Numbers, Facts and Trends Shaping Your World
Read our research on:
Full Topic List

Regions & Countries
- Publications
- Our Methods
- Short Reads
- Tools & Resources
Read Our Research On:
How Americans view electric vehicles
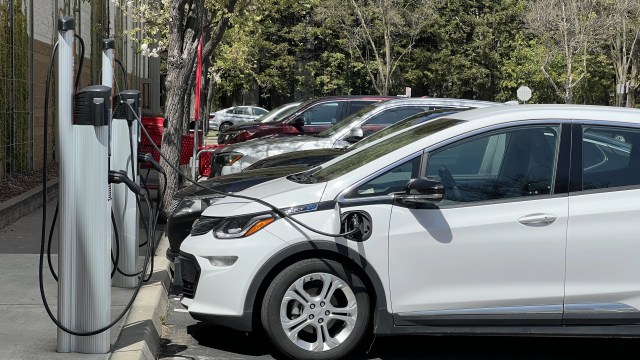
About four-in-ten Americans (38%) say they’re very or somewhat likely to seriously consider an electric vehicle (EV) for their next vehicle purchase, according to a recent Pew Research Center survey .
Pew Research Center conducted this study to understand Americans’ views on electric vehicles. We surveyed 10,329 U.S. adults from May 30 to June 4, 2023.
Everyone who took part in the survey is a member of the Center’s American Trends Panel (ATP), an online survey panel that is recruited through national, random sampling of residential addresses. This way, nearly all U.S. adults have a chance of selection. The survey is weighted to be representative of the U.S. adult population by gender, race, ethnicity, partisan affiliation, education and other categories. Read more about the ATP’s methodology .
Here are the questions used for this analysis, along with responses, and its methodology .
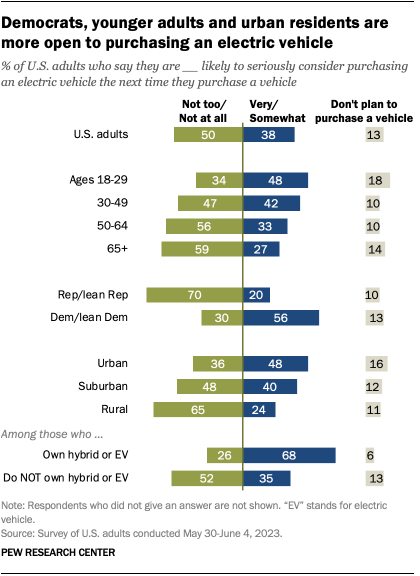
Half of U.S. adults say they are not too or not at all likely to consider purchasing an EV, while another 13% say they do not plan to purchase a vehicle. The share of the public interested in purchasing an EV is down 4 percentage points from May 2022.
Over the past year, the Biden administration has announced a range of measures aimed at increasing EV adoption, including tax credits for EV buyers and emissions limits for car manufacturers. Major automakers are increasing EV production , and electric vehicles’ share of all new U.S. car sales rose sharply over the past two years, to 8.5%.
Democrats and Democratic-leaning independents, younger adults, and people living in urban areas are among the most likely to say they would consider purchasing an EV. The 9% of U.S. adults who currently own a hybrid or electric vehicle are also particularly likely to consider an EV for their next purchase. A majority of this group (68%) says they are very or somewhat likely to seriously consider it.
Among those who would consider purchasing an EV, about seven-in-ten say helping the environment (72%) and saving money on gas (70%) are major reasons why. A small share (12%) cite keeping up with the latest trends in vehicles as a major reason.
Expectations for future electric vehicle infrastructure
One potential obstacle to greater EV adoption is the availability of public charging stations.
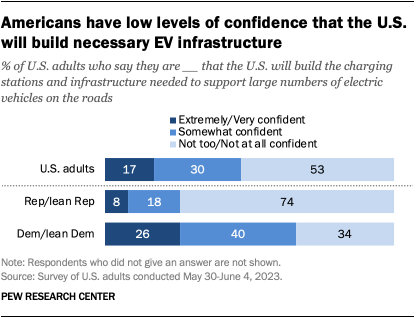
Currently, most EV owners charge their vehicles at home . Some who have used public chargers find that they are unreliable or limited in number. In September 2022, the Biden administration set aside $5 billion to create a network of EV charging stations .
Americans express limited confidence that the country will build the necessary infrastructure to support large numbers of EVs on the roads. Some 17% say they are extremely or very confident this will happen, while 30% are somewhat confident. And 53% are not too or not at all confident.
Republicans and GOP leaners are especially likely to doubt that the U.S. will build the charging stations and infrastructure needed to support EVs: 74% say they have not too much or no confidence at all in this. By comparison, 34% of Democrats and Democratic leaners say the same.
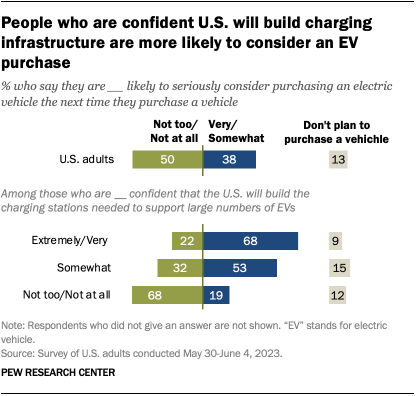
Americans who are confident the country will build the necessary infrastructure are more likely than others to say they would consider purchasing an EV.
Among those who are extremely or very confident that the U.S. will build the infrastructure needed to support EVs, 68% say they would be at least somewhat likely to consider purchasing an EV.
Just 19% of those who are not too or not at all confident in future EV infrastructure say they are at least somewhat likely to consider purchasing an EV.
Views on phasing out gasoline cars and trucks
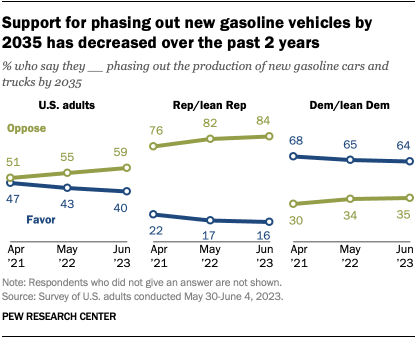
Accelerating the transition to EVs is a central part of President Joe Biden’s climate agenda. The administration has proposed new emission limits for automakers that would reduce the number of gas-powered cars and trucks they could sell. Some states have gone further, with plans to ban new gas-powered car sales by 2035.
However, the idea of phasing out the production of new gas-powered vehicles by 2035 faces more public opposition than support. About six-in-ten Americans (59%) say they oppose this, while 40% favor it.
The share of Americans who favor phasing out gas-powered vehicles has declined 7 points since 2021. Support is down among both Democrats and Republicans.
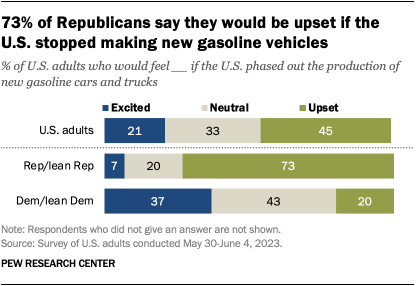
Currently, a majority of Democrats (64%) favor phasing out production of gas-powered vehicles by 2035, but 84% of Republicans oppose this.
Partisans also have different emotional reactions to the idea of ending gas-powered vehicle production. A clear majority of Republicans (73%) say they would feel upset about it, but views among Democrats are more mixed. Some 37% say they would feel excited, while 43% would feel neutral and 20% would be upset.
Note: This is an update to a post originally published June 3, 2021. Here are the questions used for this analysis, along with responses, and its methodology .
- Partisanship & Issues
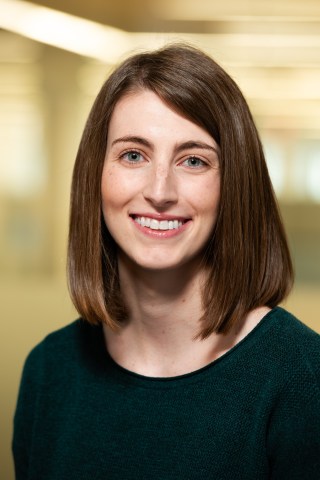
Alison Spencer is a former research analyst focusing on science and society research at Pew Research Center .
Stephanie Ross is is a former intern focusing on science and society research at Pew Research Center .
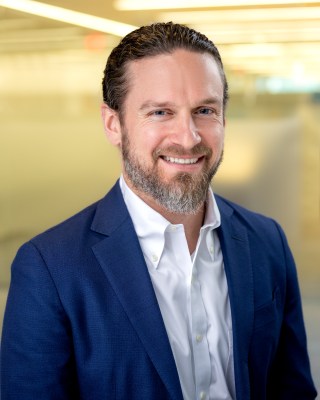
Alec Tyson is an associate director of research at Pew Research Center .
Electric Vehicle Charging Infrastructure in the U.S.
How republicans view climate change and energy issues, growing share of americans favor more nuclear power, why some americans do not see urgency on climate change, what the data says about americans’ views of climate change, most popular.
1615 L St. NW, Suite 800 Washington, DC 20036 USA (+1) 202-419-4300 | Main (+1) 202-857-8562 | Fax (+1) 202-419-4372 | Media Inquiries
Research Topics
- Email Newsletters
ABOUT PEW RESEARCH CENTER Pew Research Center is a nonpartisan fact tank that informs the public about the issues, attitudes and trends shaping the world. It conducts public opinion polling, demographic research, media content analysis and other empirical social science research. Pew Research Center does not take policy positions. It is a subsidiary of The Pew Charitable Trusts .
© 2024 Pew Research Center
Accessibility Links
- Skip to content
- Skip to search IOPscience
- Skip to Journals list
- Accessibility help
- Accessibility Help
Click here to close this panel.
Purpose-led Publishing is a coalition of three not-for-profit publishers in the field of physical sciences: AIP Publishing, the American Physical Society and IOP Publishing.
Together, as publishers that will always put purpose above profit, we have defined a set of industry standards that underpin high-quality, ethical scholarly communications.
We are proudly declaring that science is our only shareholder.
The rise of electric vehicles—2020 status and future expectations
Matteo Muratori 13,1 , Marcus Alexander 2 , Doug Arent 1 , Morgan Bazilian 3 , Pierpaolo Cazzola 4 , Ercan M Dede 5 , John Farrell 1 , Chris Gearhart 1 , David Greene 6 , Alan Jenn 7 , Matthew Keyser 1 , Timothy Lipman 8 , Sreekant Narumanchi 1 , Ahmad Pesaran 1 , Ramteen Sioshansi 9 , Emilia Suomalainen 10 , Gil Tal 7 , Kevin Walkowicz 11 and Jacob Ward 12
Published 25 March 2021 • © 2021 IOP Publishing Ltd Progress in Energy , Volume 3 , Number 2 Focus on Transport Electrification Citation Matteo Muratori et al 2021 Prog. Energy 3 022002 DOI 10.1088/2516-1083/abe0ad
Article metrics
74015 Total downloads
Permissions
Get permission to re-use this article
Share this article
Author e-mails.
Author affiliations
1 National Renewable Energy Laboratory, Golden, CO, United States of America
2 Electric Power Research Institute, Palo Alto, CA, United States of America
3 Colorado School of Mines, Golden, CO, United States of America
4 International Transport Forum in Paris, France
5 Toyota Research Institute of North America, Ann Arbour, MI, United States of America
6 University of Tennessee, Knoxville, TN, United States of America
7 University of California, Davis, CA, United States of America
8 University of California, Berkeley, CA, United States of America
9 The Ohio State University, Columbus, OH, United States of America
10 Institut VEDECOM, Versailles, France
11 Calstart, Pasadena, CA, United States of America
12 Carnegie Mellon University, Pittsburgh, PA, United States of America
Author notes
13 Author to whom any correspondence should be addressed.
Matteo Muratori https://orcid.org/0000-0003-1688-6742
Doug Arent https://orcid.org/0000-0002-4219-3950
Morgan Bazilian https://orcid.org/0000-0003-1650-8071
Ahmad Pesaran https://orcid.org/0000-0003-0666-1021
Emilia Suomalainen https://orcid.org/0000-0002-6339-2932
Gil Tal https://orcid.org/0000-0001-7843-3664
Jacob Ward https://orcid.org/0000-0002-8278-8940
- Received 3 August 2020
- Accepted 27 January 2021
- Published 25 March 2021
Peer review information
Method : Single-anonymous Revisions: 3 Screened for originality? Yes
Buy this article in print
Electric vehicles (EVs) are experiencing a rise in popularity over the past few years as the technology has matured and costs have declined, and support for clean transportation has promoted awareness, increased charging opportunities, and facilitated EV adoption. Suitably, a vast body of literature has been produced exploring various facets of EVs and their role in transportation and energy systems. This paper provides a timely and comprehensive review of scientific studies looking at various aspects of EVs, including: (a) an overview of the status of the light-duty-EV market and current projections for future adoption; (b) insights on market opportunities beyond light-duty EVs; (c) a review of cost and performance evolution for batteries, power electronics, and electric machines that are key components of EV success; (d) charging-infrastructure status with a focus on modeling and studies that are used to project charging-infrastructure requirements and the economics of public charging; (e) an overview of the impact of EV charging on power systems at multiple scales, ranging from bulk power systems to distribution networks; (f) insights into life-cycle cost and emissions studies focusing on EVs; and (g) future expectations and synergies between EVs and other emerging trends and technologies. The goal of this paper is to provide readers with a snapshot of the current state of the art and help navigate this vast literature by comparing studies critically and comprehensively and synthesizing general insights. This detailed review paints a positive picture for the future of EVs for on-road transportation, and the authors remain hopeful that remaining technology, regulatory, societal, behavioral, and business-model barriers can be addressed over time to support a transition toward cleaner, more efficient, and affordable transportation solutions for all.
Export citation and abstract BibTeX RIS
This article was updated on 29 April 2021 to add the name of the fifth author and to correct the name of the eighth author.
1. Introduction
First introduced at the end of the 1800s, electric vehicles (EVs) 12 have been experiencing a rise in popularity over the past few years as the technology has matured and costs (especially of batteries) have declined substantially. Worldwide support for clean transportation options (i.e. low emissions of greenhouse gasses [GHG] to mitigate climate change and criteria pollutants) has promoted awareness, increased charging opportunities, and facilitated adoption of EVs. EVs present numerous advantages compared to fossil-fueled internal-combustion-engine vehicles (ICEVs), inter alia: zero tailpipe emissions, no reliance on petroleum, improved fuel economy, lower maintenance, and improved driving experience (e.g. acceleration, noise reduction, and convenient home and opportunity recharging). Further, when charged with clean electricity, EVs provide a viable pathway to reduce overall GHG emissions and decarbonize on-road transportation. This decarbonization potential is important, given limited alternative options to liquid fossil fuels. The ability of EVs to reduce GHG emissions is dependent, however, upon clean electricity. Therefore, EV success is intertwined closely with the prospect of abundant and affordable renewable electricity (in particular solar and wind electricity) that is poised to transform power systems (Jacobson et al 2015 , Kroposki et al 2017 , Gielen et al 2019 , IEA 2020b ). Coordinated actions can produce beneficial synergies between EVs and power systems and support renewable-energy integration to optimize energy systems of the future to benefit users and offer decarbonization across sectors (CEM 2020 ). A cross-sectoral approach across the entire energy system is required to realise clean future transformation pathways (Hansen et al 2019 ). EVs are expected to play a critical role in the power system of the future (Muratori and Mai).
EV success is increasing rapidly since the mid-2010s. EV sales are breaking previous records every year, especially for light-duty vehicles (LDVs), buses, and smaller vehicles such as three-wheelers, mopeds, kick-scooters, and e-bikes (IEA 2017 , 2018a , 2019 , 2020 ). To date, global automakers are committing more than $140 billion to transportation electrification, and 50 light-duty EV models are available commercially in the U.S. market (Moore and Bullard 2020 ). Approximately 130 EV models are anticipated by 2023 (AFDC 2020 , Moore and Bullard 2020 ). Future projections of the role of EVs in LDV markets vary widely, with estimates ranging from limited success (∼10% of sales in 2050) to full market dominance, with EVs accounting for 100% of LDV sales well before 2050. Many studies project that EVs will become economically competitive with ICEVs in the near future or that they are already cost-competitive for some applications (Weldon et al 2018 , Sioshansi and Webb 2019 , Yale E360 2019 , Kapustin and Grushevenko 2020 ). However, widespread adoption requires more than economic competitiveness, especially for personally owned vehicles. Behavioral and non-financial preferences of individuals on different technologies and mobility options are also important (Lavieri et al 2017 , Li et al 2017 , McCollum et al 2018 , Ramea et al 2018 ). EV adoption beyond LDVs has been focused on buses, with significant adoption in several regions (especially China). Electric trucks also are receiving great attention, and Bloomberg New Energy Finance (BloombergNEF) projects that by 2025, alternative fuels will compete with, or outcompete, diesel in long-haul trucking applications (Moore and Bullard 2020 ). These recent successes are being driven by technological progress, especially in batteries and power electronics, greater availability of charging infrastructure, policy support driven by environmental benefits, and consumer acceptance. EV adoption is engendering a virtuous circle of technology improvements and cost reductions that is enabled and constrained by positive feedbacks arising from scale and learning by doing, research and development, charging-infrastructure coverage and utilization, and consumer experience and familiarity with EVs.
Vehicle electrification is a game-changer for the transportation sector due to major energy and environmental implications driven by high vehicle efficiency (EVs are approximately 3–4 times more efficient than comparable ICEVs), zero tailpipe emissions, and reduced petroleum dependency (great fuel diversity and flexibility exist in electricity production). Far-reaching implications for vehicle-grid integration extend to the electricity sector and to the broader energy system. A revealing example of the role of EVs in broader energy-transformation scenarios is provided by Muratori and Mai, who summarize results from 159 scenarios underpinning the special report on Global Warming of 1.5 °C (SR1.5) by Intergovernmental Panel on Climate Change (IPCC). Muratori and Mai also show that transportation represents only ∼2% of global electricity demand currently (with rail responsible for more than two-thirds of this total). They show that electricity is projected to provide 18% of all transportation-energy needs by 2050 for the median IPCC scenario, which would account for 10% of total electricity demand. Most of this electricity use is targeted toward on-road vehicle electrification. These projections are the result of modeling and simulations that are struggling to keep pace with the EV revolution and its role in energy-transformation scenarios as EV technologies and mobility are evolving rapidly (McCollum et al 2017 , Venturini et al 2019 , Muratori et al 2020 ). Recent studies explore higher transportation-electrification scenarios: for example, Mai et al ( 2018 ) report a scenario in which 75% of on-road miles are powered by electricity, and transportation represents almost a quarter of total electricity use during 2050.
Vehicle electrification is a disruptive element in energy-system evolution that radically changes the roles of different sectors, technologies, and fuels in long-term transformation scenarios. Traditionally, energy-system-transformation studies project minimal end-use changes in transportation-energy use over time (limited mode shifting and adoption of alternative fuels), and the sector is portrayed as a 'roadblock' to decarbonization. In many decarbonization scenarios, transportation is seen traditionally as one of the biggest hurdles to achieve emissions reductions (The White House 2016 ). These scenarios rely on greater changes in the energy supply to reduce emissions and petroleum dependency (e.g. large-scale use of bioenergy, often coupled to carbon capture and sequestration) rather than demand-side transformations (IPCC 2014 , Pietzcker et al 2014 , Creutzig et al 2015 , Muratori et al 2017 , Santos 2017 ). In most of these studies, electrification is limited to some transportation modes (e.g. light-duty), and EVs are not expected to replace ICEVs fully (The White House 2016 ). More recently, however, major mobility disruptions (e.g. use of ride-hailing and vehicle ride-sharing) and massive EV adoption across multiple applications are proposed (Edelenbosch et al 2017 , Van Vuuren et al 2017 , Hill et al 2019 , E3 2020 , Zhang and Fujimori 2020 ). These mobility disruptions allow for more radical changes and increase the decarbonization role of transportation and highlight the integration opportunities between transportation and energy supply, especially within the electricity sector. For example, Zhang and Fujimori ( 2020 ) highlight that for vehicle electrification to contribute to climate-change mitigation, electricity generation needs to transition to clean sources. They note that EVs can reduce mitigation costs, implying a positive impact of transport policies on the economic system (Zhang and Fujimori 2020 ). In-line with these projections, many countries are establishing increasingly stringent and ambitious targets to support transport electrification and in some cases ban conventional fossil fuel vehicles (Wentland 2016 , Dhar et al 2017 , Coren 2018 , CARB 2020 , State of California 2020 ).
EV charging undoubtedly will impact the electricity sector in terms of overall energy use, demand profiles, and synergies with electricity supply. Mai et al ( 2018 ) show that in a high-electrification scenario, transportation might grow from the current 0.2% to 23% of total U.S. electricity demand in 2050 and significantly impact system peak load and related capacity costs if not controlled properly. Widespread vehicle electrification will impact the electricity system across the board, including generation, transmission, and distribution. However, expected changes in U.S. electricity demand as a result of vehicle electrification are not greater than historical growth in load and peak demand. This finding suggests that bulk-generation capacity is expected to be available to support a growing EV fleet as it evolves over time, even with high EV-market growth (U.S. DRIVE 2019 ). At the same time, many studies have shown that 'smart charging' and vehicle-to-grid (V2G) services create opportunities to reduce system costs and facilitate the integration of variable renewable energy (VRE). Charging infrastructure that enables smart charging and alignment with VRE generation, as well as business models and programs to compensate EV owners for providing charging flexibility, are the most pressing required elements for successfully integrating EVs with bulk power systems. At the local level, EV charging could increase and change electricity loads significantly, which could impact distribution networks and power quality and reliability (FleetCarma 2019 ). Distribution-network impacts can be particularly critical for high-power charging and in cases in which many EVs are concentrated in a specific location, such as clusters of residential LDV charging and possibly fleet depots for commercial vehicles (Muratori 2018 ).
This paper provides a timely status of the literature on several aspects of EV markets, technologies, and future projections. The paper focuses on multiple facets that characterize technology status and the role of EVs in transportation decarbonization and broader energy-transformation pathways focusing on the U.S. context. As appropriate, global context is provided as well. Hybrid EVs (for which liquid fuel is the only source of energy) and fuel cell EVs (that power an electric powertrain with a fuel cell and on-board hydrogen storage) have some similarities with EVs and could complement them for many applications. However, these technologies are not reviewed in detail here. The remainder of this paper is structured as follows. Section 2 focuses on the status of the light-duty-EV market and provides a comparison of projections for future adoption. Section 3 provides insights on market opportunities beyond LDVs. Section 4 offers a review of cost and performance evolution for batteries, power electronics, and electric machines that are key components of EV success. Section 5 reviews charging-infrastructure status and focuses on modeling and analysis studies used to project charging-infrastructure requirements, the economics of public charging, and some considerations on cybersecurity and future technologies (e.g. wireless charging). Section 6 provides an overview of the impact of EV charging on power systems at multiple scales, ranging from bulk power systems to distribution networks. Section 7 provides insights into life-cycle cost and emissions studies focusing on EVs. Finally, section 8 touches on future expectations.
1.1. Summary of take-away points
1.1.1. ev adoption.
- The global rate of adoption of light-duty EVs (passenger cars) has increased rapidly since the mid-2010s, supported by three key pillars: improvements in battery technologies; a wide range of supportive policies to reduce emissions; and regulations and standards to promote energy efficiency and reduce petroleum consumption.
- Adoption of advanced technologies has been underestimated historically in modeling and analyses; EV adoption is projected to remain limited until 2030, and high uncertainty is shown afterward with widely different projections from different sources. However, EVs hold great promise to replace conventional LDVs affordably.
- Barriers to EV adoption to date include consumer skepticism toward new technology, high purchase prices, limited range and lack of charging infrastructure, and lack of available models and other supply constraints.
- A major challenge facing both manufacturers and end-users of medium- and heavy-duty EVs is the diverse set of operational requirements and duty cycles that the vehicles encounter in real-world operation.
- EVs appear to be well suited for short-haul trucking applications such as regional and local deliveries. The potential for battery-electric models to work well in long-haul on-road applications has yet to be established, with different studies indicating different opportunities.
1.1.2. Batteries and other EV technologies
- Over the last 10 years, the price of lithium-ion battery packs has dropped by more than 80% (from over $1000 kWh −1 to $156 kWh −1 at the end of 2019, BloombergNEF 2020 ). Further price reduction is needed to achieve EV purchase-price parity with ICEVs.
- Over the last 10 years, the specific energy of a lithium-ion battery cell has almost doubled, reaching 240 Wh kg −1 (BloombergNEF 2020 ), reducing battery weight significantly.
- Reducing or eliminating cobalt in lithium-ion batteries is an opportunity to lower costs and reduce reliance on a rare material with controversial supply chains.
- While batteries are playing a key role in the rise of EVs, power electronics and electric motors are also key components of an EV powertrain. Recent trends toward integration promise to deliver benefits in terms of increased power density, lower losses, and lower costs.
1.1.3. Charging infrastructure
- With a few million light-duty EVs on the road, currently, there is about one public charge point per ten battery electric vehicles (BEVs) in U.S. (although most vehicles have access to a residential charger).
- Given the importance of home charging (and the added convenience compared to traditional refueling at public stations), charging solutions in residential areas comprising attached or multi-unit dwellings is likely to be essential for EVs to be adopted at large scale.
- Although public charging infrastructure is clearly important to EV purchasers, how best to deploy charging infrastructure in terms of numbers, types, locations, and timing remains an active area for research.
- The economics of public charging vary with location and station configuration and depend critically on equipment and installation costs, incentives, non-fuel revenues, and retail electricity prices, which are heavily dependent on station utilization.
- The electrification of medium- and heavy-duty commercial trucks and buses might introduce unique charging and infrastructure requirements compared to those of light-duty passenger vehicles.
- Wireless charging, specifically high-power wireless charging (beyond level-2 power), could play a key role in providing an automated charging solution for tomorrow's automated vehicles.
1.1.4. Power system integration
- Accommodating EV charging at the bulk power-system level (generation and transmission) is different in each region, but there are no major known technical challenges or risks to support a growing EV fleet, especially in the near term (approximately one decade).
- At the local level, however, EV charging can increase and change electricity loads significantly, causing possible negative impacts on distribution networks, especially for high-power charging.
- The integration of EVs into power systems presents opportunities for synergistic improvement of the efficiency and economics of electromobility and electric power systems, and EVs can support grid planning and operations in several ways.
- There are still many challenges for effective EV-grid integration at large scale, linked not only to the technical aspects of vehicle-grid-integration (VGI) technology but also to societal, economic, business model, security, and regulatory aspects.
- VGI offers many opportunities that justify the efforts required to overcome these challenges. In addition to its services to the power system, VGI offers interesting perspectives for the full exploitation of synergies between EVs and VRE as both technologies promise large-scale deployment in the future.
1.1.5. Life-cycle cost and emissions
- Many factors contribute to variability in EV life-cycle emissions, mostly the carbon intensity of electricity, charging patterns, vehicle characteristics, and even local climate. Grid decarbonization is a prerequisite for EVs to provide major GHG-emissions reductions.
- Existing literature suggests that future EVs can offer 70%–90% lower GHG emissions compared to today's ICEVs, most obviously due to broad expectations for continued grid decarbonization.
- Operational costs of EVs (fuel and maintenance) are typically lower than those of ICEVs, largely because EVs are more efficient than ICEVs and have fewer moving parts.
1.1.6. Synergies with other technologies and future expectations
- Vehicle electrification fits in broader electrification and mobility macro-trends, including micro-mobility in urban areas, new mobility business models regarding ride-hailing and car-sharing, and automation that complement well with EVs.
- While EVs are a relatively new technology and automated vehicles are not readily available to the general public, the implications and potential synergies of these technologies operating in conjunction are significant.
- The coronavirus pandemic is impacting transportation markets negatively, including those for EVs, but long-term prospects remain undiminished.
- Several studies project major roles for EVs in the future, which is reflected in massive investment in vehicle development and commercialization, charging infrastructure, and further technology improvement. Consumer adoption and acceptance and technology progress form a virtuous self-reinforcing circle of technology-component improvements and cost reductions.
- EVs hold great promise to replace ICEVs affordably for a number of on-road applications, eliminating petroleum dependence, improving local air quality and enabling GHG-emissions reductions, and improving driving experiences.
- Forecasting the future, including technology adoption, remains a daunting task. However, this detailed review paints a positive picture for the future of EVs across a number of on-road applications.
2. Status of electric-LDV market and future projections
This section provides a current snapshot of the electric-LDV market in a global and U.S. context, but focuses on the latter. The global rate of adoption of electric LDVs has increased rapidly since the mid-2010s 13 . By the end of 2019, the global EV fleet reached 7.3 million units—up by more than 40% from 2018—with more than 1.25 million electric LDVs in the U.S. market alone (IEA 2020 ). EV sales totaled more than 2.2 million in 2019, exceeding the record level that was attained in 2018, despite mixed performances in different markets. Electric-LDV sales increased in Europe and stagnated or declined in other major markets, particularly in China (with a significant slowdown due to changes in Chinese subsidy policy in July 2019), Japan, and U.S. U.S. EV adoption varies greatly geographically—nine counties in California currently see EVs accounting for more than 10% of sales (8% on average for California as a whole), but national-level sales remain at less than 3% (Bowermaster 2019 ). BEV sales exceeded those of plug-in hybrid electric vehicles (PHEVs) in all regions.
The rapid increase in EV adoption is underpinned by three key pillars:
- (a) Improvements and cost reductions in battery technologies, which were enabled initially by the large-scale application of lithium-ion batteries in consumer electronics and smaller vehicles (e.g. scooters, especially in China, IEA 2017 ). These developments offer clear and growing opportunities for EVs and HEVs to deliver a reduced total cost of ownership (TCO) in comparison with ICEVs.
- (b) A wide range of supportive policy instruments for clean transportation solutions in major global markets (Axsen et al 2020 ), which are mirrored by private-sector investment. These developments are driven by environmental goals (IPCC 2014 ), including reduction of local air pollution. These policy instruments support charging-infrastructure deployment (Bedir et al 2018 ) and provide monetary (e.g. rebates and vehicle-registration discounts) and non-monetary (e.g. access to high-occupancy-vehicle lanes and preferred parking) incentives to support EV adoption (IEA 2018a , AFDC 2020 ).
- (c) Regulations and standards that support high-efficiency transportation solutions and reduce petroleum consumption (e.g. fuel-economy standards, zero-emission-vehicle mandates, and low-carbon-fuel standards). These regulations are being supported by technology-push measures, consisting primarily of economic instruments (e.g. grants and research funds) that aim to stimulate technological progress (especially batteries), and market-pull measures (e.g. public-procurement programs) that aim to support the deployment of clean-mobility technologies and enable cost reductions due to technology learning, scale, and risk mitigation.
Transport electrification also has started a virtuous self-reinforcing circle. Battery-technology developments and cost reductions triggered by EV adoption provide significant economic-development opportunities for the companies and countries intercepting the battery and EV value chains. Adoption of alternative vehicles both is enabled and constrained by powerful positive feedback arising from scale and learning by doing, research and development, consumer experience and familiarity with technologies (e.g. neighborhood effect), and complementary resources, such as fueling infrastructure (Struben and Sterman 2008 ). In this context, more diversity in make and model market offerings is supporting vehicle adoption. As of April 2020, there are 50 EV models available commercially in U.S. markets (AFDC 2020 ), and ∼130 are anticipated by 2023 (Moore and Bullard 2020 ).
Measures that support transport electrification have been, and increasingly shall be, accompanied by policies that control for the unwanted consequences. Thus, the measures need to be framed in the broader energy and industry contexts.
When looking at the future, EV-adoption forecasts remain highly uncertain. Technology-adoption projections are used by a number of stakeholders to guide investments, inform policy design and requirements (Kavalec et al 2018 ), assess benefits of previous and ongoing efforts (Stephens et al 2016 ), and develop long-term multi-sectoral assessments (Popp et al 2010 , Kriegler et al 2014 ). However, projecting the future, including technology adoption, is a daunting task. Past projections often have turned out to be inaccurate. Still, progress has been made to address projection uncertainty (Morgan and Keith 2008 , Reed et al 2019 ) and contextualize scenarios to explore alternative futures in a useful way. Scenario analysis is used largely in the energy-environment community to explore the possible implications of different judgments and assumptions by considering a series of 'what if' experiments (BP 2019 ).
Adoption of advanced technologies historically has been underestimated in modeling and analysis results (e.g. Creutzig et al 2017 ), which fail to capture the rapid technological progress and its impact on sales. Historical experiences suggest that technology diffusion, while notoriously difficult to predict, can occur rapidly and with an extensive reach (Mai et al 2018 ). Projecting personally owned LDV sales is particularly challenging because decisions are made by billions of independent (not necessarily rational) decision-makers valuing different vehicle attributes based on incomplete information (e.g. misinformation and skepticism toward new technologies) and limited financial flexibility.
Many studies make projections for future EV sales (see figure 1 for a comparison of different projections). Some organizations (e.g. Energy Information Administration [EIA]) historically have been conservative in projecting EV success, mostly due to scenario constraints and assumptions. Still, U.S. EV-sales projections from EIA in recent years are much higher than in the past. Others (e.g. BloombergNEF and Electric Power Research Institute [EPRI]) consistently have been more optimistic in terms of EV sales and continue to adjust sales projections upward. Policy ambition for EV adoption is also optimistic. For example, in September 2020, California passed new legislation that requires that by 2035 all new car and passenger-truck sales be zero-emission vehicles (and that all medium- and heavy-duty vehicles be zero-emission by 2045) (California, 2020). Projected EV sales and outcomes from major energy companies vary widely, ranging from somewhat limited EV adoption (e.g. ExxonMobil) to full market success (e.g. Shell). A survey from Columbia University (Kah 2019 ) considers 17 studies and shows that 'EV share of the global passenger vehicle fleet is not projected to be substantial before 2030 given the long lead time in turning over the global automobile fleet' and that 'the range of EVs in the 2040 fleet is 10% to 70%'. The studies compared in figure 1 show an even greater variability for 2050 projections, ranging from 13% to 100% of U.S. EV adoption for LDVs.
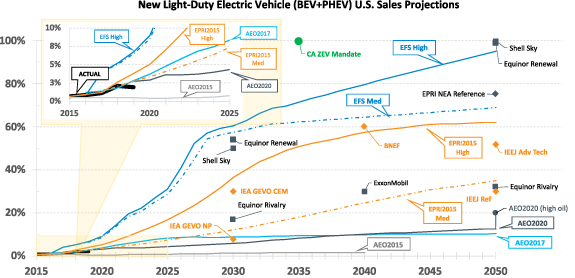
Figure 1. Electric LDV (BEV and PHEV) new sales projections from numerous international sources. Unless otherwise noted, data refer to new U.S. sales. AEO2015 = EIA Annual Energy Outlook 2015, Reference Scenario. AEO2017 = EIA Annual Energy Outlook 2017, Reference Scenario. AEO2020 = EIA Annual Energy Outlook 2020, Reference Scenario. AEO2020HO = EIA Annual Energy Outlook 2020, High Oil Scenario. EFS Med = National Renewable Energy Laboratory (NREL) Electrification Futures Study, Medium Scenario. EFS High = NREL Electrification Futures Study, High Scenario. EPRI Med = EPRI Plug-in Electric Vehicle Projections: Scenarios and Impacts, Medium Scenario. EPRI High = EPRI Plug-in Electric Vehicle Projections: Scenarios and Impacts, High Scenario. EPRI NEA = EPRI National Electrification Assessment, Reference Scenario. GEVO NP = IEA Global EV Outlook 2019, New Policies Scenario. GEVO CEM = IEA Global EV Outlook 2019, Clean Energy Ministerial 30@30 Campaign Scenario. BNEF = BloombergNEF EV Outlook 2020. Equinor Riv = Equinor 2019 Energy Perspectives, Rivalry Scenario. Equinor Ren = Equinor 2019 Energy Perspectives, Renewal Scenario. Shell Sky = Shell Sky Scenario. ExxonMobil = 2019 ExxonMobil Outlook for Energy. IEEJ Ref = The Institute of Energy Economics, Japan. 2019 Outlook, Reference Scenario (global sales). IEEJ Adv = The Institute of Energy Economics, Japan. 2019 Outlook, Advanced Technologies Scenario (global sales). CA ZEV Mandate = California zero-emission vehicle (ZEV) Executive Order N-79-20 (September 2020).
Download figure:
The future remains uncertain, but there is a clear trend in projections of light-duty EV sales toward more widespread adoption as the technology improves, consumers become more familiar with the technology, automakers expand their offerings, and policies continue to support the market.
A number of studies analyze the drivers of EV adoption (Vassileva and Campillo 2017 , Priessner et al 2018 ) and highlight several barriers for EVs to achieve widespread success, including consumer skepticism for new technologies (Egbue and Long 2012 ); uncertainty around environmental benefits (consumers wonder whether EVs actually are green; see section 7 for more clarity on the environmental benefits of EVs) and continued policy support; unclear battery aging/resale value; high costs (Haddadian et al 2015 , Rezvani et al 2015 , She et al 2017 ); lack of charging infrastructure (Melaina et al 2017 , Narassimhan and Johnson 2018 ); range anxiety (the fear of being unable to complete a trip) associated with shorter-range EVs; longer refueling times compared to conventional vehicles (Franke and Krems 2013 , Neubauer and Wood 2014 ; Melaina et al 2017 ); dismissive and deceptive car dealerships (De Rubens et al 2018 ); and other EV-supply considerations, such as limited model availability and limited supply chains.
A recent review of 239 articles published in top-tier journals focusing on EV adoption draws attention to 'relatively neglected topics such as dealership experience, charging infrastructure resilience, and marketing strategies as well as identifies much-studied topics such as charging infrastructure development, TCO, and purchase-based incentive policies' (Kumar and Alok 2019 ). Similar reviews published recently focus on different considerations, such as market heterogeneity (Lee et al 2019a ), incentives and policies (Hardman 2019 , Tal et al 2020 ), and TCO (Hamza et al 2020 ). Other than some limited discussions on business models and TCO, the literature is focused on one side of the story, namely demand. However, the availability (makes and models) of EVs is extremely limited compared to ICEVs (AFDC 2020 ). This is justified, in part, by new technologies requiring time to be introduced, and, in part, by the higher manufacturer revenues associated with selling and providing maintenance for ICEVs. Moreover, slow turnover in legacy industry (Morris 2020 ) and other supply constraints can be a major barrier to widespread EV uptake (Wolinetz and Axsen 2017 , De Rubens et al 2018 ). Kurani ( 2020 ) argues that in most cases, 'Results of large sample surveys and small sample workshops mutually reinforce the argument that continued growth of PEV markets faces a barrier in the form of the inattention to plug-in electric vehicles (PEVs) of the vast majority of car-owning and new-car-buying households even in a place widely regarded as a leader. Most car-owning households are not paying attention to PEVs or the idea of a transition to electric-drive.'
3. EVs beyond light-duty applications
While much of the recent focus on vehicle electrification is with LDVs and small two- or three-wheelers (primarily in China), major progress also is being made with the electrification of medium- and heavy-duty vehicles. This includes heavy-duty trucks of various types, urban transit buses, school buses, and medium-duty vocational vehicles. As of the end of 2019, there were about 700 000 medium- and heavy-duty commercial EVs in use around the world (EV Volumes 2020 , IEA 2020 ).
A major challenge facing both manufacturers and end-users of medium- and heavy-duty vehicles is the diverse set of operational requirements and duty cycles that the vehicles encounter in real-world operation. When designing powertrain configurations and on-board energy-storage needs for new technologies, it is of critical importance to represent vehicle behavior accurately for different operations, including possible changes triggered by electrification (Delgado-Neira 2012 ). Medium- and heavy-duty vehicles can require a large number of powertrain and battery configurations, control strategies, and charging solutions. These needs depend on vehicle type (covering the full U.S. gross vehicle weight ratings [GVWR] spectrum from class 3 to class 8, 10 001–80 000 lb [4536–36 287 kg]), commercial operational situations and activities, and diverse drive cycles and charging opportunities (e.g. depot-based operations vs. long-haul). An example of this potential variability and its effect on the required battery capacity across multiple vehicle vocations is shown in figure 2 (Smith et al 2019 ).
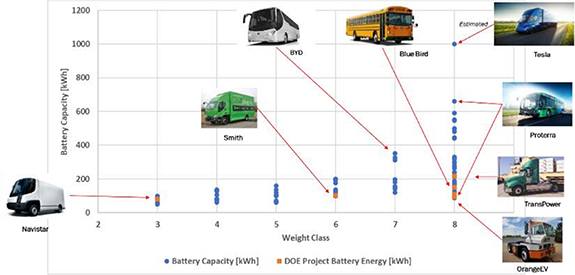
Figure 2. Battery capacity requirements vs. weight class for medium- and heavy-duty vehicles (Smith et al 2019 ).
Another example of the highly variable use cases for medium- and heavy-duty EVs shows energy efficiencies range between 0.8 kWh mile −1 and 3.2 kWh mile −1 (0.5–2.5 kWh km −1 ) (Gao et al 2018 ). If the on-board energy-storage needs for these vehicles are considered, assuming a daily operational range of between 50 miles and 200 miles (80–322 km), this results in battery-size requirements between 40 kWh and 640 kWh (assuming that the vehicle is recharged once daily). If additional charging strategies are considered (with their variability in expected charge times and associated power ratings), the range of vehicle-hardware and charging-infrastructure possibilities increases further. When adding variability across use cases with respect to temperature effects, battery-capacity degradation, payload, and road grade, it becomes clear that medium- and heavy-duty truck manufacturers face a significant challenge in designing, developing, and manufacturing systems that are able to meet the diverse operational requirements.
There are potential synergies between components of light-duty and medium- and heavy-duty electric vehicles. However, the requirements of medium- and heavy-duty vehicles place much greater burdens on powertrain components. The power and energy needs in heavy-duty applications are much larger than in light-duty applications. Heavy-duty vehicles could demand twice the peak power, four times the torque, and can consume more than five times the energy per mile (or km) driven compared to LDVs. In addition to using more energy per mile (or km) driven, typically, commercial vehicles drive many more miles (or km) per day, requiring much larger batteries and possibly much higher-power charging. Moreover, heavy-duty-vehicle users expect their vehicles to last more than a million miles, pointing to significantly higher durability requirements for heavy-duty-vehicle components (Smith et al 2019 ). Overall, these requirements, in combination with the needs for very high durability and very high-power drivelines and charging, may cause battery chemistries of heavy-duty vehicle batteries to diverge from those that are used in LDVs, hindering economies of scale. Demands for high efficiency, high power, and lower weight will put pressure on commercial vehicles to work at higher voltages than LDVs do. While LDVs are designed typically with powertrains that operate at a few hundred volts, it may be desirable to design large EVs with kilovolt powertrains. This will have a particularly significant impact on power electronics and could drive the development of wide-bandgap power electronics.
Historically, EVs have not been considered capable alternatives to heavy-duty diesel trucks (above 33 000 lb [14 969 kg] GVWR) due to high capital costs, high energy and power requirements, and weight and range-related battery constraints. International Council on Clean Transportation (ICCT), for example, suggests that while conventional EV-charging methods may be sufficient for small urban commercial vehicles, overhead catenary or in-road charging are required for heavier vehicles (Moultak et al 2017 ). Recent studies dispute this, anticipating a much greater opportunity for EVs to replace diesel trucks in the short-term, even for long-haul applications (Mai et al 2018 , McCall and Phadke 2019 , Borlaug et al Forthcoming ), but the potential for battery-electric models to work well in long-haul applications has yet to be established (NACFE 2018 ). Studies show that a significant amount of payload capacity will be consumed by batteries, potentially up to 7 tons or 28% of capacity in a truck with a 500 mile (805 km) range with 1100 kWh battery capacity (Burke and Fulton 2019 ). Thus, batteries would reduce significantly the amount of cargo that can be carried. Other studies suggest this could be much less―on the order of 4% of lost payload capacity for 500 mile range (805 km) trucks and with overall lower TCO than diesel trucks (Phadke et al 2019 ). For short-haul applications, such as port drayage and regional or local deliveries, EVs appear well suited and battery weight may not affect the cargo or payload capacity adversely. Several heavy-duty battery-electric trucks for short- and medium-haul applications have been developed and tested in recent years by Balqon, Daimler Trucks NA, Peterbilt, TransPower, Tesla, US Hybrid, Volvo, and others (AFDC 2020 ).
Urban buses are also a major emerging market for electrification. In California, Innovative Clean Transit rules require transit agencies to transition completely to zero-emission technologies (batteries or fuel cells), with all new bus purchases being zero-emission by 2029 (CARB 2018 ). Eight of the ten largest transit agencies in California already are adopting zero-emission technologies into their fleets (CARB 2018 ). In a comparative study of urban buses running on diesel, compressed natural gas, diesel hybrid, fuel cells, and batteries, the battery buses are estimated to have the lowest CO 2 emissions in both California and Finland bus duty cycles at the time of the study (Lajunen and Lipman 2016 ). This study also shows that battery buses have only slightly higher overall costs per mile (or km) than fossil-fuel-based alternatives. Future projections out to 2030 show that electric buses have the lowest overall life-cycle costs, especially when CO 2 costs are included (Lajunen and Lipman 2016 ).
Medium-duty delivery vehicles (typically 10 000–33 000 lb [4536–14 969 kg] GVWR) are another attractive emerging area for electrification. The goods-delivery market is growing at approximately 9% per year in recent years, with a projected $343 billion global industry value in 2020 (Accenture 2015 ). The 'last mile' delivery vehicles that are needed for this market are undergoing changes and present good opportunities for electrification. Amazon, for example, has announced plans to purchase 100 000 custom-designed Rivian electric delivery vans by 2030, with 10 000 of the vehicles delivered by late 2022 (Davies 2019 ).
A significant challenge with electrifying these heavy- and medium-duty vehicles revolves around the installation of the required charging infrastructure (either at depots or along highways). While LDVs typically charge at power levels of 3 kW–10 kW, and potentially 50 kW–250 kW with DC fast chargers (DCFCs), a heavy-duty vehicle may require higher-power charging, depending on its duty cycle. Fleets of these vehicles charging in one location, such as a truck depot or travel center, may require several megawatts of power. This requires expensive charging infrastructure, potentially including costly and time-consuming distribution-grid upgrades, to provide the higher voltage and current levels that are needed. For example, a single 350 kW DCFC that may be suitable for heavy-duty applications costs almost $150 000 today (Nelder and Rogers 2019 , Nicholas 2019 ). These costs would, in turn, impact the business case for vehicle electrification. Potential costs of grid upgrades to support these new electrical loads would be additional expenses that may or may not be supported by the local utility, depending on the circumstances. To enable reliable, low-cost charging, which is crucial when considering the TCO for a fleet owner, the installation and operational costs of the charging infrastructure must be optimized, requiring engagement with power-supply stakeholders.
4. Batteries, power electronics, and electric machines
Electrification is a key aspect of modern life, and electric motors and machines are prevalent in manufacturing, consumer electronics, robotics, and EVs (Zhu and Howe 2007 ). One reason for the recent success and rise in adoption of EVs is the use of advanced lithium-ion batteries with improved performance, life, and lower cost. Improved energy and power performance, increased cycle and calendar life, and lower costs are leading to EVs with longer electric range and better acceleration at lower cost premia that are attracting consumers. This section summarizes the state-of-the-art for batteries and for power electronics, electric machines, and electric traction drives in terms of cost, performance, power and energy density, and reliability, and highlights some research challenges, pathways, and targets for the future.
4.1. Batteries
Over the last 10 years, the price of a lithium-ion battery pack has dropped by almost 90% from over $1000 kWh −1 in 2010 to $156 kWh −1 at the end of 2019 (BloombergNEF 2020 ). Meanwhile, the specific energy of a lithium-ion battery cell has almost doubled from 140 Wh kg −1 to 240 Wh kg −1 during that same window of time (BloombergNEF 2020 ). The improvement in performance and cost comes mainly from engineering improvements, use of materials with higher capacities and voltages, and development of methods to increase stability for longer life and improved safety. Improvements in cell, module, and pack design also help to improve performance and lower costs. Increases in manufacturing volume due to EV sales contribute significantly to cost reductions (Nykvist and Nilsson 2015 , Nykvist et al 2019 ). However, further reductions in battery costs, along with a reduction in the cost of electric machines and power electronics, are needed for EVs to achieve purchase-price parity with ICEVs. This parity is estimated by U.S. Department of Energy (DOE) to be achieved at battery costs of ∼$100 kWh −1 (preferably less than $80 kWh −1 ) (VTO, 2020 ). At that point, EVs should have both a purchase- and a lifetime-operating-cost benefit over ICEVs. Such cost benefits are likely to trigger drastic increases in EV sales. Figure 3 shows the observed price of lithium-ion battery packs from 2010 to 2018, as well as estimated prices through 2030. BloombergNEF projects that by 2024 the price for original equipment manufacturers (OEMs) to acquire battery packs will go below $100 kWh −1 and reach ∼$60 kWh −1 by 2030 if high levels of investments continue in the future (BloombergNEF 2020 ).
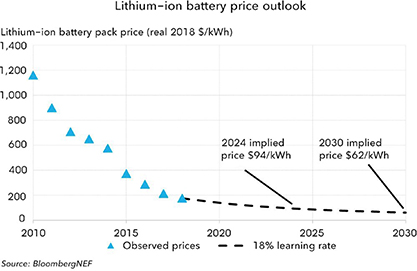
Figure 3. Evolution of battery prices over the last 10 years and future projections (Goldie-Scot 2019 ). BloombergNEF 2019.
The typical anode material that is used in most lithium-ion EV batteries is graphite (Ahmed et al 2017 ). Research is underway to utilize silicon, in addition to graphite, due to its higher specific-energy capacity. For cathodes, there is more variety (Lee et al 2019 , Manthiram 2020 ). Consumer electronics such as mobile phones and computers almost exclusively have used lithium cobalt oxide, LiCoO 2 , due to its high specific-energy density (Keyser et al 2017 ). Most EV manufacturers (except Tesla) have avoided using LiCoO 2 in EVs due to its high cost and safety concerns. Lithium iron phosphate also has been used for electric cars and buses because of its long life and better safety and power capabilities. However, due to its low specific-energy density (110 Wh kg −1 ) when paired with a graphite anode, lithium iron phosphate is not used commonly for light-duty EVs in U.S. In recent years, battery makers and vehicle OEMs have moved to lithium nickel manganese cobalt oxides (NMC) with varying ratios of the three transition metals. Initially, OEMs used NMC111 (the numbers represent the molar fractions of nickel, manganese, and cobalt, which are equal in this case), but they have transitioned to NMC532 and utilize NMC622 now while working to stabilize the NMC811 cathode structure. The goal is eventually to reduce the amount of cobalt in the cathode to less than 5% and perhaps even eliminate the use of cobalt. The use of these cathodes with higher specific-energy density and less cobalt leads to lower battery cost per unit energy ($ kWh −1 ). Table 1 shows the specific energy and estimated (bottom-up) cost from Argonne National Laboratory's BatPaC Battery Performance and Cost model (Ahmed et al 2016 ) based on large-volume material processing, cell manufacturing, and pack manufacturing.
Table 1. Calculated specific energy and cost of advanced lithium-ion batteries with different cathode/anode chemistries. Numbers are from BatPaC (Ahmed et al 2016 ) and are intended for relative comparison only. Final values can change depending on the components used and production volume, and costs reported do not reflect what a negotiated price could be between a battery and EV maker.
The cost of batteries is expected to decline in the future due to improved capacity of materials (such as Si anodes), increased percentage of active material components, use of lower-cost elements (no cobalt), improved packaging, and continued automation to increase yield while leading to a longer electric range. However, price increases for certain metals such as Ni and Li could prevent achieving those lower-battery-cost projections. Moreover, different battery chemistries can lead to very different costs and specific energies. For example, table 1 shows results obtained from bottom-up calculations with Argonne National Laboratory's BatPaC Battery Performance and Cost Model (Ahmed et al 2016 ), for a 100 kWh battery pack showing great variability in battery cost and performance for different chemistries.
Opportunities to improve performance and reduce costs further are being pursued in a number of major research areas. The battery community is investigating a number of materials, with the aim of reducing the cost and increasing the energy density of battery systems (Deign and Pyper 2018 ). Future work will involve utilizing silicon (Salah et al 2019 ) or lithium metal (Zhang et al 2020 ) as the anode while utilizing high-energy cathodes, such as NMC811 or lithium sulfur (Zhu et al 2019 ). Reducing the amount of critical material in lithium-ion batteries, especially cobalt, is an opportunity to lower the cost of batteries and improve supply-chain resilience. The private and public sectors are working toward developing new cathode materials along these lines (Li et al 2009 , 2017b ). Research and development (R&D) projects are underway to develop infrastructure and recycling technologies to collect batteries and recover the key battery materials economically and environmentally (Harper et al 2019 ). Reuse of end-of-life batteries from EVs would delay the need for additional battery materials, which should have positive environmental benefits (Neubauer et al 2012 ). Different battery technologies also are being explored. To increase energy density, reduce cost, and improve safety, the battery community is pursuing development of solid-state batteries with solid-state electrolytes (Randau et al 2020 ) that have ionic conductivities approaching those of today's liquid electrolyte systems. Solid-state lithium batteries enable the use of metallic lithium anodes, together with solid electrolytes and high-energy cathodes (such as high-nickel NMC or sulfur). Lithium-metal batteries based on solid electrolytes can, in principle, alleviate the safety concerns with current lithium-ion batteries with a flammable organic electrolyte. The main challenges facing lithium-metal anodes are dendritic growth, especially at low temperatures and higher current rates. Dendritic growth could lead to short circuit and thermal runaway and low Coulombic efficiency leading to poor cycle life (Xia et al 2019 ). Slow ion transport through the solid-state electrolyte leading to low power densities and manufacturing challenges, including poor mechanical integrity, pose additional challenges. Significant R&D activities are focused on developing solid-state electrolytes that prevent dendrite growth, have high ionic conductivity, good voltage-stability windows, and low impedance at the electrode–electrolyte interface. Recent cathode formulations in Li-S cells overcome the polysulfide problem, which could lead to lower efficiency and cycle life. Nevertheless, the deployment of cells with lower electrolyte-to-sulfur ratios for scale-up to large sizes is a remaining challenge. It may take another 5 to 10 years to mass-produce solid-state lithium batteries for EV applications.
As is discussed in section 5 , a network of fast chargers and batteries that can handle high charging-power rates is needed to address any potential barriers to widespread EV adoption. Research is focusing on developing batteries that can be charged very quickly (e.g. 80% of capacity in less than 15 min). A number of challenges to high-power charging, such as lithium plating, thermal management, and poor cycle life, need to be addressed (Ahmed et al 2017 ; DOE 2017 , Michelbacher et al 2017 ). Significant efforts also have focused on developing electrochemical and thermal modeling of batteries for EV applications (Kim et al 2011 , Chen et al 2016 , Keyser et al 2017 , Zhang et al 2017 ) to improve battery lifetime and efficiency in real-world applications. These efforts include lifetime-estimation and degradation modeling under different real-world climate and driving conditions (Hoke et al 2014 , Neubauer and Wood 2014 , Liu et al 2017b , Harlow et al 2019 , Li et al 2019 ); simplified models for control and diagnostics (e.g. state-of-charge estimation) (Muratori et al 2010 , Fan et al 2013 , Cordoba-Arenas et al 2015 , Bartlett et al 2016 ); and developing effective thermal management and control strategies (Pesaran 2001 , Serrao et al 2011 ).
Besides EV applications, batteries can offer energy-storage solutions for hybrid- or distributed-energy systems. These solutions include the use of batteries in integrated configurations with wind or solar photovoltaic (PV) systems or with EV fast-charging stations (Bernal-Agustín and Dufo-Lopez 2009 , Badwawi et al 2015 , Muratori et al 2019a ). Batteries also can provide stabilization and flexibility and can improve resilience and efficiency for power systems in general, especially for critical services or when a high share of variable power generation (e.g. from solar or wind) is expected (Divya and Østergaard 2009 , Denholm et al 2013 ; De Sisternes et al 2016 ). Lithium-ion batteries that have been developed for EV applications have found their way into stationary applications (Pellow et al 2020 ) because of their lower cost and modularity compared to other energy-storage technologies (Chen et al 2020 ). Moreover, EV batteries can be reused or repurposed at the end of their 'vehicle life' (usually considered when energy storage capacity drops below 70%–80% of the original nominal value, (Podias et al 2018 )) for stationary applications, improving their economic and environmental performance (Assuncao et al 2016 , Ahmadi et al 2017 , Martinez-Laserna et al 2018 , Olsson et al 2018 , Kamath et al 2020 ).
4.2. Power electronics, electric machines, and electric-traction-drive systems
While batteries are playing a key role in the rise of EVs, power electronics and electric motors and machines are also key components of an EV powertrain. Traditionally, the motor and power electronics drive were separate components in an EV. However, recent trends toward integration promise to deliver benefits in terms of increased power density, lower losses, and lower costs compared with separate motor and motor-drive solutions (Reimers et al 2019 ). Figure 4 shows the 2020 power density for power electronics, electric machines, and electric-traction-drive system from some example commercial vehicles as well as the 2025 DOE and U.S. DRIVE Partnership targets for near-term improvements (U.S. DRIVE 2017 , Chowdhury et al 2019 ). Commercially available vehicles exceed the 2020 power-density target. However, the 2025 target is at least a factor of six to eight higher than current commercial baselines. U.S. DRIVE Partnership also proposes electric-traction-drive-cost targets for 2020 and 2025: $8 kW −1 and $6 kW −1 , respectively, both of which are challenging targets (U.S. DRIVE 2017 , Chowdhury et al 2019 ). The authors are not aware of commercial systems meeting the 2020 target, and the 2025 target represents a further 33% reduction.
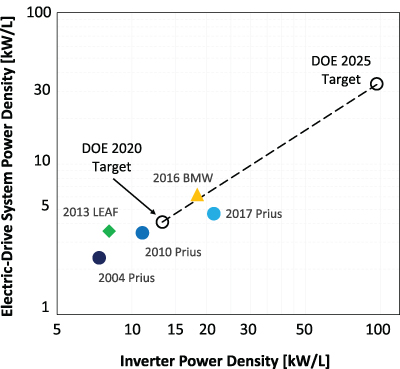
Figure 4. Integrated electric-drive system and inverter power density for several commercial light-duty vehicles and DOE targets (data from U.S. DRIVE 2017 , Chowdhury et al 2019 ).
Improvements in compact power electronics and electric machines are applicable to novel emerging wheel-integrated solutions as well (Iizuka and Akatsu 2017 , Fukuda and Akatsu 2019 ). The development of advanced electric traction drive with improved efficiency is a strategy for increasing the range of electric-drive vehicles. In addition to this, chassis light-weighting is another strategy that is being pursued by the industry and the research community for increasing EV driving ranges. There are several technical challenges in meeting the DOE power-density targets (shown in figure 4 ). Challenges in meeting related DOE cost targets remain as well. A range of integration approaches are proposed in the literature, including surface mounting the power electronics on the motor housing (Nakada, Ishikawa, and Oki 2014 ), mounting on the motor stator iron (Wheeler et al 2005 ), and piecewise integration. Piecewise integration involves modularizing both power modules and machine stators into smaller units (Brown et al 2007 ). In all cases, the close physical positioning of the power electronics relative to the machine and the associated harsh thermal environment necessitate new concepts related to the active cooling of both components. A first strategy may be to isolate the power electronics from the machine thermally using parallel cooling mechanisms (Wheeler et al 2005 ). Another approach may be to use a fully integrated, series-connected, active-cooling loop (Tenconi et al 2008 , Gurpinar et al 2018 ). In either case, cost benefits may be realized through the possible elimination or combination of cooling loops. Significant research also has been focused on reducing rare-earth and heavy-rare-earth materials within the electric machines because that is an additional important pathway to reduce costs (U.S. DRIVE 2017 ).
Higher levels of integration go hand-in-hand with the utilization of wide-bandgap (WBG) semiconductor devices, which may be used at higher operational temperatures (e.g. >200 °C versus 150 °C for silicon) with reduced switching loss (Millán et al 2014 ). However, the adoption of WBG devices requires new packaging technologies to support the end goals of high temperature, high frequency, higher voltages, and more compact footprints. High-performance electrical interconnects (Cheng et al 2013 ), die-attach (Liu et al 2020 ), encapsulation (Cao et al 2010 ), and power-module-substrate technologies (Stockmeier et al 2011 ), along with thermal management and reliability of these technologies (Moreno et al 2014 , Paret et al 2016 , 2019 ), are critical aspects to consider. The new materials, devices, and components must be cost-effective and high-temperature-capable to be compatible with WBG devices. The downsizing of passive electrical components is another added benefit of adopting WBG devices and a further necessity for integrated machine-drive packaging solutions. Fortunately, the higher switching frequencies that are supported by WBG devices enable the downsizing of both the inductors and capacitors found in a traditional power-control unit (Hamada et al 2015 ). The development of economically viable and high-temperature-capable passives, capacitors in particular (Caliari et al 2013 ), is an area of great interest.
Besides EV applications, power electronics and electric machines with low cost, high performance, and high reliability are important for numerous energy-efficiency and renewable-energy applications, such as solar inverters, generators and electric drives for wind, grid-tied medium-voltage power electronics, and sensors and electronics for high-temperature geothermal applications (PowerAmerica 2020 ).
5. Charging infrastructure
Infrastructure planning and deploying an ecosystem of cost-effective and convenient public and private chargers is central to supporting EV adoption (CEM 2020 ). The lack of a sufficient refueling infrastructure has hampered many past efforts to promote alternatives to petroleum fuels (McNutt and Rodgers 2004 ). Extensive research is being done to address the diverse challenges that are posed by a transition from fossil-fuelled ICEVs to EVs and the special role of charging infrastructure in this transition (Muratori et al 2020b ).
At the end of 2019, there were an estimated 7.3 million EV chargers (or plugs) worldwide, of which almost 0.9 million were public, including approximately 264 000 public DCFCs (81% in China) (IEA 2020 ). Significant government support and private investments are helping to expand the network of public charging stations worldwide. With about 7.2 million light-duty BEVs on the road, there is about one public charger per 10 light-duty BEVs, and most vehicles have access to a residential charger. However, the number of public chargers per BEV varies widely among the 10 countries with the most BEVs (figure 5 ) because of different strategies for deploying fast versus slow public chargers. In addition to these LDV chargers, IEA estimated there are 184 000 fast chargers dedicated to electric buses (95% in China).
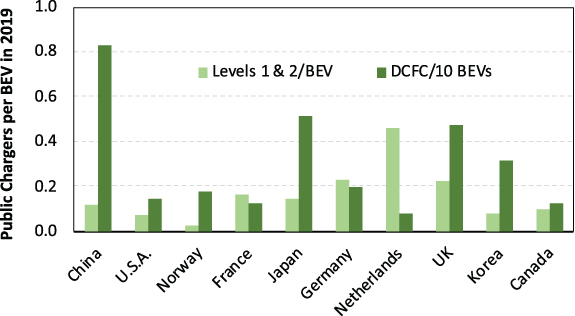
Figure 5. Public charging availability by country in 2019, measured as Level-1 and Level-2 chargers per BEV and DCFC per 10 BEVs (Data from IEA 2020 ).
Studies show consistently that today's EVs do the majority (50%–80%) of their charging at home, followed by at work (15%–25% when workers use their vehicles to commute), and using public chargers (only about 5% of charging) (Hardman et al 2018 ). PHEVs conduct more charging at home than BEVs do, and they rely more on level-1 charging (Tal et al 2019 ). While single-household detached residences readily can accommodate level-1 or -2 charging, multi-unit dwellings require curbside public charging or installations in shared parking facilities (Hall and Lutsey 2017 ). Historical data on the charging behavior of California BEV owners reveals that 11% of their charging sessions were at level 1, 72% were at level 2, and 17% used DCFCs (Tal et al 2019 ). Use of DCFCs is lowest for BEVs with less than 100 miles (161 km) of range, highest for medium-range BEVs, and lower again for BEVs with ranges of 300 miles (483 km) or more.
5.1. Charging-siting modeling
Public charging infrastructure is clearly important to EV purchasers and supports EV sales by adding value (Narassimhan and Johnson 2018 , Greene et al 2020 ). However, how best to deploy charging infrastructure, in terms of numbers, types, locations, and timing remains an active area for research (Ko et al 2017 , Funke et al 2019 provide reviews). The literature includes many examples of geographically and temporally detailed models to optimize the location, number, and types of charging stations (e.g. Wood et al 2017 , Wu and Sioshansi 2017 , Zhao et al 2019 ). Geographically and temporally detailed data recording the movements of PEVs and their charging behavior are scarce. With few exceptions (e.g. Gnann et al 2018 ), simulation analyses rely on conventional ICEV databases (e.g. Dong et al 2014 , Wood et al 2015 , 2018 ), which do not reflect the changes PEV owners will make to maximize the utility of PEVs.
Given the importance of home charging, access to chargers for on-street parking in residential areas comprising attached or multi-unit dwellings is likely to be essential for PEVs to be adopted at large scale. Grote et al ( 2019 ) employ heuristic methods with geographical-information systems to locate curbside chargers in urban areas using a combination of census and parking data. The works of Nie and Ghamami ( 2013 ), Ghamami et al ( 2016 ), and Wang et al ( 2019 ) are examples of the variety of optimization methods that are applied to design DCFC networks to support intercity travel. Despite these examples, applied research is hindered by the scarcity of data on long-distance vehicle travel by PEVs (Eisenmann and Plötz 2019 ). Jochem et al ( 2019 ) estimate that 314 DCFC stations could provide minimum coverage of EU intercity routes with approximately 0.7 charging points per 1000 BEVs. Using a database of simulated U.S. intercity travel, He et al ( 2019 ) employ a mixed-integer model to optimize the location and number of DCFCs. They conclude that 250 stations could serve 98% of the long-distance miles of BEVs with ranges of 150 miles (241 km) or greater but only 73% of the long-distance miles of 100 mile range (161 km range) BEVs. Similarly, Wood et al ( 2017 ) estimate that 400 DCFC stations are required to cover the U.S. interstate-highway network with a 40 mile (64 km) spacing between stations. Others consider the optimal location of dynamic, wireless charging in combination with stationary charging (Liu and Wang 2017 ).
Optimization models for locating chargers to support commercial PEV fleets also appear in the literature (Jung et al 2014 , Shahraki et al 2015 ). In the future, if vehicle sharing becomes much more common, the downtime for charging could be an important disadvantage for PEVs. Using an integer model to optimize station allocation and PEV assignment, Roni et al ( 2019 ) find that charging time represents 72%–75% of vehicle downtime but that charging time could be reduced by almost 50% by optimal deployment of charging stations.
5.2. Beyond LDV charging
The electrification of medium- and heavy-duty commercial trucks and buses introduces unique charging and infrastructure requirements compared to those of LDVs. These requirements stem from the significantly higher battery capacities required on-board the vehicles, potentially shorter charging-dwell times (due to the in-service time requirements of the vehicles), and the potential of large facility charging loads (due to multiple trucks or buses charging in one location). One challenge is to understand the costs associated with the multitude of charging scenarios for commercial vehicles for current operations as well as future operations. It is expected that on-road freight vehicle miles (or km) traveled will increase by 75% from 2012 to 2045 (McCall and Phadke 2019 ). This increase may bring about new business models and potentially new charging-infrastructure approaches to meet this demand with electrified trucks. California's Innovative Clean Transit regulation, which will require California transit agencies to adopt zero-emission buses by 2040, is likely to drive large charging-infrastructure investments for buses (CARB 2018 ).
Today's commercial diesel-powered trucks in small fleets typically are fueled at publicly available on-road fueling stations, while nearly half of trucks in fleets of 10+ vehicles use company-owned facilities (Davis and Boundy 2020 ). Likewise, commercial EVs are charged primarily in fleet-owned facilities as their daily schedule allows (most often overnight). This depot-charging approach, which enables seamless integration of EVs into fleet logistics, might limit the electrification of some vehicle segments in the long term due to the battery capacity that is needed to satisfy their daily-range requirements (the need to complete their full-day function) and return to the facility to recharge fully 14 . Some studies suggest that long-haul battery-electric trucks are technically feasible and economically compelling (Phadke et al 2019 ) while others are more skeptical (Held et al 2018 ). Publicly available, high-power charging or en-route charging infrastructure for commercial vehicles could enable electrification for longer-distance vehicles (by enabling smaller on-board battery-capacity needs), but this scenario has cost challenges. En-route, high-power charging of over 1 MW might be needed to enable 500 miles (805 km) or more of daily driving. Installation of a 20 MW truck-charging station in California (capable of multiple 1.5 MW charge events for heavy-duty freight vehicles) is estimated to cost as much as 15 million USD. McCall and Phadke ( 2019 ) estimate that as many as 750 of these stations are needed to electrify the fleet of California Class-8 combination trucks. Charging commercial vehicles at depots requires additional infrastructure costs to install lower-power EV-supply equipment networks (e.g. 50 kW–100 kW) capable of charging multiple vehicles at these lower rates. These depot charging systems also will challenge existing facility electrical systems by adding a significant load that was not planned previously at the facility (Borlaug et al Forthcoming ).
5.3. Economics of public charging
PEV-charging economics vary with location and station configuration and depend critically on equipment and installation costs and retail electricity prices, which are dependent on utilization (Muratori et al 2019b , Borlaug et al 2020 ). In the early stages of market development, when there are relatively few vehicles, future demand is uncertain, and most charging is done at an EV's home base (Nigro and Frades 2015 , Madina et al 2016 ). Public charging stations tend to be lightly used during these initial stages (e.g. INL 2015 ), which poses a difficult challenge for private investment. Understanding and quantifying the value of public charging is hindered by lack of experience with PEVs on the part of consumers (Ito et al 2013 , Greene et al 2020 , Miele et al 2020 ) and the complexity of network effects in the evolution of alternative-fuel-vehicle markets (Li et al 2017a ). Nevertheless, it is likely that DCFCs will be profitable with sufficient demand. Considering vehicle ranges of between 100 km and 300 km and charging-power levels of between 50 kW and 150 kW, Gnann et al ( 2018 ) conclude that charger-usage fees could be between 0.05 € kWh −1 and 0.15 € kWh −1 in addition to the cost of electricity. The estimates were based on simulations with average daily occupancy of charging points of 10%–25% and peak-hour utilization of 20%–70%. In their simulations, utilization rates increase with increasing charger power and decrease with increased EV range. For intercity travel along European Union highways, Jochem et al ( 2019 ) estimate that a surcharge of 0.05 € kWh −1 of DCFC would make a minimal coverage of 314 stations (with 20 charge plugs each) profitable, even for station capital costs of one million EUR. He et al ( 2019 ) optimize DCFC locations along U.S. intercity routes and conclude that providing an adequate nationwide charging network for long-distance travel by 100 mile (161 km) range BEVs is more economical than increasing vehicle range and reducing the number of charging stations. Muratori et al ( 2019a ) consider a set of charging scenarios from real-world data and thousands of U.S. electricity retail rates. They conclude that batteries can be highly effective at mitigating electricity costs associated with demand charges and low station utilization, thereby reducing overall DCFC costs.
Early estimates show that the cost of public DCFC in U.S. can vary widely based on the station characteristics and level of use (Muratori et al 2019a ). Numerous new technology options are being explored to provide lower-cost electricity for light-duty passenger and medium- and heavy-duty commercial BEVs. Increasing the range of EVs through higher-power public charging stations as well as accommodating new potential BEV business models, such as transportation-network companies or automated vehicles, are driving new charging-technology solutions. Managed charging solutions that are available today can provide increased value to the BEV owner (lower electricity costs), charging station owner (lower operating costs), or grid operator (lower infrastructure-investment costs). For example, a managed-charging solution has been adopted and is currently in operation at a Santa Clara Valley Transportation Authority depot to charge a fleet of Proterra electric buses optimally to ensure minimal stress on the grid (Ross 2018 ).
5.4. Emerging charging technologies
Wireless charging, specifically high-power wireless charging (beyond level-2 power levels), could play a key role in providing an automated charging solution for tomorrow's automated vehicles (Lukic and Pantic 2013 , Qiu et al 2013 , Miller et al 2015 , Feng et al 2020 ). Wireless charging also can enable significant electric range for BEVs by providing en-route opportunity charging (static or dynamic charging opportunities). If a network of wireless charging options is available to provide convenient and fast en-route charging, it could help reduce the amount of battery that is needed on-board a vehicle and reduce the cost of ownership for a BEV owner. Wireless charging is being developed for power levels of up to 300 kW for LDVs, 500 kW for medium-duty vehicles, and 1000 kW for heavy-duty vehicles. Bidirectional functionality, improved efficiency, interoperability of different systems, improved cybersecurity, and increased human-safety factors continue to be developed (Ozpineci et al 2019 ).
Connectivity and communication advances will enable new BEV-charging infrastructure and managed charging solutions. However, emerging cybersecurity threats also are being identified and should be addressed. There are concerns associated with data exchange, communications network, infrastructure, and firmware/software elements of the EV infrastructure (Chaudhry and Bohn 2012 ), and new charging-system security requirements and protocols are being developed to address these concerns (ElaadNL 2017 ). New emulation and simulation platforms also are being developed to address these threats and help understand the consequences and value of mitigating cyberattacks that could affect BEVs, electric-vehicle-supply equipment, or the electric grid (Sanghvi et al 2020 ).
6. Vehicle-grid integration (VGI)
Connecting millions of EVs to the power system, as may occur in the coming decades in major cities, regions, and countries around the world, introduces two fundamental themes: (a) challenges to meet reliably overall energy and power requirements, considering temporal load variations, and (b) VGI opportunities that leverage flexible vehicle charging ('smart charging') or V2G services to provide power-system services from connected vehicles. Multiple studies, which are reviewed in detail below, investigate the potential load growth, impact on load shapes, and infrastructure implications of increased EV adoption. These works focus especially on impact on distribution systems and opportunities for flexible charging to reshape aggregate power loads. Mai et al ( 2018 ), for example, shows that in a high-electrification scenario, transportation might grow from the current 0.2% to 23% of total U.S. electricity demand by 2050. This growth would impact system peak load and related capacity costs significantly if not controlled properly. In-depth analytics indicate a complex decision framework that requires critical understanding of potential future mobility demands and business models (e.g. ride-hailing, vehicle sharing, and mobility as a service), technology evolution, electricity-market and retail-tariff design, infrastructure planning (including charging), and policy and regulatory design (Codani et al 2016 , Eid et al 2016 , Knezovic et al 2017 , Borne et al 2018 , Hoarau and Perez 2019 , Gomes et al 2020 , Muratori and Mai 2020 , Thompson and Perez 2020 ).
While accommodating EV charging at the bulk-power (generation and transmission) level will be different in each region, no major technical challenges or risks have been identified to support a growing EV fleet, especially in the near term (FleetCarma 2019 , U.S. DRIVE 2019 , Doluweera et al 2020 ). At the same time, many studies show that smart charging and V2G create opportunities to reduce system costs and facilitate VRE integration (Sioshansi and Denholm 2010 , Weiller and Sioshansi 2014 , IRENA 2019 , Zhang et al 2019 ). Therefore, charging infrastructure that enables smart charging (e.g. widespread residential and workplace charging) and alignment with VRE generation and business models and programs to compensate EV owners for providing charging flexibility are critical elements for successful integration of EVs with bulk power systems.
6.1. Impact of EV loads on distribution systems
At the local level, EV charging can increase and change electricity loads significantly, having possible negative impacts on distribution networks (e.g. cables and distribution transformers) and power quality or reliability (Khalid et al 2019 ). Residential EV charging represents a significant increase in household electricity consumption that can require upgrades of the household electrical system which, unless managed properly, may exceed the maximum power that can be supported by distribution systems, especially for legacy infrastructure and during times of high electricity utilization (e.g. peak hours and extreme days) (IEA 2018b ). The impact of EVs on distribution systems also is influenced by the simultaneous adoption of other distributed energy resources, e.g. rooftop PV panels. While this interdependency complicates assessing the impact of EV charging, Fachrizal et al ( 2020 ) show that the two technologies support one other. Similarly, Vopava et al ( 2020 ) show that line overloads caused by rooftop PV panels can be reduced (but not avoided) by increasing EV adoption and vice versa.
The impact of EV charging on distribution systems is particularly critical for high-power charging and in cases in which many EVs are concentrated in specific locations, such as clusters of residential LDV charging and possibly fleet depots for commercial vehicles (Saarenpää et al 2013 , Liu et al 2017a , Muratori 2018 ). Smart charging, by which EV charging is timed based on signals from the grid and electricity prices that vary over time, or other forms of control, can help to minimize the impact of EV charging on distribution networks. However, smart charging requires both appropriate business models and signals (with related communication and distributed-control challenges). The market for distribution-system operators to provide such services is not mature yet (Everoze 2018 , Crozier, Morstyn, and McCulloch 2020 ). Time-varying pricing schemes, which are effective at influencing the timing of EV charging (PG&E 2017 ), typically do not include any distribution-level considerations. Thus, while consumers are responsive to such signals, the business models to include distribution-level metrics still are lacking. Moreover, price signals are offered usually to a large consumer base with the intent of reshaping the overall system load. At the local level, however, multiple consumers responding to the same signal might cause 'rebound peaks' (Li et al 2012 , Muratori and Rizzoni 2016 ) that can overstress distribution systems, calling for coordination among consumers connected to the same distribution network (e.g. direct EV-charging control from an intermediate aggregator).
Charging of larger commercial vehicles and highway fast-charging stations typically involves higher power levels: DCFC is typically at 50 kW/plug today, but power levels are increasing rapidly. Commercial charging locations with multiple plugs co-located at a specific location may lead to possible MW-level loads, which is roughly equivalent to the peak load of a large hotel. Commercial DCFC may require costly upgrades to distribution systems that can impact the cost-effectiveness of public fast charging heavily, especially if stations experience low utilization (Garrett and Nelder 2016 , Muratori et al 2019b ). While charging timing and speed at commercial stations is less flexible (consumers want to charge and leave or commercial fleets must meet business requirements), business models are often already in place to incentivize curbing maximum peak power from commercial installations. For example, demand charges (a fixed monthly payment that is proportional to the peak power that is drawn during a given month) are fairly common in U.S. retail tariffs and provide a reason to limit peak power. Furthermore, Muratori et al ( 2019a ) show that distributed batteries can be effective at mitigating the cost associated with demand charges by up to 50%, especially for 'peaky' or low-utilization EV-charging loads. Batteries also can facilitate coupling EV-charging stations with local solar electricity production or can provide grid services (Megel, Mathieu, and Andersson 2015 ), generating additional revenue.
6.2. Value of managed ('smart') EV charging for power systems
The integration of EVs into power systems presents several opportunities for synergistic improvement of the efficiency and economics of electromobility and electric power systems. These synergies stem from two inherent characteristics of EVs and power systems. Demand response and other forms of demand-side flexibility can be of value for power-system planning and operations (Albadi and El-Saadany 2007 , 2008 , Su and Kirschen 2009 , Muratori et al 2014 ). Contemporaneously, most personal-vehicle driving patterns entail vehicle-use for mobility purposes a relatively small proportion of the time (Kempton and Letendre 1997 ). If EVs are grid-connected for extended periods of time, they can provide demand-side flexibility in the form of smart charging or V2G services. Such use of an EV can improve its economics by leveraging cheaper electricity at little incremental cost (e.g. the costs of monitoring, communication, and control equipment that are needed to manage smart charging). EVs can support grid planning and operations in a number of ways. Figure 6 summarizes the key support services that EVs can provide. These services include reducing peak load and generation-, transmission-, and distribution-capacity requirements, deferring system upgrades, providing load response, supporting power-system dispatch (including VRE integration and real-time energy and operating reserves), providing energy arbitrage, and supporting power quality and end retail consumers.
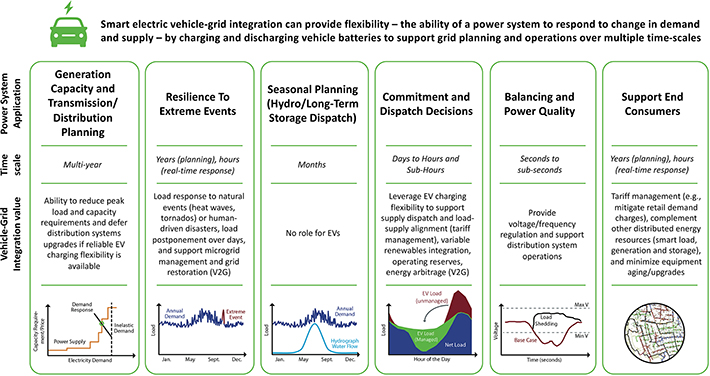
Figure 6. Summary of opportunities for EVs to provide demand-side flexibility to support power system planning and operations across multiple timescales.
Habib et al ( 2015 ) and Thompson and Perez ( 2020 ) provide detailed surveys of different potential uses of EVs for smart charging and V2G services. This includes active- and reactive-power services, load balancing, power-quality-related services (e.g. managing flicker and harmonics), retail-bill management, resource adequacy, and network deferral. In addition, Habib et al ( 2015 ) discuss different standards and technology needs relating to V2G services.
Kempton and Letendre ( 1997 ) provide the first description of the concept of EVs providing grid services, either in the form of smart charging or bidirectional V2G services (which can involve discharging EV batteries). Denholm and Short ( 2006 ) study the benefits of controlled overnight charging of PHEVs for valley-filling purposes. They demonstrate that with proper control of vehicle charging, up to 50% of the vehicle fleet could be electrified without needing new generation capacity to be built and at substantial savings compared to using liquid fuels for transportation. They show also that under conservative utility-planning practices, PHEVs could replace a significant portion of low-capacity-factor generating capacity by providing peaking V2G services. Tomić and Kempton ( 2007 ) examine the economics of using EVs for the provision of frequency reserves and demonstrate that such services can yield substantive revenues to vehicle owners in a variety of wholesale markets. Thompson and Perez ( 2020 ) conduct a meta-analysis of V2G services and value streams and find that power-focused services are of greater value than energy-focused services. They distinguish the two types of services based on the extent to which EV batteries must be discharged and degraded. Sioshansi and Denholm ( 2010 ) come to a similar conclusion in comparing the value of using PHEV batteries for energy arbitrage and operating reserves.
Another important synergy between EVs and power systems is using the flexibility of EV charging to manage the integration of VRE into power systems (Mwasilu et al 2014 , Weiller and Sioshansi 2014 ). Hoarau and Perez ( 2018 ) develop a framework for examining the synergies between EV charging and the integration of photovoltaic-solar resources into power systems. They find that the spatial footprint across which solar resources and EVs are deployed and the regulatory, policy, and market barriers to cooperation between solar resources and EVs are critical to realizing these synergies. Szinai et al ( 2020 ) find that controlled EV charging in California under its 2025 renewable-portfolio standards can reduce operational costs and renewable curtailment compared to unmanaged charging. They find that properly designed time-of-use retail tariffs can achieve some, but not all, of the benefits of controlled EV charging. They show also that these two approaches to managing EV charging (controlling EV charging directly and time-of-use tariffs) reduce the cost of infrastructure that is necessary to accommodate EV charging relative to a case of uncontrolled EV charging. Chandrashekar et al ( 2017 ) conduct an analysis of the Texas power system and find similar benefits of controlled EV charging in reducing wind-integration costs. Coignard et al ( 2018 ) show that under California's 2020 renewable-portfolio standards, controlled EV charging can deliver the same renewable-integration benefits that California's energy-storage mandate does but at substantially lower costs. They show that bidirectional V2G services deliver up to triple the value of controlled EV charging. Kempton and Tomić ( 2005 ) show that high penetrations of wind energy in U.S. could be accommodated at relatively low costs if 3% of the vehicle fleet provides frequency reserves and 8%–38% of the fleet provides operating reserves and energy-storage services to avoid wind curtailment. Loisel et al ( 2014 ) and Zhang et al ( 2019 ) conduct more forward-looking analyses of the synergies between EVs and renewables. The former examines German systems, and the latter examines California systems under potential renewable-deployment scenarios in the year 2030.
An important assumption underlying these works is that EV owners (or aggregations of EVs) are exposed to prices that signal the value of these services and that there are regulatory and business models that allow such services to be exploited (i.e. consumer are willing to engage in these programs and are compensated properly for providing flexible charging). Several pilot studies suggest that EV owners have interest in participating in utility-run controlled-charging programs and that a set of different compensation strategies beyond time-varying electricity pricing might maximize engagement (Geske and Schumann 2018 , Hanvey 2019 , Küfeoğlu et al 2019 , Delmonte et al 2020 ).
Niesten and Alkemade ( 2016 ) survey the literature on these topics and numerous European and U.S. pilot programs in terms of value generation for V2G services. They find that the ability of an aggregator to scale is related to its ability to develop a financially viable business model for V2G services. Another important consideration is the availability of control and communication technologies to manage EV charging based on power-system conditions. Key considerations in the design of control strategies are robustness in the face of uncertainty (e.g. renewable availability, EV-arrival times and charge levels upon arrivals, and EV-departure times), data privacy, and robustness to communication or other failures. Le Floch et al ( 2015a ), Le Floch et al ( 2015b ), ( 2016 ), develop a variety of distributed and partial-differential-equation-based algorithms for controlling EV charging. Rotering and Ilic ( 2011 ) develop a dynamic-optimization-based approach to control EV charging and bidirectional V2G services (with a focus on the provision of ancillary services). Donadee and Ilic ( 2014 ) develop a Markov decision process to optimize the offering behavior of EVs that participate in wholesale electricity markets to provide frequency reserves.
6.3. Remaining challenges for effective vehicle-grid integration at scale
There are still many challenges to tackle before smart charging and V2G can be deployed effectively at large scale. These challenges are linked to the technical aspects of VGI technology but also to societal, economic, security, resilience, and regulatory questions (Noel et al 2019a ). With regard to the technical challenges of VGI, existing barriers notably include battery degradation, charger availability and efficiency, communication standards, cybersecurity, and aggregation issues (Eiza and Ni 2017 , Sovacool et al 2017 , Noel et al 2019a ).
While the technical aspects of VGI are studied widely, this is much less the case for its key societal aspects. Societal issues include the environmental performance of VGI, its impact on natural resources, consumer acceptance and awareness, financing and business models, and social justice and equity (Sovacool et al 2018 ). There are also various regulatory and political challenges linked to clarifying the regulatory frameworks applicable to VGI as well as market-design issues, such as the proper valuation of VGI services and double taxation (Noel et al 2019a ) and the trade-offs between bulk power and distribution-system needs. Regulatory changes may be required to enable distribution-network operators and EV owners (or aggregators) to take a more active role in electricity markets. The Parker project, an experimental project on balancing services from an EV fleet, underlines some of the barriers to providing ancillary services, such as metering requirements (Andersen et al 2019 ). It is argued that insufficient regulatory action might keep us from attaining the full economic and environmental benefits of V2G (Thompson and Perez 2020 ) and that regulations are lagging behind technological developments (Freitas Gomes et al 2020 ). The lack of defined business models is seen by many experts as a key impediment (Noel et al 2019b ).
Major challenges that are linked to data-related aspects of VGI, including who has the right to access data from EVs (e.g. the state of EV batteries and charging) and how these data can be exploited, remain. Privacy concerns are one of the major obstacles to user acceptance (as is fear of loss of control over charging) (Bailey and Axsen 2015 ). In addition, there are also questions linked to cybersecurity (Noel et al 2019a ).
Nevertheless, VGI offers many opportunities that justify the efforts required to overcome these challenges. In addition to its services to the power system, VGI offers interesting perspectives for the full exploitation of synergies between EVs and renewable energy sources as both technologies promise large-scale deployment in the future (Kempton and Tomić 2005 , Lund and Kempton 2008 ). Exploiting EV batteries for VGI also is appealing from a life-cycle perspective, as the manufacturing of EV batteries has a non-trivial environmental footprint (Hall and Lutsey 2018 ). However, there are a few future developments that might compromise the potential of VGI, most notably cheaper batteries (including second-life EV batteries) that might compete with EVs for many potential services (Noel et al 2019b ). In addition, the impacts of new mobility business models, such as the rise of vehicle- and ride-sharing, on grid services remain unclear. Although smart charging will come first in the path toward grid integration, V2G services have the potential to provide additional value (Thingvad et al 2016 ).
7. Life-cycle cost and emissions
EVs differ from conventional ICEVs on an emissions basis. While the operation of gasoline- or diesel-powered ICEVs produces GHG and pollutant emissions that are discharged from the vehicle tailpipe, EVs have no tailpipe emissions. In a broader context, EVs still can be associated with so-called 'upstream' emissions from the processes that generate, transmit, and distribute the electricity that is used for their charging. Fueling an ICEV also involves upstream 'fuel-cycle' emissions from the raw-material extraction and transportation, refining, and final-product-delivery processes that make gasoline or diesel fuel available at a retail pump. These fuel-cycle emissions give rise to the colloquial jargon 'well-to-pump' emissions. Accordingly, a 'well-to-wheels' (WTW) life-cycle analysis (LCA) is an appropriate framework for comparing EV and ICEV emissions. WTW considers both upstream emissions from the fuel cycle ('well-to-pump') and direct emissions from vehicle operation ('pump-to-wheels') for a standardized functional unit and temporal period. WTW studies have a history of over three decades of use to evaluate direct and indirect emissions related to fuel production and vehicle operations (Wang 1996 ). WTW emissions are expressed typically on a per-mile or per-kilometer basis over a vehicle's assumed lifetime.
WTW analyses typically focus only on fuel production and vehicle operation. Some studies consider broader system boundaries that include vehicle production and decommissioning (i.e. recycling and scrappage) in an LCA framework. This broader system boundary considers what is commonly called the 'vehicle cycle' and provides a so-called 'cradle-to-grave' (or 'C2G') analysis. Vehicle-cycle emissions typically account for 5%–20% of today's ICEV C2G emissions and can be as low as 15% or as high as 80% of today's BEV emissions, depending on the underlying electricity-generation mix. Lower-carbon mixes result in vehicle-cycle emissions accounting for a greater portion of total emissions. As an extreme illustrative example, the case of zero-carbon electricity implies that vehicle-cycle emissions account for 100% of C2G emissions. In general, BEV vehicle-cycle emissions are 25% to 100% higher than their ICEV counterpart (Samaras and Meisterling 2008 , Ambrose and Kendall 2016 , Elgowainy et al 2016 , Hall and Lutsey 2018 , Ricardo 2020 ). As this section explores, higher initial BEV vehicle-cycle emissions almost always are counterbalanced by lower emissions during vehicle operation (with notable exceptions in cases in which BEVs are charged from especially high-emissions electricity).
Even including upstream emissions, EVs are championed as a critical technology for decarbonizing transportation (in line with anticipated widespread grid decarbonization). National Research Council ( 2013 ) identifies EVs as one of several technologies that could put U.S. on a path to reducing transportation-sector GHG emissions to 80% below 2005 levels in 2050. Furthermore, National Research Council ( 2013 ) estimates that BEVs would reduce emissions by 53%–72% compared to ICEVs in 2030. IEA ( 2019 ) contends, similarly, that EVs can reduce WTW GHG emissions by half versus equivalent ICEVs in 2030. Recently published literature also agrees, even on a C2G basis, estimating that future EV pathways offer 70%–90% lower GHG emissions compared to today's ICEVs (Elgowainy et al 2018 ). As such, the broad view across national, international, and academic-research perspectives is that EVs offer the potential to reduce transportation-related GHG emissions by 53% to 90% in the future.
Several studies find that EVs already reduce WTW GHG emissions today by as little as 10% or as much as 41% on average versus comparable ICEVs based on current electricity-production mixes. Samaras and Meisterling ( 2008 ), who are among the first to relate a range of potential electricity carbon intensities to associated EV-lifecycle emissions explicitly, estimate a 38%–41% GHG emissions benefit for EVs powered by the average 2008 U.S. grid. Hawkins et al ( 2012a ), informed by a meta-study of 51 previous LCAs, highlight great variations based on different electricity generation assumptions and vehicle lifetime. Hawkins et al ( 2012b ) estimate a decline of 10%–24% global warming potential (a measure proportional to GHG emissions) for EVs powered by the average 2012 European electricity mix. Elgowainy et al 2016 , 2018 ) estimate that EVs emit 20%–35% fewer GHG emissions when operating on the average 2014 U.S. grid mix.
Many factors contribute to variability in EV WTW emissions and estimated reduction opportunities compared to ICEVs—electricity-carbon intensity, charging patterns, vehicle characteristics, and even local climate (Noshadravan et al 2015 , Requia et al 2018 ). To illustrate these variabilities, figure 7 compares WTW GHG emissions of EVs versus comparable ICEVs. Relative emissions reductions are generally larger for larger vehicles. Woo et al ( 2017 ) find that electrifying SUVs reduces emissions more than electrifying sub-compact vehicles on a WTW basis versus comparable ICEVs (30%–45% and 10%–20%, respectively, assuming median national grid mixes). Ellingsen et al ( 2016 ) find that large EVs emit proportionally less than small EVs compared to comparable ICEVs on a C2G basis (27% and 19%, respectively).
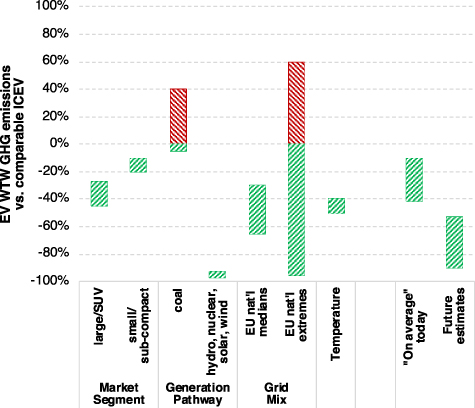
Figure 7. WTW GHG emissions for EVs versus comparable ICEVs on average and with illustrative variability by market segment, electricity generation pathway, grid mix, and ambient temperature.
Low-carbon electricity can lead to greater reductions in EV emissions. Electricity that is produced from coal, which has a high carbon intensity, can increase EV emissions by as much as 40% or decrease EV emissions by as much as 5% compared to an ICEV (depending on other assumptions). Conversely, electricity from hydropower, nuclear, solar, or wind, all of which offer near-zero carbon intensities, can decrease EV emissions by more than 95% compared to an ICEV (Woo et al 2017 ). Such variability in electricity-generation pathways affects the relative benefits of real-world grid mixes. For example, while EVs offer 30%–65% lower emissions versus comparable ICEVs on average in Europe (Woo et al 2017 , Moro and Lonza 2018 ), in individual countries relative emissions can range from as much as 95% lower to 60% higher (Orsi et al 2016 , Moro and Lonza 2018 ). Typically, U.S. EVs provide emissions reductions, but in some regions EV emissions are higher compared to an efficient ICEV (Reichmuth 2020 ). Changes in regional climate and daily weather add further variability: EV emissions can vary between 40% and 50% lower than a comparable ICEV even when charged from the same grid mix (Yuksel et al 2016 ). While outside the scope of a typical WTW comparison, the additional consideration of refueling infrastructure (i.e. gasoline stations for ICEVs and recharging equipment for EVs) is estimated to increase EV emissions by 4%–8% compared to a more modest 0.3%–0.7% increase for ICEV emissions (Lucas et al 2012 ).
When assessing EV emissions, average or marginal grid-emission factors are considered (Anair and Mahmassani 2012 , Traut et al 2013 , EPRI 2015 , Nealer and Hendrickson 2015 , Nealer et al 2015 , Elgowainy et al 2018 ), leading to significantly different results. Average emissions factors consider all electricity loads as equivalent, while marginal emission factors consider EVs as an additional load on top of existing electricity demands and estimate the associated incremental generation emissions. Marginal emissions could be higher or lower than average, depending on the relative emissions of marginal plants compared to the average in different regions. Different questions lead to using average or marginal metrics. Proper assessment of indirect EV emissions associated with electricity generation is complicated by numerous factors, including timescale (short or long term, aggregate or temporally explicit), system boundaries, impact of EV loads on power-system-expansion and -operation decisions, and non-trivial supply-demand synergies and allocation complexities. Yang ( 2013 ) reviews different grid-emissions-allocation methods concluding that there is no ideal approach to the allocation of emissions to specific end-use and stressing how different assumptions make it difficult to determine EV emissions and compare them to other alternatives and across studies. Nealer and Hendrickson ( 2015 ) discuss whether it is more appropriate to use marginal or average grid-emission factors to estimate EV emissions, concluding that 'average emissions may be the most accessible for long-term comparisons given the assumptions that must be made about the future of the electricity grid.'
Just as EVs offer typically a WTW-emissions reduction compared to ICEVs while shifting those emissions from the tailpipe to upstream, EVs shift costs as well. Operational (fuel and maintenance) costs of EVs are typically lower than those of ICEVs, largely because EVs are more efficient than ICEVs and have fewer moving parts. While data are still scarce, a recent Consumer Reports study estimates that maintenance and repair costs for EVs are about half over the life of the vehicle and that a typical EV owner who does most fueling at home can expect to save an average of $800 to $1000 a year on fuel costs over an equivalent ICEV (Harto 2020 ). Insideevs ( 2018 ) estimates a saving of 23% in servicing costs over the first 3 years and 60 000 miles (96 561 km). Borlaug et al ( 2020 ) estimate fuel savings between $3000 and $10 500 compared with gasoline vehicles (over a 15 year time horizon). However, vehicle capital costs for EVs are higher (principally due to the relatively high cost of EV batteries). In general, studies use a TCO metric to combine and compare initial capital costs with operational costs over a vehicle's lifetime. While some studies find that EVs are typically cost-competitive with ICEVs (Weldon et al 2018 ), others find that EVs are still more costly, even on a TCO basis (Breetz and Salon 2018 , Elgowainy et al 2018 ), or that the relative cost depends on other contextual factors, such as vehicle lifetime and use, economic assumptions, and projected fuel prices. Longer travel distance and smaller vehicle sizes favor relatively lower EV TCO (Wu et al 2015 ), as do lower relative electricity-versus-gasoline price differentials (Lévay et al 2017 ). Despite these differences regarding TCO conclusions across studies, there is general agreement that future EV costs will decline (Dumortier et al 2015 , Wu et al 2015 , Elgowainy et al 2018 ).
The existing literature suggests future EV emissions will decline, in large part due to expectations for continued grid decarbonization (Elgowainy et al 2016 , 2018 , Woo et al 2017 , Cox et al 2018 ). For example, Ambrose et al ( 2020 ) anticipate that evolution in vehicle types and designs could accelerate future decreases for EV GHG emissions. Several studies also posit repurposing used EV batteries for stationary applications could accrue additional GHG benefits (Ahmadi et al 2014 , 2017 , Olsson et al 2018 , Kamath et al 2020 ). Cox et al ( 2018 ) suggest future connectivity and automation technologies will enable energy-optimized EV-recharging behavior and associated lower carbon emissions. Similarly, future EV costs also are expected to decline as battery costs continue to decline (cf section 4 ), and new mobility modes such as ride-hailing lead to higher vehicle use that favors the business case for highly efficient EVs compared to ICEVs.
8. Synergies with other technologies, macro trends, and future expectations
Vehicle electrification fits within broader electrification trends, including power-system decarbonization and mobility changes. The latter include micro-mobility in urban areas, new mobility business models revolving around 'shared' services as opposed to vehicle ownership (e.g. ride-hailing and car-sharing), ride pooling, and automation. These trends are driven partially by the larger availability of efficient and cost-effective electrified technologies (Mai et al 2018 ) and the prospect of abundant and affordable renewable electricity and by other technological and behavioral changes (e.g. real-time communication). Abundant and affordable renewable electricity is a conditio sine qua non for EVs to provide a pathway to decarbonize road transportation. Direct use of PV on-board vehicles (i.e. PV-powered or solar vehicles) also is being considered. However, this concept still faces many challenges (Rizzo 2010 , Aghaei et al 2020 ). Yamaguchi et al ( 2020 ) show potential synergies for integration but also highlight that for this technology to be successful, the development of high-efficiency (>30%), low‐cost, and flexible PV modules is essential.
Urban micro-mobility is emerging recently as an alternative to traditional mobility modes providing consumers in most metropolitan areas worldwide with convenient options for last-mile transportation (Clewlow 2019 , Zarif et al 2019 , Tuncer and Brown 2020 ). Virtually all micro-mobility solutions use all-electric powertrains. Shared electric scooters and bikes (often dockless), e.g. those pioneered by Lime and Bird in the U.S., are experiencing rapid success and are 'the fastest-ever U.S. companies to reach billion-USD valuations, with each achieving this milestone within a year of inception' (Ajao 2019 ). Future expectations for micro-mobility remain uncertain due to issues related to sidewalk congestion, safety, and vandalism (heavily impacting the business case for these technologies). However, the nexus with EVs has not been questioned.
Similarly, ride-hailing—matching drivers with passengers at short notice for one-off rides through a smartphone application, which date back to Uber's introducing the concept in 2009—is an attractive alternative to traditional transportation solutions. These mobility-as-a-service solutions cater to the consumer's need for quick, convenient, and cost-effective transportation and may lead to drops in car-ownership and driver-licensure rates (Garikapati et al 2016 , Clewlow and Mishra 2017 , Movmi 2018 , Walmsley 2018 , Henao and Marshall 2019 , Arevalo 2020 ). After just over 10 years, ride-hailing is widely available and extremely successful, with hundreds of millions of consumers worldwide and 36% of U.S. consumers having used ride-hailing services (Mazareanu 2019 ). While most ride-hailing vehicles today are ICEVs (in line with the existing LDV stock), many ride-hailing companies are exploring electrification opportunities (Slowik et al 2019 ). EVs offer a number of potential advantages as high vehicle usage promotes a more favorable business model for recovering the higher EV purchase price by leveraging cheaper fuel costs (Borlaug et al 2020 ). At the same time, long-range vehicles and effective charging solutions are required for ride-hailing companies to transition to EVs (Tu et al 2019 ). Moreover, EVs can mitigate additional fuel use and emissions related to increased travel, mostly due to deadheading, which is estimated to be ∼85% (Henao and Marshall 2019 ). EVs also provide access to restricted areas in some cities (driving some regional goals for ride-hailing electrification). For example, Uber aims for half of its London fleet to be electric by 2021 and 100% electric by 2025 (Slowik et al 2019 ).
Automation trends are also poised to have the potential to disrupt transportation as we know it. The combination of electric and connected automated vehicles (CAVs) is hypothesized to offer natural synergies, including easier integration with CAV sensors and a greater affinity for cheaper fuels aligning with greater travel (Sperling 2018 ). The chief counterargument relates to high power requirements for a heavily instrumented CAV, which would deplete EV batteries quickly and may be accommodated better with PHEV powertrains. Wireless EV charging, both stationary and dynamic, increases the potential synergies enabling autonomous recharging. Also, CAVs may be required to maximize the efficiency of dynamic wireless charging. In fact, without the alignment accuracy enabled by CAVs, in-road dynamic charging may have limited efficacy. The literature on these synergies is relatively sparse, though some studies are beginning to investigate the implications of combining EV and CAV technologies.
Even though the technology is not widely available commercially, several studies are beginning to examine how consumer preferences may be influenced by the combination of connected, automated, and electric vehicles 15 . Thiel et al ( 2020 ), for example, highlight how full EV success may emerge as automated shared vehicles become predominant in a world where the border between public and private transport will cease to exist. Tsouros and Polydoropoulou ( 2020 ) develop a survey combining traditional attributes (e.g. car type and vehicle style) alongside future technology attributes (e.g. fuel type and degree of automation) and estimate preferences using a latent-class structural-regression approach. They find a specific class of consumers, described as technology-savvy, who have a high proclivity for both alternative-fuel vehicle technologies and higher degrees of automation. While the proportion of the population that can be classified as technology-savvy is unclear, Tsouros and Polydoropoulou ( 2020 ) provide early compelling evidence that consumers see explicit value in the combination of EVs and automation. Hardman et al ( 2019 ) provide a complementary perspective of early adopters of automated vehicles based on a survey of existing U.S. EV owners. Similar to the work of Tsouros and Polydoropoulou ( 2020 ), Hardman et al ( 2019 ) find that the type of consumers who would pursue automated vehicles have similar lifestyles, attitudes, and socio-demographic profiles as EV adopters. These include high-income consumers, with high levels of knowledge about technology features, who have positive perceptions of CAV attributes and technology in general, provided that safety concerns are resolved.
Another benefit of the combined technologies is the potential to integrate charging events better with the needs of the electricity grid. Several studies assess the combination of these technologies with new mobility services such as car-sharing systems to optimize VGI. Iacobucci et al ( 2018 ) consider a case study in Tokyo of the ability of connected, automated EVs to be dispatched to respond to both transportation demand and charging to meet demands and constraints of the electricity system. The authors observe the vehicles can take advantage of a variety of different time-of-day pricing structures—leading to a tradeoff between wait times and cost benefits from lower fuel prices. They find that the vehicles in Tokyo can supply on the order of 3.5 MW of charging flexibility per 1000 vehicles, even during times of high mobility demand. Miao et al ( 2019 ) conduct a similar study in a generic region. The authors develop an algorithm that simulates operational behavior of the connected, automated EV technology that includes trip demand and vehicle usage, vehicle relocation, and vehicle charging. Their results indicate that charging behavior is highly sensitive to different levels of charging due to the length of charging—which can affect service provision of trip demand.
The final topic of study considering synergistic opportunities between connected, automated vehicles and EVs focused on emissions benefits. Taiebat et al 2018 explore the environmental impacts of automated vehicles showing net positive environmental impacts at the local vehicle-urban levels due to improved efficiency, but acknowledge that greater vehicle utilization and shifts in travel patterns might to offset some of these benefits. Of course, EVs provide the significant benefit of eliminating tailpipe criteria-pollutant emissions, yielding significant human-health benefits. Regarding GHG emissions, two of the earliest studies on this topic examine the net effect of automation on reducing transport GHG emissions (Brown et al 2014 , Wadud et al 2016 ). Greenblatt and Saxena ( 2015 ) conduct a case-study application of connected and automated vehicles in taxi fleets and find large emissions benefits associated with electrification. They find a decrease of GHG emissions intensities ranging from 87% to 94% below comparable ICEVs in 2014 and 63% to 82% below hybrid electric vehicles (HEVs) in 2030. The total emissions benefit is augmented relative to privately owned vehicles due to the higher travel intensity of taxi vehicles. Following these earlier works, additional case studies examine the hypothetical application of automated and electric fleets. These include two studies in Austin, Texas. Loeb and Kockelman ( 2019 ) examine a variety of scenarios to simulate the operation of different vehicle fleets replacing current-day transportation network companies and taxis. The primary goal of their work is to estimate costs associated with operation. They find that automated EVs are the most profitable and provide the best service among the vehicle-technology options that they examine. Gawron et al ( 2019 ) also perform a case study in Austin, Texas, but focus on the emissions benefits of electrifying an automated taxi fleet. They find that nearly 60% of emissions and energy in a base case CAV fleet can be reduced by electrifying powertrains. These improvements can be pushed up to 87% when coupled with grid decarbonization, dynamic ride-sharing, and various system- and technology-efficiency improvements. These results are consistent with a more generalized study by Stogios et al ( 2019 ), who, in a similar approach simulating fleet behavior, find that emissions from CAVs are most dramatically improved via electrification.
While EVs are a relatively new technology and automated vehicles are not widely available commercially, the implications and potential synergies of electrification and automation operating in conjunction are significant. The studies mentioned in this section are investigating a broad set of impacts when CAVs are coupled with EVs. Future research is necessary to generalize and refine many of these results. However, the potential for transformative changes to transportation emissions is clear.
8.1. Expectations for the future
EVs hold great promise to replace ICEVs for a number of on-road applications. EVs can provide a number of benefits, including addressing reliance on petroleum, improving local air quality, reducing GHG emissions, and improving driving experience. Vehicle electrification aligns with broader electrification and decarbonization trends and integrates synergistically with mobility changes, including urban micro-mobility, automation, and mobility-as-a-service solutions. The effective integration of EVs into power systems presents numerous opportunities for synergistic improvement of the efficiency and economics of electromobility and electric power systems, with EVs capable of supporting power-system planning and operations in several ways. Full exploitation of the synergies between EVs and VRE sources offers a path toward affordable and clean energy and mobility for all, as both technologies promise large-scale deployment in the future. To enable such a future continued technology progress, investments in charging infrastructure (and related building codes), consumer education, effective and secure VGI programs, and regulatory and business models supporting all aspects of vehicle electrification are all critical elements.
The coronavirus pandemic is impacting LDV sales in most countries negatively, and 2020 EV sales are expected to be lower than 2019, marking the first decline in a decade (BloombergNEF 2020 ). However, sales of ICEVs are set to drop even faster and, despite the crisis, EV sales could reach a record share of the overall LDV market in 2020 (Gul et al 2020 ). Despite these short-term setbacks, long-term prospects for EVs remain undiminished (BloombergNEF 2020 ).
Several studies project major roles for EVs in the future, which is reflected in massive investment in vehicle development and commercialization, charging infrastructure, and further technology improvement, especially in batteries and their supply chains. Consumer adoption and acceptance and technology progress form a virtuous self-reinforcing circle of technology-component improvements and cost reductions that can enable widespread adoption. Forecasting the future, including technology adoption, remains a daunting task. Nevertheless, this detailed review paints a positive picture for the future of EVs for on-road transport. The authors remain hopeful that technology, regulatory, societal, behavioral, and business-model barriers can be addressed over time to support a transition toward cleaner, more efficient, and affordable mobility solutions for all.
Acknowledgments
The authors thank Paul Denholm, Elaine Hale, Trieu Mai, Caitlin, Murphy, Bryan Palmintier, and Dan Steinberg for valuable comments on figure 6 , as well as two anonymous reviewers for helpful comments on the paper. This work was co-authored by National Renewable Energy Laboratory (NREL), which is operated by Alliance for Sustainable Energy, LLC, for U.S. Department of Energy (DOE) under Contract No. DE-AC36-08GO28308. No funding was received to support this work. The views expressed in this article do not necessarily represent the views of DOE or the U.S. Government. The findings and conclusions in this publication are those of the authors alone and should not be construed to represent any official U.S. Government determination or policy, or the views of any of the institutions associated with this study's authors.
EVs are defined as vehicles that are powered with an on-board battery that can be charged from an external source of electricity. This definition includes plug-in hybrid electric vehicles (PHEVs) and battery electric vehicles (BEVs). EVs often are referred to as plug-in electric vehicles (PEVs).
Transport electrification is confined not only to electric LDVs. Transport electrification includes a wide range of other vehicles, spanning from small vehicles that are used for urban mobility, such as three-wheelers, mopeds, kick-scooters, and e-bikes, to large urban buses and delivery vehicles. In 2019, the number of electric two-wheelers on the road exceeded 300 million and buses approached 0.6 million (IEA 2019 , Business Wire 2020 ), with new deliveries in 2019 close to 100 thousand units (EV Volumes 2020 ).
Just over 10% of the U.S. heavy-duty truck (Class 7–8) population requires an operating range of 500 miles (805 km) or more, while nearly 80% operate within a 200 mile (322 km) range and around 70% within 100 miles (161 km). Only ∼25% of heavy truck VMT require an operating range of over 500 miles (805 km) (Borlaug et al Forthcoming ).
As a counterargument, Tesla states that 'all new Tesla cars come standard with advanced hardware capable of providing Autopilot features today, and full self-driving capabilities in the future—through software updates designed to improve functionality over time'.
March 12, 2024
Electric Vehicles Beat Gas Cars on Climate Emissions over Time
New research says building electric vehicles leaves a bigger carbon footprint than making gas-powered cars, though EVs make up the difference in the long run
By Mike Lee & E&E News
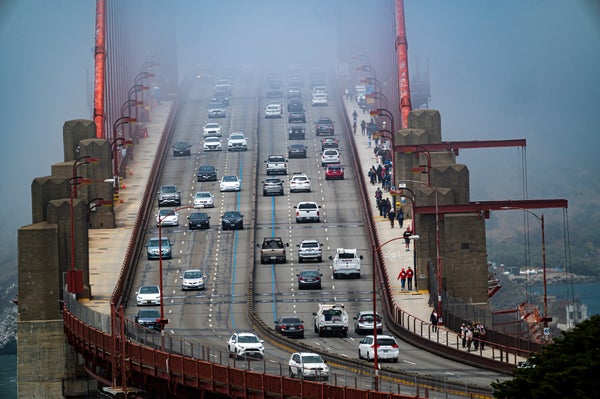
Traffic on the Golden Gate Bridge in San Francisco, California, US, on Thursday, June 29, 2023.
David Paul Morris/Bloomberg via Getty Images
CLIMATEWIRE | The production of battery-powered vehicles creates more carbon dioxide than making those that run on gasoline, a new report says. But EVs overcome the emissions difference relatively quickly.
An average EV produced in the U.S. in 2023 will close the gap in about 2.2 years or 25,000 miles, according to analysis released Monday by Bloomberg New Energy Finance.
After that, electric vehicles emit significantly less CO2 than internal combustion vehicles, particularly when considering how long a typical car stays in service. And the advantage likely will increase as electric generation becomes cleaner, the paper said.
On supporting science journalism
If you're enjoying this article, consider supporting our award-winning journalism by subscribing . By purchasing a subscription you are helping to ensure the future of impactful stories about the discoveries and ideas shaping our world today.
“Just like any car, you have to remember they’re going to be on the road about 10 years,” said Corey Cantor, a senior associate at BNEF who helped write the study.
The break-even period is longer in other countries — four years in the U.K., 5.1 years in Germany, 5.6 years in Japan and 9.6 years in China. But by 2030, it’s projected to be significantly shorter — two years in the U.K., 2.1 years in Germany, 3.1 years in Japan and 4.6 years in China.
The research addresses one of the central criticisms of electric vehicles — the amount of pollution created by the battery supply chain, which is still centered in China.
The payback period is slightly higher than the last time BNEF calculated the emissions from EVs, but that’s due in part to changes in methodology. The new version of the study is based on the larger batteries that are common in the U.S., for instance, and it assumes that batteries are produced in China rather than the United States.
Emissions from battery production are expected to fall as the Inflation Reduction Act forces companies to move production to the United States. The law also provides incentives to clean up the electric generation industry.
Reprinted from E&E News with permission from POLITICO, LLC. Copyright 2024. E&E News provides essential news for energy and environment professionals.
Print Preview
U.s. department of energy - energy efficiency and renewable energy, alternative fuels data center.
- Printable Version
- Production & Distribution
- Research & Development
- Benefits & Considerations
- Laws & Incentives
Electric Vehicle Research and Development
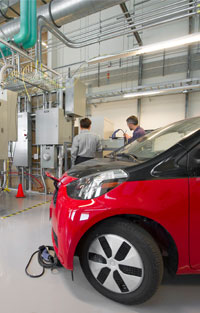
The U.S. Department of Energy (DOE) is working with its partners in the public and private sectors to research, develop, demonstrate, and deploy technologies that enhance the performance of electric-drive vehicles.
Environmental and Market Analysis
Electric vehicles (EVs) and hybrid electric vehicles (HEVs) offer reduced operating costs, fuel savings, and environmental benefits. For example, the National Renewable Energy Laboratory (NREL) and Idaho National Laboratory report, Levelized Cost of Charging Electric Vehicles in the United States , finds that driving an EV instead of a comparable conventional vehicle, can save a driver as much as $14,500 on fuel costs over 15 years. Additionally, Argonne National Laboratory’s (ANL) Autonomie model demonstrates that an all-electric vehicle is more than three times more efficient than its conventional counterpart. In Assessment of Light-Duty Plug-In Electric Vehicles in the United States, 2010-2019 , ANL estimates the electricity generation for the operation of an all-electric vehicle produces 53% less emissions than the tailpipe emissions from the operation of a gasoline vehicle.
Additionally, ANL’s An Extensive Study on Sizing, Energy Consumption, and Cost of Advanced Vehicle Technologies report demonstrates significant improvements in fuel economy over time. By 2045, HEVs could achieve a 43% to 81% improvement in fuel economy and PHEVs could achieve a 73% to 96% improvement in fuel economy. In its National Economic Value Assessment of Plug-In Electric Vehicles , NREL uses a scenario approach to estimate costs and benefits of increased EV market growth across the United States. Under various EV adoption scenarios, NREL estimates a 22% to 36% decrease in greenhouse gas emission reductions.
Further, the widespread adoption of EVs will require a robust network of charging stations, from home-based AC charging to DC powered extreme fast charging. Researchers are examining opportunities and impacts associated with a full range of charging technologies.
See the following resources for more information related to research in environmental and market analysis:
- Electric Vehicle Grid Integration
- Vehicle Electrification
- Transportation Energy Data Book: Edition 40
- National Economic Value Assessment of Plug-In Electric Vehicles
- An Extensive Study on Sizing, Energy Consumption, and Cost of Advanced Vehicle Technologies
- Cradle-to-Grave Lifecycle Analysis of U.S. Light-Duty Vehicle-Fuel Pathways: A Greenhouse Gas Emissions and Economic Assessment of Current (2015) and Future (2025-2030) Technologies
Maps & Data
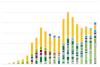
More Electricity Data | All Maps & Data
Case Studies
- Electric School Buses Clear the Air in the Mid-Atlantic
- Seattle Rideshare Fleet Adds EVs, Enjoys Success
- Massachusetts School Fleets Get Answers through Electric Bus Testing
More Electricity Case Studies | All Case Studies
Publications
- Electric Vehicles (Conceptos básicos sobre los vehículos eléctricos)
- Duluth Transit Authority Battery-Electric Bus Evaluation
- Foothill Transit Battery Electric Bus Demonstration Results: Second Report
More Electricity Publications | All Publications
- Laws and Incentives Search
- Electric Vehicle Charging Station Locations
Subscribe or renew today
Every print subscription comes with full digital access
Science News
How electric vehicles offered hope as climate challenges grew.
In the midst of a climate crisis, the EV began to gain traction

Volkswagen employees in Emden, Germany, learn how to produce electric cars, as automakers respond to new carbon dioxide emissions limits.
Sina Schuldt/picture alliance via Getty Images
Share this:
By Carolyn Gramling
December 22, 2021 at 7:00 am
This was another year of bleak climate news. Record heat waves baked the Pacific Northwest . Wildfires raged in California, Oregon, Washington and neighboring states. Tropical cyclones rapidly intensified in the Pacific Ocean. And devastating flash floods inundated Western Europe and China. Human-caused climate change is sending the world hurtling down a road to more extreme weather events, and we’re running out of time to pump the brakes, the Intergovernmental Panel on Climate Change warned in August ( SN: 9/11/21, p. 8 ).
The world needs to dramatically reduce its greenhouse gas emissions, and fast, if there’s any hope of preventing worse and more frequent extreme weather events. That means shifting to renewable sources of energy — and, importantly, decarbonizing transportation, a sector that is now responsible for about a quarter of the world’s carbon dioxide emissions.
But the path to that cleaner future is daunting, clogged with political and societal roadblocks, as well as scientific obstacles. Perhaps that’s one reason why the electric vehicle — already on the road, already navigating many of these roadblocks — swerved so dramatically into the climate solutions spotlight in 2021.
Just a few years ago, many automakers thought electric vehicles, or EVs, might be a passing fad, says Gil Tal, director of the Plug-in Hybrid & Electric Vehicle Research Center at the University of California, Davis. “It’s now clear to everyone that [EVs are] here to stay.”
Globally, EV sales surged in the first half of 2021, increasing by 160 percent compared with the previous year. Even in 2020 — when most car sales were down due to the COVID-19 pandemic — EV sales were up 46 percent relative to 2019. Meanwhile, automakers from General Motors to Volkswagen to Nissan have outlined plans to launch new EV models over the next decade: GM pledged to go all-electric by 2035, Honda by 2040. Ford introduced electric versions of its iconic Mustang and F-150 pickup truck.
Consumer demand for EVs isn’t actually driving the surge in sales, Tal says. The real engine is a change in supply due to government policies pushing automakers to boost their EV production. The European Union’s toughened CO 2 emissions laws for the auto industry went into effect in 2021, and automakers have already bumped up new EV production in the region. China mandated in 2020 that EVs make up 40 percent of new car sales by 2030. Costa Rica has set official phase-out targets for internal combustion engines.
In the United States, where transportation has officially supplanted power generation as the top greenhouse gas–emitting sector, President Joe Biden’s administration set a goal this year of having 50 percent of new U.S. vehicle sales be electric — both plug-in hybrid and all-electric — by 2030. That’s a steep rise over EVs’ roughly 2.5 percent share of new cars sold in the United States today. In September, California announced that by 2035 all new cars and passenger trucks sold in the state must be zero-emission.
There are concrete signs that automakers are truly committing to EVs. In September, Ford announced plans to build two new complexes in Tennessee and Kentucky to produce electric trucks and batteries. Climate change–related energy crises, such as the February failure of Texas’ power system, may also boost interest in EVs, Ford CEO Jim Farley said September 28 on the podcast Columbia Energy Exchange.
“We’re seeing more extreme weather events with global warming, and so people are looking at these vehicles not just for propulsion but for … other benefits,” Farley said. “One of the most popular features of the F-150 Lightning is the fact that you can power your house for three days” with the truck’s battery.
More to navigate
Although the EV market is growing fast, it’s still not fast enough to meet the Paris Agreement goals, the International Energy Agency reported this year. For the world to reach net-zero emissions by 2050 — when carbon emissions added to the atmosphere are balanced by carbon removal — EVs would need to climb from the current 5 percent of global car sales to 60 percent by 2030 , the agency found.
As for the United States, even if the Biden administration’s plan for EVs comes to fruition, the country’s transportation sector will still fall short of its emissions targets, researchers reported in 2020 in Nature Climate Change . To hit those targets, electric cars would need to make up 90 percent of new U.S. car sales by 2050 — or people would need to drive a lot less.
And to truly supplant fossil fuel vehicles, electric options need to meet several benchmarks. Prices for new and used EVs must come down. Charging stations must be available and affordable to all, including people who don’t live in homes where they can plug in. And battery ranges must be extended. Average ranges have been improving. Just five or so years ago, cars needed a recharge after about 100 miles; today the average is about 250 miles, roughly the distance from Washington, D.C., to New York City. But limited ranges and too few charging stations remain a sticking point.
Today’s batteries also require metals that are scarce, difficult to access or produced in mining operations rife with serious human rights issues . Although there, too, solutions may be on the horizon, including finding ways to recycle batteries to alleviate materials shortages ( SN: 12/4/21, p. 4 ).
EVs on their own are nowhere near enough to forestall the worst effects of climate change. But it won’t be possible to slow global warming without them.
And in a year with a lot of grim climate news — both devastating extreme events and maddeningly stalled political action — EVs offered one glimmer of hope.
“We have the technology. It’s not dependent on some technology that’s not developed yet,” Tal says. “The hope is that now we are way more willing to [transition to EVs] than at any time before.”
More Stories from Science News on Climate

Warm water is sneaking underneath the Thwaites Glacier — and rapidly melting it

‘The High Seas’ tells of the many ways humans are laying claim to the ocean

As the Arctic tundra warms, soil microbes likely will ramp up CO 2 production

A new approach to fighting wildfires combines local knowledge and AI

A ruinous hailstorm in Spain may have been supercharged by warming seas

Three reasons why the ocean’s record-breaking hot streak is devastating

Will stashing more CO 2 in the ocean help slow climate change?

A rapid shift in ocean currents could imperil the world’s largest ice shelf
Subscribers, enter your e-mail address for full access to the Science News archives and digital editions.
Not a subscriber? Become one now .
Suggestions or feedback?
MIT News | Massachusetts Institute of Technology
- Machine learning
- Social justice
- Black holes
- Classes and programs
Departments
- Aeronautics and Astronautics
- Brain and Cognitive Sciences
- Architecture
- Political Science
- Mechanical Engineering
Centers, Labs, & Programs
- Abdul Latif Jameel Poverty Action Lab (J-PAL)
- Picower Institute for Learning and Memory
- Lincoln Laboratory
- School of Architecture + Planning
- School of Engineering
- School of Humanities, Arts, and Social Sciences
- Sloan School of Management
- School of Science
- MIT Schwarzman College of Computing
Designing better batteries for electric vehicles
Press contact :.
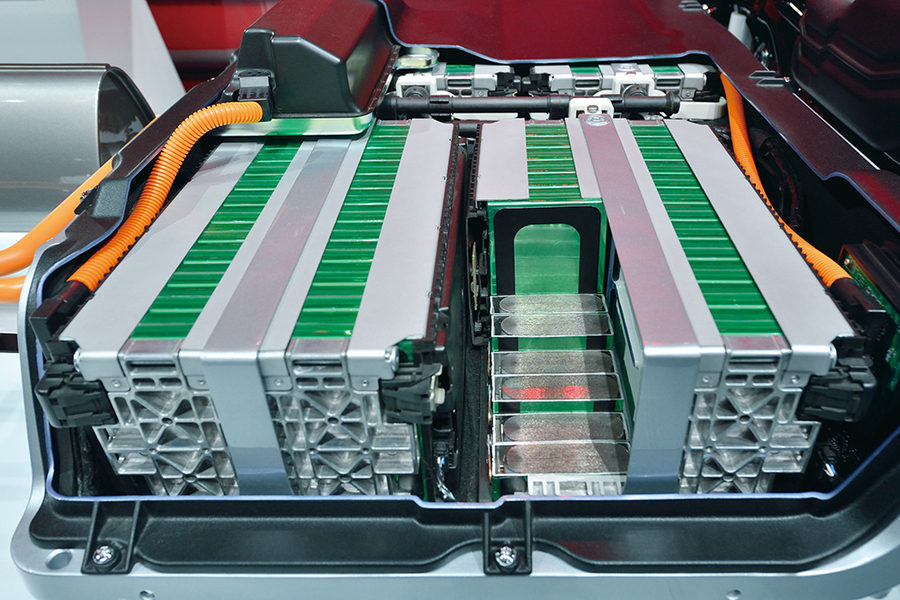
Previous image Next image
The urgent need to cut carbon emissions is prompting a rapid move toward electrified mobility and expanded deployment of solar and wind on the electric grid. If those trends escalate as expected, the need for better methods of storing electrical energy will intensify.
“We need all the strategies we can get to address the threat of climate change,” says Elsa Olivetti PhD ’07, the Esther and Harold E. Edgerton Associate Professor in Materials Science and Engineering. “Obviously, developing technologies for grid-based storage at a large scale is critical. But for mobile applications — in particular, transportation — much research is focusing on adapting today’s lithium-ion battery to make versions that are safer, smaller, and can store more energy for their size and weight.”
Traditional lithium-ion batteries continue to improve, but they have limitations that persist, in part because of their structure. A lithium-ion battery consists of two electrodes — one positive and one negative — sandwiched around an organic (carbon-containing) liquid. As the battery is charged and discharged, electrically charged particles (or ions) of lithium pass from one electrode to the other through the liquid electrolyte.
One problem with that design is that at certain voltages and temperatures, the liquid electrolyte can become volatile and catch fire. “Batteries are generally safe under normal usage, but the risk is still there,” says Kevin Huang PhD ’15, a research scientist in Olivetti’s group.
Another problem is that lithium-ion batteries are not well-suited for use in vehicles. Large, heavy battery packs take up space and increase a vehicle’s overall weight, reducing fuel efficiency. But it’s proving difficult to make today’s lithium-ion batteries smaller and lighter while maintaining their energy density — that is, the amount of energy they store per gram of weight.
To solve those problems, researchers are changing key features of the lithium-ion battery to make an all-solid, or “solid-state,” version. They replace the liquid electrolyte in the middle with a thin, solid electrolyte that’s stable at a wide range of voltages and temperatures. With that solid electrolyte, they use a high-capacity positive electrode and a high-capacity, lithium metal negative electrode that’s far thinner than the usual layer of porous carbon. Those changes make it possible to shrink the overall battery considerably while maintaining its energy-storage capacity, thereby achieving a higher energy density.
“Those features — enhanced safety and greater energy density — are probably the two most-often-touted advantages of a potential solid-state battery,” says Huang. He then quickly clarifies that “all of these things are prospective, hoped-for, and not necessarily realized.” Nevertheless, the possibility has many researchers scrambling to find materials and designs that can deliver on that promise.
Thinking beyond the lab
Researchers have come up with many intriguing options that look promising — in the lab. But Olivetti and Huang believe that additional practical considerations may be important, given the urgency of the climate change challenge. “There are always metrics that we researchers use in the lab to evaluate possible materials and processes,” says Olivetti. Examples might include energy-storage capacity and charge/discharge rate. When performing basic research — which she deems both necessary and important — those metrics are appropriate. “But if the aim is implementation, we suggest adding a few metrics that specifically address the potential for rapid scaling,” she says.
Based on industry’s experience with current lithium-ion batteries, the MIT researchers and their colleague Gerbrand Ceder, the Daniel M. Tellep Distinguished Professor of Engineering at the University of California at Berkeley, suggest three broad questions that can help identify potential constraints on future scale-up as a result of materials selection. First, with this battery design, could materials availability, supply chains, or price volatility become a problem as production scales up? (Note that the environmental and other concerns raised by expanded mining are outside the scope of this study.) Second, will fabricating batteries from these materials involve difficult manufacturing steps during which parts are likely to fail? And third, do manufacturing measures needed to ensure a high-performance product based on these materials ultimately lower or raise the cost of the batteries produced?
To demonstrate their approach, Olivetti, Ceder, and Huang examined some of the electrolyte chemistries and battery structures now being investigated by researchers. To select their examples, they turned to previous work in which they and their collaborators used text- and data-mining techniques to gather information on materials and processing details reported in the literature. From that database, they selected a few frequently reported options that represent a range of possibilities.
Materials and availability
In the world of solid inorganic electrolytes, there are two main classes of materials — the oxides, which contain oxygen, and the sulfides, which contain sulfur. Olivetti, Ceder, and Huang focused on one promising electrolyte option in each class and examined key elements of concern for each of them.
The sulfide they considered was LGPS, which combines lithium, germanium, phosphorus, and sulfur. Based on availability considerations, they focused on the germanium, an element that raises concerns in part because it’s not generally mined on its own. Instead, it’s a byproduct produced during the mining of coal and zinc.
To investigate its availability, the researchers looked at how much germanium was produced annually in the past six decades during coal and zinc mining and then at how much could have been produced. The outcome suggested that 100 times more germanium could have been produced, even in recent years. Given that supply potential, the availability of germanium is not likely to constrain the scale-up of a solid-state battery based on an LGPS electrolyte.
The situation looked less promising with the researchers’ selected oxide, LLZO, which consists of lithium, lanthanum, zirconium, and oxygen. Extraction and processing of lanthanum are largely concentrated in China, and there’s limited data available, so the researchers didn’t try to analyze its availability. The other three elements are abundantly available. However, in practice, a small quantity of another element — called a dopant — must be added to make LLZO easy to process. So the team focused on tantalum, the most frequently used dopant, as the main element of concern for LLZO.
Tantalum is produced as a byproduct of tin and niobium mining. Historical data show that the amount of tantalum produced during tin and niobium mining was much closer to the potential maximum than was the case with germanium. So the availability of tantalum is more of a concern for the possible scale-up of an LLZO-based battery.
But knowing the availability of an element in the ground doesn’t address the steps required to get it to a manufacturer. So the researchers investigated a follow-on question concerning the supply chains for critical elements — mining, processing, refining, shipping, and so on. Assuming that abundant supplies are available, can the supply chains that deliver those materials expand quickly enough to meet the growing demand for batteries?
In sample analyses, they looked at how much supply chains for germanium and tantalum would need to grow year to year to provide batteries for a projected fleet of electric vehicles in 2030. As an example, an electric vehicle fleet often cited as a goal for 2030 would require production of enough batteries to deliver a total of 100 gigawatt hours of energy. To meet that goal using just LGPS batteries, the supply chain for germanium would need to grow by 50 percent from year to year — a stretch, since the maximum growth rate in the past has been about 7 percent. Using just LLZO batteries, the supply chain for tantalum would need to grow by about 30 percent — a growth rate well above the historical high of about 10 percent.
Those examples demonstrate the importance of considering both materials availability and supply chains when evaluating different solid electrolytes for their scale-up potential. “Even when the quantity of a material available isn’t a concern, as is the case with germanium, scaling all the steps in the supply chain to match the future production of electric vehicles may require a growth rate that’s literally unprecedented,” says Huang.
Materials and processing
In assessing the potential for scale-up of a battery design, another factor to consider is the difficulty of the manufacturing process and how it may impact cost. Fabricating a solid-state battery inevitably involves many steps, and a failure at any step raises the cost of each battery successfully produced. As Huang explains, “You’re not shipping those failed batteries; you’re throwing them away. But you’ve still spent money on the materials and time and processing.”
As a proxy for manufacturing difficulty, Olivetti, Ceder, and Huang explored the impact of failure rate on overall cost for selected solid-state battery designs in their database. In one example, they focused on the oxide LLZO. LLZO is extremely brittle, and at the high temperatures involved in manufacturing, a large sheet that’s thin enough to use in a high-performance solid-state battery is likely to crack or warp.
To determine the impact of such failures on cost, they modeled four key processing steps in assembling LLZO-based batteries. At each step, they calculated cost based on an assumed yield — that is, the fraction of total units that were successfully processed without failing. With the LLZO, the yield was far lower than with the other designs they examined; and, as the yield went down, the cost of each kilowatt-hour (kWh) of battery energy went up significantly. For example, when 5 percent more units failed during the final cathode heating step, cost increased by about $30/kWh — a nontrivial change considering that a commonly accepted target cost for such batteries is $100/kWh. Clearly, manufacturing difficulties can have a profound impact on the viability of a design for large-scale adoption.
Materials and performance
One of the main challenges in designing an all-solid battery comes from “interfaces” — that is, where one component meets another. During manufacturing or operation, materials at those interfaces can become unstable. “Atoms start going places that they shouldn’t, and battery performance declines,” says Huang.
As a result, much research is devoted to coming up with methods of stabilizing interfaces in different battery designs. Many of the methods proposed do increase performance; and as a result, the cost of the battery in dollars per kWh goes down. But implementing such solutions generally involves added materials and time, increasing the cost per kWh during large-scale manufacturing.
To illustrate that trade-off, the researchers first examined their oxide, LLZO. Here, the goal is to stabilize the interface between the LLZO electrolyte and the negative electrode by inserting a thin layer of tin between the two. They analyzed the impacts — both positive and negative — on cost of implementing that solution. They found that adding the tin separator increases energy-storage capacity and improves performance, which reduces the unit cost in dollars/kWh. But the cost of including the tin layer exceeds the savings so that the final cost is higher than the original cost.
In another analysis, they looked at a sulfide electrolyte called LPSCl, which consists of lithium, phosphorus, and sulfur with a bit of added chlorine. In this case, the positive electrode incorporates particles of the electrolyte material — a method of ensuring that the lithium ions can find a pathway through the electrolyte to the other electrode. However, the added electrolyte particles are not compatible with other particles in the positive electrode — another interface problem. In this case, a standard solution is to add a “binder,” another material that makes the particles stick together.
Their analysis confirmed that without the binder, performance is poor, and the cost of the LPSCl-based battery is more than $500/kWh. Adding the binder improves performance significantly, and the cost drops by almost $300/kWh. In this case, the cost of adding the binder during manufacturing is so low that essentially all the of the cost decrease from adding the binder is realized. Here, the method implemented to solve the interface problem pays off in lower costs.
The researchers performed similar studies of other promising solid-state batteries reported in the literature, and their results were consistent: The choice of battery materials and processes can affect not only near-term outcomes in the lab but also the feasibility and cost of manufacturing the proposed solid-state battery at the scale needed to meet future demand. The results also showed that considering all three factors together — availability, processing needs, and battery performance — is important because there may be collective effects and trade-offs involved.
Olivetti is proud of the range of concerns the team’s approach can probe. But she stresses that it’s not meant to replace traditional metrics used to guide materials and processing choices in the lab. “Instead, it’s meant to complement those metrics by also looking broadly at the sorts of things that could get in the way of scaling” — an important consideration given what Huang calls “the urgent ticking clock” of clean energy and climate change.
This research was supported by the Seed Fund Program of the MIT Energy Initiative (MITEI) Low-Carbon Energy Center for Energy Storage ; by Shell, a founding member of MITEI; and by the U.S. Department of Energy’s Office of Energy Efficiency and Renewable Energy, Vehicle Technologies Office, under the Advanced Battery Materials Research Program. The text mining work was supported by the National Science Foundation, the Office of Naval Research, and MITEI.
This article appears in the Spring 2021 issue of Energy Futures , the magazine of the MIT Energy Initiative.
Share this news article on:
Related links.
- Elsa Olivetti
- MIT Energy Initiative
- Department of Materials Science and Engineering
- Energy Futures magazine
Related Topics
- Climate change
- Electric vehicles
- Automobiles
- Transportation
- Energy storage
- Lithium-ion
- Renewable energy
- Sustainability
- Supply chains
- Manufacturing
Related Articles
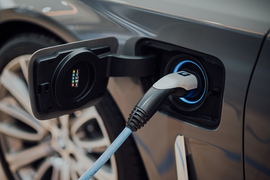
China’s transition to electric vehicles
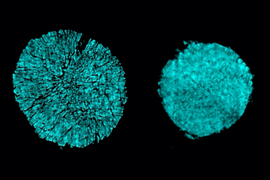
Design could enable longer lasting, more powerful lithium batteries
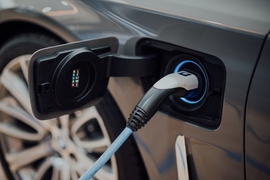
To boost emissions reductions from electric vehicles, know when to charge
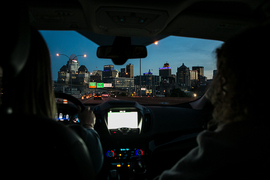
3 Questions: The price of privacy in ride-sharing app performance
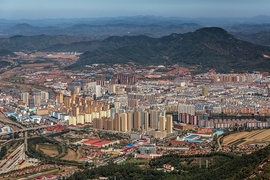
Transportation policymaking in Chinese cities
Previous item Next item
More MIT News
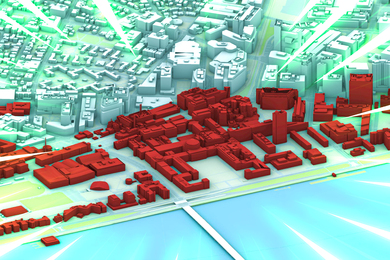
Students research pathways for MIT to reach decarbonization goals
Read full story →
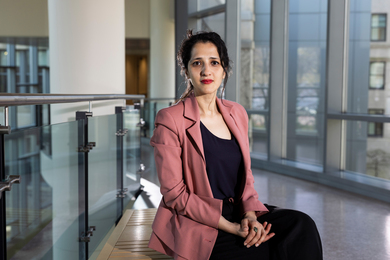
Improving working environments amid environmental distress
A data-driven approach to making better choices
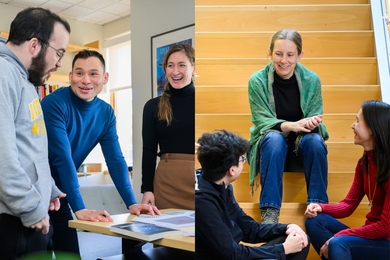
Paying it forward
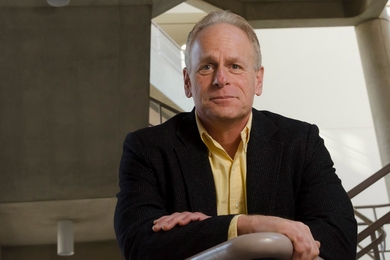
John Fucillo: Laying foundations for MIT’s Department of Biology
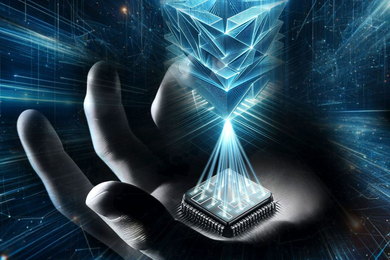
Researchers demonstrate the first chip-based 3D printer
- More news on MIT News homepage →
Massachusetts Institute of Technology 77 Massachusetts Avenue, Cambridge, MA, USA
- Map (opens in new window)
- Events (opens in new window)
- People (opens in new window)
- Careers (opens in new window)
- Accessibility
- Social Media Hub
- MIT on Facebook
- MIT on YouTube
- MIT on Instagram
Thank you for visiting nature.com. You are using a browser version with limited support for CSS. To obtain the best experience, we recommend you use a more up to date browser (or turn off compatibility mode in Internet Explorer). In the meantime, to ensure continued support, we are displaying the site without styles and JavaScript.
- View all journals
- My Account Login
- Explore content
- About the journal
- Publish with us
- Sign up for alerts
- Open access
- Published: 23 May 2023
Using machine learning methods to predict electric vehicles penetration in the automotive market
- Shahriar Afandizadeh ORCID: orcid.org/0000-0001-5137-3673 1 ,
- Diyako Sharifi 1 ,
- Navid Kalantari 2 &
- Hamid Mirzahossein ORCID: orcid.org/0000-0003-1615-9553 3
Scientific Reports volume 13 , Article number: 8345 ( 2023 ) Cite this article
9350 Accesses
6 Citations
2 Altmetric
Metrics details
- Engineering
- Environmental social sciences
Electric vehicles (EVs) have been introduced as an alternative to gasoline and diesel cars to reduce greenhouse gas emissions, optimize fossil fuel use, and protect the environment. Predicting EV sales is momentous for stakeholders, including car manufacturers, policymakers, and fuel suppliers. The data used in the modeling process significantly affects the prediction model’s quality. This research’s primary dataset contains monthly sales and registrations of 357 new vehicles in the United States of America from 2014 to 2020. In addition to this data, several web crawlers were used to gather the required information. Vehicles sale were predicted using long short-term memory (LSTM) and Convolutional LSTM (ConvLSTM) models. To enhance LSTM performance, the hybrid model with a new structure called “Hybrid LSTM with two-dimensional Attention and Residual network” has been proposed. Also, all three models are built as Automated Machine Learning models to improve the modeling process. The proposed hybrid model performs better than the other models based on the same evaluation units, including Mean Absolute Percentage Error, Normalized Root Mean Square Error, R-square, slope, and intercept of fitted linear regressions. The proposed hybrid model has been able to predict the share of EVs with an acceptable Mean Absolute Error of 3.5%.
Similar content being viewed by others
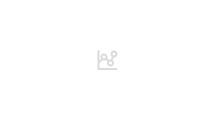
Accurate structure prediction of biomolecular interactions with AlphaFold 3
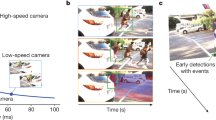
Low-latency automotive vision with event cameras
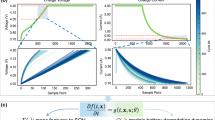
Physics-informed neural network for lithium-ion battery degradation stable modeling and prognosis
Introduction.
Emissions of greenhouse gases are increasing rapidly worldwide. According to a United States Environmental Protection Agency report released in 2020, the transportation sector produces about 27% of the entire greenhouse gas emissions in the country, which, compared to other sectors, transportation emits the most greenhouse gases 1 . EVs were introduced as an alternative to gasoline and diesel cars to reduce air pollution and greenhouse gas emissions, optimize the use of natural energy resources and protect the environment. Using electricity generated from renewable energy sources such as wind, water and sunlight for EVs can be one of the most efficient solutions to reduce emissions and climate change 2 . Although much time has passed since the invention of EVs, internal combustion vehicles are still the most popular. EV sales have been on the rise, and in January 2017, the total number of EVs sold worldwide reached two million 3 . Globally, EV sales accounted for 9% of the car market in 2021, a fourfold increase from 2019 4 .
Designing and producing vehicles is time-consuming and requires much investment, so by predicting the number of sales, automobile companies can optimize production, Furthermore, by accurately predicting the penetration of EVs in the market, it is possible to estimate their impact on reducing pollution in the coming years, which is very important from an environmental standpoint. Forecasting the sale of EVs and their penetration into the automotive market has been a significant issue for governments, policymakers, and car manufacturers to plan the production of EVs, set proper policies, and provide sufficient energy and infrastructure.
The main goal of this research is to apply Machine Learning (ML) methods to build an efficient prediction model to estimate the sale of all vehicles in the dataset, the share of EVs in each segment, and determine the main factors that influence the sales of each EV. The effect of a limited number of influencing factors on vehicle sales was examined in previous studies using different models. For this study, a wide range of information was collected, including all factors that previous studies have proven are related to car sales, and it was used in modeling. LSTM and ConvLSTM, powerful Deep Learning (DL) models, have been used for predicting vehicle sales. By combining the two-dimensional Attention model and the Residual network as the proposed hybrid model, it has been tried to improve the performance of the LSTM model. Additionally, using the collected information and the model sensitivity analysis, it was attempted to determine the most influential factors on the sale of each EV.
The literature review of this study includes two general sections. The first section examines ML methods used to predict vehicle sales, and the second section provides an overview of the features used in other methods to predict EV sales.
ML methods in predicting vehicle sales
Several studies have used ML methods to predict the sales of EVs as time-series data. Multiple Linear Regression and Support Vector Machine (SVM) models were compared for predicting vehicle sales using yearly, quarterly and monthly data (the number of new automobile registrations, the number of automobile sales, and economic indicators such as Gross Domestic Product (GDP), Available Personal Income, Consumer Price Index, Interest Rate, Unemployment Rate, Industrial Investment Demand, Petroleum Charge, Private Consumption, and Latent Replacement Demand) in a study by Brühl et al. 5 According to the results, the SVM model had better performance based on the error values (Mean Absolute Error and Mean Absolute Percentage Error), was more interpretable, and gave better results based on quarterly data. In the study of Wang et al. ML techniques were used to predict car sales based on sales quantity, economic indicators, wholesale population, unemployment rate, exchange rate, the prices of vehicles, the oil prices, and the prices of vehicle components. Based on evaluation units (R-square and Mean Squared Error), they evaluated the prediction quality of adaptive network-based fuzzy inference system (ANFIS), Artificial Neural Networks (ANN), and autoregressive integrated moving average models; the results showed that ANFIS performed better than the other models 6 . In another study, Hülsmann et al. compared the performance of linear models, such as Ordinary Least Squares and Quantile Regression, against ML methods like SVM, Decision Tree, k–Nearest Neighbor, and Random Forest for predicting vehicle sales. Based on the monthly data of vehicle sales, new car registrations, and economic indicators (such as GDP, Personal Income and Dow Jones), the Decision Tree of ML methods performed better than the other models based on Mean Absolute Percentage Error (MAPE) 7 .
Moreover, Kitabci et al. analysed the impact of economic policies on vehicle sales in Turkey as a macro-environmental factor by multiple regression and neural network methods. They assessed factors such as the vehicle loan rate presented by the banks, the income of the consumers, the tax deductions made by the government for the automobile, the inflation rate, automobile prices, the euro exchange rate, oil prices, and advertisements spent by the businesses. According to the results, neural networks were more accurate in predicting sales than regression models; some factors, including the euro exchange rate, the rates of vehicle loans offered by banks, and the government's tax deductions, have influenced automobile sales 8 . In another research, Bas et al. applied classification ML methods to predict EV adoption using ride-sourcing factors, underlying sociodemographics, and vehicle characteristics; they examined the contributions of different factors to predict outcomes using a method called “Local Interpretable Model-Agnostic Explanations”. Based on the study’s findings, ML models produced highly accurate predictions regarding EV adoption, and the frequent usage of ride-sourcing, knowledge about EVs, and environmental protection awareness were significant factors in explaining the tendency to adopt EVs 9 . In addition, Zhang et al. applied Singular Spectrum Analysis as a univariate time-series model and the Vector Auto-Regression model (VAR) as a multivariate model for forecasting EV sales. According to the results, the VAR model can significantly improve the prediction accuracy because it considers the effect of economic indicators, such as consumer prices, consumer confidence, producers' prices, fuel and vehicle prices, and Baidu data (An indicator of consumer interest and curiosity in EVs) 10 .
In another study, Kaya et al. 11 used the exchange rate, the GDP, the Consumer Confidence Index, the Consumer Price Index data and a Deep Neural Network model to predict vehicle sales; the results revealed that this ML model predicted sales accurately (based on Mean Squared Error). In another research, Xia et al. introduced the ForeXGBoost model, a vehicle sales prediction system based on large-scale datasets containing comprehensive vehicle information, including brand ID, model, engine power, and displacement. Based on Logarithmic Difference Square Root, MAPE, and running time, the XGBoost model outperforms benchmark algorithms like Linear Regression and Gradient Boosting Decision Trees 12 . Using online survey data and ML methods such as SVM, ANN, Deep Neural Networks, Gradient Boosting Models, and Random Forests, Bas et al. compared different methods for classifying potential EV buyers and identifying the features that affect the adoption of EVs. Results showed that the SVM model outperforms the other algorithms; having only partial information (e.g. only socioeconomic factors) reduces model performance, while synergy across multiple variables increases accuracy 13 . Additionally, Saxena et al. present a study that examines the use of deep learning-based models, including Autoregressive Integration Moving Averages and LSTM models, to predict future directions of vehicle sales. Based on the implementation results, the MAE and the Root Mean Square Error for LSTM-based time series forecasting were reduced, and this model could accurately predict green vehicle sales 14 .
Factors affecting the sale of EVs in other methods
Developing policies requires understanding users' behavior and prioritizing their choices. Therefore, some previous studies used survey data to predict EV demand. To assess the potential demand for EVs, Beggs et al. 15 used survey data and vehicle specifications, such as seat capacity, maximum speed, purchase price, and operating costs. In a similar study, the demand for EVs was estimated based on consumer preferences for vehicle attributes by Calfee et al. 16 The results of this research have shown that the weak performance of EVs limits their demand; however, if EVs become significantly more advanced than other cars or if gasoline becomes scarce, the demand for these vehicles will increase.
Predicting the future demand for EVs is a complex issue. As most studies for new technologies rely on survey data, market share predictions will reflect the share in the survey data, not the actual market share. Consumer opinions and the news published about EVs also influence the sales of these vehicles. Based on Mau et al. 17 research, EV sales are impacted by published information about the penetration rate of EVs, known as the “The neighbor effect”. Electric vehicles' specifications are another factor affecting their sales. According to Balducci et al. 18 study to assess plug-in hybrid EV penetration scenarios in the auto market, fuel economy and reduced motor vehicle emissions are the most important factors when purchasing hybrid EVs, while insufficient engine power, high price, and unreliability are the most important reasons for not purchasing these vehicles. Furthermore, Hess et al. used vehicle specifications such as purchase price, vehicle purchase incentives, Miles Per Gallon (MPG) or equivalent, fuel cost per year, fuel availability, refueling time, driving range, maintenance cost per year, and acceleration to explore consumers' preferences in choosing the type of vehicle and the type of fuel. The results have shown that consumers' choices are adversely affected by factors such as purchase price, operating cost, and vehicle age, whereas their choices are positively affected by factors such as better vehicle acceleration, purchase incentives, driving range, and fuel availability 19 .
The sale of EVs is also affected by improving vehicle engine performance and reducing fuel consumption. Using a discrete choice model, Bas et al. investigated EV penetration in the face of new technology for reducing fuel consumption. Results demonstrated a clear tradeoff between the cost of a gasoline-powered system and the fuel savings it provides is perceived by potential purchasers 20 . However, potential EV purchasers are not in this category since their cost–benefit analysis is adverse due to the low cost of electricity 20 . Also, the estimated market shares give a significant share of the market to alternatives that include technology to reduce consumption, due to a more favorable attitude toward environmentally friendly technologies 20 . Additionally, Shafiei et al. analysed the impact of factors such as fuel prices, vehicle attributes, consumer preferences, and social influences on the market share of EVs. The results showed that the combination of high gasoline prices, decreasing EV prices, dropping tax on EVs and eliminating consumer concerns about recharging has the most significant effect on the market share of EVs 21 . Kinski et al. 22 research shows that the information related to searching on the Internet (Google Trends) for vehicles has a positive and significant relationship with car sales.
Based on the previous research, the following two general conclusions were reached:
Firstly, ML and DL methods have been proven to be effective at predicting vehicle sales. Therefore, LSTM and ConvLSTM, powerful DL models, have been used for predicting vehicle sales in this research. Furthermore, a hybrid model was also proposed, and all three models were compared in terms of performance.
Secondly, factors and features that affect EV sales have been identified, and these features have been collected and used in this research.
Methodology
Artificial Intelligence (AI) refers to the ability of machines to perceive, synthesize, and infer information, as opposed to animals and humans displaying intelligence 23 . Machine learning, artificial neural networks, and deep learning are important tools in the development of AI systems and have been shown to perform well in predicting time series data such as vehicle sales. Recurrent neural networks (RNNs) are a type of neural network that remember what they have already processed and can learn from previous iterations 24 . In other words, an RNN is a class of ANNs where connections between nodes form a directed graph along a temporal sequence; this allows it to exhibit temporal dynamic behavior 24 .
Hochreiter and Schmidhuber introduced the LSTM network, a RNN capable of learning long-term dependencies and predicting sequential data with great accuracy 25 . An LSTM is an extension of an RNN, capable of learning patterns from long sequences of source data by retaining a long-term memory 25 . LSTMs improved the forgetfulness of RNNs. An RNN could retain a memory, but only for its immediate past. An LSTM, on the other hand, introduces loops to generate long-term gradients. While going through its loops, it can discover long-term patterns 25 . LSTM is good at storing past information and performing well when faced with vanishing gradient issues. During ANN training, each weight of the neural network receives an update proportional to the partial derivative of the error function. Vanishing gradients occur when gradients become vanishingly small, effectively preventing the weight from changing 26 .
LSTM can tie together three pieces of information at each time step: the current input data, the short-term memory it receives from the previous cell (the hidden state), and the long-term memory from cells farther away (the cell state) 27 . The LSTM unit consists of an input gate a forget gate, an output gate, and a cell state. The input gate determines how much information should be transferred from the current candidate cell state to the current cell state. The forget gate determines how much historical information should be ignored from the previous cell state. The output flow from cells to the rest of the network can be controlled through the output gate. By regulating the flow of information through the three gates, important information over time intervals can be remembered. According to Eqs. 1 – 6 , the LSTM unit process data in cell state and gates 27 . Reference 27 provides more details.
In the above equations, \(f_{t}\) , \(i_{t}\) , and \(o_{t}\) are the forget, input, and output gates, respectively; \(C_{t}\) , \(C_{t - 1}\) , and \(\tilde{C}_{t}\) are the current, previous, and candidate cell state; \(\sigma\) and tanh denotes sigmoid and hyperbolic tangent activation functions, respectively; the interconnected weight matrices for each gate and cell state are \(W_{fh}\) , \(W_{ih}\) , \(W_{oh}\) , \(W_{Ch}\) , respectively; \(W_{fx}\) , \(W_{ix}\) , \(W_{ox}\) , \(W_{Cx}\) represent the input weight matrices in the three gates and the cell state, respectively; \(b_{f}\) , \(b_{i}\) , \(b_{o}\) , \(b_{C}\) represent the respective bias terms; the Hadamard (element product) product of a matrix is denoted by \(\odot\) 27 . According to Fig. 1 , the input layer is an LSTM layer with the same number of neurons as the input data features. In the next step, one or more LSTM layers are set as the hidden layers, and in the final step, a Dense layer with the ReLU activation function is set as the output layer.
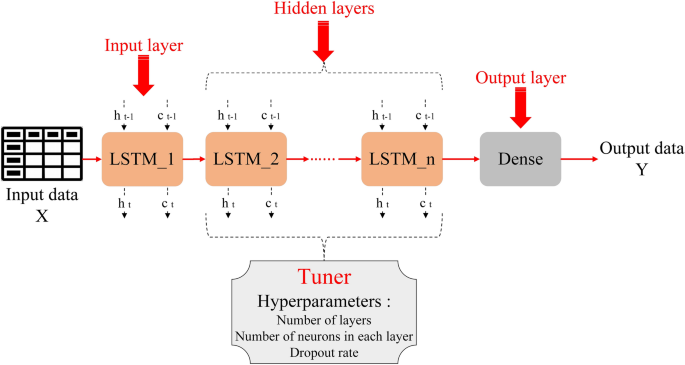
Architecture of the LSTM model.
The LSTM model is powerful for handling temporal correlation. In addition, when working with time series data with numerous features, LSTM model performance can be improved by converting the two-dimensional data to a three-dimensional tensor (Fig. 2 illustrates this), connecting states, and applying convolutional operations; this idea was the reason for creating the ConvLSTM model 28 . The ConvLSTM neural network is a fully connected LSTM network with a convolutional structure inside the LSTM cell, which does well in predicting data with temporal correlation. ConvLSTM provides a fully connected extension for data transfer between states and from inputs to states 28 . In other words, ConvLSTM determines the future state of each cell in the grid based on its inputs and neighbours' past states; this can be done by using a convolution operator in the state-to-state and input-to-state transitions 28 . In the ConvLSTM model, data in the input unit, the outputs of each cell, the hidden units, and the gates are arranged as three-dimensional tensors. ConvLSTM has similar parameters as LSTM, and the difference is in how data is transferred and convolutional multiplication is used in calculations, as expressed in Eqs. 7 – 11 28 . Reference 28 provides more details.
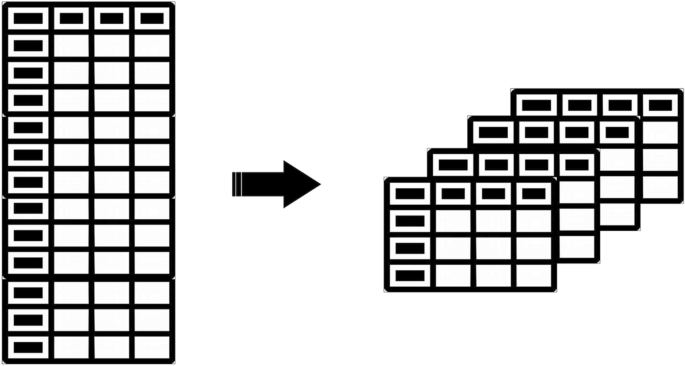
Transforming 2-D matrix into 3-D tensor.
In ConvLSTM equations, * indicates the convolution operator, and \(\odot\) indicates the Hadamard product. As shown in Fig. 3 , the input layer is a ConvLSTM layer, the hidden layers are Dense and ConvLSTM layers, and the output layer is a Dense layer with the ReLU activation function.
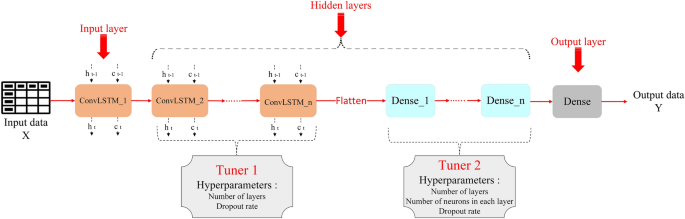
Architecture of the ConvLSTM model.
Hybrid LSTM with two-dimensional attention and residual network
Time series data have a meaningful temporal relationship. In this research, the data were transformed into three-dimensional tensors with a seven-month time window to maintain the temporal relationship; how to transform a two-dimensional matrix to a three-dimensional tensor is shown in Fig. 2 . As an innovation, the “Two-Dimensional Attention” method has been proposed in this research to determine the importance of each car's feature in a seven-month time frame and to use the weighted data in the modeling process. The two-dimensional attention method assigns weights to each feature in the time window based on how much it influences the model, allowing the features with a more significant impact to receive more attention and reduce the model's complexity. The one-dimensional attention model was proposed for the first time by Bahdanau to address the problem of the limited access of the decoder to the model's input information when the encoder vector has a fixed length in the translation machine 29 .
In the LSTM model architecture, which is shown in Fig. 1 , several LSTM layers are placed inside the hidden layer. When the number of LSTM layers in the hidden layer increases, the primary layers (the layers adjacent to the input layer) have a lesser effect on the output. The primary layers have processed the input data and learned the relationship between the data well, which is why it has been tried to improve this problem by using the Residual network in the proposed hybrid model. Using the Residual Network, the weighted data and outputs of the primary layers have been transferred to the final layers in the proposed hybrid model, as shown in Fig. 4 .
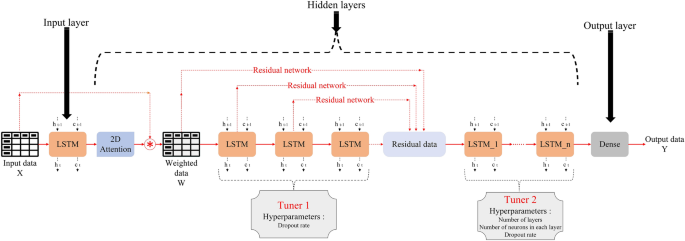
Primary architecture of the hybrid model.
In this study, each input \(x\) is represented by an \(m \times n\) matrix, where m corresponds to the previous months in the window (7), and n represents the number of vehicle features. After entering the data into the first LSTM layer, the processing is done according to Eqs. 1 –6, and the encoded hidden unit ( \(h\) ) with the exact dimensions ( \(m \times n\) ) is entered into the Attention layer. After that, the alignment score is calculated according to Eq. ( 12 ).
In Eq. ( 12 ), \(e_{i, j}\) represents the alignment score, \(W_{a}\) is the attention model’s weight (as a trainable variable), \(h\) is the encoded hidden unit of the primary LSTM layer, \(b_{a}\) is the attention model's bias (as a trainable variable), and the sign "*" denotes the Hadamard product. Since the input data for the attention layer has been encoded by an LSTM layer using tanh nonlinear activation function, tanh has also been used in the attention layer to facilitate data reading during decoding. Each input data element was assigned a degree of attention using Eq. ( 13 ).
Multiplying attention matrix \(\alpha_{i, j}\) by raw data matrix \(x_{i, j}\) yields a weighted data matrix \(W_{i, j}\) based on Eq. ( 14 ). The sign “*” denotes the Hadamard product.
Weighted data \(W_{i, j}\) is then passed through three layers of LSTM as a Residual Network; the output of each layer is combined with the weighted data at the end of the Residual Network and entered into one or more LSTM layers. A Dense layer with the ReLU activation function is the output layer. An overview of the model's architecture is illustrated in Fig. 4 .
Other architectures have also been tried in the hybrid model structure, but they were not more efficient, so only the best architecture has been mentioned.
In this study, EVs are considered as vehicles that use electric motors for propulsion and include all types of EVs. In predicting the sale of vehicles, the number of vehicles in the warehouses is an influential factor, which was not used in this modeling due to a lack of access. Since ML models are based on training, in this study, the models can predict the sales of vehicles that have been on the market for at least 24 months. Emerging vehicles (vehicles that have been on the market for less than 24 months) and cars that have not yet entered the market were not included in the modeling due to insufficient data to train the model. Therefore, the share of EVs in the Automotive Market is expressed as a share in vehicle segments and not as a share of EVs overall.
A wide range of information related to car sales has been used in this research. In the primary dataset, all the data is related to new cars, not used cars. The primary dataset contains monthly information about 357 vehicles, such as brand (or "make" in auto industry lingo, e.g., Benz), model, segmentation, category, shoppers, and sales of different types of cars in the United States from 2014 to 2020. Other information has been extracted based on the cars in this dataset. The data before the outbreak of Covid-19 disease were used since this disease had adverse impacts on the global economy.
As stated in previous studies, vehicle specifications are very effective in car sales prediction models. Vehicle specifications are changed annually. According to Alexa rating 30 and the comprehensiveness of the information presented on the “Thecarconnection” website 31 , vehicle specifications were collected through this website. In order to save time and automate the collection of information due to a large number of vehicles and changes in specifications of vehicles over time, several web crawler have been designed and used in Python programming language to collect vehicle information. Several vehicle specifications of the "CAR-MID/FULL SIZE" segment are shown in Table 1 .
There is similar information collected for gasoline and EVs; for example, the equivalent MPG in EVs. Price, MPG, max mileage, engine power, and warranty are some of the main features taken into account. Other specifications have been divided into the "safety specifications" and the "other specifications" categories. The safety specifications category includes child safety rear door locks, airbags, ABS brakes, daytime running lights, night vision, driver monitoring alerts, collision mitigation braking system, electronic stability control, and side impact beams. All other features (traction control, fog lamps, tire pressure monitoring, parking sensors, parking assist, and backup cameras) have been transferred to the other specifications category.
The second series of collected data refers to user opinions and news published on reputable websites ranked higher on Alexa 30 . Four websites were examined for this purpose: Autoblog 32 , Auto News 33 , Motor1 34 , and The Car Connection 35 . These websites were crawled using Python web crawlers to save time and collect information automatically. From 2014 to 2020, the daily news published was collected and evaluated for each type of vehicle. The Valence Aware Dictionary and sEntiment Reasoner (VADER) method was used for sentiment analysis of the text. Based on vocabulary analysis, the VADER sentiment analysis method correctly analyzes the sentiment expressed in social media and news texts. Ten independent human raters analyzed over 90,000 ratings in the VADER evaluation, which led to the adoption of 7500 linguistic features that were rated based on their valence scores, which indicate the intensity and polarity of sentiment 36 . For each vehicle, the average monthly score of news and opinions has been calculated based on their daily publication of them.
Another effective source of information about the vehicle market is various economic indicators. Using a Python web crawler, information on several economic indicators affecting the car market has been collected on the Federal Reserve website 37 . Economic indicators include GDP, Consumer Price Index (CPI), Producer Price Index, Consumer Confidence Index, Personal Income Per Capita, Interest Rates on 48-month and 60-month Loans, SP&500, and Dow Jones stock market indicators.
According to Kinski's research, using Google trends in prediction models is beneficial and practical 22 . Three keywords have been selected for Google trend data to evaluate the number of searches for each car from 2014 to 2020 and for the United States of America. The keywords are:
"Make" + "Model"
"Price" + "Make" + "Model"
"Dealer" + "Make"
All cars have the same data collected, and the features collected on a monthly basis for each car are listed in Table 2 . Several different trims were available on the market for some vehicles simultaneously, and some characteristics, such as price and MPG, had multiple values for these vehicles. Due to this, the collected values for these characteristics were divided into three categories: minimum, average, and maximum.
The sales feature has been normalized based on the maximum and minimum values from the training data set. Other features are standardized based on each feature's average and standard deviation in the training set. The input data to models are considered seven-month windows to maintain temporal correlation. For example, in the current month, the last seven months' data are input (X), and the current month's sale is output (Y). In order to achieve this, seven-month data matrices were placed consecutively in the third dimension of a three-dimensional tensor.
Validation and interpretation of results
Since the time series data in this study are monthly, eleven binary columns have been added to the dataset to reflect the effect of each month (in the first month of every year, the column corresponding to the first month is set to 1, and the column for the other months is set to 0). An example of this binary data is shown in Table 3 .
For most vehicles, data includes 79 months (January 2014 through July 2020). According to Fig. 5 , the last 14 months are selected for the testing set as rolling cross-validation. Using cross-validation on a rolling basis is one way to validate the time-series model. Starting with a subset of data for training, forecasting for later data points and then checking the accuracy of the forecasts. The same forecasted data points are included in the next training dataset, and further forecasts are made.
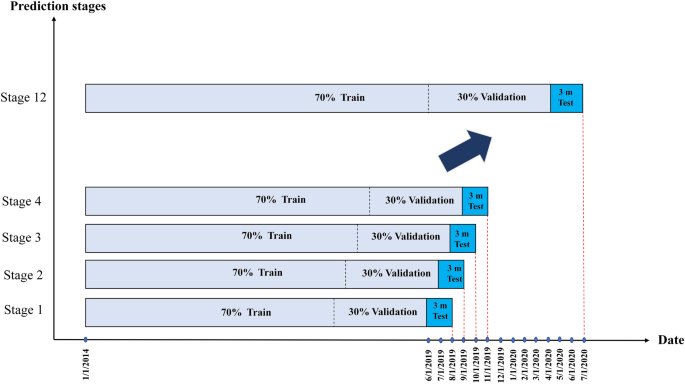
Splitting dataset into training, validation, and testing sets.
The model is cross-validated using 12 forecasting stages, with each stage predicting sales in the next three months. During each prediction stage, the preceding months are divided into training and validation (70% for training and 30% for validation. Then these data are transferred to the model, the model predicts sales in the next three months, then the forecast date is moved forward by one month, and this process has been repeated 12 times. Vehicle sales in the next three months are predicted each time the model runs, assuming most of the vehicle's characteristics remain the same. Due to fluctuation and changes in economic conditions, a three-month time horizon is used for predicting the future.
Overfitting is one of the principal problems in ANN training. The Dropout layers between the neural network layers are one of the best solutions in the ANN to avoid overfitting. During the dropout layer, the number of neurons trained in each layer and those discarded is determined randomly (rather than activating all neurons at once, only a fraction are activated) 38 . TensorFlow's early stopping tool is another basic solution to avoid overfitting. Early stopping works in the following way: during the repetition of training, the validation data is used to calculate the error value, and whenever the validation error value increases throughout several epochs, the model is ready to be stopped, and overfitting is prevented. For all three models, both solutions are used to prevent overfitting. Dimensionality reduction is another way to prevent model overfitting. In this study, Principal Component Analysis was used in several modes to reduce dimensions, but this technique was not used due to the significant decrease in model performance.
In order to improve the modeling process, all three models' hyperparameter values and network architectures were determined by Automated Machine Learning (AutoML). AutoML is the process of automating ML applications. The number of hidden layers, the number of neurons in these layers, and the dropout rate was determined by the Tuners. Several values are introduced to the Tuner for each hyperparameter. The Tuner trains different model versions and selects the best one based on the best result (lowest error or loss) on the validation data. This method sets the hyperparameters to the optimal value, and the model is then applied to a test dataset.
The model's error or loss is calculated using the Mean Absolute Error (MAE) loss function in all three models. Selecting a suitable optimization algorithm for the DL model is essential to reduce the run time and reach the desired result. Adam's optimization algorithm is used for these models, which is a generalized version of stochastic gradient descent. It reduces memory usage, converges faster, and corrects high variance and learning rates 39 .
Comparison of models
With the validation data, hyperparameters are adjusted, and the model is built to predict vehicle sales over the next three months (three months following the last validation date). The model run-time for all vehicles was very long due to the many vehicle types (357). In a random sample of 15 vehicles, different models' states were compared using fixed data, and the results were compared between the three models.
The sale of each vehicle is predicted in 12 stages; each prediction stage includes the prediction for the next three months, respectively, the first month of the prediction, the second month of the prediction, and the third month of the prediction. In total, the first predictions include 12 months, the second predictions include 12 months, and the third predictions include 12 months. Model performance was evaluated using the Mean Absolute Percentage Error (MAPE), the Root Mean Square Error normalized by the change range ( \(NRSME_{range}\) ), and the Root Mean Square Error normalized by the mean value ( \(NRSME_{mean}\) ) according to Eqs. 15 – 18 .
According to the above equations, \(y_{t}\) denotes the actual value at time t, \(\hat{y}_{t}\) denotes the predicted value at time t, \(y_{max}\) denotes the maximum actual value, \(y_{min}\) denotes the minimum actual value, \(y_{mean}\) denotes the average actual value, and T is equal to the total number of predicted samples. The average error values of all vehicles were calculated to compare the results of various models. A weighted average was calculated using the total number of sales of each car per month as a weight for the vehicle according to Eq. ( 19 ) since the numbers of vehicle sales are not on the same scale, and the error rate is more important in vehicles with high sales. A further method of checking the models' performance is to compare the R-square, slope, and intercept of the linear regressions fitted on predicted and observed data for all three models. Table 4 summarizes the evaluation results of the models.
In the proposed hybrid model, the error values are lower, the R-square accuracy is higher, the slope value is closer to 1, and the intercept is closer to 0. At this stage, the proposed hybrid model was recognized as preferable to both the LSTM and ConvLSTM models.
Implementation of the proposed hybrid model to predict the share of EVs
For all vehicles, the proposed hybrid model has been implemented, and 12 points of prediction have been used to determine the sale of all vehicles. Linear regression was fitted on the predicted sales and actual values to evaluate the model's performance, as shown in Table 5 .
Primary data segments vehicles by specifications according to segments like CAR-SMALL_COMPACT, CAR-MID_FULL SIZE, MINIVAN LARGE, and PICKUP LARGE. Each segment consists of similar vehicles in appearance and specifications that compete with one another. Segments that include EVs have been separated to determine the share of EVs. Based on actual and predicted sales, the shares of electric and gasoline vehicles have been compared and evaluated for each month of the test data. For example, the CAR-MID/FULL-SIZE segment includes 28 vehicles (23 gasoline vehicles and five EVs). Figure 6 shows the share of EVs in this segment based on twelve prediction stages (three months per stage), separately for the first, second, and third months of each prediction.
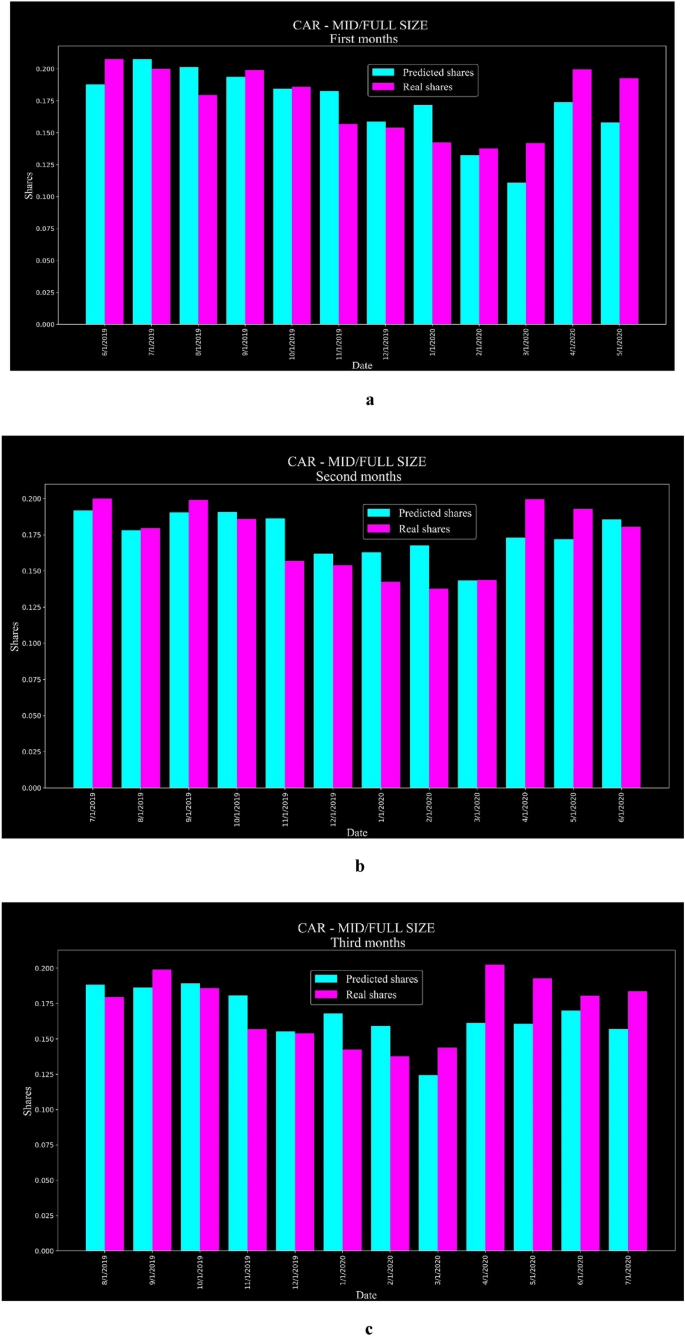
( a ) Share of EVs in CAR-MID/FULL-SIZE based on the first month of each prediction. ( b ). Share of EVs in CAR-MID/FULL-SIZE based on the second month of each prediction. ( c ) Share of EVs in CAR-MID/FULL-SIZE based on the third month of each prediction.
All segments' MAEs for EVs' share forecasting in the forecast's first, second, and third months are shown in Table 6 . The average MAE value of all segments was calculated as 3.2% for the first months, 3.8% for the second months, and 3.5% for the third months. The average value for all segments and all forecast months was calculated at about 3.5%, which shows that the proposed hybrid model performed well.
As part of the model analysis, the segments that included EVs were separated again and ranked by sales within each segment. The rankings were based on actual sales (actual rank) and predicted sales (predicted rank); the actual rank and predicted rank were used for evaluation. Kendall-Tau correlation (Kendall's correlation) is commonly used to check the concordance of two ranked lists; this technique was used to examine the actual and predicted rankings in this study. Kendall's correlation rate for two rating lists \(r_{a}\) and \(r_{b}\) ( \(\tau_{{r_{a} , r_{b} }}\) ) is represented by Eq. ( 20 ) 40 .
In Eq. ( 20 ), \(n_{c}\) represents the number of concordant pairs, \(n_{d}\) represents the number of discordant pairs, and n represents the total number of ranks in each of the rating lists 40 . The maximum number of discordant pairs between two ranking lists equals \(\frac{1}{2} n\left( {n - 1} \right)\) , and Kendall's correlation equals + 1 if all pairs of ranks are concordant and -1 if none are concordant 40 . For all segments, Kendall's correlation values were calculated separately for the first, second, and third prediction months, and the average values are shown in Table 7 . The average Kendall's correlation value of all segments was calculated as 0.76 for the first months, 0.742 for the second months, and 0.75 for the third months. The average Kendall's correlation value for all segments and all forecast months was calculated at about 0.75, which indicates the great performance of the proposed hybrid model in predicting the ranking.
Sensitivity analysis
Sensitivity analysis was performed to determine which features significantly impacted the trained model. Thus, for each vehicle, the pre-trained model that was evaluated in previous stages has once again predicted the number of vehicle sales with new input data, and its outputs have been assessed. All features, except the investigated feature, are valued at their average. For the investigated feature, the five values from the training data (the min value, the first quartile, the second quartile, the third quartile, and the max value) are taken into consideration. Five predictions were made based on these five values, and a range of changes in predicted sales was calculated. The change ranges for all features have been measured, and the four features with the most extensive range have been identified. As an example, during the sensitivity analysis of the BMW I3 for 2020, the following four features had the broadest range of changes: the Consumer Price Index (CPI), the equivalent MPG for EVs, the Google search score for car prices (Google Trends), and the car price. This EV's sensitivity analysis plots are shown in Fig. 7 .
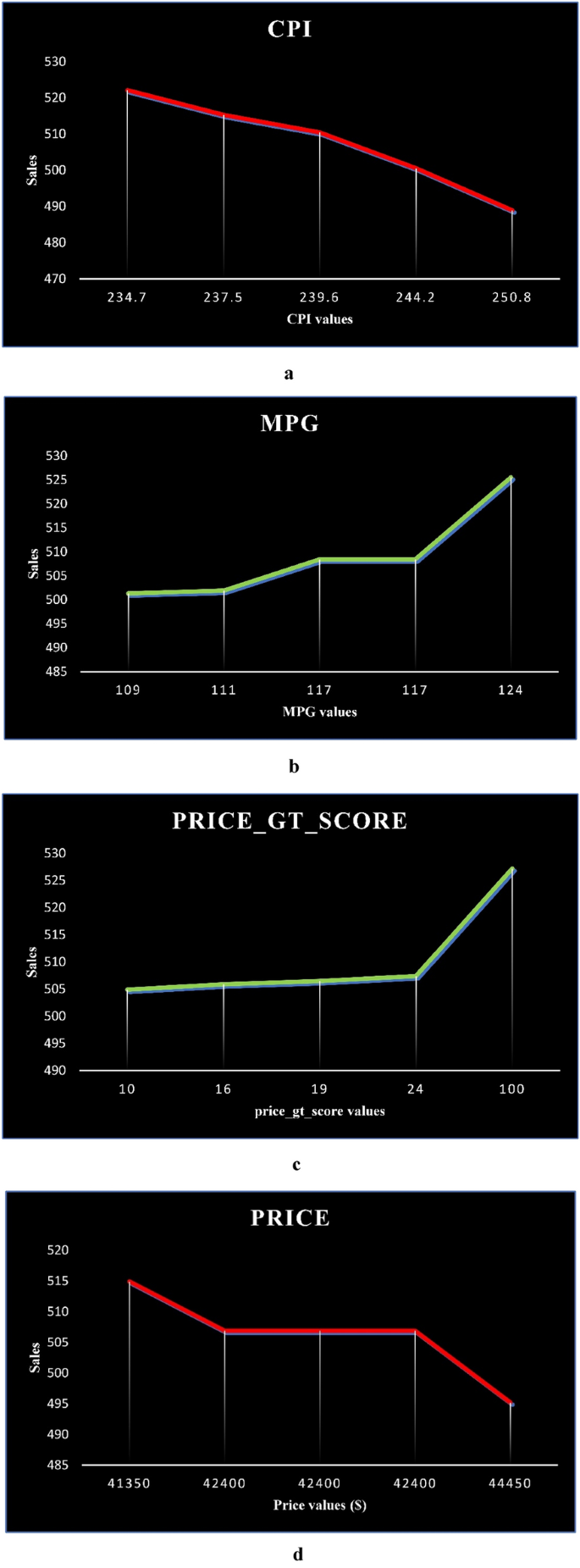
( a ) Sensitivity analysis plot of influential feature 1 for BMW I3. ( b ) Sensitivity analysis plot of influential feature 2 for BMW I3. ( c ) Sensitivity analysis plot of influential feature 3 for BMW I3. ( d ) Sensitivity analysis plot of influential feature 4 for BMW I3.
Based on Eq. ( 21 ), slope values for the four characteristics with the most extensive range of changes are calculated in different parts of the graph, and the results are summarized in Table 8 . For example, the number of sales of this EV has decreased by 8 for every thousand-dollar increase in price when the price is in the range of the minimum value to the first quarter. As the slope is zero percent in the second and third parts of the graph, the price in the first, second, and third quartiles is equal, and when the price is in the third quartile to the maximum price, the number of sales for this EV decrease by 6 for every thousand-dollar increase in price.
There has been a decrease in car sales due to the increase in the CPI. It is also true that with the increase in the CPI, the final price of the car and the price of auto parts have increased, which has led to a decrease in the desire to buy this car. The second feature is equivalent MPG for EVs, a higher equivalent MPG indicating better performance and less fuel consumption in a fixed distance has led to an increase in sales of this car. The third feature identified is the increase in the car price search score on Google (Google Trend), an indicator that buyers are more curious about this car, contributing to its sales. The fourth specified feature of the car is its price, and its sales have decreased with the increase in its price. As a result of the sensitivity analysis, the manufacturers of this car could use policies such as lowering the price of the car and its parts (CPI and car price), improving the performance of the vehicle's engine (the equivalent MPG), and developing advertisements and introducing the car to the public (Google trend score) to increase sales.
Sensitivity analysis has been conducted for each EV, and the results show different sensitivity for each vehicle. From each segment that includes EVs, one vehicle was selected as a sample, and the results of its sensitivity analysis are shown in Table 9 .
Each EV's sensitivity analysis identifies features that differ from the others, as shown in Table 9 . According to the results of the sensitivity analysis, ten features that were most frequently found in the sensitivity analysis of all the EVs were identified as the most influential features: Shoppers, Min price, CPI, Sales, Google Trends score 3 (Price), Make & model news score, Personal income per capita, Make news score, Interest Rates on 60-month, and Mean options score, respectively.
This study addresses an important topic from a business perspective. Car manufacturers can benefit from this research by understanding their market share and the effect of pricing and vehicle specification on the market share. They can use the results of this study to analyze both their EV market as well as their Non-EV market. Lower down the funnel, car dealers that operate in a highly competitive environment can strategize their sales events, marketing campaigns, and discounts to meet their business goals and target sales. Finally, the model enables the public sector to understand the effect of tax policies on the share of EV vehicles in case they like to promote them.
This study used ML methods to develop a prediction model that estimated the sale of all cars in the dataset, the share of EVs in each segment and identified the main factors affecting each EV's sales. In this research, several web crawlers have been used to collect various data, including factors that previous studies have proven to be associated with EV sales. Vehicles sale were predicted using LSTM, ConvLSTM, and the proposed hybrid model (Hybrid LSTM with two-dimensional Attention and Residual network). Several ML tools have been used to improve the model's training and the modeling process, such as transforming two-dimensional time series data into three-dimensional tensors, Dropout layers, early stopping tools, and AutoML. Because of the variety of car types and the long running time of the models, a random selection of fifteen types of cars was made. All three models are evaluated based on the same evaluation units: the MAPE, NRSME_range, and NRSME_mean, R-square, slope, and intercept of fitted linear regressions have also been assessed. The average error values in the three months of prediction were as follows:
The MAPE value of the proposed hybrid model was 4.5% less than the LSTM model and 14.4% less than the ConvLSTM model.
The NRSME_range value of the hybrid model was 0.11 less than the LSTM model and 0.22 less than the ConvLSTM model.
The NRSME_mean value of the hybrid model was 0.079 less than the LSTM model and 0.169 less than the ConvLSTM model.
As a result of fitting linear regressions to the predicted and actual values, for all three months of predictions, the proposed hybrid model has a higher R-square value, its slope is closer to one, and its intercept is closer to zero, which indicates that the hybrid model performed better than the other two. In comparing the models, it was found that the proposed hybrid model conducted better than other models and was selected to predict the sale of all vehicles in the dataset. Based on the linear regression fitted to the predicted sales and the actual sales of all vehicles, the R-square values for the first, second and third prediction months were 0.912, 0.906, and 0.917.
The predicted sales of all vehicles were used to calculate the predicted share of EVs in each segment and compare them with the actual values. Across all segments and forecasting months, the average MAE value for EV share is about 3.5%, and the hybrid model has accurately predicted the share of EVs across all segments. To further analyze the model results, the cars were ranked according to the number of actual and predicted sales within each segment. The average Kendall's correlation value for all segments and all forecast months was calculated at about 0.75, which indicates the high performance of the proposed hybrid model in predicting the ranking.
The sensitivity analysis was performed to evaluate the model further and identify its most influential features. The results have shown that each EV's sensitivity analysis identifies features that differ from the others. According to the sensitivity analysis of the BMW I3 for 2020, the following four features were most affected: the Consumer Price Index, the equivalent MPG for EVs, the Google search score, and the car price. As a result of the sensitivity analysis, the manufacturers of this car could use policies such as lowering the price of the car and its parts, improving the engine's performance, developing advertisements, and better introducing the car to increase sales (See Appendix Tables A1 to A4.2 , Fig. A1 ).
This research has achieved the following accomplishments:
A wide variety of factors have been collected and used as variables to model the sale of EVs.
LSTM and ConvLSTM, powerful DL models, have been used for predicting vehicle sales. By combining the two-dimensional Attention model and the Residual network, the performance of the LSTM model was enhanced, and the innovative hybrid model performed better than the other two.
EVs differ in terms of the most influential factors for sales depending on the sensitivity analysis results. The ten features that appeared the most in the sensitivity analysis of all EVs were identified as the most influential, including Shoppers, Min price, CPI, Sales, Google Trends score 3 (Price), News score for make and model, Personal income per capita, News score for make, Interest Rates on 60-month, and Mean options score, respectively.
Data availability
The primary dataset was taken from Autometrics, and other data were collected using web crawlers. The data is available from the corresponding author on reasonable request.
https://www.epa.gov/ghgemissions/inventory-us-greenhouse-gas-emissions-and-sinks (2020).
MacInnis, B. & Krosnick, J. Climate Insights 2020: Electric Vehicles. (2020).
https://theicct.org/the-rise-of-electric-vehicles-the-second-million/ (2020).
https://www.iea.org/fuels-and-technologies/electric-vehicles (2022).
Brühl, B., Hülsmann, M., Borscheid, D., Friedrich, C. M. & Reith, D. in Industrial Conference on Data Mining. 146–160 (Springer).
Wang, F.-K., Chang, K.-K. & Tzeng, C.-W. Using adaptive network-based fuzzy inference system to forecast automobile sales. Expert Syst. Appl. 38 , 10587–10593 (2011).
Article Google Scholar
Hülsmann, M., Borscheid, D., Friedrich, C. M. & Reith, D. General sales forecast models for automobile markets and their analysis. Trans. Mach. Learn. Data Min. 5 , 65–86 (2012).
Google Scholar
Kitapcı, O., Özekicioğlu, H., Kaynar, O. & Taştan, S. The effect of economic policies applied in Turkey to the sale of automobiles: Multiple regression and neural network analysis. Procedia Soc. Behav. Sci. 148 , 653–661 (2014).
Bas, J., Zou, Z. & Cirillo, C. An interpretable machine learning approach to understanding the impacts of attitudinal and ridesourcing factors on electric vehicle adoption. Transp. Lett. 15 , 30–41 (2023).
Zhang, Y., Zhong, M., Geng, N. & Jiang, Y. Forecasting electric vehicles sales with univariate and multivariate time series models: The case of China. PLoS ONE 12 , e0176729 (2017).
Article PubMed PubMed Central Google Scholar
Kaya, S. K. & Yildirim, Ö. A prediction model for automobile sales in turkey using deep neural networks. Endüstri Mühendisliği 31 , 57–74 (2020).
Xia, Z. et al. ForeXGBoost: Passenger car sales prediction based on XGBoost. Distrib. Parallel Databases 38 , 713–738 (2020).
Bas, J., Cirillo, C. & Cherchi, E. Classification of potential electric vehicle purchasers: A machine learning approach. Technol. Forecast. Soc. Chang. 168 , 120759 (2021).
Saxena, P., Bahad, P. & Kamal, R. Long short-term memory-RNN based model for multivariate car sales forecasting. Int. J. Adv. Sci. Technol. 29 , 4645–4656 (2020).
Beggs, S., Cardell, S. & Hausman, J. Assessing the potential demand for electric cars. J. Econom. 17 , 1–19 (1981).
Calfee, J. E. Estimating the demand for electric automobiles using fully disaggregated probabilistic choice analysis. Transp. Res. Part B Methodol. 19 , 287–301 (1985).
Mau, P., Eyzaguirre, J., Jaccard, M., Collins-Dodd, C. & Tiedemann, K. The ‘neighbor effect’: Simulating dynamics in consumer preferences for new vehicle technologies. Ecol. Econ. 68 , 504–516 (2008).
Balducci, P. J. Plug-In Hybrid Electric Vehicle Penetration Scenarios. (Pacific Northwest National Lab. (PNNL), Richland, WA (United States) (2008).
Hess, S., Fowler, M., Adler, T. & Bahreinian, A. A joint model for vehicle type and fuel type choice: Evidence from a cross-nested logit study. Transportation 39 , 593–625 (2012).
Bas, J., Zofío, J. L., Cirillo, C., Chen, H. & Rakha, H. A. Policy and industry implications of the potential market penetration of electric vehicles with eco-cooperative adaptive cruise control. Transp. Res. Part A Policy Pract. 164 , 242–256 (2022).
Shafiei, E. et al. An agent-based modeling approach to predict the evolution of market share of electric vehicles: A case study from Iceland. Technol. Forecast. Soc. Chang. 79 , 1638–1653 (2012).
Kinski, A. Google trends as complementary tool for new car sales forecasting: A cross-country comparison along the customer journey , University of Twente, (2016).
https://en.wikipedia.org/wiki/Artificial_intelligence (2023).
https://en.wikipedia.org/wiki/Recurrent_neural_network (2023).
Hochreiter, S. & Schmidhuber, J. Long short-term memory. Neural Comput. 9 , 1735–1780 (1997).
Article CAS PubMed Google Scholar
Basodi, S., Ji, C., Zhang, H. & Pan, Y. Gradient amplification: An efficient way to train deep neural networks. Big Data Min. Anal. 3 , 196–207 (2020).
Wei, X., Zhang, L., Yang, H.-Q., Zhang, L. & Yao, Y.-P. Machine learning for pore-water pressure time-series prediction: Application of recurrent neural networks. Geosci. Front. 12 , 453–467 (2021).
Article ADS Google Scholar
Shi, X. et al. Convolutional LSTM network: A machine learning approach for precipitation nowcasting. Advances in neural information processing systems 28 (2015).
Bahdanau, D., Cho, K. & Bengio, Y. Neural machine translation by jointly learning to align and translate. arXiv preprint arXiv:1409.0473 (2014).
https://www.alexa.com/ (2021).
https://www.thecarconnection.com/ (2021).
https://www.autoblog.com/news/ (2021).
https://www.autonews.com/news (2021).
https://www.motor1.com/news/ (2021).
https://www.thecarconnection.com/news (2021).
Hutto, C. & Gilbert, E. in Proceedings of the international AAAI conference on web and social media. 216–225.
https://fred.stlouisfed.org/ (2021).
Baldi, P. & Sadowski, P. J. Understanding dropout. Advances in neural information processing systems 26 (2013).
Kingma, D. P. & Ba, J. Adam. A method for stochastic optimization. arXiv preprint arXiv:1412.6980 (2014).
Bachrach, Y., Herbrich, R. & Porat, E. in International Symposium on String Processing and Information Retrieval. 344–352 (Springer).
Download references
The authors received no financial support for the research, authorship, and/or publication of this article.
Author information
Authors and affiliations.
Department of Transportation, School of Civil Engineering, Iran University of Science and Technology, Tehran, Iran
Shahriar Afandizadeh & Diyako Sharifi
AECOM, Glen Allen, VA, USA
Navid Kalantari
Department of Civil-Transportation Planning, Faculty of Technical and Engineering, Imam Khomeini International University, Qazvin, Iran
Hamid Mirzahossein
You can also search for this author in PubMed Google Scholar
Contributions
The authors confirm contribution to the paper as follows: study conception and design: S.A., D.S., N.K., H.M.; data collection: N.K., D.S.; analysis and interpretation of results: S.A., D.S., N.K.; manuscript preparation: D.S., H.M. All authors reviewed the results and approved the final version of the manuscript. Authors consent for the publication of the submitted paper and any associated data and accompanying images
Corresponding author
Correspondence to Shahriar Afandizadeh .
Ethics declarations
Competing interests.
The authors declare no competing interests.
Additional information
Publisher's note.
Springer Nature remains neutral with regard to jurisdictional claims in published maps and institutional affiliations.
Supplementary Information
Supplementary information., rights and permissions.
Open Access This article is licensed under a Creative Commons Attribution 4.0 International License, which permits use, sharing, adaptation, distribution and reproduction in any medium or format, as long as you give appropriate credit to the original author(s) and the source, provide a link to the Creative Commons licence, and indicate if changes were made. The images or other third party material in this article are included in the article's Creative Commons licence, unless indicated otherwise in a credit line to the material. If material is not included in the article's Creative Commons licence and your intended use is not permitted by statutory regulation or exceeds the permitted use, you will need to obtain permission directly from the copyright holder. To view a copy of this licence, visit http://creativecommons.org/licenses/by/4.0/ .
Reprints and permissions
About this article
Cite this article.
Afandizadeh, S., Sharifi, D., Kalantari, N. et al. Using machine learning methods to predict electric vehicles penetration in the automotive market. Sci Rep 13 , 8345 (2023). https://doi.org/10.1038/s41598-023-35366-3
Download citation
Received : 13 November 2022
Accepted : 17 May 2023
Published : 23 May 2023
DOI : https://doi.org/10.1038/s41598-023-35366-3
Share this article
Anyone you share the following link with will be able to read this content:
Sorry, a shareable link is not currently available for this article.
Provided by the Springer Nature SharedIt content-sharing initiative
This article is cited by
Optimal charge scheduling and on-board control of an urban electrified brt fleet considering synthetic representative driving cycles.
- Ahmed F. Ayad
- Mostafa Asfoor
Scientific Reports (2024)
By submitting a comment you agree to abide by our Terms and Community Guidelines . If you find something abusive or that does not comply with our terms or guidelines please flag it as inappropriate.
Quick links
- Explore articles by subject
- Guide to authors
- Editorial policies
Sign up for the Nature Briefing: Anthropocene newsletter — what matters in anthropocene research, free to your inbox weekly.

Spotlight on mobility trends
In a decade that has witnessed remarkable mobility developments, 2023 still manages to stand out. The long list of accomplishments includes some familiar topics, such as the continued growth of micromobility, steady progress with vehicle electrification, and continued investment in autonomous vehicles (AVs). Mobility stakeholders also ventured deeper into relatively new technologies and began seriously exploring the potential of generative AI to transform everything from automotive marketing and sales to R&D.
Despite mobility’s steady progress in 2023, the challenges facing the industry tended to overshadow the good news. Electric-vehicle (EV) sales slowed in some regions, technological issues and lack of public acceptance derailed some AV pilots, and concerns about battery range persisted. What’s more, 2023 provided more evidence that the global automotive market is in the midst of major disruptions. The industry’s future is clearly becoming more electric despite some regional sales dips, and Chinese EV OEMs, which hold a strong position in their home market, are now preparing to pursue global opportunities. Meanwhile, OEMs in China, Europe, and the United States continue to roll out increasingly innovative AVs, including some with advanced L3 driving technology.
Here’s a closer look at the trends that defined 2023 and will continue to shape mobility in 2024.
Changing consumer preferences
A consumer shift from private vehicles to more sustainable options.
Mobility is first and foremost about consumer choices, and the McKinsey Mobility Consumer Pulse Survey shows that preferences are indeed shifting. 1 The McKinsey Consumer Pulse Survey, conducted in December 2022, included 27,869 respondents from Brazil, China, France, Italy, Japan, Norway, South Africa, and the United States. While private cars still dominate the road, 40 percent of survey respondents now use multiple mobility modes, including eco-friendly options such as e-bikes, and 62 percent are beginning to change their transportation habits because of sustainability concerns (Exhibit 1). In a development that could affect vehicle sales, 20 percent of respondents say they would consider replacing their private vehicles with other mobility options over the next ten years, and many are open to using autonomous shuttles if they become available.
New vehicle preferences for everything from electrification to connectivity
EVs have been soaring in popularity for years and remain in high demand, despite some recent sales declines in certain regions. The McKinsey Consumer Pulse Mobility Survey provides more evidence for this trend, with 42 percent of respondents stating that they want their next car to be an EV (Exhibit 2). To be willing to switch from an internal combustion engine (ICE) vehicle to an EV, respondents say their vehicles should be able to travel an average of 437 kilometers on a full charge. In another shift, 47 percent of prospective EV buyers surveyed say they are willing to purchase their vehicles online, showing that the sales process is also evolving.
Both digital connectivity and assistance features, such as automatic braking, are increasingly important purchase considerations. For their next vehicle purchase, 69 percent of survey respondents want more car connectivity services and 34 percent want L4 automation features.
The world goes electric
Growing ev demand—with china as the hot spot.
McKinsey projects that worldwide demand for passenger cars in the battery electric vehicle (BEV) segment will grow sixfold from 2021 through 2030, with annual unit sales increasing to roughly 40.0 million, from 6.5 million, over that period. China retained its position as the world’s e-mobility hot spot in 2023, with the highest penetration rates (Exhibit 3): one out of every four cars in China in 2023 was a BEV.
BEV growth remained positive across major regions, but it was slower than in past years for several reasons, including lower consumer demand stemming from a more pessimistic macroeconomic outlook and a lack of new attractive BEV model launches globally. In Europe, BEV cash subsidies declined or ended, which further dampened demand. Plug-in hybrid electric vehicle (PHEV) sales grew in all regions except Europe.
China also hosts many local EV companies that are seeing strong domestic sales and have ambitious plans to export their vehicles. One essential factor to the country’s success: an integrated value chain that provides local companies with easy access to everything from raw materials to batteries to digital services.
Would you like to learn more about the McKinsey Center for Future Mobility ?
Chinese ev oems—high market share, lower prices.
Beyond the quality of their EVs, Chinese OEMs may have an advantage in the global market for one simple reason: their vehicles are less expensive than those from European OEMs across all vehicle segments (Exhibit 4). In fact, one leading Chinese OEM now offers its vehicles at prices that are lower than or on par with those for comparable ICE vehicles.
China’s integrated value chain contributes to the low prices for domestic OEMs. Other countries may want to consider developing similar value chains as the transition to EVs continues, since lower prices may accelerate the shift.
A growing need for batteries
The McKinsey Battery Insights team projects that the entire lithium-ion (Li-ion) battery chain, from mining through recycling, could grow by 27 percent annually from 2022 to 2030 , when it would reach a value of more than $400 billion and a market size of about 4,700 gigawatt-hours (GWh) (Exhibit 5). 2 “ Battery 2030: Resilient, sustainable, and circular ,” McKinsey, January 16, 2023. Batteries for mobility applications , such as EVs, will account for the vast bulk of demand in 2030—about 4,300 GWh, McKinsey estimates. The next two sources of demand—stationary storage and consumer electronics—will account for a much lower percentage of required GWh.
A new go-to-market model for the EV age
EV buyers value innovation, and that preference extends beyond the car to the buying process itself. McKinsey’s latest Future of Auto Retail Consumer Survey reveals that respondents who are inclined to purchase an EV are more likely to say that they want more personalization than traditional car buyers (Exhibit 6). 3 Future of Auto Retail Consumer Survey, McKinsey, August 2022. Consumers were interviewed in China, Germany, the United Kingdom, and the United States (n = 4,708). They want a smaller set of preconfigured options, for instance, and the opportunity to change configuration after purchase and before delivery. Potential EV buyers also want more simplicity, convenience, and price transparency, including the ability to prebook test drives and secure financing online. OEMs that want to create an innovative and desirable brand impression could consider heeding these preferences as electrification accelerates.
Autonomous driving
A few bumps in the road but continued progress for autonomous driving.
OEMs and tech companies alike made important advances in autonomous driving in 2023, but these were balanced by some setbacks. One tech company, for example, successfully piloted robotaxis in the United States, but another was forced to discontinue its pilot after several accidents. We now estimate that adoption of vehicles with some L4 capabilities will occur at scale around 2026, with the first applications likely involving autonomous parking, followed by highway driving (Exhibit 7). More complex applications, such as driving in an urban environment, will require longer timelines for at-scale deployment. Reflecting the technological complexities of autonomous driving, the anticipated timelines for the emergence of L4 capabilities are now longer than they were in 2021.
Mobility investment
A complex investment picture—with some signs for optimism.
Innovation does not happen overnight after an inventor has a eureka moment. Within mobility, think of the complications involved in reconfiguring the automotive value chain to support EV production or the intense testing and reworking required to build the software stack for autonomous driving. These efforts require major funding from external investors, and the early part of 2023 showed signs that they were pulling back. Funding was down by 44 percent in the first quarter of 2023, compared with the same period last year, and 13 percent lower in the second quarter (Exhibit 8). In late 2023, mobility investment showed more positive momentum, and we expect this to continue in 2024. Companies that specialize in electrification, especially batteries, are attracting the most investment, followed by those that focus on AVs.
Private-market funding and corporate investment efforts for mobility initiatives continue to be significantly more selective; however, some strongly sought-after technology clusters like EVs still show momentum.

What technology trends are shaping the mobility sector?
New types of mobility, micromobility options grew, suggesting big changes are ahead for the private-vehicle market.
Micromobility—e-scooters, bikes , mopeds—is here to stay. In the recent McKinsey ACES Consumer Survey, almost one-third of respondents say they plan to increase their use of micromobility and nearly half are planning to replace their private vehicles with other modes of transport. McKinsey estimates that the global micromobility market will reach about $360 billion by 2030, up from about $175 billion in 2022—mainly driven by e-bike sales (Exhibit 9). Europe will represent the highest share of that value.
Future air mobility made progress, but funding slowed
The future air mobility sector had a mixed year in 2023. Disclosed investment totaled $4.5 billion for the year. Although this was lower than the $4.9 billion raised in 2022, the number of deals more than doubled to 151. New aircraft orders totaled $22 billion , and the backlog of open aircraft orders, including options and letters of intent, increased to 21,300 (Exhibit 10). 4 Future Air Mobility Blog , “ Clouds or clear skies? Prospects for future air mobility ,” blog entry by Axel Esqué, Tore Johnston, and Robin Riedel, McKinsey, January 23, 2024.
At the seven leading companies specializing in electric vertical takeoff and landing (eVTOL) vehicles, the number of cumulative disclosed test flight hours now totals over 8,000. The future air mobility sector also saw an increase in the number of deals or relationships along the supply chain in 2023, as well as more commercial-drone flights and new regulatory approvals for drone flights beyond the visual line of sight.
Artificial intelligence
Applied ai still has a lead over generative ai within mobility.
Mobility actors are still heavily investing in both new and existing technologies. A McKinsey analysis of 3,500 mobility start-ups that specialize in digitization or the so-called ACES trends—autonomous driving, connectivity, electrification, and shared mobility —shows that they are more likely to invest in applied AI applications compared with other leading-edge solutions (Exhibit 11). 5 This 2023 analysis involved reviewing keywords in corporate public filings to determine their technology focus. Applied AI has a firm lead over generative AI within mobility because it enhances so many processes and addresses long-standing pain points, including those related to engineering, R&D, procurement, manufacturing, marketing and sales, and life cycle services. Companies, for instance, can use AI to identify customers who are at risk of being lost to a competitor and then create incentives to increase their satisfaction, potentially reducing churn and decreasing costs. Since many mobility stakeholders just began seriously exploring generative AI in 2023, it might soon gain more traction.
About the McKinsey Center for Future Mobility
In retrospect, 2023 was a year of “getting things done” in mobility. Despite some setbacks, mobility stakeholders kept moving toward some of the industry’s most important goals, including increasing battery life, developing more sophisticated EVs, overcoming the hurdles to L3 and L4 automation, and continuing the transformation to a less car-centric world. While external funding was down in the first quarter, it began trending upward later in the year, and select areas still generate high interest from investors. That bodes well for the entire sector, including niche areas such as future air mobility. As we move into 2024, the mobility sector is likely to experience both consolidation and scaling. As always, stakeholders will pursue innovation, but the increased attention to profitability will also persist. The resilience and persistence that they demonstrated in 2023 should also serve them well as the new year opens.

Explore a career with us
Related articles.

The micromobility city: Measuring the impact of greater bicycle use
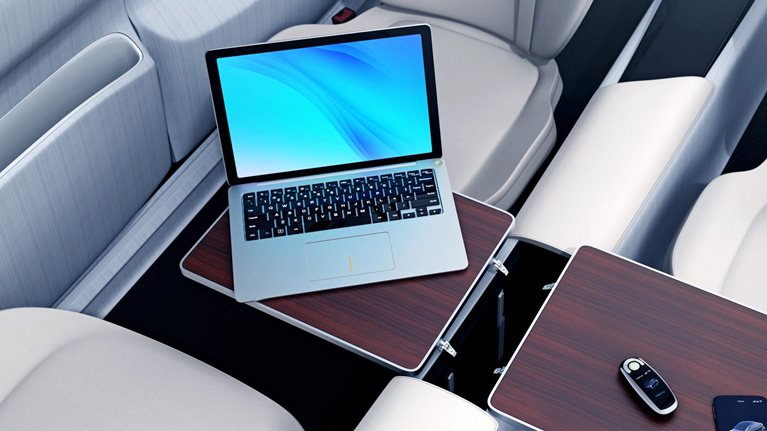
Autonomous driving’s future: Convenient and connected

Battery 2030: Resilient, sustainable, and circular
MIT Technology Review
- Newsletters
The inevitable EV: 10 Breakthrough Technologies 2023
Electric vehicles have been available for decades. Now they’ve finally become mainstream.
- James Temple archive page
BYD, Hyundai, Tesla, Volkswagen
Electric vehicles are transforming the auto industry.
While sales have slowly ticked up for years, they’re now soaring. The emissions-free cars and trucks will likely account for 13% of all new auto sales globally in 2022, up from 4% just two years earlier, according to the International Energy Agency. They’re on track to make up about 30% of those sales by the end of this decade.
A mix of forces has propelled the vehicles from a niche choice to a mainstream option.
Governments have enacted policies compelling automakers to retool and incentivizing consumers to make the switch. Notably, California and New York will require all new cars, trucks, and SUVs to be zero-emissions by 2035, and the EU had nearly finalized a similar rule at press time.
Auto companies, in turn, are setting up supply chains, building manufacturing capacity, and releasing more models with better performance, across price points and product types.
The Hongguang Mini, a tiny car that starts a little below $5,000, has become the best-selling electric vehicle in the world, reinforcing China’s dominance as the largest manufacturer of EVs.
A growing line-up of two- and three-wheelers from Hero Electric, Ather, and other companies helped EV sales triple in India over the last year (though the total number is still only around 430,000). And models ranging in size and price from the Chevy Bolt to the Ford F-150 Lightning are bringing more Americans into the electric fold.
There are still big challenges ahead. Most of the vehicles must become cheaper. Charging options need to be more convenient. Clean electricity generation will have to increase dramatically to accommodate the surge in vehicle charging. And it will be a massive undertaking to make enough batteries. But it’s now clear that the heyday of the gas-guzzler is dimming.
Climate change and energy
This classic game is taking on climate change.
What the New Energies edition of Catan says about climate technology today.
- Casey Crownhart archive page
How thermal batteries are heating up energy storage
The systems, which can store clean energy as heat, were chosen by readers as the 11th Breakthrough Technology of 2024.
How to stop a state from sinking
Louisiana’s southwestern coastline faces some of the most severe climate predictions in the US. Can a government-led project build the area up and out of crisis?
- Xander Peters archive page
Why new proposals to restrict geoengineering are misguided
We need more research, including outdoor experiments, to make better-informed decisions about such climate interventions.
- Daniele Visioni archive page
Stay connected
Get the latest updates from mit technology review.
Discover special offers, top stories, upcoming events, and more.
Thank you for submitting your email!
It looks like something went wrong.
We’re having trouble saving your preferences. Try refreshing this page and updating them one more time. If you continue to get this message, reach out to us at [email protected] with a list of newsletters you’d like to receive.

Featured Topics
Featured series.
A series of random questions answered by Harvard experts.
Explore the Gazette
Read the latest.

Bringing back a long extinct bird
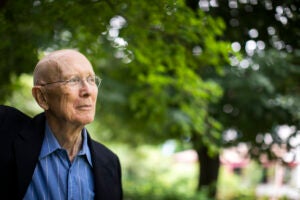
‘The scientist is not in the business of following instructions.’
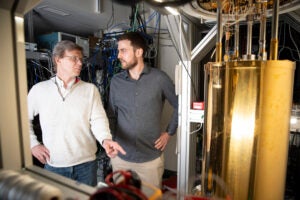
Glimpse of next-generation internet
Battery breakthrough for electric cars.
Leah Burrows
SEAS Communications
Harvard researchers design long-lasting, stable, solid-state lithium battery to fix 40-year problem
Long-lasting, quick-charging batteries are essential to the expansion of the electric vehicle market, but today’s lithium-ion batteries fall short of what’s needed — they’re too heavy, too expensive and take too long to charge.
For decades, researchers have tried to harness the potential of solid-state, lithium-metal batteries, which hold substantially more energy in the same volume and charge in a fraction of the time compared to traditional lithium-ion batteries.
“A lithium-metal battery is considered the holy grail for battery chemistry because of its high capacity and energy density,” said Xin Li, associate professor of materials science at the Harvard John A. Paulson School of Engineering and Applied Science (SEAS). “But the stability of these batteries has always been poor.”
Now, Li and his team have designed a stable, lithium-metal, solid-state battery that can be charged and discharged at least 10,000 times — far more cycles than have been previously demonstrated — at a high current density. The researchers paired the new design with a commercial high energy density cathode material.
This battery technology could increase the lifetime of electric vehicles to that of the gasoline cars — 10 to 15 years — without the need to replace the battery. With its high current density, the battery could pave the way for electric vehicles that can fully charge within 10 to 20 minutes.
The research is published in Nature.
“Our research shows that the solid-state battery could be fundamentally different from the commercial liquid electrolyte lithium-ion battery,” said Li. “By studying their fundamental thermodynamics, we can unlock superior performance and harness their abundant opportunities.”
The big challenge with lithium-metal batteries has always been chemistry. Lithium batteries move lithium ions from the cathode to the anode during charging. When the anode is made of lithium metal, needle-like structures called dendrites form on the surface. These structures grow like roots into the electrolyte and pierce the barrier separating the anode and cathode, causing the battery to short or even catch fire.
To overcome this challenge, Li and his team designed a multilayer battery that sandwiches different materials of varying stabilities between the anode and cathode. This multilayer, multimaterial battery prevents the penetration of lithium dendrites not by stopping them altogether but rather by controlling and containing them.
Think of the battery like a BLT sandwich. First comes the bread — the lithium metal anode — followed by lettuce — a coating of graphite. Next, a layer of tomatoes — the first electrolyte — and a layer of bacon — the second electrolyte. Finish it off with another layer of tomatoes and the last piece of bread — the cathode.
The first electrolyte (chemical name Li 5.5 PS 4.5 Cl 1.5 or LPSCI) is more stable with lithium but prone to dendrite penetration. The second electrolyte, (Li 10 Ge 1 P 2 S 12 or LGPS) is less stable with lithium but appears immune to dendrites. In this design, dendrites are allowed to grow through the graphite and first electrolyte but are stopped when they reach the second. In other words, the dendrites grow through the lettuce and tomato but stop at the bacon. The bacon barrier stops the dendrites from pushing through and shorting the battery.
“Our strategy of incorporating instability in order to stabilize the battery feels counterintuitive but just like an anchor can guide and control a screw going into a wall, so too can our multilayer design guide and control the growth of dendrites,” said Luhan Ye, co-author of the paper and graduate student at SEAS.
“The difference is that our anchor quickly becomes too tight for the dendrite to drill through, so the dendrite growth is stopped,” Li added.
The battery is also self-healing; its chemistry allows it to backfill holes created by the dendrites.
“This proof-of-concept design shows that lithium-metal solid-state batteries could be competitive with commercial lithium-ion batteries,” said Li. “And the flexibility and versatility of our multilayer design makes it potentially compatible with mass production procedures in the battery industry. Scaling it up to the commercial battery won’t be easy and there are still some practical challenges, but we believe they will be overcome.”
Harvard’s Office of Technology Development has protected a portfolio of intellectual property relating to this project, which is being advanced toward commercial applications with support from Harvard’s Physical Sciences and Engineering Accelerator and the Harvard Climate Change Solutions Fund .
Share this article
You might like.
Scientists sequence complete genome of bush moa, offering insights into its natural history, possible clues to evolution of flightless birds
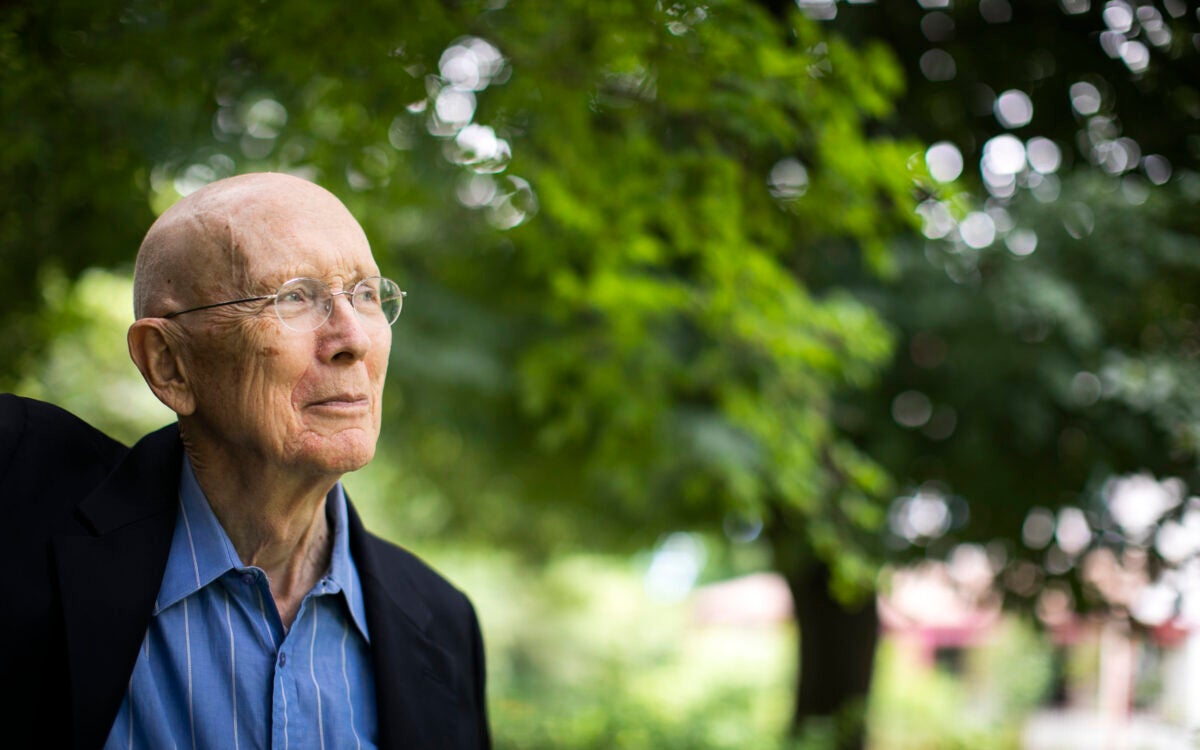
George Whitesides became a giant of chemistry by keeping it simple
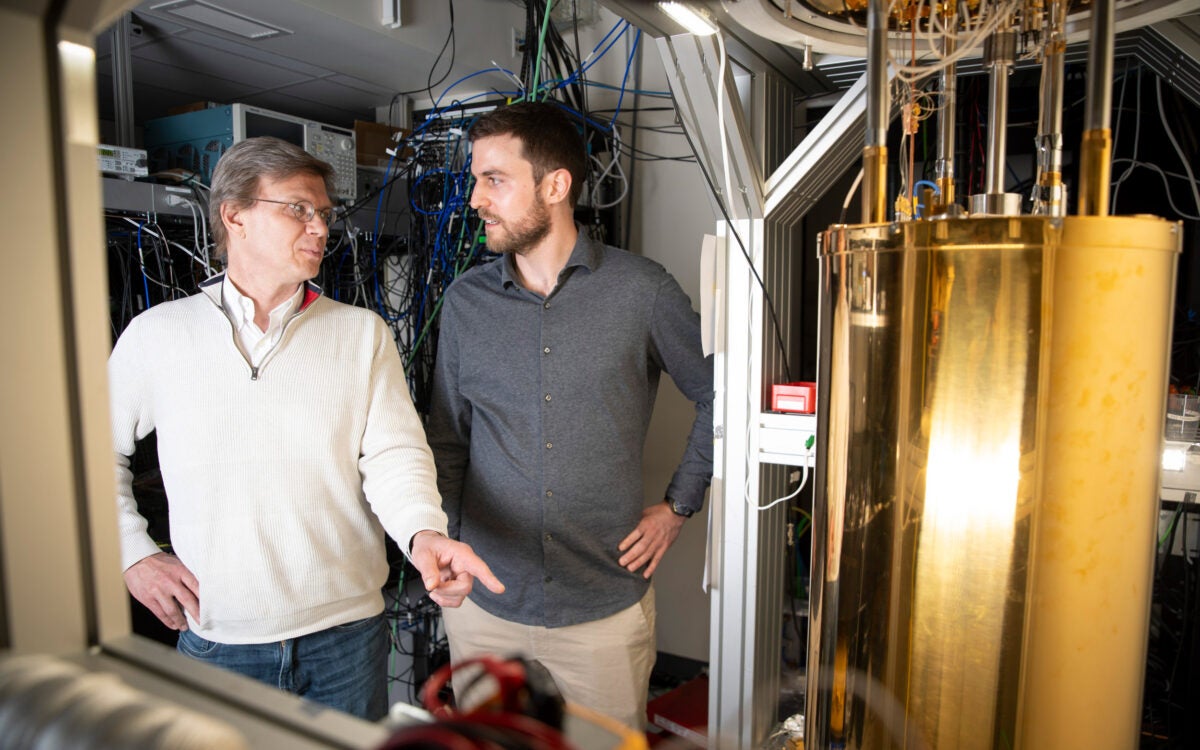
Physicists demo first metro-area quantum computer network in Boston
Women who follow Mediterranean diet live longer
Large study shows benefits against cancer, cardiovascular mortality, also identifies likely biological drivers of better health
Harvard-led study IDs statin that may block pathway to some cancers
Cholesterol-lowering drug suppresses chronic inflammation that creates dangerous cascade
Best Electric Cars of 2024 and 2025
The edmunds experts test electric car contenders on our test track and in the real world. our ratings assess performance, comfort, technology, utility, value and more. how we rank, best electric cars.
Mainstream electric cars are the entry point for many EV shoppers, providing a driving range of anywhere from about 100 miles to well over 200. Expect spirited acceleration, too.

Hyundai IONIQ 6
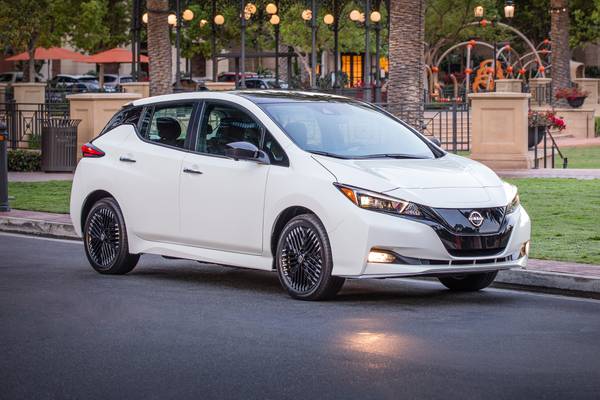
Nissan LEAF
In the world of cost-effective EVs, the Leaf's game is strong. Along with a host of standard advance driving aids, the Leaf also delivered a respectable 237 miles of range on a single charge when driven on Edmunds' real-world EV test route. Read full review about Nissan LEAF

MINI Hardtop 2 Door
The Mini Cooper SE is an electric version of the regular Hardtop 2 Door. This means less cargo room and rear seat space compared to other EVs, but also some classic Mini benefits such as quick acceleration and nimble handling. Range is more modest than most, however, traveling 150 miles in Edmunds' real-world range test. Read full review about MINI Hardtop 2 Door
Best Electric SUVs
The line between SUV and hatchback gets blurry in this segment, but you can count on versatile cargo space and an elevated driving position.

Kia EV9 GT-Line

Hyundai Ioniq 5 Limited AWD
The Hyundai Ioniq 5 springs out of the gate with retro-themed styling and the latest in electric vehicle design. It's also comfortable, practical and generously equipped. While it can't quite match the range of the equivalent Tesla Model Y or Ford Mustang Mach-E models, there's still plenty to love about this all-new all-electric SUV. Read full review about Hyundai Ioniq 5 Limited AWD

Kia Niro EV
The Niro EV isn't the best in any one particular area, but it does a lot of things very well. It's comfortable, packed with in-car tech, and has impressive real-world range. It's not the cheapest EV out there, but Kia's long list of standard features and excellent warranty help offset that. Read full review about Kia Niro EV
Best All-wheel-drive electric trucks
As the dominant truck brands branch out into electric territory, the race is on to provide traditional truck attributes in a battery-powered package.

Ford F-150 Lightning Platinum
Best electric vans.
SUVs tend to get all the press, but electric vans quietly get the job done. A van gives you the most practical shape for hauling people and cargo alike.

Ford E-Transit Cargo Van
Best luxury electric cars.
Luxury electric cars tend to cost a lot more than mainstream models, but you also get a lot more, typically including sports-car acceleration and the latest interior tech.

BMW i5 eDrive40

Tesla Model 3 Long Range
The Tesla Model 3 offers an enticing combination of performance, comfort and range, and its price continues to undercut the competition. The updated Model 3 also impresses with its improved ride comfort and added amenities such as ventilated front seats. It's a great choice for a well-rounded EV. Read full review about Tesla Model 3 Long Range

Mercedes-Benz EQE 350 4Matic
The Mercedes-Benz EQE pretty much does everything the E-Class does, just with electric power. It's comfortable, quiet and packed full of features. It also has a roomy cargo area and a long range on a full charge. There are a few drawbacks, but overall we think the EQE is a smart pick for a luxury EV. Read full review about Mercedes-Benz EQE 350 4Matic
Best Luxury electric SUVs
Given the popularity of luxury SUVs, it's no surprise that luxury electric SUVs are on the rise. Versatile interior space, cutting-edge technology and impressive power are par for the course.

BMW iX xDrive50

Genesis Electrified GV70
The electrified version of the Genesis GV70 compromises little and presents major gains in acceleration and smoothness compared to its gasoline-burning counterpart. Its lackluster range aside, the Electrified GV70 resets the bar on what a luxury SUV can be. Read full review about Genesis Electrified GV70

Tesla Model Y Long Range
The Model Y Long Range might not have the crazy acceleration of the Performance model, but it's the version we prefer. It's still pleasingly quick but is smoother riding, and as its name states, has a longer range. This is one stylish and roomy electric SUV with strong appeal. Read full review about Tesla Model Y Long Range
Best Luxury electric trucks
Electric trucks tend to cost a pretty penny, so we consider them luxury vehicles by default. But if you can get past the price tags, you'll find that this new pickup breed offers a beguiling blend of performance, utility and, yes, luxury features.

Best Super luxury electric cars
Want to get the most range out of your executive-class EV? This is the segment for you. Because these cars lack all-wheel drive, they maximize the platform's range potential.

BMW i7 xDrive60

Porsche Taycan Performance Battery Plus
While the 4S, GTS, Turbo and Turbo S variants return stunning performance, the base rear-wheel-drive Taycan model doesn't excite in quite the same way. We found it competent, comfortable and highly customizable, but it lacks the performance punch you expect from the brand. Read full review about Porsche Taycan Performance Battery Plus

Mercedes-Benz EQS 580 4Matic
The EQS 580 is one of the best luxury EVs around thanks to its impressive technology, driver comfort and exceptional range. Easy to drive and easy to use, the EQS 580 is a clear indication of what's to come from Mercedes-Benz. We just wish there was a little more S-Class in the EQS. Read full review about Mercedes-Benz EQS 580 4Matic
Best Super luxury electric SUVs

Mercedes-Benz EQS SUV 450+
Best super luxury electric trucks.

GMC Hummer EV Edition 1
Best performance electric cars.
Pretty much every electric car with more than one motor is going to be fast, but some EVs prioritize performance above all else. These are the true athletes of the EV world.

Best Performance electric SUVs
Want your performance wrapped in a more versatile body style? These speedy SUVs are inherently taller and heavier than their car counterparts, but it's hard to argue with the extra space.

Hyundai IONIQ 5 N
The words "fun" and "EV" are no longer mutually exclusive. The Hyundai Ioniq 5 N is just as impressive on a twisty back road as it is blistering quick to 60 mph. On top of all its mind-bending performance, the 5 N retains all the creature comforts of the standard Ioniq 5. If you want a modern, electric interpretation of a hot hatchback, the Ioniq 5 N is essentially unmatched. Read full review about Hyundai IONIQ 5 N

Genesis GV60 Performance
The GV60 is brimming with technology, power and comfort. It's the first of many electric vehicles from Genesis that's sure to put Tesla and company on notice. Sharp design, super-quick charging capability and an unbeatable warranty round out what is simply one of the best EVs on sale. Read full review about Genesis GV60 Performance
Best Exotic performance electric cars
If cost is no object and you like feeling nauseous when you floor it, try one of these electron-munching speed demons. EV acceleration is a whole new ballgame, and these cars epitomize it.

Mercedes-Benz EQS AMG 53 4Matic+
Porsche taycan 4s.
The Taycan represents Porsche's foray into the electric vehicle space, and it does not disappoint. The Taycan is a technological masterpiece that manages to deliver incredible comfort and effortless driving one moment and outstanding performance the next. Read full review about Porsche Taycan 4S

We love the all-electric BMW i7 for its super-smooth ride quality, library-quiet interior and long range. The M70 version largely builds on those attributes with increased performance at a commensurately elevated price. Read full review about BMW i7 M70
Browse other types
- Best Sedans
- Best Coupes
- Best Hatchbacks
- Best Trucks
- Best Wagons
- Best Convertibles
- Best Minivans
- Best Electrics
- Best Hybrids
Latest electric vehicle reviews
- 2024 Chevrolet Silverado EV
- 2025 Hyundai IONIQ 6
- 2025 Tesla Model Y
- 2025 Hyundai IONIQ 5
- 2025 BMW iX
- 2024 Honda Prologue
Video reviews
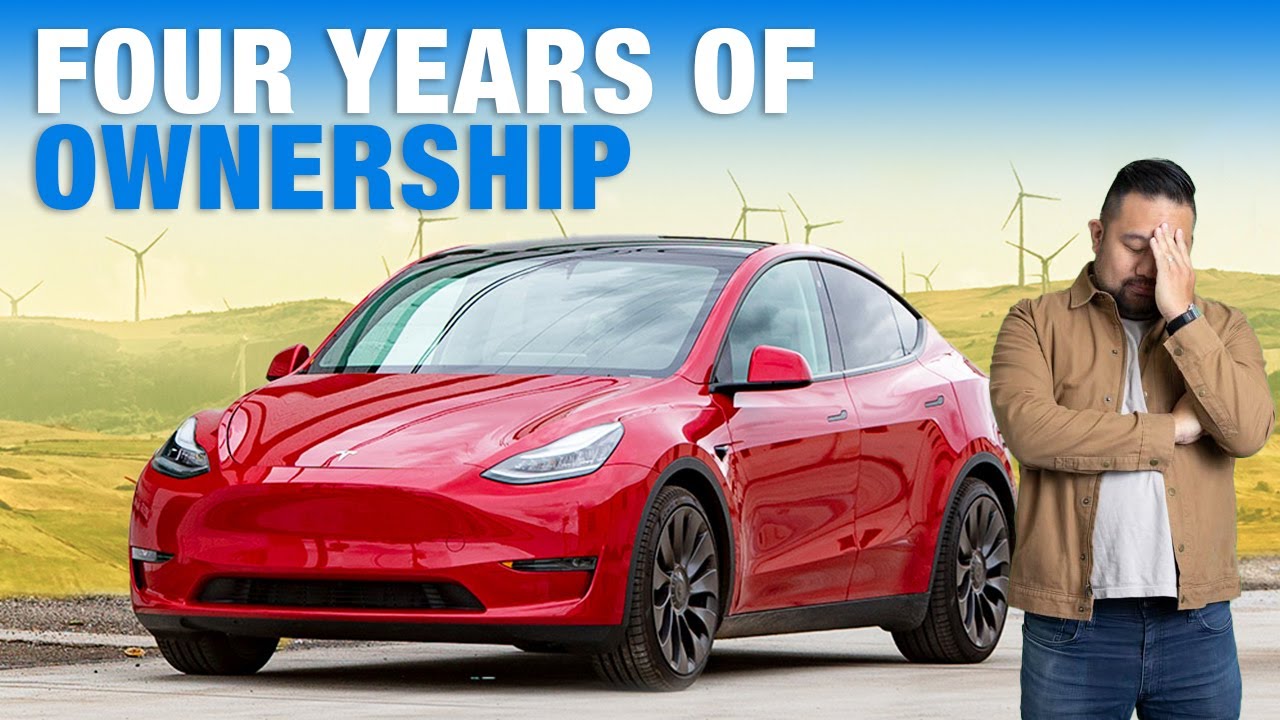
40,000 Miles in Our Tesla Model Y Performance | What It's Like to Live With & How It's Held Up
- 40,000 Miles in Our Tesla Model Y Performance | What It's Li...
- Fastest & Slowest Charging EVs | When Speed Really Matters |...
- The Hyundai Ioniq 5 N Is the Most Fun Electric Car You Can B...
- The G-Wagen Goes Electric | 2025 Mercedes-Benz G580 with EQ ...
- DRIVEN: The All-Electric 2025 Porsche Macan Will Make You Fo...
- Fisker Ocean Long-Term Test Update: New Software, New Proble...
- The Porsche Taycan Turbo GT Is an Electrified Monster | Driv...
- We Bought a 2024 Tesla Model 3! | Better Than Before? | Full...
- 17,000 Miles in the BMW iX | What It's Like to Live with BMW...
- Tesla Cybertruck vs. Rivian R1T vs. Ford F-150 Lightning! | ...
- FIRST LOOK: ALL-NEW 2024 Dodge Challenger Daytona! | Saying ...
- 18,000 Miles in the Ford Mustang Mach-E | What It's Like to ...
- RANGE TESTED: Tesla Cybertruck! | Edmunds EV Range Test
- U-DRAG RACE: Acura NSX Type S vs. Lucid Air Grand Touring | ...
- The Cheapest EVs You Can Buy Today | Most Affordable Electri...
- 2025 Porsche Taycan Highway Range Test | Refreshed & Ready t...
- 2024 Porsche Macan EV First Look | Macan Goes Electric | Int...
- Edmunds Top Rated 2024 | The Best Cars, Trucks and SUVs for ...
- We Bought a $70,000 FISKER OCEAN! | What We Bought & Why | L...
- Porsche Macan EV Highway Range Test | Behind the Wheels of P...
What are the best electric vehicles on the market?
What is the top-rated electric vehicle for 2019, what is the top-rated electric vehicle for 2018, what are the best used electric vehicles to buy, browse electric vehicles by brand.
- Mercedes-Benz
Edmunds Latest Electric Car News
Edmunds tested: electric car range and consumption, mercedes-benz eqs 450+ is the longest-range electric suv we've ever tested, jeep is racing tesla to the $25,000 ev, edmunds top rated kia ev9 joins our long-term test fleet, related information, recent automotive news.
- 2025 Ford Expedition Will Get the Huge Dash Screen from the Lincoln Nautilus
- 2024 Jeep Wagoneer S First Look: Jeep's First EV Goes 300 Miles, Has Massive Wing
- The Jeep Wagoneer S Trailhawk Concept Is the Off-Road EV We Really Want
- How We'd Spec It: 2025 Porsche 911 GTS Hybrid
- CarCast+Edmunds Podcast: Is now a good time to buy a car?
- 2024 Chevy Equinox EV First Drive: A Winning Recipe
- 2024 Ford Mustang Mach-E Rally First Drive: It Does What It Says on the Door
- Here's How Our Long-Term Tesla Model Y Held Up After 4 Years
- 2025 Lincoln Navigator Spied With a Fancy New Face
- Chevy Corvette E-Ray Battles BMW M8 Competition in Edmunds U-Drags
Popular new car reviews and ratings
- Mercedes Benz Eqc
- CLS Amg 63 Amg
- 2023Audi RS3
- Toyota Corolla Hybrid Cars
- Toyota Land Cruiser
Lease deals by make
- Hyundai Lease Deals
- Genesis Lease Deals
- GMC Lease Deals
- Cadillac Lease Deals
- Ford Lease Deals
- Buick Lease Deals
- BMW Lease Deals
- Honda Lease Deals
- Audi Lease Deals
- Chevrolet Lease Deals
Lease deals by model
- Audi R8 Lease Deals
- Chevrolet Corvette Lease Deals
- Audi Q8 Lease Deals
- Audi A7 Lease Deals
- Audi Q5 Lease Deals
- Chevrolet Bolt EV Lease Deals
- Chevrolet Blazer Lease Deals
- Chevrolet Camaro Lease Deals
- Audi A5 Lexus RX-350 Lease Deals
- Audi Q7 Lease Deals
Join Edmunds
Receive pricing updates, shopping tips & more!
- Share full article
Advertisement
Supported by
Electric Cars Are Suddenly Becoming Affordable
More efficient manufacturing, falling battery costs and intense competition are lowering sticker prices for battery-powered models to within striking distance of gasoline cars.

By Jack Ewing
Alex Lawrence, a dealer in Salt Lake City who specializes in used electric cars, has seen a change over the last year in the kinds of customers who are coming into his showroom. They used to be well-heeled professionals who could drop $70,000 on a Rivian luxury pickup truck.
Recently, Mr. Lawrence said, customers have been snapping up used Teslas for a little over $20,000, after applying a $4,000 federal tax credit.
“We’re seeing younger people,” Mr. Lawrence said. “We are seeing more blue-collar and entry-level white-collar people. The purchase price of the car has suddenly become in reach.”
Regarded by conservative politicians and other critics as playthings of the liberal elite, electric vehicles are fast becoming more accessible. Prices are falling because of increased competition, lower raw-material costs and more efficient manufacturing. Federal tax credits of up to $7,500 for new electric cars, often augmented by thousands of dollars in state incentives, push prices even lower.
At the same time, technology is improving quickly and making electric vehicles more practical. Cars that can travel more than 300 miles on a fully charged battery are becoming common, and charging times are dropping below 30 minutes. The number of fast chargers, which can top up a battery in less than half an hour, grew 36 percent from April 2023 to April 2024.
Carmakers including Tesla, Ford, General Motors and Stellantis, the owner of Jeep, have announced plans for electric vehicles that would sell new for as little as $25,000.
“The E.V. market has hit an inflection point,” said Randy Parker, chief executive of Hyundai Motor America, which will begin producing electric vehicles at a factory in Georgia by the end of the year. “The early adopters have come. They’ve got their cars. Now you’re starting to see us transition to a mass market.”
All this is good news for proponents of electric vehicles and the Biden administration, which is aiming for half of new cars sold to be electric by 2030 as part of the president’s plan to combat climate change. Even if Republicans gain control of the White House and Congress and follow through on promises to dismantle electric vehicle subsidies, they may not be able to undo the market forces pushing down prices.
“There may be some hiccups in the exact pace and scale of E.V. sales if there are major policy changes, but I wouldn’t expect the E.V. market to flatline,” said Peter Slowik, who leads research on passenger cars at the International Council on Clean Transportation, a research organization. “Most automakers are committed to an all-electric future, and many are planning on a timeline that goes far beyond the next administration.”
Electric cars, sales of which have slowed in recent months, are still more expensive than gasoline models, costing an average of $55,252 in the United States in April, according to estimates by Kelley Blue Book. That is a decline of 9 percent from April 2023, but still about $6,700 more than the average for all vehicles.
But Mr. Slowik’s group estimates that cars and sport-utility vehicles capable of traveling 400 miles on a full battery will cost less than cars with internal combustion engines in 2030, even before taking into account government subsidies. (Pickup trucks, which require bigger batteries, will take a little longer, not reaching parity for 400-mile models until 2033.)
Those calculations do not take into account lower fuel and maintenance costs that strengthen the financial argument for electric vehicles. Electricity is almost always cheaper per mile than gasoline, and battery-powered vehicles don’t need oil changes, engine air filters or spark plugs. For people who drive a lot, electric cars may already be a better deal. At the same time, some automakers are offering strong discounts on E.V. models as an enticement for buyers.
While prices are clearly trending downward, there are risks. China supplies more than half of the lithium-ion batteries used in cars sold in the United States, according to Interact Analysis, a research firm. Those batteries will become more expensive because the Biden administration announced in May that it would raise tariffs on them to 25 percent from 7.5 percent.
Many companies are building battery factories in the United States and Canada , but most of these won’t produce enough batteries to replace China for several years.
Raw materials are another risk. The price of lithium and other materials required for batteries has plunged in the last 12 months, making electric cars cheaper. But commodity prices could soar again.
The recent slowdown in the growth of electric car sales has prompted Tesla, Ford and others to delay plans to expand manufacturing. But many analysts expect sales to pick up as a glut of models pushes down prices, and as the charging network grows. High prices and the fear of not being able to find a place to recharge are the two biggest reasons people hesitate to buy an electric vehicle, surveys show.
For many people, the car’s price is not the only expense to consider. People who live in apartments often depend on public charging plugs. Public charging, besides being less convenient, tends to be more costly than charging at home.
Still, the forces pushing prices down are powerful. Manufacturing costs are dropping as traditional carmakers, who were slow to sell electric vehicles, start to apply their decades of experience with mass production to the new technology.
Later this year, for example, General Motors will begin selling an electric version of its Chevrolet Equinox sport-utility vehicle that will have a range of more than 300 miles and sell for less than $30,000 after the $7,500 federal tax credit. And the company plans to sell an even cheaper car, a new Chevrolet Bolt, next year.
The Equinox and Bolt will be built on G.M.’s Ultium platform, a collection of components that can be used for a variety of vehicles including pickups and luxury Cadillacs. G.M., which has cut costs by using the same batteries and parts for different models, has said its electric vehicles will become profitable in the second half of this year.
Electric cars still cost more to manufacture than cars with internal combustion engines, said Prateek Biswas, an analyst at Wood Mackenzie, a research firm. But costs will come down as companies learn how to produce the cars more efficiently, Mr. Biswas said — for example, by eliminating rare minerals from electric motors or replacing copper wiring with aluminum.
At the same time, the cost to make a gasoline car is rising because of stricter emissions regulations. “At some point it will be easier to just move toward E.V.s,” Mr. Biswas said.
Competition is also intensifying. Toyota and other Japanese carmakers with a reputation for delivering reliable and affordable vehicles are belatedly offering electric vehicles. Honda plans to begin producing them at an Ohio factory next year.
There will be more than 100 fully electric models for sale in the United States by next year, according to Cars.com, an online sales platform, double the number available last year. “We’re at the point now where anybody that wants an E.V. for a price point can actually get an E.V.,” said Rebecca Lindland, senior director of industry data at Cars Commerce, which operates Cars.com.
Used car prices are arguably more important than prices of new cars. Most people buy used cars. A vibrant used market vastly increases the number of people who can consider an electric vehicle.
Models from Tesla, Nissan or G.M. have been on the road for three years or more, generating inventory for dealers as the original owners buy new ones. More than half of the used electric vehicles on the market sell for less than $30,000, according to Recurrent, a research firm that focuses on the used E.V. market.
Jesse Lore, owner of Green Wave Electric Vehicles in North Hampton, N.H., recently sold a used Chevy Bolt for $15,000. After applying a federal tax credit for used electric vehicles, the price was $11,000. Besides the lure of affordable prices, he noted, his customers like that electric vehicles are quieter than gasoline models, better for the environment, and faster because an electric motor generates instant torque.
“The car is more fun than whatever they’re driving now,” Mr. Lore said.
Jack Ewing writes about the auto industry with an emphasis on electric vehicles. More about Jack Ewing
The current research on electric vehicle
Ieee account.
- Change Username/Password
- Update Address
Purchase Details
- Payment Options
- Order History
- View Purchased Documents
Profile Information
- Communications Preferences
- Profession and Education
- Technical Interests
- US & Canada: +1 800 678 4333
- Worldwide: +1 732 981 0060
- Contact & Support
- About IEEE Xplore
- Accessibility
- Terms of Use
- Nondiscrimination Policy
- Privacy & Opting Out of Cookies
A not-for-profit organization, IEEE is the world's largest technical professional organization dedicated to advancing technology for the benefit of humanity. © Copyright 2024 IEEE - All rights reserved. Use of this web site signifies your agreement to the terms and conditions.
Electric Vehicles Research Service

Latest Research

Industrial Lift Truck Market: Key Trends and Expanding Automation

Securing the EV Supply Chain: Battery Recycling and Circularity Regulation in the EU

The EV Market Slowdown

Key Takeaways From NVIDIA GTC 2024

Securing the EV Supply Chain: Battery Recycling in the United States
Related spotlights.

View All Research
Companies Covered
Battery technologies inc.
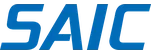
Analog Devices Inc
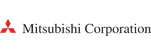
Ford Motor Company
Volkswagen group, ads-tec energy, general motors corporation.
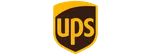
Analyst Support
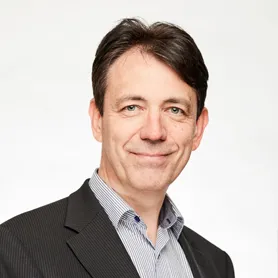
Electric Vehicles Coverage

Coverage Areas Include
- Lithium Metal Battery Chemistries and Novel Alternatives
- Silicon Anode
- Battery Management Systems
- Removing Rare Earth Metals and Scarce Materials
- Scaling EV Battery Production and Cost Reduction
- EV Propulsion
- Smart Charging, Vehicle-to-Grid (V2G), and Bi-Directional Energy Flow
- PEV versus Hydrogen Fuel Cell (HFC)-EV in Commercial Vehicles
- Electrified Micromobility
- Battery Lifecycle Management and Safety
- Wireless Charging
- Buffer Battery Charging and Microgrids
- EV Supply Chain Consolidation
- Battery Swapping
Deliverables
Latest news & resources for electric vehicles, filter by type, reports & data.
Research Report | 2Q 2024 | AN-5555
Research Report | 2Q 2024 | AN-5804
Research Report | 2Q 2024 | AN-6131
Whitepaper | 1Q 2024 | WP-200
Research Report | 1Q 2024 | AN-5803
Key Takeaways From CES 2024
Whitepaper | 1Q 2024 | WP-1037
82 Technology Trends That Will—And Will Not—Shape 2024
Whitepaper | 1Q 2024 | WP-1035
55 Technology Leaders To Watch In 2024
Whitepaper | 4Q 2023 | WP-1034
Accelerating EV Charging Rates
Research Report | 4Q 2023 | AN-5810
Electric Vehicle Charging Infrastructure
Market Data | 4Q 2023 | MD-EVCI-23
The Sustainable Future is Here. Are You Ready?
Whitepaper | 4Q 2023 | WP-1026
Electric Vehicles
Market Data | 3Q 2023 | MD-EV-102
Next-Generation Electric Vehicle Batteries
Research Report | 3Q 2023 | AN-5808
Chinese Electric Vehicle OEMs
Research Report | 3Q 2023 | AN-5809
Battery Management Systems for Electric Vehicles
Research Report | 2Q 2023 | AN-5807
Zero-Emission Vehicle Adoption in Fleets: Investments, Use Cases, Reduced Costs, Regulations, and Infrastructure
Research Report | 2Q 2023 | AN-5483
Market Data | 1Q 2023 | MD-EV-101
37 Technology Stats You Need to Know for 2023
Whitepaper | 1Q 2023 | WP-1013
Electric Vehicle Smart Charging Platforms
Research Report | 4Q 2022 | AN-5545
Bringing Electric Vehicles into the Mainstream
Research Report | 1Q 2022 | AN-5480
Connected Services for Electric Vehicles
Presentation | 2Q 2020 | PT-2331
Electric Vehicle Battery and Charging Technologies
Research Report | 3Q 2019 | AN-5152
Vehicle-to-Grid Technologies and Applications
Research Report | 3Q 2018 | AN-4995
Connected Vehicle Developments Heat up in the Southeast Asian Region: What Opportunities Lie ahead for CSPs?
Insight | 2Q 2024 | IN-7358
Addressing EV Charging Pain Points with Artificial Intelligence
Insight | 2Q 2024 | IN-7368
Biden’s Tariffs Will Keep Chinese EVs out of the United States, and Mexico Will Not Serve as a Backdoor
Insight | 2Q 2024 | IN-7318
BMW’s Investments Combined with Its Multi-pronged Strategy Demonstrate How Traditional Automotive OEMs Can Prepare for the EV Era
Insight | 2Q 2024 | IN-7310
North American OEMs Launch Their Own Charging Network to Fight Against a Tesla Monopoly
Insight | 2Q 2024 | IN-7297
Modern Wheels Coupled with Outdated Infrastructure: EVs Facing Increasing Cybersecurity Vulnerabilities
Insight | 1Q 2024 | IN-7259
VinFast Makes Headlines at CES 2024, but Supplier Beware
Insight | 1Q 2024 | IN-7228
CES 2024: Key Highlights from A Supply Chain Lens
Insight | 1Q 2024 | IN-7224
BYD Overtakes Tesla, Presenting a Growing Threat to Legacy OEMs
Insight | 1Q 2024 | IN-7218
The EV Market in Indonesia: A Push to Become a Leading Manufacturing Base in the Southeast Asian Region
Insight | 1Q 2024 | IN-7188
Stellantis Follows Volkswagen by Buying a Stake in a Chinese OEM, but Not for the Same Reasons
Insight | 4Q 2023 | IN-7165
Tesla Revamps the Model 3, Staying Ahead of the Competition in the West but Fighting for Market Share in China
Insight | 4Q 2023 | IN-7090
CarbonScape Invests US$18 Million in Biographite Production in Europe and the United States to Tackle Material Scarcity for EVs and Battery Storage
Insight | 3Q 2023 | IN-7087
CCS Is Dead in North America as Tesla’s NACS Plug Takes Over
Insight | 3Q 2023 | IN-7005
Dassault Systèmes’ 2023 Analyst Days: The Strategy Is to Focus on Experiences, Outcomes, and Health
Insight | 2Q 2023 | IN-6976
JLR Partners with Elektrobit to Enable Cutting-Edge In-Car Digital Experiences in Its Next-Generation Vehicles
Insight | 2Q 2023 | IN-6955
NIO Takes On Tesla and Aims to Disrupt the Global Fast Charging Market with Its Latest Battery Swap Technology
Insight | 2Q 2023 | IN-6908
The European Union Will Ban All Polluting Cars from 2035. Who’s Ready for It?
Insight | 1Q 2023 | IN-6868
Supply Chain Challenges to Curtail Electric Vehicle Production over the Next Decade
Insight | 3Q 2022 | IN-6625
Why are Electric Vehicles at the Forefront of Vehicle Tech?
Insight | 2Q 2022 | IN-6570
Identifying and Addressing the Challenges to Mainstream Electric Vehicles
Insight | 1Q 2022 | IN-6399
How Electric Vehicle Charging Systems are Accelerating the Clean Energy Revolution
Insight | 1Q 2021 | IN-6034
Subscribe now for data-driven insight and expert analysis to fuel your business.

Cyber & Digital Security
Industrial & manufacturing, sustainable technologies, all other services.
- Resource Center
- Journalist Inquiry
- ABI Research News
- Management Team
- Office Locations
©2024 Allied Business Intelligence, Inc. All Rights Reserved
We've detected unusual activity from your computer network
To continue, please click the box below to let us know you're not a robot.
Why did this happen?
Please make sure your browser supports JavaScript and cookies and that you are not blocking them from loading. For more information you can review our Terms of Service and Cookie Policy .
For inquiries related to this message please contact our support team and provide the reference ID below.
- My View My View
- Following Following
- Saved Saved
How China's EV makers aim to beat Tesla, legacy automakers in Europe
- Medium Text
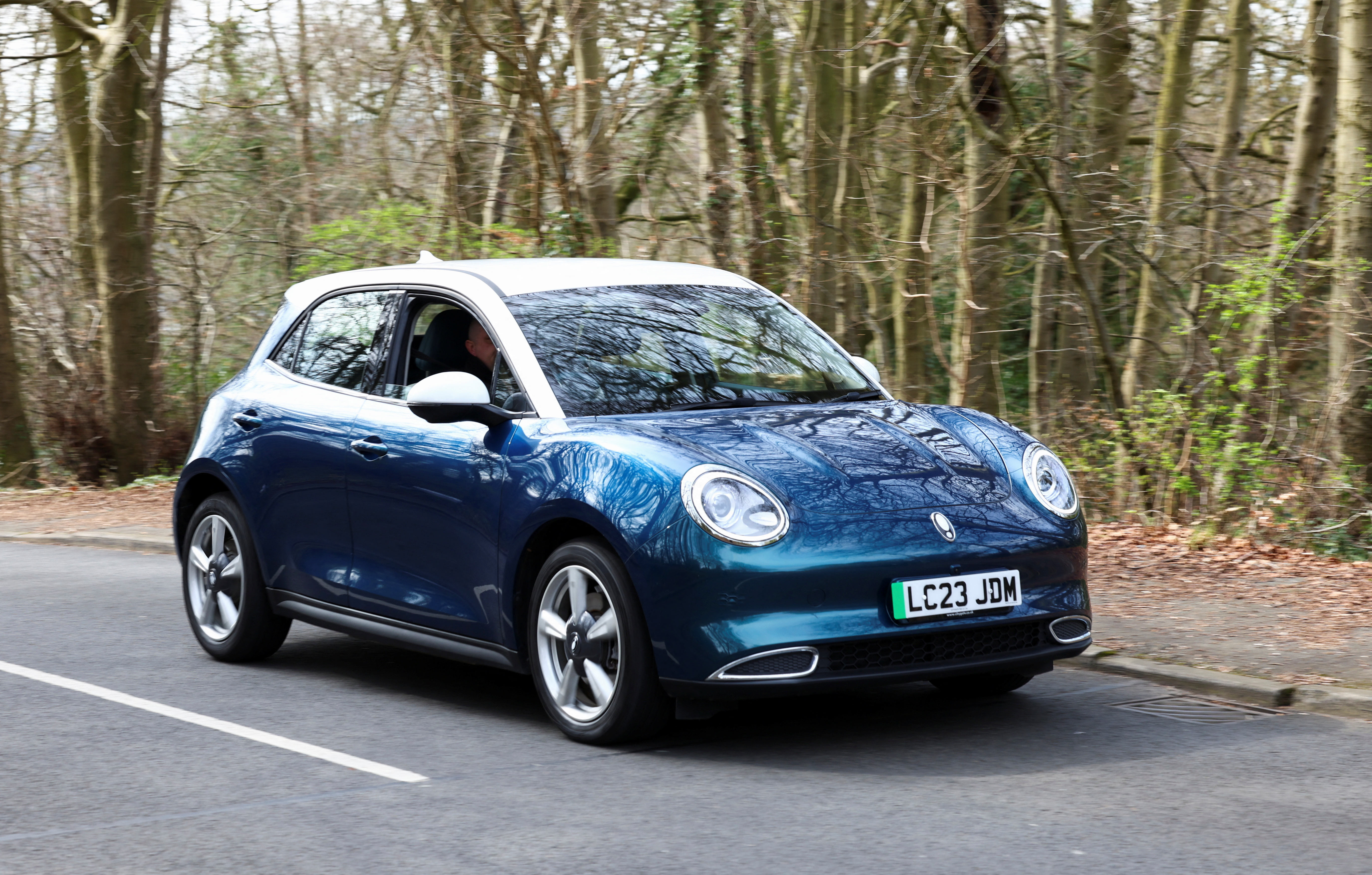
- Company Ald Sa Follow
- Company BYD Co Ltd Follow
- Company Chery Automobile Co Ltd Follow
COST ADVANTAGES
Long-term investing.
Sign up here.
Reporting by Nick Carey, Giulio Piovaccari, Marie Mannes and Christina Amann; Editing by Brian Thevenot and Daniel Flynn
Our Standards: The Thomson Reuters Trust Principles. New Tab , opens new tab
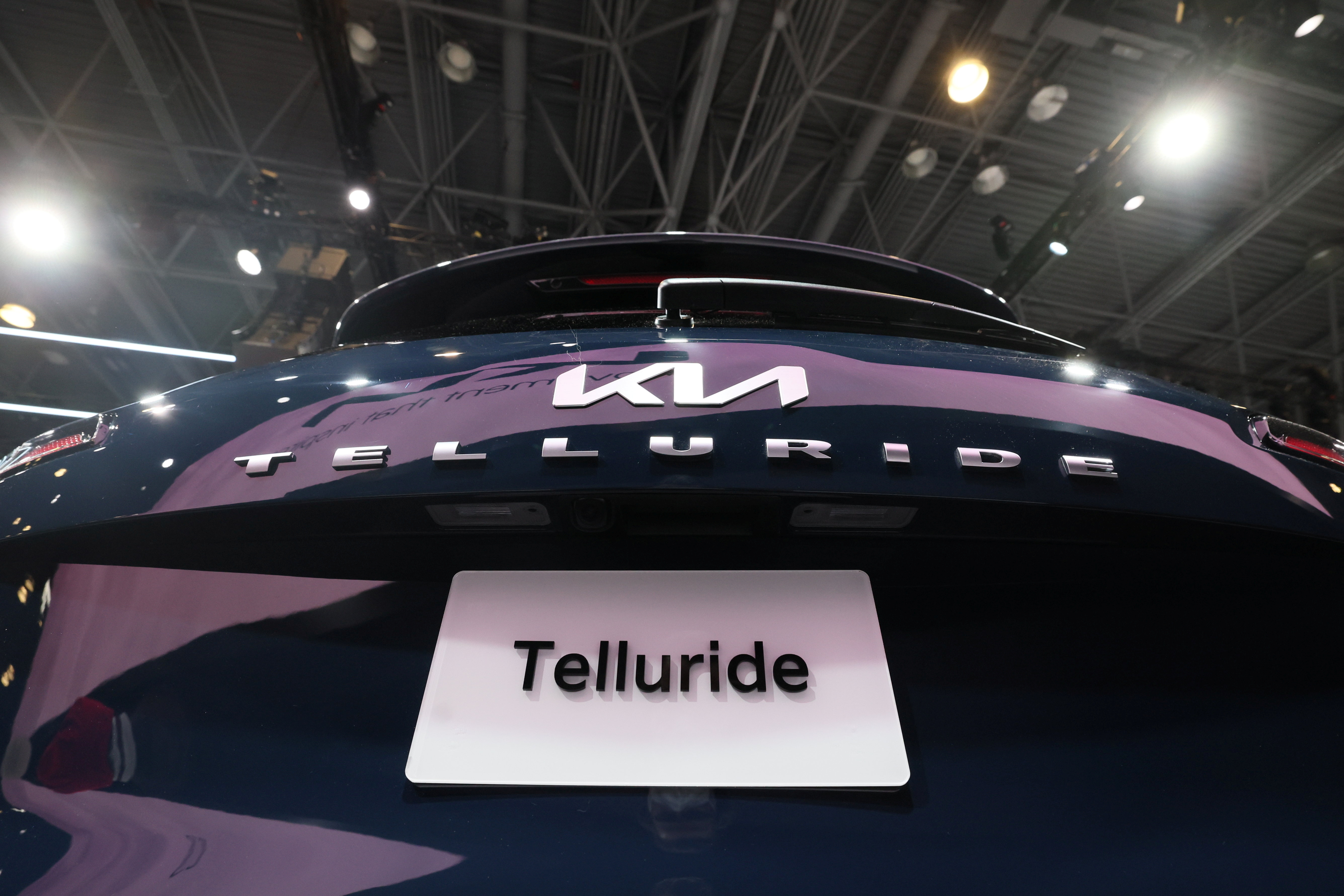
Business Chevron
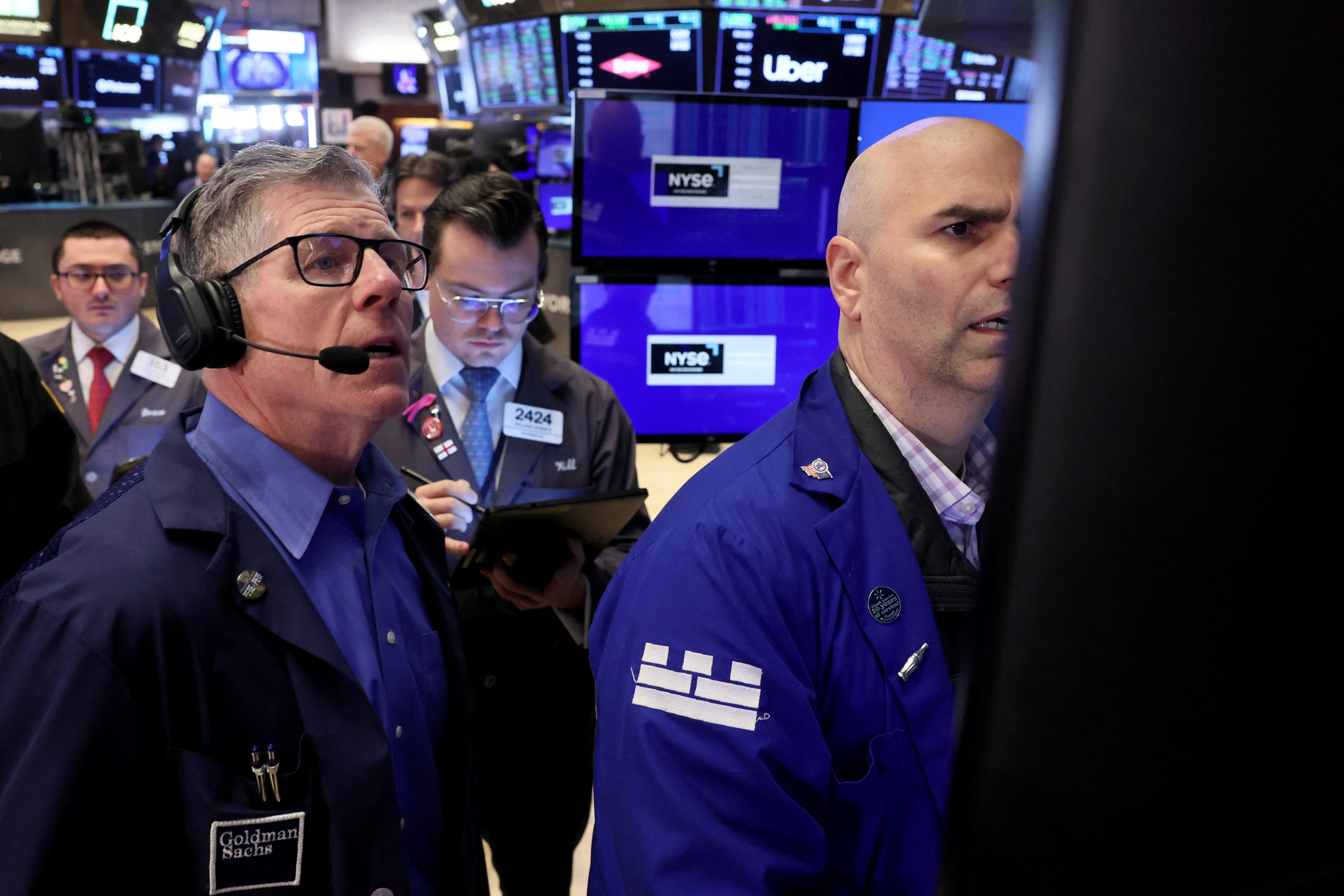
Wall Street stocks close slightly lower; jobs data strong but rates still high
Wall Street stocks ended slightly lower on Friday in choppy trading after stronger-than-expected U.S. jobs data pointed to a robust economy but prompted worries the Federal Reserve may wait longer to cut interest rates than many investors had hoped.
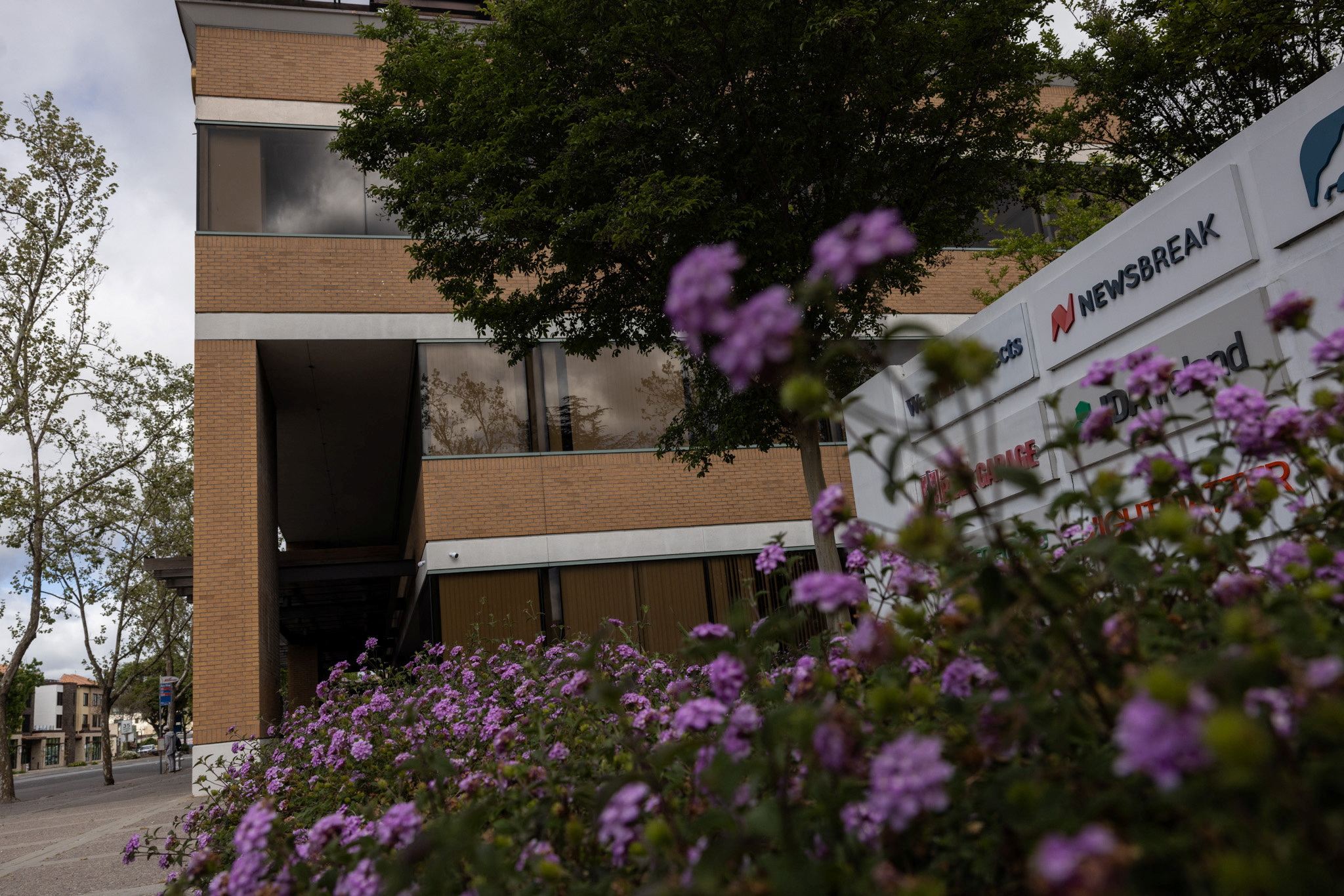
AAA Newsroom
Automotive, Travel, and Traffic Safety Information
Is the EV Hype Over?
Aaa finds interest in evs declining as americans turn their attention to hybrids.
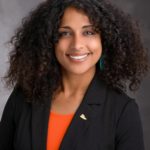
ORLANDO, Fla. (June 6, 2024) – The most recent annual consumer survey by AAA on Electric Vehicles (EVs) indicates a decline in consumer interest in purchasing EVs. Only 18% of U.S. adults say they would be “very likely” or “likely” to buy a new or used EV (not a hybrid) – down from 23% last year . Even more revealing, 63% cited “unlikely or very unlikely” to purchase an EV for their next car purchase.
“Early adopters who wanted an EV already have one,” said Greg Brannon, director of automotive research at AAA. “The remaining group of people who have yet to adopt EVs consider the practicality, cost, convenience, and ownership experience, and for some, those are big enough hurdles to keep them from making the jump to fully electric.”
AAA found the main hesitations in purchasing an EV continue to be cost, lack of convenient charging options, and range anxiety. Three in ten also cited the inability to install a charging station where they live.
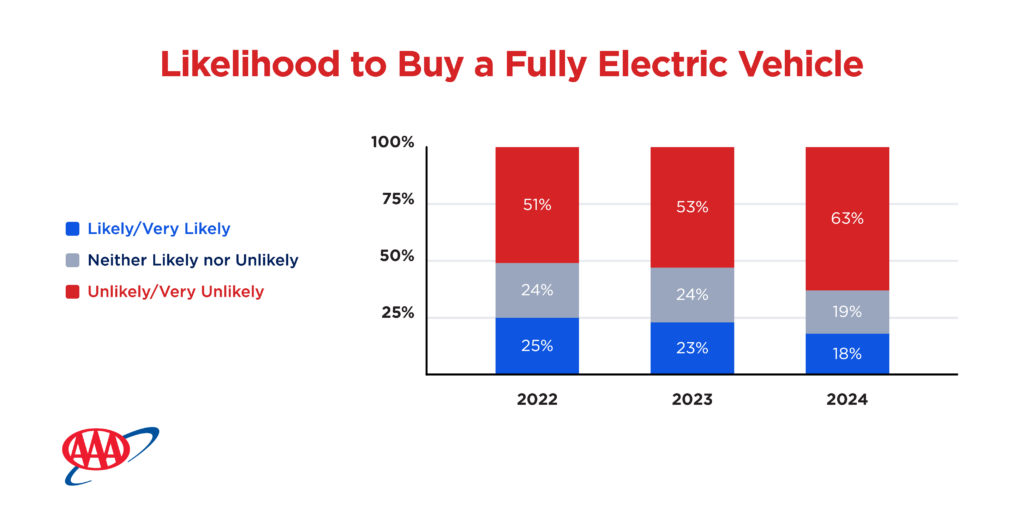
Accessible, reliable, affordable, and convenient charging is key to growing EV interest and adoption. For people who live in an apartment or condo, at-home charging options are likely not possible. An EV might be a great choice for households with 2+ cars, but it might not fit the consumer who has to rely on their car for everyday use and travel.
AAA believes there may be a near-term ceiling related to consumer adoption of battery electric vehicles due to their costs, charging accessibility, and range anxiety. However, hybrid options could bridge these gaps, broadening consumer interest in owning an EV. AAA’s survey also found that one in three U.S. adults (31%) say they would be “very likely” or “likely” to buy a hybrid. Access to a hybrid vehicle lessens the anxiety for consumers because it allows people to enjoy the benefits of electrification without feeling like they are disrupting their current lifestyle or travel plans (longer distance driving, less charging options, etc.).
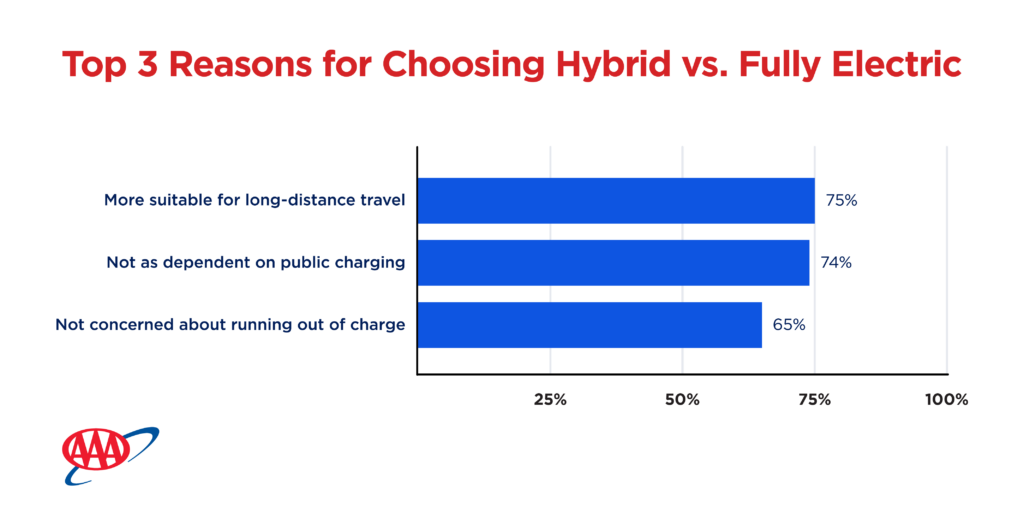
“Deciding to make the leap to full electric may feel overwhelming for many consumers, and a hybrid option may be the way to bridge this gap,” continued Brannon. “Consumer demand will ultimately dictate the future, and my prediction is that we will have a mix of EVs, hybrids, and internal combustion vehicles in dealerships and on the roads in the US for many decades ahead.”
To help educate the public, AAA conducts ongoing research on EVs, including consumer sentiment surveys, testing to determine factors impacting electric vehicle range, the true cost of electric vehicle ownership, and a survey on consumers’ experience with going electric.
AAA has a range of resources and services for EV owners, those interested in making the switch, or those who want to try a rental.
- EV 101 : Online resource for anyone interested in going electric, whether shopping for new OR used.
- The “CARBs” of Driving an EV : An acronym for 1st time EV Drivers that survey Point of View (POV) survey from 1 st- time drivers on how to remember EV-based driving tips.
- Your Driving Cost Calculator : An online resource available to consumers that gives a yearly breakdown of EV ownership costs
- #AdultingwithAAA: Car Care Electric Vehicles – Low Maintenance – No Problem: AAA series for young adults providing helpful tips, advice, and life hacks on all things “Adulting.”
- Mobile EV Charging Pilot : Available in select cities, this service is now part of our roadside assistance and will get EV owners back on the go if they run out of charge. That’s just one of the many benefits and services we offer our EV members.
- EV Research : AAA in-house experts research consumer sentiment around EVs and their functionality.
- AAA Car Guide: For those in the market for newer EVs, the online resources also contain links to the two prior editions in 2022 and 2023.
Methodology
The survey was conducted April 4-8, 2024, using a probability-based panel designed to be representative of the U.S. household population overall. The panel provides sample coverage of approximately 97% of the U.S. household population. Most surveys were completed online; consumers without Internet access were surveyed over the phone.
A total of 1,152 interviews were completed among U.S. adults, 18 years of age or older. The margin of error for the study overall is +/- 4% at the 95% confidence level. Smaller subgroups have larger error margins.
About AAA
Started in 1902 by automotive enthusiasts who wanted to chart a path for better roads in America and advocate for safe mobility, AAA has transformed into one of North America’s largest membership organizations. Today, AAA provides roadside assistance, travel, discounts, financial and insurance services to enhance the life journey of over 64 million members across North America, including over 57 million in the United States. To learn more about all AAA offers or become a member visit AAA.com .
Create an account
Create a free IEA account to download our reports or subcribe to a paid service.
SUVs are setting new sales records each year – and so are their emissions

Cite commentary
IEA (2024), SUVs are setting new sales records each year – and so are their emissions , IEA, Paris https://www.iea.org/commentaries/suvs-are-setting-new-sales-records-each-year-and-so-are-their-emissions, Licence: CC BY 4.0
Share this commentary
- Share on Twitter Twitter
- Share on Facebook Facebook
- Share on LinkedIn LinkedIn
- Share on Email Email
- Share on Print Print
The large, heavy passenger vehicles were responsible for over 20% of the growth in global energy-related CO2 emissions last year
SUVs accounted for 48% of global car sales in 2023, reaching a new record and further strengthening the defining automobile trend of the early 21 st century – the shift towards ever larger and heavier cars. There are various driving forces behind this trend, from the appeal of SUVs as a status symbol and their potential enhancements in comfort, to the marketing strategies of leading automakers.
New car registrations by size and powertrain, 2010-2023
In advanced economies, SUV sales reached around 20 million last year, surpassing a market share of 50% for the first time. This preference for larger vehicles extends to emerging and developing economies, too, where the share of SUVs in total car sales mirrors this trend. Today, more than one in four cars on the road worldwide are SUVs, the majority of which are conventional internal combustion engine (ICE) vehicles. While only 5% of SUVs currently on the road are electric, they account for a growing share of electric car sales. In 2023, more than 55% of new electric car registrations were SUVs.
If SUVs were a country, they would be the world’s fifth largest emitter of CO2
SUVs weigh 200-300 kg more than an average medium-sized car, and typically take up nearly 0.3 m 2 more space – emitting roughly 20% more carbon dioxide (CO 2 ) emissions. The trend towards heavier and less fuel-efficient cars increases energy demand, including oil and electricity use, as well as demand for basic metals and critical minerals needed for battery production. Over the course of 2022 and 2023, global oil consumption directly related to SUVs rose by a total of over 600 000 barrels per day, accounting for more than a quarter of the overall annual growth in oil demand.
Combustion-related CO2 emissions from SUVs and the 10 highest-emitting countries, 2023
In 2023, there were more than 360 million SUVs on the roads worldwide, resulting in combustion-related CO 2 emissions of 1 billion tonnes, an increase of around 100 million tonnes from the previous year. This accounted for more than 20% of the growth in global energy-related CO 2 emissions last year. The annual increase in CO 2 emissions attributed to the rise of SUVs is equivalent to about half of the emissions growth stemming from the global electricity sector. Compared with smaller cars, SUVs are also associated with higher indirect emissions resulting from producing the materials used to manufacture them. If ranked among countries, the global fleet of SUVs would be the world’s fifth largest emitter of CO 2 , exceeding the emissions of Japan and various other major economies.
Global electric vehicles fleet and sales of SUVs, 2023
Around 30 million SUVs with internal combustion engines were added to the global fleet in 2023, comparable to the total number of electric cars on the roads today. In 2023, there were 500 electric car models available worldwide, of which 60% fell under the SUV category, marking a significant increase from previous years. This trend is further reinforced as automakers plan to introduce a greater number of electrified SUV models in the near future.
Globally, SUVs now account for approximately 45% of the electric car fleet, a share that would be even higher were it not for the strong growth of small electric cars in urban areas in China. In advanced economies, the share of SUVs among electric cars is even higher at 55%. This is due to limited availability of smaller and more affordable compact models.
Despite advances in fuel efficiency and electrification, the trend toward heavier and less efficient vehicles such as SUVs, which emit roughly 20% more emissions than an average medium-sized car, has largely nullified the improvements in energy consumption and emissions achieved elsewhere in the world’s passenger car fleet in recent decades. Larger vehicles also pose challenges related to their increased use of critical minerals, as they are equipped with larger batteries. Additionally, they raise questions around pedestrian safety in constrained urban environments due to their higher front ends. On top of this, their greater parking space requirements, approximately 10% more than for medium-sized cars, can limit the use of valuable space in dense urban areas for other purposes.
To respond to some of these challenges, countries such as France, Norway, and Ireland have either established or are exploring legislative frameworks to reign in demand for SUVs. Major cities like Paris and Lyon are implementing higher parking charges specifically targeting SUVs in urban areas.
Shifting from fossil-fuelled cars to electric vehicles is a key strategy for reaching international energy and climate goals. However, measures such as rightsizing EV battery packs, tailoring fuel efficiency standards based on car size, and investing in innovative battery technologies with enhanced performance and durability, as well as lower material demand requirements, are also essential for a sustainable future.
This analysis was supported by the work of IEA Energy Analysts Mathilde Huismans and Jules Sery.
Subscription successful
Thank you for subscribing. You can unsubscribe at any time by clicking the link at the bottom of any IEA newsletter.

IMAGES
VIDEO
COMMENTS
Electric car sales neared 14 million in 2023, 95% of which were in China, Europe and the United States. Almost 14 million new electric cars1 were registered globally in 2023, bringing their total number on the roads to 40 million, closely tracking the sales forecast from the 2023 edition of the Global EV Outlook (GEVO-2023). Electric car sales in 2023 were 3.5 million higher than in 2022, a 35 ...
Source: Adapted from G. Harper et al. Nature 575, 75-86 (2019) and G. Offer et al. Nature 582, 485-487 (2020) Today, most electric cars run on some variant of a lithium-ion battery. Lithium is ...
Here, an integrative and bird's-eye view of scholarly research on Electric Vehicles (EV) is provided with a focus on an objective and quantitative determination of research trends. ... The most prominent example of market support is probably Norway which also has the largest market share with 86% of new sold cars in 2021 (IEA, 2022).
About six-in-ten Americans (59%) say they oppose this, while 40% favor it. The share of Americans who favor phasing out gas-powered vehicles has declined 7 points since 2021. Support is down among both Democrats and Republicans. Currently, a majority of Democrats (64%) favor phasing out production of gas-powered vehicles by 2035, but 84% of ...
First introduced at the end of the 1800s, electric vehicles (EVs) 12 have been experiencing a rise in popularity over the past few years as the technology has matured and costs (especially of batteries) have declined substantially. Worldwide support for clean transportation options (i.e. low emissions of greenhouse gasses [GHG] to mitigate climate change and criteria pollutants) has promoted ...
New research says building electric vehicles leaves a bigger carbon footprint than making gas-powered cars, though EVs make up the difference in the long run. By Mike Lee & E&E News.
Researchers at MIT have developed a cathode, the negatively-charged part of an EV lithium-ion battery, using "small organic molecules instead of cobalt," reports Hannah Northey for Energy Wire.The organic material, "would be used in an EV and cycled thousands of times throughout the car's lifespan, thereby reducing the carbon footprint and avoiding the need to mine for cobalt," writes ...
The new estimates update a study published in 2016 and add to a growing body of research underscoring the potential lifetime savings of electric cars. Comparing individual cars can be useful ...
By 2045, HEVs could achieve a 43% to 81% improvement in fuel economy and PHEVs could achieve a 73% to 96% improvement in fuel economy. In its National Economic Value Assessment of Plug-In Electric Vehicles, NREL uses a scenario approach to estimate costs and benefits of increased EV market growth across the United States.
Electric Vehicles (EVs) are gaining momentum due to several factors, including the price reduction as well as the climate and environmental awareness. This paper reviews the advances of EVs regarding battery technology trends, charging methods, as well as new research challenges and open opportunities. More specifically, an analysis of the worldwide market situation of EVs and their future ...
To hit those targets, electric cars would need to make up 90 percent of new U.S. car sales by 2050 — or people would need to drive a lot less. And to truly supplant fossil fuel vehicles ...
Caption. Solid-state batteries now being developed could be key to achieving the widespread adoption of electric vehicles — potentially a major step toward a carbon-free transportation sector. A team of researchers from MIT and the University of California at Berkeley has demonstrated the importance of keeping future low-cost, large-scale ...
This research's primary dataset contains monthly sales and registrations of 357 new vehicles in the United States of America from 2014 to 2020. In addition to this data, several web crawlers ...
Growing EV demand—with China as the hot spot. McKinsey projects that worldwide demand for passenger cars in the battery electric vehicle (BEV) segment will grow sixfold from 2021 through 2030, with annual unit sales increasing to roughly 40.0 million, from 6.5 million, over that period. China retained its position as the world's e-mobility ...
What's next for batteries. Expect new battery chemistries for electric vehicles and a manufacturing boost thanks to government funding this year. By. Casey Crownhart. January 4, 2023. BMW plans ...
The emissions-free cars and trucks will likely account for 13% of all new auto sales globally in 2022, up from 4% just two years earlier, according to the International Energy Agency. They're on ...
This battery technology could increase the lifetime of electric vehicles to that of the gasoline cars — 10 to 15 years — without the need to replace the battery. With its high current density, the battery could pave the way for electric vehicles that can fully charge within 10 to 20 minutes. The research is published in Nature.
The research proposes a new sinusoidal pulse width modulation (PWM) strategy in a way that the two carriers of the six-phase inverter are out of phase during four-pole operation and are in phase during two-pole operation. ... The electric vehicle market includes electric cars, hybrid electric cars, electric scooters and motorcycles, electric ...
Mitsubishi. Nissan. smart. Tesla. Volkswagen. See new electric vehicle s for sale. Edmunds expert reviewers rank the best electric vehicles of 2024 and 2025 on a 10-point scale that includes ...
Electric vehicles (EVs) have advanced significantly this decade, owing in part to decreasing battery costs. Yet EVs remain more costly than gasoline fueled vehicles over their useful life. This paper analyzes the additional advances that will be needed, if electric vehicles are to sig-nificantly penetrate the passenger vehicle fleet. Battery Prices
863. Dealers specializing in used electric cars say prices have come down so much that they are becoming affordable to many more people. Tristan Spinski for The New York Times. By Jack Ewing. June ...
In 21st century, Electric vehicle(EV) as a green transportation tool, EV has won great attention from researchers, leading to a series of intensive and extensive studies. With the development of high-storage battery and EV, the number of the EV will rise dramatically, so that the random charging and discharging behavior will bring new challenges to safe and stable operation of power grids. The ...
ABI Research's Electric Vehicles Research Service focuses on Electric Vehicle (EV) trends and technologies in passenger, commercial, and two-wheel vehicles. From scaling production to optimizing operation and facilitating end-of-life, the Electric Vehicle Research Service will provide holistic coverage of the new materials, chemistries, software, and services powering the zero-emission ...
Almost one-third of new EVs in the US are being shipped to California, Florida or Texas. As the US car market swiftly and steadily goes electric, the Volkswagen ID.4 remains near the front of an ...
Item 1 of 4 A person drives a GWM ORA 03 electric car in Wycombe, Britain, March 26, 2024. ... opens new tab, ... According to research in March from online car marketplace Carwow, 50% of ...
CNN —. Jeep, widely known for making rugged off-road vehicles, has unveiled its first fully electric SUV for the North American market. Jeep already offers the small Avenger EV in Europe but the ...
ORLANDO, Fla. (June 6, 2024) - The most recent annual consumer survey by AAA on Electric Vehicles (EVs) indicates a decline in consumer interest in purchasing EVs. Only 18% of U.S. adults say they would be "very likely" or "likely" to buy a new or used EV (not a hybrid) - down from 23% last year.Even more revealing, 63% cited "unlikely or very unlikely" to purchase an EV for ...
The 2024 Jeep Wagoneer S employs dual electric motors—one at each axle—giving the electric crossover AWD capability. Horsepower and torque figures are 600 and 617 pound-feet, respectively. The SUV uses a 400-volt architecture and draws power from a 100-kilowatt-hour (kWh) lithium-ion battery pack.
The large, heavy passenger vehicles were responsible for over 20% of the growth in global energy-related CO2 emissions last year. SUVs accounted for 48% of global car sales in 2023, reaching a new record and further strengthening the defining automobile trend of the early 21 st century - the shift towards ever larger and heavier cars.