- Open access
- Published: 15 April 2024

Social media addiction: associations with attachment style, mental distress, and personality
- Christiane Eichenberg 1 ,
- Raphaela Schneider 1 &
- Helena Rumpl 1
BMC Psychiatry volume 24 , Article number: 278 ( 2024 ) Cite this article
641 Accesses
1 Altmetric
Metrics details
Social media bring not only benefits but also downsides, such as addictive behavior. While an ambivalent closed insecure attachment style has been prominently linked with internet and smartphone addiction, a similar analysis for social media addiction is still pending. This study aims to explore social media addiction, focusing on variations in attachment style, mental distress, and personality between students with and without problematic social media use. Additionally, it investigates whether a specific attachment style is connected to social media addiction.
Data were collected from 571 college students (mean age = 23.61, SD = 5.00, 65.5% female; response rate = 20.06%) via an online survey administered to all enrolled students of Sigmund Freud PrivatUniversity Vienna. The Bergen Social Media Addiction Scale (BSMAS) differentiated between students addicted and not addicted to social media. Attachment style was gauged using the Bielefeld Partnership Expectations Questionnaire (BFPE), mental distress by the Brief Symptom Inventory (BSI-18), and personality by the Big Five Inventory (BFI-10).
Of the total sample, 22.7% of students were identified as addicted to social media. For personality, it was demonstrated that socially media addicted (SMA) students reported significantly higher values on the neuroticism dimension compared to not socially media addicted (NSMA) students. SMA also scored higher across all mental health dimensions—depressiveness, anxiety, and somatization. SMA more frequently exhibited an insecure attachment style than NSMA, specifically, an ambivalent closed attachment style. A two-step cluster analysis validated the initial findings, uncovering three clusters: (1) secure attachment, primarily linked with fewer occurrences of social media addiction and a lower incidence of mental health problems; (2) ambivalent closed attachment, generally associated with a higher rate of social media addiction and increased levels of mental health problems; and (3) ambivalent clingy attachment, manifesting a medium prevalence of social media addiction and a relatively equitable mental health profile.
Conclusions
The outcomes are aligned with previous research on internet and smartphone addiction, pointing out the relevance of an ambivalent closed attachment style in all three contexts. Therapeutic interventions for social media addiction should be developed and implemented considering these findings.
Peer Review reports
Introduction
Digital media have become ubiquitous. As of April 2023, 5.18 billion people worldwide use the Internet [ 1 ]. On average, global Internet users spend 6 h and 43 min online daily [ 2 ]. In 2023, social media platforms engage 4.8 billion users worldwide, a significant rise from 2.46 billion in 2017 [ 1 , 2 ]. These users spend an average of 2 h and 25 min on social networks each day and have, on average, 8.9 social media accounts [ 2 ]. Smartphones, now an essential device for many, are especially popular among the youth. Specifically, teenagers aged 14 to 24 access their phones approximately 214 times daily [ 3 ]. While social media networks have grown in importance, they also introduce challenges. Issues such as social media fatigue manifest in negative emotional responses like burnout, exhaustion, and frustration during social network activities [ 4 ]. Another possible negative consequence of social media activity is addictive behavior that is reported prior in the context of internet addiction.
Classification and definition of social media addiction
Digital media addictions, with a particular emphasis on social media addictions, are increasingly prevalent in psychotherapy, especially among younger demographics [ 5 , 6 ]. The concern for social media addiction is heightened among females, who show a higher propensity towards this addiction [ 7 , 8 ]. Despite its growing prevalence, social media addiction is yet to be fully acknowledged in diagnostic classification systems. The term “addiction” is therefore only used in this context for the sake of simplicity, as it is not yet officially recognized. The concept of ‘behavioral addiction,’ which characterizes excessive, rewarding behaviors leading to psychological addiction symptoms [ 9 ], is applicable here, though social media addiction still lacks distinct recognition in diagnostic manuals like the ICD and DSM. This gap highlights the need for more comprehensive research and understanding.
Prior research conforms mainly to differentiate between generalized and specific internet addictions [ 10 , 11 , 12 , 13 ]. The first means a multidimensional misuse of the internet using multiple internet functions, whereas the ladder aims a sole specific internet function (e.g., gaming, gambling, social media etc.) [ 13 , 14 ]. Social Media Addiction, encompassing variants like Facebook addiction and general addictive use of social networking sites (SNSs), is characterized as a maladaptive psychological dependency on SNSs, leading to behavioral addiction symptoms [ 15 , 16 , 17 ]. Currently, Social Media Addiction assessment relies on questionnaires like the Bergen Social Media Addiction Scale (BSMAS [ 18 ]),, which is momentarily the most widely used tool and applies criteria such as salience, mood modification, tolerance, withdrawal, conflict, and relapse [ 19 ] to evaluate addictive behaviors [ 10 ].
Prevalence rates and mental stress correlations of social media addiction
Data regarding the prevalence of social media addiction indicate a range between 1% and 18.7% [ 20 ]. However, the accuracy of these rates is debated. Cheng et al. [ 21 ] suggest that estimates of social media addiction are often either under- or overestimated. Their recent meta-analysis revealed prevalence rates ranging from 0 to 82%, a wide disparity stemming from differing theoretical frameworks and measurement instruments. Depending on the strictness of the classification system used, the researchers identified three mean prevalence benchmarks: 5%, 13%, and 25%. Frequently, individuals with problematic social media use also grapple with other mental health issues. Depression [ 20 , 22 ] and social anxiety [ 23 ] are commonly co-occurring disorders, as are challenges related to self-esteem (ibid.). Particularly, young women often feel dissatisfied with their bodies due to social media engagement. The frequent exposure to manipulated and idealized images of models or influencers fuels a comparison culture. As a result, many young women develop a desire to alter their appearance [ 24 ]. The number of “likes” they receive on platforms becomes a proxy for their self-worth, heavily influencing their self-esteem [ 25 ]. Several studies highlight that young adults spending over two hours daily on social media tend to exhibit higher rates of anxiety, depression, and sleep disturbances.
Personality traits and social media addiction
The personality trait neuroticism, and the “fear of missing out” or FOMO [ 26 ], have been identified as predictors of Social Media Addiction [ 27 ]. Conversely, extraversion’s link to social media use is debated. While some evidence suggests extraversion is not a significant factor [ 28 ], other research indicates extraverted individuals are more prone to social media use and potential addiction. Kuss & Griffiths [ 29 ] offer a more nuanced view in their literature review. According to them, extraverted individuals might use social media to augment their social interactions, i.e. they use social media in a positive manner to expand opportunities to interact with others in more ways. Introverted users, on the other hand, use social media to compensate for a perceived social deficit. For them, using social media is a way to connect with others in a way that they feel is not sufficiently possible in real life.
Attachment styles and social media addiction
Extensive research has been conducted on the association between insecure attachment and substance addictions [ 30 , 31 ]. The attachment system, which comprises secure, insecure, and disorganized categories, is a biologically and evolutionarily rooted motivational and behavioral system that operates through attachment figures [ 32 ]. Schuhler et al. [ 33 ] proposed a model elucidating the link between internet addiction and attachment, suggesting that addictive behaviors may arise as a means to compensate for attachment issues. From this perspective, digital addiction represents a flawed attempt to address early attachment deficiencies [ 33 , 34 ]. In a related vein, Brisch [ 35 ] introduced a model that positions the ‘reference object’ as central to the understanding of addictions. According to this model, the primary function of social media addiction isn’t to escape negative emotions, as is often the case with substance addictions. Instead, it’s seen as an excessive digitally-mediated social behavior aiming to substitute for insecure attachments. Supporting this, Eichenberg et al. [ 34 ] showed that insecure attachment style is correlated with problematic smartphone usage and problematic internet usage [ 36 ]. Notably, an ambivalently attached style was identified as particularly relevant in both contexts. A plethora of studies showed a link between social media addiction and attachment in general [ 37 , 38 , 39 , 40 , 41 , 42 , 43 ]. But the question arises whether the specific attachment style as has shown relevant for internet and smartphone addiction will also be prominent for social media addiction.
Research objectives and questions
The primary objective of this study is to explore whether an insecure attachment style correlates with addictive social media use, and to pinpoint which specific style is most relevant. While research has identified an ambivalent closed insecure attachment style as being significant in the context of internet and smartphone addiction, a detailed examination specific to social media addiction remains lacking.
Moreover, this study seeks to gather further information regarding the still emerging psychopathology, specifically focusing on the personality traits neuroticism and extraversion, as well as mental stress.
Mental health
The research questions will be, whether social media addicted students report higher levels of depression, anxiety, and somatization.
- Personality
Further, it will be explored whether neuroticism and extraversion influence an individual’s susceptibility to social media addiction.
Recruitment
A comprehensive survey ( N = 2846, response rate = 20.06%) was created with the SoSci Survey online survey tool [ 44 ] and was conducted among students at the Sigmund Freud PrivatUniversität in Vienna, Austria. The data collection took place from January to March 2021, resulting in a final sample of 571 respondents. To distribute the online questionnaire, the Study Service Centers from the faculties of psychology, psychotherapy, law, and medicine were approached. They were requested to email the link to the questionnaire, accompanied by a pre-written invitation text, to all actively enrolled students at the Sigmund Freud PrivatUniversität Vienna. Once the participants provided informed consent and completed the survey, they were redirected to a debriefing page. This page detailed the study’s objectives and offered the contact information of the researchers, in case the participants sought support related to the survey topics or had additional inquiries. The survey received approval from the Ethics Commission of the Faculty of Psychotherapy Science and the Faculty of Psychology of the Sigμund Freud University PrivatVienna. Recognizing the sensitive nature of the topic, paramount emphasis was placed on safeguarding the confidentiality of participants’ responses. Furthermore, participants had the liberty to opt out of the study at any juncture. Should they wish to have their data expunged, they could simply reach out to a researcher via email, referencing an unique anonymized code. This would enable the researcher to identify and delete the participant’s anonymized data.
Survey structure
The survey, created using Sosci-Survey, began with a brief that outlined the research rationale and the survey’s objectives. Participants affirmed their agreement with the study’s privacy policy through a checkbox.
Section 1 asked about socio-demographic factors, including age, gender, and study subject. Subsequently, it touched upon matters related to social media:
Services most used : Participants identified which social media services they frequently use, answered dichotomously (yes/no).
Usage frequency : Choices ranged from “less than 30 minutes” to “more than four hours per day” on a seven-point scale.
Social Media Importance : Participants rated from “very significant” to “not significant” on a four-point scale.
Purposes of Use : Employing a five-point scale, respondents indicated frequency, ranging from 1 (“never”) to 5 (“several times a day”).
Perceived downsides : Participants assessed their sentiments on a five-point scale from 1 (“not true at all”) to 5 (“completely true”).
In light of evidence suggesting a discrepancy between objective and self-reported usage time—where users often overestimate their screen time [ 45 ]—the survey did not deploy open-ended questions concerning usage duration. Instead, participants were presented with predefined categories to streamline their responses.
Section 2 incorporated standardized questionnaires to examine further social media addiction, mental distress, personality traits, and attachment styles.
Bergen social media addiction scale BSMAS [ 18 ]
The Bergen Social Media Addiction Scale (BSMAS) [ 18 ] categorizes users into two groups: those addicted to social media and those not addicted. All six items pertain to one’s experience with social media over the past 12 months. It employs a five-point scale, ranging from 1 (“very rarely”) to 5 (“very often”). The scale asks at the beginning of each item “How often during the last year have you…” and continues with “…spent a lot of time thinking about social media or planned use of social media?” (i.e., salience) or “…become restless or troubled if you have been prohibited from using social media?” (i.e., withdrawal). A higher BSMAS score indicates a heightened risk of social media addiction. As suggested by a substantial Hungarian study involving 6000 adolescents [ 20 ], a cutoff score of 19 out of 30 was adopted. The scale was repeatedly reported with high internal consistency, e.g., α = 0.97 [ 46 ] and α = 0.82 (at baseline) plus α = 0.86 (at follow-up) [ 10 ]. Chen et al. [ 10 ] confirm the single-factor structure of the scale, report only medium correlations with scales close to the construct (SABAS/smartphone addiction, IGDS-SF9/internet gaming disorder, r =.06 and 0.42), and showed invariance across three months among young adults. They presented a good test–retest reliability after three months ( ICC = 0.86, p <.001).
Brief symptom inventory BSI-18 [ 47 ]
The BSI-18 is a brief, reliable instrument for assessing mental stress. It contains the three subscales somatization, depression, and anxiety, comprising 6 items, as well as the Global Severity Index (GSI) including all 18 items. Response format of the 18 items is a five-point scale (0=”not at all” to 4=”very strong”). The scale asks at the beginning of a symptoms list: “How much have you had within the past 7 days…”. Examples for the symptoms on this list are “Nausea or upset stomach” for somatization, “Feelings of worthlessness” for depression”, and “Spells of terror or panic” for anxiety. The BSI-18 is the newest and shortest of the multidimensional versions of the Symptom Checklist 90-R. The BSI-18 assesses validly mental stress in both normal population [ 48 ] and clinical populations [ 49 ]. Confirmatory analyses confirm the three-factor structure [ 48 ]. Franke et al. [ 49 ] report good internal consistencies of the scales fear of rejection (BSI-18 (α (somatization) = 0,79, α (depression) = 0,84, α (anxiety) = 0,84, α (GSI) = 0,91).
Big five inventory BFI-10 [ 50 ]
The questionnaire is based on the Big Five personality traits model, also called OCEAN model that is the most widely used model for describing overall personality [ 51 ]. Theoretical background is the sedimentation hypothesis that assumes that every personality trait must be represented in language and, therefore, factor analyses were used to find universal personality dimensions [ 52 ]. Multiple analyses by various researchers resulted repeatedly in the OCEAN model, which consists of the five dimensions agreeableness, neuroticism, conscientiousness, openness to experience, and extraversion. The BFI-10 [ 50 ] contains 10 items, two for each of the five dimensions. The scale asks, “How well do the following statements describe your personality?” and starts a list of attitudes with “I see myself as someone who…“. Example answers are: “…does a thorough job” (i.e., conscientiousness) or “…is outgoing, sociable” (i.e., extraversion). Respondents answered a five-point rating scale from “does not apply at all” (1) to “applies completely” (5) for each item. Rammstedt und John [ 50 ] report moderate test–retest reliability after 6 weeks in a student sample (agreeableness: rtt = 0.58, neuroticism: rtt = 0.74, conscientiousness: rtt = 0.77, openness to experience: rtt = 0.72, extraversion: rtt = 0.84). In a representative sample, however, the retest coefficients are lower overall ranging from ( rtt =.62) for openness to experience to ( rtt =.49) for neuroticism [ 51 ]. Rammstedt et al. [ 51 ] report sufficient construct validity correlating the BFI-10 with the NEO-PI-R and factorial validity by conducting principal component analyses on a representative sample.
Bielefeld questionnaire on partnership expectations BFPE [ 53 ]
The BFPE operationalizes attachment styles of adults by recording self-reports on three scales: conscious need for care (8 items), fear of rejection (11 items), and readiness for self-disclosure (11 items) [ 53 ]. Example items are: “Knowing myself as I do, I can hardly imagine that my partner will appreciate me” (i.e., fear of rejection), “I prefer to talk with my partner about facts rather than about feelings” (i.e., readiness for self- disclosure), and “It’s important for me that my partner thinks of me often, even when we are not together” (i.e., conscious need for care). The first of the 31 items serves as an icebreaker item and is not evaluated. The degree of expression of each item is indicated on a 5-point scale (1= “does not apply at all” to 5 = “applies exactly”). From the aggregate scores of these scales, one of five attachment styles can be determined: secure, two variations of ambivalent/anxious (closed and clinging), and two variations of the avoidant style (closed and conditionally secure). For simplification purposes, these styles can be dichotomized into two primary categories: secure (which includes both secure and conditionally secure types) and insecure (encompassing avoidant-closed, ambivalent-clingy, and ambivalent-closed types). These distinct attachment styles emerged originally from cluster analysis research [ 53 ]. Höger and Buschkämper [ 53 ] report good internal consistencies of the scales fear of rejection (Cronbach’s α = 0.88), readiness for self-disclosure (Cronbach’s α = 0.89), and conscious need for care (Cronbach’s α = 0.77). The split-half reliabilities calculated according to Guttman and Spearman-Brown are also similarly good for the three scales (fear of rejection = 0.91, readiness for self-disclosure = 0.89, and conscious need for care = 0.77). A validation is based on a German translation of the “Adult Attachment Scale” (AAS [ 54 ]),.
Statistical analysis
The Statistical Package for the Social Sciences Program (SPSS version 27) was used for data input, processing, and statistical analyses. The participants were divided into social media addicted (SMA) and not addicted (NSMA) using the cut-off score according to Bányai et al. [ 20 ]. Additionally, the percentage of social media dependent students has been calculated. To evaluate differences between SMA and NSMA in social media usage, Mann-Whitney U tests for two independent samples were analyzed for differences in downsides of social media and usage purposes, and chi-square tests for differences in social media services, usage frequency, and social media importance, as the corresponding data were not normally distributed. Based on the data obtained with the BFPE, participants were allocated (see above) to the five attachment styles “secure,” “conditionally secure,” “ambivalent clingy,” “ambivalent closed,” and “avoidant closed.” Subsequently, the five attachment styles were dichotomized into the variables “secure” and “insecure” attachment styles. Subsequently, the five attachment styles were dichotomized into the variables “secure” and “insecure” attachment styles. Finally, using the chi-square tests, attachment styles and social media addiction were tested for significance differences. While chi-square tests provide valuable insights into individual associations, a two-step cluster analysis was conducted to gain a comprehensive understanding of how these variables collectively group participants. Two-step cluster analysis was chosen due to its capacity to handle both continuous and categorical variables. The number of clusters was determined based on the Schwarz Bayesian Criterion (BIC), and the selected model was further validated by examining the silhouette measure of cohesion and separation. Since gender and age are variables that could influence social media addiction, they were included in the cluster analysis to investigate their distribution over the resulting clusters. To maintain robustness of analyses, the non-binary gender category was omitted due to very small case number.
The total sample ( N = 571) consisted of 65.5% female students ( n = 374) 33.3% male students ( n = 190), and 1.2% those who did not wish to be defined by these two genders ( n = 7). Participants were between 18 and 60 years old ( M = 23.61 years, SD = 5.00, median = 23, modus = 22). The distribution of study subject was the following: medicine ( n = 344, 59.7%), psychology ( n = 121, 21.0%), psychotherapy ( n = 79, 13.7%), and law ( n = 32, 5.6%) (some students studied two subjects).
- Social media addiction
A total of 131 people (22.7% of the total sample) could be classified as addicted to social media. In addition, it was also relevant how genders were distributed between the two groups. Of the total number of participants classified as addicted participants ( N = 131), 79.39% were female, 19.08% male, and 1.53% non-binary. These values are to be contrasted with the group of not addicted ( N = 440), in which 61.36% were female, 37.5% male, and 1.14% non-binary.
Social media usage
Among the various social media platforms, “WhatsApp” was the predominant choice with 99.1% usage. It was trailed by “YouTube” at 91.2%, “Instagram” at 82.1%, “Facebook” at 66.9%, “Snapchat” at 63.7%, “Facebook Messenger” at 35.6%, “Pinterest” at 32.9%, and “Twitter” at 10.5%. In addressing frequency of use, a significant 91% indicated they access social media multiple times per day. Delving into the duration of daily usage: 12.8% were on for less than an hour, 25.6% used it for around an hour, 32.7% for two hours, 16.8% for three hours, and 12.1% devoted more than three hours. When participants were asked about the significance of social media, 8.9% viewed it as very important, 55.1% as important, 31.3% as less important, and a mere 4.7% as not important. Participants predominantly engaged with social media for “entertainment” ( M = 4.17, SD = 1.05), staying “up to date” ( M = 4.12, SD = 1.03), combating “boredom” ( M = 3.94, SD = 1.22), maintaining “contact with family” ( M = 3.86, SD = 1.2), and for “music” ( M = 3.55, SD = 1.4). They also sought “inspiration (e.g., fashion, interior)” with a mean score of ( M = 3.35, SD = 1.29). However, not all experiences were positive. Downsides associated with social media usage were led by “comparison with others” ( M = 3.19, SD = 1.3), followed by “dissatisfaction with own body” ( M = 2.55, SD = 1.38), “negative self-esteem in contact with influencers” ( M = 2.23, SD = 1.32), and encountering “insults, intrusive behavior” ( M = 1.88, SD = 1.3). Distinguishing between SMA and NSMA users, differences emerged in their consumption patterns (see for details Table 1 ). SMA users predominantly gravitated towards image-centric platforms such as “Instagram” (93.1% SMA vs. 78.9% NSMA) and “Pinterest” (46.6% SMA vs. 28.9% NSMA). Remarkably, SMA users expressed heightened concerns regarding the downsides “comparison with others” ( M = 4.06, SD = 1.03 for SMA vs. M = 2.94, SD = 1.26 for NSMA), “dissatisfaction with own body (when viewing idealized bodies online)” (M = 3.45, SD = 1.34 for SMA vs. M = 2.28, SD = 1.28 for NSMA), and “negative self-esteem in contact with influencers” ( M = 3.16, SD = 1.34 for SMA vs. M = 1.95, SD = 1.18 for NSMA). It became evident that SMA users faced enhanced negative repercussions, especially in terms of body perception when comparing themselves with images of others. In addition, SMA use social media as tool for more purposes than NSMA. Not addicted report here, to use social media only for contact with family and music equally often.
Attachment style
Since 12 participants did not completely fill in the BFPE, the number of participants regarding attachment is 559. Frequencies and percentages of each attachment style can be seen in Table 2 . A small part of the student population was securely bound ( n = 88, 15.7%) with the biggest part being insecurely bound ( n = 471, 84.3%). Secure attachment style (corrected residuals: 3.1) is related to a disproportionately higher number of NSMA and insecure attachment style (corrected residuals: 3.1) is related to a disproportionately higher number of SMA, χ² (1) = 9.28, p = .002, C = 0.13 (see Fig. 1 , see Table 3 ). The five individual attachment styles differ in the frequency distribution of social media addiction, χ² (4) = 30.75, p < .001, C = 0.24, with avoidant closed (corrected residuals:3.2) having disproportionately more NSMA, ambivalent closed (corrected residuals: 4.8) having disproportionately more SMA, and conditionally secure (corrected residuals: 2.4) having disproportionately more NSMA (see Fig. 2 ). So, findings show that participants with social media addiction had a significant higher likelihood to have an ambivalent closed attachment style.
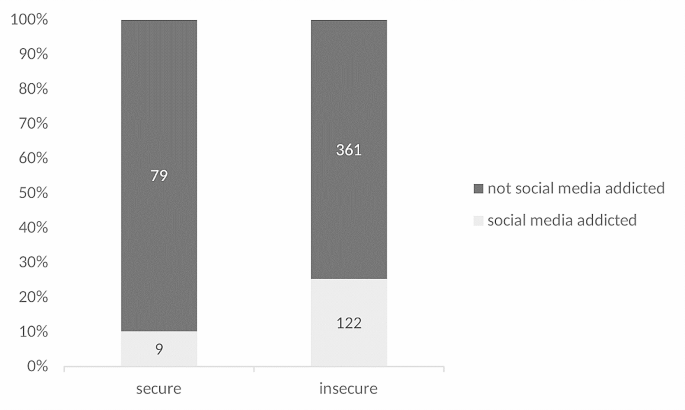
Relationship between attachment style and social media addiction. This stacked bar chart depicts the proportion of participants with ‘secure’ and ‘insecure’ attachment styles as determined by the Bielefeld Questionnaire on Partnership Expectations (BFPE). Attachment styles are defined by responses to three scales: conscious need for care, fear of rejection, and readiness for self-disclosure. These styles are subsequently dichotomized into ‘secure’ (including secure and conditionally secure styles) and ‘insecure’ (including avoidant-closed, ambivalent-clingy, and ambivalent-closed styles). Dark gray bars represent participants not addicted to social media, while light gray bars represent those with a self-reported addiction determined by the Bergen Social Media Addiction Scale (BSMAS). The numbers within the bars indicate the count of participants in each category
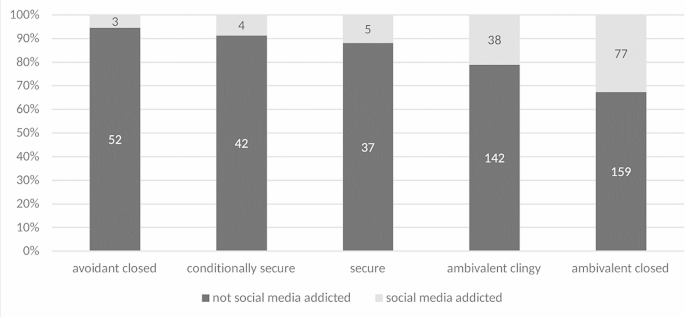
Distribution of five attachment styles and social media addiction. This bar chart visualizes the proportion of participants classified into five distinct attachment styles according to the Bielefeld Questionnaire on Partnership Expectations (BFPE) alongside their social media addiction status, as measured by the Bergen Social Media Addiction Scale (BSMAS). The attachment styles represented are ‘avoidant closed’, ‘conditionally secure’, ‘secure’, ‘ambivalent clingy’, and ‘ambivalent closed’. Dark gray bars indicate participants not identified as addicted to social media, while light gray bars represent those who meet the criteria for addiction according to the BSMAS. The numbers within the bars denote the count of participants corresponding to each category
Regarding extraversion, the total sample ( M = 3.58, SD = 0.92, modus = 5, Md = 3.5) is slightly but significantly less open-minded than a norm sample having same age and education ( M = 3.93, SD = 0.83, Rammstedt et al. 2012) ( t (570)=-9.23, p < .001) and regarding neuroticism, the sample ( M = 3.09, SD = 0.87, modus = 2.5, Md = 3) is significantly more neurotic than a comparable norm sample ( M = 2.25, SD = 0.69, Rammstedt et al. 2012) ( t (570) = 23.15, p < .001). Further, it was found that SMA ( M = 3.40, SD = 0.85) scored significantly higher than NSMA ( M = 3.00, SD = 0.85) on the dimension of neuroticism and thus could be classified as more emotionally unstable ( U = 20636.50, Z = -5.02, p < .001). However, on the dimension of extraversion, SMA ( M = 3.56, SD = 0.85) did not differ from NSMA ( M = 3.58, SD = 0.94) ( U = 28408.5, Z = − 0.25, p = .801).
- Mental distress
The total sample showed in comparison with a norm sample high levels of each of the three dimensions of depression ( M = 4.18; SD = 4.52 vs. M norm =1.27; SD norm =2.5, Franke et al. 2017) ( t (570) = 15.40, p < .001), anxiety ( M = 3.67; SD = 4.30, vs. M norm =1.09; SD norm =2.1, ibd.) ( t (570) = 14.35, p < .001), and somatization ( M = 2.23, SD = 3.00, vs. M norm =0.70; SD norm =14.8, ibd.) ( t (570) = 12.18, p < .001). Moreover, SMA reported still higher scores on all three scales of the BSI-18: depression (SMA M = 7.93, SD = 5.25, NSMA M = 3.06, SD = 3.59) ( U = 11,606, Z = -10.47, p < .001), anxiety (SMA M = 6.18, SD = 5.34, NSMA M = 2.92, SD = 3.61) ( U = 16,841, Z = -7.31, p < .001), and somatization (SMA M = 3.60, SD = 4.02, NSMA M = 1.82, SD = 2.48) ( U = 19,730, Z = -5.64, p < .001) than NSMA. Spitzer et al. (2011) reported BSI-18 patient scores relatively close to SMA scores for depression (mean scores ranging from 6.17 to 11.61) and anxiety (mean scores ranging from 6.26 to 9.51), but not for somatization (mean scores ranging from 6.47 to 6.90). It can therefore be assumed that students in this sample are generally more mentally stressed, with students who are addicted to social media being particularly mentally stressed. This finding could be explained due to the high distress and burden in the early phase of the COVID19 pandemic.
Two-step cluster analysis
The two-step cluster analysis suggested a three-cluster solution as the most appropriate fit. Evaluation of the centroids of continuous variables (Table 4 ) and frequencies of the categorical cluster composition (Table 5 ) result in the following clusters:
The Cluster ambivalent clingy attachment (ACA) ( N = 178) is relatively balanced in terms of extraversion, neuroticism, depression, anxiety, and somatization. They are uniquely characterized by the ambivalent clingy attachment style with a balanced representation of social media dependence.
The Cluster secure attachment (SA) ( N = 140) is characterized by individuals who are slightly extroverted, less neurotic, and show lower levels of depression, anxiety, and somatization. This cluster stands out due to its representation of secure and rather secure attachment styles and has the lowest proportion of individuals who are addicted to social media.
The Cluster ambivalent closed attachment (AVA) ( N = 231) is slightly introverted, more neurotic, and exhibits higher levels of depression and anxiety. Participants of this cluster are exclusively of the ambivalent closed attachment style, and a significant portion seems more susceptible to social media addiction.
For the validation of the derived clustering solution, the Bayesian Information Criterion (BIC) was employed as a model selection criterion to identify the optimal number of clusters. The BIC is advantageous in balancing the goodness of fit of the model against its complexity, penalizing models with more parameters to avoid overfitting. Various numbers of clusters were considered, ranging from 1 to 15, and the corresponding BIC values were calculated for each cluster solution. Table 6 presents the BIC values obtained for different cluster solutions. The BIC drops substantially from 1 cluster to 2 clusters, indicated by a change of -1180.384. There is a smaller but still notable drop from 2 clusters to 3 clusters, with a change of -605.464. After 3 clusters, the BIC drops more slowly, with smaller changes for each additional cluster. Even if the ratio for the change from 2 to 3 clusters is 0.512, the ratio of distance measures that indicates how distinct the clusters are from each other is for the 3-cluster solution still 1.780, which suggests that the 3-cluster solution is equally well-defined compared to the 2-cluster solution. Given this information, we opt for the 3-cluster solution, since the BIC drops more slowly beyond this point, suggesting diminishing returns in terms of model fit as more clusters are added and the 3-cluster solution offers a sufficient granular segmentation. The distribution of age (Table 4 ) and gender (Table 5 ) was relatively even.
Principal results
This study aimed to examine social media addiction with a focus on differences in attachment style, mental distress, and personality between students with and without social media addiction. For personality, it was shown that SMA had significantly higher values on the neuroticism dimension than NSMA, but they did not differ in the extraversion dimension. Thus, SMA can be classified as more emotionally unstable in comparison with NSMA. Further, SMA scored significantly higher on all three levels—depressiveness, anxiety, and somatization—than the group of NSMA, i.e., social media addicted users are comparatively more mentally stressed. At least for attachment style, the assumption that SMA are more likely to show an insecure attachment was confirmed here. In more detail, most SMA displayed an ambivalent closed attachment style. Two-step cluster analysis yielded a holistic insight into the collective grouping of cases by these variables. It corroborated the findings of the univariate analyses, revealing three predominant clusters, chiefly characterized by three attachment styles and varying levels of social media addiction: (a) secure attachment, predominantly associated with fewer instances of social media addiction and lower prevalence of mental health problems; (b) ambivalent closed attachment, typically marked by a higher frequency of social media addiction and elevated levels of mental health problems; and (c) ambivalent clingy attachment, presenting a moderate incidence of social media addiction and a relatively balanced mental health profile.
Prevalence rate of social media addiction (22.8%) lies within the literature reported prevalence of the used instrument (BSMAS), since Chen et al. [ 10 ] specify < 10–40% for the BSMAS. SMA differ from NSMA in their usage of social media, exhibiting higher values in usage frequency, time spent, and perceived importance. Notably, SMA are more active on image-oriented services such as “Instagram” and “Pinterest”. They also report higher levels of “comparison with others”, “dissatisfaction with their own body (especially when exposed to idealized online images)”, and “negative self-esteem when interacting with influencers”. This suggests that SMA may experience heightened negative body awareness when comparing themselves to online images. Moreover, SMA use social media for a broader range of purposes compared to NSMA.
SMA scored significantly higher on the neuroticism dimension than NSMA, suggesting that they tend to be more emotionally unstable and easily irritable. Conversely, no difference was observed in the extraversion dimension. Previous research supports the idea that internet-related addictions are linked to higher scores on the neuroticism dimension. Blackwell et al. [ 27 ] demonstrated that neuroticism predicts social media use. Moreover, a study by Müller [ 55 ] suggests that Internet addiction correlates with increased neuroticism scores. Interestingly, individuals with elevated neuroticism scores associate Internet topics with significantly stronger positive arousal compared to a healthy control group [ 56 ]. Social media addiction has also been positively linked to neuroticism [ 27 , 28 ], and individuals scoring high on this trait are drawn to social networks as they offer recognition and validation [ 27 ]. Marengo et al. [ 28 ] align with our findings by not observing a relationship between social media addiction and extraversion. The contrasting findings presented by Kuss and Griffiths [ 29 ] relate extraversion more to older individuals and openness more to younger ones. Given our primary focus on younger participants, our results are consistent with these observations.
SMA display significantly higher values for depression, anxiety, and somatization compared to NSMA, even considering the evident distress in the overall sample. This suggests that SMA may be mentally more strained than NSMA. Consequently, further evidence for the connection between mental disorders and internet-related addictions in terms of comorbidity was found in the present study. This augments the extant research on depression, anxiety, and internet addiction. Kırcaburun [ 57 ] also identified a significant positive relationship between depressive symptoms, internet use, and social media addiction. In his study, the level of depression in adolescents was indirectly influenced by social media addiction; addicts spent more time online, amplifying the risk of depressive symptoms. Similarly, Wu et al. [ 58 ] found that internet addiction correlates with depression in adolescents, exerting direct, mediated, and moderating effects on depression levels. For anxiety, there’s also documented evidence of a positive association with problematic social media consumption. Baltaci [ 23 ] highlighted social anxiety as a predictor for social media addiction among university students. Other studies have shown a positive correlation between internet addiction and general anxiety levels in students [ 59 , 60 ]. As for somatization, there’s a documented positive correlation with internet addiction in adolescents [ 61 , 62 , 63 ]. Research on somatization and smartphone addiction is somewhat limited [ 63 ]. Results here confirm the positive correlation adding to this research corpus also heightened somatic symptomatology in social media addicted students.
Users with an insecure attachment style are significantly more likely to exhibit social media addiction than those with a secure attachment style. These findings align with a substantial body of research that establishes a connection between insecure attachment styles and internet-related addictions. A systematic review has provided evidence linking insecure attachment styles with both internet addiction in general and social media addiction in particular [ 64 ]. Moreover, certain studies suggest that difficulties in relational behavior or the presence of insecure attachment styles can act as risk factors for smartphone addiction. For instance, Baek et al. [ 65 ] identified a correlation between attachment behavior (specifically internalization problems) and smartphone usage. Other research [ 66 , 67 ] has indicated a mediating effect of attachment style on smartphone addiction. Anxiously attached individuals showed patterns of self-regulation that directly influenced their susceptibility to smartphone addiction. While a secure attachment style offered a protective effect, an anxious attachment style increased vulnerability to addiction. In contrast, an avoidant attachment style didn’t significantly influence addiction development.
For social media addiction, several studies have highlighted its relationship with attachment. For instance, Hart et al. [ 37 ] demonstrated a link between dysfunctional attachment qualities and problematic social media use. A study involving Turkish students revealed that insecure attachment styles might serve as risk factors for social media addiction [ 38 ]. Conversely, secure attachment and high self-esteem can act as protective factors against such addiction [ 38 ]. Numerous studies have established a connection between an anxious attachment style and both heavy social media use [ 39 , 40 , 41 ] and addiction to it [ 42 ]. Specifically, Yaakobi and Goldenberg [ 43 ] identified a positive correlation between an anxious attachment style and the amount of time spent on social media. This same study found that an anxious attachment style negatively predicts the number of online friends. Oldmeadow et al. [ 41 ] also discovered a relationship between anxious attachment and seeking comfort on Facebook, noting an increase in Facebook usage, especially during negative emotional states.
Currently, no studies explore the relationship between an ambivalent closed attachment style and social media addiction. However, the findings in this study indicate that an ambivalent closed attachment style is significantly associated with social media addiction more frequently. These results are consistent with previous data suggesting this style is prevalent for internet-related addictions, as observed in the context of both smartphone [ 34 ] and internet [ 36 ] addictions. According to Höger and Buschkämper [ 53 ], individuals with an ambivalent attachment style exhibit an increased need for attention and concurrently face heightened acceptance issues. This pattern suggests heightened anxiety and a secondary hyperactivating (ambivalent) strategy (ibid.). It’s plausible that the social-compensatory component is particularly influential in this context when it comes to social media [ 34 ]. Individuals with an ambivalent-closed attachment style might turn to online platforms, especially social media, to mitigate their interpersonal relationship deficits (ibid.). The anonymity afforded by the internet allows a new representation of the self to be created, helping this group to compensate for feared problems of acceptance (ibid.). Based on the data, it appears this new representation of the self is often facilitated through image-focused platforms like “Instagram” and “Pinterest”. However, this may inadvertently expose SMA users to the pitfalls of social media, such as body dissatisfaction and reduced self-esteem when interacting with influencers. This dynamic could exacerbate their acceptance issues, perpetuating a detrimental cycle.
The ambivalent clinging and closed attachment styles differ primarily in their perceived willingness to open up. The former demonstrates a moderate willingness, allowing for the expression of strong attachment needs associated with the hyperactivated attachment system, while the latter exhibits a notably low willingness to open [ 53 ]. The findings presented in this study indicate that the degree of openness (for attachment) may play a crucial role in determining the severity of problematic user behavior. Specifically, the more receptive a user is to attachment, the less likely they are to exhibit addictive behaviors. Cluster analysis supports this interpretation. It identified three clusters with varying susceptibilities to social media addiction: those with secure attachment exhibit the lowest likelihood, those with ambivalent clingy attachment have a medium likelihood, and those with ambivalent closed attachment display the highest likelihood. This potential correlation warrants further exploration in subsequent research. Moreover, given that a mediating effect of mentalization between attachment style and both emotion dysregulation [ 68 ] and psychopathology [ 69 ] has been demonstrated, future research should delve deeper into exploring the relationships between mentalization, attachment style, and internet-related addictions.
Limitations
It should be noted that the data are based on self-reporting in an online survey. Response rate is comparable with other online-survey studies [ 70 ]. So, possible self-selection processes could be of importance since online surveys are prone to an inherent selection bias. Social media users may find it appealing to participate for trying to relativize the negative image of social media addiction. Further, the sample is due to the narrow age distribution and educational level not representative. Even if cluster analysis shows no noteworthy age distribution for the clusters, future research should collect sufficient case number for each age group or limit age to a homogenous group. Female students contributed disproportionately here. Which in turn can affect the prevalence of social media addiction since there is evidence that women are more prone to social media addiction [ 8 ]. Though, this gender bias has been frequently observed in online surveys [ 71 ]. Cluster analysis did not reveal any conspicuous distribution for gender either. Altogether, future studies with a broader recruitment strategy may provide more representative data and confirm discussed results. Further, it could be discussed that the design of the study is cross-sectional. Since there is evidence for differences in age, at least for personality dimensions, comparison of two points in time or more can corroborate data or reduce it to differences in generation cohort. Furthermore, since mental health is a key variable, future studies should check psychiatric history of participants.
This study enhances our understanding of how specific attachment problems could contribute to the development of social media addiction, reaffirming findings related to internet and smartphone addiction. It reveals that an avoidant closed attachment style, characterized by a pronounced need for attention, acceptance issues, and notably low openness for attachment, is frequently associated with this addiction. Such a deficit in openness may prompt compensatory behavior to satiate the intensive need for attention in the manageable environment of the digital world, where any conversation can be terminated with a click. This intense attention-seeking behavior seems to find satisfaction through image-centric services on social media, instigating negative comparative processes with others and potentially reinforcing acceptance issues in a self-perpetuating cycle, with mental stress being a substantial correlate.
To break this cycle, therapeutic interventions should consider these interrelations and specifically target critical areas. This could include conducting a thorough media anamnesis, educating about the effects of image-focused services and comparative processes, and establishing a robust and consistent therapeutic alliance—a cornerstone of successful addiction treatment [ 34 ]. The incorporation of attachment-oriented strategies is vital, as attachment-related aspects have yet to be integrated into existing internet addiction treatment protocols [ 34 , 36 ]. In addition, since research showed a good impact of whole school attachment-based interventions [ 72 ], prevention programs to combat digital addictions in schools and universities should also include content that promotes secure attachment behavior, especially to young people with a high need for attention, acceptance issues, and notably low openness for attachment. Beyond individual treatment, the implementation of these strategies has the potential to foster a healthier approach to digital media usage across society, thereby contributing to a more informed and mindful engagement with social media platforms, which can finally lead to a reduction in the prevalence and impact of social media addiction on a broader scale.
Data availability
The raw data supporting the conclusions of this article will be made available by the authors, without undue reservation.
Abbreviations
Big Five Inventory
Bielefelder Fragebogen zu Partnerschaftserwartungen
Brief Symptom Inventory
Bergen Social Media Addiction Scale
Not Social Media Addicted
Social Media Addicted
Statista. digital-population-worldwide. 2023. www.statista.com/statistics/617136/digital-population-worldwide . Accessed 14 Jun 2023.
Ahlgren M. 100 + Internet-Statistiken und Fakten zu 2022. 2022. www.websiterating.com/de/research/internet-statistics-facts/#references . Accessed 31 Aug 2022.
Scholz M, Studie. Wir nutzen unsere Smartphones 1.500 Mal pro Woche. mobile zeitgeist (We use our smartphones 1.500 times a week. Mobile zeitgeist). 2017. www.mobile-zeitgeist.com/studie-wir-nutzen-unsere-smartphones-1-500-mal-pro-woche/ . Accessed 18 Nov 2022.
Zheng H, Ling R. Drivers of social media fatigue: a systematic review. Telematics Inform. 2021;64:101696.
Article Google Scholar
Kapus K, Nyulas R, Nemeskeri Z, Zadori I, Muity G, Kiss J, et al. Prevalence and risk factors of internet addiction among Hungarian High School Students. IJERPH. 2021;18:6989.
Article PubMed PubMed Central Google Scholar
Rumpf HJ, Meyer C, Kreuzer A, John U, Vermulst A, Merkeerk G-J. Prävalenz der Internetabhängigkeit (PINTA). Bericht an das Bundesministerium für Gesundheit (prevalence of internet addiction (PINTA). Report to the federal ministry of health). 2011.
Baloğlu M, Şahin R, Arpaci I. A review of recent research in problematic internet use: gender and cultural differences. Curr Opin Psychol. 2020;36:124–9.
Article PubMed Google Scholar
Bischof G, Bischof A, Meyer C, John U, Rumpf HJ. Prävalenz Der Internetabhängigkeit– Diagnostik und Risikoprofile (PINTA-DIARI). Kompaktbericht an das Bundesministerium für Gesundheit (Prevalence of Internet Addiction - Diagnostics and Risk profiles (PINTA-DIARI). Compact report to the Federal Ministry of Health). Lübeck; 2013.
Grüßer-Sinopoli SM, Thalemann CN, Verhaltenssucht. Diagnostik, Therapie, Forschung. 1. Aufl. Bern: Huber; 2006.
Chen I-H, Strong C, Lin Y-C, Tsai M-C, Leung H, Lin C-Y, et al. Time invariance of three ultra-brief internet-related instruments: Smartphone Application-based addiction scale (SABAS), Bergen Social Media Addiction Scale (BSMAS), and the nine-item internet gaming disorder scale- short form (IGDS-SF9) (Study Part B). Addict Behav. 2020;101:105960.
Montag C, Bey K, Sha P, Li M, Chen Y, Liu W, et al. Is it meaningful to distinguish between generalized and specific internet addiction? Evidence from a cross-cultural study from G ermany, S weden, T aiwan and C hina. Asia-Pacific Psychiatry. 2015;7:20–6.
Brand M, Young KS, Laier C. Prefrontal control and internet addiction: a theoretical model and review of neuropsychological and neuroimaging findings. Front Hum Neurosci. 2014;8.
Griffiths M, Pontes H, Kuss D. Clinical psychology of internet addiction: a review of its conceptualization, prevalence, neuronal processes, and implications for treatment. NAN. 2015;11.
Davis RA. A cognitive-behavioral model of pathological internet use. Comput Hum Behav. 2001;17:187–95.
Cao X, Gong M, Yu L, Dai B. Exploring the mechanism of social media addiction: an empirical study from WeChat users. Internet Res. 2020;30:1305–28.
Chen A. From attachment to addiction: the mediating role of need satisfaction on social networking sites. Comput Hum Behav. 2019;98:80–92.
Turel O, Serenko A. The benefits and dangers of enjoyment with social networking websites. Eur J Inform Syst. 2012;21:512–28.
Andreassen CS, Billieux J, Griffiths MD, Kuss DJ, Demetrovics Z, Mazzoni E, et al. The relationship between addictive use of social media and video games and symptoms of psychiatric disorders: a large-scale cross-sectional study. Psychol Addict Behav. 2016;30:252–62.
Griffiths M. A ‘components’ model of addiction within a biopsychosocial framework. J Subst Use. 2005;10:191–7.
Bányai F, Zsila Á, Király O, Maraz A, Elekes Z, Griffiths MD, et al. Problematic social media use: results from a large-scale nationally representative adolescent sample. PLoS ONE. 2017;12:e0169839.
Cheng C, Lau YC, Chan L, Luk JW. Prevalence of social media addiction across 32 nations: meta-analysis with subgroup analysis of classification schemes and cultural values. Addict Behav. 2021;117:106845. https://doi.org/10.1016/j.addbeh.2021.106845 .
Pantic I. Online social networking and mental health. Cyberpsychology Behav Social Netw. 2014;17:652–7.
Ahi Evran University, Baltacı Ö. The predictive relationships between the social media addiction and social anxiety, loneliness, and happiness. IJPE. 2019;15:73–82.
Status of mind. Social media and young people ́s mental health. 2017.
Tiggemann M, Hayden S, Brown Z, Veldhuis J. The effect of Instagram likes on women’s social comparison and body dissatisfaction. Body Image. 2018;26:90–7.
Milyavskaya M, Saffran M, Hope N, Koestner R. Fear of missing out: prevalence, dynamics, and consequences of experiencing FOMO. Motiv Emot. 2018;42:725–37.
Blackwell D, Leaman C, Tramposch R, Osborne C, Liss M. Extraversion, neuroticism, attachment style and fear of missing out as predictors of social media use and addiction. Pers Indiv Differ. 2017;116:69–72.
Marengo D, Poletti I, Settanni M. The interplay between neuroticism, extraversion, and social media addiction in young adult Facebook users: testing the mediating role of online activity using objective data. Addict Behav. 2020;102:106150.
Kuss DJ, Griffiths MD. Online social networking and addiction—a review of the psychological literature. IJERPH. 2011;8:3528–52.
Borhani Y. Substance abuse and Insecure attachment styles: a relational study. lux. 2013;2:1–13.
Unterrainer HF, Hiebler-Ragger M, Rogen L, Kapfhammer HP. Sucht als Bindungsstörung. Nervenarzt. 2018;89:1043–8.
Article CAS PubMed Google Scholar
Ainsworth MDS. The Bowlby-Ainsworth attachment theory. Behav Brain Sci. 1978;1:436–8.
Schuhler P, Vogelgesang M, Petry J. Pathologischer PC-/Internetgebrauch: Krankheitsmodell, diagnostische und therapeutische Ansätze. Psychotherapeut. 2009;54:187–92.
Eichenberg C, Schott M, Schroiff A. Comparison of students with and without problematic smartphone use in light of attachment style. Front Psychiatry. 2019;10:681.
Brisch KH. Bindungsstörungen: von der Bindungstheorie zur Therapie. 17. Auflage. Stuttgart: Klett-Cotta; 2020.
Eichenberg C, Schott M, Decker O, Sindelar B. Attachment style and internet addiction: an online survey. J Med Internet Res. 2017;19:e170.
Hart J, Nailling E, Bizer GY, Collins CK. Attachment theory as a framework for explaining engagement with Facebook. Pers Indiv Differ. 2015;77:33–40.
Demircioğlu ZI, Köse AG. Mediating effects of self-esteem in the relationship between attachment styles and social media addiction among university students. 2020.
Jenkins-Guarnieri MA, Wright SL, Hudiburgh LM. The relationships among attachment style, personality traits, interpersonal competency, and Facebook use. J Appl Dev Psychol. 2012;33:294–301.
Liu H, Shi J, Liu Y, Sheng Z. The moderating role of attachment anxiety on social network site use intensity and social capital. Psychol Rep. 2013;112:252–65.
Oldmeadow JA, Quinn S, Kowert R. Attachment style, social skills, and Facebook use amongst adults. Comput Hum Behav. 2013;29:1142–9.
Eroglu Y. Interrelationship between attachment styles and Facebook addiction. J Educ Train Stud. 2016;4:150–60.
Google Scholar
Yaakobi E, Goldenberg J. Social relationships and information dissemination in virtual social network systems: an attachment theory perspective. Comput Hum Behav. 2014;38:127–35.
SoSci. Survey online survey tool.
Deng T, Kanthawala S, Meng J, Peng W, Kononova A, Hao Q, et al. Measuring smartphone usage and task switching with log tracking and self-reports. Mob Media Communication. 2019;7:3–23.
Monacis L, Palo VD, Griffiths MD, Sinatra M. Validation of the internet gaming disorder scale– short-form (IGDS9-SF) in an italian-speaking sample. J Behav Addictions. 2016;5:683–90.
Spitzer C, Hammer S, Löwe B, Grabe H, Barnow S, Rose M, et al. Die kurzform des brief symptom inventory (BSI– 18): erste Befunde zu den psychometrischen Kennwerten Der Deutschen version. Fortschr Neurol Psychiatr. 2011;79:517–23.
Franke GH, Jaeger S, Glaesmer H, Barkmann C, Petrowski K, Braehler E. Psychometric analysis of the brief symptom inventory 18 (BSI-18) in a representative German sample. BMC Med Res Methodol. 2017;17:14.
Franke GH, Ankerhold A, Haase M, Jäger S, Tögel C, Ulrich C, et al. Der Einsatz Des brief symptom inventory 18 (BSI-18) Bei psychotherapiepatienten. Psychother Psych Med. 2011;61:82–6.
Rammstedt B, John OP. Measuring personality in one minute or less: a 10-item short version of the big five inventory in English and German. J Res Pers. 2007;41:203–12.
Rammstedt B, Kemper CJ, Klein MC, Beierlein C, Kovaleva A. Eine Kurze Skala Zur Messung Der fünf Dimensionen Der Persönlichkeit: big-five-Inventory-10 (BFI-10). Mannheim: GESIS - Leibniz-Institut für Sozialwissenschaften; 2012.
Asendorpf JB, Neyer FJ. Psychologie Der Persönlichkeit. Berlin, Heidelberg: Springer Berlin Heidelberg; 2012.
Book Google Scholar
Höger D, Buschkämper S. Der Bielefelder Fragebogen zu Partnerschaftserwartungen. Z für Differentielle und Diagnostische Psychologie. 2002;23:83–98.
Collins NL, Read SJ. Adult attachment, working models, and relationship quality in dating couples. J Personal Soc Psychol. 1990;58:644–63.
Article CAS Google Scholar
Müller K. Wer ist gefährdet? Risikofaktoren für Internetsucht. Spielwiese internet. Berlin, Heidelberg: Springer Berlin Heidelberg; 2013. pp. 67–84.
Chapter Google Scholar
Wölfling K, Beutel M, Dreier M, Müller K. Risikofaktoren Von Verhaltenssucht: Explizite Persönlichkeitsfaktoren Und Implizite Assoziationsstärken Bei Pathologischem Glücksspiel Und Internetsucht. Suchttherapie. 2015;16:s–0035.
Kircaburun K, Self-Esteem. Daily internet use and social media addiction as predictors of depression among Turkish adolescents. J Educ Pract. 2016;7:64–72.
Wu AMS, Li J, Lau JTF, Mo PKH, Lau MMC. Potential impact of internet addiction and protective psychosocial factors onto depression among Hong Kong Chinese adolescents– direct, mediation and moderation effects. Compr Psychiatr. 2016;70:41–52.
Azher M, Khan RB, Salim M, Bilal M, Hussain A, Haseeb M, et al. The relationship between internet addiction and anxiety among students of University of Sargodha. Int J Humanit Social Sci. 2014;4:288–93.
Younes F, Halawi G, Jabbour H, El Osta N, Karam L, Hajj A, et al. Internet addiction and relationships with insomnia, anxiety, depression, stress and self-esteem in University students: a cross-sectional designed study. PLoS ONE. 2016;11:e0161126.
Cerutti R, Spensieri V, Amendola S, Presaghi F, Fontana A, Faedda N, et al. Sleep disturbances partially mediate the association between problematic internet use and somatic symptomatology in adolescence. Curr Psychol. 2021;40:4581–9.
Cerruti R, Spensieri V, Presaghi F, Valastro C, Fontana A, Guidetti V. An exploratory study on internet addiction, somatic symptoms and emotional and behavioral functioning in school-aged adolescents. Clin Neuropsychiatry. 2017.
Cerutti R, Presaghi F, Spensieri V, Valastro C, Guidetti V. The potential impact of internet and mobile use on headache and other somatic symptoms in adolescence. A Population-Based Cross‐Sectional Study. Headache. 2016;56:1161–70.
D’Arienzo MC, Boursier V, Griffiths MD. Addiction to social media and attachment styles: a systematic literature review. Int J Ment Health Addict. 2019;17:1094–118.
Baek HW, Shin YM, Shin KM. Emotional and behavioral problems related to smartphone overuse in elementary school children. J Korean Neuropsychiatr Assoc. 2014;53:320.
Kwan HC, Leung MT. The path model of parenting style, attachment style, self-regulation and smartphone addiction. Applied psychology. Concorde Hotel. Singapore: WORLD SCIENTIFIC; 2015. pp. 196–214.
Yelpaze İ. The mediator role of self-control in the relationship between insecure attachment styles and problematic smartphone use in adolescents. Turkish Psychol Couns Guidance J. 2020;10:457–73.
Parada-Fernández P, Herrero‐Fernández D, Oliva‐Macías M, Rohwer H. Analysis of the mediating effect of mentalization on the relationship between attachment styles and emotion dysregulation. Scandinavian J Psychol. 2021;62:312–20.
Santoro G, Midolo LR, Costanzo A, Schimmenti A. The vulnerability of insecure minds: the mediating role of mentalization in the relationship between attachment styles and psychopathology. Bull Menninger Clin. 2021;85:358–84.
Sax LJ, Gilmartin SK, Bryant AN. Assessing response rates and nonresponse bias in web and paper surveys. Res High Educt. 2003;44:409–32.
Jackob N, Schoen H, Zerback T, editors. Sozialforschung Im Internet. Wiesbaden: VS Verlag für Sozialwissenschaften; 2009.
Rose J, McGuire-Snieckus R, Gilbert L, McInnes K. Attachment aware schools: the impact of a targeted and collaborative intervention. Pastoral Care Educ. 2019;37:162–84.
Download references
Acknowledgements
Not applicable.
Author information
Authors and affiliations.
Faculty of Medicine, Institute of Psychosomatics, Sigmund Freud Private University, Freudplatz 3, Vienna, 1020, Austria
Christiane Eichenberg, Raphaela Schneider & Helena Rumpl
You can also search for this author in PubMed Google Scholar
Contributions
CE was involved in planning, supervising the work, and writing the manuscript. HR collected survey data and did the data curation. RS analyzed data and was involved in writing the manuscript.
Corresponding author
Correspondence to Raphaela Schneider .
Ethics declarations
Ethics approval and consent to participate.
The study involving human participants was reviewed and approved by the Ethics Commission of the Faculty of Psychotherapy Science and the Faculty of Psychology of the Sigmund Freud Private University Vienna. The patients/participants provided their written informed consent to participate in this study.
Consent for publication
Competing interests.
The authors declare no competing interests.
Additional information
Publisher’s note.
Springer Nature remains neutral with regard to jurisdictional claims in published maps and institutional affiliations.
Rights and permissions
Open Access This article is licensed under a Creative Commons Attribution 4.0 International License, which permits use, sharing, adaptation, distribution and reproduction in any medium or format, as long as you give appropriate credit to the original author(s) and the source, provide a link to the Creative Commons licence, and indicate if changes were made. The images or other third party material in this article are included in the article’s Creative Commons licence, unless indicated otherwise in a credit line to the material. If material is not included in the article’s Creative Commons licence and your intended use is not permitted by statutory regulation or exceeds the permitted use, you will need to obtain permission directly from the copyright holder. To view a copy of this licence, visit http://creativecommons.org/licenses/by/4.0/ . The Creative Commons Public Domain Dedication waiver ( http://creativecommons.org/publicdomain/zero/1.0/ ) applies to the data made available in this article, unless otherwise stated in a credit line to the data.
Reprints and permissions
About this article
Cite this article.
Eichenberg, C., Schneider, R. & Rumpl, H. Social media addiction: associations with attachment style, mental distress, and personality. BMC Psychiatry 24 , 278 (2024). https://doi.org/10.1186/s12888-024-05709-z
Download citation
Received : 31 January 2024
Accepted : 22 March 2024
Published : 15 April 2024
DOI : https://doi.org/10.1186/s12888-024-05709-z
Share this article
Anyone you share the following link with will be able to read this content:
Sorry, a shareable link is not currently available for this article.
Provided by the Springer Nature SharedIt content-sharing initiative
- Internet related addiction
- Insecure attachment style
BMC Psychiatry
ISSN: 1471-244X
- Submission enquiries: [email protected]
- General enquiries: [email protected]
Advertisement
The Relationships Among Social Media Intensity, Smartphone Addiction, and Subjective Wellbeing of Turkish College Students
- Published: 15 July 2020
- Volume 16 , pages 1999–2021, ( 2021 )
Cite this article
- Tuğba Koç ORCID: orcid.org/0000-0002-2454-9992 1 &
- Aykut Hamit Turan ORCID: orcid.org/0000-0002-8855-4643 1
2883 Accesses
17 Citations
Explore all metrics
Excessive use of new technologies appears to be potentially addictive for a minority of young people. The aim of the present study was to examine the relationship between personal environment factors (self-esteem and subjective wellbeing) and behaviors (social network site [SNS] intensity and smartphone addiction) drawing upon the Social Cognitive Theory and utilizing structural equation modeling. The sample comprised 734 undergraduate Business School students. Findings indicated that young people tend to use SNSs to enhance their extrinsic outcome expectation (network size) rather than intrinsic one (subjective well-being). Based on our knowledge, the present study is the first to address SNS usage behavior to assess extrinsic and intrinsic factors separately. Results also show that high SNS intensity is associated with high levels of smartphone usage, which decreases the individuals’ level of subjective wellbeing. It is also proved that low self-esteem causes smartphone addiction. Lastly, there is a weak but significant relation between SNS intensity and network size is also found. Overall, the present study contributes to our understanding of problematic smartphone and social media use.
This is a preview of subscription content, log in via an institution to check access.

Access this article
Price includes VAT (Russian Federation)
Instant access to the full article PDF.
Rent this article via DeepDyve
Institutional subscriptions
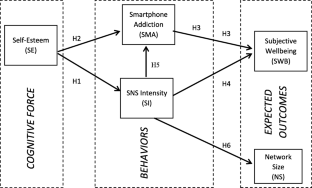
Similar content being viewed by others
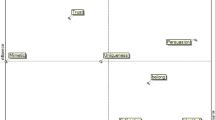
The effect of social media influencers' on teenagers Behavior: an empirical study using cognitive map technique
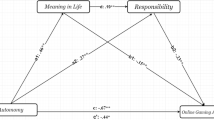
Online Gaming Addiction and Basic Psychological Needs Among Adolescents: The Mediating Roles of Meaning in Life and Responsibility
Social media effects on young women’s body image concerns: theoretical perspectives and an agenda for research.
Aiken, L. R. (1980). Content Validity and Reliability of Single Items or Questionnaires. Educational and Psychological Measurement, 40 , 955–959.
Article Google Scholar
Ainin, S., Naqshbandi, M. M., Moghavvemi, S., & Jaafar, N. I. (2015). Facebook usage, socialization and academic performance. Computers and Education, 83 , 64–73. https://doi.org/10.1016/j.compedu.2014.12.018 .
Akers, R. L. (1991). Addiction: The Troublesome Concept. Journal of Drug Issues, 21 (4), 777–793. https://doi.org/10.1177/002204269102100407 .
Alavi, S., Ferdosi, M., Jannatifard, F., Eslami, M., Alaghemandan, H., & Setare, M. (2012). Behavioral addiction versus substance addiction: correspondence of psychiatric and psychological views. International Journal of Preventive Medicine, 3 (4), 290–294.
Google Scholar
Andreassen, C. S. (2015). Online Social Network Site Addiction: A Comprehensive Review. Current Addiction Reports, 2 (2), 175–184. https://doi.org/10.1007/s40429-015-0056-9 .
Andreassen, C. S., & Pallesen, S. (2014). Social Network Site Addiction-An Overview. Current Pharmaceutical Design, 20 , 1–9 Retrieved from http://freepsychologypdf.com/wp-content/uploads/2018/07/Andreassen-2014-Social-network-site-addiction-An-overview.pdf .
Andreassen, C., Pallesen, S., & Griffiths, M. (2016). The relationship between addictive use of social media, narcissism, and self-esteem: Findings from a large national survey. Addictive Behaviors, 64 , 287–293.
Aydın, B., & Sarı, S. V. (2011). Internet addiction among adolescents: The role of self-esteem. In Procedia - Social and Behavioral Sciences (Vol. 15, pp. 3500–3505). https://doi.org/10.1016/j.sbspro.2011.04.325
Bae, S.-M. (2019). The relationship between smartphone use for communication, social capital, and subjective well-being in Korean adolescents: Verification using multiple latent growth modeling. Children and Youth Services Review, 96 , 93–99.
Baek, Y., Bae, Y., & Jang, H. (2013). Social and parasocial relationships on social network sites and their differen- tial relationships with users’ psychological well-being. Cyberpsychology, Bahevior, and Social Networking, 16 , 512–517.
Baker, J., & Moore, S. (2008). Distress, coping, and blogging: comparing new MySpace users by their intention to blog. CyberPsychology & Behavior, 11 , 81–85.
Bandura, A. (1986). Social foundations of thought and action: A social cognitive theory . Englewood Cliffs: Prentice-Hall.
Bányai, F., Zsila, Á., Király, O., Maraz, A., Elekes, Z., Griffiths, M., … Demetrovics, Z. (2017). Problematic social media use: Results from a large-scale nationally representative adolescent sample . PLoS ONE, 12 (1), 10–14. https://doi.org/10.1371/journal.pone.0169839
Barnes, S. J., Pressey, A. D., & Scornavacca, E. (2019). Mobile ubiquity: Understanding the relationship between cognitive absorption, smartphone addiction and social network services. Computers in Human Behavior, 90 (September 2018), 246–258. https://doi.org/10.1016/j.chb.2018.09.013 .
Bianchi, A., & Phillips, J. G. (2005). Psychological Predictors of Problem Mobile Phone Use. CyberPsychology & Behavior, 8 (1), 39–51. https://doi.org/10.1089/cpb.2005.8.39 .
Billieux, J. (2012). Problematic use of the mobile phone: a literature review and a pathways model. Current Psychiatry Reviews, 8 (4), 299–307.
Billieux, J., Maurage, P., Lopez-Fernandez, O., Kuss, D. J., & Griffiths, M. D. (2015). Can Disordered Mobile Phone Use Be Considered a Behavioral Addiction? An Update on Current Evidence and a Comprehensive Model for Future Research. Current Addiction Reports, 2 (2), 156–162. https://doi.org/10.1007/s40429-015-0054-y .
Chan, M. (2015). Mobile phones and the good life: Examining the relationships among mobile use, social capital and subjective well-being. New Media and Society, 17 (1), 96–113. https://doi.org/10.1177/1461444813516836 .
Chan, M. (2018). Mobile-mediated multimodal communications, relationship quality and subjective well-being: An analysis of smartphone use from a life course perspective. Computers in Human Behavior, 87 , 254–262. https://doi.org/10.1016/j.chb.2018.05.027 .
Charlton, J. P. & Danforth, I. D. W. (2010). Validating the distinction between computer addiction and engagement: Online game playing and personality. Behaviour & Information Technology, 29 , 601–613.
Chiu, C. M., Hsu, M. H., & Wang, E. T. G. (2006). Understanding knowledge sharing in virtual communities: An integration of social capital and social cognitive theories. Decision Support Systems, 42 (3), 1872–1888. https://doi.org/10.1016/j.dss.2006.04.001 .
Choi, J., & Kim, H. J. (2016). Influence of SNS User Innovativeness and Public Individuation on SNS Usage Patterns and Social Capital Development: The Case of Facebook. International Journal of Human-Computer Interaction, 32 (12), 921–930. https://doi.org/10.1080/10447318.2016.1220067 .
Chou, H.-T. G., & Edge, N. (2012). “They Are Happier and Having Better Lives than I Am”: The Impact of Using Facebook on Perceptions of Others’ Lives. Cyberpsychology, Behavior, and Social Networking, 15 (2), 117–121. https://doi.org/10.1089/cyber.2011.0324 .
Davis, K. (2012). Friendship 2.0: Adolescents’ experiences ofbelonging and self-disclosure online. Journal of Adolescence, 35 , 1527–1536.
Dayapoğlu, N., Kavurmaci, M., & Karaman, S. (2016). The Relationship between the Problematic Mobile Phone Use and Life Satisfaction, Loneliness, and Academic Performance in Nursing Students. International Journal of Caring Sciences May, 9 (2), 2–647 Retrieved from www.internationaljournalofcaringsciences.org .
Diener, E., Emmons, R. A., Larsen, R. J., & Griffin, S. (1985). The satisfaction with life scale. Journal of Personality Assessment, 49 (1), 71–75.
Duffy, B., Smith, K., Terhanian, G., & Bremer, J. (2005). Comparing data from online and face-to-face surveys. International Journal of Market Research, 47 (6), 615–630. https://doi.org/10.1177/147078530504700602 .
Ehrenberg, A., Juckes, S., White, K. M., & Walsh, S. P. (2008). Personality and Self-Esteem as Predictors of Young People’s Technology Use. CyberPsychology & Behavior, 11 (6), 739–741. https://doi.org/10.1089/cpb.2008.0030 .
Elhai, J. D., Dvorak, R. D., Levine, J. C., & Hall, B. J. (2017). Problematic smartphone use: A conceptual overview and systematic review of relations with anxiety and depression psychopathology. Journal of Affective Disorders, 207 (August 2016), 251–259. https://doi.org/10.1016/j.jad.2016.08.030 .
Ellison, N. B., Steinfield, C., & Lampe, C. (2007). The benefits of facebook “friends:” Social capital and college students’ use of online social network sites. Journal of Computer-Mediated Communication, 12 (4), 1143–1168. https://doi.org/10.1111/j.1083-6101.2007.00367.x .
Fornell, C., & Larcker, D. F. (1981). Evaluating Structural Equation Models with Unobservable Variables and Measurement Error. Journal of Marketing Research, 18 (1), 39. https://doi.org/10.2307/3151312 .
Ganley, D., & Lampe, C. (2009). The ties that bind: Social network principles in online communities. Decision Support Systems, 47 (3), 266–274.
Gezgin, D. M. (2018). Understanding Patterns for Smartphone Addiction: Age, Sleep Duration, Social Network Use and Fear of Missing Out. Cypriot Journal of Educational Sciences, 13 (2), 166–177.
Greenwood, J., Guner, N., Santos, C., & Kocharkov, G. (2016). Technology and the changing family: a unified model of marriage, divorce, educational attainment and marred female labor-force participation. American Economic Journal: Macroeconomics, 8 (1), 1–41. https://doi.org/10.1257/mac.20130156 .
Griffiths, M. (1996). Gambling on the internet: A brief note. Journal of Gambling Studies, 12 (4), 471–473.
Griffiths, M. (1999). Internet addiction: Internet fuels other addictions. Student British Medical Journal, 7 , 428–429.
Griffiths, M. (2009). A ‘components’ model of addiction within a biopsychosocial framework. Journal of Substance Use, 10 (4), 191–197
Griffiths, M., Kuss, D., & Demetrovics, Z. (2014). Social Networking Addiction: An Overview of Preliminary Findings. In K. Rosenberg & C. Feder (Eds.), Behavioral addictions: Criteria, evidence, and treatment (pp. 119–141). London: Academic Press.
Chapter Google Scholar
Gutiérrez, J. D. S., de Fonseca, F. R., & Rubio, G. (2016). Cell-phone addiction: A review. Frontiers in Psychiatry, 7 (Article 175), 1–15. https://doi.org/10.3389/fpsyt.2016.00175 .
Ha, J., Chin, B., Park, D., Ryu, S., & Yu, J. (2008). Characteristics of excessive cellular phone use in Korean adolescents. CyberPsychology & Behavior, 11 (6), 783–784.
Hair, J. F. J., Hult, G. T. M., Ringle, C. M., Sarstedt, M., (2014). A primer on partial least squares structural equation modeling (PLS-SEM). Thousand Oaks, CA: SAGE Publications.
Haug, S., Castro, R. P., Kwon, M., Filler, A., Kowatsch, T., & Schaub, M. P. (2015). Smartphone use and smartphone addiction among young people in Switzerland. Journal of Behavioral Addictions, 4 (4), 299–307. https://doi.org/10.1556/2006.4.2015.037 .
Hawi, N., & Samaha, M. (2017). The Relations Among Social Media Addiction, Self-Esteem, and Life Satisfaction in University Students. Social Science Computer Review, 35 (5), 576–586.
Hawi, N., & Samaha, M. (2018). Identifying commonalities and differences in personality characteristics of Internet and social media addiction profiles: traits, self-esteem, and self-construal. Behaviour & Information Technology, 38 (2), 110–119. https://doi.org/10.1080/0144929X.2018.1515984 .
Hong, F. Y., Chiu, S. I., & Huang, D. H. (2012). A model of the relationship between psychological characteristics, mobile phone addiction and use of mobile phones by Taiwanese university female students. Computers in Human Behavior, 28 (6), 2152–2159. https://doi.org/10.1016/j.chb.2012.06.020 .
Hormes, J. M., Kearns, B., & Timko, C. A. (2014). Craving Facebook? Behavioral addiction to online social networking and its association with emotion regulation deficits . Addiction (Vol. 109). https://doi.org/10.1111/add.12713
Horwood, S., & Anglim, J. (2019). Problematic smartphone usage and subjective and psychological well-being. Computers in Human Behavior, 97 (March), 44–50. https://doi.org/10.1016/j.chb.2019.02.028 .
Hu, X., Kim, A., Siwek, N., & Wilder, D. (2017). The Facebook Paradox: Effects of Facebooking on individuals’ social relationships and psychological well-being. Frontiers in Psychology, 8 (JAN), 1–8. https://doi.org/10.3389/fpsyg.2017.00087 .
Huang, C. (2017). Time Spent on Social Network Sites and Psychological Well-Being: A Meta-Analysis. Cyberpsychology, Behavior, and Social Networking, 20 (6), 346–354. https://doi.org/10.1089/cyber.2016.0758 .
Hussain, Z., Simonovic, B., Stupple, E. J. N., & Austin, M. (2019). behavioral sciences Using Eye Tracking to Explore Facebook Use and Associations with Facebook Addiction, Mental, (February), 1–12. https://doi.org/10.3390/bs9020019
Jia, L., Hall, D., & Sun, S. (2014). The Effect of Technology Usage Habits on Consumers’ Intention to Continue Use Mobile Payments. Americas Conference on Information Systems , 1–12.
Jin, B., & Park, N. (2013). Mobile voice communication and loneliness: Cell phone use and the social skills deficit hypothesis. New Media & Society, 15 , 1094–1111.
Kang, S., & Jung, J. (2014). Mobile communication for human needs: A comparison of smartphone use between the US and Korea. Computers in Human Behavior, 35 , 376–387.
Kassel, J. (2010). Substance abuse and emotion . Washington, DC: American Psychological Association.
Book Google Scholar
Kim, E., & Koh, E. (2018). Avoidant attachment and smartphone addiction in college students: The mediating effects of anxiety and self-esteem. Computers in Human Behavior, 84 , 264–271. https://doi.org/10.1016/j.chb.2018.02.037 .
Kim, J., LaRose, R., & Peng, W. (2009). Loneliness as the cause and the effect of problematic Internet use: the relationship between Internet use and psychological well-being. CyberPsychology & Behavior, 12 (4), 451–455.
Kircaburun, K., Demetrovics, Z., & Tosuntaş, Ş. B. (2018). Analyzing the Links Between Problematic Social Media Use, Dark Triad Traits, and Self-esteem. International Journal of Mental Health and Addiction. https://doi.org/10.1007/s11469-018-9900-1 .
Koo, H. J., & Kwon, J. H. (2014). Risk and protective factors of internet addiction: A meta-analysis of empirical studies in Korea. Yonsei Medical Journal, 55 (6), 1691–1711. https://doi.org/10.3349/ymj.2014.55.6.1691 .
Körmendi, A., Brutóczki, Z., Végh, B. P., & Székely, R. (2016). Smartphone use can be addictive? A case report. Journal of Behavioral Addictions, 5 (3), 548–552. https://doi.org/10.1556/2006.5.2016.033 .
Krasnova, H., Wenninger, H., Widjaja, T., & Buxmann, P. (2013). Envy on Facebook: A Hidden Threat to Users’ Life Satisfaction? Wirtschaftsinformatik Proceedings , 92.
Kring, A., Davison, G., Neale, J., & Johnson, S. (2007). Abnormal psychology . Hoboken: John Wiley & Sons.
Kross, E., Verduyn, P., Demiralp, E., Park, J., Lee, D. S., Lin, N., … Ybarra, O. (2013). Facebook Use Predicts Declines in Subjective Well-Being in Young Adults. PLoS ONE, 8 (8), 1–6. https://doi.org/10.1371/journal.pone.0069841
Kuss, D. J., & Griffiths, M. D. (2011). Online social networking and addiction-A review of the psychological literature. International Journal of Environmental Research and Public Health, 8 (9), 3528–3552. https://doi.org/10.3390/ijerph8093528 .
Kuss, D. J., & Griffiths, M. D. (2017). Social networking sites and addiction: Ten lessons learned. International Journal of Environmental Research and Public Health , 14 (3). https://doi.org/10.3390/ijerph14030311
Kwon, M., Lee, J.-Y., Won, W.-Y., Park, J.-W., Min, J.-A., Hahn, C., … Kim, D.-J. (2013). Development and validation of a smartphone addiction scale (SAS). PloS One , 8 (2), e56936. https://doi.org/10.1371/journal.pone.0056936
La Barbera, D., La Paglia, F., & Valsavoia, R. (2009). Social network and addiction. Annual Review of CyberTherapy and Telemedicine, 7 (1), 33–36. https://doi.org/10.3233/978-1-60750-017-9-33 .
Lee, J., Sung, M. J., Song, S. H., Lee, Y. M., Lee, J. J., Cho, S. M., … Shin, Y. M. (2018). Psychological Factors Associated With Smartphone Addiction in South Korean Adolescents. Journal of Early Adolescence , 38 (3), 288–302. https://doi.org/10.1177/0272431616670751
Lee-Won, R. J., Shim, M., Joo, Y. K., & Park, S. G. (2014). Who puts the best “face” forward on Facebook?: Positive self-presentation in online social networking and the role of self-consciousness, actual-to-total Friends ratio, and culture. Computers in Human Behavior, 39 , 413–423. https://doi.org/10.1016/j.chb.2014.08.007 .
Leung, L. (2008). Leisure boredom, sensation seeking, self-esteem, and addiction: Symptoms and patterns of cell phone use. In E. A. Konjin, S. Utz, M. Tanis, & S. B. Bames (Eds.), Mediated Interrpersonal Communication (pp. 359–381). New York: Taylor & Francis.
Lin, H. C., & Chang, C. M. (2018). What motivates health information exchange in social media? The roles of the social cognitive theory and perceived interactivity. Information and Management, 55 (6), 771–780. https://doi.org/10.1016/j.im.2018.03.006 .
Lin, T. C., & Huang, C. C. (2008). Understanding knowledge management system usage antecedents: An integration of social cognitive theory and task technology fit. Information and Management, 45 (6), 410–417. https://doi.org/10.1016/j.im.2008.06.004 .
Lin, T. C., & Huang, C. C. (2010). Withholding effort in knowledge contribution: The role of social exchange and social cognitive on project teams. Information and Management, 47 (3), 188–196. https://doi.org/10.1016/j.im.2010.02.001 .
Ling, R. (2004). The mobile connection: The cellphone’s impact on society . San Francisco: Morgan Kauffman.
Malik, S., & Khan, M. (2015). Impact of Facebook addiction on narcissistic behavior and self-esteem among students. Journal of Pakistan Medical Association, 65 , 260–263.
McDaniel, B. (2015). ‘Technoference’: Everyday intrusions and inter- ruptions of technology in couple and family relationships. C. Bruess içinde, Family Communication in the Age of Digital and Social Media. New York: Peter Lang Publishing.
McDonald, R. P. (1978). Generalizability in factorable domains: Domain validity and reliability. Educational and Psychological Measurement, 38 , 75–79.
Mcintosh, C. N., Edwards, J. R., & Antonakis, J. (2014). Reflections on Partial Least Squares Path Modeling. Organizational Research Methods, 17 (2), 210–251. https://doi.org/10.1177/1094428114529165 .
Meena, P. S., Soni, R., Jain, M., & Paliwal, S. (2015). Social networking sites addiction and associated psychological problems among young adults: a study from North India. Sri Lanka Journal of Psychiatry, 6 (1), 14. https://doi.org/10.4038/sljpsyc.v6i1.8055 .
Mehdizadeh, S. (2010). Self-Presentation 2.0: Narcissism and Self-Esteem on Facebook. Cyberpsychology, Behavior, and Social Networking, 13 (4), 357–364. https://doi.org/10.1089/cyber.2009.0257 .
Meier, A., Reinecke, L., & Meltzer, C. E. (2016). Facebocrastination? Predictors of using Facebook for procrastination and its effects on students’ well-being. Computers in Human Behavior, 64 , 65–76. https://doi.org/10.1016/j.chb.2016.06.011 .
Montag, C., Błaszkiewicz, K., Lachmann, B., Sariyska, R., Andone, I., Trendafilov, B., & Markowetz, A. (2015). Recorded Behavior as a Valuable Resource for Diagnostics in Mobile Phone Addiction: Evidence from Psychoinformatics. Behavioral Sciences, 5 (4), 434–442. https://doi.org/10.3390/bs5040434 .
Moqbel, M., & Kock, N. (2018). Unveiling the dark side of social networking sites: Personal and work-related consequences of social networking site addiction. Information and Management, 55 (1), 109–119. https://doi.org/10.1016/j.im.2017.05.001 .
Munzel, A., Meyer-Waarden, L., & Galan, J. P. (2018). The social side of sustainability: Well-being as a driver and an outcome of social relationships and interactions on social networking sites. Technological Forecasting and Social Change, 130 (July 2017), 14–27. https://doi.org/10.1016/j.techfore.2017.06.031 .
Ng, Y.-K. (2015). Happiness, life satisfaction, or subjective well-being? A measurement and moral philosophical approach (pp. 1–27). Singapore: Nanyang Technological University.
Nunnally, J. C. (1978). Psychometric theory (2nd ed.). New York: McGraw-Hill.
Padilla-Walker, L., Coyne, S., & Fraser, A. (2012). Getting a High-Speed Family Connection:Associations Between Family Media Useand Family Connection. Family Relations: Inderdisciplinary Journal of Applied Family Science, 61 (3), 426–440.
Pavis, S., Hubbard, G., & Platt, S. (2001). Young people in rural areas: Social excluded or not? Work, Employment & Society, 15 , 291–309.
Pearson, A., Mack, E., & Namanya, J. (2017). Mobile Phones and Mental Well-Being: Initial Evidence Suggesting the Importance of Staying Connected to Family in Rural, Remote Communities in Uganda. PLoS One, 12 (1), e0169819.
Phu, B., & Gow, A. J. (2019). Facebook use and its association with subjective happiness and loneliness. Computers in Human Behavior, 92 (June 2018), 151–159. https://doi.org/10.1016/j.chb.2018.11.020 .
Pugh, S. (2017). Investigating the relationship between: Smartphone Addiction, Social Anxiety, Self-Esteem, Age and Gender . Retrieved from https://esource.dbs.ie/bitstream/handle/10788/3329/ba_pugh_s_2017.pdf?sequence=3
Punamäki, R.-L., Wallenius, M., Nygård, C.-S., Saarni, L., & Rimpelä, A. (2007). Use of information and communication technology (ICT) and perceived health in adolescence: The role of sleeping habits and waking-time tiredness. Journal of Adolescence, 30 (4), 569–585.
Rae, J. R., & Lonborg, S. D. (2015). Do motivations for using Facebook moderate the association between Facebook use and psychological well-being? Frontiers in Psychology, 6 (JUN), 1–9. https://doi.org/10.3389/fpsyg.2015.00771 .
Rashid, U. K., Ahmed, O., & Hossain, M. A. (2019). Relationship between Need for Belongingness and Facebook Addiction: Mediating Role of Number of Friends on Facebook. International Journal of Social Science Studies, 7 (2), 36. https://doi.org/10.11114/ijsss.v7i2.4017 .
Roberts, J., Yaya, L., & Manolis, C. (2014). The invisible addiction: Cell-phone activities and addiction among male and female college students. Journal of Behavioral Addictions, 3 (4), 254–265. https://doi.org/10.1556/JBA.3.2014.015 .
Roberts, J. A., Pullig, C., & Manolis, C. (2015). I need my smartphone: A hierarchical model of personality and cell-phone addiction. Personality and Individual Differences, 79 , 13–19. https://doi.org/10.1016/j.paid.2015.01.049 .
Robinson, M. A. (2018). Using multi-item psychometric scales for research and practice in human resource management. Human Resource Management, 57 (3), 739–750
Rosen, L., Lim, A., Felt, J., Carrier, L., Cheever, N., Lara-Ruiz, J., et al. (2014). NIH Public Access, 6 , 247–253. https://doi.org/10.1111/j.1743-6109.2008.01122.x.Endothelial .
Rosenberg, M. (1965). Society and the adolescent self-image . Princeton: Princeton University Press.
Ross, C., Orr, E., Sisic, M., Arseneault, J., Simmering, M., & Orr, R. (2009). Personality and motivations associated with Facebook use. Computers in Human Behavior, 25 (2), 578–586.
Rothen, S., Briefer, J. F., Deleuze, J., Karila, L., Andreassen, C. S., Achab, S., … Billieux, J. (2018). Disentangling the role of users’ preferences and impulsivity traits in problematic Facebook use. PLoS ONE , 13 (9), 1–13. https://doi.org/10.1371/journal.pone.0201971
Rotondi, V., Stanca, L., & Tomasuolo, M. (2017). Connecting alone: Smartphone use, quality of social interactions and well-being. Journal of Economic Psychology, 63 , 17–26.
Sagioglou, C., & Greitemeyer, T. (2014). Facebook’s emotional consequences: Why Facebook causes a decrease in mood and why people still use it. Computers in Human Behavior, 35 , 359–363.
Salehan, M., & Negahban, A. (2013). Social networking on smartphones: When mobile phones become addictive. Computers in Human Behavior, 29 (6), 2632–2639. https://doi.org/10.1016/j.chb.2013.07.003 .
Samaha, M., & Hawi, N. S. (2016). Relationships among smartphone addiction, stress, academic performance, and satisfaction with life. Computers in Human Behavior, 57 , 321–325. https://doi.org/10.1016/j.chb.2015.12.045 .
Satici, S., & Uysal, R. (2015). Well-being and problematic Facebook use. Computers in Human Behavior, 49 , 185–190.
Shankar, V., Venkatesh, A., Hofacker, C., & Naik, P. (2010). Mobile Marketing in the Retailing Environment: Current Insights and Future Research Avenues. Journal of Interactive Marketing, 24 (2), 111–120.
Smetaniuk, P. (2014). A preliminary investigation into the prevalence and prediction of problematic cell phone use. Journal of Behavioral Addictions, 3 (1), 41–53. https://doi.org/10.1556/JBA.3.2014.004 .
Steinfield, C., Ellison, N. B., & Lampe, C. (2008). Social capital, self-esteem, and use of online social network sites: A longitudinal analysis. Journal of Applied Developmental Psychology, 29 (6), 434–445. https://doi.org/10.1016/j.appdev.2008.07.002 .
Techspirited Staff. (2018). Facebook Addiction . Retrieved from https://techspirited.com/facebook-addiction .
Tufekci, Z. (2010). Who Acquires Friends hrough Social Media and Why? “Rich Get Richer” versus “Seek and Ye Shall Find” . Proceedings of the Fourth International AAAI Conference on Weblogs and Social Media (s. 170–177). Washington, D.C.: Association for the Advancement of Artificial Intelligence.
Valenzuela, S., Park, N., & Kee, K. F. (2009). Is There Social Capital in a Social Network Site?: Facebook Use and College Students’ Life Satisfaction, Trust, and Participation. Journal of Computer-Mediated Communication, 14 (4), 875–901. https://doi.org/10.1111/j.1083-6101.2009.01474.x .
Valkenburg, P., & Jochen, P. (2007). Online communication and aOnline communication and adolescent well-being: Testing the stimulation versus the displacement hypothesis. Journal of Computer-Mediated Communication, 12 , 1169–1182.
van Rooij, A., Ferguson, C., van de Mheen, D., & Schoenmakers, T. (2017). Time to Abadon Internet Addiction? Predicting Problematic Internet, Game, and Social Media Use from Psychosocial Well-Being and Application Use. Clinical Neuropsychiatry, 14 (1), 115–121.
Volkmer, S. A., & Lermer, E. (2019). Unhappy and addicted to your phone? – Higher mobile phone use is associated with lower well-being. Computers in Human Behavior, 93 (November 2018), 210–218. https://www.sciencedirect.com/science/article/pii/S0747563218306010 .
Walsh, S. P., White, K. M., Cox, S., & Young, R. M. D. (2011). Keeping in constant touch: The predictors of young Australians’ mobile phone involvement. Computers in Human Behavior, 27 (1), 333–342. https://doi.org/10.1016/j.chb.2010.08.011 .
Wang, J. L., Gaskin, J., Rost, D. H., & Gentile, D. A. (2018). The Reciprocal Relationship Between Passive Social Networking Site (SNS) Usage and Users’ Subjective Well-Being. Social Science Computer Review, 36 (5), 511–522. https://doi.org/10.1177/0894439317721981 .
Wegmann, E., Stodt, B., & Brand, M. (2015). Addictive use of social networking sites can be explained by the interaction of Internet use expectancies, Internet literacy, and psychopathological symptoms. Journal of Behavioral Addictions, 4 (3), 155–162. https://doi.org/10.1556/2006.4.2015.021 .
Wei, R., & Lo, V.-H. (2006). Staying connected while on the move: Cell phone use and social connectedness. New Media & Society, 8 (1), 53–72.
Wilcox, K., & Stephen, A. (2013). Are Close Friends the Enemy? Online Social Networks, Self-Esteem, and Self-Control. Journal of Consumer Research, 40 (1), 90–103.
Wilson, K., Fornasier, S., & White, K. M. (2010). Psychological Predictors of Young Adults’ Use of Social Networking Sites. Cyberpsychology, Behavior, and Social Networking, 13 (2), 173–177. https://doi.org/10.1089/cyber.2009.0094 .
Wold, H. (1982). Soft Modeling: The Basic Design and Some Extensions. In K. G. Joreskog & H. Wold (Eds.), Systems under Indirect Observations, Part II (pp. 1–54). Amsterdam: North-Holland.
Woollaston, V. (2013). How often do you check your phone? Science & Tech: Retrieved from https://www.dailymail.co.uk/sciencetech/article-2449632/How-check-phone-The-average-person-does-110-times-DAY-6-seconds-evening.html .
Wu, A. M. S., Cheung, V. I., Ku, L., & Hung, E. P. W. (2013). Psychological risk factors of addiction to social networking sites among Chinese smartphone users. Journal of Behavioral Addictions, 2 (3), 160–166. https://doi.org/10.1556/jba.2.2013.006 .
Xu, C., Ryan, S., Prybutok, V., & Wen, C. (2012). It is not for fun: An examination of social network site usage. Information and Management, 49 (5), 210–217. https://doi.org/10.1016/j.im.2012.05.001 .
Yang, S., Liu, Y., & Wei, J. (2016). Social capital on mobile SNS addiction: A perspective from online and offline channel integrations. Internet Research, 26 (4), 982–1000. https://doi.org/10.1108/MRR-09-2015-0216 .
You, Z., Zhang, Y., Zhang, L., Xu, Y., & Chen, X. (2019). How does self-esteem affect mobile phone addiction? The mediating role of social anxiety and interpersonal sensitivity. Psychiatry Research, 271 (December 2018), 526–531. https://doi.org/10.1016/j.psychres.2018.12.040 .
Young, K. (1999). Internet addiction: Symptoms, evaluation and treatment. Innovations in Clinical Practice: A Source Book, 17 , 19–31.
Zhou, J., Zuo, M., Yu, Y., & Chai, W. (2014). How fundamental and supplemental interactions affect users’ knowledge sharing in virtual communities? A social cognitive perspective. Internet Research, 24 (5), 566–586. https://doi.org/10.1108/MRR-09-2015-0216 .
Download references
Author information
Authors and affiliations.
Management Information Systems Department, Sakarya University, Serdivan/Sakarya, Turkey
Tuğba Koç & Aykut Hamit Turan
You can also search for this author in PubMed Google Scholar
Corresponding author
Correspondence to Tuğba Koç .
Ethics declarations
The study was approved by the first author’s university ethics committee. All participants were informed about the study and the procedures were carried out according to Helsinki Declaration.
Conflict of Interest
The authors have no conflict of interest to declare.
Informed Consent
Verbal informed consent was obtained from students who participated in the study.
Additional information
Publisher’s note.
Springer Nature remains neutral with regard to jurisdictional claims in published maps and institutional affiliations.
Appendix 1. Survey Instrument
RSES1. On the whole, I am satisfied with myself.
RSES2. At times, I think I am no good at all.
RSES3. I feel that I have a number of good qualities.
RSES4. I am able to do things as well as most other people.
RSES5. I do not have much to be proud of.
RSES6. I certainly feel useless at times.
RSES7. I feel that I am a person of worth, at least on an equal plane with others.
RSES8. I wish I had more respect for myself.
RSES9. I am inclined to feel that I am a failure.
RSES10. I take a positive attitude towards myself.
SIS1. Visiting social networking sites is part of my everyday activity.
SIS2. I check my social networking site(s) almost every day.
SIS3. I feel out of touch when I haven’t logged onto my social networking site(s) for a day.
SIS4. I feel I am part of the community of my social networking site at the campus.
SIS5. I would be sorry if my social networking site shuts down.
SWLS1.In most ways my life is close to my ideal.
SWLS2. The conditions of my life are excellent.
SWLS3. I am satisfied with my life.
SWLS4. So far, I have gotten important things I want in life.
SWLS5. If I could live my time at over, I would change almost nothing.
SMA1. I feel lost when I do not have my mobile phone with me.
SMA2. When I do not have my mobile phone, I feel disconnected.
SMA3. I feel uneasy in places where mobile phone usage is prohibited.
SMA4. I feel the need to check again immediately after using the mobile phone.
SMA5. My excessive use of the mobile phone causes me to have problems with those around me.
SMA6. I use my mobile phone longer than I intend.
SMA7. I can’t do without my mobile phone.
Rights and permissions
Reprints and permissions
About this article
Koç, T., Turan, A.H. The Relationships Among Social Media Intensity, Smartphone Addiction, and Subjective Wellbeing of Turkish College Students. Applied Research Quality Life 16 , 1999–2021 (2021). https://doi.org/10.1007/s11482-020-09857-8
Download citation
Received : 28 February 2020
Accepted : 06 July 2020
Published : 15 July 2020
Issue Date : October 2021
DOI : https://doi.org/10.1007/s11482-020-09857-8
Share this article
Anyone you share the following link with will be able to read this content:
Sorry, a shareable link is not currently available for this article.
Provided by the Springer Nature SharedIt content-sharing initiative
- Social media use
- Smartphone addiction
- Social media addiction
- Problematic smartphone use
- Problematic social media use
- Find a journal
- Publish with us
- Track your research
Research trends in social media addiction and problematic social media use: A bibliometric analysis
Affiliations.
- 1 Sasin School of Management, Chulalongkorn University, Bangkok, Thailand.
- 2 Business Administration Division, Mahidol University International College, Mahidol University, Nakhon Pathom, Thailand.
- PMID: 36458122
- PMCID: PMC9707397
- DOI: 10.3389/fpsyt.2022.1017506
Despite their increasing ubiquity in people's lives and incredible advantages in instantly interacting with others, social media's impact on subjective well-being is a source of concern worldwide and calls for up-to-date investigations of the role social media plays in mental health. Much research has discovered how habitual social media use may lead to addiction and negatively affect adolescents' school performance, social behavior, and interpersonal relationships. The present study was conducted to review the extant literature in the domain of social media and analyze global research productivity during 2013-2022. Bibliometric analysis was conducted on 501 articles that were extracted from the Scopus database using the keywords social media addiction and problematic social media use. The data were then uploaded to VOSviewer software to analyze citations, co-citations, and keyword co-occurrences. Volume, growth trajectory, geographic distribution of the literature, influential authors, intellectual structure of the literature, and the most prolific publishing sources were analyzed. The bibliometric analysis presented in this paper shows that the US, the UK, and Turkey accounted for 47% of the publications in this field. Most of the studies used quantitative methods in analyzing data and therefore aimed at testing relationships between variables. In addition, the findings in this study show that most analysis were cross-sectional. Studies were performed on undergraduate students between the ages of 19-25 on the use of two social media platforms: Facebook and Instagram. Limitations as well as research directions for future studies are also discussed.
Keywords: bibliometric analysis; problematic social media use; research trends; social media; social media addiction.
Copyright © 2022 Pellegrino, Stasi and Bhatiasevi.
Publication types
- Systematic Review

An official website of the United States government
The .gov means it’s official. Federal government websites often end in .gov or .mil. Before sharing sensitive information, make sure you’re on a federal government site.
The site is secure. The https:// ensures that you are connecting to the official website and that any information you provide is encrypted and transmitted securely.
- Publications
- Account settings
Preview improvements coming to the PMC website in October 2024. Learn More or Try it out now .
- Advanced Search
- Journal List
- Indian J Psychiatry
- v.63(1); Jan-Feb 2021
Addiction-like behavior associated with social media usage in undergraduate students of a government medical college in Delhi, India
Saurav basu.
Department of Community Medicine, Maulana Azad Medical College, New Delhi, India
Ragini Sharma
Pragya sharma, nandini sharma, background:.
Excessive use of social media is increasingly being recognized as a source of technological addiction in young people globally.
The aim of this study is to assess social media addiction in medical students using a self-designed questionnaire.
Materials and Methods:
We collected data from undergraduate medical students (MBBS) in Delhi, India using a self-administered 20-item social media addiction questionnaire (SMAQ) to measure addiction-like behavior, and the Pittsburgh Sleep Quality Index (PSQI) to assess sleep quality.
We enrolled 264 (62.3%) male and 160 (37.7%) female participants of mean (standard deviation) age 19.83 (1.6) years. The Cronbach's alpha of the SMAQ was 0.879. A principal component analysis revealed a 4-component SMAQ structure based on eigenvalue cutoff (>1), loading score >0.3, and inspection of the Scree-plot that explained 54.7% of the total variance. We observed strong loadings of impaired control items on Component 1, decreased alternate pleasure items on Component 2, intense desire items on Component 3, and harmful use items on Component 4. The mean SMAQ score was significantly higher in the students reporting poor sleep quality and older students.
Conclusion:
The SMAQ has acceptable psychometric properties, with higher scores associated with sleep deprivation. A majority of students were unable to reduce their time spent on social media despite wanting to do so, signifying the presence of tolerance and impaired control.
INTRODUCTION
Social media constitutes a variety of internet applications that have provided >3 billion people with a platform to connect in real-time and share texts, messages, photos, and videos. The popularity of social media networks has exponentially increased in the previous decade due to the dissemination of affordable Internet and smartphone technology in developing countries, especially among their younger populations.[ 1 ] However, there is growing recognition that excessive use of social media is a form of behavioral addiction associated with high levels of anxiety and depressive symptomatology.[ 2 , 3 ] Some researchers have even compared extreme cases of addiction to social media comparable to substance-related addictions involving tobacco smoking or alcohol.[ 4 ]
Social media can promote the feeling of social envy and anxiety, and can potentially interfere with sleep.[ 5 ] Responding to late-night social media ping notifications on smartphones can reduce sleep quality, whereas blue light emissions can increase sleep latency by disturbing the circadian rhythm.[ 6 ] Furthermore, an extended number of hours spent on social media contributes to sleep displacement.[ 7 ] In younger age-group students, poor sleep quality can cause cognitive impairment and reduced concentration.[ 8 ] Studies have also linked excessive social media use with diminished academic performance in young students.[ 9 ]
The prevalence of social media addiction has been estimated to be particularly high among adolescents and young people in developed countries.[ 10 , 11 ] India, the country with the largest youth population globally, has become a world leader in the adoption of smartphones and mobile data in the past 5 years, which has materialized the growth and popularity of social media use.[ 12 ] However, there are very few studies that have evaluated social media addiction in India, especially with locally validated questionnaires. A recent study estimated that 36.9% of students in Southern India had social media addiction by using a questionnaire adapted from Young's internet addiction test.[ 13 ] A previous study among medical students in Delhi had reported a high prevalence of mobile phone addiction, which coupled with academic stress and anxiety, possibly renders them susceptible to social media addiction and sleep deprivation.[ 14 ]
We conducted the present study to assess social media addiction in medical students using a self-designed questionnaire.
MATERIALS AND METHODS
Design, setting, and participants.
We conducted a cross-sectional study among adult medical undergraduate (MBBS) and interns of a government medical college in Delhi situated in Northern India during September–November 2019. All the students could read, comprehend, and respond in the English language, and it is the official medium of instruction.
Study instruments
- We constructed a 20-item social media addiction questionnaire (SMAQ) in the English language after the application of the following steps: (a). Identification of domains that corresponded to the International Classification of Diseases, 10 th Revision criteria for substance dependence syndrome,[ 15 ] and the inclusion of items from the literature search and previously validated questionnaires related to behavioral and technological addiction.[ 14 , 16 , 17 ] The SMAQ assessed the presence of intense desire (Q.1, 2, 3, 4, 5, 6, 7, 8, 9, 10, 19), impaired control (Q. 3, 11, 12, 15, 16, 19), withdrawal (Q. 13), tolerance (Q. 5, 6, 11, 12, 16, 18), decreased alternate pleasure (Q. 5, 6, 7, 8, 9, 10, 13), and harmful use (Q. 14, 15, 17, 18, 19, 20). The SMAQ also corresponded to the four components of the CAGE screening questionnaire for potential drinking-related problems[ 18 ] and Griffith's six core components of addictions,[ 19 ] (b) Pretesting of the questionnaire was conducted in 10 students who were not part of the final study, (c) Content validity of the items was determined by two experts on behavioral health who went through each of the items and their pretest responses. Subsequently, they, by consensus, accepted the appropriateness of all the items subject to language modification in certain items. The response to the each of the SMAQ items was self-rated by the students on a 6-point Likert scale with options being 1 (strongly disagree), 2 (disagree), 3 (weakly disagree), 4 (weakly agree), 5 (agree), and 6 (strongly agree). The total SMAQ score was the sum of individual scores for all the 20 items of the questionnaire, with higher scores indicating a greater risk of addiction
- Pittsburgh Sleep Quality Index (PSQI) - The PSQI is a valid instrument used to differentiate “poor” from “good” sleep by measuring seven domains: subjective sleep quality, sleep latency, sleep duration, habitual sleep efficiency, and sleep disturbances, use of sleep medication, and daytime dysfunction over the last month.[ 20 ] The cutoff for poor and good sleepers was considered at a PSQI score >6, which has been previously validated in Indian university students.[ 21 ]
Sampling and data collection
The college has five batches of undergraduate medical students for the MBBS course lasting for five and a half years duration. We selected a total of 95 students from among the 250 cohorts in each batch, with final year students and interns considered as one-batch during participant selection. A sample size of ≥400 with item-to-respondent ratio of 20:1 is considered excellent for factor analysis.[ 22 ] We selected the participants by simple random sampling using a computer-generated set of random numbers for selection through student roll numbers. The selected participants were administered the questionnaires in groups in a separate lecture room. An investigator was present at these sites during this time to resolve any participant query regarding filling of the questionnaires and also prevent any interpersonal interaction among the participants.
Statistical analysis
We analyzed the data with the IBM SPSS Statistics for Windows, Version 25.0 (Armonk, NY: IBM Corp.).
Handling of missing data
We assumed that the missing data was at random. We excluded questionnaires with more than ≥4 incomplete entries in the social media questionnaire. Missing items were imputed, taking the item median score as per the gender and age-group to which the participant belonged.
The quantitative data were expressed in terms of mean, median, and standard deviations (SDs) and qualitative data in terms of frequency and proportions. The significance of the association between categorical variables was determined by Chi-square/Fisher's exact test. The independent samples t -test was applied to test for the significance of the mean difference between groups. A P < 0.05 was considered statistically significant. We also conducted a principal component analysis (PCA) of the SMAQ.
Ethical considerations
The study was approved with an exemption from full review by the Institutional Ethics Committee of the medical college. We obtained written and informed consent from all the study participants, and we maintained data confidentiality, with the data being used for research purposes only.
Participant characteristics
We distributed a total of 475 questionnaires to medical undergraduate students and interns of the selected medical college with a response rate of 100%. We excluded 51 questionnaires with incomplete responses, and hence, the effective sample size was 424. We enrolled 264 (62.3%) male and 160 (37.7%) female participants. The mean (SD) age of the participants was 19.83 (1.6) years.
Social media usage
The total typical weekly mean (SD) time spent on social media by the participants was 21.8 (11.2) hours, with adolescents reporting significantly lower time spent on social media compared to older participants ( P < 0.001), although no association existed with participant gender ( P = 0.165). Popular social media platforms such as Facebook and YouTube were accessed more frequently by older or male students compared to adolescent or female students [ Figure 1 ].

Social media usage in medical students of Delhi ( n = 424). *Mean number of days accessed in a typical week
Psychometric properties of the social media addiction questionnaire
The Cronbach's alpha of the 20-item SMAQ was 0.879 and the split-half reliability coefficient was 0.765, indicating very good reliability. The dataset was assessed to be suitable for the application of PCA in terms of sampling adequacy. The overall Kaiser-Meyer-Olkin (KMO) measure was 0.89 classifications of “Meritorious” according to Kaiser. All the individual KMO measures were >0.8. The correlation matrix showed that all variables had at least one correlation coefficient >0.3. The Bartlett's test of Sphericity was statistically significant ( P < 0.0001).
We found four components that had eigenvalues >1, which explained 30.4%, 10.5%, 7.4%, and 6% of the total variance, respectively, thereby cumulatively explaining 54.7% of the total variance. Visual inspection of the Scree plot also indicated that four components should be retained [ Figure 2 ]. We observed strong loadings of impaired control items on Component 1, decreased alternate pleasure items on Component 2, intense desire items on Component 3, and harmful use items on Component 4. Component loadings and communalities of the rotated solution are presented in Table 1 .

Scree plot of a 4-component social media addiction questionnaire
Rotated structure matrix for principal component analysis with varimax rotation of a 4-component social media addiction questionnaire showing major loadings
Extraction Method: Principal Component Analysis Rotation method: Varimax with Kaiser normalization
Construct validity of the social media addiction questionnaire
On dichotomizing responses into “agree” and “disagree” categories, higher mean scores were seen for items 1, 2, 3, 6 (eye-opener/intense desire), 11 (cut-down/tolerance) and 11, 15, 16 (impaired control) [ Table 2 ]. Older students compared to their junior counterparts were significantly more likely to agree that “life would be less interesting,” “they could not imagine living” and “felt unhappy and annoyed when not connected” to social media. The students with poor quality sleep were also significantly more likely to agree regarding the harmful consequences of social media use compared to good sleepers [ Table 3 ]. The average time spent on social media showed a moderate correlation with the SMAQ score ( r = 0.4, P < 0.001).
Distribution of responses to the social media addiction questionnaire in medical students ( n =424)
Item agreement on the social media addiction questionnaire and association with age, gender, and sleep quality among medical students ( n =424)
*Statistically significant
Association with sleep quality
The mean (SD) global PSQI score was 5.66 (2.6). We classified 276 (65.1%) participants as having a good sleep (PSQI ≤6) and 148 (34.9) as having a poor sleep (PSQI ≥7). The mean SMAQ score was significantly higher in the students reporting poor sleep compared to those showing good sleep quality [ Table 4 ].
Construct validity of the social media addiction questionnaire in medical students ( n =424)
CI – Confidence interval; SMAQ – Social media addiction questionnaire; SD – Standard deviation
The exponential increase in social media users globally intensifies the need for its measurement, which is particularly challenging in the absence of validated instruments.[ 23 ] We found the 20-item SMAQ displayed acceptable psychometric properties for the assessment of self-reported social media addiction in young medical undergraduates. Social media addiction scores were significantly higher in poor sleepers compared to good sleepers. Furthermore, increased time spent on social media also correlated with higher addiction scores. The evaluation of item-responses of the SMAQ revealed that a majority of participants were unable to reduce their time spent on social media despite wanting to do so signifying the presence of tolerance and impaired control.
Although it is well-established that school-going adolescents are at high risk of social media addiction,[ 10 ] in the present study, college-going adolescents reported less likelihood of addiction-like behavior compared to their senior counterparts reiterating the persistent addiction potential of the technology among youth.
In this study, gender was not associated with the potential for social media addiction in contradiction to a previous study conducted in India that found male gender to be a risk factor.[ 13 ] However, another large-scale study in Norway found women to be predisposed to a higher risk of addiction to activities on social media[ 11 ] indicating nonavailability of any definite evidence in studies limited by the cross-sectional design.
The study revealed that one in three medical undergraduates experienced poor sleep, with bad sleepers more likely to report excessive social media use compared to good sleepers. This corroborates the evidence from previous studies, which also found worsening sleep with increasing duration of social media use.[ 7 ] Poor sleep has been previously linked to diminished academic performance.[ 7 , 24 ] In this study, nearly half (46.5%) of the students were in agreement with the view that excessive social media use was negatively affecting their academic performance. However, another study in Eastern India reported a majority of medical students having a positive perception of social media impact on their academic performance.[ 25 ]
Finally, most participants did not agree that social media diminished their interpersonal relationships, promoted narcissism, or impacted their self-esteem, in contradiction to a study in Norway,[ 11 ] suggesting significant cross-cultural differences in the long-term influence of social media on an individual's personality.
In conclusion, self-reported social media overuse leading to addiction-like symptoms was reported by a large proportion of medical undergraduates when assessed with a self-designed SMAQ. Despite the lack of compelling evidence for recognizing social media addiction as a disorder,[ 23 ] we found it was significantly associated with objective parameters like poor sleep quality.
There are certain limitations to the study. In the absence of an objective or gold standard for the measurement of social media addiction,[ 26 ] we could not determine a cutoff point for distinguishing addiction and lack of addiction. Moreover, as the collected data were self-reported, it is likely to be influenced by the social desirability bias. The test–retest reliability analysis was also not performed. The cross-sectional study design precluded the identification of any potential causality between addiction and adverse health, academic or interpersonal problems in the students. The estimated time spent on social media could be overestimated since multiple social media accounts can be handled simultaneously and often in short bursts rather than in prolonged sessions. Finally, we conducted the study in medical students who are more likely to have a better awareness of addiction compared to other student populations, reducing the generalizability of the study findings.
Financial support and sponsorship
Conflicts of interest.
There are no conflicts of interest.
Numbers, Facts and Trends Shaping Your World
Read our research on:
Full Topic List
Regions & Countries
- Publications
- Our Methods
- Short Reads
- Tools & Resources
Read Our Research On:
Teens and social media: Key findings from Pew Research Center surveys
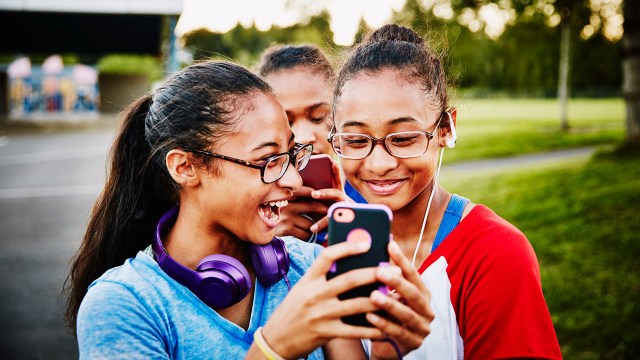
For the latest survey data on social media and tech use among teens, see “ Teens, Social Media, and Technology 2023 .”
Today’s teens are navigating a digital landscape unlike the one experienced by their predecessors, particularly when it comes to the pervasive presence of social media. In 2022, Pew Research Center fielded an in-depth survey asking American teens – and their parents – about their experiences with and views toward social media . Here are key findings from the survey:
Pew Research Center conducted this study to better understand American teens’ experiences with social media and their parents’ perception of these experiences. For this analysis, we surveyed 1,316 U.S. teens ages 13 to 17, along with one parent from each teen’s household. The survey was conducted online by Ipsos from April 14 to May 4, 2022.
This research was reviewed and approved by an external institutional review board (IRB), Advarra, which is an independent committee of experts that specializes in helping to protect the rights of research participants.
Ipsos invited panelists who were a parent of at least one teen ages 13 to 17 from its KnowledgePanel , a probability-based web panel recruited primarily through national, random sampling of residential addresses, to take this survey. For some of these questions, parents were asked to think about one teen in their household. (If they had multiple teenage children ages 13 to 17 in the household, one was randomly chosen.) This teen was then asked to answer questions as well. The parent portion of the survey is weighted to be representative of U.S. parents of teens ages 13 to 17 by age, gender, race, ethnicity, household income and other categories. The teen portion of the survey is weighted to be representative of U.S. teens ages 13 to 17 who live with parents by age, gender, race, ethnicity, household income and other categories.
Here are the questions used for this report, along with responses, and its methodology .
Majorities of teens report ever using YouTube, TikTok, Instagram and Snapchat. YouTube is the platform most commonly used by teens, with 95% of those ages 13 to 17 saying they have ever used it, according to a Center survey conducted April 14-May 4, 2022, that asked about 10 online platforms. Two-thirds of teens report using TikTok, followed by roughly six-in-ten who say they use Instagram (62%) and Snapchat (59%). Much smaller shares of teens say they have ever used Twitter (23%), Twitch (20%), WhatsApp (17%), Reddit (14%) and Tumblr (5%).
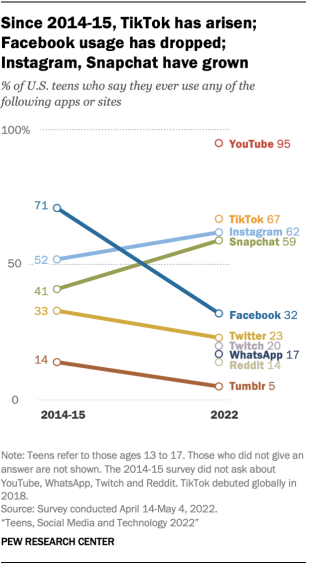
Facebook use among teens dropped from 71% in 2014-15 to 32% in 2022. Twitter and Tumblr also experienced declines in teen users during that span, but Instagram and Snapchat saw notable increases.
TikTok use is more common among Black teens and among teen girls. For example, roughly eight-in-ten Black teens (81%) say they use TikTok, compared with 71% of Hispanic teens and 62% of White teens. And Hispanic teens (29%) are more likely than Black (19%) or White teens (10%) to report using WhatsApp. (There were not enough Asian teens in the sample to analyze separately.)
Teens’ use of certain social media platforms also varies by gender. Teen girls are more likely than teen boys to report using TikTok (73% vs. 60%), Instagram (69% vs. 55%) and Snapchat (64% vs. 54%). Boys are more likely than girls to report using YouTube (97% vs. 92%), Twitch (26% vs. 13%) and Reddit (20% vs. 8%).
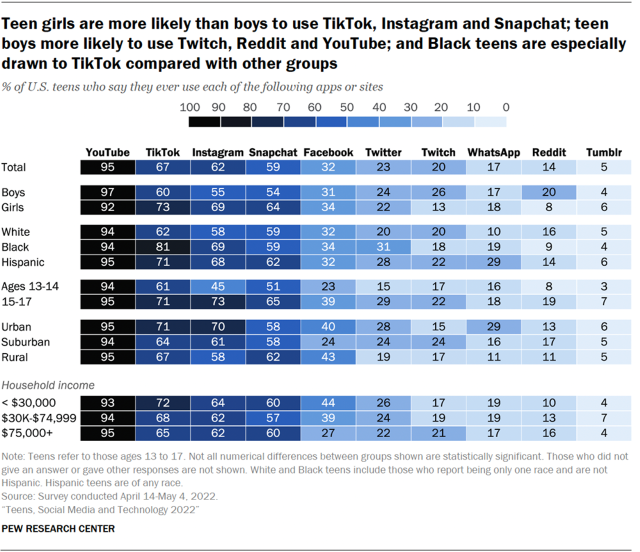
Majorities of teens use YouTube and TikTok every day, and some report using these sites almost constantly. About three-quarters of teens (77%) say they use YouTube daily, while a smaller majority of teens (58%) say the same about TikTok. About half of teens use Instagram (50%) or Snapchat (51%) at least once a day, while 19% report daily use of Facebook.
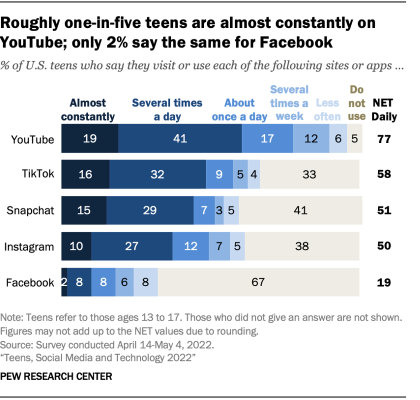
Some teens report using these platforms almost constantly. For example, 19% say they use YouTube almost constantly, while 16% and 15% say the same about TikTok and Snapchat, respectively.
More than half of teens say it would be difficult for them to give up social media. About a third of teens (36%) say they spend too much time on social media, while 55% say they spend about the right amount of time there and just 8% say they spend too little time. Girls are more likely than boys to say they spend too much time on social media (41% vs. 31%).
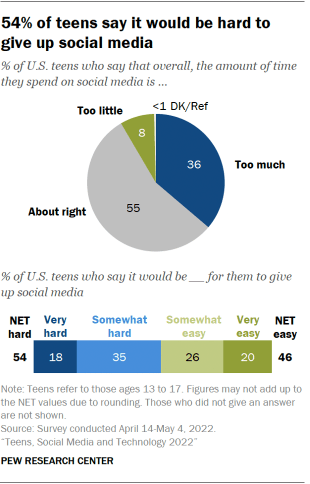
Teens are relatively divided over whether it would be hard or easy for them to give up social media. Some 54% say it would be very or somewhat hard, while 46% say it would be very or somewhat easy.
Girls are more likely than boys to say it would be difficult for them to give up social media (58% vs. 49%). Older teens are also more likely than younger teens to say this: 58% of those ages 15 to 17 say it would be very or somewhat hard to give up social media, compared with 48% of those ages 13 to 14.
Teens are more likely to say social media has had a negative effect on others than on themselves. Some 32% say social media has had a mostly negative effect on people their age, while 9% say this about social media’s effect on themselves.
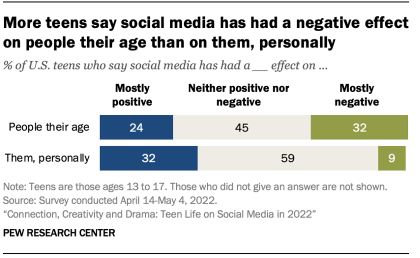
Conversely, teens are more likely to say these platforms have had a mostly positive impact on their own life than on those of their peers. About a third of teens (32%) say social media has had a mostly positive effect on them personally, while roughly a quarter (24%) say it has been positive for other people their age.
Still, the largest shares of teens say social media has had neither a positive nor negative effect on themselves (59%) or on other teens (45%). These patterns are consistent across demographic groups.
Teens are more likely to report positive than negative experiences in their social media use. Majorities of teens report experiencing each of the four positive experiences asked about: feeling more connected to what is going on in their friends’ lives (80%), like they have a place where they can show their creative side (71%), like they have people who can support them through tough times (67%), and that they are more accepted (58%).
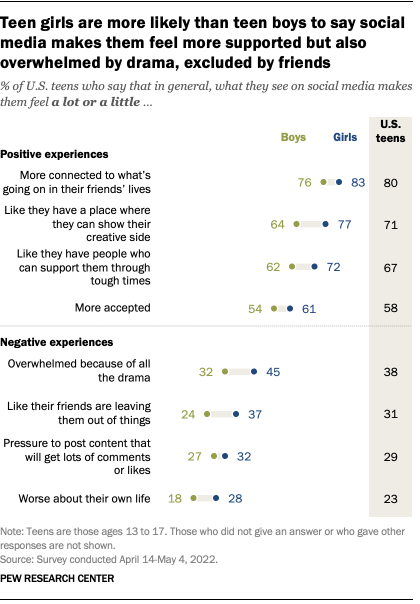
When it comes to negative experiences, 38% of teens say that what they see on social media makes them feel overwhelmed because of all the drama. Roughly three-in-ten say it makes them feel like their friends are leaving them out of things (31%) or feel pressure to post content that will get lots of comments or likes (29%). And 23% say that what they see on social media makes them feel worse about their own life.
There are several gender differences in the experiences teens report having while on social media. Teen girls are more likely than teen boys to say that what they see on social media makes them feel a lot like they have a place to express their creativity or like they have people who can support them. However, girls also report encountering some of the pressures at higher rates than boys. Some 45% of girls say they feel overwhelmed because of all the drama on social media, compared with 32% of boys. Girls are also more likely than boys to say social media has made them feel like their friends are leaving them out of things (37% vs. 24%) or feel worse about their own life (28% vs. 18%).
When it comes to abuse on social media platforms, many teens think criminal charges or permanent bans would help a lot. Half of teens think criminal charges or permanent bans for users who bully or harass others on social media would help a lot to reduce harassment and bullying on these platforms.
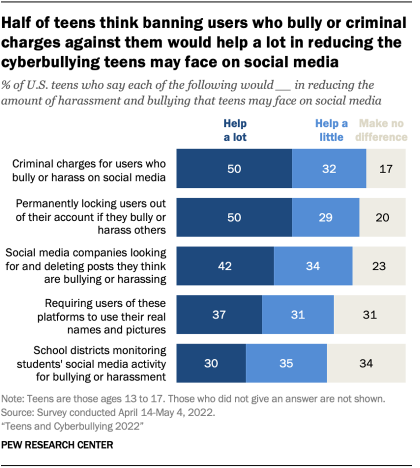
About four-in-ten teens say it would help a lot if social media companies proactively deleted abusive posts or required social media users to use their real names and pictures. Three-in-ten teens say it would help a lot if school districts monitored students’ social media activity for bullying or harassment.
Some teens – especially older girls – avoid posting certain things on social media because of fear of embarrassment or other reasons. Roughly four-in-ten teens say they often or sometimes decide not to post something on social media because they worry people might use it to embarrass them (40%) or because it does not align with how they like to represent themselves on these platforms (38%). A third of teens say they avoid posting certain things out of concern for offending others by what they say, while 27% say they avoid posting things because it could hurt their chances when applying for schools or jobs.
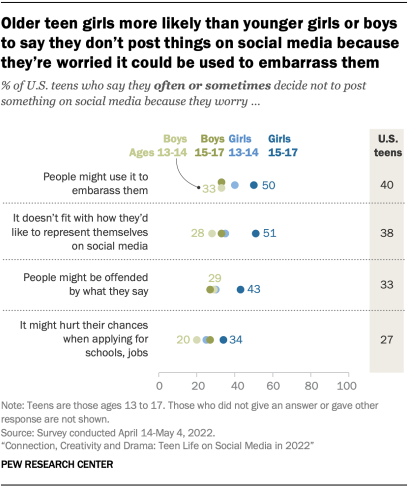
These concerns are more prevalent among older teen girls. For example, roughly half of girls ages 15 to 17 say they often or sometimes decide not to post something on social media because they worry people might use it to embarrass them (50%) or because it doesn’t fit with how they’d like to represent themselves on these sites (51%), compared with smaller shares among younger girls and among boys overall.
Many teens do not feel like they are in the driver’s seat when it comes to controlling what information social media companies collect about them. Six-in-ten teens say they think they have little (40%) or no control (20%) over the personal information that social media companies collect about them. Another 26% aren’t sure how much control they have. Just 14% of teens think they have a lot of control.
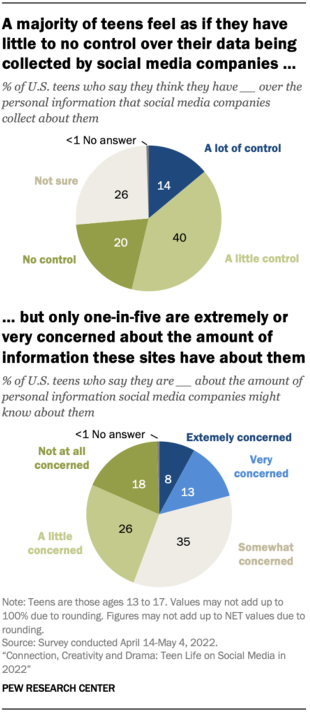
Despite many feeling a lack of control, teens are largely unconcerned about companies collecting their information. Only 8% are extremely concerned about the amount of personal information that social media companies might have and 13% are very concerned. Still, 44% of teens say they have little or no concern about how much these companies might know about them.
Only around one-in-five teens think their parents are highly worried about their use of social media. Some 22% of teens think their parents are extremely or very worried about them using social media. But a larger share of teens (41%) think their parents are either not at all (16%) or a little worried (25%) about them using social media. About a quarter of teens (27%) fall more in the middle, saying they think their parents are somewhat worried.
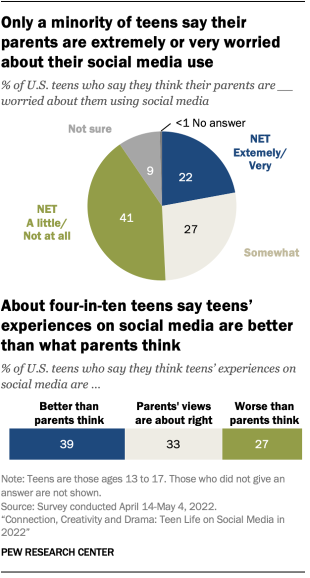
Many teens also believe there is a disconnect between parental perceptions of social media and teens’ lived realities. Some 39% of teens say their experiences on social media are better than parents think, and 27% say their experiences are worse. A third of teens say parents’ views are about right.
Nearly half of parents with teens (46%) are highly worried that their child could be exposed to explicit content on social media. Parents of teens are more likely to be extremely or very concerned about this than about social media causing mental health issues like anxiety, depression or lower self-esteem. Some parents also fret about time management problems for their teen stemming from social media use, such as wasting time on these sites (42%) and being distracted from completing homework (38%).
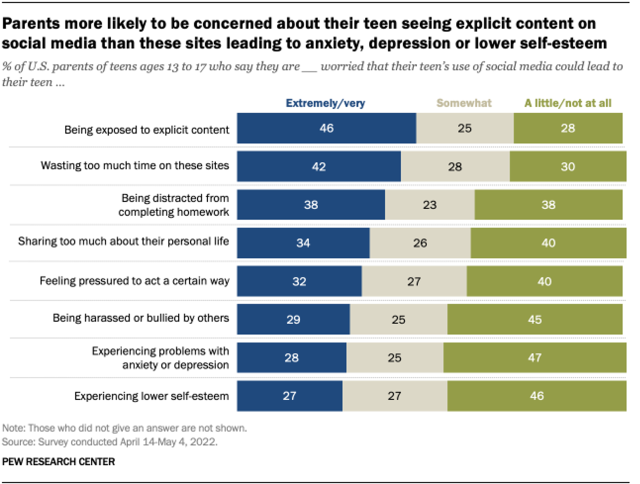
Note: Here are the questions used for this report, along with responses, and its methodology .
CORRECTION (May 17, 2023): In a previous version of this post, the percentages of teens using Instagram and Snapchat daily were transposed in the text. The original chart was correct. This change does not substantively affect the analysis.
- Age & Generations
- Age, Generations & Tech
- Internet & Technology
- Platforms & Services
- Social Media
- Teens & Tech
- Teens & Youth
Emily A. Vogels is a former research associate focusing on internet and technology at Pew Research Center
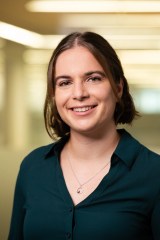
Risa Gelles-Watnick is a research analyst focusing on internet and technology research at Pew Research Center
How Teens and Parents Approach Screen Time
Who are you the art and science of measuring identity, u.s. centenarian population is projected to quadruple over the next 30 years, older workers are growing in number and earning higher wages, teens, social media and technology 2023, most popular.
1615 L St. NW, Suite 800 Washington, DC 20036 USA (+1) 202-419-4300 | Main (+1) 202-857-8562 | Fax (+1) 202-419-4372 | Media Inquiries
Research Topics
- Coronavirus (COVID-19)
- Economy & Work
- Family & Relationships
- Gender & LGBTQ
- Immigration & Migration
- International Affairs
- Methodological Research
- News Habits & Media
- Non-U.S. Governments
- Other Topics
- Politics & Policy
- Race & Ethnicity
- Email Newsletters
ABOUT PEW RESEARCH CENTER Pew Research Center is a nonpartisan fact tank that informs the public about the issues, attitudes and trends shaping the world. It conducts public opinion polling, demographic research, media content analysis and other empirical social science research. Pew Research Center does not take policy positions. It is a subsidiary of The Pew Charitable Trusts .
Copyright 2024 Pew Research Center
Terms & Conditions
Privacy Policy
Cookie Settings
Reprints, Permissions & Use Policy

IMAGES
VIDEO
COMMENTS
In terms of bibliometric analysis of social media addiction research, few studies have attempted to review the existing literature in the domain extensively. ... The study reveals that there is a lack of screening instruments and diagnostic criteria to assess social media addiction. Validated DSM-V-based instruments could shed light on the ...
Social media addiction (SMA) led to the formation of health-threatening behaviors that can have a negative impact on the quality of life and well-being. ... Dwivedi Y, Nerur S. Advances in social media research: Past, present and future. Inf Syst Front. 2018; 20:531-58. [Google Scholar] 5. Leong LY, Ooi KB, Lee VH, Lee V, Hew Ju. A hybrid SEM ...
social media addiction is negatively associated, in which the. higher the addiction in social media, the lower the young. people's academic performance (Hou et al., 2019). This i s. because ...
As a result, social media addiction, a type of behavioral addiction related to the compulsive use of social media and associated with adverse outcomes, has been discussed by scholars and ...
The Internet addiction instruments from which SMA scales are generally derived initially focused on three dimensions related to obsession or salience, negative life impact, and lack of control. ... Social media addiction: ... A., & Bhatiasevi, V. (2022). Research trends in SMA and problematic social media use: A bibliometric analysis. Frontiers ...
Excessive and compulsive use of social media may lead to social media addiction (SMA). The main aim of this study was to investigate whether demographic factors (including age and gender), impulsivity, self-esteem, emotions, and attentional bias were risk factors associated with SMA. The study was conducted in a non-clinical sample of college ...
Problematic social media use has been identified as negatively impacting psychological and everyday functioning and has been identified as a possible behavioural addiction (social media addiction; SMA). Whether SMA can be classified as a distinct behavioural addiction has been debated within the literature, with some regarding SMA as a premature pathologisation of ordinary social media use ...
Other scales were utilised to screen participants for Social Media Addiction (n:1), Internet Gaming Disorder (n:5), Smartphone Addiction (n:6), and Internet Addiction (n:12). One scale was used to screen participants for both Internet Addiction and Internet Gaming Disorder [30]. The screening instrument list is shown in Table 1. Of the studies ...
Abstract. This work endeavours to present the important measures of social media addiction in the existing academic literature. It outlines the evolution of social media addiction measures in the last 10 years from specific to broader platforms. It discusses the important works and postulations referring to the concerned topic.
Social media bring not only benefits but also downsides, such as addictive behavior. While an ambivalent closed insecure attachment style has been prominently linked with internet and smartphone addiction, a similar analysis for social media addiction is still pending. This study aims to explore social media addiction, focusing on variations in attachment style, mental distress, and ...
The study was administered to 513 senior high students with social media accounts. It was found out that 294 out of 513 are social media addicts and mostly use Facebook. The majority of social ...
Excessive use of new technologies appears to be potentially addictive for a minority of young people. The aim of the present study was to examine the relationship between personal environment factors (self-esteem and subjective wellbeing) and behaviors (social network site [SNS] intensity and smartphone addiction) drawing upon the Social Cognitive Theory and utilizing structural equation ...
Much research has discovered how habitual social media use may lead to addiction and negatively affect adolescents' school performance, social behavior, and interpersonal relationships. The present study was conducted to review the extant literature in the domain of social media and analyze global research productivity during 2013-2022.
Social media addiction was measured using Dr Kimberly ... scholars reported Young's IAT as a reliable instrument for measuring internet addiction particularly among college ... & Primack, B. A. (2016). Association between social media use and depression among U.S. Young adults: Research article: Social media and depression. Depression and ...
4.2.3. Social Media Addiction . The Bergen Social Media Addiction Scale (BSMAS) was designed by Andreassen et al. to measure problematic social media use behaviours over 12 months. It consists of 6 items that are rated on a 5-point Likert scale, with options ranging from "very rarely" (1) to "very often" (5).
In 2015, the average social media user spent 1.7 h per day on social media in the USA and 1.5 h in the UK, with social media users in the Philippines having the highest daily use at 3.7 h . This suggests social media use has become an important leisure activity for many, allowing individuals to connect with one another online irrespective of ...
Therefore, the present study aims to develop and validate a new instrument for measuring social media addiction - that is, the Social Media Disorder (SMD) Scale. Currently, the research field of social media addiction largely lags behind research on game addiction.
The aim of this research is to develop a measurement tool with a high level of reliability and validity for determining the social media addiction levels of university students. The researcher created 54 items related to its structure through a literature review, and seven experts were consulted for their opinions of the form prepared by the researcher. The scale, graded in five categories ...
The Social Media Addiction Scale was developed by Arslan and Kırık (Citation 2013) and it comprises 25 questions designed to assess time spent on social media, the priority placed on social media platforms, and how the usage affects their interaction with people. For the DASS, the instrument contains 42 items for assessing depression, anxiety ...
In the current research, we examined the relations of social media addiction to college students' mental health and academic performance. and the role of self-esteem as a mediator for the ...
In a study conducted by Kirik et al. on social media addiction with 271 undergraduates, no significant difference was found in terms of gender either. There are also studies in the literature showing that social media addiction of men is higher than in women [30,31,32]. Participants in the current study were using social media for an average of ...
Congruent with the growth of social media use, there are also increasing worries that social media might lead to social anxiety in users (Jelenchick et al., 2013).Social anxiety is one's state of avoiding social interactions and appearing inhibited in such interactions with other people (Schlenker & Leary, 1982).Scholars indicated that social anxiety could arise from managing a large network ...
Twice a year, in an effort to get out ahead of digital addiction, students at Gustavus Adolphus College in St. Peter are encouraged to take a deeper look at their social media use and its impact ...
The prevalence of social media addiction has been estimated to be particularly high among adolescents and young people in developed countries.[10,11] India, the country with the largest youth population globally, has become a world leader in the adoption of smartphones and mobile data in the past 5 years, which has materialized the growth and ...
Girls are more likely than boys to say it would be difficult for them to give up social media (58% vs. 49%). Older teens are also more likely than younger teens to say this: 58% of those ages 15 to 17 say it would be very or somewhat hard to give up social media, compared with 48% of those ages 13 to 14. Teens are more likely to say social ...
The present study evaluated the psychometric properties of four instruments related to internet use, namely the Bergen Social Media Addiction Scale (BSMAS), Smartphone Application-Based Addiction ...