- Encyclopedia of Management
- Problem Solving

PROBLEM SOLVING

A managerial problem can be described as the gap between a given current state of affairs and a future desired state. Problem solving may then be thought of as the process of analyzing the situation and developing a solution to bridge the gap. While it is widely recognized that different diagnostic techniques are appropriate in different situations, problem solving as a formal analytical framework applies to all but the simplest managerial problems. The framework is analogous to the scientific method used in chemistry, astronomy, and the other physical sciences. In both cases, the purpose underlying the analytic process is to minimize the influence of the investigator's personal biases, maximize the likelihood of an accurate result, and facilitate communication among affected parties.
Problem solving was popularized by W. Edwards Deming and the expansion of the total quality management movement in the 1980s. While Deming described what he called the Shewhart cycle, the technique is more commonly known as the Deming Wheel or simply as the PDCA cycle. Regardless of the name, a problem solver is urged to follow a step-by-step approach to problem solving-plan, do, check, act (hence the PDCA acronym).
In the planning stage, a manager develops a working hypothesis about why a given problem exists and then develops a proposed solution to the problem. The second step is to implement, or do, the proposed remedy. Next, the manager studies or checks the result of the action taken. The focus of this review is to determine whether the proposed solution achieved the desired result-was the problem solved? The fourth step then depends upon the interpretation of the check on results. If the problem was solved, the manager acts to institutionalize the proposed solution. This might mean establishing controls or changing policy manuals to ensure that the new way of doing business continues. However, if the check indicates that the problem was not solved or was only partially corrected, the manager acts by initiating a new cycle. Indeed, the technique is represented as a cycle based on the belief that many problems are never fully solved. For example, suppose that the problem in a given manufacturing facility is determined to be that labor productivity is too low. A change in processing methods may be found to successfully increase labor productivity. However, this does not preclude additional increases in labor productivity. Therefore, the PDCA cycle suggests that managers should pursue a course of continuous improvement activity.
The problem-solving framework can be used in a wide variety of business situations, including both large-scale management-change initiatives and routine improvement or corrective activity. Indeed, management consultants may be thought of as professional problem solvers. By relying on the proven problem-solving framework, external consultants are often able to overcome their lack of specific industry experience or knowledge of an organization's internal dynamics to provide meaningful analysis and suggestions for improvement. To more fully explore the issues presented by problem solving, the four-step PDCA cycle is expanded to a nine-step framework in the next section.
Perhaps the only generalizable caveat regarding problem solving is to guard against overuse of the framework. For example, Florida Power & Light became well known for their problem-solving ability in the late 1980s. One of their most successful initiatives was to institute an aggressive tree-trimming program to anticipate and prevent power failure due to downed limbs falling on electrical lines during storms. They were so successful that they integrated the problem-solving framework into their day-to-day managerial decision making and organizational culture. While this resulted in well reasoned decisions, it also meant that implementing even simple changes like moving a filing cabinet closer to the people using it required an overly bureaucratic approval process. This phenomenon is commonly referred to as paralysis of analysis. Therefore, managers should remain aware of the costs in both time and resources associated with the problem-solving framework. Accordingly, the nine-step framework described below is offered as a suggested guide to problem solving. Managers should feel free to simplify the framework as appropriate given their particular situation.
THE PROBLEM-SOLVING FRAMEWORK
Problem identification..
Although business problems in the form of a broken piece of machinery or an irate customer are readily apparent, many problems present themselves in a more subtle fashion. For example, if a firm's overall sales are increasing, but its percentage of market share is declining, there is no attention-grabbing incident to indicate that a problem exists. However, the problem-solving framework is still helpful in analyzing the current state of affairs and developing a management intervention to guide the firm toward the future desired state. Therefore, a solid approach to problem solving begins with a solid approach to problem identification. Whatever techniques are used, a firm's approach to problem identification should address three common identification shortfalls. First and most obviously, the firm wants to avoid being blindsided. Many problems develop over time; however, unless the firm is paying attention, warning signals may go unheeded until it is too late to effectively respond. A second common error of problem identification is not appropriating properly. This means that although a firm recognizes that an issue exists, they do not recognize the significance of the problem and fail to dedicate sufficient resources to its solution. It can be argued that not prioritizing properly has kept many traditional retail firms from responding effectively to emerging internet-based competitors. Finally, a third common error in problem identification is overreaction-the Chicken Little syndrome. Just as every falling acorn does not indicate that the sky is falling, neither does every customer complaint indicates that a crisis exists. Therefore, a firm's problem identification methods should strive to present an accurate assessment of the problems and opportunities facing the firm.
While no specific problem-identification technique will be appropriate for every situation, there are several techniques that are widely applicable. Two of the most useful techniques are statistical process control (SPC) and benchmarking. SPC is commonly used in the repetitive manufacturing industries, but can also prove useful in any stable production or service-delivery setting. A well formulated SPC program serves to inform managers when their operational processes are performing as expected and when something unexpected is introducing variation in process outputs. A simplified version of SPC is to examine performance outliers-those instances when performance was unusually poor or unusually good. It is believed that determining what went wrong, or conversely what went right, may inspire process or product modifications. Competitive benchmarking allows managers to keep tabs on their competition and thereby gauge their customers' evolving expectations. For instance, benchmarking might involve reverse engineering-disassembling a competitor's product-to study its design features and estimate the competitor's manufacturing costs. Texas Nameplate Company, Inc., a 1998 Malcolm Baldrige National Quality Award winner, uses competitive benchmarking by periodically ordering products from their competitors to compare their delivery-time performance.
Additional listening and problem identification techniques include the time-tested management-by-walking-around, revamped with a Japanese influence as going to gemba. The technique suggests that managers go to where the action is-to the production floor, point of delivery, or even to the customer's facilities to directly observe how things are done and how the product is used. Other methods include active solicitation of customer complaints and feedback. Bennigan's Restaurants offer a five-dollar credit toward future purchases to randomly selected customers who respond to telephone surveys on their satisfaction with their most recent restaurant visit. Granite Rock Company, a 1992 Baldrige Award winner, goes even farther by allowing customers to choose not to pay for any item that fails to meet their expectations. All that Granite Rock asks in return is an explanation of why the product was unsatisfactory.
PROBLEM VERIFICATION.
The amount of resources that should be dedicated to verification will vary greatly depending upon how the problem itself is manifested. If the problem is straightforward and well-defined, only a cursory level of verification may be appropriate. However, many business problems are complex and ill defined. These situations may be similar to the case of a physician who is confronted with a patient that has self-diagnosed his medical condition. While considering the patient's claim, the doctor will conduct her own analysis to verify the diagnosis. Similarly, the need for verification is especially important when a manager is asked to step in and solve a problem that has been identified by someone else. The introduction of the manager's fresh perspective and the possibility of a hidden agenda on the part of the individual who initially identified the issue under consideration suggests that a "trust, but verify" approach may be prudent. Otherwise, the manager may eventually discover she has expended a great deal of time and effort pursuing a solution to the wrong problem.
In the case of particularly ambiguous problems, McKinsey & Company, a management-consulting group, uses a technique they call Forces at Work. In this analysis, McKinsey's consultants review the external pressures on the client firm arising from suppliers, customers, competitors, regulators, technology shifts, and substitute products. They then attempt to document the direction and magnitude of any changes in the various pressures on the firm. In addition, they review any internal changes, such as shifts in labor relations or changes in production technology. Finally, they look at how the various factors are impacting the way the firm designs, manufactures, distributes, sells, and services its products. Essentially, McKinsey attempts to create comprehensive before-and-after snapshots of their client's business environment. Focusing on the differences between the two, they hope to identify and clarify the nature of the challenges facing the firm.
PROBLEM DEFINITION.
The next step in problem solving is to formally define the problem to be addressed. This is a negotiation between the individuals tasked with solving the problem and the individuals who over-see their work. Essentially, the parties need to come to an agreement on what a solution to the problem will look like. Are the overseers anticipating an implementation plan, a fully operational production line, a recommendation for capital investment, or a new product design? What metrics are considered important-cycle time, material costs, market share, scrap rates, or warranty costs? Complex problems may be broken down into mutually exclusive and collectively exhaustive components, allowing each piece to be addressed separately. The negotiation should recognize that the scope of the problem that is defined will drive the resource requirements of the problem solvers. The more focused the problem definition, the fewer resources necessary to generate a solution. Finally, the time frame for problem analysis should also be established. Many business problems require an expedited or emergency response. This may mean that the problem solvers need to generate a temporary or interim solution to the problem before they can fully explore the underlying causes of the problem. Ensuring that the overseers recognize the limitations inherent in an interim solution serves to preserve the credibility of the problem solvers.
ROOT-CAUSE ANALYSIS.
Now that the problem has been formally defined, the next step is for the problem solvers to attempt to identify the causes of the problem. The ultimate goal is to uncover the root cause or causes of the problem. The root cause is defined as that condition or event that, if corrected or eliminated, would prevent the problem from occurring. However, the problem solver should focus on potential root causes they are within the realm of potential control. For example, finding that a particular weight of motor oil is insufficient to protect an engine from overheating readily leads to an actionable plan for improvement. Finding that the root cause of a problem is gravity does not.
A common technique for generating potential root causes is the cause-and-effect diagram (also known as the fishbone or Ishikawa diagram). Using the diagram as a brainstorming tool, problem solvers traditionally review how the characteristics or operation of raw materials, labor inputs, equipment, physical environment, and management policies might cause the identified problem. Each branch of the diagram then becomes a statement of a causal hypothesis. For example, one branch of the diagram might suggest that low salaries are leading to high employee turnover, which in turn results in inexperienced operators running the machinery, which leads to a high scrap rate and ultimately higher material costs. This analysis suggests that to address the problem of high material costs, the firm may have to address the root cause of insufficient salaries.
Collection and examination of data may also lead the problem solver toward causal hypotheses. Check sheets, scatter plots, Pareto diagrams, data stratification, and a number of other graphical and statistical tools can aid problem solvers as they look for relationships between the problems identified and various input variables. Patterns in the data, changes in a variable over time, or comparisons to similar systems may all be useful in developing working theories about why something is happening. The problem solver should also consider the possibility of multiple causes or interaction effects. Perhaps the problem manifests only when a specific event occurs and certain conditions are met-the temperature is above 85 degrees or the ambient humidity is abnormally low.
Once the problem solver has identified the likely root causes of the problem, an examination of the available evidence should be used to confirm or disconfirm which potential causes actually are present and impacting the performance under consideration. This might entail developing an experiment where the candidate cause is controlled to determine whether its manipulation influences the presence of the problem. At this stage of the analysis, the problem solver should remain open to disconfirming evidence. Many elegant theories fail to achieve the necessary confirmation when put to the test. At this stage of the analysis it is also common for the problem solver to discover simple, easily implemented actions that will solve all or part of the problem. If this occurs, then clearly the problem solver should grasp the opportunity to "pick the low hanging fruit." Even if only a small component of the problem is solved, these interim wins serve to build momentum and add credibility to the problem-solving process.
ALTERNATIVE GENERATION.
Once the root causes of the problem have been identified, the problem solver can concentrate on developing approaches to prevent, eliminate, or control them. This is a creative process. The problem solver should feel free to challenge assumptions about how business was conducted in the past. At times, an effective approach is to generalize the relationship between the cause and the problem. Then the problem solver can look for similar relationships between other cause and effects that might provide insight on how to address the issues at hand. In general, it is useful to attempt to generate multiple candidate solutions. By keeping the creative process going, even after a viable solution is proposed, the problem solver retains the possibility of identifying a more effective or less expensive solution to the problem.
EVALUATION OF ALTERNATIVES.
Assuming that the problem was well defined, evaluation of the effectiveness of alternative solutions should be relatively straightforward. The issue is simply to what extent each alternative alleviates the problem. Using the metrics previously identified as important for judging success, the various alternatives can generally be directly compared. However, in addition to simply measuring the end result, the problem solvers may also want to consider the resources necessary to implement each solution. Organizations are made up of real people, with real strengths and weaknesses. A given solution may require competencies or access to finite resources that simply do not exist in the organization. In addition, there may be political considerations within the organization that influence the desirability of one alternative over another. Therefore, the problem solver may want to consider both the tangible and intangible benefits and costs of each alternative.
IMPLEMENTATION.
A very common problem-solving failure is for firms to stop once the plan of action is developed. Regardless of how good the plan is, it is useless unless it is implemented. Therefore, once a specific course of action has been approved, it should continue to receive the necessary attention and support to achieve success. The work should be broken down into tasks that can be assigned and managed. Specific mile-stones with target dates for completion should be established. Traditional project management techniques, such as the critical path method (CPM) or the program evaluation and review technique (PERT) are very useful to oversee implementation efforts.
POST-IMPLEMENTATION REVIEW.
Another common failure is for firms to simply move on after a solution has been implemented. At a minimum, a post-implementation evaluation of whether or not the problem has been solved should be conducted. If appropriate and using the metrics that were established earlier, this process should again be relatively straightforward-were the expected results achieved? The review can also determine whether additional improvement activities are justified. As the PDCA cycle suggests, some problems are never solved, they are only diminished. If the issue at hand is of that nature, then initiating a new cycle of problem-solving activity may be appropriate.
A secondary consideration for the post-implementation review is a debriefing of the problem solvers themselves. By its very nature, problem solving often presents managers with novel situations. As a consequence, the problem-solving environment is generally rich in learning opportunities. To the extent that such learning can be captured and shared throughout the organization, the management capital of the firm can be enhanced. In addition, a debriefing may also provide valuable insights into the firm's problem-solving process itself. Given the firm's unique competitive environment, knowing what worked and what did not may help focus future problem-solving initiatives.
INSTITUTIONALIZATION AND CONTROL.
The final step in problem solving is to institutionalize the results of the initiative. It is natural for any system to degrade over time. Therefore, any changes made as a result of the problem-solving effort should be locked in before they are lost. This might entail amending policy manuals, establishing new control metrics, or even rewriting job descriptions. In addition, the firm should also consider whether the problem addressed in the initiative at hand is an isolated incident or whether the solution can be leveraged throughout the organization. Frequently, similar problems are present in other departments or other geographic locations. If this is the case, institutionalization might involve transferring the newly developed practices to these new settings.
SEE ALSO: Project Management
Daniel R. Heiser
Revised by Badie N. Farah
FURTHER READING:
Deming, W. Edwards. Out of the Crisis. Cambridge: Massachusetts Institute of Technology, Center for Advanced Engineering Study, 1992.
Ketola, Jeanne and Kathy Roberts. Correct! Prevent! Improve!: Driving Improvement Through Problem Solving and Corrective and Preventive Action. Milwaukee: ASQ Quality Press, 2003.
National Institute of Standards and Technology. "Award Recipients." Malcolm Baldrige National Quality Award Program, 1999. Available from http://www.quality.nist.gov.
Rasiel, Ethan M. The McKinsey Mind: Using the Techniques of the World's Top Strategic Consultants to Help You and Your Business. New York: McGraw-Hill, 2001.
——. The McKinsey Way—Understanding and Implementing the Problem-Solving Tools and Management Techniques of the World's Top Strategic Consulting Firm. New York: McGraw-Hill, 1999.
Smith, Gerald F. Quality Problem Solving. Milwaukee: ASQ Quality Press, 1998.
User Contributions:
Comment about this article, ask questions, or add new information about this topic:.
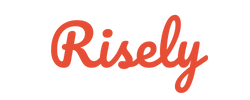
Problem Solving 101 for Managers: 5 Essential Skills and Tips
Understanding problem solving, the 5 essential skills for effective problem solving, tips for successful problem solving, case studies of successful problem solving in the business world, developing your problem-solving skills and mindset as a manager.
Other Related Blogs
What is problem solving for managers?
The importance of problem solving for managers, analytical thinking, communication skills, emotional intelligence, creative thinking, adaptability and flexibility.
- Just in Time Training: Boost Performance with Timely Learning Resources
- Problem-solving in Leadership: How to Master the 5 Key Skills
- 6 Effective Employee Development Ideas For Managers
- The Power of Storytelling in Training: Top 6 Tips
- Effective 5-Step Paced Decision Making Model For Managers
- 6 Must Read Growth Mindset Books For People Managers
- Leadership Is A Continuous Learning Process: How Do Successful Leaders Focus
- Creating an inclusive workplace: How to welcome new team members effectively
- Assertive communication styles: Learn about your communication style as a manager
- 9 Workplace Communication Problems: How Can Managers Beat Them?
Brainstorm Possible Solutions
Evaluate and choose the best solution, monitor the progress of implemented solutions, learn from the outcome and make adjustments.
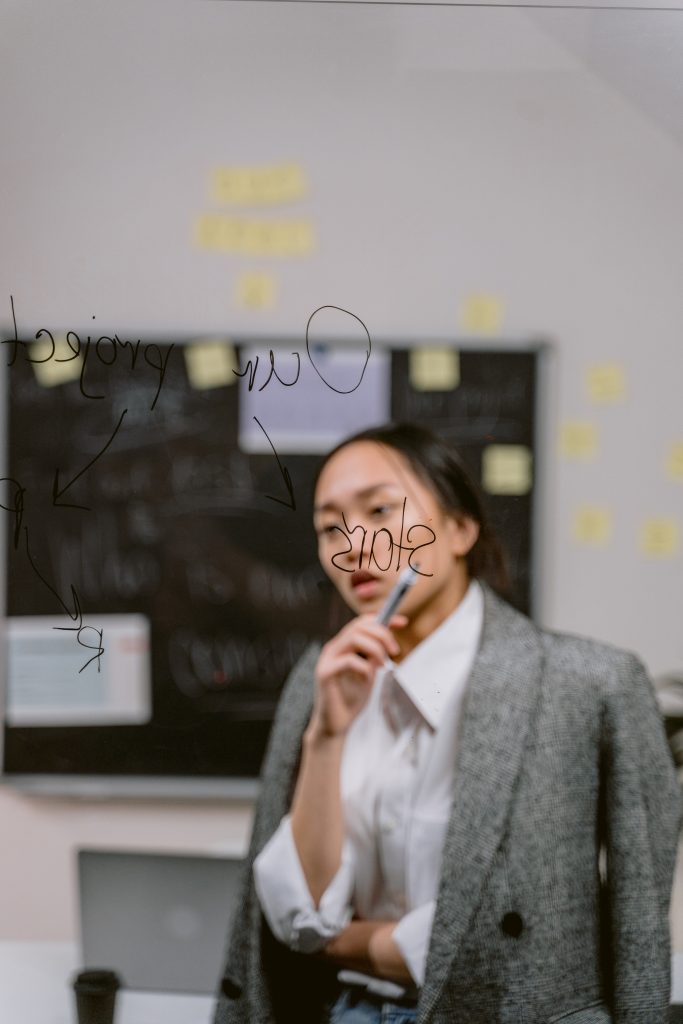
How Amazon used problem-solving Skills to Launch its Amazon Go Stores
The importance of problem-solving in the growth of zoom, the power of collaborative problem solving, practicing computational thinking for better problem solving, test your problem-solving skills, ready to take your problem-solving skills to the next level.
Download our free toolkit on developing effective problem-solving skills for managers to get started today!
Download Now
What are strong problem solving skills?
What are problem-solving skills of leaders, why problem-solving is important for managers.
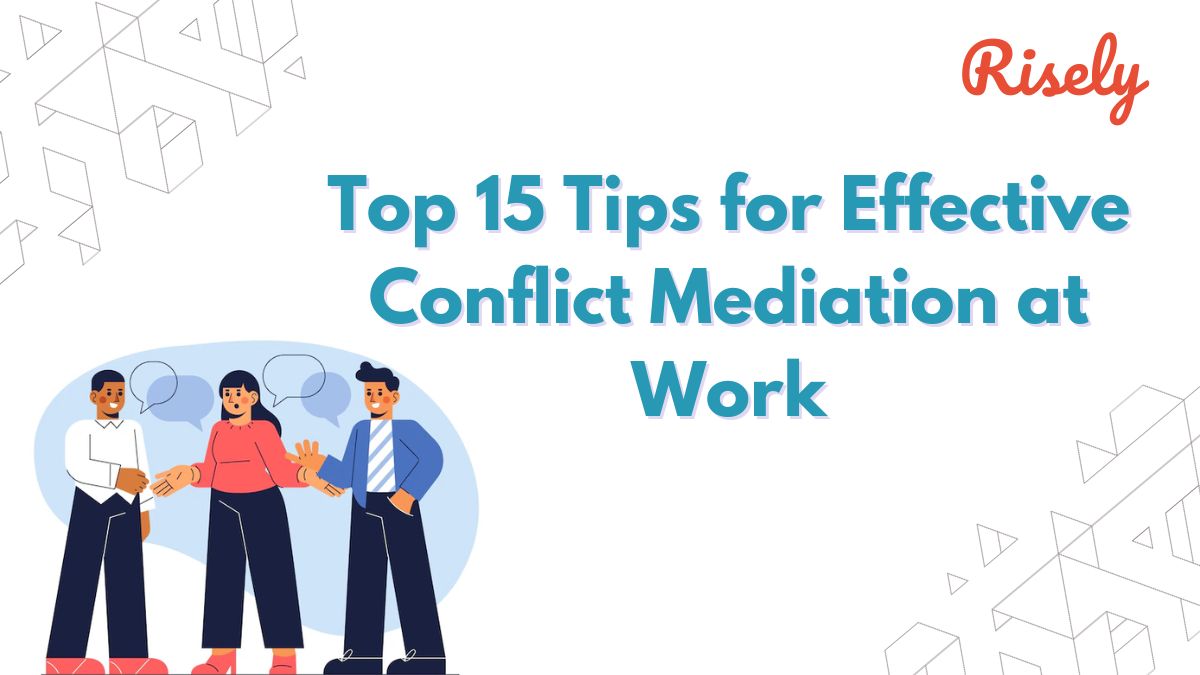
Top 15 Tips for Effective Conflict Mediation at Work
Top 10 games for negotiation skills to make you a better leader, manager effectiveness: a complete guide for managers in 2024, 5 proven ways managers can build collaboration in a team.
Last updated 27/06/24: Online ordering is currently unavailable due to technical issues. We apologise for any delays responding to customers while we resolve this. For further updates please visit our website: https://www.cambridge.org/news-and-insights/technical-incident
We use cookies to distinguish you from other users and to provide you with a better experience on our websites. Close this message to accept cookies or find out how to manage your cookie settings .
Login Alert

- > Managerial Economics
- > Nature, scope and methods of managerial economics
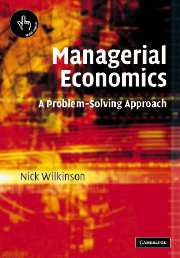
Book contents
- Frontmatter
- Acknowledgements
- Detailed contents
- PART I INTRODUCTION
- Chapter 1 Nature, scope and methods of managerial economics
- Chapter 2 The theory of the firm
- PART II DEMAND ANALYSIS
- PART III PRODUCTION AND COST ANALYSIS
- PART IV STRATEGY ANALYSIS
Chapter 1 - Nature, scope and methods of managerial economics
Published online by Cambridge University Press: 05 June 2012
To introduce and define managerial economics.
To outline the types of issue which are addressed by managerial economics.
To explain the difference between positive and normative economics.
To explain the relationship between managerial economics, economic theory and the decision sciences.
To explain how managerial economics is related to other disciplines in business, such as marketing and finance.
To identify the main subject areas in managerial economics, explain how they are related to each other, and describe how they are organized and presented in the text.
To explain the methods used in the development of scientific theories and show their relevance to managerial economics.
To explain how economic theory is presented from a pedagogical viewpoint, and how this relates to the organization and presentation of the material in the text.
Introduction
What is managerial economics about? What kind of issues does it deal with? How can it help us make better decisions, in business or elsewhere? These are fundamental questions which any student may ask when first approaching the subject. It is therefore a good idea to make a start by examining a situation that has become increasingly high on the economic and political agenda on a global basis over many years; yet it is not a situation where it might seem at first sight that managerial economics is particularly relevant. We shall see, to the contrary, that the methods studied and implemented in managerial economics are vital to identifying solutions to the problems raised.
Access options
Save book to kindle.
To save this book to your Kindle, first ensure [email protected] is added to your Approved Personal Document E-mail List under your Personal Document Settings on the Manage Your Content and Devices page of your Amazon account. Then enter the ‘name’ part of your Kindle email address below. Find out more about saving to your Kindle .
Note you can select to save to either the @free.kindle.com or @kindle.com variations. ‘@free.kindle.com’ emails are free but can only be saved to your device when it is connected to wi-fi. ‘@kindle.com’ emails can be delivered even when you are not connected to wi-fi, but note that service fees apply.
Find out more about the Kindle Personal Document Service .
- Nature, scope and methods of managerial economics
- Nick Wilkinson , Richmond: The American International University in London
- Book: Managerial Economics
- Online publication: 05 June 2012
- Chapter DOI: https://doi.org/10.1017/CBO9780511810534.004
Save book to Dropbox
To save content items to your account, please confirm that you agree to abide by our usage policies. If this is the first time you use this feature, you will be asked to authorise Cambridge Core to connect with your account. Find out more about saving content to Dropbox .
Save book to Google Drive
To save content items to your account, please confirm that you agree to abide by our usage policies. If this is the first time you use this feature, you will be asked to authorise Cambridge Core to connect with your account. Find out more about saving content to Google Drive .
1.3 The Nature of Management
- What is expected of a manager?
If organizations are to be successful in meeting these challenges, management must lead the way. With effective management, contemporary companies can accomplish a great deal toward becoming more competitive in the global environment. On the other hand, ineffective management dooms the organization to mediocrity and sometimes outright failure. Because of this, we turn now to a look at the nature of management. However, we want to point out that even though our focus is on managers, what we discuss is also relevant to the actions of nonmanagers. On the basis of this examination, we should be ready to begin our analysis of what managers can learn from the behavioral sciences to improve their effectiveness in a competitive environment.
What Is Management?
Many years ago, Mary Parker Follett defined management as “the art of getting things done through people.” A manager coordinates and oversees the work of others to accomplish ends they could not attain alone. Today this definition has been broadened. Management is generally defined as the process of planning, organizing, directing, and controlling the activities of employees in combination with other resources to accomplish organizational objectives. In a broad sense, then, the task of management is to facilitate the organization’s effectiveness and long-term goal attainment by coordinating and efficiently utilizing available resources. Based on this definition, it is clear that the topics of effectively managing individuals, groups, or organizational systems is relevant to anyone who must work with others to accomplish organizational objectives.
Management exists in virtually all goal-seeking organizations, whether they are public or private, large or small, profit-making or not-for-profit, socialist or capitalist. For many, the mark of an excellent company or organization is the quality of its managers.
Managerial Responsibilities
An important question often raised about managers is: What responsibilities do managers have in organizations? According to our definition, managers are involved in planning, organizing, directing, and controlling. Managers have described their responsibilities that can be aggregated into nine major types of activities. These include:
- Long-range planning . Managers occupying executive positions are frequently involved in strategic planning and development.
- Controlling . Managers evaluate and take corrective action concerning the allocation and use of human, financial, and material resources.
- Environmental scanning . Managers must continually watch for changes in the business environment and monitor business indicators such as returns on equity or investment, economic indicators, business cycles, and so forth.
- Supervision . Managers continually oversee the work of their subordinates.
- Coordinating . Managers often must coordinate the work of others both inside the work unit and out.
- Customer relations and marketing . Certain managers are involved in direct contact with customers and potential customers.
- Community relations . Contact must be maintained and nurtured with representatives from various constituencies outside the company, including state and federal agencies, local civic groups, and suppliers.
- Internal consulting. Some managers make use of their technical expertise to solve internal problems, acting as inside consultants for organizational change and development.
- Monitoring products and services . Managers get involved in planning, scheduling, and monitoring the design, development, production, and delivery of the organization’s products and services.
As we shall see, not every manager engages in all of these activities. Rather, different managers serve different roles and carry different responsibilities, depending upon where they are in the organizational hierarchy. We will begin by looking at several of the variations in managerial work.
Variations in Managerial Work
Although each manager may have a diverse set of responsibilities, including those mentioned above, the amount of time spent on each activity and the importance of that activity will vary considerably. The two most salient perceptions of a manager are (1) the manager’s level in the organizational hierarchy and (2) the type of department or function for which they are responsible. Let us briefly consider each of these.
Management by Level. We can distinguish three general levels of management: executives, middle management , and first-line management (see Exhibit 1.6 ). Executive managers are at the top of the hierarchy and are responsible for the entire organization, especially its strategic direction. Middle managers, who are at the middle of the hierarchy, are responsible for major departments and may supervise other lower-level managers. Finally, first-line managers supervise rank-and-file employees and carry out day-to-day activities within departments.
Exhibit 1.7 shows differences in managerial activities by hierarchical level. Senior executives will devote more of their time to conceptual issues, while first-line managers will concentrate their efforts on technical issues. For example, top managers rate high on such activities as long-range planning , monitoring business indicators, coordinating, and internal consulting. Lower-level managers, by contrast, rate high on supervising because their responsibility is to accomplish tasks through rank-and-file employees. Middle managers rate near the middle for all activities. We can distinguish three types of managerial skills: 8
- Technical skills . Managers must have the ability to use the tools, procedures, and techniques of their special areas. An accountant must have expertise in accounting principles, whereas a production manager must know operations management. These skills are the mechanics of the job.
- Human relations skills . Human relations skills involve the ability to work with people and understand employee motivation and group processes. These skills allow the manager to become involved with and lead his or her group.
- Conceptual skills . These skills represent a manager’s ability to organize and analyze information in order to improve organizational performance. They include the ability to see the organization as a whole and to understand how various parts fit together to work as an integrated unit. These skills are required to coordinate the departments and divisions successfully so that the entire organization can pull together.
As shown in Exhibit 1.7 , different levels of these skills are required at different stages of the managerial hierarchy. That is, success in executive positions requires far more conceptual skill and less use of technical skills in most (but not all) situations, whereas first-line managers generally require more technical skills and fewer conceptual skills. Note, however, that human or people skills remain important for success at all three levels in the hierarchy.
Management by Department or Function. In addition to level in the hierarchy, managerial responsibilities also differ with respect to the type of department or function. There are differences found for quality assurance, manufacturing, marketing, accounting and finance, and human resource management departments. For instance, manufacturing department managers will concentrate their efforts on products and services, controlling, and supervising. Marketing managers, in comparison, focus less on planning, coordinating, and consulting but more on customer relations and external contact. Managers in both accounting and human resource management departments rate high on long-range planning, but will spend less time on the organization’s products and service offerings. Managers in accounting and finance are also concerned with controlling and with monitoring performance indicators, while human resource managers provide consulting expertise, coordination, and external contacts. The emphasis on and intensity of managerial activities varies considerably by the department the manager is assigned to.
At a personal level, knowing that the mix of conceptual, human, and technical skills changes over time and that different functional areas require different levels of specific management activities can serve at least two important functions. First, if you choose to become a manager, knowing that the mix of skills changes over time can help you avoid a common complaint that often young employees want to think and act like a CEO before they have mastered being a first-line supervisor. Second, knowing the different mix of management activities by functional area can facilitate your selection of an area or areas that best match your skills and interests.
In many firms, managers are rotated through departments as they move up in the hierarchy. In this way they obtain a well-rounded perspective on the responsibilities of the various departments. In their day-to-day tasks they must emphasize the right activities for their departments and their managerial levels. Knowing what types of activity to emphasize is the core of the manager’s job. In any event, we shall return to this issue when we address the nature of individual differences in the next chapter.
The Twenty-First Century Manager
We discussed above many of the changes and challenges facing organizations in the twenty-first century. Because of changes such as these, the managers and executives of tomorrow will have to change their approaches to their jobs if they are to succeed in meeting the new challenges. In fact, their profiles may even look somewhat different than they often do today. Consider the five skills that Fast Company predicts that successful future managers, compared to the senior manager in the year 2000, will need. The five skills are: the ability to think of new solutions, being comfortable with chaos, an understanding of technology, high emotional intelligence, and the ability to work with people and technology together.
For the past several decades, executive profiles have typically looked like this: The person started out in finance with an undergraduate degree in accounting, then methodically worked their way up through the company from the controller’s office in a division, to running that division, to the top job. The military background shows. They are used to giving orders—and to having them obeyed. As head of the philanthropic efforts, they are important in the community. However, the first time the individual traveled overseas on business was as chief executive. Computers, which became ubiquitous, make them nervous. 9
Now compare this with predictions about what a twenty-first-century executive will look like:
Their undergraduate degree might be in French literature, but they also have a joint MBA/engineering degree. The individual started in research and was quickly picked out as a potential CEO. They are able to think creatively and thrives in a chaotic environment. They zigzagged from research to marketing to finance, are comfortable with technology and people, and have a high degree of emotional intelligence. The executive proved valuable in Brazil by turning around a failing joint venture. This person speaks multiple languages and is on a first-name basis with commerce ministers in half a dozen countries.
Clearly, the future holds considerable excitement and promise for future managers and executives who are properly prepared to meet the challenges. How do we prepare them? One study suggested that the manager of the future must be able to fill at least the following four roles: 10
Global strategist. Executives of the future must understand world markets and think internationally. They must have a capacity to identify unique business opportunities and then move quickly to exploit them.
Master of technology. Executives and managers of the future must be able to get the most out of emerging technologies, whether these technologies are in manufacturing, communications, marketing, or other areas.
Leadership that embraces vulnerability. The successful executive of the future will understand how to cut through red tape to get a job done, how to build bridges with key people from highly divergent backgrounds and points of view, and how to make coalitions and joint ventures work.
Follow-from-the-front motivator. Finally, the executive of tomorrow must understand group dynamics and how to counsel, coach, and command work teams and individuals so they perform at their best. Future organizations will place greater emphasis on teams and coordinated efforts, requiring managers to understand participative management techniques.
Great communicator. To this list of four, we would add that managers of the future must be great communicators. They must be able to communicate effectively with an increasingly diverse set of employees as well as customers, suppliers, and community and government leaders.
Whether these predictions are completely accurate is difficult to know. Suffice it to say that most futurists agree that the organizational world of the twenty-first century will likely resemble, to some extent, the portrait described here. The task for future managers, then, is to attempt to develop these requisite skills to the extent possible so they will be ready for the challenges of the next decade.
Concept Check
- Define management.
- How does the nature of management change according to one’s level and function in the organization?
As an Amazon Associate we earn from qualifying purchases.
This book may not be used in the training of large language models or otherwise be ingested into large language models or generative AI offerings without OpenStax's permission.
Want to cite, share, or modify this book? This book uses the Creative Commons Attribution License and you must attribute OpenStax.
Access for free at https://openstax.org/books/organizational-behavior/pages/1-introduction
- Authors: J. Stewart Black, David S. Bright
- Publisher/website: OpenStax
- Book title: Organizational Behavior
- Publication date: Jun 5, 2019
- Location: Houston, Texas
- Book URL: https://openstax.org/books/organizational-behavior/pages/1-introduction
- Section URL: https://openstax.org/books/organizational-behavior/pages/1-3-the-nature-of-management
© Jan 9, 2024 OpenStax. Textbook content produced by OpenStax is licensed under a Creative Commons Attribution License . The OpenStax name, OpenStax logo, OpenStax book covers, OpenStax CNX name, and OpenStax CNX logo are not subject to the Creative Commons license and may not be reproduced without the prior and express written consent of Rice University.
To read this content please select one of the options below:
Please note you do not have access to teaching notes, managerial problem solving: a cultural perspective.
Management Decision
ISSN : 0025-1747
Article publication date: 1 October 1994
Illustrates how organizational culture can stifle effective problem solving and decision making. In the organization in question this had critical implications for quality, staff retention and organizational change. The myopic, non‐innovative generation of apparent solutions to major organizational problems made the organization a victim, not a master, of change. Precedent determined action so that “more of the same” becomes the forte of the organization. Investigates the nature of the organizational culture and the mechanisms of influence it exerted over the problem‐solving process. The research utilized a qualitative methodology well suited to investigating organizational culture and was based in a civil service agency. Suggests an alternative, or model, culture, the adoption of which would enable the organization to cope with environmental and organizational change. Offers recommendations which derive from the research.
- Corporate culture
- Decision making
- Organizational change
- Problem solving
- Public sector
Brooks, I. (1994), "Managerial Problem Solving: A Cultural Perspective", Management Decision , Vol. 32 No. 7, pp. 53-59. https://doi.org/10.1108/00251749410068120
Copyright © 1994, MCB UP Limited
Related articles
All feedback is valuable.
Please share your general feedback
Report an issue or find answers to frequently asked questions
Contact Customer Support

- > Economics
- > Financial Analytics
What is Managerial Economics? Definition, Types, Nature, Principles, and Scope
- Ritesh Pathak
- Nov 26, 2020
- Updated on: Nov 21, 2023
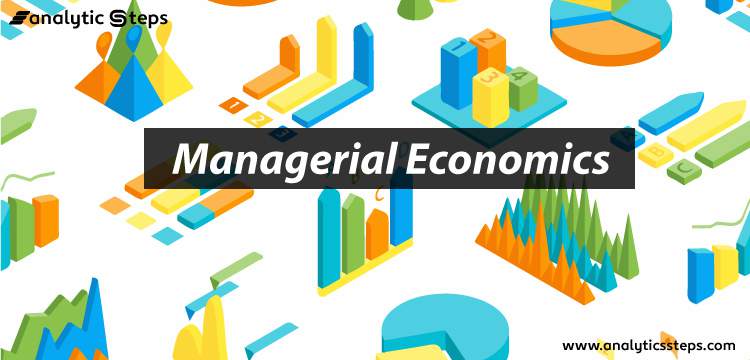
Businesses run on various theories that are explained in Economics. Managerial Economics is the stream of management studies that emphasizes solving problems in businesses using the theories in micro and macroeconomics . This branch of economics is used by firms to not only find a solution to problems in daily running but also for long-term planning. We can also say that Managerial economics is a practical application of theories in economics.
“Managerial economics is concerned with the application of economic concepts and economic analysis to the problems of formulating rational managerial decisions.” - Edwin Mansfield, Economics Professor, University of Pennsylvania
We should also look here at What is economics? Economics is an inevitable part of any business. All the business assumptions, forecasting, and investments are based on this one single concept. Investopedia explains “Economics is a social science concerned with the production, distribution, and consumption of goods and services. It studies how individuals, businesses, governments, and nations make choices about how to allocate resources.” So, theories in economics are not just some statements written but rather they act as fuel for a firm. In the broader picture, economics also helps nations in policy formation.
So, in this blog, we will discuss the branch of economics that helps businesses to find a solution to almost every problem they may face. We will discuss the definition of managerial economics, its nature, its scope in businesses, and the principles of managerial economics.
Also Read | 5 Key elements of Financial Analysis
Definition of Managerial Economics
Managerial economics is defined as the branch of economics which deals with the application of various concepts, theories, methodologies of economics to solve practical problems in business management . It is also reckoned as the amalgamation of economic theories and business practices to ease the process of decision making. Managerial economics is also said to cover the gap between the problems of logic and problems of policy.
Managerial economics is used to find a rational solution to problems faced by firms. These problems include issues around demand, cost, production, marketing, and it is used also for future planning. The best thing about managerial economics is that it has a logical solution to almost every problem that may arise during business management and that too by sticking to the microeconomic policies of the firm.
When we talk of managerial economics as a subject, it is a branch of management studies that emphasizes solving business problems using theories of micro and macroeconomics . Spencer and Siegelman have defined the subject as “the integration of economic theory with business practice to facilitate decision making and planning by management.” The study of managerial economics helps the students to enhance their analytical skills, developing a mindset that enables them to find rational solutions.
Nature of Managerial Economics
We know about managerial economics like what it is and how different people define it. Managerial Economics is an essential scholastic field. It can be termed as a science in the sense that it fulfills the criteria of being a science.
We all know science as a systematic body of knowledge and it is based on methodological observations. Similarly, Managerial Economics is also a science of making decisions and finding alternatives, keeping the scarce of resources in mind.
In science, we arrive at any conclusion after continuous experimentation. Similarly, in managerial economics policies are formed after constant testing and trailing.
In science, principles are universally acceptable and in managerial economics, policies are universally applicable at least partially if not fully.
Nature of Managerial Economics
We will now look at the characteristics of managerial economics in brief.
Art and Science
Managerial Economics requires a lot of creativity and logical thinking to come up with a solution. A managerial economist should possess the art of utilizing his capabilities, knowledge, and skills to achieve the organizational objective. Managerial Economics is also considered as a stream of science as it involves the application of different economic principles, techniques, and methods, to solve business problems.
Microeconomics
In managerial economics, problems of a particular organization are looked upon rather than focusing on the whole economy. Therefore it is termed as a part of microeconomics.
Uses Macroeconomics
Any organization operates in a market that is a part of the whole economy, so external environments affect the decisions within the organization. Managerial Economics uses the concepts of macroeconomics to solve problems. Managers analyze the macroeconomic factors like market conditions, economic reforms, government policies to understand their impact on the organization.
Multi-disciplinary
Managerial Economics uses different tools and principles from different disciplines like accounting, finance, statistics, mathematics, production, operation research, human resource, marketing, etc. This helps in coming up with a perfect solution.
Management oriented and pragmatic
Managerial economics is a tool in the hands of managers that aids them in finding appropriate solutions to business-related problems and uncertainties. As mentioned above, managerial economics also helps in goal establishment, policy formation, and effective decision making. It is a practical approach to find solutions.
Types of Managerial Economics
Everyone has their perceiving ability, so the same goes with managerial economics. All managers perceive the concept of managerial economics differently. For some, customers’ satisfaction can be the priority while some may focus on efficient production. This leads us to different types of managerial economics. So, let us explore the different approaches to managerial economics.
Liberal Managerialism
Market is a free and democratic place in terms of decision making. Customers get a lot many options to choose from. So, companies have to modify their policies according to consumers’ demands and market trends. If not done so, it may result in business failures. This is what we call liberal managerialism.
Normative Managerialism
The normative view of managerial economics means that the decisions taken by the administration would be normal, based on real-life experiences and practices. The decisions reflect a practical approach regarding product design, forecasting, marketing, supply and demand analysis, recruitments, and everything else that is concerned with the growth of a business.
Radical Managerialism
Radical managerialism means to come up with revolutionary solutions. Sometimes, when the conventional approach to a problem doesn’t work, radical managerialism may have the solution. However, it requires the manager to possess some extraordinary skills and thinking to look beyond. In radical managerialism, consumer needs and satisfaction are prioritized over profit maximization.
So, these were the three different types of managerial economics. These are decided based on the different approaches by managers.
Recommended Blog: Stripe vs Paypal: How to Decide the Right Payment Platform for Online Business
Principles of Managerial Economics
The great macroeconomist N. Gregory Mankiw has given ten principles to explain the significance of managerial economics in business operations which can be further classified into three categories.
Principles of How People Make Decisions
Based on the real-life decision-making processes, four principles are recalled in Managerial Economics.
1. People Face Tradeoffs
There are enormous options in the market. So, people have to make choices among the various options available.
2. Opportunity Cost
Every decision involves an opportunity cost that is the cost of those options which we let go of while selecting the most appropriate one.
3. Rational People Think at the Margin
When we make choices from the various options available and before investing the capital or resources we look at the profit margin we would make in the investment.
4. People Respond to Incentives
It is human nature to look for something extra while purchasing something. Decision-making is affected by the incentives attached to a particular product or service. Positive incentive motivates people to opt for the particular product while negative incentive discourages.
Principles of How People Interact
Communication with the audience plays a vital role in good performance. Over the years, organizations have realized the need to communicate well with their audience. Based on this, three principles are given in Managerial Economics.
1. Trade can Make Everyone Better Off
This principle states that trade is a medium to exchange services and products. Everyone gets a fair chance to offer products and services which they are good at making and also to purchase those products and services. Also Read: The success story of Delhivery
2. Markets Are Usually A Good Way to Organize Economic Activity
Market is a place where buyers and sellers interact with each other. Consumers put in their demands and requirements and the producers decide on the production and supply of those products and services.
3. Government can better the market outcomes
Government intervenes in business operations whenever there are unfavorable market conditions like the current pandemic situation or also for the welfare of society. One example of the latter is deciding the minimum wage for laborers.
Referred Blog | How is India recovering from the economic slowdown .
Principle of How Economy Works as a Whole
Three principles are given to explain the role of the economy in the functioning of an organization.
1. A Country’s Standard of Living Depends on the Goods and Services produced
The role of organizations in the economic growth of a country is one of the major, so, the organizations must be capable enough to produce goods and services for the population. This ultimately raises the standard of living and also contributes to GDP growth.
2. Price Rises When Government Prints Too Much Money
If there is surplus money available with people, their spending capacity increases, ultimately leading to a rise in demand. When the producers are unable to meet the consumer’s demand, inflation takes place. Referred blog : What does the 24% shrink in India’s GDP mean?
3. Society Faces a Short-Run Tradeoff between Inflation and Unemployment
Government bring-in policies to tackle the problem of unemployment and boost the economy in the short run as well. This further leads to inflation.
Scope of Managerial Economics
Managerial Economics has a more narrow scope. It solves a firm’s problem using microeconomics. In the situation of scarce resources, managerial economics ensures that managers make effective and efficient decisions that are equally beneficial to customers, suppliers, and the organization. The fact of scarcity of resources gives rise to three fundamental questions-
What to produce?
How to produce?
For whom to produce?
To answer these questions, a firm makes use of managerial economics principles.
Managerial Economics is not only applicable to profit-making business organizations, but also to non- profit organizations such as hospitals, schools, government agencies, etc.
Read this article to know about the scope of Managerial Economics in detail.
We tried to explain Managerial Economics through this blog. The definition of Managerial Economics says that it is a branch of economics that deals with the application of various theories, concepts, and methodologies to solve business problems. It is said to cover the gap between problem of logic and problem of policy.
For any firm to be successful, it needs to solve its problems logically and rationally. Managerial Economics helps the managers to make effective and efficient decisions using the concepts of microeconomics. One of the top characteristics of Managerial Economics is that it uses the different factors of macroeconomics helping firms to act according to the market trends.
Share Blog :

Be a part of our Instagram community
Trending blogs
5 Factors Influencing Consumer Behavior
Elasticity of Demand and its Types
An Overview of Descriptive Analysis
What is PESTLE Analysis? Everything you need to know about it
5 Factors Affecting the Price Elasticity of Demand (PED)
6 Major Branches of Artificial Intelligence (AI)
Dijkstra’s Algorithm: The Shortest Path Algorithm
Different Types of Research Methods
Latest Comments
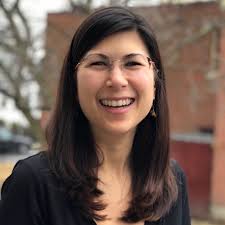
Mary Robinson
Good day to everyone reading my post, i'm here to appreciate a legitimate spell caster call Dr Kachi who can help you winning the lottery draw, i have never win a biggest amount in lottery unite the day i saw good reviews about DR Kachi how he has helped a lot of people in different ways both financially/martially and i have been playing Mega Million for 8years now, but things suddenly change the moment i contacted Dr Kachi and explained everything to me about the spell and I accepted. I followed his instructions and played the Mega Million with the numbers he gave me, now i am a proud lottery winner with the help of Dr Kachi spell, i win $640 Million Dollars in Mega Millions Ticket, i am making this known to everyone out there who have been trying all day to win the lottery jackpot, believe me this is the only way to win the lottery, this is the real secret we all have been searching for. I want to thank Dr Kachi for his endless help and his from the United States. you can contact via email [email protected] or through Text and Call Number: +1 (209) 893-8075 his website: https://drkachispellcaster.wixsite.com/my-site

johncase3976c2ff74a8e374e04
Hi there, everyone, I’m John, please to meet you all. I work as a security with a fair rent since I live with a family who doesn’t want me to live alone and have a good paying job. My goal is to build a good credit score to get a good credit card. In April I had 140 dollars or so collections from a gym saying I never canceled my membership. I wasn’t getting charged the monthly fee for it. So, I randomly go on credit karma and see the collections. Few days after I contacted PINNACLE CREDIT SPECIALIST, they increased my score to 809 and improved my credit profile by removing the collections, charge off and late payments. I just saw today it says no more derogatory on my credit Karma. My score went up. I sincerely acknowledge their relentless efforts and urge you to contact PINNACLE CREDIT SPECIALIST for any credit related issue. Contact info: [email protected] Or Call 409 231 0041. Honestly, I do not enjoy writing much, but I have let the world know about this genius that helped me.
Dara Pellar
TROPICAL DELIGHT RECOVERY- A TRUSTWORTHY CRYPTOCURRENCY RECOVERY HACKER. Tropical Delight Recovery has been a game changer in cryptocurrency recovery. They are extremely swift and efficient, ensuring that every operation runs smoothly and without difficulty. Their service is unparalleled in terms of dependability, and their customer care is always available and helpful. I've never felt more secure or certain that I could fully restore my crypto assets. Tropical Delight Recovery stands out in the business for their remarkable speed, efficiency, and dependability in recovering cryptocurrency, and they come highly recommended! You can contact them at: Whatsapp: +1(347). 913. 658 1, Signal: +1 .614 568.38.73 Telegram: @tropicaldelightrecoveryhacker Email : tropicaldelightrecoveryhacker @ out. Look . Website: htt. ps : // dev - t ropicaldelightrecovery .pantheonsite .io
vernonjesse8153eace3e9a25e4325
There was a time, I was a credit disaster, I knew absolutely nothing about how credit works. I’d pay bills late thinking paying late was better than not paying at all. To be honest, I didn’t even know negatives lasted on your reports for 7 years. Why did I pay late? Because at the time, I’d think I needed to buy something shiney and then pay bills. To further my reckless behaviors, I had my house foreclosed on. To be honest, I don’t know why I checked my credit, which ultimately led me to this site where I found PINNACLE CREDIT SPECIALIST, but I’m glad I did, reading as much testimonies as I could. My starting score was (low 500’s). All thanks to PINNACLE CREDIT SPECIALIST, as of today, I’m sitting at EX: 808, TU: 812 and my lowest is EQ: 801. If I can offer one piece of advice that helped me, I’ll advise you to contact PINNACLE by email: [email protected] Or call +1 (409) 231-0041. The first time I’m seeing my scores reach the 800 mark, that feeling is right up there with having my first child, marriage.
lauraani626b9c6da6ad7814e7c
HOW TO RECOVER LOST CRYPTO BACK FROM FAKE PLATFORMS. I’ve read many stories about GEO COORDINATES RECOVERY HACKER COMPANY. How the company is helping people recover their funds after losing them to scammers. It is indeed surprising to know you can recover all lost bitcoin back from scam companies or any from any fake investment site. I used to be a victim of an online scam trading platform where I invested about 97,000 usd worth of Btc and lost everything. GEO COORDINATES RECOVERY HACKER COMPANY. As the only trusted cyber security company, the hacker recovered all my lost money. I’m highly recommending them to everyone who wishes to recover their funds. You can also contact GEO COORDINATES RECOVERY HACKER COMPANY. Contact the following address: Email: geovcoordinateshacker@protonme Email: [email protected] Telegram ( @Geocoordinateshacker ) Website; https://geovcoordinateshac.wixsite.com/geo-coordinates-hack
michaelwatson108642225ff3ab3d44a1
I just want to share how I became a homeowner with the help of PINNACLE CREDIT SPECIALIST for fixing my credit report. The negative items on my credit report deprived me of so many opportunities alongside 5 derogatory and 2 collections which messed up my report and lowered my credit score to EQ: 547, TU 564, EX 521. After my mom told me about PINNACLE CREDIT SPECIALIST, I reached out to them immediately and complied with their proposal. After five days he helped me boost my credit score to EQ: 809, TU 801, EX 811 excellent standard and he also deleted all negative collections and derogatory ones on my credit report. These finally got me a mortgage for my new house and credit cards. Contact him now by email: [email protected] Or call +1 (409) 231-0041.

Managerial Problem Definition: A Descriptive Study of Problem Definers
PDF Version Also Available for Download.
Description
This research examines problem definition as the first step in a sequential problem solving process. Seventy-seven managers in four diverse organizations were studied to determine common characteristics of problem definers. Among the variables considered as differentiating problem definers from non-problem definers were cognitive style, personal need characteristics, preference for ideation, experience, level of management, and type and level of education. Six hypotheses were tested using the following instruments: the Problem Solving Inventory, the Myers-Briggs Type Indicator Schedule, the Preference for Ideation Scale, the Edwards Personal Preference Schedule, a Problem Definition Exercise, and a Personal Data Questionnaire. Among the managers studied, … continued below
Physical Description
ii, 136 leaves : ill.
Creation Information
Phillips Danielson, Waltraud August 1985.
This dissertation is part of the collection entitled: UNT Theses and Dissertations and was provided by the UNT Libraries to the UNT Digital Library , a digital repository hosted by the UNT Libraries . It has been viewed 2271 times. More information about this dissertation can be viewed below.
People and organizations associated with either the creation of this dissertation or its content.
- Phillips Danielson, Waltraud
- Powell, James Don Major Professor
Committee Members
- Starling, Jack Minor Professor
- Glass, James J. Minor Professor
- North Texas State University Publisher Info: www.unt.edu Place of Publication: Denton, Texas
Rights Holder
For guidance see Citations, Rights, Re-Use .
Provided By
Unt libraries.
The UNT Libraries serve the university and community by providing access to physical and online collections, fostering information literacy, supporting academic research, and much, much more.
Descriptive information to help identify this dissertation. Follow the links below to find similar items on the Digital Library.
Degree Information
- Department: College of Business
- Discipline: Organization Theory and Policy Analysis
- Level: Doctoral
- Name: Doctor of Philosophy
- PublicationType: Doctoral Dissertation
- Grantor: North Texas State University
This research examines problem definition as the first step in a sequential problem solving process. Seventy-seven managers in four diverse organizations were studied to determine common characteristics of problem definers. Among the variables considered as differentiating problem definers from non-problem definers were cognitive style, personal need characteristics, preference for ideation, experience, level of management, and type and level of education. Six hypotheses were tested using the following instruments: the Problem Solving Inventory, the Myers-Briggs Type Indicator Schedule, the Preference for Ideation Scale, the Edwards Personal Preference Schedule, a Problem Definition Exercise, and a Personal Data Questionnaire. Among the managers studied, only twelve were found to be problem definers. Such small numbers severely limit the ability to generalize about problem definers. However, it is possible that problem definers are scarce in organizations. In terms of cognitive style, problem definers were primarily thinking types who preferred evaluation to ideation in dealing with problems, making judgmental decisions on the basis of collected facts. Problem definers were not predominant at lower levels of the organization. One-third of the problem definers held upper level management positions while another one-fourth were responsible for specialized activities within their organizations, overseeing special projects and individuals much like upper level managers. Sixty-eight of the problem definers had non-business educations with none having more than a bachelors degree. As knowledge and judgment on which to base evaluation expands, managers may become less adept at defining problems and more adept at selecting and implementing alternatives. Several tentative hypotheses can be tested in future research including: 1) determining whether problem definers are scarce in organizations, 2) determining whether problem definers are more prevalent in some types of organizations than others, 3) verifying unique cognitive and personal need characteristics, 4) determining whether non-managers rather than managers have problem defining skills.
- problem definers
- sequential problem solving process
Library of Congress Subject Headings
- Problem solving
- Thesis or Dissertation
Unique identifying numbers for this dissertation in the Digital Library or other systems.
- Accession or Local Control No : 1002779272-Danielson
- Call Number : 379 N81d no. 2390
- UNT Catalog No. : b1339432 | View in Discover
- OCLC : 13703594
- Archival Resource Key : ark:/67531/metadc331384
Collections
This dissertation is part of the following collection of related materials.
UNT Theses and Dissertations
Theses and dissertations represent a wealth of scholarly and artistic content created by masters and doctoral students in the degree-seeking process. Some ETDs in this collection are restricted to use by the UNT community .
What responsibilities do I have when using this dissertation?
Digital Files
- 143 image files available in multiple sizes
- 1 file (.pdf)
- Metadata API: descriptive and downloadable metadata available in other formats
Dates and time periods associated with this dissertation.
Creation Date
- August 1985
Added to The UNT Digital Library
- Aug. 22, 2014, 6 p.m.
Description Last Updated
- Nov. 21, 2017, 10:15 a.m.
Usage Statistics
When was this dissertation last used?
Interact With This Dissertation
Here are some suggestions for what to do next.
Search Inside
- or search this site for other thesis or dissertations
Start Reading
- All Formats
Citations, Rights, Re-Use
- Citing this Dissertation
- Responsibilities of Use
- Licensing and Permissions
- Linking and Embedding
- Copies and Reproductions
International Image Interoperability Framework

We support the IIIF Presentation API
Print / Share
Links for robots.
Helpful links in machine-readable formats.
Archival Resource Key (ARK)
- ERC Record: /ark:/67531/metadc331384/?
- Persistence Statement: /ark:/67531/metadc331384/??
International Image Interoperability Framework (IIIF)
- IIIF Manifest: /ark:/67531/metadc331384/manifest/
Metadata Formats
- UNTL Format: /ark:/67531/metadc331384/metadata.untl.xml
- DC RDF: /ark:/67531/metadc331384/metadata.dc.rdf
- DC XML: /ark:/67531/metadc331384/metadata.dc.xml
- OAI_DC : /oai/?verb=GetRecord&metadataPrefix=oai_dc&identifier=info:ark/67531/metadc331384
- METS : /ark:/67531/metadc331384/metadata.mets.xml
- OpenSearch Document: /ark:/67531/metadc331384/opensearch.xml
- Thumbnail: /ark:/67531/metadc331384/thumbnail/
- Small Image: /ark:/67531/metadc331384/small/
- In-text: /ark:/67531/metadc331384/urls.txt
- Usage Stats: /stats/stats.json?ark=ark:/67531/metadc331384
Phillips Danielson, Waltraud. Managerial Problem Definition: A Descriptive Study of Problem Definers , dissertation , August 1985; Denton, Texas . ( https://digital.library.unt.edu/ark:/67531/metadc331384/ : accessed June 27, 2024 ), University of North Texas Libraries, UNT Digital Library, https://digital.library.unt.edu ; .
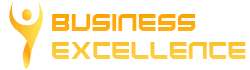
Mastering Managerial Problem-Solving Strategies: A Comprehensive Guide
- Posted by: Thamizharasu Gopalsamy
- Category: Management
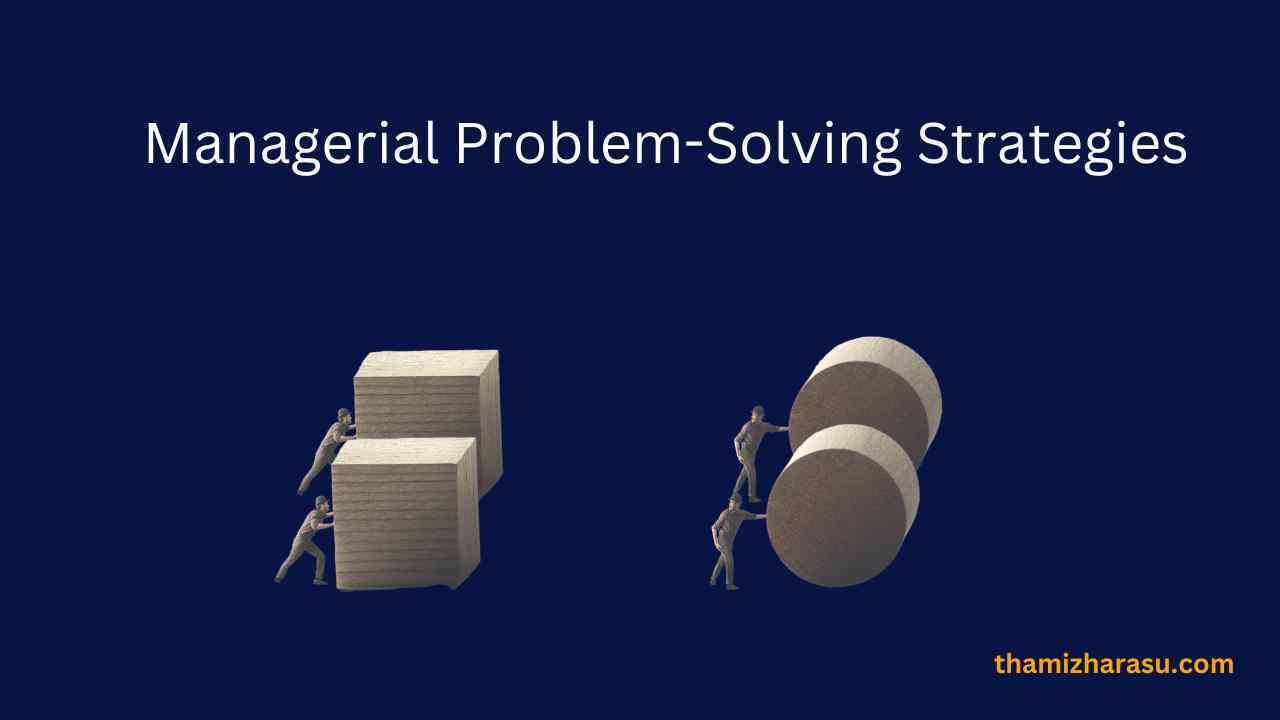
Table of Contents
1. Essential Elements of Managerial Problem-Solving Strategies
Problem-solving is a vital skill for managers, as it enables them to identify, analyze, and resolve issues effectively. In a managerial context, problem-solving strategies involve several essential elements to ensure success. In this section, we’ll discuss the fundamental components of effective managerial problem-solving.
1. Defining the Problem
Clearly identifying the problem is the first step in effective problem-solving. Managers must accurately assess the situation, gather relevant information, and determine the root cause of the issue. This allows for the development of targeted solutions that directly address the underlying problem.
2. Analyzing the Problem
Once the problem has been defined, managers must analyze the issue from multiple perspectives. This involves considering the problem’s impact on various stakeholders, the resources available to address the issue, and the potential consequences of different actions. Analyzing the problem thoroughly helps managers make well-informed decisions.
3. Generating Solutions
The next step involves brainstorming and generating a wide range of potential solutions. Managers should encourage creative thinking and open discussions among team members. This process promotes the development of innovative and effective ideas to address the problem at hand.
4. Evaluating and Selecting Solutions
After generating potential solutions, managers must carefully evaluate each option based on their feasibility, cost-effectiveness, and potential impact. This process helps in narrowing down the list of potential solutions and selecting the best one. Managers should consider both short-term and long-term consequences of each solution to ensure the chosen strategy aligns with organizational goals.
5. Implementing the Chosen Solution
Once a solution has been selected, managers must develop a detailed action plan to implement it. This involves assigning tasks and responsibilities to team members, setting deadlines, and allocating resources. Effective communication is crucial during this stage to ensure everyone understands their role in implementing the solution.
6. Monitoring and Reviewing Progress
After the solution has been implemented, managers must continuously monitor and review its progress to ensure it is effectively addressing the problem. This involves tracking key performance indicators (KPIs), gathering feedback from stakeholders, and making adjustments as necessary. Regular review helps identify any potential issues early on, allowing for timely corrective action.
7. Learning and Continuous Improvement
Effective problem-solving is an ongoing process that involves learning from past experiences and continuously improving strategies. Managers should encourage a culture of continuous learning and improvement within the organization. This involves reflecting on the problem-solving process, identifying areas for improvement, and implementing changes to enhance future problem-solving efforts.
By incorporating these essential elements into their problem-solving strategies, managers can effectively address issues and make informed decisions that benefit the organization and its stakeholders.

2. Top Managerial Problem-Solving Strategies for Improved Decision-Making
Effective decision-making is crucial for managers to navigate complex business situations and lead their teams towards success. By employing various problem-solving strategies, managers can enhance their decision-making capabilities. Here are some top managerial problem-solving strategies to improve decision-making:
1. Root Cause Analysis
Root cause analysis is a systematic approach to identifying the underlying cause of a problem. By addressing the root cause, managers can prevent the issue from recurring and find long-lasting solutions. Techniques like the 5 Whys and Fishbone Diagram (Ishikawa Diagram) can be employed for effective root cause analysis.
2. Brainstorming
Brainstorming is a creative process that encourages the free flow of ideas from all team members. By fostering an open and inclusive environment, managers can generate a diverse range of potential solutions and gain valuable insights from different perspectives. This approach leads to innovative solutions and improved decision-making.
3. SWOT Analysis
A SWOT (Strengths, Weaknesses, Opportunities, and Threats) analysis is a strategic planning tool that helps managers assess their organization’s internal and external factors. By evaluating these factors, managers can identify potential opportunities and challenges, leading to more informed decision-making.
4. Cost-Benefit Analysis
The cost-benefit analysis involves comparing the costs and benefits associated with each potential solution to determine the most effective course of action. This strategy helps managers make data-driven decisions based on the potential return on investment (ROI) and the overall impact of each solution.
5. Decision Matrix
A decision matrix is a quantitative tool that helps managers compare different options based on specific criteria. By assigning weights to each criterion and scoring the options, managers can rank the solutions and choose the best one. This structured approach ensures a more objective and rational decision-making process.
6. Scenario Planning
Scenario planning involves envisioning multiple possible future scenarios and assessing the potential impact of each on the organization. By considering different outcomes, managers can make more informed decisions and develop contingency plans to adapt to changing circumstances.
7. Proactive Problem-Solving
Proactive problem-solving involves anticipating potential issues and developing strategies to mitigate them before they occur. By regularly reviewing processes, analyzing trends, and seeking feedback, managers can identify potential problems early on and take preventive action.
8. Collaborative Problem-Solving
Collaborative problem-solving involves working closely with team members and stakeholders to develop and implement solutions. By leveraging the collective knowledge and expertise of the group, managers can arrive at more effective and well-rounded decisions.
By employing these managerial problem-solving strategies, managers can enhance their decision-making abilities and lead their teams more effectively. Incorporating a diverse range of approaches will help managers navigate various challenges and make better-informed decisions that drive organizational success.
3. Implementing Managerial Problem-Solving Strategies: A Step-by-Step Guide
Effectively implementing problem-solving strategies is crucial for managers to address challenges and make informed decisions. Here is a practical, step-by-step guide to implementing problem-solving strategies in a managerial setting:
Step 1: Identify the Problem
Start by clearly defining the issue you are facing. Gather all relevant information, assess the situation, and determine the root cause of the problem. Make sure you have a comprehensive understanding of the problem before moving forward.
Step 2: Assemble a Problem-Solving Team
Select a diverse group of team members with different skill sets and perspectives to form a problem-solving team. Ensure that the team is equipped with the necessary resources and authority to address the issue.
Step 3: Choose an Appropriate Problem-Solving Strategy
Based on the nature of the problem and your organizational context, choose a problem-solving strategy that best fits your needs. Consider factors like time constraints, available resources, and the complexity of the issue when selecting a strategy.
Step 4: Analyze the Problem
Thoroughly analyze the issue from multiple perspectives, considering its impact on various stakeholders, available resources, and potential consequences of different actions. Use tools like SWOT analysis or root cause analysis to gain a deeper understanding of the problem.
Step 5: Generate Potential Solutions
Encourage open discussions and brainstorming sessions with your team to generate a wide range of potential solutions. Ensure that all team members have the opportunity to contribute their ideas and perspectives.
Step 6: Evaluate and Select the Best Solution
Carefully evaluate each proposed solution based on factors such as feasibility, cost-effectiveness, and potential impact. Use tools like cost-benefit analysis or a decision matrix to help guide your decision-making process. Once you have assessed all options, choose the best solution that aligns with your organizational goals and addresses the root cause of the problem.
Step 7: Develop an Implementation Plan
Create a detailed action plan outlining the steps needed to implement the chosen solution. Assign specific tasks and responsibilities to team members, set deadlines, and allocate the necessary resources. Ensure that everyone involved understands their role and the expectations.
Step 8: Communicate the Plan
Effectively communicate the implementation plan to all relevant stakeholders, including your team, other departments, and upper management. Make sure everyone is aware of the chosen solution, the rationale behind it, and their role in the process.
Step 9: Implement the Solution
Monitor the progress of the solution implementation closely and provide support to your team as needed. Be prepared to make adjustments to the plan if unforeseen challenges arise.
Step 10: Evaluate and Review the Results
After the solution has been implemented, evaluate its effectiveness by tracking key performance indicators (KPIs) and gathering feedback from stakeholders. Assess whether the solution has successfully addressed the problem and identify any areas for improvement.
Step 11: Learn and Adapt
Reflect on the problem-solving process and use the insights gained to improve future problem-solving efforts. Foster a culture of continuous learning and improvement within your organization to enhance overall performance.
By following this step-by-step guide, managers can effectively implement problem-solving strategies and address challenges in a systematic and organized manner. This approach will lead to better decision-making and improved organizational performance.
4. Overcoming Barriers to Effective Managerial Problem-Solving Strategies
Managers often encounter various challenges when solving problems, which can hinder the effectiveness of their problem-solving strategies. Identifying these barriers and finding ways to overcome them is crucial for successful problem-solving. Here are some common challenges and tips to overcome them:
1. Lack of Clear Problem Definition
Challenge: Managers sometimes struggle to define the problem clearly, leading to ineffective solutions that do not address the root cause.
Solution: Invest time in understanding the issue, gather relevant information, and use tools like the 5 Whys or Fishbone Diagram to determine the root cause. A clear problem definition will guide the problem-solving process and ensure more targeted solutions.
2. Insufficient Information or Resources
Challenge: Limited access to information or resources can hinder the problem-solving process and lead to suboptimal decisions.
Solution: Seek out additional data sources, consult with experts, or leverage your network to gather more information. Assess the available resources and consider reallocating them to address the problem effectively.
3. Groupthink and Limited Perspectives
Challenge: Groupthink can occur when team members conform to the dominant opinion, resulting in a lack of diverse perspectives and innovative solutions.
Solution: Encourage open communication and create an environment where team members feel comfortable sharing their ideas. Include diverse perspectives by involving people from different backgrounds, departments, or roles in the problem-solving process.
4. Time Constraints
Challenge: Tight deadlines and time pressure can lead to rushed decisions and poorly thought-out solutions.
Solution: Prioritize tasks and allocate sufficient time for each step of the problem-solving process. If necessary, break the problem down into smaller, more manageable parts and address them sequentially.
5. Resistance to Change
Challenge: Implementing new solutions may face resistance from team members or stakeholders, who are reluctant to change existing processes or practices.
Solution: Communicate the rationale behind the chosen solution clearly, address concerns, and involve stakeholders in the problem-solving process. Demonstrating the benefits of the change and providing support during the transition can help overcome resistance.
6. Ineffective Communication
Challenge: Poor communication can result in misunderstandings, misaligned expectations, and suboptimal solutions.
S olution: Develop strong communication skills and use clear, concise language when discussing the problem and potential solutions. Ensure all team members and stakeholders are on the same page and understand their roles in the problem-solving process.
7. Inability to Measure Results
Challenge: Managers may struggle to evaluate the effectiveness of their problem-solving strategies due to a lack of measurable outcomes.
Solution: Establish key performance indicators (KPIs) to track the progress and effectiveness of the implemented solution. Regularly review the results and make adjustments as needed to improve the problem-solving strategy.
By recognizing and addressing these common challenges , managers can overcome barriers to effective problem-solving and make more informed decisions that benefit their organizations.
5. Managerial Problem-Solving Strategies: The Role of Emotional Intelligence
Emotional intelligence (EI) plays a significant role in effective problem-solving and decision-making for managers. EI refers to the ability to recognize, understand, and manage one’s emotions and the emotions of others. Integrating emotional intelligence into managerial problem-solving strategies can lead to better decision-making, improved team dynamics , and increased organizational success. Here are some ways in which emotional intelligence contributes to effective problem-solving for managers:
1. Empathy and Perspective-Taking
Emotionally intelligent managers can put themselves in others’ shoes, enabling them to understand different perspectives and consider the impact of potential solutions on various stakeholders. This broader perspective helps in the development of well-rounded, inclusive solutions that address diverse needs and concerns.
2. Effective Communication
Emotionally intelligent managers are skilled at expressing their thoughts and ideas clearly and empathetically. They can also actively listen and respond to the concerns and ideas of others. Effective communication is essential in the problem-solving process, as it facilitates open discussion, collaborative decision-making, and smooth implementation of solutions.
3. Emotional Regulation
Managers with high emotional intelligence can regulate their emotions during stressful or challenging situations. By staying calm and composed, they can make more rational and objective decisions, ensuring that their problem-solving strategies are not clouded by emotional biases or stress-induced reactions.
4. Conflict Resolution
Emotionally intelligent managers can navigate conflicts and disagreements effectively, turning potentially destructive situations into opportunities for growth and collaboration. By addressing the underlying emotions and fostering open communication, managers can resolve conflicts in a way that strengthens relationships and enhances the problem-solving process.
5. Team Motivation and Engagement
Managers with high emotional intelligence can effectively motivate and engage their teams during the problem-solving process. They can recognize the emotions and needs of team members, provide support, and create a positive work environment that encourages participation, creativity, and commitment to shared goals.
6. Adaptability and Resilience
Emotionally intelligent managers can adapt to changing circumstances and bounce back from setbacks during the problem-solving process. Their resilience and ability to manage emotions help them maintain focus and stay committed to finding effective solutions, even in the face of adversity.
7. Building Trust and Collaboration
Emotional intelligence enables managers to build strong relationships with their team members and other stakeholders based on trust, understanding, and mutual respect. This fosters a collaborative work environment where individuals feel comfortable sharing their ideas, contributing to more effective problem-solving and decision-making.
Incorporating emotional intelligence into managerial problem-solving strategies is essential for effective decision-making, strong team dynamics , and overall organizational success. By developing their emotional intelligence skills, managers can enhance their problem-solving abilities and make more informed, empathetic decisions that benefit all stakeholders.
6. Case Studies: Managerial Problem-Solving Strategies in Action
Here are three real-life examples of successful problem-solving strategies employed by managers in various industries:
1. Toyota and the 5 Whys Technique
Industry: Automotive Manufacturing
Toyota, a leading automobile manufacturer, is well-known for its effective problem-solving strategies, particularly the 5 Whys technique. This approach involves asking “why” five times to identify the root cause of a problem. In one instance, Toyota faced issues with its assembly line , where a welding robot stopped working, causing a production delay. The manager employed the 5 Whys technique, which led to the discovery that the root cause was a lack of proper maintenance on the robot’s electrical components. By addressing the core issue, Toyota not only solved the immediate problem but also implemented preventive measures to avoid similar issues in the future.
2. Starbucks and Collaborative Problem-Solving
Industry: Food and Beverage
In 2008, Starbucks faced a significant decline in sales due to increased competition and the global financial crisis. The company’s CEO, Howard Schultz, decided to close all Starbucks stores for a day to engage the entire organization in a collaborative problem-solving effort. Employees were asked to share their ideas and suggestions on how to improve the company’s performance. This inclusive approach led to several innovative solutions, such as revamping the menu, improving the in-store experience, and expanding the company’s digital presence. By involving employees at all levels, Starbucks successfully turned around its business and re-established its position as a market leader.
3. LEGO and Scenario Planning
Industry: Toy Manufacturing
In the early 2000s, LEGO faced severe financial difficulties due to a combination of factors, including increased competition, changing consumer preferences, and an overly diversified product portfolio. The company’s management team decided to employ scenario planning as a problem-solving strategy to envision various future scenarios and their potential impact on the business. This exercise helped LEGO identify its core strengths and prioritize strategic initiatives , such as streamlining its product portfolio, focusing on innovation, and leveraging digital technologies. By employing scenario planning, LEGO was able to make informed decisions that ultimately led to a successful turnaround and a resurgence in its popularity.
These case studies demonstrate how effective problem-solving strategies can be employed by managers across different industries to address complex challenges and drive organizational success. By adopting a systematic and collaborative approach to problem-solving, managers can develop innovative solutions, make better-informed decisions, and improve the overall performance of their organizations.
7. The Future of Managerial Problem-Solving Strategies: Adapting to a Changing Business Environment
The business landscape is evolving rapidly, driven by factors such as globalization, technological advancements, and shifting consumer preferences. As a result, managers must adapt their problem-solving strategies to meet the demands of this changing environment. Here are some ways in which managerial problem-solving strategies must evolve to stay relevant and effective:
1. Embracing Digital Technologies
Managers must become adept at leveraging digital technologies, such as data analytics, artificial intelligence (AI), and machine learning, to enhance their problem-solving capabilities. These technologies can help managers make data-driven decisions, automate routine tasks, and gain valuable insights into customer behavior and market trends. By incorporating digital technologies into their problem-solving strategies, managers can improve efficiency, accuracy, and innovation.
2. Fostering Agility and Flexibility
In a rapidly changing business environment, the ability to adapt quickly to new challenges and opportunities is crucial. Managers must develop agile problem-solving strategies that allow them to pivot and adjust their approach as needed. This may involve adopting iterative processes, embracing a fail-fast mentality, and fostering a culture of continuous learning and improvement.
3. Promoting Innovation and Creativity
To stay competitive in a constantly evolving landscape, managers must prioritize innovation and creativity in their problem-solving strategies. This involves encouraging a culture of experimentation, embracing risk-taking, and empowering employees to think outside the box. By fostering an environment that supports innovation, managers can drive organizational growth and stay ahead of emerging trends and challenges.
4. Enhancing Collaboration and Cross-Functional Problem-Solving
As businesses become more interconnected and complex, managers must facilitate cross-functional collaboration to address multifaceted problems effectively. This includes breaking down silos, fostering open communication, and leveraging the diverse skills and expertise of employees across the organization. By promoting cross-functional problem-solving, managers can develop comprehensive solutions that address various aspects of a problem.
5. Prioritizing Sustainability and Social Responsibility
In an increasingly eco-conscious and socially aware business landscape, managers must consider the environmental and social impacts of their problem-solving strategies. This involves integrating sustainability and social responsibility into decision-making processes and evaluating potential solutions based on their long-term consequences. By prioritizing sustainable and responsible problem-solving, managers can contribute to the overall well-being of society and the environment while also enhancing their organization’s reputation and competitiveness.
6. Developing Global Mindsets
Globalization has made the business landscape more interconnected than ever before. Managers must develop a global mindset and consider the implications of their problem-solving strategies on diverse stakeholders, markets, and cultures. By adopting a global perspective, managers can make more informed decisions and develop solutions that resonate with audiences worldwide.
8. How to Measure the Success of Your Managerial Problem-Solving Strategies
Evaluating the effectiveness of your problem-solving strategies is crucial for continuous improvement and ensuring that your decisions lead to the desired outcomes. Here are some tips on how to track and measure the success of your managerial problem-solving strategies:
1. Establish Clear Objectives and Key Performance Indicators (KPIs)
Before implementing a problem-solving strategy, define clear objectives and set measurable KPIs to track progress and success. KPIs should be specific, quantifiable, and relevant to the problem at hand. They will serve as a benchmark to evaluate the effectiveness of your strategy and help you determine if adjustments are needed.
2. Monitor Progress Regularly
Regularly review the progress of your problem-solving strategy by comparing the actual results against your KPIs. This will help you identify any deviations from the plan, allowing you to address issues promptly and make necessary adjustments. Establish a consistent schedule for monitoring progress, whether it’s weekly, monthly, or quarterly, depending on the nature of the problem and the strategy implemented.
3. Gather Feedback from Stakeholders
Involve relevant stakeholders, such as team members, customers, or suppliers, in the evaluation process. Seek their feedback on the effectiveness of the problem-solving strategy and its impact on their work or experience. This qualitative input can provide valuable insights into areas for improvement and help you fine-tune your approach.
4. Conduct a Post-Implementation Review
After the completion of your problem-solving strategy, conduct a post-implementation review to assess its overall effectiveness. Analyze the outcomes, compare them against your initial objectives and KPIs, and identify any lessons learned. This review will help you understand what worked well, what didn’t, and how you can improve your problem-solving strategies moving forward.
5. Utilize Data Analytics
Leverage data analytics tools to measure the success of your problem-solving strategies. These tools can help you collect, analyze, and visualize data, making it easier to identify trends, patterns, and areas for improvement. By harnessing the power of data analytics, you can make more informed decisions about your problem-solving strategies and their effectiveness.
6. Benchmark Against Industry Standards or Competitors
Compare the results of your problem-solving strategies against industry standards or competitors to gain an understanding of your relative performance. This benchmarking can provide valuable insights into your organization’s strengths and weaknesses, helping you identify areas for improvement and opportunities for growth.
7. Assess the Impact on Organizational Goals
Evaluate the effectiveness of your problem-solving strategies by considering their impact on your organization’s broader goals and objectives. Assess how the implemented solutions contribute to the overall success of the company and whether they align with your strategic vision.
By tracking and evaluating the success of your managerial problem-solving strategies, you can ensure their effectiveness, make informed adjustments, and drive continuous improvement. Remember that problem-solving is an ongoing process, and regular evaluation is essential for maximizing the positive impact of your strategies on your organization.
Conclusion:
Effective managerial problem-solving strategies are crucial for organizational success in today’s rapidly evolving business environment. By understanding and implementing a range of problem-solving approaches, overcoming common barriers, and leveraging emotional intelligence, managers can make informed decisions that benefit their organizations. Adapting to the changing business landscape, fostering collaboration, and continuously evaluating the success of problem-solving strategies are essential for staying ahead of the competition and driving sustainable growth.
Collaborative Problem-Solving in Knowledge-Rich Domains: A Multi-Study Structural Equation Model
- Open access
- Published: 24 June 2024
Cite this article
You have full access to this open access article
- Laura Brandl ORCID: orcid.org/0000-0001-7974-7892 1 ,
- Matthias Stadler 1 , 2 ,
- Constanze Richters 1 ,
- Anika Radkowitsch 3 ,
- Martin R. Fischer 2 ,
- Ralf Schmidmaier 4 &
- Frank Fischer 1
123 Accesses
Explore all metrics
Collaborative skills are crucial in knowledge-rich domains, such as medical diagnosing. The Collaborative Diagnostic Reasoning (CDR) model emphasizes the importance of high-quality collaborative diagnostic activities (CDAs; e.g., evidence elicitation and sharing), influenced by content and collaboration knowledge as well as more general social skills, to achieve accurate, justified, and efficient diagnostic outcomes (Radkowitsch et al., 2022). However, it has not yet been empirically tested, and the relationships between individual characteristics, CDAs, and diagnostic outcomes remain largely unexplored. The aim of this study was to test the CDR model by analyzing data from three studies in a simulation-based environment and to better understand the construct and the processes involved ( N = 504 intermediate medical students) using a structural equation model including indirect effects. We found various stable relationships between individual characteristics and CDAs, and between CDAs and diagnostic outcome, highlighting the multidimensional nature of CDR. While both content and collaboration knowledge were important for CDAs, none of the individual characteristics directly related to diagnostic outcome. The study suggests that CDAs are important factors in achieving successful diagnoses in collaborative contexts, particularly in simulation-based settings. CDAs are influenced by content and collaboration knowledge, highlighting the importance of understanding collaboration partners’ knowledge. We propose revising the CDR model by assigning higher priority to collaboration knowledge compared with social skills, and dividing the CDAs into information elicitation and sharing, with sharing being more transactive. Training should focus on the development of CDAs to improve CDR skills.
Similar content being viewed by others
Diagnosing Collaboratively: A Theoretical Model and a Simulation-Based Learning Environment
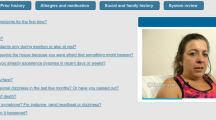
Diagnosing virtual patients: the interplay between knowledge and diagnostic activities
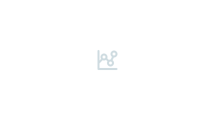
Medical Students’ Clinical Reasoning During a Simulated Viral Pandemic: Evidence of Cognitive Integration and Insights on Novices’ Approach to Diagnostic Reasoning
Avoid common mistakes on your manuscript.
Introduction
Collaborative skills are highly relevant in many situations, ranging from computer-supported collaborative learning to collaborative problem-solving in professional practice (Fiore et al., 2018 ). While several broad collaborative problem-solving frameworks exist (OECD, 2017 ), most of them are situated in knowledge-lean settings. However, one example of collaborative problem-solving of knowledge-rich domains is collaborative diagnostic reasoning (CDR; Radkowitsch et al., 2022 )—which aligns closely with medical practice—as this is a prototypical knowledge-rich domain requiring high collaboration skills in daily practice. In daily professional practice, physicians from different specialties often need to collaborate with different subdisciplines to solve complex problems, such as diagnosing, that is, determining the causes of a patient’s problem. Moreover, research in medical education and computer-supported collaborative learning suggests that the acquisition of medical knowledge and skills is significantly enhanced by collaborative problem-solving (Hautz et al., 2015 ; Koschmann et al., 1992 ). For problem-solving and learning, it is crucial that all relevant information (e.g., evidence and hypotheses) is elicited from and shared with the collaboration partner (Schmidt & Mamede, 2015 ). However, CDR is not unique to the medical field but also relevant in other domains, such as teacher education (Heitzmann et al., 2019 ).
The CDR model has been the basis of empirical studies and describes how individual characteristics and the diagnostic process are related to the diagnostic outcome. However, it has not yet been empirically tested, and the relationships between individual characteristics, diagnostic process, and diagnostic outcome remain mostly unexplored (Fink et al., 2023 ). The aim of this study is to test the CDR model by analyzing data from three studies with similar samples and tasks investigating CDR in a simulation-based environment. By undertaking these conceptual replications, we aspire to better understand the construct and the processes involved. As prior research has shown, collaboration needs to be performed at a high quality to achieve accurate problem solutions respectively learning outcomes (Pickal et al., 2023 ).
Collaborative Diagnostic Reasoning (CDR) Model
Diagnosing can be understood as the process of solving complex diagnostic problems through “goal-oriented collection and interpretation of case-specific or problem-specific information to reduce uncertainty” in decision-making through performing diagnostic activities at a high quality (Heitzmann et al., 2019 , p. 4). To solve diagnostic problems, that is, to identify the causes of an undesired state, it is increasingly important to collaborate with experts from different fields, as these problems become too complex to be solved individually (Abele, 2018 ; Fiore et al., 2018 ). Collaboration provides advantages such as the division of labor, access to diverse perspectives and expertise, and enhanced solution quality through collaborative sharing of knowledge and skills (Graesser et al., 2018 ).
The CDR model is a theoretical model focusing on the diagnostic process in collaborative settings within knowledge-rich domains (Radkowitsch et al., 2022 ). The CDR model is based on scientific discovery as a dual-search model (SDDS; Klahr & Dunbar, 1988 ) and its further development by van Joolingen and Jong ( 1997 ). The SDDS model describes individual reasoning as the coordinated search through hypothetical evidence and hypotheses spaces and indicates that for successful reasoning it is important not only that high-quality cognitive activities within these spaces are performed but also that one is able to coordinate between them (Klahr & Dunbar, 1988 ). In the extended SDDS model (van Joolingen & Jong, 1997 ) focusing on learning in knowledge-rich domains, a learner hypothesis space was added including all the hypotheses one can search for without additional knowledge. Although Dunbar ( 1995 ) found that conceptual change occurs more often in groups than in individual work, emphasizing the importance of collaborative processes in scientific thinking and knowledge construction, the SDDS model has hardly been systematically applied in computer-supported collaborative learning and collaborative problem-solving.
Thus, the CDR model builds upon these considerations and describes the relationship between individual characteristics, the diagnostic process, and the diagnostic outcome. As in the SDDS model we assume that CDR involves activities within an evidence and hypotheses space; however, unlike the SDDS in the CDR model, these spaces are understood as cognitive storages of information. Which aligns more to the extended dual search space model of scientific discovery learning (van Joolingen & Jong, 1997 ). In summary we assume that coordinating between evidence (data) and hypothesis (theory) is essential for successful diagnosing. Further, the CDR model is extended to not only individual but also collaborative cognitive activities and describes the interaction of epistemic activities (F. Fischer et al., 2014 ) and collaborative activities (Liu et al., 2016 ) to construct a shared problem representation (Rochelle & Teasley, 1995 ) and effectively collaborate. Thus, we define CDR as a set of skills for solving a complex problem collaboratively “by generating and evaluating evidence and hypotheses that can be shared with, elicited from, or negotiated among collaborators” (Radkowitsch et al., 2020 , p. 2). The CDR model also makes assumptions about the factors necessary for successful CDR. First, we look at what successful CDR means, why people differ, and what the mediating processes are.
Diagnostic Outcome: Accuracy, Justification, and Efficiency
The primary outcome of diagnostic processes, such as CDR, is the accuracy of the given diagnosis, which indicates problem-solving performance or expertise (Boshuizen et al., 2020 ). However, competence in diagnostic reasoning, whether it is done individually or collaboratively, also includes justifying the diagnosis and reaching it effectively. This is why, in addition to diagnostic accuracy, diagnostic justification and diagnostic efficiency should also be considered as secondary outcomes of the diagnostic reasoning process (Chernikova et al., 2022 ; Daniel et al., 2019 ). Diagnostic justification makes the reasoning behind the decision transparent and understandable for others (Bauer et al., 2022 ). Good reasoning entails a justification including evidence, which supports the reasoning (Hitchcock, 2005 ). Diagnostic efficiency is related to how much time and effort is needed to reach the correct diagnosis; this is important for CDR, as diagnosticians in practice are usually under time pressure (Braun et al., 2017 ). Both diagnostic justification and diagnostic efficiency are thus indicators of a structured and high-quality reasoning process. So, while in many studies, the focus of assessments regarding diagnostic reasoning is on the accuracy of the given diagnosis (Daniel et al., 2019 ), the CDR model considers all three facets of the diagnostic outcome as relevant factors.
Individual Characteristics: Knowledge and Social Skills
Research has shown that content knowledge, social skills, and, in particular, collaboration knowledge are important prerequisites for, and outcomes of, computer-supported collaborative learning (Jeong et al., 2019 ; Vogel et al., 2017 ). CDR has integrated these dependencies into its model structure. Thus, the CDR model assumes that people engaging in CDR differ with respect to their content knowledge, collaboration knowledge, and domain general social skills.
Content knowledge refers to conceptual and strategic knowledge in a specific domain (Förtsch et al., 2018 ). Conceptual knowledge encompasses factual understanding of domain-specific concepts and their interrelations. Strategic knowledge entails contextualized knowledge regarding problem-solving during the diagnostic process (Stark et al., 2011 ). During expertise development, large amounts of content knowledge are acquired and restructured through experience with problem-solving procedures and routines (Boshuizen et al., 2020 ). Research has repeatedly shown that having high conceptual and strategic knowledge is associated with the diagnostic outcome (e.g., Kiesewetter et al., 2020 ; cf. Fink et al., 2023 ).
In addition to content knowledge, the CDR model assumes that collaborators need collaboration knowledge. A key aspect of collaboration knowledge (i.e., being aware of knowledge distribution in the group; Noroozi et al., 2013 ) is the pooling and processing of non-shared information, as research shows that a lack of collaboration knowledge has a negative impact on information sharing, which in turn has a negative impact on performance (Stasser & Titus, 1985 ).
Finally, general social skills influence the CDR process. These skills mainly influence the collaborative aspect of collaborative problem-solving and less the problem-solving aspect (Graesser et al., 2018 ). Social skills are considered particularly important when collaboration knowledge is low (F. Fischer et al., 2013 ). CDR assumes that in particular the abilities to share and negotiate ideas, to coordinate, and to take the perspective are relevant for the diagnostic process and the diagnostic outcome (Radkowitsch et al., 2022 ; see also Liu et al., 2016 , and Hesse et al., 2015 ).
Diagnostic Process: Collaborative Diagnostic Activities
The diagnostic process is thought to mediate the effect of the individual characteristics on the diagnostic outcome and is described in the CDR model using collaborative diagnostic activities (CDAs), such as evidence elicitation, evidence sharing, and hypotheses sharing (Heitzmann et al., 2019 ; Radkowitsch et al., 2022 ). One of the main functions of CDAs is to construct a shared problem representation (Rochelle & Teasley, 1995 ) by sharing and eliciting relevant information, as information may not be equally distributed among all collaborators initially. To perform these CDAs at a high quality, each collaborator needs to identify information relevant to be shared with the collaboration partner as well as information they need from the collaboration partner (OECD, 2017 ).
Evidence elicitation involves requesting information from a collaboration partner to access additional knowledge resources (Weinberger & Fischer, 2006 ). Evidence sharing and hypothesis sharing involve identifying the information needed by the collaborator to build a shared problem representation. This externalization of relevant information can be understood as the novelty aspect of transactivity (Vogel et al., 2023 ). However, challenges arise from a lack of relevant information due to deficient sharing, which can result from imprecise justification and insufficient clustering of information. In particular, research has shown that collaborators often lack essential information-sharing skills, such as identifying information relevant for others from available data, especially in the medical domain (Kiesewetter et al., 2017 ; Tschan et al., 2009 ).
It is crucial for the diagnostic outcome that all relevant evidence and hypotheses are elicited and shared for the specific collaborators (Tschan et al., 2009 ). However, diagnostic outcomes seem to be influenced more by the relevance and quality of the shared information than by their quantity (Kiesewetter et al., 2017 ; Tschan et al., 2009 ). In addition, recent research has shown that the diagnostic process is not only an embodiment of individual characteristics but also adds a unique contribution to diagnostic outcome (Fink et al., 2023 ). However, it remains difficult to assess and foster CDAs.
Collaboration in Knowledge-Rich Domains: Agent-Based Simulations
There are several challenges when it comes to modelling collaborative settings in knowledge-rich domains for both learning and research endeavors. First, many situations are not easily accessible, as they may be scarce (e.g., natural disasters) or too critical or overwhelming to be approached by novices (e.g., some medical procedures). In these cases, the use of simulation-based environments allows authentic situations approximating real-life diagnostic problems to be provided (Cook et al., 2013 ; Heitzmann et al., 2019 ). Further, the use of technology-enhanced simulations allows data from the ongoing CDR process to be collected in log files. This enables researchers to analyze process data without the need for additional assessments with dedicated tests. Analyzing process data instead of only product data (the assessment’s outcome) permits insights into the problem-solving processes leading to the eventual outcome (e.g., Goldhammer et al., 2017 ). Second, when using human-to-human collaboration, the results of one individual are typically influenced by factors such as group composition or motivation of the collaboration partner (Radkowitsch et al., 2022 ). However, we understand CDR as an individual set of skills enabling collaboration, as indicated by the broader definition of collaborative problem-solving (OECD, 2017 ). Thus, the use of simulated agents as collaboration partners allows a standardized and controlled setting to be created that would otherwise be hard to establish in collaborations among humans (Rosen, 2015 ). There is initial research showing that performance in simulations using computerized agents is moderately related to collaborative skills in other operationalizations (Stadler & Herborn et al., 2020 ). Thus, computerized agents allow for enhanced control over the collaborative process without significantly diverging from human-to-human interaction (Graesser et al., 2018 ; Herborn et al., 2020 ). Third, in less controlled settings it is hard to ensure a specific process is taking place during collaborative problem-solving. For example, when using a human-to-human setting, it is possible that, even though we envision measuring or fostering a specific activity (i.e. hypotheses sharing), it is not performed by the student. Through using an agent-based simulated collaboration partner, we can ensure that all required processes are taking place while solving the problem (Rosen, 2015 ).
Summarizing, by fostering a consistent and controlled setting, simulated agents facilitate the accurate measurement and enhancement of collaborative problem-solving. Evidential support for the application of simulated agents spans a variety of contexts, including tutoring, collaborative learning, knowledge co-construction, and collaborative problem-solving itself, emphasizing their versatility and effectiveness in educational settings (Graesser et al., 2018 ; Rosen, 2015 ).
Research Question and Current Study
In computer-supported collaborative learning there has been the distinction between approaches addressing collaboration to learn and approaches focusing on learning to collaborate. Our study is best understood as addressing the second approach, learning to collaborate. We want to better understand CDR to be able to facilitate collaborative problem-solving skills in learners. Thus, in this paper, we examine what it takes to be able to collaborate in professional practice of knowledge-rich domains, such as medical diagnosing.
When solving diagnostic problems, such as diagnosing a patient, it is often necessary to collaborate with experts from different fields (Radkowitsch et al., 2022 ). In CDR, the diagnostic outcome depends on effectively eliciting and sharing relevant evidence and hypotheses among collaborators, who often lack information-sharing skills (Tschan et al., 2009 ). Thus, the CDR model emphasizes the importance of high-quality CDAs influenced by content and collaboration knowledge as well as social skills to achieve accurate, justified, and efficient diagnostic outcomes (Radkowitsch et al., 2022 ).
This study reviews the relationships postulated in CDR model across three studies to test them empirically and investigate the extent to which the relationships in the CDR model are applicable across studies . By addressing this research question, the current study contributes to a better understanding of the underlying processes in collaborative problem-solving.
We derived a model (Fig. 1 ) from the postulated relationships made by the CDR model. We assume that the individual characteristics are positively related to the CDAs (Hypotheses 1–3), as well as that the CDAs are positively related to the diagnostic outcome (Hypotheses 4–6). Further, we expect that the relationship between the individual characteristics and the diagnostic outcome is partially mediated by the CDAs (Hypotheses 7–15).
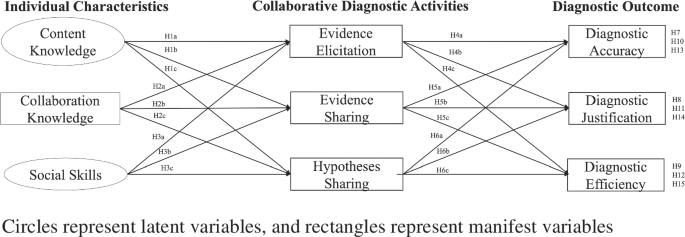
Visualization of hypothesized relationships between individual characteristics, collaborative diagnostic activities, and diagnostic outcome
We used data from three studies with similar samples and tasks investigating CDR in an agent-based simulation in the medical domain. The studies can therefore be considered conceptual replication studies. Furthermore, we decided to use an agent-based simulation of a typical collaboration setting in diagnostic reasoning, namely the interdisciplinary collaboration between an internist and a radiologist (Radkowitsch et al., 2022 ).
To test the hypotheses, three studies were analyzed. Footnote 1 Study A was carried out in a laboratory setting in 2019 and included medical students in their third to sixth years. Study B included medical students in their fifth to sixth years. Data collection for this study was online due to the pandemic situation in 2020 and 2021. In both studies, participation was voluntary, and participants were paid 10 per hour. Study C was embedded as an online session in the curriculum of the third year of medical school in 2022. Participation was mandatory, but permission to use the data for research purposes was given voluntarily. All participants took part in only one of the three studies. All three studies received ethical approval from LMU Munich (approval numbers 18-261, 18-262 & 22-0436). For a sample description of each study, see Table 1 . We would like to emphasize that none of the students were specializing in internal medicine, ensuring that the study results reflect the competencies of regular medical students without specialized expertise.
Each of the three studies was organized in the same way, with participants first completing a pretest that included a prior knowledge test, socio-demographic questions, and questions about individual motivational-affective characteristics (e.g., social skills, interest, and motivation). Participants then moved on to the CDR simulation and worked on the patient case. The patient case was the same for studies B and C, but was different for study A. The complexity and difficulty of the patient case did not vary systematically between the patient cases.
Simulation and Task
In the CDR simulation, which is also used as a learning environment, the task was to take over the role of an internist and to collaborate with an agent-based radiologist to obtain further information by performing radiological examinations to diagnose fictitious patient cases with the chief symptom of fever. Medical experts from internal medicine, radiology, and general medicine constructed the patient cases. Each case was structured in the same way: by studying the medical record individually, then collaborating with an agent-based radiologist, and finally reporting the final diagnosis and its justification again individually. For a detailed description on the development and validation of the simulation, see Radkowitsch and colleagues ( 2020 ).
Before working within the simulation, participants were presented with an instruction for the simulated scenario and informed what they were to do with it. Then, we instructed participants how to access further information in the medical record by clicking on hyperlinks, as well as how they could use the toolbar to make notes for the later in the process. Furthermore, we acquainted the students with how they could request further information through collaborating with a radiologist.
During the collaboration with an agent-based radiologist, participants were asked to fill out request forms to obtain further evidence from radiological examinations needed to diagnose the patient case. To effectively collaborate with radiologists, it is crucial for internists to clearly communicate the type of evidence required to reduce uncertainty (referred to as “evidence elicitation”) and share any relevant patient information such as signs, symptoms, and medical history (referred to as “evidence sharing”) as well as suspected diagnoses under investigation (referred to as “hypotheses sharing”) that may impact the radiologists’ diagnostic process. Only when participants shared evidence and hypotheses appropriately for their requested examination did they receive a description and evaluation of the radiologist’s radiologic findings. What was considered appropriate was determined by medical experts for each case and examination in preparation of the cases. Therefore, this scenario involves more than a simple division of tasks, as the quality of one person’s activity (i.e., description and evaluation of the radiologic findings) depends on the collaborative efforts (i.e., CDAs) of the other person (OECD, 2017 )
Measures—Individual Characteristics
The individual characteristics were measured in the pretest. The internal consistencies of each measure per study are displayed in Table 4 in the Results section. We want to point out that the internal consistency of knowledge as a construct—determined by the intercorrelations among knowledge pieces—typically exhibits a moderate level. Importantly, recent research argues that a moderate level of internal consistency does not undermine the constructs’ capacity to explain a significant amount of variance (Edelsbrunner, 2024 ; Stadler et al., 2021 ; Taber, 2018 ).
Content knowledge was separated into radiology and internal medicine knowledge, as these two disciplines play a major role in the diagnosis of the simulated patient cases. For each discipline, conceptual and strategic knowledge was assessed (Kiesewetter et al., 2020 ; Stark et al., 2011 ). The items in each construct were presented in a randomized way in each study. However, the items for study C were shortened due to the embedding of the data collection in the curriculum. Therefore, items with a very high or low item difficulty in previous studies were excluded (Table 2 ).
Conceptual knowledge was measured using single-choice questions including five options adapted from a database of examination questions from the Medical Faculty of the LMU Munich, focusing on relevant and closely related diagnoses of the patient cases used in the simulation. A mean score of 0–1 was calculated, representing the percentage of correct answers and indicating the average conceptual knowledge of the participant per medical knowledge domain.
Strategic content knowledge was measured contextually using key features questions (M. R. Fischer et al., 2005 ). Short cases were introduced followed by two to three follow up questions (e.g., What is your most likely suspected diagnosis?, What is your next examination?, What treatment do you choose?). Each question had eight possible answers, from which the learners were asked to choose one. Again, a mean score of 0–1 was calculated, representing the percentage of correct responses, indicating the average strategic content knowledge of the participant per domain.
The measure of collaboration knowledge was consistent across the three studies and specific to the simulated task. Participants were asked to select all relevant information for seven different patient cases with the cardinal symptom of fever (internal medicine). The patient cases were presented in a randomized order and always included 12 pieces of information regarding the chief complaints, medical history, and physical examination of the patient cases. We then assessed whether each piece of information was shared correctly (i.e. whether relevant information was shared and irrelevant information was not shared) and assigned 1 point and divided it by the maximum of 12 points to standardized the range of measure to 0–1. Then we calculated a mean score for each case and then across all cases, resulting in a range of 0–1 indicating the participants’ collaboration knowledge
The construct of social skills was consistent across the three data collections and was measured on the basis of self-report on a 6-point Likert scale ranging from total disagreement to total agreement. The construct was measured using 23 questions divided into five subscales; for example items, see Table 3 . Five questions aimed to measure the overall construct, and the other four subscales were identified using the complex problem-solving frameworks of Liu et al. ( 2016 ) and Hesse et al. ( 2015 ): perspective taking (four questions), information sharing (five questions), negotiation (four questions), and coordination (five questions). For the final score, the mean of all subcategories was calculated, ranging from 1 to 6, representing general social skills.
Measures—Collaborative Diagnostic Activities (CDA)
We operationalize CDAs in the pretest case in terms of quality of evidence elicitation, evidence sharing, and hypotheses sharing. The internal consistencies of each measure per study are displayed in Table 4 in the Results section.
The quality of evidence elicitation was measured by assessing the appropriateness of the requested radiological examination for the indicated diagnosis. An expert solution was developed to indicate which radiological examinations were appropriate for each of the possible diagnoses. If participants requested an appropriate radiological examination for the indicated diagnoses, they received 1 point for that request attempt. Finally, a mean score across all request attempts (maximum of 3) was calculated and scored. The final mean score was transformed into a binary indicator, with 1 indicating that all requested radiological examinations were appropriated and 0 indicating that inappropriate radiological examinations were also requested, due to the categorical nature of the original data and its skewed distribution, with a majority of responses concentrated in a single category.
The quality of evidence sharing was measured using a precision indicator. This was calculated as the proportion of shared relevant evidence out of all shared evidence. Relevant evidence is defined per case and per diagnosis and indicated by the expert solution. The precision indicator was first calculated per radiological request. We then calculated the mean score, summarizing all attempts in that patient case. This resulted in a range from 0 points, indicating that only irrelevant evidence was shared, to 1 point, indicating that only relevant evidence was shared.
The quality of hypotheses sharing was also measured using a precision indicator. For each patient case, the proportion of diagnoses relevant for the respective patient case to all shared diagnoses was calculated. Which diagnoses were considered relevant for a specific case was determined by an expert solution. As with evidence elicitation, this score was evaluated and converted into a binary variable, where 1 indicated that only relevant diagnoses were shared and 0 indicated that also irrelevant diagnoses were shared, due to the categorical nature of the original data and its skewed distribution, with a majority of responses concentrated in a single category.
Measures—Diagnostic Outcome
We operationalize diagnostic outcome in the pretest case in terms of diagnostic accuracy, diagnostic justification, and diagnostic efficiency.
For diagnostic accuracy, a main diagnosis was assigned to each patient case as expert solution. After working on the patient case and requesting the radiological examination, participants indicated their final diagnosis. To do this, they typed in the first three letters of their desired diagnosis and then received suggestions from a list of 249 possible diagnoses. Diagnostic accuracy was then calculated by coding the agreement between the final diagnosis given and the expert solution. Accurate diagnoses (e.g., hospital-acquired pneumonia) were coded as 1, correct but inaccurate diagnoses (e.g., pneumonia) were coded as 0.5, and incorrect diagnoses were coded as 0. A binary indicator was used for the final diagnostic accuracy score, with 0 indicating an incorrect diagnosis and 1 indicating an at least inaccurate diagnosis, due to the categorical nature of the original data and its skewed distribution, with a majority of responses concentrated in a single category.
A prerequisite for diagnostic justification and diagnostic efficiency is the provision of at least an inaccurate diagnosis. If a participant provided an incorrect diagnosis (coded as 0), diagnostic justification and diagnostic efficiency were immediately scored as 0.
After choosing a final diagnosis, participants were asked to justify their decision in an open text field. Diagnostic justification was then calculated as the proportion of relevant reported information out of all relevant information that would have fully justified the final accurate diagnosis. Again, medical experts agreed on an expert solution that included all relevant information to justify the correct diagnosis. The participants’ solution was coded by two independent coders, each coding the full data, and differences in coding were discussed until the coders agreed. We obtained a range from 0 points, indicating a completely inadequate justification, to 1 point, indicating a completely adequately justified final diagnosis.
Diagnostic efficiency was defined as diagnostic accuracy (non-binary version) divided by the minutes required to solve the case.
Statistical Analyses
To answer the research question, a structural equation model (SEM) was estimated using MPlus Editor, version 8 (Muthén & Muthén, 2017 ). We decided to use a SEM, as it is a comprehensive statistical approach widely used in psychology and educational sciences for its ability to model complex relationships among observed and latent variables while accounting for measurement error (Hilbert & Stadler, 2017 ). SEM support the development and verification of theoretical models, enabling scholars to refine theories and interventions in psychology and education based on empirical evidence, as not only can one relationship be investigated but a system of regressions is also considered simultaneously (Nachtigall et al., 2003 ).
We included all links between the variables and applied a two-step approach, using mean-adjusted and variance-adjusted unweighted least squares (ULSMV, Savalei & Rhemtulla, 2013 ) as the estimator and THETA for parametrization, first examining the measurement model and then the structural model. The assessment of model fit was based on chi-square (χ2), root mean square error of approximation (RMSEA), and comparative fit index (CFI). Model fit is generally indicated by small chi-squared values; RMSEA values of < 0.08 (acceptable) and < 0.06 (excellent), and CFI values ≥ 0.90. We do not consider standardized root mean squared residual (SRMR), because, according to the definition used in MPlus, this index is not appropriate when the sample size is 200 or less, as natural variation in such small samples contributes to larger SRMR values (Asparouhov & Muthén, 2018 ). For hypotheses 1–6, we excluded path coefficients < 0.1 from our interpretation, as they are relatively small. In addition, at least two interpretable path coefficients, of which at least one is statistically significant, are required to find support for the hypothesis. For hypotheses 7–15, specific indirect effects (effect of an individual characteristic on diagnostic outcome through a specific CDA) and total indirect effects (mediation of the effect of an individual characteristic on diagnostic outcome through all mediators) were estimated.
We reported all measures in the study and outlined differences between the three samples. All data and analysis code have been made publicly available at the Open Science Framework (OSF) and can be accessed at https://osf.io/u8t62 . Materials for this study are available by email through the corresponding author. This study’s design and its analysis were not pre-registered.
The descriptive statistics of each measure per study are displayed in Table 4 . The intercorrelations between the measures per study can be found in Appendix Table 7 .
Overall Results of the SEM
All loadings were in the expected directions and statistically significant, except for conceptual knowledge in internal medicine in study C (λ = 0.241, p = .120), conceptual knowledge in radiology in study A (λ = 0.398, p = .018), and strategic knowledge in internal medicine (λ = 0.387, p = .206) and radiology (λ = -0.166, p = .302) in study B. Standardized factor loadings of the measurement model are shown in Appendix Table 8 .
The SEM has a good fit for study A [ X 2 (75) = 74.086, p = .508, RMSEA = 0.00, CFI = 1.00], study B [ X 2 (75) = 68.309, p = .695, RMSEA = 0.000, CFI = 1.00], and study C [ X 2 (75) = 93.816, p = .070, RMSEA = 0.036, CFI = 1.00].
Paths between Individual Characteristics, CDAs, and Diagnostic Outcome
The standardized path coefficients and hypotheses tests for the theoretical model are reported in Table 5 . An overview of the paths supported by the data is shown in Fig. 2 .
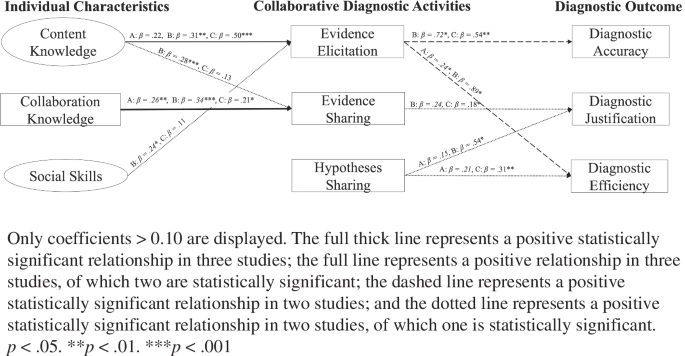
Evidence on supported relationships between individual characteristics, collaborative diagnostic activities, and diagnostic outcome
Overall, the R 2 for the CDAs ranged from medium to high for evidence elicitation and evidence sharing, depending on the study, and were consistently low for hypotheses sharing across all three studies. Looking at diagnostic outcome, R 2 is consistently large for diagnostic accuracy and medium to large for diagnostic justification and diagnostic efficiency (Table 6 ).
The path from content knowledge to evidence elicitation was positive and > 0.1 in all three studies, as well as statistically significant in two of them; therefore, we consider Hypothesis 1a supported. The path from content knowledge to evidence sharing was positive and > 0.1 in two studies, as well as statistically significant in one of them; therefore, Hypothesis 1b is also supported. In contrast, the path from content knowledge to hypotheses sharing was indeed also positive in two studies, but as neither was statistically significant, we conclude that Hypothesis 1c was not supported. The path from collaboration knowledge to evidence elicitation was positive and > 0.1 in only one study, but also not statistically significant. Thus, we found that Hypothesis 2a was not supported. For the path from collaboration knowledge to evidence sharing, we found relevant positive and statistically significant coefficients in all three studies. Hypothesis 2b is therefore fully supported by the data. This is not the case for Hypothesis 2c, for which we found no coefficient > 0.1 for the path from collaboration knowledge to hypotheses sharing. For the path from social skills to evidence elicitation, we found positive coefficients > 0.1 in two out of three studies, of which one was also statistically significant. Thus, we consider Hypothesis 3a to be supported. For the path from social skills to evidence sharing, we again found one statistically significant positive coefficient, but in the other two studies it was < 0.1. Therefore, we do not consider Hypothesis 3b to be supported by the data. The same applies to the path from social skills to hypotheses sharing, where the coefficient is < 0.1 in two studies. We therefore do not consider Hypothesis 3c to be supported.
The path from evidence elicitation to diagnostic accuracy was statistically significant and large in magnitude in two out of three studies. Hypothesis 4a is therefore supported. The path from evidence elicitation to diagnostic justification was only positive and > 0.1 in one study, which was also not statistically significant. Therefore, we find no support for Hypothesis 4b. In contrast, the path from evidence elicitation to diagnostic efficiency was positive and statistically significant in two out of three studies, with one large effect. Hypothesis 4c is therefore supported. The path from evidence sharing to diagnostic accuracy was only positive and reasonably large in one study. Therefore, we do not find support for Hypothesis 5a. The path from evidence sharing to diagnostic justification was positive and > 0.1 in two studies as well as statistically significant in one of them, so Hypothesis 5b is supported. In contrast, we did not find a positive coefficient > 0.1 for the path from evidence sharing to diagnostic efficiency. Therefore, Hypothesis 5c is not supported by the data. Although we found coefficients > 0.1 in two studies for the path from hypotheses sharing to diagnostic accuracy, we found no support for Hypothesis 6a, as none of these was statistically significant. This is different for Hypothesis 6b, as we found two positive paths from hypotheses sharing to diagnostic justification, one of which was statistically significant and large. Finally, we found two positive paths from evidence sharing to diagnostic efficiency in three studies, one of which was statistically significant. Hypothesis 6c is therefore supported.
Indirect Effects between Individual Characteristics, CDA, and Diagnostic Outcome
Indirect effects of CDAs on the effect of individual characteristics on the diagnostic outcome in CDR were estimated to test hypotheses 7–15. Although we found a mediating effect of all CDAs (β = .31, p = .008), and specifically for evidence elicitation (β = .27, p = .021) from content knowledge on diagnostic accuracy in study C, and some significant overall and direct effects for other relationships (Appendix Table 9 ), none of these were consistent across all of the studies. Thus, we conclude no consistent support for any of the Hypotheses 7–15.
The aim of the current study was to investigate the extent to which the relationships specified in the CDR model (Radkowitsch et al., 2022 ) are applicable across studies, to better understand the processes underlying CDR in knowledge-rich domains. Not only is this exploration crucial for the medical field or collaborative problem-solving in knowledge-rich domains, but it also offers valuable insights for computer-supported collaborative learning research. Despite CDR’s specific focus, the principles and findings have relevant implications for understanding and enhancing collaborative processes in various educational and professional settings.
Specifically, we investigated how individual learner characteristics, the CDAs, and the diagnostic outcome are related. We therefore analyzed data from three independent studies, all from the same context, a simulation-based environment in the medical domain. Our study found positive relationships between content knowledge and the quality of evidence elicitation as well as the quality of evidence sharing, but not for the quality of hypotheses sharing. Furthermore, collaboration knowledge is positively related to the quality of evidence sharing, but not to the quality of evidence elicitation and the quality of hypotheses sharing. Social skills are only positively related to the quality of evidence elicitation. This underscores the multifaceted nature of collaborative problem-solving situations. Thus, effective CDR, a form of collaborative problem-solving, necessitates a nuanced understanding of the interplay between individual characteristics and CDAs.
The relevance of content knowledge for diagnostic competence is well established in research (Chernikova et al., 2020 ). To develop any diagnostic skills in knowledge-rich domains, learners need to acquire large amounts of knowledge and to restructure it through experience with problem-solving procedures and routines (Boshuizen et al., 2020 ). In the case of CDR this enables the diagnostician to come up with an initial suspected diagnosis, which is likely to be relevant information for the collaboration partner and to guide the further CDAs effectively. The finding that content knowledge only has a relation to the quality of evidence elicitation but none of the other CDAs can be explained by the fact that evidence elicitation is the least transactive CDA within the collaborative decision-making process. When eliciting evidence, the collaboration partner is used as an external knowledge resource (Weinberger & Fischer, 2006 ). So, despite being a collaborative activity, evidence elicitation is about what information from the collaboration partner is needed rather than what the collaboration partner needs. Thus, elicitation is less transactive than sharing, which is focused at what the collaboration partner needs.
Not only content knowledge but also collaboration knowledge is related to the quality of evidence sharing. This finding implies that collaboration knowledge may influence the CDR above and beyond individual content knowledge. It also supports the differentiation of knowledge types made in the CDR model (Radkowitsch et al., 2022 ). Thus, it is important to learn not only the conceptual and strategic medical knowledge that is required for diagnosing but also knowledge about what information is relevant for specific collaboration partners when diagnosing collaboratively. This finding underpins the importance of being aware of the knowledge distribution among collaboration partners and the relevance of the transactive memory (Wegner, 1987 ). Thus, for collaborative problem-solving in knowledge-rich domains—as for computer-supported collaborative learning more generally—knowledge and information awareness is crucial (Engelmann & Hesse, 2010 ).
Thus, the relevance of collaboration knowledge in collaborative problem-solving is an important finding of our study, highlighting that it is critical in facilitating effective collaborative processes and outcomes. The current findings emphasize the need for educational strategies that explicitly target the development of collaborative knowledge to ensure that learners have the knowledge and skills necessary to participate in productive collaborative problem-solving and computer-supported collaborative learning processes. In doing so, the CDR model emphasizes the need for learners to master collaborative skills and build shared problem representations to take full advantage of collaborative learning opportunities.
As CDR is conceptualized to be an interplay of cognitive and social skills (Hesse et al., 2015 ), we also assumed that social skills are related to CDAs. However, we only found evidence of the expected relationship between social skills and CDAs for the quality of evidence elicitation. One explanation could be that collaboration knowledge was relatively high in all three samples, outweighing the influences of general skills. This is consistent with the assumption of the CDR model that the influence of more general social skills is reduced with an increasing level of professional collaboration knowledge (Radkowitsch et al., 2022 ). When collaboration knowledge is available to the diagnosticians, it becomes more important than social skills. This finding again underlines the importance of collaboration knowledge, which can be seen as a domain- and profession-specific development of social skills. However, another explanation could be that, when collaborating with an agent, the effect of social skills decreases, as the agent was not programmed to respond to social nuances. The design of the simulation would thus buffer against the effect of social skills. Although the study by Herborn et al. ( 2020 ) found no differences between human-to-human and human-to-agent collaboration, this does not necessarily invalidate the potential variability in outcomes associated with the social skills incorporated into the agent. For a thorough investigation into the impact of social skills, the agent would need variable social abilities, enabling the variation of the importance of basic social skills for successful collaboration.
Further, we need to conclude that there is no support for a relationship between the individual characteristics and hypotheses sharing, as we found no stable support for the relationship between any of the individual characteristics and the quality of hypotheses sharing. One possible explanation could be that the binary precision measure used to operationalize quality in hypotheses sharing is not sensitive enough or is not capturing the relevant aspect of quality in that activity. Another explanation could be that there is no direct relationship between the individual characteristics and hypotheses sharing, as this relationship is mediated by evidence sharing and thus influenced by the activated knowledge scripts (Schmidt & Rikers, 2007 ).
Looking at the relationships between CDAs and the diagnostic outcome, the current results highlight the need to distinguish between primary (diagnostic accuracy) and secondary (diagnostic justification and efficiency) outcomes of diagnostic reasoning (Daniel et al., 2019 ). Achieving diagnostic accuracy, a purely quantitative outcome measure, is less transactive than other aspects of the diagnostic outcome. This is also where we find the link to evidence elicitation, as we consider this to be the least transactive CDA within the collaborative decision-making process. However, the ability to justify and reach this decision efficiently is then highly dependent on evidence sharing and hypotheses sharing, activities that are more focused on transactivity within CDR (Weinberger & Fischer, 2006 ).
Although individual learner characteristics are found to have an effect on CDAs, and CDAs impact the diagnostic outcome, the effect is not mediated by CDAs across studies. Thus, we assume that, for effective collaborative problem-solving in knowledge-rich domains, such as CDR, it is not enough to have sufficient content and collaboration knowledge; it is also necessary to be able to engage in high quality CDAs to achieve a high-quality diagnostic outcome. This is consistent with research on individual diagnostic reasoning, which shows that diagnostic activities have a unique contribution to the diagnostic outcome after controlling for content knowledge (Fink et al., 2023 ).
In summary, we explored evidence elicitation, evidence sharing, and hypotheses sharing as crucial CDAs. The findings revealed diverse associations of these CDAs with individual characteristics and facets of the diagnostic outcome, supporting the notion that the CDR-process involves a variety of different skills (instead of being one overarching skill). On the basis of these results, we propose categorizing CDAs into activities primarily focused on individual goals and needs (e.g., elicitation) and more transactive activities directly targeted at the collaborator (e.g., sharing). To enhance quality in CDAs, instructional support should be considered. For instance, providing learners with an adaptive collaboration script has been shown to improve evidence sharing quality and promote the internalization of collaboration scripts, fostering the development of collaboration knowledge (Radkowitsch et al., 2021 ). Further, group awareness tools, such as shared concept maps, should be considered to compensate for deficits in one’s collaboration knowledge (Engelmann & Hesse, 2010 ). However, what is required to engage in high-quality CDAs remains an open question. One starting point is domain-general cognitive skills. These could influence CDAs, particularly in the early stages of skill development (Hetmanek et al., 2018 ). Previous research showed that, in diagnostic reasoning, instructional support is more beneficial when being domain-specific than domain-general (Schons et al., 2022 ). Thus, there is still a need for further research on how such instructional support might look like.
Future Research
Although we used data from three studies, all of them were in the same domain; thus, it remains an open question whether these findings are applicable across domains. The CDR model claims that the described relationships are not limited to the medical domain, but rather are valid across domains for collaboratively solving complex problems in knowledge-rich domains. Future research should explore generalizability, for example, for teacher education, which is a distinct field that also requires diagnosing and complex problem-solving (Heitzmann et al., 2019 ).
Regardless of domain, the non-mediating relationship of CDAs between individual characteristics and diagnostic outcomes, as well as the found effects of the CDAs in the current study, suggests that an isolated analysis of CDAs does not fully represent the complex interactions and relationships among activities, individual characteristics, and diagnostic outcomes. Future studies might assess CDAs as a bundle of necessary activities, including a focus on their possible non-linear interactions. We propose to use process data analysis to account for the inherent complexity of the data, as different activities in different sequences can lead to the same outcome (Y. Chen et al., 2019 ). More exploratory analyses of fine-grained, theory-based sequence data are needed to provide insights into more general and more specific processes involved in successful solving complex problems collaboratively (Stadler et al., 2020 ).
As our results have shown, collaboration knowledge and thus awareness of the knowledge distribution among collaboration partners is highly relevant. While a recent meta-analyses showed a moderate effect of group awareness of students’ performance in computer-supported collaborative learning (D. Chen et al., 2024 ), it has so far not been systematically investigated in collaborative problem-solving. Thus, more research on the influence collaboration knowledge in collaborative problem-solving is needed.
Further, additional factors associated with success in collaborative problem-solving—not yet incorporated into the model and thus not yet investigated systematically—include communication skills (OECD, 2017 ), the self-concept of problem-solving ability (Scalise et al., 2016 ), and positive activating emotions during problem-solving tasks (Camacho-Morles et al., 2019 ).
Limitations
There are, however, some limitations to be considered. One is that we have only considered CDAs and how they relate to individual characteristics and outcomes. However, the CDR model also introduces individual diagnostic activities, such as the generation of evidence and the drawing of conclusions. These occur before and after the CDAs and may therefore also have an impact on the described relationships. However, we decided to focus on the CDAs within the CDR process because they are particularly relevant for constructing a shared problem representation, being central to CDR. Future research might consider these individual diagnostic activities, as they could, for example, further explain the how content knowledge is related to the diagnostic outcome.
Another limitation of the current analyses is the operationalization of quality for the CDAs. We chose the appropriateness of radiological examination for the indicated diagnosis for quality of evidence elicitation and precision for quality of evidence sharing and hypotheses sharing. However, all of these only shed light on one perspective of each activity, while possibly obscuring others. For example, it may be that content knowledge is not related to the precision of hypotheses sharing, but this may be different when looking at other quality indicators, such as sensitivity or specificity. However, we decided to use the precision aspect of activities, as research shows that collaborators often fail to identify relevant information, and the amount of information is not related to performance (Tschan et al., 2009 ). Future research may explore a broader variety of quality indicators to be able to assess the quality of CDAs as comprehensively as possible. It should also be noted that in study B a suppression effect (Horst, 1941 ) between hypothesis sharing and evidence elicitation artificially inflated the observed effect size. This is to be expected with process data that can be highly correlated and needs to be considered when interpreting the effect sizes.
In addition, it should be noted that the omega values obtained for the conceptual and strategic knowledge measures were below the commonly accepted threshold of 0.7. While we chose to use omega values as a more appropriate measure of reliability in our context, given the complex and multifaceted nature of the knowledge constructs, these lower-than-expected values raise important questions about the quality of the data and the robustness of the findings. Thus, it is important to understand that knowledge constructs, by their very nature, may not always exhibit high levels of internal consistency due to the diverse and interrelated components they encompass (Edelsbrunner, 2024 ; Stadler et al., 2021 ; Taber, 2018 ). This complexity may be reflected in the moderate omega values observed, which, while seemingly counterintuitive, does not invalidate the potential of the constructs to account for substantial variance in related outcomes. However, findings related to these constructs should be interpreted with caution, and the results presented should be considered tentative. Future research should further explore the implications of using different reliability coefficients in assessing complex constructs within the learning sciences, potentially providing deeper insights into the nuanced nature of knowledge and its measurement.
Another limitation of this study is related to the agent-based collaboration, as a predictive validation of collaborative problem-solving for later human-to-human collaboration in comparable contexts has not yet been systematically conducted. Although the agent-based collaboration situation used has been validated in terms of perceived authenticity, it still does not fully correspond to a real collaboration situation (Rosen, 2015 ). This could be an explanation for the low influence of social skills, as the setting might not require the application of a broad set of social skills (Hesse et al., 2015 ; Radkowitsch et al., 2020 ). In a real-life collaboration, the effects of social skills might be more pronounced. However, research showed that the human-to-agent approach did not lead to different results in collaborative problem-solving than the human-to-human approach in the 2015 PISA study, and correlations with other measures of collaborative skills have been found (Herborn et al., 2020 ; Stadler, Herborn et al., 2020 ). Future studies should specifically test the relevance of social skills for CDR in a human-to-human setting to strengthen the generalizability of our findings.
In conclusion, the current study highlights the importance of individual characteristics and CDAs as independent predictors for achieving good diagnoses in collaborative contexts, at least in the simulation-based settings we used in the studies included in our analysis. Collaboration knowledge emerged as a critical factor, demonstrating its importance over early acquired, general social skills. Therefore, it is imperative to revise the CDR approach by giving higher priority to the proficiency of collaboration knowledge compared with social skills. Furthermore, we conclude that, in simulation-based CDR, content knowledge does not play such a crucial role in predicting diagnostic success compared with many other educational settings, most probably because of the endless opportunities for retrying and revising in simulation-based learning environments.
With respect to CDAs, we suggest refining the perspective on the quality of CDAs and consider revising the CDR model by summarizing CDAs as information elicitation and information sharing, with the former being less transactive, and thus, less demanding than the latter. Adequate performance in both types of CDA is presumed to result in a high-quality shared problem representation, resulting in good diagnostic outcome. Collaborative problem-solving skills are highly relevant in professional practice of knowledge-rich domains, highlighting the need to strengthen these skills in students engaged in CDR and to provide learning opportunities accordingly. Further, the ability to effectively collaborate and construct shared problem representations is important, not only in CDR but also in collaborative problem-solving and computer-supported collaborative learning more in general, highlighting the need for integrating such skills into curricula and instructional design.
By emphasizing these aspects, we can improve the diagnostic skills of individuals in collaborative settings. Through advancing our understanding of CDR, we are taking a key step forward in optimizing collaborative problem-solving and ultimately contributing to improved diagnostic outcomes in various professional domains beyond CDR in medical education. In particular, integrating collaboration knowledge and skills into computer-supported collaborative learning environments can enrich learning experiences and outcomes in various knowledge-rich domains.
Please note that the data employed in this study have been used in previous publications (e.g., Brandl et al., 2021 ; Radkowitsch, et al., 2021 ; Richters et al., 2022 ). However, the research question and the results reported in this study are completely unique to this study.
Abele, S. (2018). Diagnostic problem-solving process in professional contexts: theory and empirical investigation in the context of car mechatronics using computer-generated log-files. Vocations and Learning, 11 (1), 133–159. https://doi.org/10.1007/s12186-017-9183-x
Article Google Scholar
Asparouhov, T., & Muthe´n, B. (2018). SRMR in Mplus . https://www.statmodel.com/download/SRMR2.pdf
Bauer, E., Sailer, M., Kiesewetter, J., Fischer, M. R., & Fischer, F. (2022). Diagnostic argumentation in teacher education: Making the case for justification, disconfirmation, and transparency. Frontiers in Education , 7 , Article 977631. https://doi.org/10.3389/feduc.2022.977631
Boshuizen, H. P., Gruber, H., & Strasser, J. (2020). Knowledge restructuring through case processing: the key to generalise expertise development theory across domains? Educational Research Review, 29 , 100310. https://doi.org/10.1016/j.edurev.2020.100310
Brandl, L., Richters, C., Radkowitsch, A., Obersteiner, A., Fischer, M. R., Schmidmaier, R., Fischer, F., & Stadler, M. (2021). Simulation-based learning of complex skills: Predicting performance with theoretically derived process features. Psychological Test and Assessment Modeling, 63 (4), 542–560. https://www.psychologie-aktuell.com/fileadmin/Redaktion/Journale/ptam-2021-4/PTAM__4-2021_6_kor.pdf
Braun, L. T., Zottmann, J. M., Adolf, C., Lottspeich, C., Then, C., Wirth, S., Fischer, M. R., & Schmidmaier, R. (2017). Representation scaffolds improve diagnostic efficiency in medical students. Medical Education, 51 (11), 1118–1126. https://doi.org/10.1111/medu.13355
Camacho-Morles, J., Slemp, G. R., Oades, L. G., Morrish, L., & Scoular, C. (2019). The role of achievement emotions in the collaborative problem-solving performance of adolescents. Learning and Individual Differences, 70 , 169–181. https://doi.org/10.1016/j.lindif.2019.02.005
Chen, D., Zhang, Y., Luo, H., Zhu, Z., Ma, J., & Lin, Y. (2024). Effects of group awareness support in CSCL on students’ learning performance: a three-level meta-analysis. International Journal of Computer-Supported Collaborative Learning, 19 (1), 97–129. https://doi.org/10.1007/s11412-024-09418-3
Chen, Y., Li, X., Liu, J., & Ying, Z. (2019). Statistical analysis of complex problem-solving process data: an event history analysis approach. Frontiers in Psychology , 10 , Article 486. https://doi.org/10.3389/fpsyg.2019.00486
Chernikova, O., Heitzmann, N., Fink, M. C., Timothy, V., Seidel, T., & Fischer, F. (2020). Facilitating diagnostic competences in higher education—a meta-analysis in medical and teacher education. Educational Psychology Review, 32 (1), 157–196. https://doi.org/10.1007/s10648-019-09492-2
Chernikova, O., Heitzmann, N., Opitz, A., Seidel, T., & Fischer, F. (2022). A theoretical framework for fostering diagnostic competences with simulations in higher education. In F. Fischer & A. Opitz (Eds.), Learning to Diagnose with Simulations . Springer, Cham. https://doi.org/10.1007/978-3-030-89147-3_2
Cook, D. A., Brydges, R., Zendejas, B., Hamstra, S. J., & Hatala, R. M. (2013). Technology-enhanced simulation to assess health professionals: a systematic review of validity evidence, research methods, and reporting quality. Academic Medicine: Journal of the Association of American Medical Colleges, 88 (6), 872–883. https://doi.org/10.1097/ACM.0b013e31828ffdcf
Daniel, M., Rencic, J., Durning, S. J., Holmboe, E. S., Santen, S. A., Lang, V., Ratcliffe, T., Gordon, D., Heist, B., Lubarsky, S., Estrada, C. A., Ballard, T., Artino, A. R., Da Sergio Silva, A., Cleary, T., Stojan, J., & Gruppen, L. D. (2019). Clinical reasoning assessment methods: a scoping review and practical guidance. Academic Medicine: Journal of the Association of American Medical Colleges, 94 (6), 902–912. https://doi.org/10.1097/ACM.0000000000002618
Dunbar, K. (1995). How scientists really reason: scientific reasoning in real-world laboratories. In R. J. Sternberg & J. E. Davidson (Eds.), The nature of insight (pp. 365–395). MIT Press.
Google Scholar
Edelsbrunner, P. A. (2024). Does interference between intuitive conceptions and scientific concepts produce reliable inter-individual differences? Science & Education. Advance online publication. https://doi.org/10.1007/s11191-024-00500-8
Book Google Scholar
Engelmann, T., & Hesse, F. W. (2010). How digital concept maps about the collaborators’ knowledge and information influence computer-supported collaborative problem solving. International Journal of Computer-Supported Collaborative Learning, 5 (3), 299–319. https://doi.org/10.1007/s11412-010-9089-1
Fink, M. C., Heitzmann, N., Reitmeier, V., Siebeck, M., Fischer, F., & Fischer, M. R. (2023). Diagnosing virtual patients: the interplay between knowledge and diagnostic activities. Advances in Health Sciences Education : Theory and Practice , 1–20. https://doi.org/10.1007/s10459-023-10211-4
Fiore, S. M., Graesser, A. C., & Greiff, S. (2018). Collaborative problem-solving education for the twenty-first-century workforce. Nature Human Behaviour, 2 (6), 367–369. https://doi.org/10.1038/s41562-018-0363-y
Fischer, F., Kollar, I., Stegmann, K., & Wecker, C. (2013). Toward a script theory of guidance in computer-supported collaborative learning. Educational Psychologist, 48 (1), 56–66. https://doi.org/10.1080/00461520.2012.748005
Fischer, F., Kollar, I., Ufer, S., Sodian, B., Hussmann, H., Pekrun, R., Neuhaus, B. J., Dorner, B., Pankofer, S., Fischer, M. R., Strijbos, J.‑W., Heene, M., & Eberle, J. (2014). Scientific reasoning and argumentation: advancing an interdisciplinary research agenda in education. Frontline Learning Research , 2 (3), 28–45. https://doi.org/10.14786/flr.v2i2.96
Fischer, M. R., Kopp, V., Holzer, M., Ruderich, F., & Jünger, J. (2005). A modified electronic key feature examination for undergraduate medical students: validation threats and opportunities. Medical Teacher, 27 (5), 450–455. https://doi.org/10.1080/01421590500078471
Förtsch, C., Sommerhoff, D., Fischer, F., Fischer, M. R., Girwidz, R., Obersteiner, A., Reiss, K., Stürmer, K., Siebeck, M., Schmidmaier, R., Seidel, T., Ufer, S., Wecker, C., & Neuhaus, B. J. (2018). Systematizing professional knowledge of medical doctors and teachers: development of an interdisciplinary framework in the context of diagnostic competences. Education Sciences, 8 (4), 207. https://doi.org/10.3390/educsci8040207
Goldhammer, F., Naumann, J., Rölke, H., Stelter, A., & Tóth, K. (2017). Relating product data to process data from computer-based competency assessment. In D. Leutner, J. Fleischer, J. Grünkorn, & E. Klieme (Eds.), Methodology of educational measurement and assessment. competence assessment in education (pp. 407–425). Springer International Publishing. https://doi.org/10.1007/978-3-319-50030-0_24
Graesser, A. C., Fiore, S. M., Greiff, S., Andrews-Todd, J., Foltz, P. W., & Hesse, F. W. (2018). Advancing the science of collaborative problem solving. Psychological Science in the Public Interest: A Journal of the American Psychological Society, 19 (2), 59–92. https://doi.org/10.1177/1529100618808244
Hautz, W. E., Kämmer, J. E., Schauber, S. K., Spies, C. D., & Gaissmaier, W. (2015). Diagnostic performance by medical students working individually or in teams. JAMA, 313 (3), 303–304. https://doi.org/10.1001/jama.2014.15770
Heitzmann, N., Seidel, T., Hetmanek, A., Wecker, C., Fischer, M. R., Ufer, S., Schmidmaier, R., Neuhaus, B. J., Siebeck, M., Stürmer, K., Obersteiner, A., Reiss, K., Girwidz, R., Fischer, F., & Opitz, A. (2019). Facilitating diagnostic competences in simulations in higher education: a framework and a research agenda. Frontline Learning Research , 1–24. https://doi.org/10.14786/flr.v7i4.384
Herborn, K., Stadler, M., Mustafić, M., & Greiff, S. (2020). The assessment of collaborative problem solving in PISA 2015: can computer agents replace humans? Computers in Human Behavior, 104 , 105624. https://doi.org/10.1016/j.chb.2018.07.035
Hesse, F. W., Care, E., Buder, J., Sassenberg, K., & Griffin, P. (2015). A framework for teachable collaborative problem solving skills. In P. Griffin & E. Care (Eds.), Assessment and Teaching of 21 st Century Skills (pp. 37–56). Springer.
Chapter Google Scholar
Hetmanek, A., Engelmann, K., Opitz, A., & Fischer, F. (2018). Beyond intelligence and domain knowledge. In F. Frank, C. Clark A., E. Katharina, O. Jonathan, F. Fischer, C. A. Chinn, K. Engelmann, & J. Osborne (Eds.), Scientific reasoning and argumentation (pp. 203–226). Routledge. https://doi.org/10.4324/9780203731826-12
Hilbert, S., & Stadler, M. (2017). Structural equation models. In V. Zeigler-Hill & T. K. Shackelford (Eds.), Encyclopedia of Personality and Individual differences (pp. 1–9). Springer International Publishing. https://doi.org/10.1007/978-3-319-28099-8_1285-1
Hitchcock, D. (2005). Good reasoning on the Toulmin model. Argumentation, 19 (3), 373–391. https://doi.org/10.1007/s10503-005-4422-y
Horst, P. (1941). The prediction of personnel adjustment. Socia LScience Research and Council Bulletin (48), 431–436.
Jeong, H., Hmelo-Silver, C. E., & Jo, K. (2019). Ten years of computer-supported collaborative learning: a meta-analysis of CSCL in STEM education during 2005–2014. Educational Research Review, 28 , 100284. https://doi.org/10.1016/j.edurev.2019.100284
Kiesewetter, J., Fischer, F., & Fischer, M. R. (2017). Collaborative clinical reasoning—a systematic review of empirical studies. The Journal of Continuing Education in the Health Professions, 37 (2), 123–128. https://doi.org/10.1097/CEH.0000000000000158
Kiesewetter, J., Sailer, M., Jung, V. M., Schönberger, R., Bauer, E., Zottmann, J. M., Hege, I., Zimmermann, H., Fischer, F., & Fischer, M. R. (2020). Learning clinical reasoning: how virtual patient case format and prior knowledge interact. BMC Medical Education, 20 (1), 73–83. https://doi.org/10.1186/s12909-020-1987-y
Klahr, D., & Dunbar, K. (1988). Dual space search during scientific reasoning. Cognitive Science, 12 (1), 1–48. https://doi.org/10.1207/s15516709cog1201_1
Koschmann, T. D., Feltovich, P. J., Myers, A. C., & Barrows, H. S. (1992). Implications of CSCL for problem-based learning. ACM SIGCUE Outlook, 21 (3), 32–35. https://doi.org/10.1145/130893.130902
Liu, L., Hao, J., Davier, A. A. von, Kyllonen, P., & Zapata-Rivera, J.‑D. (2016). A tough nut to crack: measuring collaborative problem solving. In J. Keengwe, Y. Rosen, S. Ferrara, & M. Mosharraf (Eds.), Handbook of Research on Technology Tools for Real-World Skill Development (pp. 344–359). IGI Global. https://doi.org/10.4018/978-1-4666-9441-5.ch013
Muthén, L. K., & Muthén, B. O. (2017). Mplus: Statistical Analysis with Latent Variables: User’s Guide (Version 8) [Computer software]. Authors.
Nachtigall, C., Kroehne, U., Funke, F., & Steyer, R. (2003). (Why) should we use SEM? Pros and cons of structural equation modeling. Methods of Psychological Research Online, 8 (2), 1–22.
Noroozi, O., Biemans, H. J., Weinberger, A., Mulder, M., & Chizari, M. (2013). Scripting for construction of a transactive memory system in multidisciplinary CSCL environments. Learning and Instruction, 25 , 1–12. https://doi.org/10.1016/j.learninstruc.2012.10.002
OECD. (2017). PISA 2015 Assessment and Analytical Framework: Science, Reading, Mathematic, Financial Literacy and Collaborative Problem Solving, revised edition. PISA, OECD Publishing. https://doi.org/10.1787/9789264281820-en
Pickal, A. J., Engelmann, K., Chinn, C. A., Girwidz, R., Neuhaus, B. J., & Wecker, C. (2023). Fostering the collaborative diagnosis of cross-domain skills in video-based simulations. In Proceedings of the International Conference on Computer-supported for Collaborative Learning, Proceedings of the 16 th International Conference on Computer-Supported Collaborative Learning — CSCL 2023 (pp. 139–146). International Society of the Learning Sciences. https://doi.org/10.22318/cscl2023.638463
Radkowitsch, A., Fischer, M. R., Schmidmaier, R., & Fischer, F. (2020). Learning to diagnose collaboratively: Validating a simulation for medical students. GMS Journal for Medical Education, 37(5), Doc51. https://doi.org/10.3205/zma001344
Radkowitsch, A., Sailer, M., Fischer, M. R., Schmidmaier, R., & Fischer, F. (2022). Diagnosing collaboratively: A theoretical model and a simulation-based learning environment. In F. Fischer & A. Opitz (Eds.), Learning to Diagnose with Simulations . Springer, Cham. https://doi.org/10.1007/978-3-030-89147-3_10
Radkowitsch, A., Sailer, M., Schmidmaier, R., Fischer, M. R., & Fischer, F. (2021). Learning to diagnose collaboratively—effects of adaptive collaboration scripts in agent-based medical simulations. Learning and Instruction, 75 , 101487. https://doi.org/10.1016/j.learninstruc.2021.101487
Richters, C., Stadler, M., Radkowitsch, A., Behrmann, F., Weidenbusch, M., Fischer, M. R., Schmidmaier, R., & Fischer, F. (2022). Making the rich even richer? Interaction of structured reflection with prior knowledge in collaborative medical simulations. In A. Weinberger, W. Chen, D. Hernández-Leo, & B.Che (Chair), International Society of the Learning Sciences. Hiroshima, Japan.
Rochelle, J., & Teasley, S. (1995). The construction of shared knowledge in collaborative problem solving. In C. O’Malley (Ed.), Computer-Supported Collaborative Learning (pp. 66–97). Springer.
Rosen, Y. (2015). Computer-based assessment of collaborative problem solving: exploring the feasibility of human-to-agent approach. International Journal of Artificial Intelligence in Education, 25 (3), 380–406. https://doi.org/10.1007/s40593-015-0042-3
Savalei, V., & Rhemtulla, M. (2013). The performance of robust test statistics with categorical data. British Journal of Mathematical and Statistical Psychology, 66 (2), 201–223. https://doi.org/10.1111/j.2044-8317.2012.02049.x
Scalise, K., Mustafic, M., & Greiff, S. (2016). Dispositions for collaborative problem solving. In S. Kuger, E. Klieme, N. Jude, & D. Kaplan (Eds.), Methodology of Educational Measurement and Assessment. Assessing Contexts of Learning (pp. 283–299). Springer International Publishing. https://doi.org/10.1007/978-3-319-45357-6_11
Schmidt, H. G., & Mamede, S. (2015). How to improve the teaching of clinical reasoning: a narrative review and a proposal. Medical Education, 49 (10), 961–973. https://doi.org/10.1111/medu.12775
Schmidt, H. G., & Rikers, R. M. J. P. (2007). How expertise develops in medicine: knowledge encapsulation and illness script formation. Medical Education, 41 (12), 1133–1139. https://doi.org/10.1111/j.1365-2923.2007.02915.x
Schons, C., Obersteiner, A., Reinhold, F., Fischer, F., & Reiss, K. (2022). Developing a simulation to foster prospective mathematics teachers’ diagnostic competencies: the effects of scaffolding . Advance online publication. https://doi.org/10.1007/s13138-022-00210-0
Stadler, M., Herborn, K., Mustafić, M., & Greiff, S. (2020). The assessment of collaborative problem solving in PISA 2015: an investigation of the validity of the PISA 2015 CPS tasks. Computers & Education, 157 , 103964. https://doi.org/10.1016/j.compedu.2020.103964
Stadler, M., Hofer, S., & Greiff, S. (2020). First among equals: log data indicates ability differences despite equal scores. Computers in Human Behavior, 111 , 106442. https://doi.org/10.1016/j.chb.2020.106442
Stadler, M., Sailer, M., & Fischer, F. (2021). Knowledge as a formative construct: a good alpha is not always better. New Ideas in Psychology , 60. https://doi.org/10.1016/j.newideapsych.2020.100832
Stark, R., Kopp, V., & Fischer, M. R. (2011). Case-based learning with worked examples in complex domains: two experimental studies in undergraduate medical education. Learning and Instruction, 21 (1), 22–33. https://doi.org/10.1016/j.learninstruc.2009.10.001
Stasser, G., & Titus, W. (1985). Pooling of unshared infomration in group decision making: biased information sampling during discussion. Journal of Personality and Social Psychology, 48 (6), 1467–1478.
Taber, K. S. (2018). The use of Cronbach’s alpha when developing and reporting research instruments in science education. Research in Science Education, 48 (6), 1273–1296. https://doi.org/10.1007/s11165-016-9602-2
Tschan, F., Semmer, N. K., Gurtner, A., Bizzari, L., Spychiger, M., Breuer, M., & Marsch, S. U. (2009). Explicit reasoning, confirmation bias, and illusory transactive memory: a simulation study of group medical decision making. Small Group Research, 40 (3), 271–300. https://doi.org/10.1177/1046496409332928
van Joolingen, W. R., & de Jong, T. (1997). An extended dual search space model of scientific discovery learning. Instructional Science, 25 (5), 307–346. https://doi.org/10.1023/A:1002993406499
Vogel, F., Wecker, C., Kollar, I., & Fischer, F. (2017). Socio-cognitive scaffolding with computer-supported collaboration scripts: a meta-analysis. Educational Psychology Review, 29 (3), 477–511. https://doi.org/10.1007/s10648-016-9361-7
Vogel, F., Weinberger, A., Hong, D., Wang, T., Glazewski, K., Hmelo-Silver, C. E., Uttamchandani, S., Mott, B., Lester, J., Oshima, J., Oshima, R., Yamashita, S., Lu, J., Brandl, L., Richters, C., Stadler, M., Fischer, F., Radkowitsch, A., Schmidmaier, R., . . . Noroozi, O. (2023). Transactivity and knowledge co-construction in collaborative problem solving. In Proceedings of the International Conference on Computer-supported for Collaborative Learning, Proceedings of the 16 th International Conference on Computer-Supported Collaborative Learning — CSCL 2023 (pp. 337–346). International Society of the Learning Sciences. https://doi.org/10.22318/cscl2023.646214
Wegner, D. M. (1987). transactive memory: a contemporary analysis of the group mind. In B. Mullen & G. R. Goethals (Eds.), Theories of Group Behavior (pp. 185–208). Springer New York. https://doi.org/10.1007/978-1-4612-4634-3_9
Weinberger, A., & Fischer, F. (2006). A framework to analyze argumentative knowledge construction in computer-supported collaborative learning. Computers & Education, 46 (1), 71–95. https://doi.org/10.1016/j.compedu.2005.04.003
Download references
Open Access funding enabled and organized by Projekt DEAL. The research presented in this contribution was funded by a grant of the Deutsche Forschungsgemeinschaft (DFG, FOR 2385) to Frank Fischer, Martin R. Fischer and Ralf Schmidmaier (FI 792/11-1 & FI 792/11-2)
Author information
Authors and affiliations.
Department of Psychology, Ludwig-Maximilians-Universität München, Leopoldstr. 13, 80802, Munich, Germany
Laura Brandl, Matthias Stadler, Constanze Richters & Frank Fischer
Institute of Medical Education, LMU University Hospital, Ludwig-Maximilians-Universität München, Munich, Germany
Matthias Stadler & Martin R. Fischer
IPN Leibniz Institute for Science and Mathematics Education, Department of Mathematics Education, Kiel, Germany
Anika Radkowitsch
Medizinische Klinik und Poliklinik IV, LMU University Hospital, Ludwig-Maximilians-Universität München, Munich, Germany
Ralf Schmidmaier
You can also search for this author in PubMed Google Scholar
Corresponding author
Correspondence to Laura Brandl .
Ethics declarations
Conflict of interest statement.
On behalf of all authors, the corresponding author states that there is no conflict of interest.
Additional information
Publisher's note.
Springer Nature remains neutral with regard to jurisdictional claims in published maps and institutional affiliations.
Please note that the data employed in this study have been used in previous publications (e.g., Brandl et al., 2021; Radkowitsch, et al., 2021; Richters et al., 2022 ). However, the research question and the results reported in this study are completely unique to this study. An initial version of this article is presented as a poster at ISLS 2024.
see Tables 7 , 8 and 9
Rights and permissions
Open Access This article is licensed under a Creative Commons Attribution 4.0 International License, which permits use, sharing, adaptation, distribution and reproduction in any medium or format, as long as you give appropriate credit to the original author(s) and the source, provide a link to the Creative Commons licence, and indicate if changes were made. The images or other third party material in this article are included in the article's Creative Commons licence, unless indicated otherwise in a credit line to the material. If material is not included in the article's Creative Commons licence and your intended use is not permitted by statutory regulation or exceeds the permitted use, you will need to obtain permission directly from the copyright holder. To view a copy of this licence, visit http://creativecommons.org/licenses/by/4.0/ .
Reprints and permissions
About this article
Brandl, L., Stadler, M., Richters, C. et al. Collaborative Problem-Solving in Knowledge-Rich Domains: A Multi-Study Structural Equation Model. Intern. J. Comput.-Support. Collab. Learn (2024). https://doi.org/10.1007/s11412-024-09425-4
Download citation
Received : 18 September 2023
Accepted : 09 May 2024
Published : 24 June 2024
DOI : https://doi.org/10.1007/s11412-024-09425-4
Share this article
Anyone you share the following link with will be able to read this content:
Sorry, a shareable link is not currently available for this article.
Provided by the Springer Nature SharedIt content-sharing initiative
- Collaborative Problem-solving
- Simulation-based Learning Environment
- Diagnostic Activities
- Diagnostic Reasoning
- Medical Education
- Find a journal
- Publish with us
- Track your research
Thank you for visiting nature.com. You are using a browser version with limited support for CSS. To obtain the best experience, we recommend you use a more up to date browser (or turn off compatibility mode in Internet Explorer). In the meantime, to ensure continued support, we are displaying the site without styles and JavaScript.
- View all journals
- Explore content
- About the journal
- Publish with us
- Sign up for alerts
- Open access
- Published: 28 June 2024
Research on distribution strategy of logistics enterprise alliance based on three-party evolution game
- Wenxue Ran 1 ,
- Dandan He 1 ,
- Zhaoxia Li 1 ,
- Yun Xue 1 ,
- Zhenzhen He 2 &
- Aravinda Dananjaya Basnayaka Basnayaka Gunarathnage 1
Scientific Reports volume 14 , Article number: 14894 ( 2024 ) Cite this article
Metrics details
- Climate-change mitigation
- Sustainability
Currently, collaborative distribution models have not reached the optimal state of carbon emissions. The cost of additional low-carbon expenditures and the problem of carbon data verification have led to the lack of motivation for reducing emissions among collaborative distribution enterprises. Therefore, how to incentivize them to adopt the low-carbon model is crucial for achieving low-carbon goal. By relying on a government-led digital platform, this paper designs a dual low-carbon incentive strategy to encourage enterprise-alliance to adopt a low-carbon distribution model. In this paper, we first construct an evolutionary game model of the government, enterprise-alliance and end-users; then we explore the conditions of the three-party equilibrium evolution strategy by solving the model and analyzing the stability; and finally, we conduct simulation validation and results analysis with the help of MATLAB. In summary, we found that government punishment is more effective at regulating enterprise-alliance than reward. End-users’ behavior is affected by the costs they need to bear, and they no longer support enterprise-alliance to carry out collaborative low-carbon distribution above a certain threshold.
Introduction
In recent years, China's carbon emissions trading market has experienced unprecedented development, and the carbon trading mechanism has entered a new phase. At the same time, consumer attitudes have also changed, shifting from the original affordable to low-carbon environmental protection. Research shows that consumers' increased preference for low-carbon makes them pay more attention to the low-carbon attributes of products when they buy them, and they are willing to pay a high price for them. As a result, the end-users' low-carbon preference has become an important consideration in logistics distribution decisions 1 .
Logistics enterprise-alliance is a cooperative organization formed by a number of logistics enterprises to improve the overall efficiency and competitiveness of the logistics industry. Logistics enterprise alliance as a kind of enterprise relationship concluded voluntarily between enterprises. It has the characteristics of resource sharing, platform building and result sharing, so it can meet the individualized needs of logistics enterprises and make the relationship between logistics enterprises stable and long-term 2 . With the goal of "exploring the best opportunities for collective competitive advantage", the Alliance draws on the management philosophy of virtual enterprises and integrates them according to specific needs 3 . At present, there are enterprise alliances, such as cold chain logistics alliance, sea-railway logistics alliance, railroad logistics alliance, e-commerce logistics alliance, transportation logistics alliance, cross-border logistics alliance and so on.
Under the traditional model, logistics distribution enterprises have difficulties monitoring and managing carbon emissions, leading to inaccurate data and nontransparent supervision. To solve this problem, a collaborative distribution model with a digital platform has emerged 4 . Collaborative distribution refers to the modernized distribution method in which logistics enterprise alliance use online platforms to integrate distribution orders from different enterprises and jointly complete distribution tasks. In this way, distribution efficiency can be improved, and distribution costs and carbon emissions can be significantly reduced 5 . Collaborative distribution becomes more important with the boom of low-carbon goals and the in-depth study of low-carbon concepts. The digitization platform mentioned in this paper is led by the government and is currently free for distribution enterprises. In the initial stage, the government-run digital platform can incentivize distribution enterprises to participate in collaboration, account for carbon emissions in the distribution process, provide data support for low-carbon incentives, and effectively connect with the carbon trading market 6 . The flowchart of the enterprise-alliance for collaborative distribution in this paper is shown in Fig. 1 .
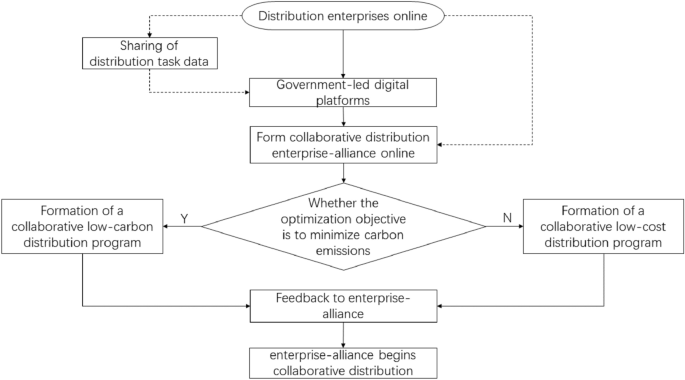
Schematic diagram of enterprise-alliance collaborative distribution.
There are many studies on collaborative distribution among enterprises. Rao et al. designed an optimal price discount strategy to incentivize customers to participate in collaborative distribution 7 . Zhou et al. studied the optimization problem of collaborative distribution using vehicle piggybacking to carry out collaboration 8 . Qie et al. developed a cost sharing model based on the consensual function to solve the problem of cost allocation among enterprises 9 . Chu et al. argued that the joint distribution improves the delivery rate of express delivery in the last-mile logistics 10 . Rao et al. constructed a cost minimization collaborative distribution model to analyze the necessity of coalition splitting 11 . Improving vehicle utilization through a collaborative distribution approach enables firms to contribute to the three pillars of sustainable development by reducing fuel consumption and emissions, increasing profitability, and improving customer satisfaction 12 . Rao et al. proposed a vehicle make-up strategy of finding idle vehicles from within the alliance and then leasing vehicles from outside the alliance, and constructed a quantitative model for the cost of multi-party collaborative distribution considering the constraints on the distribution capacity of the enterprises 13 . Han et al. argued that the distribution of common profit has always been a key obstacle to the effective development of joint distribution in the context of green and low-carbon, and explored a fairer and more reasonable profit distribution scheme 14 . Chen et al. develop a cold chain logistics model considering joint distribution and carbon trading mechanism 15 ; Rao et al. design a default recovery and loss compensation mechanism for members' withdrawal from an alliance in collaborative distribution 16 .
Evolutionary game theory is widely used to analyze the strategy selection problem under the participation of multiple subjects. Li et al. constructed a developer-government-consumer three-way evolutionary game model by combining the carbon trading prospect theory 17 . Based on evolutionary game theory, Zeng et al. explored the influence of platform reward and punishment mechanisms on the low-carbon strategy selection of manufacturing enterprises 18 . Lin et al. studied the strategic decision-making process of two participants in the context of medical malpractice based on the evolutionary game model of doctors and patients 19 . Wu et al. developed a game theory model involving packaging suppliers and logistics companies and based on the theory of corporate social responsibility 20 . Tang et al. constructed a three-party evolutionary game model to analyze the strategic choices of automobile companies, consumers and the Chinese government 21 . Gong et al. constructed a three-party evolutionary game model of cloud manufacturing platform, service provider and service demander, and analyzed the evolutionary stabilization strategy 22 . Yuan et al. constructed a three-party evolutionary game model to study the allocation of medical supplies in the rescue environment of public health emergencies under the condition of information incompleteness 23 . Wang et al. constructed an evolutionary game model of value co-creation in a cloud manufacturing innovation ecosystem, and studied the relationship between platform enterprises and government participation behaviors under dynamic and static reward and punishment mechanisms 24 . Qiu et al. constructed a three-party evolutionary game model of e-commerce platforms, merchants, and consumers, and examined the impacts of the influencing factors on the strategic choices of each party 25 . Chen et al. analyzed the game between the government and polluting enterprises under the environmental tax system through an evolutionary game model 26 .
From the above review, it can be seen that current researches on the collaborative distribution of logistics enterprise alliance have focused mainly on alliance splitting strategies, cost sharing methods and the mechanism of recovery and compensation for members' withdrawal from the alliance. Researches on collaborative distribution participants has considered only the influence of government regulatory decisions. In this paper, the distribution strategy of logistics enterprise alliance is investigated considering government reward and punishment as well as end-users' low-carbon preference. Therefore, this paper constructs an evolutionary game model of the government, enterprise-alliance and end-users, to consider the impact of the government's reward and punishment mechanism and end-users' low-carbon preference on the distribution strategy of enterprise-alliance. The research questions that are addressed in this paper are as follows: (1) From the analysis of the evolutionary route, what are the differences in the dynamic evolutionary process of the game subjects under different strategy choices of multiple subjects? (2) From the perspective of supply-side government policy analysis, what is the impact of government reward and punishment mechanism on the distribution strategy of logistics enterprise-alliance? (3) From the analysis of end-user behavior on the demand side, how does the end-user's low-carbon preference affect the distribution strategy of logistics enterprise-alliance?
Model construction
Description of the problem.
The collaborative distribution of logistics enterprise-alliance can be categorized into collaborative low-cost distribution and collaborative low-carbon distribution according to the distribution mode 27 . The enterprise-alliance usually strives to maximize profits, so it prefers to choose collaborative low-cost distribution, while the government is committed to maximizing social benefits, and hopes that the enterprise-alliance will choose collaborative low-carbon distribution. Taxation is a kind of environmental regulation policy of the government, which can improve the environmental protection willingness of enterprise-alliance by levying environmental tax. At the same time, from the implementation level, the government needs to implement certain regulatory measures on the collaborative distribution strategy of enterprise-alliance. Reward for collaborative distribution enterprise-alliance that aim to minimize carbon emissions, and punishment for collaborative distribution enterprise-alliance that aim to minimize costs. The government, through taxation and reward and punishment mechanisms, encourages the alliance to optimize the distribution plan with the goal of carbon emissions, so as to achieve the goal of energy saving and emission reduction. Similarly, the government grants distribution subsidies to end-users with low carbon preferences, and imposes a personal environmental tax on end-users without low carbon preferences in order to optimize emissions reduction. Finally, end-users with low-carbon preferences are willing to pay additional low-carbon distribution subsidies to enterprise-alliance, which provide logistics and distribution services to end-users. Therefore, the mechanism of the three-way game between the government, enterprise-alliance and end-users in the distribution process is shown in the following Fig. 2 .
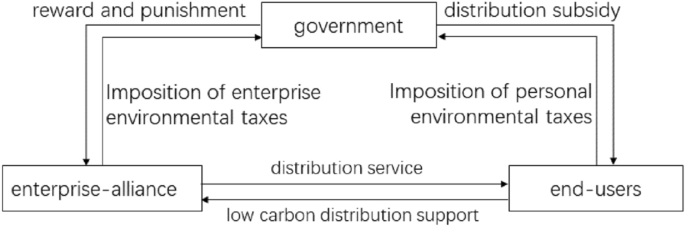
Logical relationship diagram of the evolution of the three-party evolutionary game.
Model assumptions and parameter design
In assumption 1 for the natural environment, subject 1 for the government subgroup, subject 2 for the enterprise-alliance subgroup, and subject 3 for the end-user subgroup, assuming that the participating subjects have finite rationality, the model of the game parties is in the initial stage, the game strategy selection over time gradually evolves and stabilizes in the optimal strategy and the game process does not take into account the impact of other subjects on the game 28 , 29 .
Assumption 2 enterprise-alliance: the set of actions available to the enterprise-alliance is assumed to be{collaborative low-carbon distribution, collaborative low-cost distribution} 30 . Collaborative low-cost distribution refers to an enterprise-alliance that minimizes costs as the goal of distribution route design, and collaborative low-carbon distribution refers to the enterprise-alliance that minimizes carbon emissions as the goal of distribution route design. Let the probability of collaborative low-carbon distribution of enterprise-alliance be \(x\) ,the probability of collaborative low-cost distribution is \(1-x\) where \(0\le x\le 1\) .
Assumption 3 government: the set of actions available to the government is assumed to be{reward and punishment combination policy, flow in the form of policy} 31 . The meaning of the policy of combining reward and punishment is that when the enterprise-alliance chooses low-carbon distribution or low-cost distribution, the government, as an external regulator, gives flexible reward or punishment to regulate the market, and the meaning of flow in the form of policy is that the government uses fixed rather than flexible reward or punishment to regulate the market. Let the probability that the government adopts the policy of combining reward and punishment be as follows \(\text{y}\) ,the probability of adopting the flow in the form of policy is \(1-\text{y}\) where \(0\le \text{y}\le 1\) .
Assumption 4 end-users: the set of actions available to the end-users is assumed to be{with low-carbon preference , without low-carbon preference} . End-users with low-carbon preference are willing to spend more money supporting collaborative low-carbon distribution through enterprise-alliance; End-users without low-carbon preference are more likely to support collaborative low-cost distribution through enterprise-alliance. Let the probability that end-users with low-carbon preference be \(\text{z}\) , the probability of without low-carbon preference is \(1-\text{z}\) where \(0\le \text{z}\le 1\) . End-users who support low-carbon distribution have to pay higher distribution costs. To promote the development of low-carbon distribution, the government provides end-users with a certain amount of low-carbon distribution subsidies.
Assumption 5 in a scenario where end-users demand for goods remains constant but the unit price of distribution increases due to end-users' low-carbon preference, the benefits of collaborative distribution for the enterprise-alliance increase accordingly. When an enterprise-alliance chooses collaborative low-cost distribution or collaborative low-carbon distribution strategies, differences in optimized routes lead to differences in distribution costs. Therefore, different collaborative distribution strategies chosen by enterprises will affect the collaborative distribution costs, carbon emissions, and revenues of the enterprise-alliance. In addition, the distribution process includes the cost of cargo deployment in addition to the cost generated by the distribution itself 30 .
Assumption 6 revenues 32 : revenues from collaborative distribution by the enterprise-alliance \(\left({\varphi }_{1},{\varphi }_{2}\right)\) , low-carbon distribution support \(\beta {\mathcal{V}}\) that the enterprise-alliance receives from end-users; environmental taxes levied by the government on the enterprise-alliance \(\left({\mathcalligra{m}}{\mathcal{E}}_{1},{\mathcalligra{m}}{\mathcal{E}}_{2}\right)\) and personal environmental taxes levied by the government on the end-users \(\left(\gamma L,{D}_{2}\right)\) , and penalties \(A\) imposed by the government on enterprise-alliance for low-carbon distribution; end-users with low-carbon preference can receive the government's distribution subsidy \(\left(\alpha {\mathcal{W}},{D}_{1}\right)\) .
Assumption 7 costs 32 : the cost of collaborative distribution by the enterprise-alliance \(\left({\mathcal{C}}_{x1},{\mathcal{C}}_{x2}\right)\) , the deployment cost of collaborative distribution by the enterprise-alliance \(\uptheta\) ; the regulatory costs invested by the government \(\left({\mathcal{C}}_{y1},{\mathcal{C}}_{y2}\right)\) , the government's bonus to the enterprise-alliance when it distributes low carbon \(S\) , and distribution subsidies for end-users with low-carbon preferences \(\left(\alpha {\mathcal{W}},{D}_{1}\right)\) ; the end-users' costs are mainly the time cost \(\updelta\) needed to support low-carbon distribution in terms of the low-carbon distribution support \(\beta {\mathcal{V}}\) they are willing to pay to the enterprise-alliance.
Based on the above assumptions, the parameters and their significance required to comprehensively set up the three-party evolutionary game model are shown below:
Enterprise-alliance:
\({\mathcal{C}}_{x1}\) : Distribution costs of collaborative low-carbon distribution \({\mathcal{C}}_{x1}>0\)
\({\mathcal{C}}_{x2}\) : Distribution costs for collaborative low-cost distribution \({\mathcal{C}}_{x2}>0\)
\(\uptheta\) : Redeployment costs for collaborative distribution \(\uptheta >0\)
\({\mathcal{E}}_{1}\) : Carbon emissions when collaborating on low-carbon distribution \({\mathcal{E}}_{1}>0\)
\({\mathcal{E}}_{2}\) : Carbon emissions when collaborating on low-cost distribution \({\mathcal{E}}_{2}>0\)
\({\varphi }_{1}\) : Revenues of collaborative low-carbon distribution \({\varphi }_{1}>0\)
\({\varphi }_{2}\) : Revenues of collaborative low-cost distribution \({\varphi }_{2}>0\)
Governments:
\({\mathcalligra{m}}\) : Government's unit penalty factor for carbon emissions from corporate alliances (environmental tax rate 30 ) \(0\le {\mathcalligra{m}}\le 1\)
\(S\) : Government reward for enterprise-alliance when collaborating on low-carbon distribution \(S>0\)
\(A\) : Government punishment when enterprise-alliance collaborate on low-cost distribution \(A>0\)
\({\mathcal{C}}_{y1}\) : Regulatory costs incurred by the government in adopting the policy of reward and punishment combination \({\mathcal{C}}_{y1}>0\)
\({\mathcal{C}}_{y2}:\) Regulatory costs incurred by governments in adopting the policy of flow in the form \({\mathcal{C}}_{y2}>0\)
\(\omega\) : Environmental revenues to governments when enterprise-alliance collaborate on low-carbon distribution \(\omega >0\)
\(\alpha{ \mathcal{W}}\) : Distribution subsidies for end-users with low-carbon preference when the government adopts a policy of reward and punishment. \({\mathcal{W}}\) is the cap of government reward
\(\gamma L\) : Individual environmental taxes on end-users without low-carbon preferences when the government adopts a policy of reward and punishment, 32 \(L\) is the upper limit of the government's tax revenue
\({D}_{1}\) : Distribution subsidies for end-users with low-carbon preference when the government adopt flow in the form policy
\({D}_{2}\) : Individual environmental taxes levied on end-users without low-carbon preferences when governments adopt flow in the form policy.
\(\beta {\mathcal{V}}\) : End-users with low-carbon preference are willing to pay for low-carbon distribution support \({\mathcal{V}}\) is the upper limit of what end-users are willing to pay
\(\updelta\) : Cost of time for end-users to support low-carbon distribution.
Game model construction and analysis
When logistics enterprise alliance choose distribution strategies, their behaviors are constrained by a variety of factors. To analyze the influencing factors of the behavior of each participating subject, evolutionary game theory can be used to construct an evolutionary game model 33 . First, according to the assumptions, the benefits of enterprise-alliance, end-users and government under different behavioral strategies are listed, and then their average expected benefits are calculated, and the replicated dynamic equations of each party are finally derived. The three parties, the enterprise-alliance, end-user and government obtain the benefits according to the benefit payment matrix in Tables 1 and 2 below , where the benefits of each subject is equal to the revenues of each subject minus its corresponding costs (the equations in rows 1–3 of each decision combination in the payment matrix represent the expected benefits of the government, the enterprise-alliance and the end-user under the decisions of the corresponding parties).
Enterprise-alliance, end-users, and government behaviors are interactive, and the three parties utilize continuous evolution to achieve mutual benefits. In the following, the replicated dynamic equations are developed and solved for evolutionary stabilization strategies.
The payment matrices in Tables 1 and 2 show the expected benefits of collaborative low-carbon distribution and collaborative low-cost distribution for enterprise-alliance \({E}_{11}\) and \({E}_{12}\) :
The average expected return of the enterprise-alliance \({E}_{1}\) is:
This leads to the following equation for the replication dynamics of the enterprise-alliance \({F}_{\left(x\right)}\) as 34 :
Similarly, it follows that the expected returns to the government's adoption of the policy of reward and punishment combination and the policy of flow in the form \({E}_{21}\) and \({E}_{22}\) respectively:
The average expected return to the government \({E}_{2}\) is:
This leads to the following equation for the replication dynamics of the government \({F}_{\left(y\right)}\) as:
Similarly, the expected returns of the end-users with and without low-carbon preference are \({E}_{31}\) and \({E}_{32}\) respectively:
The average expected return to the end-users \({E}_{3}\) is:
This leads to the following equation for the dynamics of end-users replication \({F}_{\left(z\right)}\) as:
Game model solving and analysis
Analysis of the stability of single subject strategies, strategic stability analysis of enterprise-alliance.
From the above, the replication dynamic equation of the corporate alliance game strategy is Eq. ( 4 ):
At this point the first order derivative of \(x\) the first-order derivative of \({\mathcalligra{d}}\left({F}_{\left(x\right)}\right)/{\mathcalligra{d}}x=\left(1-2x\right){H}_{\left(\text{y}\right)}\)
When \(\text{y}=\widehat{\text{y}}\) and \({F}_{\left(x\right)}=0\) , regardless of whether \(x\) takes any value, the enterprise-alliance ' strategy tends to stabilize, which indicates that the enterprise-alliance is a stable strategy regardless of what strategy it chooses 34 , as shown in Fig. 3 a.
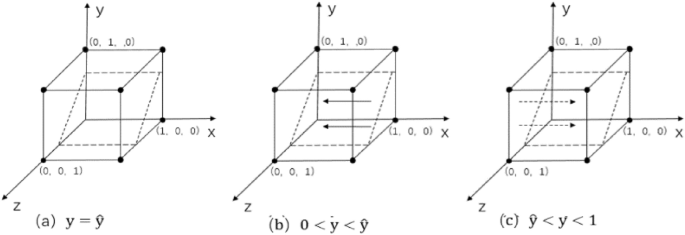
Phase diagram of replication dynamics for enterprise-alliance.
When \(\text{y}\ne \widehat{\text{y}}\) , order \({F}_{\left(x\right)}=0\) , there exist two equilibrium points: \({x}_{1}^{*}=0\) and \({x}_{2}^{*}=1\) .According to the stability theorem of differential equations, realizing the stabilization needs to satisfy both \({F}_{\left(x\right)}=0\) and \({\mathcalligra{d}}\left({F}_{\left(x\right)}\right)/{\mathcalligra{d}}x<0\) . \({\mathcalligra{d}}{H}_{\left(\text{y}\right)}/{\mathcalligra{d}}\text{y}=\text{S}-\text{A}>0\) and \({H}_{\left(\text{y}\right)}\) is an increasing function.
When \(0<\text{y}<\widehat{\text{y}}\) and \({H}_{\left(\text{y}\right)}<0\) , \({\mathcalligra{d}}\left({F}_{\left(x\right)}\right)/{\mathcalligra{d}}x{|}_{{x}_{1}^{*}=0}<0\) , \(\left({F}_{\left(x\right)}\right)/{\mathcalligra{d}}x{|}_{{x}_{1}^{*}=1}>0\) .Therefore \({x}_{1}^{*}=0\) is an evolutionarily stable strategy. The adoption of collaborative low-cost distribution by the enterprise-alliance is the only evolutionary stable strategy globally, as shown in Fig. 3 b. Similarly, when \(\widehat{\text{y}}<\text{y}<1\) , \(\left({F}_{\left(x\right)}\right)/{\mathcalligra{d}}x{|}_{{x}_{2}^{*}=1}<0\) , and \({x}_{2}^{*}=1\) is the stable strategy, the enterprise-alliance adopts collaborative low-carbon distribution as the global unique evolutionary stable strategy as shown in Fig. 3 c.
Strategic stability analysis of government
From the above, the replication dynamic equation for the government game strategy is:
At this point the first order derivative of \(\text{y}\) the first-order derivative of \({\mathcalligra{d}}\left({F}_{\left(\text{y}\right)}\right)/{\mathcalligra{d}}\text{y}=\left(1-2\text{y}\right){G}_{\left(\text{z}\right)}\)
When \(\text{z}=\widehat{\text{z}}\) and \({F}_{\left(\text{y}\right)}=0\) ,regardless of whether \(\text{y}\) takes any value, the government's strategy tends to stabilize, which indicates that the government's strategy is stable regardless of what it chooses, as shown in Fig. 4 a.
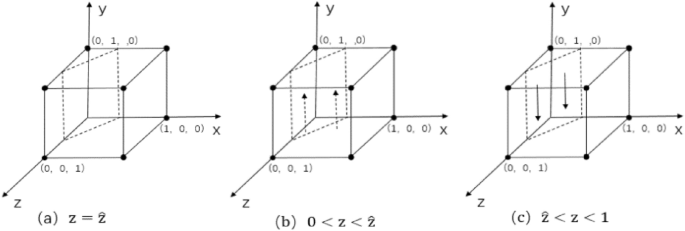
Phase diagram of replication dynamics for government.
When \(\text{z}\ne \widehat{\text{z}}\) , order \({F}_{\left(\text{y}\right)}=0\) , there exist two equilibrium points: \({\text{y}}_{1}^{*}=0\) and \({\text{y}}_{2}^{*}=1\) .According to the stability theorem of differential equations, realizing the strategy stabilization needs to satisfy both \({F}_{\left(\text{y}\right)}=0\) and \({\mathcalligra{d}}\left({F}_{\left(\text{y}\right)}\right)/{\mathcalligra{d}}\text{y}<0\) . \({\mathcalligra{d}}{G}_{\left(\text{z}\right)}/{\mathcalligra{d}}\text{z}={D}_{1}+{D}_{2}-\alpha \mathcal{W}-\gamma L<0\) and \({G}_{\left(\text{z}\right)}\) is a decreasing function.
When \(0<\text{z}<\widehat{\text{z}}\) and \({G}_{\left(\text{z}\right)}>0\) , \({\mathcalligra{d}}\left({F}_{\left(\text{y}\right)}\right)/{\mathcalligra{d}}\text{y}{|}_{{\text{y}}_{1}^{*}=0}>0\) , \(\left({F}_{\left(\text{y}\right)}\right)/{\mathcalligra{d}}\text{y}{|}_{{\text{y}}_{2}^{*}=1}<0\) , therefore \({\text{y}}_{2}^{*}=1\) is an evolutionarily stabilization strategy. The government adopts the flow in the form policy as the only evolutionary stabilization strategy in the whole world, as shown in Fig. 4 b. Similarly, when \(\widehat{\text{z}}<\text{z}<1\) and \(\left({F}_{\left(\text{y}\right)}\right)/{\mathcalligra{d}}\text{y}{|}_{{\text{y}}_{1}^{*}=0}<0\) , \({\text{y}}_{1}^{*}=0\) is an evolutionarily stable strategy. The government adopts the policy of reward and punishment combination as the only evolutionary stable strategy in the whole situation, as shown in Fig. 4 c.
Strategy stability analysis for end-users
From the above, the replication dynamic equation for the end-user game strategy is:
At this point the first order derivative of \(\text{z}\) the first-order derivative of \({\mathcalligra{d}}\left({F}_{\left(\text{z}\right)}\right)/{\mathcalligra{d}}\text{z}=\left(1-2\text{z}\right){R}_{\left(x\right)}\)
When \(x=\widehat{x}\) and \({F}_{\left(\text{z}\right)}=0\) , regardless of whether \(\text{z}\) takes any value, the end-users' strategy tends to stabilize, which indicates that the end-users' strategy is a stable strategy regardless of the strategy chosen, as shown in Fig. 5 a.
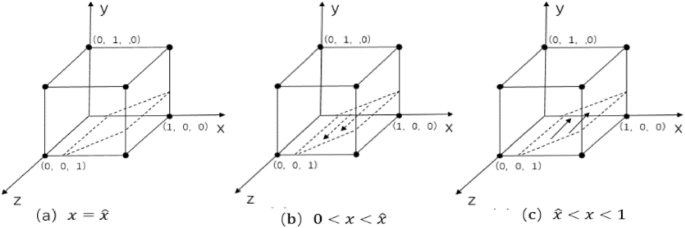
Phase diagram of replication dynamics for end-users'.
When \(x\ne \widehat{x} ,\text{order}{F}_{\left(\text{z}\right)}=0\) , there exist two equilibrium points: \({\text{ z}}_{1}^{*}=0\) and \({\text{z}}_{2}^{*}=1\) . According to the stability theorem of differential equations, realizing strategy stabilization needs to satisfy both \({F}_{\left(\text{z}\right)}=0\) and \({\mathcalligra{d}}\left({F}_{\left(\text{z}\right)}\right)/{\mathcalligra{d}}\text{z}<0\) . Since \({\mathcalligra{d}} {R}_{\left(x\right)}/{\mathcalligra{d}}x=-\left(\beta \mathcal{V}+\delta \right)<0\) , \({R}_{\left(x\right)}\) is a decreasing function.
When \(0<x<\widehat{x}\) and \({R}_{\left(x\right)}>0\) , \({\mathcalligra{d}}\left({F}_{\left(\text{z}\right)}\right)/{\mathcalligra{d}}\text{z}{|}_{{\text{z}}_{1}^{*}=0}>0\) , \(\left({F}_{\left(\text{z}\right)}\right)/{\mathcalligra{d}}\text{z}{|}_{{\text{z}}_{2}^{*}=1}<0\)
Therefore \({\text{z}}_{2}^{*}=1\) is an evolutionarily stable strategy. End-users without low-carbon preference is the only evolutionarily stable strategy globally, as shown in Fig. 5 b. Similarly, when \(\widehat{x}<x<1\) and \(\left({F}_{\left(\text{z}\right)}\right)/{\mathcalligra{d}}\text{z}{|}_{{\text{z}}_{1}^{*}=0}<0\) , the \({\text{z}}_{1}^{*}=0\) is an evolutionarily stable strategy. End-users with low-carbon preference is the global unique evolutionary stable strategy, as shown in Fig. 5 c.
Stability analysis of the equilibrium point of the tripartite evolutionary game system
Three-party game model ess solving.
The previous section analyzed the equilibrium conditions for each game subject to reach the stabilization strategy from the perspective of a single game subject, but in essence, the achievement of the final stable state of the system requires the joint action of the three parties 35 . Therefore, in this section, the asymptotic stability of the equilibrium point is determined by constructing a Jacobi matrix and solving for the eigenvalues, taking into account the existing studies 36 , 37 . According to the replicated dynamic equations \({F}_{\left(x\right) },{F}_{\left(y\right)}\) and \({F}_{\left(\text{z}\right)}\) ,the of \(x\) , \(y\) and \(\text{z}\) derivation yield the Jacobian matrix 38 . In fact, let the replicated dynamic equation of the three parties of interest be 0. Eight pure strategy Nash equilibrium points can be obtained: \(\left(0,0,0\right)\left(0,0,1\right)\left(0,1,0\right)\left(1,0,0\right)\left(1,1,0\right)\left(1,0,1\right)\left(0,1,1\right)\left(1,1,1\right)\) .The Jacobi matrix is obtained from the above replicated dynamic equation as shown in Eq. ( 19 ):
Among them:
Equilibrium point stability analysis
From evolutionary game theory 39 , the stability of the equilibrium point of the system can be judged by the eigenvalues of the Jacobi matrix proposed by Friedman 40 . According to Lyapunov’s 41 , 42 first method, when the eigenvalues are negative, the equilibrium point is stable; when the eigenvalues are positive, the equilibrium point is unstable. The above eight equilibrium points are substituted into the Jacobi matrix to obtain the corresponding eigenvalues, as shown in the following table:
The local equilibrium points in the table are stable points if the three corresponding eigenvalues are not greater than zero. By further solving the eigenvalues in Table 3 , we find that there are six stable points in the evolutionary game, and the specific scenarios are as follows:
Scenario 1 \(\left(1,1,1\right)\) : When \(\left(S-A\right)+\left({\mathcal{C}}_{x2}-{\mathcal{C}}_{x1}\right)+{\mathcalligra{m}}\left({\mathcal{E}}_{2}-{\mathcal{E}}_{1}\right)+\beta \mathcal{V}>{\varphi }_{2}-{\varphi }_{1}\) , \({\mathcal{C}}_{y1}-{\mathcal{C}}_{y2}<{D}_{1}-\alpha \mathcal{W}-S\) and \(\beta \mathcal{V}+\delta <\alpha \mathcal{W}+\gamma L\) ,the stable strategy of the evolutionary game is (collaborative low-carbon distribution, reward and punishment combination, with low-carbon preference). In this scenario, the benefits of the enterprise-alliance when it engages in low-carbon distribution are supplemented by the government and end-users, and the benefits of collaborative low-carbon distribution are much greater than the benefits of collaborative low-cost distribution, so the enterprise-alliance group will choose to engage in collaborative low-carbon distribution. The net benefit 43 to the government of adopting the policy of reward and punishment combination is greater than the net benefit of adopting flow in the form of policy, so the government group will choose to adopt policy of reward and punishment combination to guide the enterprise-alliance and the end-users, and the government can obtain the greatest environmental benefits. The benefits to end-users with low-carbon preference outweigh the costs, so the end-users will gradually support low-carbon distribution through enterprise-alliance.
Scenario 2 \(\left(0,1,1\right)\) : When \(\left(S-A\right)+\left({\mathcal{C}}_{x2}-{\mathcal{C}}_{x1}\right)+{\mathcalligra{m}}\left({\mathcal{E}}_{2}-{\mathcal{E}}_{1}\right)+\beta \mathcal{V}<{\varphi }_{2}-{\varphi }_{1}\) , \({\mathcal{C}}_{y1}-{\mathcal{C}}_{y2}<A-{D}_{1}-\alpha \mathcal{W}\) , the evolutionary game stabilizes the strategy as (collaborative low-cost distribution, reward-punishment combination policy, with low-carbon preference). In this scenario, the enterprise-alliance group will prefer collaborative low-cost distribution because the revenue subsidies to the enterprise-alliance from the government and end-users are smaller than the revenue difference between the enterprise-alliance performing low-cost distribution and low-carbon distribution. The government will prefer reward and punishment combination policy because the government receives a penalty for low-carbon distribution, which is greater than the difference between the government's costs of adopting the two policies.
Scenario 3 \(\left(1,0,1\right)\) : When \(\left({\mathcal{C}}_{x2}-{\mathcal{C}}_{x1}\right)+{\mathcalligra{m}}\left({\mathcal{E}}_{2}-{\mathcal{E}}_{1}\right)+\beta \mathcal{V}>{\varphi }_{2}-{\varphi }_{1}\) , \(S+{D}_{1}-\alpha \mathcal{W}<{\mathcal{C}}_{y1}-{\mathcal{C}}_{y2}\) and \(\beta \mathcal{V}+\delta <{D}_{1}+{D}_{2}\) ,the stable strategy of the evolutionary game is (collaborative low-carbon distribution, flow in the form of policy, with low-carbon preference). In this scenario, although the enterprise-alliance does not have government incentives, the end-users with low-carbon preference is willing to pay a certain distribution cost. The income of the enterprise-alliance's collaborative low-carbon distribution is greater than income of the collaborative low-cost distribution, so the enterprise-alliance group still chooses the collaborative low-carbon distribution. When the government adopts flow in the form of policy, the incentives for end-users are greater than the costs that end-users with low-carbon preference need to pay, so the end-users group will gradually have low-carbon preference and support the enterprise-alliance in collaborating on low-carbon distribution.
Scenario 4 \(\left(1,1,0\right)\) : When \(\left(\text{S}-\text{A}\right)+\left({\mathcal{C}}_{x2}-{\mathcal{C}}_{x1}\right)+{\mathcalligra{m}}\left({\mathcal{E}}_{2}-{\mathcal{E}}_{1}\right)<{\varphi }_{2}-{\varphi }_{1}\) , \(S+\gamma L-{D}_{2}>{\mathcal{C}}_{y1}-{\mathcal{C}}_{y2}\) , \(\text{and }\alpha \mathcal{W}+\gamma L<\beta \mathcal{V}+\delta\) , the stable strategy of the evolutionary game is (collaborative low-carbon distribution, reward and punishment combination policy, without low-carbon preference). In this scenario, the reward received by the end-users are less than the costs they need to bear to support low-carbon distribution, so the end-users group's low-carbon preference will gradually decrease until it disappears. Although the enterprise-alliance does not support of end-users, the benefit of collaborative low-carbon distribution is still greater than benefit of collaborative low-cost distribution under the government subsidy, so the enterprise-alliance group will choose collaborative low-carbon distribution. Similarly, the benefit to the government of adopting reward and punishment combination policy is greater than benefit of adopting flow in the form of policy, so the government group will choose to adopt policy of reward and punishment combination.
Scenario 5 \(\left(1,0,0\right)\) : When \(\left({\mathcal{C}}_{x2}-{\mathcal{C}}_{x1}\right)+{\mathcalligra{m}}\left({\mathcal{E}}_{2}-{\mathcal{E}}_{1}\right)>{\varphi }_{2}-{\varphi }_{1}\) , \(\gamma L-S-{D}_{2}<{\mathcal{C}}_{y1}-{\mathcal{C}}_{y2}\) , \(\text{and }{D}_{1}+{D}_{2}<\beta \mathcal{V}+\delta\) , the evolutionary game stabilizes the strategy (collaborative low-carbon distribution, flow in the form of policy, without low- carbon preference). In this scenario, the government subsidy to end-users is less than their cost of supporting low-carbon distribution, so the end-users group's low-carbon preference fades. The benefit to the enterprise-alliance of undertaking collaborative low-carbon distribution is greater than benefit of undertaking collaborative low-cost distribution, so the enterprise-alliance group will undertake collaborative low-carbon distribution.
Scenario 6 \(\left(0,0,1\right)\) : When \(\left({\mathcal{C}}_{x2}-{\mathcal{C}}_{x1}\right)+{\mathcalligra{m}}\left({\mathcal{E}}_{2}-{\mathcal{E}}_{1}\right)+\beta \mathcal{V}<{\varphi }_{2}-{\varphi }_{1}\) , \(A+{D}_{1}-\alpha \mathcal{W}<{\mathcal{C}}_{y1}-{\mathcal{C}}_{y2}\) ,the stable strategy of the evolutionary game is (collaborative low-cost distribution, flow in the form of policy, with low-carbon preference). In this scenario, although the end-users have low-carbon preference, the cost they are willing to pay is lower, and the enterprise-alliance adopts the flow in the form of policy, therefore the enterprise-alliance group will choose the collaborative low-cost distribution based on the principle of profit maximization. The benefits to the government of adopting reward and punishment combination policy are lower than those of adopting flow in the form of policy, so the government group will also choose to adopt flow in the form of policy.
Data simulation
To verify the validity of the evolutionary stability analysis, more intuitively present the evolutionary stability process and explore the influence of relevant factors on the strategic behavior of each subject, this paper uses MATLAB R2021 (Full name of software and Version number: Matlab R2021a Win64 Crack. URL link: https://matlab.waltzsy.com/ ) software to carry out sensitivity analysis and numerical simulation on the equilibrium strategies of each subject 44 . At the same time, the parameters involved in the replicated dynamic equation system assumed to meet the corresponding conditions of different evolutionary stable states, to make them as close as possible to the actual case situation and to representatively present the model's evolution of the game laws and characteristics 45 , 46 .
First, assume that array 1 satisfies Case 1: \({\mathcal{C}}_{x1}\) = 50, \({\mathcal{C}}_{x2}\) = 40, \({\mathcal{E}}_{1}=50\) , \({\mathcal{E}}_{2}=35\) , \({\varphi }_{1}=300\) , \({\varphi }_{2}=250\) , \({\mathcalligra{m}}=0.5\) , \(S=5\) , \(A=1\) , \({\mathcal{C}}_{y1}=15\) , \({\mathcal{C}}_{y2}=20\) , \(\alpha =0.1\) , \(\mathcal{W}=10\) , \(\gamma =0.7\) , \(L=10\) , \({D}_{1}=4\) , \({D}_{2}=1\) , \(\beta =0.5\) , \(\mathcal{V}=6\) , \(\delta =1\) . The array 1 \(,x\) , \(y\) and \(\text{z}\) evolutionary simulation routes under different initial value states are explored to verify the validity of the parameters. The initial values of \(x\) , \(y\) and \(\text{z}\) are set to 0.2, 0.5, and 0.7, respectively, and the results are shown in Figs. 6 , 7 and 8 : according to the model results, the initial values of \(x\) , \(y\) , \(\text{z}\) converge to 1 in the end, which is consistent with the evolutionary equilibrium point of case 1 \(\left(1,1,1\right)\) , therefore the model and parameters are valid.
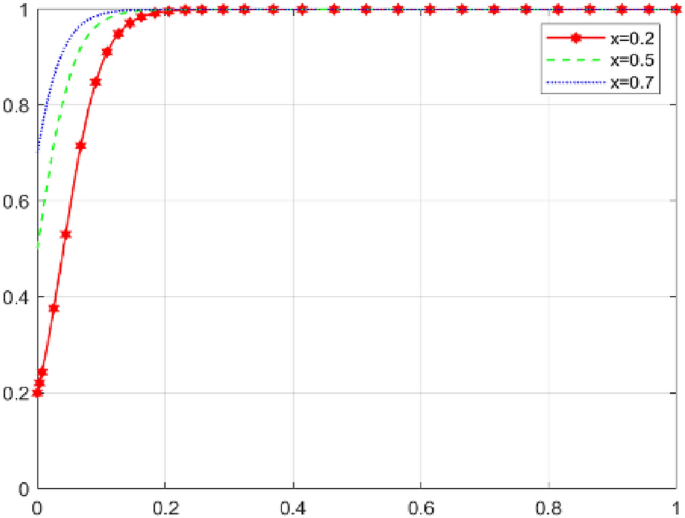
Evolutionary game diagram with different times for x.
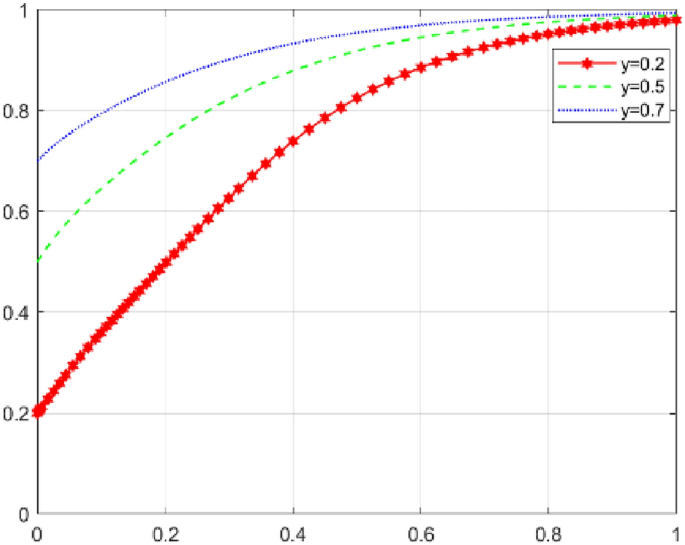
Evolutionary game diagram with different times for y.
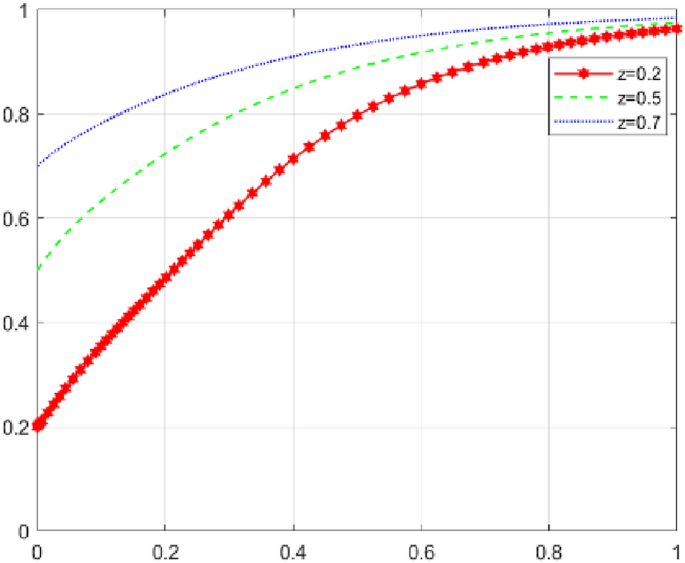
Evolutionary game diagram with different times for z.
Analysis of the impact of different strategy choices of multiple subjects on the outcome of the evolutionary game
The data simulation of the six evolutionary stabilization scenarios proposed in “ Game model solving and analysis ” is shown in Figs. 9 , 10 , 11 , 12 , 13 and 14 : from the evolutionary results, it can be seen that array 1 satisfies the conditions of scenario 1, and at this time, there exists an evolutionary equilibrium point of the system \(\left(1,1,1\right)\) , the combination of the strategies of the enterprise-alliance, the government, and the end-user is (collaborative low-carbon distribution, reward and punishment combination policy, with low-carbon preference). By changing the value of \({\varphi }_{1}\) the value of the array 2 is adjusted to obtain array 2 satisfies the following case 2 conditions: \({\mathcal{C}}_{x1}\) =50 , \({\mathcal{C}}_{x2}\) =40, \({\mathcal{E}}_{1}=50\) , \({\mathcal{E}}_{2}=35\) , \({\varphi }_{1}=255\) , \({\varphi }_{2}=250\) , \({\mathcalligra{m}}=0.5\) , \(S=5\) , \(A=1\) , \({\mathcal{C}}_{y1}=15\) , \({\mathcal{C}}_{y2}=20\) , \(\alpha =0.1\) , \(\mathcal{W}=10\) , \(\gamma =0.7\) , \(L=10\) , \({D}_{1}=4\) , \({D}_{2}=1\) , \(\beta =0.5\) , \({\mathcal{V}}=6\) , \(\delta =1\) . According to the simulation results Fig. 9 , the evolutionary equilibrium point of the system at this time becomes \(\left(0,1,1\right)\) , and the strategy combinations of enterprise-alliance, government and end-users are (collaborative low-cost distribution, reward and punishment combination policy, with low-carbon preference). Therefore, when the revenue difference between collaborative low-cost distribution and collaborative low-carbon distribution of enterprise-alliance is relatively small, it will tend to choose collaborative low-carbon distribution, on the contrary, if the revenue difference is large, enterprise-alliance will tend to choose collaborative low-cost distribution. This result suggests that enterprise-alliance are not only seeking to maximize economic benefits, but are also willing to take part of the social responsibility of reducing carbon emissions. However, when the difference between the two revenues is large, the enterprise-alliance will still choose collaborative low-cost distribution by focusing on economic benefits. The simulation analysis is consistent with the conclusions of the stability analysis of each party's strategy and has the validity, which is of practical significance in guiding the choice of distribution strategy for enterprise alliances (other cases will not be verified for reasons of space).
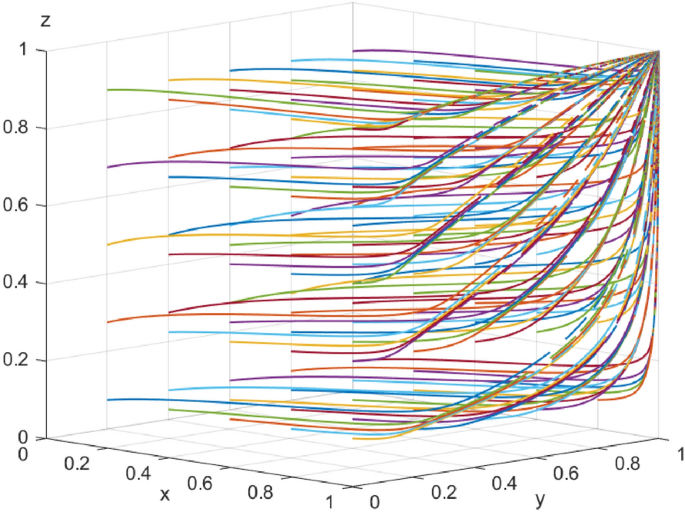
Simulation results of scenario 1 evolution.
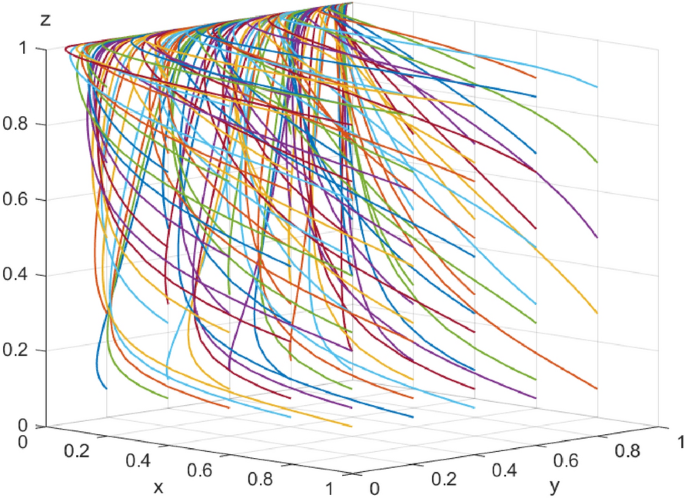
Simulation results of scenario 2 evolution.
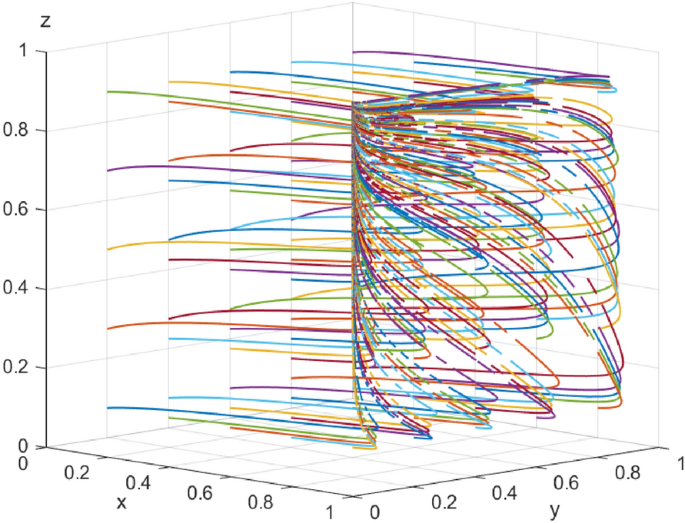
Simulation results of scenario 3 evolution.
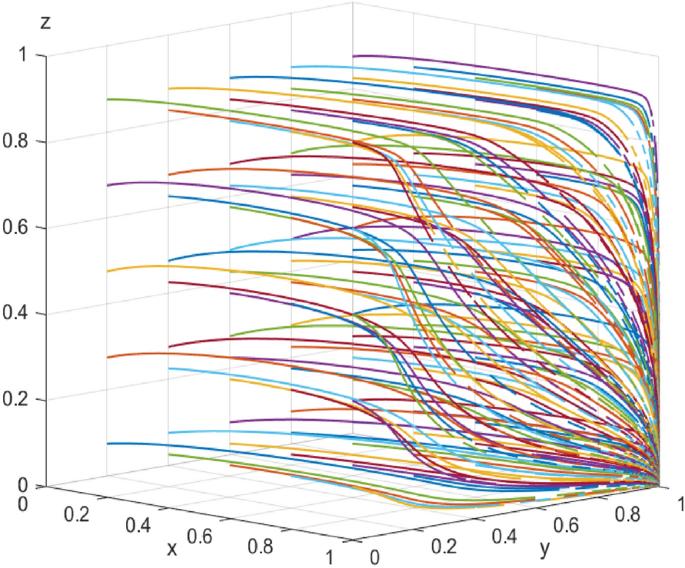
Simulation results of scenario 4 evolution.
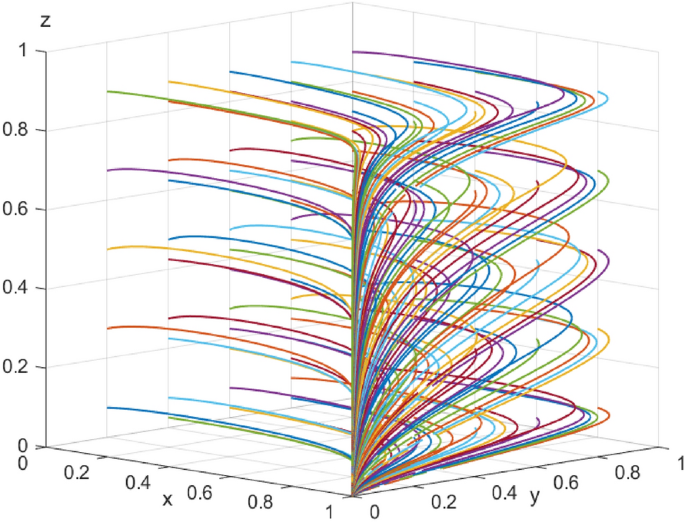
Simulation results of scenario 5 evolution.
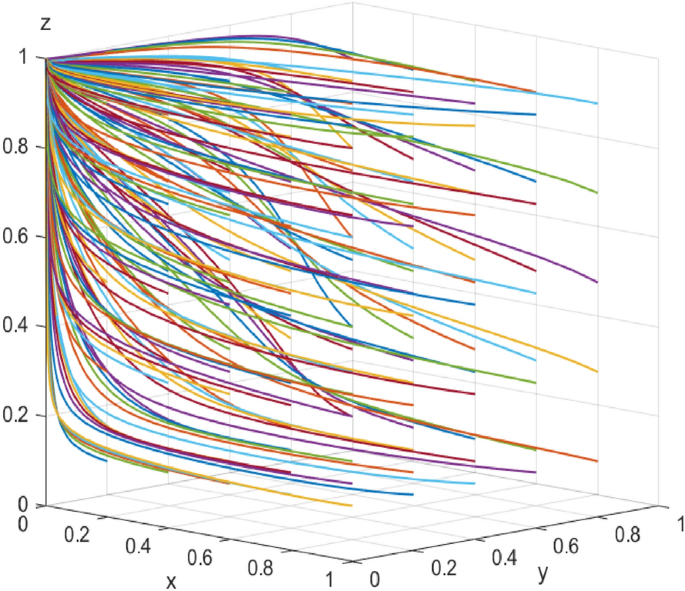
Simulation results of scenario 6 evolution.
The impact of supply-side government policies on enterprise-alliance distribution strategies
Since the combination of strategies in Case 1 (collaborative low-carbon distribution, reward and punishment combination policy, with low-carbon preference) is a desirable state of social operation, based on Case 1, the impact of government reward on enterprise-alliance distribution strategies is further discussed. When \(S=(1,5,10),\) the results of 100 simulations of the replicable dynamic equation system evolving over time are shown in Fig. 15 : in the process of the system evolving to a stable point, an increase in the government reward can accelerate the evolution of the enterprise-alliance to carry out collaborative low-carbon distribution. Enterprise-alliance will engage in collaborative low-carbon distribution in order to maintain their reputation with the government and end-users because of the rewards they receive from the government. The stronger the incentives from the government, the more stable the strategy of the enterprise-alliance in choosing collaborative low-carbon distribution. But with an increase in the government's financial burden, the government's financial burden increases, so the evolution of the government's policy of combining reward and punishment slows down when \(S=10\) the financial burden is too heavy, and the government will turn to flow in the form of policy by reducing the cost of regulation or reducing incentives.
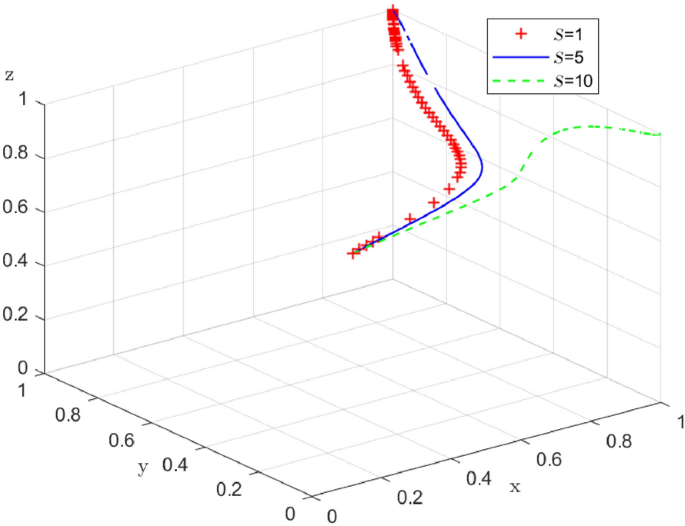
Impact of government reward on system evolution.
Similarly, when enterprise-alliance collaborate on low-cost distribution, and government punishment \(A=(1,10,20)\) , the simulation results of replicating the system of dynamic equations evolving over time 100 times are shown in Fig. 16 . During the evolution of the system to the stable point, an increase of government punishment can accelerate the evolution of the enterprise-alliance to carry out collaborative low-carbon distribution, and the enterprise-alliance will continue choosing collaborative low-carbon distribution in this case as long as the government's punishment for collaborative low-cost distribution is \(A>0\) .When the government punishment collaborative low-cost distribution, the enterprise-alliance will always choose collaborative low-carbon distribution in this case. This suggests that government punishment have a greater impact on enterprise-alliance's strategy choices than reward, because government punishment affect enterprise-alliance 's reputation in the industry as well as its credit rating.
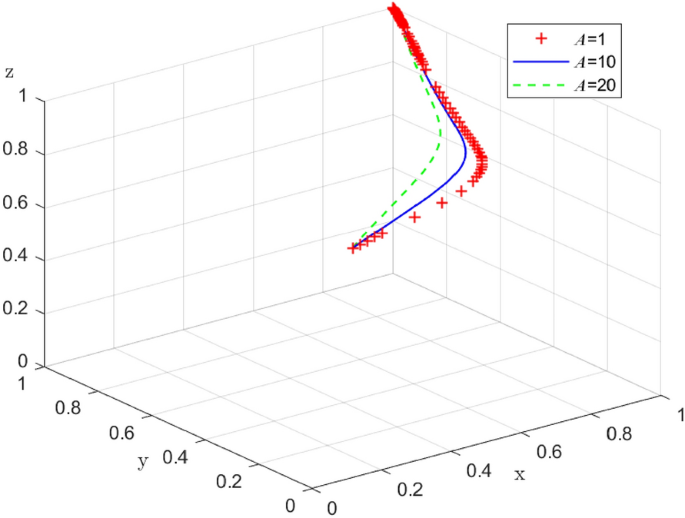
Impact of government punishment on system evolution.
The impact of demand-side end-users’ behavior on the distribution strategies of enterprise-alliance
Additionally, based on Case 1, we analyze the impact of end-users behavior on the distribution strategy of the enterprise-alliance, and first explore the impact of end-users with low-carbon preference who are willing to pay the low-carbon distribution subsidy coefficient \(\beta\) .The influence of the value of the subsidy coefficient of low-carbon preference is investigated \(\beta =0.5\) .On the basis of \(\beta =0.1\) and \(\beta =1.2\) , the simulation results of 100 repetitions of the dynamic equation system evolving over time are shown in Fig. 17 . According to the evolution results, when \(\beta =0.1\text{and}0.5\) , the end-users will tend to choose the low-carbon preference; when the subsidy coefficient \(\beta\) is lower, the end-users with low-carbon preference consider it acceptable to spend extra distribution costs. However, when \(\beta =1.2\) , end-users no longer support the enterprise alliance in low-carbon distribution from the perspective of maximizing their own interests because the low-carbon distribution subsidy they have to pay is too high. It follows that the behavior of end-users is influenced by the costs they need to bear, and above a certain threshold they will no longer support enterprise-alliance for collaborative low-carbon distribution.
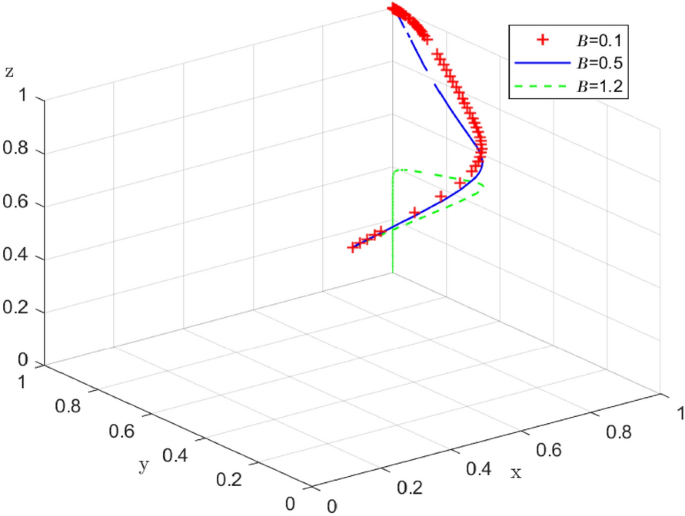
Impact of end-users’ low-carbon preference on system evolution.
Similarly, the simulation results of replicating the dynamic system of equations with time evolution 100 times when the time cost δ = (1, 3,6) that the end-users need to pay to support low-carbon distribution are shown in Fig. 18 . From the evolution results, it can be seen that, when δ = 1 and 3, the end-users will tend to choose to support the enterprise-alliance in carrying out low-carbon distribution; But when δ = 6, the end-users will not support the enterprise-alliance in carrying out low-carbon distribution due to the high time cost that they need to pay. The current end-user requirements for the speed of logistics and distribution are increasingly high, if the enterprise-alliance in the collaboration of low-carbon distribution at the same time, logistics efficiency has not been improved, it will lead to the end-user's low-carbon preference is reduced until there is none.
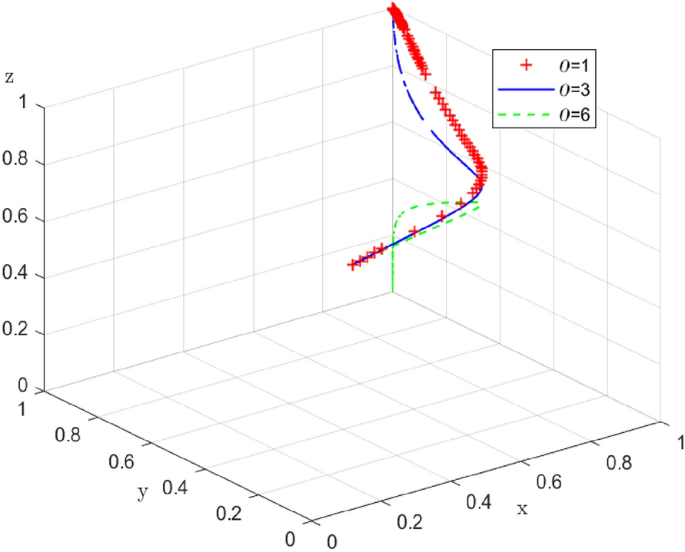
Impact of time cost on system evolution
Conclusions and implications
This study aims to explore the dual effects of government reward and punishment mechanisms and end-users low-carbon preference on the choice of distribution strategies of enterprise-alliance. Through the government-led digitalization platform, the accurate accounting of cost data and carbon data of each distribution enterprise is realized, and the carbon trading market is effectively docked. Loss-of-benefit and carbon emission quantification models of collaborative low-carbon distribution modes and collaborative low-cost distribution modes are established, a dual incentive strategy considering government reward and punishment and end-user subsidies is proposed, and the effectiveness and feasibility of the models and strategies are verified through numerical experiments The main conclusions of this paper are as follows.
From the viewpoint of supply-side policy, the government should macroscopically control the reward and punishment mechanism according to the actual situation of the market 47 . Blindly increasing the reward will impose a heavy financial burden to the government, and with the development of the carbon trading market and increase in the carbon price, the government will gradually withdraw from the reward mechanism and play a major role in punishment. Compared with reward, strict punishment have a more obvious effect on the regulation of enterprise-alliance, so the government should consider local actuality, adopt a policy combination of dynamic reward and static punishment, further improve the construction of the carbon market, guide enterprises to actively participate in carbon trading, and give full play to the role of the carbon market.
Regarding demand-side end-users’ behavior, the end-user's support for the low-carbon distribution of the enterprise-alliance has a threshold, and after exceeding the threshold, the end-users will no longer support the enterprise-alliance in carrying out low-carbon distribution from the perspective of maximizing self-interest, so the enterprise-alliance should reasonably formulate the cost of low-carbon distribution, and guide the end-users to participate in the construction of the carbon market 48 .
The research presented in this paper further improves standard emissions and designs reasonable reward and punishment mechanisms 49 . However, the choice of enterprise-alliance strategies in real situations is often influenced by other constraints, while the evolutionary game model in this study is based on assumptions and the choice of parameters is idealized. Future research directions should combine the actual situation of distribution development and comprehensively consider the multiple factors involved in enterprise-alliance distribution.
Data availability
The datasets used and analysed during the current study available from the corresponding author on reasonable request.
Liu, X. Supply Chain Carbon Emission Reduction Decision-Making with Consumer Low-Carbon Preference and Financing Dual Constraints (Hunan University, 2018).
Google Scholar
Meisheng, K. & Liangtao, Y. Research on the impact of logistics enterprise alliance network and resource integration on service performance. R. Enterprise Econ. 19 , 104–107 (2023).
Zhang, X., Chen, C. & Zhang, X. How 3PL and 4PL build agile logistics service alliance. Sci. Technol. Manag. 12 , 105–107 (2003).
Weizhen, R. et al. Research on effective methods of collaborative distribution cost sharing relying on platforms. J. Manag. Sci. 24 (09), 105–126. https://doi.org/10.19920/j.cnki.jmsc.2021.09.006 (2021).
Yuan, Y. et al. Research on the interaction influence mechanism of urban traffic congestion and air pollution—Based on the big data analysis of drip travel. J. Manag. Sci. 23 (02), 54–73 (2020).
Weizhen, R. et al. Research on dual low-carbon incentive strategies for collaborative distribution relying on platforms in the context of carbon trading[J/OL]. China Manag. Sci 3 , 1–18 (2024).
Weizhen, R., Xinru, P. & Qinghua, Z. Research on optimal price discount incentive strategy for customer participation in logistics collaborative distribution[J/OL]. Syst. Eng. Theory Pract. 5 , 1–20 (2024).
Zhou, L. et al. Optimization of cooperative distribution based on crowdsourcing and piggybacking. Oper. Res. Manag. 32 (07), 78–84 (2023).
CAS Google Scholar
Qie, B., Weng, X., Sun, Z. et al . Location-routing and cost-sharing models under joint distribution. Cluster Comput . (2024).
Chu, X. et al. Enabling joint distribution with blockchain technology in last-mile logistics. Comput. Indus. Eng. 1 , 187 (2024).
Weizhen, R., Luxia, Y. & Liu, Lu. Research on platform-based online large-scale collaborative distribution alliance splitting strategy. Syst. Eng. Theory Pract. 43 (05), 1425–1445 (2023).
Benli, D., Çimen, M. & Soysal, M. Sustainable vehicle allocation decisions under a vertical logistics collaboration setting. J. Clean. Prod. 453 , 14226 (2024).
Rao, W.-Z., Miao, X. & Liu, L. Research on collaborative distribution model and cost sharing impact considering enterprise distribution capacity constraint[J/OL]. China Manag. Sci. 3 , 1–13 (2024).
Han, R. & Yang, M. Profit distribution and stability analysis of joint distribution alliance based on tripartite evolutionary game theory under the background of green and low carbon. Environ. Sci. Pollut. Res. Int. 29 (39), 59633–59652. https://doi.org/10.1007/s11356-022-19712-y (2022).
PubMed Google Scholar
Chen, Y., Gan, H. & Cheng, L. A joint distribution cold chain logistics model and its solution algorithm in the context of “double carbon”. Control Decis. Mak. 38 (07), 1951–1959 (2023).
Weizhen, R., Feng, X. & Zhongfei, D. Default recovery and loss compensation mechanism for members’ withdrawal from alliance in collaborative distribution. Syst. Eng. Theory Pract. 41 (12), 3260–3281 (2021).
Li, H. et al. Simulation analysis of the three-party evolutionary game of green building market players under carbon trading policy. Environ. Sci. Pollut. Res. 30 , 117591–117608 (2023).
Zhenxiang, Z., Jingzhao, S. & Chunyu, L. A study on low-carbon strategy selection and game evolution of manufacturing enterprises under cloud manufacturing model. J. Syst. Sci. 30 (03), 102–107+113 (2022).
Lin, S. et al. Evolutionary game theory and simulations based on doctor and patient medical malpractice under government regulation. Sci. Rep. https://doi.org/10.1038/s41598-023-44915-9 (2023).
PubMed PubMed Central Google Scholar
Wu, X. & Zhao, S. Research on green development strategy of logistics packaging based on corporate social responsibility: An evolutionary game analysis. Environ. Dev. Sustain. https://doi.org/10.1007/s10668-023-04362-y (2024).
Tang, X., Feng, J., Feng, B. et al. Policy analysis on the promotion of new energy vehicles in China considering consumers’ car purchasing choices in the “post-subsidy era”: Based on the study of a three-party evolutionary game. Environ. Dev. Sustain. ( 2024 ).
Ting, G. & Feng, W. Three-way evolutionary game analysis of user skip order behavior in cloud manufacturing platform[J/OL]. Indus. Eng. Manag. 3 , 1–16 (2024).
Yuan, Y. et al. Allocation strategy of medical supplies during a public health emergency: A tripartite evolutionary game perspective. Sci. Rep. 13 , 9571 (2023).
ADS CAS PubMed PubMed Central Google Scholar
Wang, J., Yang, B. & Wei, L. Research on the evolutionary game of value co-creation in cloud manufacturing innovation ecosystem under low-carbon background[J/OL]. Comput. Integr. Manuf. Syst. 12 , 1–18 (2023).
Qiu, Z. et al. Research on credit regulation mechanism of e-commerce platform based on evolutionary game theory. Syst. Sci. Syst. Eng. 33 , 1 (2024).
Chen, Z. & Wang, W. Analysis of evolutionary game between government and polluting enterprises under environmental tax system. Manag. Rev. 29 (05), 226–236. https://doi.org/10.14120/j.cnki.cn11-5057/f.2017.05.021 (2017).
Weizhen, R. et al. Research on collaborative distribution problem and cost sharing method considering service quality difference of enterprises. Syst. Eng. Theory Pract. 42 (10), 2721–2739 (2022).
Chen, J. et al. Research on credit evolution of shared logistics market players based on three-party game[J/OL]. Complex Syst. Complex. Sci. 5 , 1–12 (2024).
Chen, W., Tang, Y. & Zhang, T. Stochastic evolutionary analysis of strategy behavior of related subjects under environmental credit regulation[J/OL]. Complex Syst. Complex. Sci 5 , 1–10 (2024).
Rao, W.-Z., Duan, Z.-F. & Zhang, Z.-W. A study on the evolutionary stability of government regulation and firms’ collaborative distribution strategies under environmental regulation. Comput. Integr. Manuf. Syst. 30 (04), 1537–1550 (2024).
Wang, H. et al. A study on automobile manufacturers’ production decisions considering consumers’ low-carbon preferences under government reward and punishment mechanisms. Syst. Eng. Theory Pract. 43 (09), 2669–2684 (2023).
Chu, T. et al. Study on the carbon neutral pathway of home appliance industry considering consumers’ carbon responsibility. Syst. Eng. Theory Pract. 44 (03), 1018–1037 (2024).
Yi, W., Xiuhui, L. & Xinggang, L. A tripartite evolutionary game analysis of new retail platform, riders and consumers considering delivery traffic risk. J. Syst. Manag. 33 (01), 46–58 (2024).
Ji, Y., Xie, Y. & Chai, J. A three-party evolutionary game and simulation study to promote the development of rural revitalization industry[J/OL]. Syst. Sci. Math. 2024 , 1–21 (2024).
Li, X. et al. A tripartite evolutionary game of participating in the enhancement of regional public brands of agricultural products. China Manag. Sci. 30 (08), 196–209 (2022).
Yang, N. et al. A study on the dynamic evolution of corporate technological innovation behavior in complex product R&D networks. China Manag. Sci. 32 (02), 242–253. https://doi.org/10.16381/j.cnki.issn1003-207x.2021.1296 (2024).
Zhou, X. et al. How government subsidies and cost sharing affect platform and firm strategy choices-based on a three-way evolutionary game. Control Decis. Mak. 37 (02), 293–302. https://doi.org/10.13195/j.kzyjc.2021.0853 (2022).
Tanimoto, J. Fundamentals of evolutionary game and applications. SpringJapan 2015 , 7–14 (2015).
Kalman, R. E. & Bertram, J. F. Control system analysis and design via the second method of Lyapunov. J. Basic Eng. 82 (2), 394–400 (1960).
Friedman, D. Evolutionary games I2 economics. Econometrica 59 (3), 637–666 (1991).
MathSciNet Google Scholar
Khalil, H. K. & Grizzle, J. W. Nonlinear Systems (Prentice Hall, 2002).
Wang, G. et al. A comprehensive review of research works based on evolutionary game theory for sustainable energy development. Energy Rep. 8 , 114–136 (2022).
Han, P., Ye, D. & Gu, L. A game study on the evolution of community garbage sorting behavior under reward and punishment mechanism[J/OL]. Complex Syst. Complex. Sci. 5 , 1–9 (2024).
Huang, J. et al. Evolutionary game analysis of food safety regulation based on blockchain technology. Syst. Sci. Math. 43 (11), 2885–2900 (2023).
Zhang, J. et al. Research on the three-party evolutionary game of standard essential patent licensing in the era of digital economy. Manag. Rev. 35 (02), 268–279. https://doi.org/10.14120/j.cnki.cn11-5057/f.2023.02.002 (2023).
Zhou, X. et al. Evolutionary strategy of tripartite collaboration among industrial internet platforms, developers and enterprises—Analyzing the role of government subsidies and revenue sharing. China Manag. Sci. 32 (01), 276–287. https://doi.org/10.16381/j.cnki.issn1003-207x.2021.2558 (2024).
Wang, W. et al. Impact of government reward and punishment mechanism on WEEE recycling under the tripartite evolutionary game[J/OL]. China Manag. Sci. 5 , 1–13 (2024).
Haoyan, F. & Li, S. Differential game strategy for energy sharing in industrial clusters under low carbon preference. Comput. Integr. Manuf. Syst. 29 (09), 3111–3122 (2023).
Zhou, K., Wang, Q. & Tang, J. Evolutionary game analysis of environmental pollution control under the government regulation. Sci. Rep. 12 (1), 474. https://doi.org/10.1038/s41598-021-04458-3 (2022).
Download references
Author information
Authors and affiliations.
School of Logistics and Management Engineering, Yunnan University of Finance and Economics, Kunming, 650221, China
Wenxue Ran, Dandan He, Zhaoxia Li, Yun Xue & Aravinda Dananjaya Basnayaka Basnayaka Gunarathnage
School of Accounting, Lanzhou University of Finance and Economics, Lanzhou, 730000, China
Zhenzhen He
You can also search for this author in PubMed Google Scholar
Contributions
Wenxue Ran: Conceptualization, Funding acquisition, Project administration, Dandan He: Conceptualization, Methodology, Software, Writing-original draft, Zhaoxia Li: Data curation, Writing-original draft, Yun Xue: Data curation, Writing-original draft, Zhenzhen He: Methodology, Software, Writing-original draft, Aravinda Dananjaya Basnayaka Basnayaka Gunarathnage: Writing-original draft, Resources.
Corresponding author
Correspondence to Wenxue Ran .
Ethics declarations
Competing interests.
The authors declare no competing interests.
Additional information
Publisher's note.
Springer Nature remains neutral with regard to jurisdictional claims in published maps and institutional affiliations.
Rights and permissions
Open Access This article is licensed under a Creative Commons Attribution 4.0 International License, which permits use, sharing, adaptation, distribution and reproduction in any medium or format, as long as you give appropriate credit to the original author(s) and the source, provide a link to the Creative Commons licence, and indicate if changes were made. The images or other third party material in this article are included in the article's Creative Commons licence, unless indicated otherwise in a credit line to the material. If material is not included in the article's Creative Commons licence and your intended use is not permitted by statutory regulation or exceeds the permitted use, you will need to obtain permission directly from the copyright holder. To view a copy of this licence, visit http://creativecommons.org/licenses/by/4.0/ .
Reprints and permissions
About this article
Cite this article.
Ran, W., He, D., Li, Z. et al. Research on distribution strategy of logistics enterprise alliance based on three-party evolution game. Sci Rep 14 , 14894 (2024). https://doi.org/10.1038/s41598-024-65723-9
Download citation
Received : 14 April 2024
Accepted : 24 June 2024
Published : 28 June 2024
DOI : https://doi.org/10.1038/s41598-024-65723-9
Share this article
Anyone you share the following link with will be able to read this content:
Sorry, a shareable link is not currently available for this article.
Provided by the Springer Nature SharedIt content-sharing initiative
- Reward and punishment mechanism
- Low-carbon preference
- Collaborative distribution
- Evolutionary game
By submitting a comment you agree to abide by our Terms and Community Guidelines . If you find something abusive or that does not comply with our terms or guidelines please flag it as inappropriate.
Quick links
- Explore articles by subject
- Guide to authors
- Editorial policies
Sign up for the Nature Briefing newsletter — what matters in science, free to your inbox daily.


IMAGES
VIDEO
COMMENTS
The second step in problem-solving and decision-making, covered pre viously, was to understand. and define tangential issues and consider and assess alternative solutions which could handle each ...
How to solve problems as a manager. Consider these steps to help you solve problems as a manager in your workplace: 1. Define the problem. You must first identify what the problem is by talking to colleagues, conducting research and using your observational skills. Once you understand the challenge you want to overcome, try to define it as ...
A managerial problem can be described as the gap between a given current state of affairs and a future desired state. Problem solving may then be thought of as the process of analyzing the situation and developing a solution to bridge the gap. While it is widely recognized that different diagnostic techniques are appropriate in different ...
Problem solving is identifying and solving problems by applying critical thinking, creativity, communication, and analytical skills. It's an essential skill for managers because it helps them make informed decisions that can impact their team's productivity and the company's bottom line. In this blog post, we'll cover the five essential ...
What are the basic characteristics of managerial decision-making? Decision-making is the action or process of thinking through possible options and selecting one.. It is important to recognize that managers are continually making decisions, and that the quality of their decision-making has an impact—sometimes quite significant—on the effectiveness of the organization and its stakeholders.
Executive Overview An emerging problem-finding and problem-solving approach suggests that management's ability to discover problems to solve, opportunities to seize, and challenges to respond to is vital to organizations. This paper explores the extent to which the problem-finding and problem-solving approach can provide a foundation for joining the capabilities, dynamic capabilities, and ...
The key to effective and inventive problem solutions is often the ability to identify the "correct" and/or interesting problems (Getzels, 1975). Problem finding may be conceptualized as the very first stage in problem solving, i.e. the phase where problems are brought to awareness and initially interpreted.
Problem-solving A problem-solving decision-making style allows for management to create solutions to issues that exist within the workplace. This is a common style of decision-making, since a key role of management involves resolving workplace issues to improve workflow and create a positive environment for team members.
Problem solving. Process improvement. Source: Harvard Business Press Chapters. Product #: 2430BC-PDF-ENG. Length: 46 page (s) Unless managers and their teams clearly understand the roots of today's barriers to achieving strategic opportunities, their attempts to solve these p.
Managerial problem solving, action-oriented thought in organizational contexts, is a key target of DSS intervention and has been extensively researched from a number of perspectives. ... on the contributions of individuals. consider both problem and problem solver, the Narrowly construed, problem solving is action-ori- nature of the task as ...
Despite increased attention towards problem finding, the initial activity in problem solving, no integrated theory or common conceptual framework has been developed. This can partly be explained by competing vocabularies and perspectives due to the interdisciplinary nature of the topic.
This Course, MBA 835: Managerial Problem Solving is a course in the School of Management Sciences. It has been arranged into three modules which are all related, beginning with a kind of introduction to what management is, then ... Unit 1 The Nature of Management and Managerial Work Unit 2 Values of Management: Is Management an Art or Science?
Managerial problem solving models exhibit commonalities in form and content. Problem solving models have developed from learning theory but have not reached the refinement of expectancy motivation models which were derived from the same sources. An integrative problem solving model is proposed which includes applicable constructs from.
Nick Wilkinson adopts a user-friendly problem-solving approach which takes the reader in gradual steps from simple problems through increasingly difficult material to complex case studies, providing an understanding of how the relevant principles can be applied to real-life situations involving managerial decision-making. ... Nature, scope and ...
Summary. Objectives. To introduce and define managerial economics. To outline the types of issue which are addressed by managerial economics. To explain the difference between positive and normative economics. To explain the relationship between managerial economics, economic theory and the decision sciences. To explain how managerial economics ...
evolutionary approach to problem-solving behaviour (PSB) in firms, contrasting these with the. 1 The terms organisational form, structure and governance arrangements are used interchangeably in ...
Problem-solving vs. decision-making Problem-solving is an analytical method to identify potential solutions to a situation. It's a complex process and judgment calls, or decisions, may have to be made on the way. The primary goal is to find the best solution. Problem-solving involves identifying an issue, finding causes, asking questions and ...
The emphasis on and intensity of managerial activities varies considerably by the department the manager is assigned to. At a personal level, knowing that the mix of conceptual, human, and technical skills changes over time and that different functional areas require different levels of specific management activities can serve at least two ...
Investigates the nature of the organizational culture and the mechanisms of influence it exerted over the problem‐solving process. The research utilized a qualitative methodology well suited to investigating organizational culture and was based in a civil service agency. Suggests an alternative, or model, culture, the adoption of which would ...
A managerial problem solving methodology (MPSM) has been developed and extensively used [1-7] to solve Process problems. A Process is defined as an operational combination of man-machine systems which transforms basic (to the organization) inputs ($'s) to primary (to survival) outputs (products/services). A problem is defined by state-space ...
Managerial problem solving, action-oriented thought in organizational contexts, is a key target of DSS intervention and has been extensively researched from a number of perspectives. ... Existing theoretical work is reviewed in light of the nature of domain phenomena. A new theory building strategy, focusing on the conscious representational ...
Managerial Economics is the stream of management studies that emphasizes solving problems in businesses using the theories in micro ... So, in this blog, we will discuss the branch of economics that helps businesses to find a solution to almost every problem they may face. We will discuss the definition of managerial economics, its nature, its ...
This research examines problem definition as the first step in a sequential problem solving process. Seventy-seven managers in four diverse organizations were studied to determine common characteristics of problem definers. Among the variables considered as differentiating problem definers from non-problem definers were cognitive style, personal need characteristics, preference for ideation ...
Based on the nature of the problem and your organizational context, choose a problem-solving strategy that best fits your needs. Consider factors like time constraints, available resources, and the complexity of the issue when selecting a strategy. ... Managerial Problem-Solving Strategies: The Role of Emotional Intelligence.
Managerial economics is a stream of management studies that focus on decision-making and problem-solving. Both microeconomics and macroeconomics theories are applied. It focuses on the efficient utilization of scarce resources. It is a discipline that brings together the concepts of business and economics.
Collaborative skills are highly relevant in many situations, ranging from computer-supported collaborative learning to collaborative problem-solving in professional practice (Fiore et al., 2018).While several broad collaborative problem-solving frameworks exist (OECD, 2017), most of them are situated in knowledge-lean settings.However, one example of collaborative problem-solving of knowledge ...
Managerial problem-solving is a complex process with a high degree of ambiguity, since very little information is available to the manager, ... & Miller, S. L. (1994). The nature and constructs of relative expertise in economic problem-solving. Paper presented at the Annual Meeting of the American Educational Research Association, New Orleans, LA.
By further solving the eigenvalues in Table 3, we find that there are six stable points in the evolutionary game, and the specific scenarios are as follows: Table 3 Stability analysis of ...