Reflection about Statistics and Probability – Essay Sample [New]
Reflection paper about statistics and probability: introduction, what i learned in statistics and probability, reflection about statistics and probability: data analysis, reflection paper about statistics: future studies, statistics reflection paper: conclusion.
Learning statistics is viewed as an essential subject. It is also crucial to do statistics and probability reflection about their role in math, data management, and one’s daily life as a student. This statistics essay sample is going to cover what I have learned in statistics and probability. Essay will talk about my experience of learning the necessary skills for analyzing data and predicting possible outcomes.
I have extensively studied statistics and probability throughout this course. The probability course appeared to be a useful tool to apply in areas of statistical analysis. However, the part of learning statistics is much more prominent.
Statistical knowledge for both statisticians and non-statisticians is essential (Broers, 2006). So it is recommended that people from all fields be given the necessary statistical skills.
For that kind of reason, to gain quantitative skills to be applied and worked on in many ways, I followed this course. In this regard, I had hoped to acquire knowledge in designing experiments. I had wanted to grow in collecting and analyzing data, interpreting results, and drawing conclusions as well (Broers, 2006). I summarized and analyzed the results in my reflection about statistics and probability.
I can proudly say now that I have learned many useful things. I now understand math applications very clearly. I know how to collect, arrange, and explain the details. In data analysis, I may apply central tendency measurements such as mean, mode, and median.
I may also use dispersion measures to explain the data, such as standard deviation and variance. Furthermore, I have a detailed understanding of probability distribution. I can see what conditions are to be fulfilled for a normal distribution (Broers, 2006).
For example, I am aware of conditional probability and its applications. I also know how to use The Poisson process, Brownian motion process, Stochastic processes, Stationary processes, and Markovian processes. I learned the Ehrenfest model of diffusion, the symmetric random walk, queuing models, insurance risk theory, and Martingale theory.
Now, I can determine the relation between the two data sets. I can distinguish dependent and independent variables as well as the sort of relationship between them. I will tell you whether one random variable is causal to another. I am also able to determine positive, negative, and minimal correlations (Broers, 2006).
In most instances, data collection on an entire population is delicate (Chance, 2002). I got the necessary skills in sampling techniques in this respect. I have the skills to analyze sample data. Now I can draw inferences about the entire population using statistical probabilities and hypothesis testing.
In hypothesis testing, one seeks to determine whether the outcomes of a given sample are due to chance or known cause (Chance, 2002). Knowledge is used in the implementation of significance level, critical value, degrees of freedom, and p-value. One must be able to present the null hypothesis and the alternative hypotheses (Chance, 2002).
Now I’m able to use a t-test to assess if there are statistically significant variations between two data sets. In this regard, I understand the required assumptions for the t-test to be applied. I have a clear insight into the analysis of variance (ANOVA), both single and bidirectional. However, I feel that further practice would improve my knowledge of all of those applications (Chance, 2002).
This program has provided me with a good understanding of how statistics play a major part in life. Among other areas, statistics is the most commonly used research method in medicine, education, psychology, business, and economics (Rumsey, 2002). It helps to shape the choices people make in their everyday lives. Statistical studies may provide a clear picture of the consequences. For example, the results of such activities as smoking and contribute to corrective steps.
I was always keen to build a stable scientific career. I have now decided to major in statistics after taking this course. I would like to have advanced statistical skills that will help me to manage and evaluate complex research problems. The knowledge I’ve already obtained in this case will give me a strong foothold.
The goals I had hoped to accomplish by following this cause were well achieved. Now I can conduct experiments and collect, analyze, and interpret data. In real-life scenarios, I can apply that information and draw conclusions that will help develop answers to some issues.
Also, I have thoroughly studied and understood various causes of probability. I will be able to apply this knowledge where needed. Nevertheless, statistics will remain my main field of research.
This course, therefore, gave me the desire to seek additional statistical knowledge. For this reason, I intend to be in a better position in statistics to deal with more complex research issues.
- Broers, N. J. Learning goals: the primacy of statistical knowledge. 2006, Maastricht: Maastricht University.
- Chance, B. L. Components of statistical thinking and implications for instruction and assessment . 2002, Journal of Statistics Education, 10(3)
- Rumsey, D. J. Statistical literacy as a goal for introductory statistics courses. 2002, Journal of Statistics Education, 10(3)

What should I include in my statistics reflection paper?
Your statistics essay should contain a number of research data. Thoroughly research your subject. You can also include visual support like graphs or diagrams. Make sure the statistics are broken down and provide a general image of the issue.
Why are statistics so difficult?
Much of statistics makes no sense to students as it’s taught out of context. Many people often do not understand anything until they begin to examine data in their studies. You need to gain academic knowledge before you can understand statistics.
How do you start a reflection on statistics?
The good idea is to start your essay on statistics with a choice of the right topic. Make sure to research everything thoroughly and take notes of interesting observations. You should have a detailed understanding of a problem, and work well with data.
What is the aim of statistics?
Statistics aims to help you to use the right methods to gather the data. It ensures you use analysis correctly and present the results effectively. Statistics are essential to make science-based discoveries, make data-based decisions, and predict possible results.
What is the importance of statistics and probability?
Statistics is the mathematics that we use to gather, organize, and interpret numerical information. Probability is the study of possible events. It is often used in the analysis of chance games, genetics, and weather forecasting. A myriad of other everyday occurrences can be examined.
Cite this paper
- Chicago (N-B)
- Chicago (A-D)
StudyCorgi. (2020, July 8). Reflection about Statistics and Probability – Essay Sample [New]. https://studycorgi.com/reflection-about-statistics-essay-sample-new/
"Reflection about Statistics and Probability – Essay Sample [New]." StudyCorgi , 8 July 2020, studycorgi.com/reflection-about-statistics-essay-sample-new/.
StudyCorgi . (2020) 'Reflection about Statistics and Probability – Essay Sample [New]'. 8 July.
1. StudyCorgi . "Reflection about Statistics and Probability – Essay Sample [New]." July 8, 2020. https://studycorgi.com/reflection-about-statistics-essay-sample-new/.
Bibliography
StudyCorgi . "Reflection about Statistics and Probability – Essay Sample [New]." July 8, 2020. https://studycorgi.com/reflection-about-statistics-essay-sample-new/.
StudyCorgi . 2020. "Reflection about Statistics and Probability – Essay Sample [New]." July 8, 2020. https://studycorgi.com/reflection-about-statistics-essay-sample-new/.
This paper, “Reflection about Statistics and Probability – Essay Sample [New]”, was written and voluntary submitted to our free essay database by a straight-A student. Please ensure you properly reference the paper if you're using it to write your assignment.
Before publication, the StudyCorgi editorial team proofread and checked the paper to make sure it meets the highest standards in terms of grammar, punctuation, style, fact accuracy, copyright issues, and inclusive language. Last updated: November 10, 2023 .
If you are the author of this paper and no longer wish to have it published on StudyCorgi, request the removal . Please use the “ Donate your paper ” form to submit an essay.
- Skip to main content
- Keyboard shortcuts for audio player
13.7 Cosmos & Culture
How i learned to love statistics — and why you should, too.
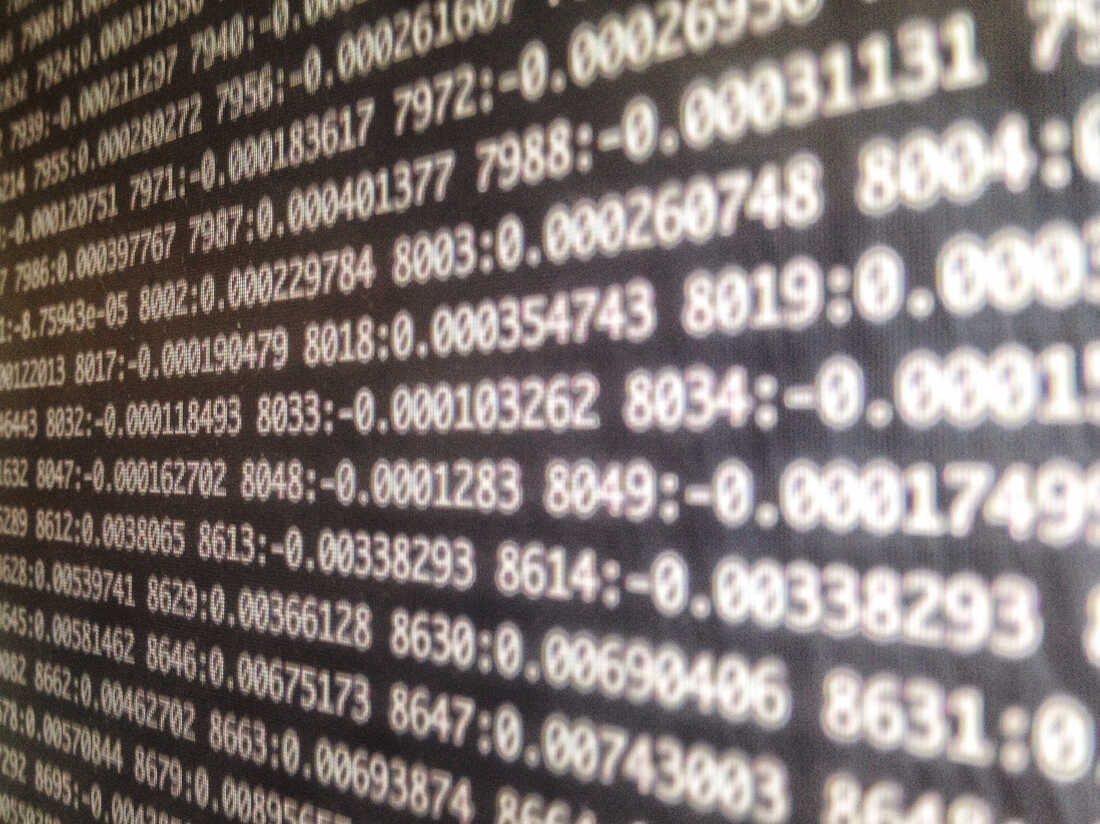
I always hated statistics. I mean really, really, really hated it.
Recently though, I've had a change of heart about the subject. In response, I find statistics changing my mind, or at least changing my perspective.
Let me explain.
When I was an undergraduate physics major, lab classes were mandatory. One of the most important parts of lab was doing error analysis — and that meant applying basic statistical ideas like calculating averages and measures of variability (like standard deviations ).
After a few weeks of this, I happily changed my major from physics to math-physics. The latter, I learned, came mercifully without the lab and its statistics requirement.
The problem for me wasn't doing the statistical calculations. They were OK. Instead, it was the idea of statistics that bummed me out. What I loved about physics were its laws. They were timeless. They were eternal. Most of all, I believed they fully and exactly determined everything about the behavior of the cosmos.
Statistics, on the other hand, was about the imperfect world of imperfect equipment taking imperfect data. For me, that realm was just a crappy version of the pure domain of perfect laws I was interested in. Measurements, by their nature, would always be messy. A truck goes by and jiggles your equipment. The kid you paid to do the observations isn't really paying attention. The very need to account for those variations made me sad.
Now, however, I see things very differently. My change of heart can be expressed in just two words — Big Data. Over the last 10 years, I've been watching in awe as the information we have been inadvertently amassing has changed society for better and worse. There is so much power, promise and peril for everyone in this brave new world that I knew I had to get involved . That's where my new life in statistics began.
The whole point of Big Data is to understand how to quickly and intelligently shift through peta-bytes of information and extract relationships. That means applying statistics-based methods to the numbers, names and other quantities that are what we mean by "The Data."
But to get anywhere with Big Data, I need to learn everything I can about statistics as fast as I can. My first refresher and guide in this effort has been the Coursera Course of Matthijs Rooduijn and Emiel van Loon of the University of Amsterdam. So far, I've only made it through the first week of their online lectures, but my platonic-oriented mind is already being retuned. The thing that's really getting to me is pretty simple, so I hope you'll excuse my naïve enthusiasm.
The issue is the world that's out there, independent of us. With my platonic-theoretical-physicist glasses on, I have always been happy to claim that we already know the exact laws exactly governing that independent world. But really, a claim like that is kind of bull. The real, independent world is way more complex than my theoretical physics equations can handle. This is particularly true when it comes to biology or, even more to the point, human society with its economy and culture and politics and elections.
So what can we do to understand the complexity of economies, cultures, politics and elections? We can take data. We can go out and measure whatever we can get our hands on. And it's right there that the light snaps on and vaults me past in my old distaste for statistics.
The problem with taking data is you don't know what it's telling you. It's always only a partial representation of the thing you are trying to understand. That means there is only one way to make clear links between the data you have taken and what the world wants to understand. You have to be very clear and very clever about interrogating the data. You have to develop methods — statistical methods — that extract answers you can trust.
Even more important, you need methods — statistical methods — for knowing exactly what the limits of trust are. Without these methods we would literally be lost. We'd be unable to see what data to take, what that data can tell us and when the data can't tell us anything at all.
Of course, what I'm saying will elicit a giant snooze for anyone who has thought even a bit about statistics and their use. But for us statistic-haters, the deeper philosophical basis of its methods in representing the world are worth consideration. That's because the effectiveness of all those algorithms creeping into every aspect of our lives hinge exactly on understanding the essential gap between the data we collect and the world it's meant to describe.
So now, finally , I can see the great range and beauty in the ideas behind statistics. Better late than never, at least on average.
Adam Frank is a co-founder of the 13.7 blog, an astrophysics professor at the University of Rochester, a book author and a self-described "evangelist of science." You can keep up with more of what Adam is thinking on Facebook and Twitter: @adamfrank4
- theoretical physics
Browse Course Material
Course info, instructors.
- Dr. Jeremy Orloff
- Dr. Jennifer French Kamrin
Departments
- Mathematics
As Taught In
- Discrete Mathematics
- Probability and Statistics
Learning Resource Types
Introduction to probability and statistics, why teach probability and statistics together.
Below, Dr. Jeremy Orloff and Dr. Jennifer French Kamrin describe how probability and statistics relate to one another, and why they are well suited to being taught together as a single subject.
Statistics is applied probability, and probability is the mathematical description of random events. While probability is a deductive science—starting from definitions we can prove mathematical theorems such as the law of large numbers—statistics is as much art as science. The students in 18.05 are typically not math majors, and their interests lie in the application more than the theory. Accordingly, the class is roughly 1/3 probability and 2/3 statistics. We provide the essential underpinnings of probability necessary to understand the meaning and justification of statistical methods. Statistics uses probability to describe and draw inferences from data. However, there are myriad ways that statistics are misunderstood and even abused—lies, damn lies, and statistics. This happens in both popular and technical presentations of experimental results. By providing enough of the underlying “why,” we are arming students with not only the knowledge of how to perform statistics, but perhaps more importantly, the ability to read and understand statistical arguments in research papers and media. This allows them to avoid common statistical pitfalls and to understand precisely what the statistics are suggesting.

You are leaving MIT OpenCourseWare
If you're seeing this message, it means we're having trouble loading external resources on our website.
If you're behind a web filter, please make sure that the domains *.kastatic.org and *.kasandbox.org are unblocked.
To log in and use all the features of Khan Academy, please enable JavaScript in your browser.
Unit 7: Probability
About this unit.
Probability tells us how often some event will happen after many repeated trials. You've experienced probability when you've flipped a coin, rolled some dice, or looked at a weather forecast. Go deeper with your understanding of probability as you learn about theoretical, experimental, and compound probability, and investigate permutations, combinations, and more!
Basic theoretical probability
- Intro to theoretical probability (Opens a modal)
- Probability: the basics (Opens a modal)
- Simple probability: yellow marble (Opens a modal)
- Simple probability: non-blue marble (Opens a modal)
- Intuitive sense of probabilities (Opens a modal)
- The Monty Hall problem (Opens a modal)
- Simple probability Get 5 of 7 questions to level up!
- Comparing probabilities Get 5 of 7 questions to level up!
Probability using sample spaces
- Probability with counting outcomes (Opens a modal)
- Example: All the ways you can flip a coin (Opens a modal)
- Die rolling probability (Opens a modal)
- Subsets of sample spaces (Opens a modal)
- Subsets of sample spaces Get 3 of 4 questions to level up!
Basic set operations
- Intersection and union of sets (Opens a modal)
- Relative complement or difference between sets (Opens a modal)
- Universal set and absolute complement (Opens a modal)
- Subset, strict subset, and superset (Opens a modal)
- Bringing the set operations together (Opens a modal)
- Basic set notation Get 5 of 7 questions to level up!
Experimental probability
- Experimental probability (Opens a modal)
- Theoretical and experimental probabilities (Opens a modal)
- Making predictions with probability (Opens a modal)
- Simulation and randomness: Random digit tables (Opens a modal)
- Experimental probability Get 5 of 7 questions to level up!
- Making predictions with probability Get 5 of 7 questions to level up!
Randomness, probability, and simulation
- Experimental versus theoretical probability simulation (Opens a modal)
- Theoretical and experimental probability: Coin flips and die rolls (Opens a modal)
- Random number list to run experiment (Opens a modal)
- Random numbers for experimental probability (Opens a modal)
- Statistical significance of experiment (Opens a modal)
- Interpret results of simulations Get 3 of 4 questions to level up!
Addition rule
- Probability with Venn diagrams (Opens a modal)
- Addition rule for probability (Opens a modal)
- Addition rule for probability (basic) (Opens a modal)
- Adding probabilities Get 3 of 4 questions to level up!
- Two-way tables, Venn diagrams, and probability Get 3 of 4 questions to level up!
Multiplication rule for independent events
- Sample spaces for compound events (Opens a modal)
- Compound probability of independent events (Opens a modal)
- Probability of a compound event (Opens a modal)
- "At least one" probability with coin flipping (Opens a modal)
- Free-throw probability (Opens a modal)
- Three-pointer vs free-throw probability (Opens a modal)
- Probability without equally likely events (Opens a modal)
- Independent events example: test taking (Opens a modal)
- Die rolling probability with independent events (Opens a modal)
- Probabilities involving "at least one" success (Opens a modal)
- Sample spaces for compound events Get 3 of 4 questions to level up!
- Independent probability Get 3 of 4 questions to level up!
- Probabilities of compound events Get 3 of 4 questions to level up!
- Probability of "at least one" success Get 3 of 4 questions to level up!
Multiplication rule for dependent events
- Dependent probability introduction (Opens a modal)
- Dependent probability: coins (Opens a modal)
- Dependent probability example (Opens a modal)
- Independent & dependent probability (Opens a modal)
- The general multiplication rule (Opens a modal)
- Dependent probability (Opens a modal)
- Dependent probability Get 3 of 4 questions to level up!
Conditional probability and independence
- Calculating conditional probability (Opens a modal)
- Conditional probability explained visually (Opens a modal)
- Conditional probability using two-way tables (Opens a modal)
- Conditional probability tree diagram example (Opens a modal)
- Tree diagrams and conditional probability (Opens a modal)
- Conditional probability and independence (Opens a modal)
- Analyzing event probability for independence (Opens a modal)
- Calculate conditional probability Get 3 of 4 questions to level up!
- Dependent and independent events Get 3 of 4 questions to level up!
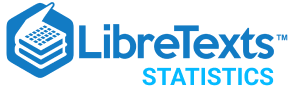
- school Campus Bookshelves
- menu_book Bookshelves
- perm_media Learning Objects
- login Login
- how_to_reg Request Instructor Account
- hub Instructor Commons
- Download Page (PDF)
- Download Full Book (PDF)
- Periodic Table
- Physics Constants
- Scientific Calculator
- Reference & Cite
- Tools expand_more
- Readability
selected template will load here
This action is not available.
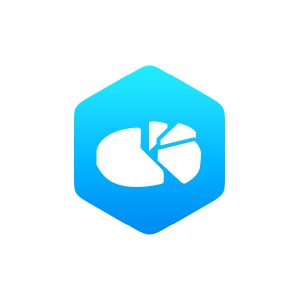
3: Probability Topics
- Last updated
- Save as PDF
- Page ID 692
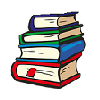
Probability theory is concerned with probability, the analysis of random phenomena. The central objects of probability theory are random variables, stochastic processes, and events: mathematical abstractions of non-deterministic events or measured quantities that may either be single occurrences or evolve over time in an apparently random fashion.
- 3.1: Introduction You have, more than likely, used probability. In fact, you probably have an intuitive sense of probability. Probability deals with the chance of an event occurring. Whenever you weigh the odds of whether or not to do your homework or to study for an exam, you are using probability. In this chapter, you will learn how to solve probability problems using a systematic approach.
- 3.2: Terminology In this module we learned the basic terminology of probability. The set of all possible outcomes of an experiment is called the sample space. Events are subsets of the sample space, and they are assigned a probability that is a number between zero and one, inclusive.
- 3.3: Independent and Mutually Exclusive Events Two events A and B are independent if the knowledge that one occurred does not affect the chance the other occurs. If they are not independent, then they are dependent. In sampling with replacement, with selecting each member with the possibility of being chosen more than once, and the events are considered to be independent. In sampling without replacement, each member may be chosen only once, and the events are considered not to be independent. When events do not share outcomes, they are mutu
- 3.4: Two Basic Rules of Probability The multiplication rule and the addition rule are used for computing the probability of A and B, and the probability of A or B for two given events A, B. In sampling with replacement each member has the possibility of being chosen more than once, and the events are considered to be independent. In sampling without replacement, each member may be chosen only once, and the events are not independent. The events A and B are mutually exclusive events when they have no common outcomes.
- 3.5: Contingency Tables There are several tools you can use to help organize and sort data when calculating probabilities. Contingency tables help display data and are particularly useful when calculating probabilites that have multiple dependent variables.
- 3.6: Tree and Venn Diagrams A tree diagram use branches to show the different outcomes of experiments and makes complex probability questions easy to visualize. A Venn diagram is a picture that represents the outcomes of an experiment. It generally consists of a box that represents the sample space S together with circles or ovals. The circles or ovals represent events. A Venn diagram is especially helpful for visualizing the OR event, the AND event, and the complement of an event and for understanding conditional probabi
- 3.7: Probability Topics (Worksheet) The student will use theoretical and empirical methods to estimate probabilities. The student will appraise the differences between the two estimates. The student will demonstrate an understanding of long-term relative frequencies.
- 3.E: Probability Topics (Exericses) These are homework exercises to accompany the Textmap created for "Introductory Statistics" by OpenStax.
- Essay Editor
Reflection on Statistics Learning Goals Essay
1. introduction.
This essay is based on my Unit Three Statistical Learning and Thinking course reflection paper. This course was an important step for me in learning to properly conduct research and to be able to validate the results. This is important because proper statistical analysis can lead to more meaningful results, which will then lead to conclusions that are based on the evidence provided. This is important in all fields, whether it be relaying a message to your children and providing evidence on why they should or shouldn't do something, or trying to get a new drug past the FDA. The more evidence and findings to support your case, the stronger the conclusion that can be drawn. This can save time, money, and resources. One main thing that I learned throughout the course is that we as analysts must keep an open mind and be willing to accept and consider our results no matter what they are. This is not always an easy feat. Many times we go into research with preconceived expected results. If said results are not attained, then we question the methods of the research. Sometimes this is necessary, while at other times this would be considered altering the data just so that the conclusion will agree with the original hypothesis. This is a common mistake in the course of an experiment and is often overlooked.
1.1. Purpose of the essay
It is well known that learning is a fundamental goal of education, and knowing the appropriate tools for learning is very important. Statistics holds an essential position in research, which is the process of learning and answering questions. Hence, statistics is a fundamental tool in learning and explains the process of how to obtain knowledge. Unfortunately, not all people can learn statistics effectively. It is argued that statistics is hard and tests related to statistics are not necessary. This is proven by many students not taking any advantages from learning statistics because they find it difficult to understand the concept and the process. The only way to learn statistics is to do the practices and then understand the concept. This is the first year for me studying statistics using SPSS software, which is a user-friendly software that will help a lot in conducting statistical analysis. This is very important because nowadays the usage of statistics is growing due to the advances in IT/technology. Learning statistics needs a lot of practice and understanding the concept. This can be done if the data obtained can be analyzed continuously. Unfortunately, I do not have any dataset on statistics to enable me to do the practices and understand the concept. So, by taking the Basic Statistics for Data Analysis course, it is very suitable for me because through this course I can learn statistics and at the same time I can use statistics to analyze my research data. This will give a lot of benefits for me by using only one method to achieve two different goals. Another advantage of learning statistics through this course is that I can learn statistics step-by-step and I can repeat learning each concept because the assessment for this course is based on my understanding of the statistics topic. This is far different from my previous experience learning statistics where I needed to rush to understand each topic to answer the test given.
1.2. Background information
Over the course of this semester, I have become a statistician/data analyst and learned to synthesize a large amount of data and determine the significance of the results. The importance of this has been demonstrated throughout my daily life in giving me a foundation for evidence-based decision making. These decisions, whether in my career as an analyst, a student working towards a degree, or a part-time coach, require the ability to assess the probability of certain events. This probability can be determined through statistical analysis and provides an educated approach to making a decision. This is the reasoning behind one of the six major learning goals for the statistics department: to assess the probability of a claim and its underlying assumptions in order to provide an informed decision. Synthesis is the ability to combine multiple concepts into a new whole. Oftentimes, this is more easily explained as taking information from multiple sources and putting it together. This skill is very important in today's data-laden world. With the infinite amounts of data available, being able to extract only what is needed and combine it with other data to form a new whole is a valuable skill. I have practiced and learned synthesis throughout this course and aim to further this skill by continuing on to a Masters in Business Analytics. A degree such as this and a career in the field would apply statistics to many types of businesses with the purpose of making more informed decisions and providing a foundation for those decisions. This can be seen as assessing a probability and thus ties back to one of the other major goals.
2. Statistics Learning Goals
Learning statistics involves a lot of learning goals that need to be achieved by students. There are numerous very specific statistical methods and techniques that can be used for something. Also, the interpretation of results is very crucial in whether the analysis is successful or the data obtained can be used. These aspects may help in achieving success in a career later on. However, the main focus in learning statistics is to achieve these learning goals: 2.1. Understanding statistical concepts. This is the most fundamental thing in learning statistics. Students need to understand the meaning of a technique, when to use it, how to use it, and also the assumptions of the technique. To have an understanding of statistical concepts, students need to be able to differentiate a few things: a) Know the meaning of populations, parameters, and sample statistics. This is important so that students can understand the root of statistical techniques later, which involves using sample statistics to estimate population parameters. It is a common mistake among students where they keep on interchanging these 3 things, and this shows that they are not clear about it. b) Know the difference between estimation and hypothesis testing. These are two types of inference in making conclusions about a population based on a sample. Estimation involves estimating a likely range for a population parameter, while hypothesis testing is evaluating two competing statements regarding a population parameter. Then students need to understand the various techniques used in estimation and hypothesis testing.
2.1. Understanding statistical concepts
An understanding of statistical concepts is vital if I am to succeed in this course. My long-term retention of all other learning goals depends on it. I need to have a deep conceptual understanding rather than just knowing the technical procedures involved. Often, people can be trained to carry out procedures of statistical analysis without understanding when and why these methods should be used. This increases the likelihood of incorrect analysis of data and thus incorrect conclusions being drawn. While it is relatively easy to use software to carry out statistical analysis, the difficult part is knowing what analysis to do and making sure that you understand the results. Throughout this course, I should always be asking myself things like "why am I doing this analysis?", "what does this analysis tell me?", "what are the assumptions behind this analysis?", and "how can I explain what I learned to someone else?". If at any time I am unclear on the answers to these questions, I know that I need to spend more time on the core statistical concepts. An understanding of statistical concepts is also important for research methodology in the behavioral sciences. This understanding aids in the design of experiments or surveys in the most appropriate manner. It helps in understanding the various limitations and errors associated with each method of data collection and in the most valid method of drawing conclusions from data. At the stage of learning what is the best way to determine research objectives and hypothesizing testing, I will do my best to relate any newly learned concepts to the context of behavioral sciences. During all stages of learning, I will use simple problems and examples to make sure I understand why and how to apply each method of statistical analysis.
2.2. Applying statistical techniques
I have mentioned in my learning goals that my second goal is to be able to apply statistical techniques to the data to make decisions. In order to work on this goal, I need to be solving problems that require the use of stats. The best practice is to head back to my texts and notes and see what will help me to answer the questions the best way possible. During this course, I will get the chance to do this more often as we have a stats software that will do the work for me, but I will still need to know what technique to use and be able to interpret the output. This will be very helpful and will give me a chance to improve my stats knowledge outside of class. By using R and interpreting the output, I will be able to improve my stats knowledge as well as my overall knowledge of the subject related to the data. Given that there are R problems assigned in the book, I can also use the data that I gather in response to those problems and still learning to use particular stats techniques. This is a very important goal as it will help me learn more about the techniques themselves with the intention that I will be able to make better use of stats in the future. I have no doubt that the best way to learn is by direct problem solving, but at the same time I may need to teach somebody how to do a technique or just what it is used for. This could happen with me tutoring stats or someone from another field who is simply looking for some stats help.
2.3. Interpreting statistical results
The process of interpreting statistical results is a complex one. At one level, it involves understanding the results of a particular study. But more than this, it involves understanding the meaning of the results in the context of the study. This will mostly likely require the researcher to return to the literature to understand what those results mean in terms of the statistical hypothesis under investigation. For practitioners of statistics, the ability to interpret the results of statistical analysis is an essential skill. Thus, if we want to teach someone statistics or a statistical technique, understanding how they will interpret the eventual results of their study will have implications for how we teach them statistics or that technique. This is a point that educators need to consider when teaching statistics. A new generation of statistical techniques is making huge inferential breakthroughs, beginning with a departure from some kind of Null Hypothesis significance Testing (NHST) approach to some level of significance and leading to concepts of sample to population generalisation, estimation, and comparison in terms of probability of effect. These techniques are not simply "testing ones" and their results are not immediately simple to understand. Methods involved in understanding these new kinds of statistical inferences will also be new and will differ across various analyses. It is important to consider how best to teach students how to interpret such results. The ability to interpret the results of data analysis starts with simple graphical representations of data and leads up to complex multivariate results in terms of underlying hypotheses. It is a key learning goal for a student of statistics. At the purely data level, this involves understanding key characteristics of data sets and being able to decide what kinds of data analysis are appropriate. These are global skills that apply to any analysis and any statistical software, and there is some methodology to teaching this kind of stuff. A typical way to teach someone how to choose the right data analysis is to use tone of example data analyses and some kind of simple flowchart based on the characteristics of that data set. This stage in teaching will be greatly facilitated by emphasis of the consensus on the GAISE recommendations. An important tool to help students interpret the results of statistical analysis is conducting the analysis and interpreting it within the context of a research project from their own discipline. Such analysis is more akin to the real data analysis students will do in the future and will help students transcend language and technical barriers. But from here on, it becomes very hard to teach how to interpret the kind of statistical results we have been discussing.
3. Reflection on Learning Process
When I began the statistics course, I faced many challenges in my learning of the course material. Although it was a subject I had never encountered before, I quickly learned to cope with the learning style. I became acquainted with various statistical tools to analyze data, draw conclusions, and make predictions. Before each class, I would complete the assigned reading, taking notes and jotting down any questions I had. Then in class, I would hear the material again during the lecture, and if by chance I still didn't understand the topic, I was always asking questions to clarify any confusion. As the content became more difficult, so did the frequency of questions that I had. In order to improve my understanding of the material, I soon found myself visiting my professor during office hours to help me make the connection of the material to real-life situations. This was when doing the work for the course was most difficult and frustrating for me. I was used to math being very black and white, with a formula to use for every problem. I soon found statistics was more of an art, with statistical tools used in various situations, but with different interpretations of the output. Many times I did not know if I was using the correct procedure to analyze data, and if the output was meaningful. This was something that frustrated me, which led to my frequent visits to the professor and increased reliance on the text to further my understanding of the material. But, by far my biggest challenge in learning of the course material came in our learning of statistical inference. This was the turning point that changed my understanding of statistics from pure memorization of steps and formulas to deep analytical thinking.
3.1. Challenges encountered
During the course of the statistics learned, I encountered many difficulties, some more easily overcome and some which had a ponderous weight on my learning process. The more trying difficulties I faced were understanding many concepts and definitions that were unclear or too technical for me to understand. An example of this was understanding the concept of a null hypothesis. Before it was mentioned what a null hypothesis actually was, I just assumed it was a hypothesis that was incorrect or 'null and void'. Applying more meaning to the term caused confusion. In an attempt to clear my confusion, I took to the internet to find a simpler explanation (this was often a good plan) and tried asking my tutor to explain it in simpler terms. He explained that it was the hypothesis that there was no difference, which I understood, but then went on to explain that he liked to think of it as the 'status quo'. This I felt was an unnecessary use of jargon to describe a jargon term. This was cleared in the following lecture but I feel a simpler explanation earlier would have been more beneficial. Another problem was my general constant muddling up of confidence intervals and tests of significance. I always knew how to do the procedures for these, but I would constantly be tripped up by getting the definitions of these mixed up. I would often find myself saying 'tests of confidence' and 'confidence levels', which wasn't helped by the fact that alpha is the level of significance, and 1-alpha is the confidence level. I finally cleared this up by writing the two definitions down in big letters on a sheet of paper and sticking it on my wall so that I was constantly reminded of them. This was a strange idea of mine, but my history of constantly muddling these two things made me think it would be a smart idea. This was the case as I never muddled them up again, and the piece of paper containing the definitions only came down after the exams.
3.2. Strategies for overcoming difficulties
First and foremost, I must reinforce the fact that having a clear goal of what I wanted to achieve in statistics was essential in shrugging off the problems encountered. One major difficulty was in the understanding of the course content and assimilating the relevant information from the irrelevant. Given that statistics is a highly technical subject, much effort was needed in internalizing the various concepts and theories. Understanding usually came about by reading the textbook several times. Furthermore, in a bid to identify the important areas, I found that attempting the tutorial questions prior to the commencement of the chapter proved to be a more efficient method compared to glancing through the chapter. This is because the tutorial questions directly tested the understanding of the important concepts. Hence, in trying to answer them, one would naturally have to go through the chapter in a more thorough manner and it also highlighted any misconceptions in one's understanding early. Last of all, to check for understanding, I would consistently consult with the professors and/or students who had understood the concept to ensure accuracy. Another very trying problem faced was the low confidence in attempting the homework and test questions despite having done the readings. This at times led to frustration and eventual resorting to looking at the answers too quickly. The turning point came when I realized that simply reading the course content did not guarantee immediate success and I failed to take into account that understanding comes at a different pace for different people. This realization was pivotal, as I began to adopt more patience in letting new concepts sink in rather than force it out of frustration. To tackle the confidence issue, I began to utilize a study group where I expressed my thoughts on a particular question and attempted to teach them the relevant concept. The positive feedback from this spurred an increase in confidence and more often than not, I was able to narrow the differences in understanding of a particular question between me and my friends. It was also a revelation to realize that the tuition of others indirectly strengthened my understanding due to having to explain the concept in a class environment.
3.3. Personal growth and improvement
I have certainly grown as a learner. My current knowledge of statistics is much more thorough and satisfying. Previous courses in statistics and probability were taken simply to achieve a grade sufficient for acceptance into a post-secondary program; as such, little aid to my general understanding and knowledge of these subjects was gained. The mathematical approach was far too complex, with little relevant example to aid understanding. At times I simply had to memorize a formula with little knowledge of what it truly meant or was impossible to apply due to confusion about the conditions for its use. This course has provided a comfortable learning environment and teachings with excellent clarification and intuitive relevance to aid understanding. I can now apply most of Descriptive Analysis and basic Probability with ease and would feel confident in teaching others. This was my main goal and I have certainly achieved it.
4. Conclusion
Statistics, which I did not know before doing the statistics course, I feel I know much more about the subject and its relevance to the world around me. I enjoyed learning about the ideas of the subject and applying them to real-life situations. My knowledge of statistics has increased dramatically while doing this course, and I believe I can carry this knowledge with me into the future. I have learned about statistical studies and the best ways to carry them out. This has given me broader knowledge and understanding of the scientific method and the importance of collecting data in today's modern world. Probability was a large part of the course and has given me invaluable information that can be applied to everyday life. I learned about the normal distribution and how many things today can be related to a normal distribution. This has given me the opportunity to see the world in a statistical way, an invaluable skill that has been taught to me by this course. Multiple data analysis was a topic that can provide unequaled assistance in future studies and employment. The ability to compare two sets of data and determine if there is a strong correlation between the two can be invaluable in determining many real-life situations. And finally, learning about statistical inference has given me a greater understanding of the information provided by many reports and studies today. I feel after completing this course that I can now read a newspaper and understand any studies produced with greater clarity and understanding than ever before.
4.1. Summary of key insights
This essay has proved very enlightening to my learning experience in statistics. Assessing my initial learning goals, it is evident I have met most of them and have gained much knowledge in this area of study. The challenge for me in the future is to try and maintain and remember what I have learned and also to keep abreast of what is happening in the world of statistical analysis. My main goal in studying statistics was to be able to do students justice as a science teacher when explaining this area of study. I believe my understanding of the topics, my learning of the language and terminology used in statistics, and also the practical meaning of many of the methods involved will enable me to do a better job than I could have previously. Due to the fact that I feel I understand these basic ideas, I am not going to forget things quickly – but I need to try and keep in touch with statistics to help build on my understanding and knowledge. An example of this would be to create resources to aid the teaching and learning of various statistical methods.
4.2. Future learning goals
Throughout the semester, my basic goal was to get a solid understanding of statistics. I know that this is a very general goal, but I specifically tried to learn the material in a way that made it useful outside of the classroom. I wanted to avoid simply memorizing formulas, and then regurgitating them on the exam without really understanding what they meant. To meet this goal, I did practice problems throughout each chapter to make sure I understood the material as it was being taught. I also met with the professor a couple times to clarify concepts that I was unsure about. This was helpful because if I had a misunderstanding, I could get direction on where to further study. Going to office hours is something I have done in the last few semesters to assure complete understanding of the material being taught. I plan to go into a field where statistics is regularly used, so it is important to keep the knowledge fresh. I think the best way to do this is to review problems a couple times a month to make sure the material is not lost. I have also just purchased a statistics-based software that I can use to further cement the concepts that I have learned. Coming out of this course, I think I have a firm grasp on the basics. If I can maintain that level of understanding and build from it, I think I will be in good shape in the future.
Related articles
The six facets of understanding essay.
1. Introduction Héctor Díaz-Zermeo, former Assistant Secretary of Education, said about the national education system in 1986, ". . . there is a lamentable convergence of pay in competence in all school systems across the country." Competence and students' low qualification are the principal complaints that give rise to Competitive Education for the Excellence in the Knowledge Society in the 21st Century. Thus it needs human beings with intellectual capabilities of high quality and a strong for ...
Rancho Solano Preparatory School's Closure Process
1. Introduction The parents of the Rancho Solano Preparatory School community trust that the school offers the exceptionally high standards that many search for in the private modern educational system. It is with this insight and trust that the school reports that Rancho Solano Preparatory School is closed at the end of the 2020-2021 academic year. Rancho Solano values the immense pride each family invests in being a Wildcat and deeply appreciates the continued loyalty, patience, and understan ...
Sexual Harassment in Schools - 840 Words | Essay Example
1. Introduction Although sexual harassment is often seen as a problem that affects adult women in the workplace, surveys have shown that girls in the United States experience sexual harassment that is similar to the experiences of adult women. This most deleterious of girls' experiences is common in the extreme. Harassment of girls centered on their sexuality is the most pervasive form of harassment and is common from the earliest grades onward, occurring in grades 6 through 12 in schools all a ...

Child Abuse: Preventive Measures Essay
1. Introduction Child abuse is a grievous historical problem. Its origins are not recent, and there are acceptable reasons why our attention is now directed towards it. In the last few decades, the civilizational process has been gathering hold of certain aspects of human behavior, in particular, preserving the rights of all individuals, including the provision of all essential needs of children. As a consequence, society is more sensitive to the plights of children. It is paradoxical in human ...
Hospitality Management Education and Career - 1403 Words
1. Introduction Although training for hospitality management has been in existence in Turkey for more than a hundred years, the first formal and official education took place in 1982 with the establishment of Hacettepe University Tourism and Hotel Management Department. While successful graduates were met with appreciation and attention in tourism, the number of students, in parallel with the increase in the quality of education programs, increased rapidly to train qualified personnel for the h ...
Negative Effects of Excessive Positive Reinforcement Essay
1. Introduction Behavior therapists typically advocate the use of reinforcement to influence a client's behavior. However, excessive positive reinforcement may have the unintended effect of reinforcing negative behavior in individuals. Traditional behavior management strategies recommend rewarding a client's positive behavior to foster a change in negative off-task, inappropriate, or disruptive behaviors. However, there is some scientific evidence to suggest that excessive positive reinforcemen ...
Importance of Teamwork Skills - 471 Words | Essay Example
1. Introduction The core of this chapter is the understanding of team skills and working groups in the context of organizations and business in general. The main goal is to show the importance of team skills and team development both for organizations and universities. The main questions are: Why is there such a great focus on these topics today? Why should organizations spend time and resources developing teams? Why are companies seeking young talents with team skills? Why should young talents ...
Water Cycle: Lesson Plan for 5th Graders Coursework
1. Introduction The water cycle is one of the major scientific concepts students learn about in elementary school. A thorough understanding of the water cycle is important for students, not just as scientists, but as inhabitants on the planet because the water cycle is essential to life. However, when students leave elementary school, they still struggle with understanding the water cycle as a system. It is important for students to understand such a complex system at a young age because it pav ...

Statistics Made Easy
Why is Statistics Important? (10 Reasons Statistics Matters!)
The field of statistics is concerned with collecting, analyzing, interpreting, and presenting data.
As technology becomes more present in our daily lives, more data is being generated and collected now than ever before in human history.
Statistics is the field that can help us understand how to use this data to do the following things:
- Gain a better understanding of the world around us.
- Make decisions using data.
- Make predictions about the future using data.
In this article we share 10 reasons for why the field of statistics is so important in modern life.
Reason 1: To Use Descriptive Statistics to Understand the World
Descriptive statistics are used to describe a chunk of raw data. There are three main types of descriptive statistics:
- Summary statistics
Each of these can help us gain a better understanding of existing data.
For example, suppose we have a set of raw data that shows the test scores of 10,000 students in a certain city. We can use descriptive statistics to:
- Calculate the average test score and the standard deviation of test scores.
- Generate a histogram or boxplot to visualize the distribution of test scores.
- Create a frequency table to understand the distribution of test scores.
Using descriptive statistics, we can understand the test scores of the students much more easily compared to just staring at the raw data.
Reason 2: To Be Wary of Misleading Charts
There are more charts being generated in journals, news outlets, online articles, and magazines than ever before. Unfortunately, charts can often be misleading if you don’t understand the underlying data.
For example, suppose some journal publishes a study that finds a negative correlation between GPA and ACT scores for students at a a certain university.
However, this negative correlation only occurs because the students who have both a high GPA and ACT score may go to an elite university while students who have both a low GPA and ACT score do not get admitted at all.

Although the correlation between ACT and GPA is positive in the population, the correlation appears to be negative in the sample.
This particular bias is known as Berkson’s bias . By being aware of this bias, you can avoid being mislead by certain charts.
Reason 3: To Be Wary of Confounding Variables
One important concept that you’ll learn about in statistics is the concept of confounding variables .
These are variables that are unaccounted for and can confound the results of an experiment and lead to unreliable findings.
For example, suppose a researcher collects data on ice cream sales and shark attacks and finds that the two variables are highly correlated. Does this mean that increased ice cream sales cause more shark attacks?
That’s unlikely. The more likely cause is the confounding variable temperature . When it is warmer outside, more people buy ice cream and more people go in the ocean.

Reason 4: To Make Better Decisions Using Probability
One of the most important sub-fields of statistics is probability . This is the field that studies how likely events are to happen.
By having a basic understanding of probability, you can make more informed decisions in the real world.
For example, suppose a high school student knows that they have a 10% chance of being accepted to a given university. Using the formula for the probability of “at least one” success , this student can find the probability that they’ll get accepted to at least one university they apply for and can adjust the number of universities they apply for accordingly.
Reason 5: To Understand P-Values in Research
Another important concept that you’ll learn about in statistics is p-values .
The textbook definition of a p-value is:
A p-value is the probability of observing a sample statistic that is at least as extreme as your sample statistic, given that the null hypothesis is true.
For example, suppose a factory claims that they produce tires that have a mean weight of 200 pounds. An auditor hypothesizes that the true mean weight of tires produced at this factory is different from 200 pounds so he runs a hypothesis test and finds that the p-value of the test is 0.04.
Here is how to interpret this p-value:
If the factory does indeed produce tires that have a mean weight of 200 pounds, then 4% of all audits will obtain the effect observed in the sample, or larger, because of random sample error. This tells us that obtaining the sample data that the auditor did would be pretty rare if indeed the factory produced tires that have a mean weight of 200 pounds.
Thus, the auditor would likely reject the null hypothesis that the true mean weight of tires produced at this factory is indeed 200 pounds.
Reason 6: To Understand Correlation
Another important concept that you’ll learn about in statistics is correlation , which tells us the linear association between two variables.
The value for a correlation coefficient always ranges between -1 and 1 where:
- -1 indicates a perfectly negative linear correlation between two variables
- 0 indicates no linear correlation between two variables
- 1 indicates a perfectly positive linear correlation between two variables
By understanding these values, you can understand the relationship between variables in the real world.
For example, if the correlation between advertisement spending and revenue is 0.87, then you can understand that there is a strong positive relationship between the two variables. As you spend more money on advertising, you can expect a predictable increase in revenue.
Reason 7: To Make Predictions About the Future
Another important reason to learn statistics is to understand basic regression models such as:
- Simple linear regression
- Multiple linear regression
- Logistic Regression
Each of these models allow you to make predictions about the future value of some response variable based on the value of certain predictor variables in the model.
For example, multiple linear regression models are used all the time in the real world by businesses when they use predictor variables such as age, income, ethnicity, etc. to predict how much customers will spend at their stores.
Similarly, logistics companies use predictor variables like total demand, population size, etc. to forecast future sales.
No matter which field you’re employed in, the odds are good that regression models will be used to predict some future phenomenon.
Reason 8: To Understand Potential Bias in Studies
Another reason to study statistics is to be aware of all the different types of bias that can occur in real-world studies.
Some examples include:
- Observer Bias
- Self-Selection Bias
- Referral Bias
- Omitted Variable Bias
- Undercoverage Bias
- Nonresponse Bias
By having a basic understanding of these types of biases, you can avoid committing them when performing research or be aware of them when reading through other research papers or studies.
Reason 9: To Understand the Assumptions Made by Statistical Tests
Many statistical tests make assumptions about the underlying data under study.
When reading the results of a study or even performing your own study, it’s important to understand what assumptions need to be made in order for the results to be reliable.
The following articles share the assumptions made in many commonly used statistical tests and procedures:
- What is the Assumption of Equal Variance in Statistics?
- What is the Assumption of Normality in Statistics?
- What is the Assumption of Independence in Statistics?
Reason 10: To Avoid Overgeneralization
Another reason to study statistics is to understand the concept of overgeneralization .
This occurs when the individuals in a study are not representative of the individuals in the overall population and therefore it’s inappropriate to generalize the conclusions from a study to the larger population.
For example, suppose we want to know what percentage of students at a certain school prefer “drama” as their favorite movie genre. If the total student population is a mix of 50% boys and 50% girls, then a sample with a mix of 90% boys and 10% girls might lead to biased results if far fewer boys prefer drama as their favorite genre.
Ideally, we want our sample to be like a “mini version” of our population. So, if the overall student population is composed of 50% girls and 50% boys, our sample would not be representative if it included 90% boys and only 10% girls.

Thus, whether you’re conducting your own survey or you’re reading about the results of a survey, it’s important to understand whether the sample data is representative of the total population and whether the findings of the survey can be generalized to the population with confidence.
Additional Resources
Check out the following articles to gain a basic understanding of the most important concepts in introductory statistics:
Descriptive vs. Inferential Statistics Population vs. Sample Statistic vs. Parameter Qualitative vs. Quantitative Variables Levels of Measurement: Nominal, Ordinal, Interval and Ratio
Featured Posts

Hey there. My name is Zach Bobbitt. I have a Masters of Science degree in Applied Statistics and I’ve worked on machine learning algorithms for professional businesses in both healthcare and retail. I’m passionate about statistics, machine learning, and data visualization and I created Statology to be a resource for both students and teachers alike. My goal with this site is to help you learn statistics through using simple terms, plenty of real-world examples, and helpful illustrations.
One Reply to “Why is Statistics Important? (10 Reasons Statistics Matters!)”
Wonderful article. Gave a gist of statistics in a nutshell. Never read such a good article about statistics. Thank you for your Statology site. I am able to understand the right way of doing and interpreting results statistical methods using your articles .
Leave a Reply Cancel reply
Your email address will not be published. Required fields are marked *
Join the Statology Community
I have read and agree to the terms & conditions
Home — Essay Samples — Science — Mathematics in Everyday Life — The Benefits and Importance of Statistics in Daily Life

The Benefits and Importance of Statistics in Daily Life
- Categories: Mathematics in Everyday Life
About this sample

Words: 649 |
Published: Dec 11, 2018
Words: 649 | Page: 1 | 4 min read
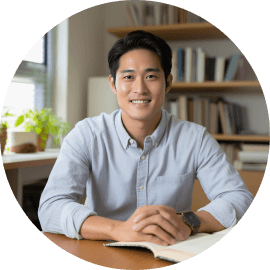
Cite this Essay
Let us write you an essay from scratch
- 450+ experts on 30 subjects ready to help
- Custom essay delivered in as few as 3 hours
Get high-quality help

Dr. Karlyna PhD
Verified writer
- Expert in: Science
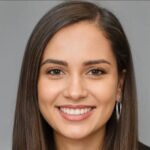
+ 120 experts online
By clicking “Check Writers’ Offers”, you agree to our terms of service and privacy policy . We’ll occasionally send you promo and account related email
No need to pay just yet!
Related Essays
1 pages / 470 words
2 pages / 1086 words
1 pages / 582 words
1 pages / 555 words
Remember! This is just a sample.
You can get your custom paper by one of our expert writers.
121 writers online
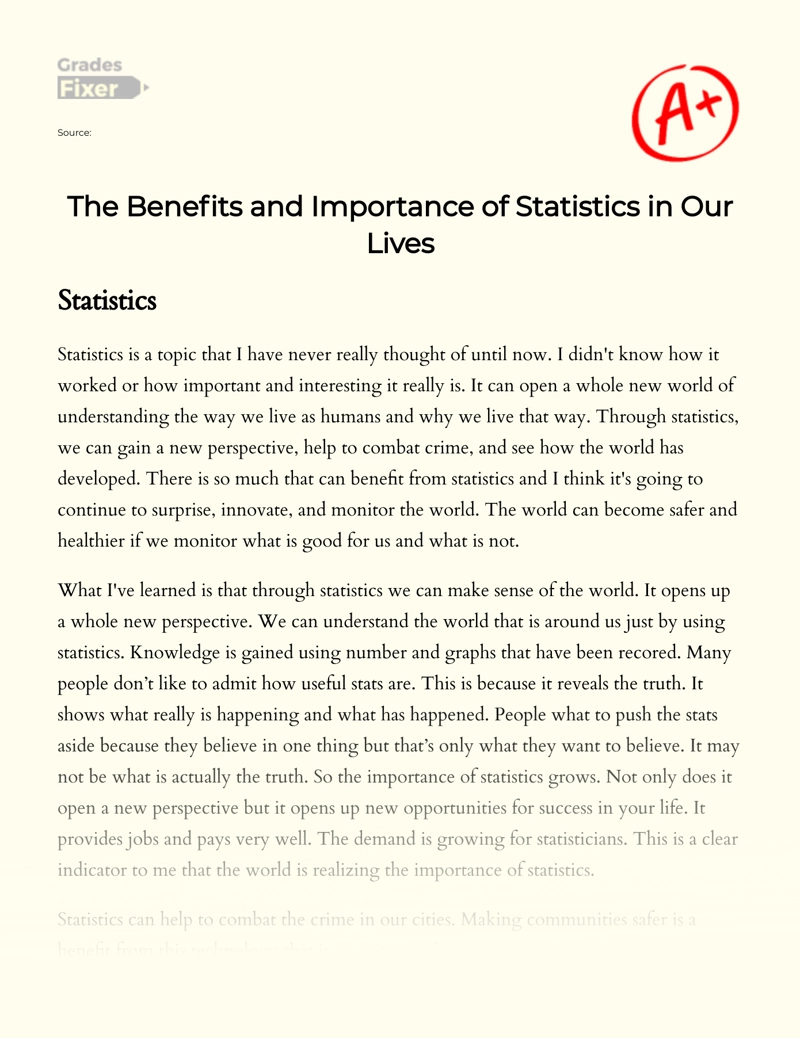
Still can’t find what you need?
Browse our vast selection of original essay samples, each expertly formatted and styled
Related Essays on Mathematics in Everyday Life
Mathematics has thousands of branches, and each branch means something different to every person. Some may know it as a useful tool that is a key to getting civilizations rolling. Others may just see it as bothersome and a tough [...]
The importance of the Fibonacci sequence in our daily life cannot be understated. This fascinating mathematical sequence, named after the Italian mathematician Leonardo of Pisa, also known as Fibonacci, holds significant [...]
Math has always been a subject that has both fascinated and challenged me. As a child, I struggled with the concept of numbers and equations, finding it difficult to understand the logic behind them. However, as I grew older and [...]
John Cassidy's College Calculus is an essential textbook for college students studying calculus. This essay will provide an in-depth analysis of the book, focusing on its content, approach, and effectiveness in teaching calculus [...]
Golden ration is a common mathematical ratio existing in the nature that is used to construct compositions in design work. The Golden ratio describes the perfectly symmetrical relationship between two proportions. It has been in [...]
It has many thing to do with our lives, it is everywhere. Are we going to be good businessmen if we don’t know how to add, subtract, divide, multiply, how to find probabilities, the statistic for the growths? Are we going to be [...]
Related Topics
By clicking “Send”, you agree to our Terms of service and Privacy statement . We will occasionally send you account related emails.
Where do you want us to send this sample?
By clicking “Continue”, you agree to our terms of service and privacy policy.
Be careful. This essay is not unique
This essay was donated by a student and is likely to have been used and submitted before
Download this Sample
Free samples may contain mistakes and not unique parts
Sorry, we could not paraphrase this essay. Our professional writers can rewrite it and get you a unique paper.
Please check your inbox.
We can write you a custom essay that will follow your exact instructions and meet the deadlines. Let's fix your grades together!
Get Your Personalized Essay in 3 Hours or Less!
We use cookies to personalyze your web-site experience. By continuing we’ll assume you board with our cookie policy .
- Instructions Followed To The Letter
- Deadlines Met At Every Stage
- Unique And Plagiarism Free
We can help you reset your password using the email address linked to your Project Euclid account.

- Subscription and Access
- Library Resources
- Publisher Tools
- Researcher Resources
- About Project Euclid
- Advisory Board
- News & Events
Editor(s) Deborah Nolan , Terry Speed
This volume is our tribute to David A. Freedman, whom we regard as one of the great statisticians of our time. He received his B.Sc. degree from McGill University and his Ph.D. from Princeton, and joined the Department of Statistics of the University of California, Berkeley, in 1962, where, apart from sabbaticals, he has been ever since.
In a career of over 45 years, David has made many fine contributions to probability and statistical theory, and to the application of statistics. His early research was on Markov chains and martingales, and two topics with which he has had a lifelong fascination: exchangeability and De Finetti’s theorem, and the consistency of Bayes estimates. His asymptotic theory for the bootstrap was also highly influential. David was elected to the American Academy of Arts and Sciences in 1991, and in 2003 he received the John J. Carty Award for the Advancement of Science from the U.S. National Academy of Sciences.
In addition to his purely academic research, David has extensive experience as a consultant, including working for the Carnegie Commission, the City of San Francisco, and the Federal Reserve, as well as several Departments of the U.S. Government–Energy, Treasury, Justice, and Commerce. He has testified as an expert witness on statistics in a number of law cases, including Piva v. Xerox (employment discrimination), Garza v. County of Los Angeles (voting rights), and New York v. Department of Commerce (census adjustment).
Lastly, he is an exceptionally good writer and teacher, and his many books and review articles are arguably his most important contribution to our subject. His widely used elementary text Statistics , written with R. Pisani and R. Purves, now in its 4th edition, is rightly regarded as a classic introductory exposition, while his second text Statistical Models (2005) is set to become just as successful in its field.
The roles of theoretical researcher, consultant, and expositor are not disjoint aspects of David’s personality, but fully integrated ones. For over 20 years now, he has been writing extensively on statistical modeling. He has contributed to theory, and prepared illuminating expositions and given penetrating critiques of old and new models and methods in a wide range of contexts. The result is a quite remarkable body of research on the theory and application of statistics, particularly to the decennial U.S. census, the social sciences (especially econometrics, political science and the law), and epidemiology. These themes are reflected in this volume of papers by friends and colleagues of David’s. We’d like to thank him for his wonderful body of work, and to wish him well for the future.
Information
Rights: Copyright © 2008, Institute of Mathematical Statistics
Published: 2008 First available in Project Euclid: 7 April 2008
Digital Object Identifier: 10.1214/imsc/1207580069
ISBN: 9780940600744
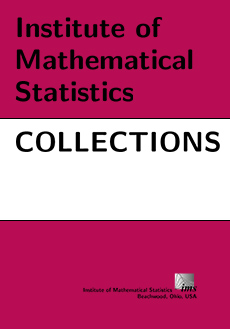
This paper argues for the status of formal probability theory as a mathematical, rather than a scientific, theory. David Freedman and Philip Stark’s concept of model based probabilities is examined and is used as a bridge between the formal theory and applications.
In this expository paper we describe a relatively elementary method of establishing the existence of a Dutch book in a simple multivariate normal prediction setting. The method involves deriving a nonstandard predictive distribution that is motivated by invariance. This predictive distribution satisfies an interesting identity which in turn yields an elementary demonstration of the existence of a Dutch book for a variety of possible predictive distributions.
We give an example of a transient reversible Markov chain that almost surely has only a finite number of cutpoints. We explain how this is relevant to a conjecture of Diaconis and Freedman and a question of Kaimanovich. We also answer Kaimanovich’s question when the Markov chain is a nearest-neighbor random walk on a tree.
We solve the moment problem for convex distribution functions on [0, 1] in terms of completely alternating sequences. This complements a recent solution of this problem by Diaconis and Freedman, and relates this work to the Lévy-Khintchine formula for the Laplace transform of a subordinator, and to regenerative composition structures.
Motivated by Lévy’s characterization of Brownian motion on the line, we propose an analogue of Brownian motion that has as its state space an arbitrary closed subset of the line that is unbounded above and below: such a process will be a martingale, will have the identity function as its quadratic variation process, and will be “continuous” in the sense that its sample paths don’t skip over points. We show that there is a unique such process, which turns out to be automatically a reversible Feller-Dynkin Markov process. We find its generator, which is a natural generalization of the operator f ↦½ f'' .
We then consider the special case where the state space is the self-similar set {± q k : k ∈ℤ}∪{0} for some q >1. Using the scaling properties of the process, we represent the Laplace transforms of various hitting times as certain continued fractions that appear in Ramanujan’s “lost” notebook and evaluate these continued fractions in terms of basic hypergeometric functions (that is, q -analogues of classical hypergeometric functions). The process has 0 as a regular instantaneous point, and hence its sample paths can be decomposed into a Poisson process of excursions from 0 using the associated continuous local time. Using the reversibility of the process with respect to the natural measure on the state space, we find the entrance laws of the corresponding Itô excursion measure and the Laplace exponent of the inverse local time – both again in terms of basic hypergeometric functions. By combining these ingredients, we obtain explicit formulae for the resolvent of the process. We also compute the moments of the process in closed form. Some of our results involve q -analogues of classical distributions such as the Poisson distribution that have appeared elsewhere in the literature.
Assessment of learning in higher education is a critical concern to policy makers, educators, parents, and students. And, doing so appropriately is likely to require including constructed response tests in the assessment system. We examined whether scoring costs and other concerns with using open-end measures on a large scale (e.g., turnaround time and inter-reader consistency) could be addressed by machine grading the answers. Analyses with 1359 students from 14 colleges found that two human readers agreed highly with each other in the scores they assigned to the answers to three types of open-ended questions. These reader assigned scores also agreed highly with those assigned by a computer. The correlations of the machine-assigned scores with SAT scores, college grades, and other measures were comparable to the correlations of these variables with the hand-assigned scores. Machine scoring did not widen differences in mean scores between racial/ethnic or gender groups. Our findings demonstrated that machine scoring can facilitate the use of open-ended questions in large-scale testing programs by providing a fast, accurate, and economical way to grade responses.
The U.S. Census Bureau provides an estimate of the true population as a supplement to the basic census numbers. This estimate is constructed from data in a post-censal survey. The overall procedure is referred to as dual system estimation. Dual system estimation is designed to produce revised estimates at all levels of geography, via a synthetic estimation procedure.
We design three alternative formulas for dual system estimation and investigate the differences in area estimates produced as a result of using those formulas. The primary target of this exercise is to better understand the nature of the homogeneity assumptions involved in dual system estimation and their consequences when used for the enumeration data that occurs in an actual large scale application like the Census. (Assumptions of this nature are sometimes collectively referred to as the “synthetic assumption” for dual system estimation.)
The specific focus of our study is the treatment of the category of census counts referred to as imputations in dual system estimation. Our results show the degree to which varying treatment of these imputation counts can result in differences in population estimates for local areas such as states or counties.
This paper tries to tell the story of the general linear model, which saw the light of day 200 years ago, and the assumptions underlying it. We distinguish three principal stages (ignoring earlier more isolated instances). The model was first proposed in the context of astronomical and geodesic observations, where the main source of variation was observational error. This was the main use of the model during the 19th century.
In the 1920’s it was developed in a new direction by R.A. Fisher whose principal applications were in agriculture and biology. Finally, beginning in the 1930’s and 40’s it became an important tool for the social sciences. As new areas of applications were added, the assumptions underlying the model tended to become more questionable, and the resulting statistical techniques more prone to misuse.
Over the past two decades, a variety of methods have been used to count the homeless in large metropolitan areas. In this paper, we report on an effort to count the homeless in Los Angeles County, one that employed the sampling of census tracts. A number of complications are discussed, including the need to impute homeless counts to areas of the County not sampled. We conclude that, despite their imperfections, estimated counts provided useful and credible information to the stakeholders involved.
The Women’s Health Initiative randomized clinical trial of hormone therapy found no benefit of hormones in preventive cardiovascular disease, a finding in striking contrast with a large body of observational research. Understanding whether better methodology and/or statistical adjustment might have prevented the erroneous conclusions of observational research is important. This is a re-analysis of data from a case-control study examining the relationship of postmenopausal hormone therapy and the risks of myocardial infarction (MI) and ischemic stroke in which we reported no overall increase or decrease in the risk of either event. Variables measuring health behavior/lifestyle that are not likely to be causally with the risks of MI and stroke (e.g., sunscreen use) were included in multivariate analysis along with traditional confounders (age, hypertension, diabetes, smoking, body mass index, ethnicity, education, prior coronary heart disease for MI and prior stroke/TIA for stroke) to determine whether adjustment for the health behavior/lifestyle variables could reproduce or bring the results closer to the findings in a large and definitive randomized clinical trial of hormone therapy, the Women’s Health Initiative.
For both MI and stroke, measures of health behavior/lifestyle were associated with odds ratios (ORs) less than 1.0. Adjustment for traditional cardiovascular disease confounders did not alter the magnitude of the ORs for MI or stroke. Addition of a subset of these variables selected using stepwise regression to the final MI or stroke models along with the traditional cardiovascular disease confounders moved the ORs for estrogen and estrogen/progestin use closer to values observed in the Women Health Initiative clinical trial, but did not reliably reproduce the clinical trial results for these two endpoints.
We propose a general and formal statistical framework for multiple tests of association between known fixed features of a genome and unknown parameters of the distribution of variable features of this genome in a population of interest. The known gene-annotation profiles, corresponding to the fixed features of the genome, may concern Gene Ontology (GO) annotation, pathway membership, regulation by particular transcription factors, nucleotide sequences, or protein sequences. The unknown gene-parameter profiles, corresponding to the variable features of the genome, may be, for example, regression coefficients relating possibly censored biological and clinical outcomes to genome-wide transcript levels, DNA copy numbers, and other covariates. A generic question of great interest in current genomic research regards the detection of associations between biological annotation metadata and genome-wide expression measures. This biological question may be translated as the test of multiple hypotheses concerning association measures between gene-annotation profiles and gene-parameter profiles. A general and rigorous formulation of the statistical inference question allows us to apply the multiple hypothesis testing methodology developed in [ Multiple Testing Procedures with Applications to Genomics (2008) Springer, New York] and related articles, to control a broad class of Type I error rates, defined as generalized tail probabilities and expected values for arbitrary functions of the numbers of Type I errors and rejected hypotheses. The resampling-based single-step and stepwise multiple testing procedures of [ Multiple Testing Procedures with Applications to Genomics (2008) Springer, New York] take into account the joint distribution of the test statistics and provide Type I error control in testing problems involving general data generating distributions (with arbitrary dependence structures among variables), null hypotheses, and test statistics.
The proposed statistical and computational methods are illustrated using the acute lymphoblastic leukemia (ALL) microarray dataset of [ Blood 103 (2004) 2771–2778], with the aim of relating GO annotation to differential gene expression between B-cell ALL with the BCR/ABL fusion and cytogenetically normal NEG B-cell ALL. The sensitivity of the identified lists of GO terms to the choice of association parameter between GO annotation and differential gene expression demonstrates the importance of translating the biological question in terms of suitable gene-annotation profiles, gene-parameter profiles, and association measures. In particular, the results reveal the limitations of binary gene-parameter profiles of differential expression indicators, which are still the norm for combined GO annotation and microarray data analyses. Procedures based on such binary gene-parameter profiles tend to be conservative and lack robustness with respect to the estimator for the set of differentially expressed genes. Our proposed statistical framework, with general definitions for the gene-annotation and gene-parameter profiles, allows consideration of a much broader class of inference problems, that extend beyond GO annotation and microarray data analysis.
This paper presents exploratory techniques for multivariate data, many of them well known to French statisticians and ecologists, but few well understood in North American culture. We present the general framework of duality diagrams which encompasses discriminant analysis, correspondence analysis and principal components, and we show how this framework can be generalized to the regression of graphs on covariates.
A quarter-century of statistical research has shown that census coverage surveys, valuable as they are in offering a report card on each decennial census, do not provide usable estimates of geographical differences in coverage. The determining reason is the large number of “doubly missing” people missing both from the census enumeration and from coverage survey estimates. Future coverage surveys should be designed to meet achievable goals, foregoing efforts at spatial specificity. One implication is a sample size no more than about 30,000, setting free resources for controlling processing errors and investing in coverage improvement. Possible integration of coverage measurement with the American Community Survey would have many benefits and should be given careful consideration.
Hawaiian monk seals ( Monachus schauinslandi ) are endemic to the Hawaiian Islands and are the most endangered species of marine mammal that lives entirely within the jurisdiction of the United States. The species numbers around 1300 and has been declining owing, among other things, to poor juvenile survival which is evidently related to poor foraging success. Consequently, data have been collected recently on the foraging habitats, movements, and behaviors of monk seals throughout the Northwestern and main Hawaiian Islands.
Our work here is directed to exploring a data set located in a relatively shallow offshore submerged bank (Penguin Bank) in our search of a model for a seal’s journey. The work ends by fitting a stochastic differential equation (SDE) that mimics some aspects of the behavior of seals by working with location data collected for one seal. The SDE is found by developing a time varying potential function with two points of attraction. The times of location are irregularly spaced and not close together geographically, leading to some difficulties of interpretation. Synthetic plots generated using the model are employed to assess its reasonableness spatially and temporally. One aspect is that the animal stays mainly southwest of Molokai. The work led to the estimation of the lengths and locations of the seal’s foraging trips.
This paper develops projection pursuit for discrete data using the discrete Radon transform. Discrete projection pursuit is presented as an exploratory method for finding informative low dimensional views of data such as binary vectors, rankings, phylogenetic trees or graphs. We show that for most data sets, most projections are close to uniform. Thus, informative summaries are ones deviating from uniformity. Syllabic data from several of Plato’s great works is used to illustrate the methods. Along with some basic distribution theory, an automated procedure for computing informative projections is introduced.
When a defendant’s DNA matches a sample found at a crime scene, how compelling is the match? To answer this question, DNA analysts typically use relative frequencies, random-match probabilities or likelihood ratios. They compute these quantities for the major racial or ethnic groups in the United States, supplying prosecutors with such mind-boggling figures as “one in nine hundred and fifty sextillion African Americans, one in one hundred and thirty septillion Caucasians, and one in nine hundred and thirty sextillion Hispanics.” In People v. Prince , a California Court of Appeals rejected this practice on the theory that only the perpetrator’s race is relevant to the crime; hence, it is impermissible to introduce statistics about other races. This paper critiques this reasoning. Relying on the concept of likelihood, it presents a logical justification for referring to a range of races and identifies some problems with the one-race-only rule. The paper also notes some ways to express the probative value of a DNA match quantitatively without referring to variations in DNA profile frequencies among races or ethnic groups.
Statistical tests of earthquake predictions require a null hypothesis to model occasional chance successes. To define and quantify ‘chance success’ is knotty. Some null hypotheses ascribe chance to the Earth: Seismicity is modeled as random. The null distribution of the number of successful predictions – or any other test statistic – is taken to be its distribution when the fixed set of predictions is applied to random seismicity. Such tests tacitly assume that the predictions do not depend on the observed seismicity. Conditioning on the predictions in this way sets a low hurdle for statistical significance. Consider this scheme: When an earthquake of magnitude 5.5 or greater occurs anywhere in the world, predict that an earthquake at least as large will occur within 21 days and within an epicentral distance of 50 km. We apply this rule to the Harvard centroid-moment-tensor (CMT) catalog for 2000–2004 to generate a set of predictions. The null hypothesis is that earthquake times are exchangeable conditional on their magnitudes and locations and on the predictions–a common “nonparametric” assumption in the literature. We generate random seismicity by permuting the times of events in the CMT catalog. We consider an event successfully predicted only if (i) it is predicted and (ii) there is no larger event within 50 km in the previous 21 days. The P -value for the observed success rate is <0.001: The method successfully predicts about 5% of earthquakes, far better than ‘chance,’ because the predictor exploits the clustering of earthquakes – occasional foreshocks – which the null hypothesis lacks. Rather than condition on the predictions and use a stochastic model for seismicity, it is preferable to treat the observed seismicity as fixed, and to compare the success rate of the predictions to the success rate of simple-minded predictions like those just described. If the proffered predictions do no better than a simple scheme, they have little value.
It has been widely realized that Monte Carlo methods (approximation via a sample ensemble) may fail in large scale systems. This work offers some theoretical insight into this phenomenon in the context of the particle filter. We demonstrate that the maximum of the weights associated with the sample ensemble converges to one as both the sample size and the system dimension tends to infinity. Specifically, under fairly weak assumptions, if the ensemble size grows sub-exponentially in the cube root of the system dimension, the convergence holds for a single update step in state-space models with independent and identically distributed kernels. Further, in an important special case, more refined arguments show (and our simulations suggest) that the convergence to unity occurs unless the ensemble grows super-exponentially in the system dimension. The weight singularity is also established in models with more general multivariate likelihoods, e.g. Gaussian and Cauchy. Although presented in the context of atmospheric data assimilation for numerical weather prediction, our results are generally valid for high-dimensional particle filters.
We present a theory of point and interval estimation for nonlinear functionals in parametric, semi-, and non-parametric models based on higher order influence functions (Robins (2004), Section 9; Li et al. (2004), Tchetgen et al. (2006), Robins et al. (2007)). Higher order influence functions are higher order U-statistics. Our theory extends the first order semiparametric theory of Bickel et al. (1993) and van der Vaart (1991) by incorporating the theory of higher order scores considered by Pfanzagl (1990), Small and McLeish (1994) and Lindsay and Waterman (1996). The theory reproduces many previous results, produces new non-$\sqrt{n}$ results, and opens up the ability to perform optimal non-$\sqrt{n}$ inference in complex high dimensional models. We present novel rate-optimal point and interval estimators for various functionals of central importance to biostatistics in settings in which estimation at the expected $\sqrt{n}$ rate is not possible, owing to the curse of dimensionality. We also show that our higher order influence functions have a multi-robustness property that extends the double robustness property of first order influence functions described by Robins and Rotnitzky (2001) and van der Laan and Robins (2003).

KEYWORDS/PHRASES
Publication title:, publication years.

- Why Study Statistics?
- Descriptive & Inferential Statistics
- Fundamental Elements of Statistics
- Quantitative and Qualitative Data
- Measurement Data Levels
- Collecting Data
- Ethics in Statistics
- Describing Qualitative Data
- Describing Quantitative Data
- Stem-and-Leaf Plots
- Measures of Central Tendency
- Measures of Variability
- Describing Data using the Mean and Standard Deviation
- Measures of Position
- Counting Techniques
- Simple & Compound Events
- Independent and Dependent Events
- Mutually Exclusive and Non-Mutually Exclusive Events
- Permutations and Combinations
- Normal Distribution
- Central Limit Theorem
- Confidence Intervals
- Determining the Sample Size
- Hypothesis Testing
- Hypothesis Testing Process
The field of statistics is the science of learning from data. Statistical knowledge helps you use the proper methods to collect the data, employ the correct analyses, and effectively present the results. Statistics is a crucial process behind how we make discoveries in science, make decisions based on data, and make predictions. Statistics allows you to understand a subject much more deeply.

Statistics is an exciting field about the thrill of discovery, learning, and challenging your assumptions. Statistics facilitates the creation of new knowledge. Bit by bit, we push back the frontier of what is known.

- << Previous: Statistics
- Next: Descriptive & Inferential Statistics >>
- Last Updated: Apr 20, 2023 12:47 PM
- URL: https://libraryguides.centennialcollege.ca/c.php?g=717168
Help | Advanced Search
Mathematics > Probability
Title: probability and statistics: essays in honor of david a. freedman.
Abstract: This volume is our tribute to David A. Freedman, whom we regard as one of the great statisticians of our time. He received his this http URL . degree from McGill University and his Ph.D. from Princeton, and joined the Department of Statistics of the University of California, Berkeley, in 1962, where, apart from sabbaticals, he has been ever since. In a career of over 45 years, David has made many fine contributions to probability and statistical theory, and to the application of statistics. His early research was on Markov chains and martingales, and two topics with which he has had a lifelong fascination: exchangeability and De Finetti's theorem, and the consistency of Bayes estimates. His asymptotic theory for the bootstrap was also highly influential. David was elected to the American Academy of Arts and Sciences in 1991, and in 2003 he received the John J. Carty Award for the Advancement of Science from the U.S. National Academy of Sciences. In addition to his purely academic research, David has extensive experience as a consultant, including working for the Carnegie Commission, the City of San Francisco, and the Federal Reserve, as well as several Departments of the U.S. Government--Energy, Treasury, Justice, and Commerce. He has testified as an expert witness on statistics in a number of law cases, including Piva v. Xerox (employment discrimination), Garza v. County of Los Angeles (voting rights), and New York v. Department of Commerce (census adjustment). Lastly, he is an exceptionally good writer and teacher, and his many books and review articles are arguably his most important contribution to our subject.
Submission history
Access paper:.
- Other Formats
References & Citations
- Google Scholar
- Semantic Scholar
BibTeX formatted citation

Bibliographic and Citation Tools
Code, data and media associated with this article, recommenders and search tools.
- Institution
arXivLabs: experimental projects with community collaborators
arXivLabs is a framework that allows collaborators to develop and share new arXiv features directly on our website.
Both individuals and organizations that work with arXivLabs have embraced and accepted our values of openness, community, excellence, and user data privacy. arXiv is committed to these values and only works with partners that adhere to them.
Have an idea for a project that will add value for arXiv's community? Learn more about arXivLabs .
Statistics, Its Importance and Application Essay
Importance of statistics, examples of how statistics can be used.
Statistics is a science that helps businesses in decision-making. It entails the collection of data, tabulation, and inference making. In essence, Statistics is widely used in businesses to make forecasts, research on the market conditions, and ensure the quality of products. The importance of statistics is to determine the type of data required, how it is collected, and the way it is analyzed to get factual answers.
Statistics is the collection of numerical facts and figures on such things as population, education, economy, incomes, etc. Figures collected are referred to as data. The collection, analysis, and interpretation of data are referred to as statistical methods (Lind, Marchal, & Wathen, 2011).
Two subdivisions of the statistical method are:
- Descriptive statistics: Deals with compilation and presentation of data in various forms such as tables, graphs, and diagrams from which conclusions can be drawn and decisions made. Businesses, for example, use descriptive statistics when presenting their annual accounts and reports.
- Mathematical/inferential/inductive statistics: This deals with the tools of statistics. These are the techniques that are used to analyze, make estimates, inferences, and conclude the data collected (McClave, Benson, & Sincish, 2011).
Statistics have been collected since the earliest times in history. Rulers needed to have data on population and wealth so that taxes could be levied to maintain the state and the courts. Details on the composition of the population were necessary to determine the strength of the nation. With the growth of the population and the advent of the industrial revolution in the 18 th and 19 th centuries, there was a need for greater volumes of statistics in an increasing variety of subjects such as production, expenditure, incomes, imports, and exports. In the 19 th and 20 th centuries, governments worldwide took more control in economic activities such as education and health. This led to the enormous expansion of statistics collected by governments (Lind, Marchal, & Wathen, 2011).
The government’s economic activities have expanded in the last three centuries and so have the companies/businesses grown, as well. Indeed, some have grown to such an extent that their annual turnover is greater than the annual budgets of some governments. Big firms have to make decisions based on data. The companies collect data on their own other than these sources to establish:
- Competition
- Customer needs
- Production and personnel costs
- Accounting reports on liabilities, assets, losses, and income
The tools of statistics are important for companies in areas such as planning, forecasting, and quality control (McClave, Benson, & Sincish, 2011).
To Ensure Quality
A continuous check into quality using programs is very helpful in ensuring that only quality products come out of production firms. This, in turn, ensures that there is minimum wastage or errors in the production of goods and services (McClave, Benson, & Sincish, 2011).
Making Connections
Statistics are good in revealing relationships between variables – a good example is when a company makes a close relationship between the numbers of dissatisfied customers and the turnover. Indeed, there is an inverse relationship between the number of dissatisfied customers and turnover.
Backing Judgment
With only a small sample of the population studied, the management can come up with a concrete understanding of how the customers will relate to their products. This, therefore, will help them decide on whether to or not continue with that line of production (Lind, Marchal, & Wathen, 2011).
Lind, D., Marchal, G., & Wathen, A. (2011). Basic statistics for business and economics (7 th ed.). New York, NY: McGraw-Hill/Irwin.
McClave, T., Benson, G., & Sincish, T. (2011). Statistics for business and economics (11 th ed.). Boston, MA: Pearson-Prentice Hall.
- Chicago (A-D)
- Chicago (N-B)
IvyPanda. (2024, January 30). Statistics, Its Importance and Application. https://ivypanda.com/essays/statistics-its-importance-and-application/
"Statistics, Its Importance and Application." IvyPanda , 30 Jan. 2024, ivypanda.com/essays/statistics-its-importance-and-application/.
IvyPanda . (2024) 'Statistics, Its Importance and Application'. 30 January.
IvyPanda . 2024. "Statistics, Its Importance and Application." January 30, 2024. https://ivypanda.com/essays/statistics-its-importance-and-application/.
1. IvyPanda . "Statistics, Its Importance and Application." January 30, 2024. https://ivypanda.com/essays/statistics-its-importance-and-application/.
Bibliography
IvyPanda . "Statistics, Its Importance and Application." January 30, 2024. https://ivypanda.com/essays/statistics-its-importance-and-application/.
- Descriptive Statistics and Probability
- Descriptive Statistics in Nursing
- Descriptive Statistics Method: Household Income Analysis
- Hypothesis Testing in Practical Statistics
- Applied Statistics for Healthcare Professionals
- Time Series and Causal Models in Forecasting
- Study Hours and Grades in Educational Institutions
- The Repeated-Measures ANOVA in a General Context

COMMENTS
This statistics essay sample is going to cover what I have learned in statistics and probability. Essay will talk about my experience of learning the necessary skills for analyzing data and predicting possible outcomes. Reflection Paper about Statistics and Probability: Introduction.
I now have a clear understanding of statistical applications. I know how to collect, organize and describe data. I can apply measures of central tendency such as mean, mode and median in data description. I can also use measures of dispersion such as standard deviation and variance to describe data.
Instead, it was the idea of statistics that bummed me out. What I loved about physics were its laws. They were timeless. They were eternal. Most of all, I believed they fully and exactly ...
Personally this Statistics class has taught me things I have never thought of. For instance, the first lesson taught me that statistics is composed of collecting, organizing, summarizing, analyzing, and interpreting data of a population. Another thing I've learned in chapter 2 is that graph...
Unit 7: Probability. 0/1600 Mastery points. Basic theoretical probability Probability using sample spaces Basic set operations Experimental probability. Randomness, probability, and simulation Addition rule Multiplication rule for independent events Multiplication rule for dependent events Conditional probability and independence.
Without statistics we have no way of making an educated decision between the two possibilities. Statistics, however, provides us with a tool to make an educated decision. We will be able to decide which of the two possibilities is more likely to be true. We will base this decision on our knowledge of probability and inferential statistics.
The utilization of probability concepts is a manner of articulating knowledge or conviction that an incident will happen or has taken place (Anderson, Sweeney, Williams, Camm, & Cochran, 2013). Such concepts have been offered a precise mathematical significance in the probability theory, which is employed broadly in fields such as statistics ...
We provide the essential underpinnings of probability necessary to understand the meaning and justification of statistical methods. Statistics uses probability to describe and draw inferences from data. However, there are myriad ways that statistics are misunderstood and even abused—lies, damn lies, and statistics.
Probability tells us how often some event will happen after many repeated trials. You've experienced probability when you've flipped a coin, rolled some dice, or looked at a weather forecast. Go deeper with your understanding of probability as you learn about theoretical, experimental, and compound probability, and investigate permutations, combinations, and more!
For example, when deciding on the marketing strategy of the business, statistics helps in getting the actual data that can be used to advertise the products of a business. Pages: 3. Words: 925. We will write a custom essay specifically for you. for only 11.00 9.35/page. 809 certified writers online.
Statistics is a crucial process behind how we make discoveries in science, make decisions based on data, and make predictions. Statistics allows you to understand a subject much more deeply. In this post, I cover two main reasons why studying the field of statistics is crucial in modern society. First, statisticians are guides for learning from ...
In this chapter, you will learn how to solve probability problems using a systematic approach. 3.2: Terminology In this module we learned the basic terminology of probability. The set of all possible outcomes of an experiment is called the sample space. Events are subsets of the sample space, and they are assigned a probability that is a number ...
Table of contents. Step 1: Write your hypotheses and plan your research design. Step 2: Collect data from a sample. Step 3: Summarize your data with descriptive statistics. Step 4: Test hypotheses or make estimates with inferential statistics.
Published: April 9, 2024. 1. Introduction. This essay is based on my Unit Three Statistical Learning and Thinking course reflection paper. This course was an important step for me in learning to properly conduct research and to be able to validate the results. This is important because proper statistical analysis can lead to more meaningful ...
probability and statistics, the branches of mathematics concerned with the laws governing random events, including the collection, analysis, interpretation, and display of numerical data.Probability has its origin in the study of gambling and insurance in the 17th century, and it is now an indispensable tool of both social and natural sciences. . Statistics may be said to have its origin in ...
Statistics is the field that can help us understand how to use this data to do the following things: Gain a better understanding of the world around us. Make decisions using data. Make predictions about the future using data. In this article we share 10 reasons for why the field of statistics is so important in modern life.
Statistics can help to combat the crime in our cities. Making communities safer is a benefit from this technology that is growing in the world. I learned that there is a program that tracks crime in cities and displays it for citizens to look at and stay safe around their neighborhoods. This is a great safety feature that is powered by statistics.
The result is a quite remarkable body of research on the theory and application of statistics, particularly to the decennial U.S. census, the social sciences (especially econometrics, political science and the law), and epidemiology. These themes are reflected in this volume of papers by friends and colleagues of David's.
Statistics allows you to understand a subject much more deeply. There are two main reasons why studying the field of statistics is crucial in modern society. First, statisticians are guides for learning from data and navigating common problems that can lead you to incorrect conclusions. Second, given the growing importance of decisions and ...
On a daily basis, people collect and analyze a lot of information presented in numbers, and it is closely associated with different aspects of their lives. Thus, it is typical to apply the elementary statistical approaches to examining the learned material about everyday activities. It can help to get the average results in relation to actual ...
This is the fourth of seven courses in the Google Advanced Data Analytics Certificate. In this course, you'll discover how data professionals use statistics to analyze data and gain important insights. You'll explore key concepts such as descriptive and inferential statistics, probability, sampling, confidence intervals, and hypothesis testing.
5 Intuitions and learning dificulties in probability. Successful probability teaching requires foreseeing the infor-mal ideas and dificulties that students bring to the class-room. Part of the research on this area continues previous psychological studies on reasoning biases summarised in Gilovich et al. (2002).
In a career of over 45 years, David has made many fine contributions to probability and statistical theory, and to the application of statistics. His early research was on Markov chains and martingales, and two topics with which he has had a lifelong fascination: exchangeability and De Finetti's theorem, and the consistency of Bayes estimates.
Statistics is a science that helps businesses in decision-making. It entails the collection of data, tabulation, and inference making. In essence, Statistics is widely used in businesses to make forecasts, research on the market conditions, and ensure the quality of products. The importance of statistics is to determine the type of data ...