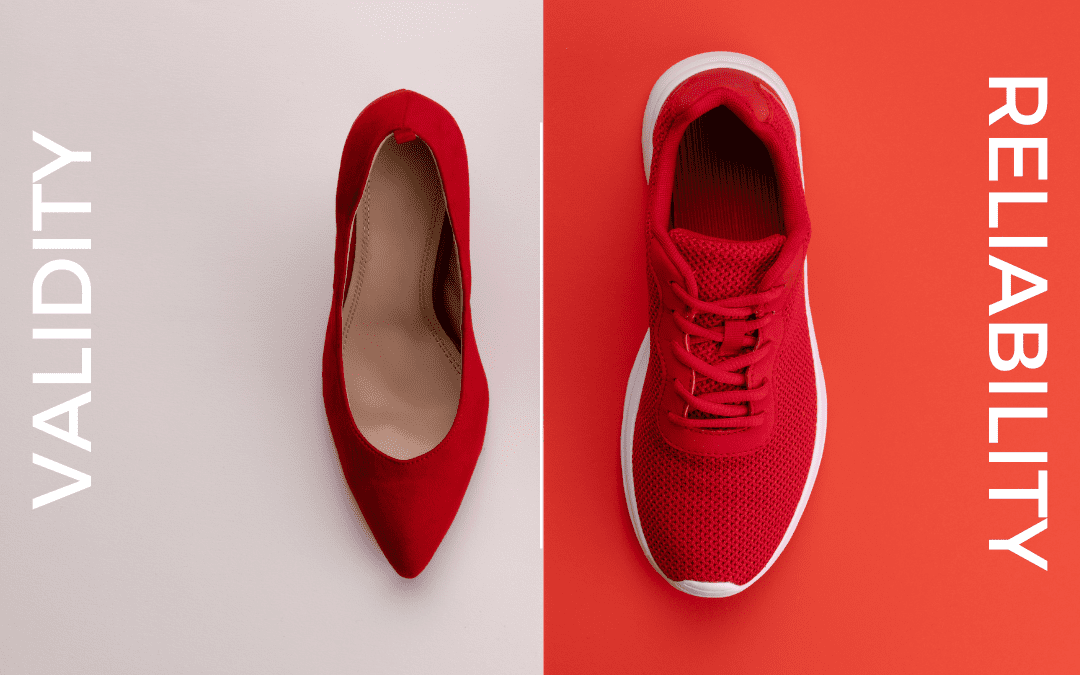
Validity & Reliability: Explained Simply
Learn about validity and reliability within the context of research methodology. Plain-language explainer video with loads of examples.
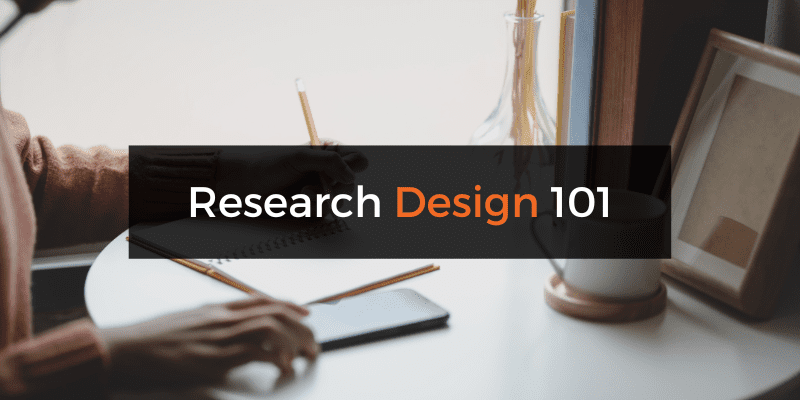
Research Design 101: Qualitative & Quantitative
Learn about research design for both qualitative and quantitative studies. Includes plain-language explanations and examples.
📄 FREE TEMPLATES
Research Topic Ideation
Proposal Writing
Literature Review
Methodology & Analysis
Academic Writing
Referencing & Citing
Apps, Tools & Tricks
The Grad Coach Podcast
Thank you. I will try my best to write my results.
Awesome content 👏🏾
this was great explaination
Submit a Comment Cancel reply
Your email address will not be published. Required fields are marked *
Save my name, email, and website in this browser for the next time I comment.
Submit Comment
- Print Friendly
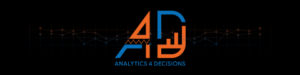
11 Tips For Writing a Dissertation Data Analysis
Since the evolution of the fourth industrial revolution – the Digital World; lots of data have surrounded us. There are terabytes of data around us or in data centers that need to be processed and used. The data needs to be appropriately analyzed to process it, and Dissertation data analysis forms its basis. If data analysis is valid and free from errors, the research outcomes will be reliable and lead to a successful dissertation.
Considering the complexity of many data analysis projects, it becomes challenging to get precise results if analysts are not familiar with data analysis tools and tests properly. The analysis is a time-taking process that starts with collecting valid and relevant data and ends with the demonstration of error-free results.
So, in today’s topic, we will cover the need to analyze data, dissertation data analysis, and mainly the tips for writing an outstanding data analysis dissertation. If you are a doctoral student and plan to perform dissertation data analysis on your data, make sure that you give this article a thorough read for the best tips!
What is Data Analysis in Dissertation?
Dissertation Data Analysis is the process of understanding, gathering, compiling, and processing a large amount of data. Then identifying common patterns in responses and critically examining facts and figures to find the rationale behind those outcomes.
Even f you have the data collected and compiled in the form of facts and figures, it is not enough for proving your research outcomes. There is still a need to apply dissertation data analysis on your data; to use it in the dissertation. It provides scientific support to the thesis and conclusion of the research.
Data Analysis Tools
There are plenty of indicative tests used to analyze data and infer relevant results for the discussion part. Following are some tests used to perform analysis of data leading to a scientific conclusion:
11 Most Useful Tips for Dissertation Data Analysis
Doctoral students need to perform dissertation data analysis and then dissertation to receive their degree. Many Ph.D. students find it hard to do dissertation data analysis because they are not trained in it.
1. Dissertation Data Analysis Services
The first tip applies to those students who can afford to look for help with their dissertation data analysis work. It’s a viable option, and it can help with time management and with building the other elements of the dissertation with much detail.
Dissertation Analysis services are professional services that help doctoral students with all the basics of their dissertation work, from planning, research and clarification, methodology, dissertation data analysis and review, literature review, and final powerpoint presentation.
One great reference for dissertation data analysis professional services is Statistics Solutions , they’ve been around for over 22 years helping students succeed in their dissertation work. You can find the link to their website here .
For a proper dissertation data analysis, the student should have a clear understanding and statistical knowledge. Through this knowledge and experience, a student can perform dissertation analysis on their own.
Following are some helpful tips for writing a splendid dissertation data analysis:
2. Relevance of Collected Data
It involves data collection of your related topic for research. Carefully analyze the data that tends to be suitable for your analysis. Do not just go with irrelevant data leading to complications in the results. Your data must be relevant and fit with your objectives. You must be aware of how the data is going to help in analysis.
If the data is irrelevant and not appropriate, you might get distracted from the point of focus. To show the reader that you can critically solve the problem, make sure that you write a theoretical proposition regarding the selection and analysis of data.
3. Data Analysis
For analysis, it is crucial to use such methods that fit best with the types of data collected and the research objectives. Elaborate on these methods and the ones that justify your data collection methods thoroughly. Make sure to make the reader believe that you did not choose your method randomly. Instead, you arrived at it after critical analysis and prolonged research.
Data analysis involves two approaches – Qualitative Data Analysis and Quantitative Data Analysis. Qualitative data analysis comprises research through experiments, focus groups, and interviews. This approach helps to achieve the objectives by identifying and analyzing common patterns obtained from responses.
On the other hand, quantitative analysis refers to the analysis and interpretation of facts and figures – to build reasoning behind the advent of primary findings. An assessment of the main results and the literature review plays a pivotal role in qualitative and quantitative analysis.
The overall objective of data analysis is to detect patterns and inclinations in data and then present the outcomes implicitly. It helps in providing a solid foundation for critical conclusions and assisting the researcher to complete the dissertation proposal.
4. Qualitative Data Analysis
Qualitative data refers to data that does not involve numbers. You are required to carry out an analysis of the data collected through experiments, focus groups, and interviews. This can be a time-taking process because it requires iterative examination and sometimes demanding the application of hermeneutics. Note that using qualitative technique doesn’t only mean generating good outcomes but to unveil more profound knowledge that can be transferrable.
Presenting qualitative data analysis in a dissertation can also be a challenging task. It contains longer and more detailed responses. Placing such comprehensive data coherently in one chapter of the dissertation can be difficult due to two reasons. Firstly, we cannot figure out clearly which data to include and which one to exclude. Secondly, unlike quantitative data, it becomes problematic to present data in figures and tables. Making information condensed into a visual representation is not possible. As a writer, it is of essence to address both of these challenges.
Qualitative Data Analysis Methods
Following are the methods used to perform quantitative data analysis.
- Deductive Method
This method involves analyzing qualitative data based on an argument that a researcher already defines. It’s a comparatively easy approach to analyze data. It is suitable for the researcher with a fair idea about the responses they are likely to receive from the questionnaires.
- Inductive Method
In this method, the researcher analyzes the data not based on any predefined rules. It is a time-taking process used by students who have very little knowledge of the research phenomenon.
5. Quantitative Data Analysis
Quantitative data contains facts and figures obtained from scientific research and requires extensive statistical analysis. After collection and analysis, you will be able to conclude. Generic outcomes can be accepted beyond the sample by assuming that it is representative – one of the preliminary checkpoints to carry out in your analysis to a larger group. This method is also referred to as the “scientific method”, gaining its roots from natural sciences.
The Presentation of quantitative data depends on the domain to which it is being presented. It is beneficial to consider your audience while writing your findings. Quantitative data for hard sciences might require numeric inputs and statistics. As for natural sciences , such comprehensive analysis is not required.
Quantitative Analysis Methods
Following are some of the methods used to perform quantitative data analysis.
- Trend analysis: This corresponds to a statistical analysis approach to look at the trend of quantitative data collected over a considerable period.
- Cross-tabulation: This method uses a tabula way to draw readings among data sets in research.
- Conjoint analysis : Quantitative data analysis method that can collect and analyze advanced measures. These measures provide a thorough vision about purchasing decisions and the most importantly, marked parameters.
- TURF analysis: This approach assesses the total market reach of a service or product or a mix of both.
- Gap analysis: It utilizes the side-by-side matrix to portray quantitative data, which captures the difference between the actual and expected performance.
- Text analysis: In this method, innovative tools enumerate open-ended data into easily understandable data.
6. Data Presentation Tools
Since large volumes of data need to be represented, it becomes a difficult task to present such an amount of data in coherent ways. To resolve this issue, consider all the available choices you have, such as tables, charts, diagrams, and graphs.
Tables help in presenting both qualitative and quantitative data concisely. While presenting data, always keep your reader in mind. Anything clear to you may not be apparent to your reader. So, constantly rethink whether your data presentation method is understandable to someone less conversant with your research and findings. If the answer is “No”, you may need to rethink your Presentation.
7. Include Appendix or Addendum
After presenting a large amount of data, your dissertation analysis part might get messy and look disorganized. Also, you would not be cutting down or excluding the data you spent days and months collecting. To avoid this, you should include an appendix part.
The data you find hard to arrange within the text, include that in the appendix part of a dissertation . And place questionnaires, copies of focus groups and interviews, and data sheets in the appendix. On the other hand, one must put the statistical analysis and sayings quoted by interviewees within the dissertation.
8. Thoroughness of Data
It is a common misconception that the data presented is self-explanatory. Most of the students provide the data and quotes and think that it is enough and explaining everything. It is not sufficient. Rather than just quoting everything, you should analyze and identify which data you will use to approve or disapprove your standpoints.
Thoroughly demonstrate the ideas and critically analyze each perspective taking care of the points where errors can occur. Always make sure to discuss the anomalies and strengths of your data to add credibility to your research.
9. Discussing Data
Discussion of data involves elaborating the dimensions to classify patterns, themes, and trends in presented data. In addition, to balancing, also take theoretical interpretations into account. Discuss the reliability of your data by assessing their effect and significance. Do not hide the anomalies. While using interviews to discuss the data, make sure you use relevant quotes to develop a strong rationale.
It also involves answering what you are trying to do with the data and how you have structured your findings. Once you have presented the results, the reader will be looking for interpretation. Hence, it is essential to deliver the understanding as soon as you have submitted your data.
10. Findings and Results
Findings refer to the facts derived after the analysis of collected data. These outcomes should be stated; clearly, their statements should tightly support your objective and provide logical reasoning and scientific backing to your point. This part comprises of majority part of the dissertation.
In the finding part, you should tell the reader what they are looking for. There should be no suspense for the reader as it would divert their attention. State your findings clearly and concisely so that they can get the idea of what is more to come in your dissertation.
11. Connection with Literature Review
At the ending of your data analysis in the dissertation, make sure to compare your data with other published research. In this way, you can identify the points of differences and agreements. Check the consistency of your findings if they meet your expectations—lookup for bottleneck position. Analyze and discuss the reasons behind it. Identify the key themes, gaps, and the relation of your findings with the literature review. In short, you should link your data with your research question, and the questions should form a basis for literature.
The Role of Data Analytics at The Senior Management Level
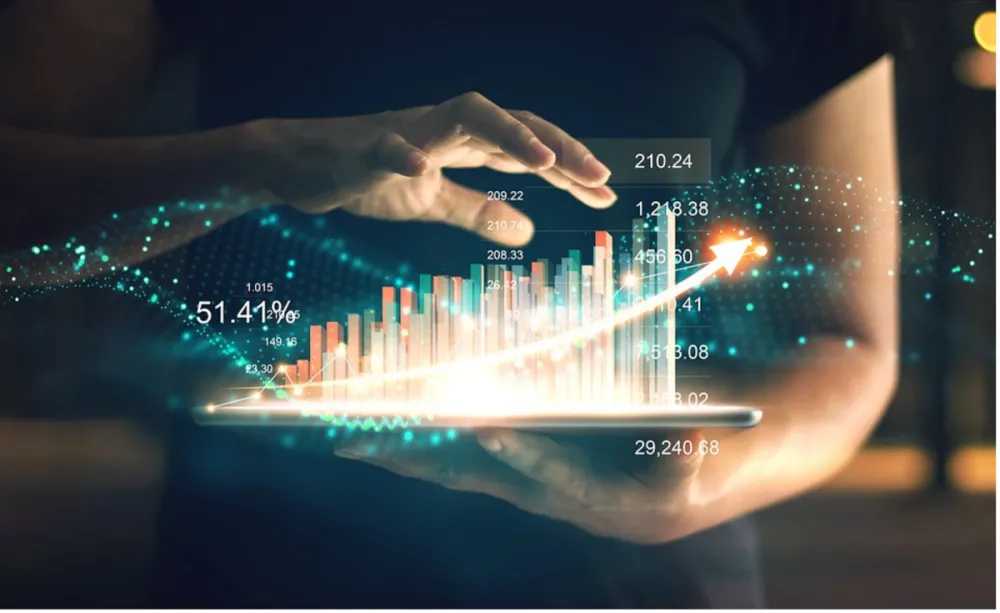
From small and medium-sized businesses to Fortune 500 conglomerates, the success of a modern business is now increasingly tied to how the company implements its data infrastructure and data-based decision-making. According
The Decision-Making Model Explained (In Plain Terms)

Any form of the systematic decision-making process is better enhanced with data. But making sense of big data or even small data analysis when venturing into a decision-making process might
13 Reasons Why Data Is Important in Decision Making
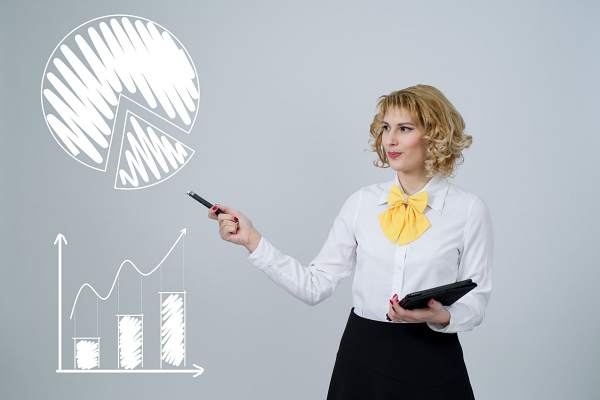
Data is important in decision making process, and that is the new golden rule in the business world. Businesses are always trying to find the balance of cutting costs while
Wrapping Up
Writing data analysis in the dissertation involves dedication, and its implementations demand sound knowledge and proper planning. Choosing your topic, gathering relevant data, analyzing it, presenting your data and findings correctly, discussing the results, connecting with the literature and conclusions are milestones in it. Among these checkpoints, the Data analysis stage is most important and requires a lot of keenness.
In this article, we thoroughly looked at the tips that prove valuable for writing a data analysis in a dissertation. Make sure to give this article a thorough read before you write data analysis in the dissertation leading to the successful future of your research.
Oxbridge Essays. Top 10 Tips for Writing a Dissertation Data Analysis.
Emidio Amadebai
As an IT Engineer, who is passionate about learning and sharing. I have worked and learned quite a bit from Data Engineers, Data Analysts, Business Analysts, and Key Decision Makers almost for the past 5 years. Interested in learning more about Data Science and How to leverage it for better decision-making in my business and hopefully help you do the same in yours.
Recent Posts
Causal vs Evidential Decision-making (How to Make Businesses More Effective)
In today’s fast-paced business landscape, it is crucial to make informed decisions to stay in the competition which makes it important to understand the concept of the different characteristics and...
Bootstrapping vs. Boosting
Over the past decade, the field of machine learning has witnessed remarkable advancements in predictive techniques and ensemble learning methods. Ensemble techniques are very popular in machine...

IMAGES
VIDEO
COMMENTS
The first step to writing a successful data analysis dissertation is to choose a topic you’re passionate about. Not only will this make the research and writing process more enjoyable, but it will also ensure that you produce a high-quality paper.
Master the art of analysing and interpreting data for your dissertation with our comprehensive guide. Learn essential techniques for quantitative and qualitative analysis, data preparation, and effective presentation to enhance the credibility and impact of your research.
Learn how to write up the quantitative results/findings/analysis chapter for your dissertation or thesis. Step-by-step guide + examples.
Writing data analysis in the dissertation involves dedication, and its implementations demand sound knowledge and proper planning. Choosing your topic, gathering relevant data, analyzing it, presenting your data and findings correctly, discussing the results, connecting with the literature and conclusions are milestones in it.
Learn about data analysis techniques and how they affect your replication-based dissertation.
A results section is where you report the main findings of the data collection and analysis you conducted for your thesis or dissertation. You should report all relevant results concisely and objectively, in a logical order.