
- Cambridge Libraries

Study Skills
Research skills.
- Searching the literature
- Note making for dissertations
Research Data Management
- Copyright and licenses
- Publishing in journals
- Publishing academic books
- Depositing your thesis
- Research metrics
- Build your online profile
- Finding support
Welcome to this module, where we will cover all the main aspects of looking after your research data, including:
- how to store and backup up data
- how to organise data
- what to do with protected data (personal or commercially sensitive)
- why sharing data is important and how to do it
- writing Data Management Plans
Data can take many forms: not only spreadsheets, but also images, interview recordings and transcripts, old texts, survey results, protocols... the list goes on.
To complete this section, you will need:

- Approximately 60 minutes.
- Access to the internet. All the resources used here are available freely.
- Some equipment for jotting down your thoughts, a pen and paper will do, or your phone or another electronic device.
Where did it all go wrong?
Lack of planning at the start of a project can cause problems (and much more work!) later on. Think of data management as a time investment to make sure that the data you collect is used effectively and remains usable over time.
Watch this video by the NYU Health Sciences Library as an example of poor data management and take some brief notes on any mistakes you spot. When you’re done, compare your notes with our answers underneath.
Check your answers
What did this researcher do wrong?
Here are all the mistakes we spotted: -he did not consider how others may want to reuse his data -he did not share the data in a repository -he was not aware of his funder and publisher requirements -he did not have multiple backups -he did not keep the data in a safe place -data on a USB stick is easy to lose -he did not use a safe way to share data (the post could have been lost) -he did not save the data in a common format -he did not save instructions on how to open the data -he did not plan for long-term preservation -he did not give variables intuitive names -he did not save metadata on what the variable names mean -he relied on knowledge found only in the brain of one person, rather than writing metadata
Keeping your data safe and up to date
Ensuring your data are safe is crucial to any research project. A good storage and backup strategy will help prevent potential data loss. Explore this scenario to see if your choices align with good research practice. Click on the link below to begin
Note: scenario opens in new window. Please view the scenario in full-screen. Return to this window to continue with the module, or if you wish to restart the scenario
Data storage and backup - why bother?
- Please visit the UK Data Service for more detailed tips on Storage and Backup of research data
- Branching scenario built using YoScenario
- All photos are CC-0 from Pexels
Organising data
Once you are sure that your data is safe from accidental loss, you should be thinking about how to organise it. Are your computer files ‘an amorphous plethora of objects’? In this video by the University of Edinburgh Data Library, Professor Jeff Haywood talks about his experiences of organising data.
If you want to read more about organising your data, including folder structures and file naming, there is a detailed guide on the Cambridge data website.
If you are at the start of a project, spend some time now preparing an organisational structure for your data. Create all the folders you are likely to need and a few named placeholders for files you will create. If you would like some feedback on it, email me .
Actvity - What should a PhD student do with her data?
Follow Martha in our scenario and help her make the best choices!
Sharing your data
Take a look a this video of Cambridge researchers talking about their experience of sharing data.
Using repositories
So what does it mean in practice to share your data? All you have to do is upload your dataset and information about it on a repository, either a subject-specific one, an institutional one like Apollo, or a general one. The repository then lets people find and download the data. Find out more in the video below.
Useful resources related to the video:
- Link to upload in Apollo
- Cornell metadata guide
- Funders policies on data website
- License Selector Tool
- Re3data
- Blog post by Blair Fix
- Bioinformatics Training Facility courses
- Slides for Sharing your data in repositories
Protected data
If your research data is of a personal or sensitive nature, you must make sure you understand and respect the additional requirements associated with managing it. If possible, get in touch with your department’s ethics committee, or your industrial sponsor to check what they expect of you. Additional help can be sought from the Research Data team , the Research Integrity team , and the Information Compliance Office .

What are personal and sensitive data?
Personal data is data relating to a living individual, which allows the individual to be identified from the information itself or from the information plus any other information held by the 'data controller' (or from information available in the public domain). The University of Cambridge as a whole is the data controller. Sensitive data is personal data about: racial or ethnic origin, political opinions, religious beliefs, Trade Union membership, physical and mental health, sexual life, or criminal offences and court proceedings about these.
What are the legal requirements for data protection?
The The EU General Data Protection Regulation (GDPR), coupled with the UK Data Protection Act 2018 (DPA 2018) gives individuals certain rights and imposes obligations on those who record and use personal information to be open about how information is used and to follow eight data protection principles. Personal data must be: processed fairly, lawfully and transparently; obtained for specified, explicit and lawful purposes; adequate, relevant and not excessive; accurate and, where necessary, kept up-to-date; not kept for longer than necessary; processed in accordance with the subject's rights; kept secure; not transferred abroad without adequate protection
How should I store my sensitive or confidential data?
You should limit physical access to sensitive data or encrypt it (speak with your local IT/Computing Officer or the University Information Services Help Desk for help in doing this). To avoid accidentally compromising the data at some future date, you should always store information about the data's sensitivity and any available information on participants' consent or use agreements from your data provider with the data itself (i.e. put information about lawful and ethical data use in your data documentation or metadata description).
Data supporting my research is personal or sensitive. How do I share these data?
There can be a potential conflict between abiding by data protection legislation and ethical guidelines, whilst at the same time fulfilling funder's and individual's requirements to make research results available. Consult your ethics committee before deciding to share participants’ data. Your plans for research data processing, storage and sharing should be considered at the start of each project and reflected in both your data management plan and consent form. For example, you can inform your participants that anonymised data will be shared via the University of Cambridge data repository. There is good guidance on consent forms at the UK Data Archive (www.ukdataservice.ac.uk). The UK Data Archive also provides a sample consent form. Your Department’s Ethics Committee may also provide sample consent forms.
If you would like to learn more about personal and sensitive data and do some practical exercises on identifying these data types, the University of Cambridge offers short 30-mins long online courses on personal and sensitive data .
You should also consider whether your data is commercially sensitive: do you or a sponsor plan to profit from the research in the future? There should be a collaboration agreement in place from the start to clarify the terms of any commercial collaboration. The Research Operations Office can help with this. If you are working with both public funders and commercial partners, clarify early what data can be shared and what can’t, so you can make this clear to all parties.
Data Management Plans
Throughout this module we have seen how important it is to plan the way you will manage your data right at the start of a project. A Data Management Plan (DMP) is a document that captures that process.

To end this module and pull together everything you have learnt, we recommend you write your own DMP for a project you are about to start or have recently started. Use these instuctions as a guide.
- DMP activity
Did you know?

How did you find this Research Skills module

- << Previous: Note making for dissertations
- Next: Copyright and licenses >>
- Last Updated: Apr 11, 2024 9:35 AM
- URL: https://libguides.cam.ac.uk/research-skills
© Cambridge University Libraries | Accessibility | Privacy policy | Log into LibApps

Ontario Human Rights Commission
Language selector, ohrc social links.

Main Navigation
- The Ontario Human Rights Code
- The Human Rights System
- The Ontario Human Rights Commission
- The Human Rights Legal Support Centre
- The Human Rights Tribunal of Ontario
- Frequently asked questions
- Family and marital status
- Gender identity and gender expression
- Race and related grounds
- Receipt of public assistance
- Record of offences
- Sexual orientation
- Goods, services and facilities
- Membership in vocational associations and trade unions
- Teaching human rights in Ontario
- Public education requests
- The OHRC at 60
- Backgrounders and research
- Brochures, fact sheets and guides
- Papers and reports
- Policies and guidelines
- Submissions
You are here
6. what is involved in collecting data – six steps to success, page controls, page content.
If an organization is considering whether to collect data on its own or get help from an external consultant, it will need to have enough information to make an informed decision about how to proceed.
This section outlines some of the key considerations that may arise during various steps in the data collection process. There is no requirement that these steps be followed or pursued in the order that they are written. The model presented is offered as a reference tool. How data is gathered and analyzed depends on many factors, including the context, the issue that needs to be monitored, the purpose of the data collection, and the nature and size of the organization.
The main consideration is to make sure that any information collected is done in a way and for a purpose that is consistent with the Code and complies with freedom of information and privacy protection legislation. In the interest of effectiveness and efficiency, it is recommended that efforts be made to collect data that will shed light on issues or opportunities. To protect the credibility and reliability of data, information should be gathered using accepted data collection techniques.
Step 1: Identify issues and/or opportunities for collecting data
The first step is to identify issues and/or opportunities for collecting data and to decide what next steps to take. To do this, it may be helpful to conduct an internal and external assessment to understand what is happening inside and outside of your organization.
Some organizations, like FCP and Legislated Employment Equity Plan (LEEP) [21] employers, are given specific direction on what issues should be explored and how data must be collected. Other organizations may have more flexibility to decide when and how to collect information to achieve certain goals. Some of the non-exhaustive questions identified below may apply to a diverse range of organizations and audiences, including employees and service users. Depending on the organization, these questions may be considered at Step 1, or at different stages in a data collection process.
Conduct a review of all policies, practices and procedures applicable to employees, service users or another appropriate audience:
- Does the organization have human resources and human rights policies, practices and procedures that are accessible to all employees or to the people they serve?
- Does the organization have clear, transparent and fair complaint procedures in place to deal with allegations of discrimination, harassment or systemic barriers?
- Do any signal barriers to persons protected under the Code and/or other individuals/groups in society based on a non- Code ground?
- Have any been dealt with appropriately and in accordance with existing polices, practices and procedures?
Explore organizational culture from a human rights, diversity and equity-inclusion lens:
- What are the organization's mandate, goals and core values?
- What is the history of the organization?
- Are equity, diversity and inclusiveness supported, reflected and promoted by senior leaders throughout the organization?
- Are performance measures in place to motivate the achievement of an organization’s strategic human resources, human rights, equity and diversity goals?
- Do employees feel that the organization is diverse, inclusive, and provides equal opportunity for learning and advancement?
- How are decisions made?
- How are employment, programming or service delivery opportunities advertised?
- Does the organization have formal, transparent and fair processes in place to recruit, hire, promote, terminate and retire staff?
- Is this system perceived to be applied fairly and consistently?
- Do service users feel that they are welcome, valued, and able to use the services offered by the organization?
Assess external context:
- Are there best practices in the industry/sector or among similar organizations that can be learned from?
- Are there objective data or research studies showing that discrimination or systemic barriers exist or do not exist in the organization, industry/sector or similar organizations?
- Is there evidence from other organizations or jurisdictions that a policy, program or practice, similar to one in place at the organization, has had a positive or negative impact on Code-protected persons or other marginalized persons in society?
- How is the organization perceived by the community it operates in?
- Have the media or advocacy groups complimented or criticized the organization about human rights, human resource or equity issues?
- Are the demographics changing or projected to change in the future?
- Is the organization proactively looking at ways to make sure that it has the skills and knowledge to meet the potential needs and concerns of this changing demographic?
Check representation:
- Compare the organization’s workforce makeup to the availability of labour or the demographics of the service users in the community, city, region, province and/or country it operates in.
- Is the organization representative of and responsive to the needs of the community it serves?
- At this stage, a detailed comparison is not needed. The goal here is to identify key issues and/or opportunities that may need further study by noting obvious gaps, disparities or trends.
- Estimate how people or groups identified by Code grounds and other persons/groups are represented and distributed among their employees or service users by levels of responsibility, occupation, branch, department or other appropriate measure.
- Are there any areas in the organization or in service delivery where the persons or groups seem to be obviously over-represented or under-represented?
Finding the above information can be challenging for smaller organizations, but the internet offers a wealth of resources to choose from. Media reports may offer insights, as well as on-line resources offered by the OHRC, Statistics Canada, [22] the City of Toronto, [23] government agencies, and community organizations that focus on Code and non- Code ground-related topics. Information may also be gathered from various sources using accepted data collection research methodologies discussed in Step 3.
It is to be expected that an internal and external assessment of the organization, in light of the questions listed above, may result in a number of potential issues and/or opportunities for exploring data collection. Before proceeding to Step 2, organizations may wish to consider whether there are any preliminary actions that can be taken to address these issues and/or opportunities, without collecting data ( e.g. training, policy development).
Example: The review in Step 1 may have identified the following issues and/or opportunities for collecting data:
- Positive public feedback received about a pilot community policing project in high-crime neighbourhoods
- Unclear and inconsistent human rights policies and procedures in place to address sexual harassment.
The above examples present a potential opportunity or problematic human rights issue, respectively, and could lend themselves to data collection. Decisions need to be made about how best to address the identified opportunities and/or issues and whether it would be appropriate to act, based on the assessments in Step 1 (either instead of or together with further data collection).
If the results of the internal and external assessment seem to show that the organization does not have any pressing problems with discrimination and/or systemic barriers, and is generally in compliance with the Code and OHRC policies, consider whether the organization could still benefit from proactively implementing a data collection initiative (for example, to help monitor the ongoing effectiveness and suitability of policies, programs and intervention strategies).
Step 2: Select issue(s) and/or opportunity(ies) and set goals
The focus of Step 2 is choosing a priority issue(s) and/or opportunity(ies) for collecting data, and then setting goals and objectives.
The organization reviews the issues and/or opportunities identified from the internal and external assessment done in Step 1, and picks one or more specific issues and/or opportunities for starting a data collection project from among the list of priorities. Some of the questions an organization can consider when deciding to prioritize an issue and/or opportunity for gathering data include:
- Is there a fundamental reason or opportunity to collect data from which other issues and/or opportunities seem to arise?
Example: An aging taxpayer base provides a government body with a pressing reason to collect data on this group’s projected size, needs and revenue base. This changing demographic also presents an opportunity for the government body to ensure that it is proactively developing policies, programs and services that are accessible and appropriate to meet the needs and concerns of these taxpayers.
- Did the internal and external assessment of the organization in Step 1 reveal any critical gaps or trends that are apparent in the organization, industry/sector or similar organizations?
- Is there one particular area that has drawn positive/negative media attention or been subject to multiple complaints, internal rumours and concerns?
- Does there seem to be a greater diversity or lack of diversity in one area compared to others?
Goal-setting
While the organization may intend to collect data relating to multiple issues and/or opportunities at the same time, the next steps, including goal-setting, should be individualized for each issue and/or opportunity.
The specific goal(s) defined for each issue and/or opportunity may depend on a hypothesis or guess about what is happening that can be tested using data collection techniques and analysis.
Example: A downtown Toronto hotel receives complaints from guests, who self-identify as being gay, about the unwelcome treatment they received from staff. A hypothesis might be that hotel staff lack sufficient awareness and training about how to deal respectfully with guests who are gay, or are perceived to be from the larger LGBT community. The goal is to get enough evidence to test this hypothesis.
Step 2 can also involve an organization brainstorming a smaller set of questions that may be answered by collecting data. Rather than asking a general question like, “Is there any evidence of discrimination on the basis of sexual orientation or gender identity in this hotel?” one might ask, “What percentage of hotel guests self-identify as being part of the LGBT community?” and “What are the perceptions of the service received by self-identified LGBT patrons?” Ultimately, data that is collected should be rationally connected to the goals set and the overall purpose for collecting the data.
Step 3: Plan an approach and methods
In Step 3, organizations will make decisions about who will be surveyed, how data will be collected, the sources of data that will be used, and the duration of the data collection project, among other questions. These decisions may be made in consultation with an expert. The methods and approaches will flow from the goals set in Step 2, and will vary significantly depending on a number of factors, including the organization’s context, size, resources, and the purpose and complexity of the issue(s) or opportunity(ies) selected.
Some of the questions to consider at this stage include:
- Who will the data be collected about?
The “group of interest” (e.g. youth service users of a local community centre who cannot read and speak English as a second language) will be the focus of the study, and the data collection methods used will refer to this group, or the persons within it, depending on the goals of the project.
Understanding discrimination
- When thinking about who the data will be collected about, it is important to consider who you think will be most affected by, for example, the discrimination or inequities that you wish to measure. Is it a broad category (e.g. all service users who cannot read), or a sub-set of that category (e.g. youth service users who cannot read)? The italicized words refer to a unique characteristic about a broader group that an organization may wish to gather information about.
- Depending on factors like the goals of the data collection project, the organization’s size, resources and time, data may be gathered about many sub-sets within a broader group of interest (e.g. youth service users who cannot read and who speak English as a second language ).
- Collecting data about a group of interest that shares characteristics, based on several Code or non- Code grounds, can help an organization understand the behaviour, perceptions, values and demographic makeup of services users and other subjects of interest. Generally speaking, gathering data that reflects more than one Code and/or non- Code ground can allow for richer, nuanced information and more complex analysis.
- It is important to recognize that based on their unique combination of identities, people may be exposed to particular forms of discrimination. Multiple forms of discrimination can intersect and compound to form a unique experience of discrimination. This perspective is referred to as an “intersectional” analysis of discrimination.
Example: A South Asian male youth service user, who cannot read and speaks limited English, may face discrimination on any of the grounds of age, race, colour, ancestry, ethnic origin, place of origin, gender, disability or perceived disability ( e.g. could be seen as having a learning disability). However, he may also be exposed to discrimination on intersecting grounds based on being identified as a “young, illiterate Indian male from a foreign country,” based on the various assumptions or stereotypes that are uniquely associated with this socially significant interaction of multiple identity factors. To better understand the potential impact of multiple identity factors, or intersectionality, when collecting and analyzing data about a group of interest, it may be helpful to consult with communities, and review applicable research and other relevant documents that highlight how the dynamic of discrimination and disadvantage can play out in a practical way for persons identified by Code and non- Code grounds. The OHRC’s recent edition of Human Rights at Work is a useful reference for this purpose. The OHRC has also developed policies and guidelines that provide a more detailed outline of how the Code applies to the various grounds (see Appendix G for a list of OHRC guides, policies and guidelines).
- Who will the group of interest be compared to?
The “comparator group” [24] should be persons who share one or more characteristics with the persons in the group of interest, but differ in the key characteristic(s) being studied (e.g. youth service users who cannot read but can speak English fluently). The experiences of youth service users who cannot read and who speak English as a second language can then be compared to youth service users who cannot read but can speak English fluently.
- What locations or geographical areas will the data be gathered from?
Some data collection initiatives require gathering data from multiple sizes, groups or communities located in different locations and geographical areas. When determining where to collect information from, key factors to consider include who the data will be collected about and who the data will be compared to. Example: A local community centre is interested in making its current youth literacy program more responsive to the needs of an increased number of youth in the surrounding area who cannot read and who speak English as a second language. The community centre plans to gather information about the community it serves and the geographical region it is located in. Data is gathered from the community centre’s pre-existing records relating to its service users, including people who attend the youth literacy program or have expressed an interest in it. Publicly available information about the characteristics of the surrounding neighbourhood is also explored, among other data sources.
- What categories will be used to identify the group of interest and comparator group?
Choosing categories provides a way to organize the information that is collected. This can be done either before collecting data, as discussed in this step, or after data is collected (see Step 5).
In some cases, although it is not required, it is preferable to use pre-determined categories such as those developed by Statistics Canada. There are certain benefits to this approach. Example: Organizations can be confident that the 12 racial groups used by Statistics Canada will represent how the majority of Canadians racially classify themselves. In addition, use of these categories is most likely to produce reliable and valid results and enable researchers to directly compare the results of their studies to Census data collected by Statistics Canada. [25] The limitations are that if these categories are used, some respondents may not identify with them or may object to them. Another limitation is that Statistics Canada does not produce Census data on all grounds (for example, on sexual orientation). [26] For a fee, Statistics Canada will customize its data. For example, it can break it down to “disaggregated” data for a local labour market or for a particular occupational category. [27] Another limitation is that the Statistics Canada categories may be too broad depending on the goals selected in Step 2. Example: Using a broad category such as “racialized” can mask important differences between racialized groups, since racialized groups are not subject to exactly the same experiences, racial stereotypes and types of discrimination. [28] However, when it is necessary to describe people collectively, the term “racialized person” or “racialized group” is preferred over terms like “racial minority,” “visible minority,” “person of colour” or “non-White” as it expresses race as a social construct rather than as a description based on perceived biological traits. Also, these other terms treat “White” as the norm that racialized persons are to be compared to, and have a tendency to group all racialized persons in one category, as if they are all the same. [29] Consider other categories to describe the groups selected (for example, relating to job or service categories). Organizations may ultimately choose the categories that best reflect where the organization is at in terms of achieving its human rights, equity and diversity goals.
How should data be collected?
In the context of human rights, social-science researchers [30] are commonly asked to lead or help with data collection projects. Two types of data are used in social science research: qualitative and quantitative. A good research effort involves the use of both types. Both approaches, while distinct, can overlap and rely on the other to produce meaningful data, analysis and results.
Qualitative data:
- Typically, data is called “qualitative” if it is in the form of words, but may also include any information that is not numerical in form, such as photographs, videos and sound recordings.
- Qualitative methods are aimed at describing a specific context, event, people or relationship in a broad contextual way, by trying to understand the underlying reasons for behaviour, thoughts and feelings.
- Common qualitative research methods include observation, one-on-one interviews, focus groups and intensive case studies.
Example: A restaurant chain wants to improve service and access to customers with disabilities. Management decides to collect qualitative information using focus groups consisting of a range of stakeholders, including customers and representatives of organizations from the disability community.
Potential strengths:
- qualitative data excels at "telling the story" from the participant's viewpoint (it helps participants feel like they have been heard)
- can help others better understand the issue or problem by providing the rich descriptive detail that explains the human context of numerical results
Potential weaknesses:
- perceived that the accuracy of qualitative data can be influenced by false, subjective or manipulated testimonies. Good qualitative data, checked by a professional researcher and gathered using accepted data collection research methods, can address the impact of such factors
- depending on the nature and size of the project, as well as the sophistication of the methods and analysis used, can take a significant amount of time, be very labour-intensive, and yield results that may not be general enough for policy-making and decision-making purposes.
Quantitative data:
- Typically, data is called “quantitative” if it is in the form of numbers.
- A quantitative approach can be used to count events or the number of people who represent a particular background.
- Common quantitative tools include surveys, questionnaires and statistical data (such as Statistics Canada census information).
- It is important to note that all quantitative data is based on qualitative judgment. In other words, numbers cannot be interpreted by themselves, without understanding the assumptions that underlie them.
Example: A simple 1- 5 rating variable for the survey statement, “My union handles human rights grievances in a sensitive and efficient manner” gives respondents the option of circling: 1 (Strongly Disagree), 2 (Disagree), 3 (Neutral) 4 (Agree) and 5 (Strongly Agree). A respondent circles “2 = Disagree.” To understand the value of “2” here, a researcher must consider some of the judgments and assumptions that are behind this choice. Did the respondent understand the term "human rights grievance"? Has the respondent had experience filing a grievance with the union? Does the respondent like unions generally?
- perceived to be more credible and reliable than qualitative data because of the use of numbers, which are seen as an objective source of data. This is not necessarily the case. The accuracy of quantitative data can be influenced by manipulation and bias of the researcher, among other factors, unless checked by the researcher’s professionalism and the use of accepted data collection research methods
- quantitative data excels at summarizing, organizing and comparing large amounts of information, and drawing general conclusions about a research topic of interest
- can help measure progress and success
- good at identifying trends and determining the magnitude of a research topic of interest.
a focus on numbers and rankings alone can overly simplify or lead to an inaccurate understanding of complex situations and realities, unless a broader context is provided
Example: An employment data survey of the Custodial Services Division of a large organization reveals that 80% of the cleaning staff are women and that 6 of 7 Custodial Services supervisors are men. A comparison between these figures and gap data from Human Resources and Skills Development Canada (HRSDC) shows that, while there is an overrepresentation of women in the ranks of cleaners, there is no gap for women in the ranks of supervisors. The reason for the seeming discrepancy is that HRSDC gap data is based on availability. Nationally, so few women are Custodial Services supervisors that there is a statistically insignificant availability, giving rise to the conclusion that there is no numerical gap with respect to women supervisors. This conclusion, however, does not make sense since the organization knows that the 200:40 women to men cleaning staff ratio is supervised by a 6:1 male to female supervisory staff ratio. The organization decides to ignore the HRSDC data and apply common sense by setting up career advancement mentoring and other policies and programs to increase the number of female supervisors in its workforce.
- subject to multiple interpretations of what the numbers actually mean, which can lead to a distorted understanding of a research topic of interest. This potential weakness can be minimized by using accepted quantitative research methods and identifying appropriate warnings to explain the parameters and assumptions underlying the research
- depending on the nature and size of the project, as well as the sophistication of the methods and analysis used, it can be costly to gather the required information
- in areas of research that are relatively new or where tools, indicators, procedures and sources are far from settled, statistical data can be lacking or of unequal quality, causing problems for comparisons. These difficulties are often compounded by other issues, like definition problems (e.g. the meaning of the word “freedom” – depending on the interpretation of the word chosen, it may produce different issues and results).
What sources of data should be used to collect information?
Qualitative and quantitative data are generally gathered from more than one source. Where possible, two or more of the following sources should be used together to strengthen reliability and consistency in results.
Pre-existing or official data
Pre-existing or official data is information that has already been documented (e.g. newspaper clippings, case law, Statistics Canada census data, photographs) or is created by an organization during its routine business operations (e.g. employee personnel files, student registration forms, annual reports, occurrence reports). This data may contain information that directly relates to specific Code grounds like race, but more commonly will relate only indirectly (for example, in the form of names, place of origin or ethnicity). This type of information could be used as proxies or stand-ins for race, but would be less reliable than actually having self-reported racial data.
- is efficient. Avoids the time, energy, expense and disruption involved in collecting data as a separate step from running daily operations
Example: Outcomes of workplace recruitment, hiring, promotions and terminations can be recorded, as can events such as interventions by security guards and customer complaints. When recording these events, relevant Code ground and non- Code classifications could also be included. This data could then be examined for trends over time to show whether discrimination or systemic barriers exist, may exist or do not exist.
- to be a useful source of information, organizations need to be willing to collect the data as part of their ordinary record-keeping procedures
- the reliability of this data will depend on the diligence and accuracy of the reporting done by the people collecting it.
Survey data
Survey research is a broad area and generally includes any measurement procedures that involve asking respondents questions. A "survey" can range from a short paper-and-pencil questionnaire to an in-depth one-on-one interview (interviews will be discussed further below). In designing a survey, it is important to consider the specific characteristics of the respondents, to make sure that the questions are relevant, clear, accessible and easy to understand. Some practical considerations to keep in mind are whether the respondents can read, have language or cultural barriers, have disabilities, and can be easily reached.
- very useful for documenting an individual’s perceptions and perceived experiences of an organization’s work culture, service delivery or other areas of interest
Example: The TDSB’s 2006 Student Census, Grades 7-12 System Overview included a component on how senior and secondary school students generally perceived their schooling and out-of-school experiences in 10 areas, including school safety and home support and involvement.
- can contain questions that are quantitative or qualitative in nature, or a combination of both
- can be conducted on a small or large scale.
- quality and reliability of survey data depends on factors like the expertise of the people conducting them, the design and appropriateness of the questions asked, and the credibility of the methods used to analyze and interpret the results
- may not provide an accurate measure of how others perceive a person’s background or experience.
Example: A transgender employee may self-identify as female but a third party may identify her as male.
Focus groups and interviews
Interviews and focus groups (also referred to as “group interviews”) allow for information to be provided orally, either individually or in a group setting. The data can be recorded in a wide variety of ways including written notes, audio recording and video recording.
Focus groups:
In focus groups, the interviewer facilitates the session. A select group of people are brought together, asked questions, encouraged to listen to each other's comments, and have their answers recorded. The same set of questions may be used for a number of different groups, each of which is constituted slightly differently, and for a range of purposes. Focus groups may be facilitated by professionals, but this is not always needed. The decision to hire a professional facilitator may depend on the goals of the focus group research, the nature of the questions asked, the skills and experience of staff taking part, and the need for confidentiality or anonymity. Example: To get the unique perspective of each group, an organization may wish to hold separate focus groups for representatives of each of the organization’s internal and external stakeholder groups, such as senior management, front-line employees, service users, union representatives and community groups. Or, it may be of greater value to organize a group that includes people representing all key internal and external stakeholders, to allow for contrasting ideas to be expressed and discussed. Whatever format is chosen, it is important that the focus group is structured and managed in a way that cultivates a “safe space” for people to share their experiences. In some cases, this may not be possible without setting up separate focus groups or hiring a professional facilitator who is not connected to the organization.
- focus groups allow for multiple narratives to be voiced in one “interview” about a research topic of interest
- act as tools for education because discussion among participants can illuminate the participants’ and the researcher’s views, helping to further refine research about a particular topic of interest.
Potential weakness:
- does not allow participants to fully express their individual opinions and narratives, or ask questions when they immediately come to mind, because of the need to hear and accommodate other voices.
Interviews:
Typically, interviews involve a set of standard questions being asked of all respondents, on a one-on-one basis, so that accurate trends and gaps can be drawn from the data. Interviews are commonly conducted face-to-face, but for more rapid results, can also be done over the telephone, or, as technology advances, through video-conferencing and other means.
- interviews can provide a rich, detailed perspective, impression or story on a research topic of interest
- the interviewer generally has the opportunity to probe more deeply or ask follow-up questions than when in a focus group setting
- data from both focus groups and interviews can provide valuable context for understanding and informing research, numbers, events, behaviour and other research goals
- depending on the size of the organization, the purpose of the data collection, the internal expertise available and other factors, focus groups and interviews can be done with relatively little expense.
- one-on-one interviews allow for just one narrative or perspective on a research topic of interest
- can be very time consuming and resource intensive
- respondents in interviews and focus groups generally want to "look good" in the eyes of others. Depending on the questions asked, they might “spin” their response to avoid being embarrassed, particularly in a face-to-face setting. Skilled interviewers may be able to address this potential weakness by doing a few things, like designing good questions, being perceptive, asking follow-up questions and cross-checking responses with other credible sources of information
- interviewers, in both individual and focus group settings, may distort an interview by not, for example, asking questions that make them uncomfortable or not listening carefully to respondents on topics that they have strong opinions on. The impact of this potential weakness can be addressed by taking steps like making sure that interviewers are properly trained and using standard interview questions.
Observed data
Trained staff or external experts can gather data by identifying and recording the characteristics and behaviour of research subjects through observation, either within or outside of an organization. Observed data can include information gathered using all of the senses available to the researcher, including sight, hearing, smell, taste and touch. Example: A human rights organization that offers a mediation service hires a mediation expert to observe mediators and service users and provide feedback about any issues of concern related to human rights. To minimize potential stress and anxiety experienced by the people being observed, staff and service users are informed in advance of the purpose and goals of the exercise. Service users’ consent is sought. Staff is advised that the observed data gathered will only be used for research purposes and not shared with their managers. The expert maintains access to the data, and the results are reported on an aggregated and summarized basis to prevent individuals from being identified. Hiring experts, while potentially expensive, can add validity and credibility to research analysis because they are often perceived as having no vested interest in the research results. Information gathered using observation techniques differs from interviewing, because the observer does not actively ask the respondent questions. Observed data can include everything from field research, where someone lives in another context or culture for a period of time (participant observation), to photographs that show the interaction between service providers and service users (direct observation). The data can be recorded in many of the same ways as interviews (taking notes, audio, video) and through pictures, photos or drawings.
- an effective and capable observer can provide an objective third viewpoint on what is going on, and draw out implications that are not obvious or that people are unaware of
- can be relatively inexpensive depending on factors like the size of the project, its goals, the organization’s resources and the duration of the project.
- an observer, trained or otherwise, can influence the behaviour of the people being observed (for example, people could be motivated to behave better while under observation), which may ultimately affect the accuracy of observed results
- can cause potential stress and anxiety for the people being observed, more than the use of other data collection methods. Efforts can be made to minimize stress and anxiety by using effective communication strategies to inform participants, in advance, of the purpose, goals, confidentiality measures, duration of the project and other key information
- an observer, trained or otherwise, may not always be able to accurately differentiate within or between certain groups of people, particularly when an identity (ies) is/are non-evident (e.g. religion, mental illness, sexual orientation). A survey requesting self-identification information might be more effective in this regard.
Each source of data used to collect information has its strengths and weaknesses. Some of the more common potential strengths and weaknesses identified above have been highlighted. Analyzing data from multiple perspectives and relying on data from different sources can strengthen the conclusions drawn from research. A combination of statistical analysis, observational data, legal analysis, documentary analysis, in-depth interviews and external and/or internal consultation can help maximize understanding of a given situation. [31] Organizations should choose the sources of data that best suit their program goals, context, resources and organizational culture.
How long will the data be collected (the scope of data collection)?
Data can be collected and analyzed on a short-term or project basis in response to situations or needs that arise from time to time. A short-term data collection project would include a start and a finish date, with set deliverables to be carried out over a certain period of time. The best practice is to collect data on an ongoing, permanent basis, and to analyze this data as often as is needed to identify, address and monitor barriers to Code -protected persons or other persons based on non- Code grounds. Data collected in a time-limited study may be less complete than data collected through ongoing monitoring. This is because short-term studies do not allow for the assessment of trends, patterns or changes over time. However, where costs, time and resources are a factor, short-term studies may be the preferred choice to fulfil a need and project goals. Other factors may also influence the reliability of the data. For example, people may modify behaviour while under scrutiny during the data collection period.
Step 4: Collect data
When planning on how best to collect data in Step 4, it is important to be aware of the practical considerations and best practices for addressing logistical challenges organizations often face at this stage of the process. Implementing a data collection plan requires attention to matters such as:
- Getting buy-in from senior leadership and key stakeholders, in or outside of the organization. This group could include boards of directors, management committees, union representatives, employees, community groups, tenants, customers and service users.
- Establishing a steering committee or selecting a person(s) to be consulted and held accountable for all major decisions about the data collection process, such as design, logistics, communication management, coordination and finances.
- Determining who will collect the data (e.g., experts or trained employees).
- Identifying the logistics, resources, technology and people needed to develop and implement a data collection initiative.
- Anticipating and addressing key stakeholder concerns and questions about the project.
- Designing a communication and consultation strategy that will explain the data collection initiative and encourage the highest possible participation rate.
- Protecting privacy and personal information by using carefully controlled procedures for collecting, storing and accessing data that comply with privacy, human rights and other legislation. Dignity and confidentiality must be respected.
- Minimizing the impact and inconvenience for the people affected in the workplace or service environment, which includes choosing the best time to collect the data.
- Aiming for flexibility to allow for changes without great expense or inconvenience.
- Considering a test period or a pilot phase to allow you to improve and modify data collection methods, as may be needed.
Step 5: Analyze and interpret data
Step 5 involves analyzing and interpreting the data collected. Whether quantitative and/or qualitative methods of gathering data are used, the analysis can be complex, or less so, depending on the methods used and the amount of data collected.
Explaining the technical steps involved in analyzing and interpreting data is beyond the scope of this guide. An organization will have to determine whether it has the internal capacity and expertise to analyze and interpret data itself, or whether it will need the help of an external consultant.
A smaller organization that has basic data collection needs may be able to rely on internal expertise and existing resources to interpret the meaning of gathered data.
Example: An organization with 50 employees wants to find out if it has enough women working in management positions, and if there are barriers to equal opportunity and advancement. The organization counts the number of female employees it has (25), and determines how many of these employees are working in supervisory and management positions (two). A few motivated employees identify some issues of concern, like gender discrimination, that may have broader implications for the organization as a whole.
After deciding to do an internal and external assessment (Step 1), and gather qualitative data using focus groups and interviews with current and past employees, senior leadership decides that barriers exist for women in the organization’s recruitment, hiring, promotion and human resources policies, processes and practices. Efforts are made to work with female employees, human resources and other staff to address these barriers. The organization makes a commitment to foster a more equitable, inclusive work environment for all employees.
Step 6: Act on results
Once an organization has analyzed and interpreted the results of the data collected, it may decide to act on the data, collect more of the same type of data or modify its approach.
Quantitative and qualitative information can provide a solid basis for creating an effective action plan designed to achieve strategic organizational human resources, human rights, equity and diversity goals identified through the data collection process. If an organization feels it has enough information to develop an action plan, it should consider including the following elements:
- a summary of the results of the analysis and interpretation of the data
- identification of the barriers, gaps and opportunities that exist or may exist for Code-protected persons and other individuals/groups based on non- Code grounds
- steps that will be taken to address these barriers, gaps or opportunities now and in the future
- realistic, attainable goals with short-term and longer-term timelines
- input sought from stakeholders and affected communities
- how progress in meeting these goals will be monitored, evaluated and reported.
In some cases, an organization may decide that it needs to collect more information because there are gaps in the data collected, or areas where the data is unclear or inconclusive. This may prompt them to conduct a more detailed internal and external assessment (go back to Step 1) or try another approach.
In the end, there is no one or “right way” to conduct a data collection initiative. The experiences of Mount Sinai Hospital, KPMG Canada, the Keewatin-Patricia District School Board, TD Bank Financial Group, the University of Guelph and the DiverseCity Counts project and featured in the Appendices reflect this statement, yet also show some similarities in terms of the best practices and lessons learned.
Six steps to success
Step 1: Identify issues and/or opportunities for collecting data Step 2: Select issue(s) and/or opportunity(ies) and set goals Step 3: Plan an approach and methods
- Qualitative Data
- Quantitative Data
- Pre-existing or official data
- Survey data
- Interviews and focus groups
- Observed data
How long will the data be collected (the scope of data collection)? Step 4: Collect data Step 5: Analyze and interpret data Step 6: Act on result
[21] The Employment Equity Act (the Act ) applies to federally regulated employers, like banks, transportation and communication companies with 100 or more employees, as well as to Crown corporations and the federal public service. Employers covered by the Act are known as Legislated Employment Equity Plan (LEEP) employers. [22] Statistics Canada online: www.statcan.gc.ca . [23] The City of Toronto offers many publications and reports on its website relating to an array of topics by sector or topic, including the labour force. See City of Toronto, Publications and reports , online: www.toronto.ca/business_publications/publications.htm . [24] The term “comparator group” is used to determine whether human rights “discrimination” in fact exists in a scenario. Comparison is made between a group claiming discrimination and another group that shares the relevant characteristics, to determine if disadvantage, denial, devaluation, oppression or marginalization has been experienced. A comparator group must share relevant characteristics with the group of interest in the area being questioned for comparison to be meaningful. Who the appropriate comparator group is will depend on the context and is often contested between litigants. Often the comparator group is a more privileged group in society, often the dominant group. [25] S. Wortley, The Collection of Race-Based Statistics Within the Criminal Justice and Educational Systems: A Report for the Ontario Human Rights Commission (Centre of Criminology, University of Toronto) [unpublished], online: www.ohrc.on.ca . [26] Data collection based on certain grounds, such as ethnic origin, sex and disability, has been done for many years under federal employment equity legislation, the national census that takes place every five years or in accordance with international requirements. In comparison, data collection on other grounds, such as sexual orientation, has not been done much in the past. Notably, the national Census does not include a question about sexual orientation, although sexual orientation has been included on other non-mandatory surveys and has been the subject of testing. Statistics Canada, Ministry of Industry “2006 Census Content Consultation Report, Catalogue No. 92-130-XE (2003, Revised in February 2004). [27] C. Agocs, “Surfacing Racism in the Workplace: Qualitative and Quantitative Evidence of Systemic Discrimination” (2004) 3:3 Canadian Diversity at 26, online: www.ohrc.on.ca . For more information about Statistics Canada’s “custom services” see Statistics Canada, supra note 27. [28] S. Wortley, The Collection of Race-Based Statistics Within the Criminal Justice and Educational Systems: A Report for the Ontario Human Rights Commission (Centre of Criminology, University of Toronto) [unpublished], online: www.ohrc.on.ca at 6. [29] See Ontario Human Rights Commission’s Policy and Guidelines on Racism and Racial Discrimination (2005), online: www.ohrc.on.ca at 9-10. [30] Social Science is defined as the scientific study of human society and social relationships. The Concise Oxford Dictionary Ninth Edition , s.v. “social science.” [31] J.-C. Icart, M. Labelle, R. Antonius , Indicators for Evaluating Municipal Policies Aimed at Fighting Racism and Discrimination, Report presented to UNESCO, Fight against Discrimination and Racism Section, Division of Human Rights and Fight against Discrimination Sector for Social and Human Sciences (MontréaI, Québec: International Observatory of Racism and Discrimination: Centre for Research on Immigration, Ethnicity and Citizenship (CRIEC), Université du Québec à Montréal, 2005) at 47, online: CRIEC www.criec.uqam.ca/pdf/CRIEC%20Cahier%2028%20 (en).pdf .
Book Prev / Next Navigation

Research Data Management Self-Assessment
- Self-Assessment Rubric for researchers
- Planning for Data
Organizing Data
- Saving Data
- Preparing Data
- Analyzing Data
- Sharing Data
Organizing data involves ensuring that you can find your data and other research materials (including documentation, code, and physical samples) when you need to and ensuring that data and materials that go together are connected in a meaningful way.
What does it mean to organize data?
Organizing data means arranging your data and other research materials so they can be found – by yourself and by others – as needed. Here are four factors to consider when organizing data. Remember, you can’t use data you can’t find.
Requirements and How to Meet Them
There are specific requirements about how some human subjects data can be organized. Under most circumstances, data containing sensitive or potentially identifying information should be stored separately from data that does not. However, you should apply the same organizational principles to both.
Things to Think About
- There may or may not be standard organizational schemes that fit your data. Whenever possible, you should try to adopt the standards of your research community. For assistance in identifying the right organization scheme for your data, contact the LRDS team at [email protected] .
- You should document your file naming and structuring schemes. Such documentation may take the form of a data dictionary or ReadMe file and should enable somebody other than you to understand how your research materials are organized.
- The size and content of your data will determine the degree of flexibility you have about keeping it organized. It is very likely that your organizational scheme will not be perfect. There may be times when you’ll need to rearrange your files.
- Versioning your data may be a good way of keep it organized, as long as it is done in a consistent and descriptive manner. Data_v2.csv may be informative, Data_NewEdits is less so.
- These principles (naming, hierarchies, linking, documentation) also apply within data files. For example, variables names within a file should be consistent and descriptive and you should maintain documentation about what they refer to.
- << Previous: Planning for Data
- Next: Saving Data >>
- Last Updated: Mar 1, 2024 4:25 PM
- URL: https://libguides.chapman.edu/support_your_data

- Data Science
Caltech Bootcamp / Blog / /
Data Collection Methods: A Comprehensive View
- Written by John Terra
- Updated on February 21, 2024
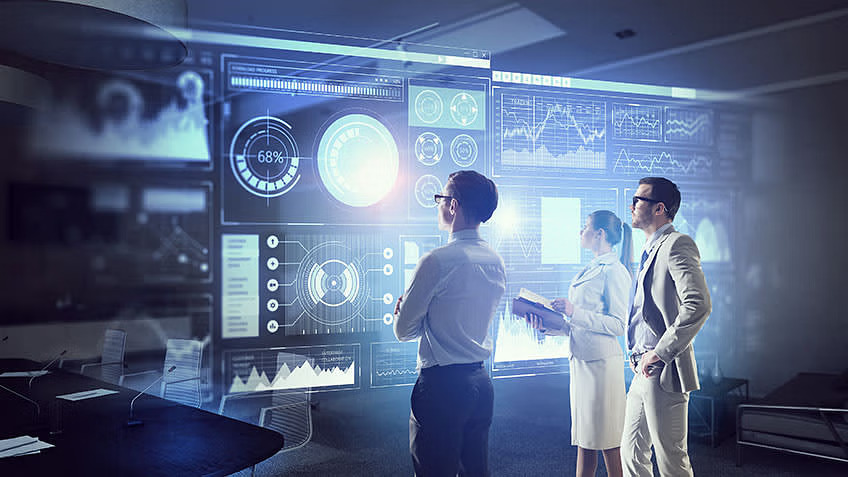
Companies that want to be competitive in today’s digital economy enjoy the benefit of countless reams of data available for market research. In fact, thanks to the advent of big data, there’s a veritable tidal wave of information ready to be put to good use, helping businesses make intelligent decisions and thrive.
But before that data can be used, it must be processed. But before it can be processed, it must be collected, and that’s what we’re here for. This article explores the subject of data collection. We will learn about the types of data collection methods and why they are essential.
We will detail primary and secondary data collection methods and discuss data collection procedures. We’ll also share how you can learn practical skills through online data science training.
But first, let’s get the definition out of the way. What is data collection?
What is Data Collection?
Data collection is the act of collecting, measuring and analyzing different kinds of information using a set of validated standard procedures and techniques. The primary objective of data collection procedures is to gather reliable, information-rich data and analyze it to make critical business decisions. Once the desired data is collected, it undergoes a process of data cleaning and processing to make the information actionable and valuable for businesses.
Your choice of data collection method (or alternately called a data gathering procedure) depends on the research questions you’re working on, the type of data required, and the available time and resources and time. You can categorize data-gathering procedures into two main methods:
- Primary data collection . Primary data is collected via first-hand experiences and does not reference or use the past. The data obtained by primary data collection methods is exceptionally accurate and geared to the research’s motive. They are divided into two categories: quantitative and qualitative. We’ll explore the specifics later.
- Secondary data collection. Secondary data is the information that’s been used in the past. The researcher can obtain data from internal and external sources, including organizational data.
Let’s take a closer look at specific examples of both data collection methods.
Also Read: Why Use Python for Data Science?
The Specific Types of Data Collection Methods
As mentioned, primary data collection methods are split into quantitative and qualitative. We will examine each method’s data collection tools separately. Then, we will discuss secondary data collection methods.
Quantitative Methods
Quantitative techniques for demand forecasting and market research typically use statistical tools. When using these techniques, historical data is used to forecast demand. These primary data-gathering procedures are most often used to make long-term forecasts. Statistical analysis methods are highly reliable because they carry minimal subjectivity.
- Barometric Method. Also called the leading indicators approach, data analysts and researchers employ this method to speculate on future trends based on current developments. When past events are used to predict future events, they are considered leading indicators.
- Smoothing Techniques. Smoothing techniques can be used in cases where the time series lacks significant trends. These techniques eliminate random variation from historical demand and help identify demand levels and patterns to estimate future demand. The most popular methods used in these techniques are the simple moving average and the weighted moving average methods.
- Time Series Analysis. The term “time series” refers to the sequential order of values in a variable, also known as a trend, at equal time intervals. Using patterns, organizations can predict customer demand for their products and services during the projected time.
Qualitative Methods
Qualitative data collection methods are instrumental when no historical information is available, or numbers and mathematical calculations aren’t required. Qualitative research is closely linked to words, emotions, sounds, feelings, colors, and other non-quantifiable elements. These techniques rely on experience, conjecture, intuition, judgment, emotion, etc. Quantitative methods do not provide motives behind the participants’ responses. Additionally, they often don’t reach underrepresented populations and usually involve long data collection periods. Therefore, you get the best results using quantitative and qualitative methods together.
- Questionnaires . Questionnaires are a printed set of either open-ended or closed-ended questions. Respondents must answer based on their experience and knowledge of the issue. A questionnaire is a part of a survey, while the questionnaire’s end goal doesn’t necessarily have to be a survey.
- Surveys. Surveys collect data from target audiences, gathering insights into their opinions, preferences, choices, and feedback on the organization’s goods and services. Most survey software has a wide range of question types, or you can also use a ready-made survey template that saves time and effort. Surveys can be distributed via different channels such as e-mail, offline apps, websites, social media, QR codes, etc.
Once researchers collect the data, survey software generates reports and runs analytics algorithms to uncover hidden insights. Survey dashboards give you statistics relating to completion rates, response rates, filters based on demographics, export and sharing options, etc. Practical business intelligence depends on the synergy between analytics and reporting. Analytics uncovers valuable insights while reporting communicates these findings to the stakeholders.
- Polls. Polls consist of one or more multiple-choice questions. Marketers can turn to polls when they want to take a quick snapshot of the audience’s sentiments. Since polls tend to be short, getting people to respond is more manageable. Like surveys, online polls can be embedded into various media and platforms. Once the respondents answer the question(s), they can be shown how they stand concerning other people’s responses.
- Delphi Technique. The name is a callback to the Oracle of Delphi, a priestess at Apollo’s temple in ancient Greece, renowned for her prophecies. In this method, marketing experts are given the forecast estimates and assumptions made by other industry experts. The first batch of experts may then use the information provided by the other experts to revise and reconsider their estimates and assumptions. The total expert consensus on the demand forecasts creates the final demand forecast.
- Interviews. In this method, interviewers talk to the respondents either face-to-face or by telephone. In the first case, the interviewer asks the interviewee a series of questions in person and notes the responses. The interviewer can opt for a telephone interview if the parties cannot meet in person. This data collection form is practical for use with only a few respondents; repeating the same process with a considerably larger group takes longer.
- Focus Groups. Focus groups are one of the primary examples of qualitative data in education. In focus groups, small groups of people, usually around 8-10 members, discuss the research problem’s common aspects. Each person provides their insights on the issue, and a moderator regulates the discussion. When the discussion ends, the group reaches a consensus.
Also Read: A Beginner’s Guide to the Data Science Process
Secondary Data Collection Methods
Secondary data is the information that’s been used in past situations. Secondary data collection methods can include quantitative and qualitative techniques. In addition, secondary data is easily available, so it’s less time-consuming and expensive than using primary data. However, the authenticity of data gathered with secondary data collection tools cannot be verified.
Internal secondary data sources:
- CRM Software
- Executive summaries
- Financial Statements
- Mission and vision statements
- Organization’s health and safety records
- Sales Reports
External secondary data sources:
- Business journals
- Government reports
- Press releases
The Importance of Data Collection Methods
Data collection methods play a critical part in the research process as they determine the accuracy and quality and accuracy of the collected data. Here’s a sample of some reasons why data collection procedures are so important:
- They determine the quality and accuracy of collected data
- They ensure the data and the research findings are valid, relevant and reliable
- They help reduce bias and increase the sample’s representation
- They are crucial for making informed decisions and arriving at accurate conclusions
- They provide accurate data, which facilitates the achievement of research objectives
Also Read: What Is Data Processing? Definition, Examples, Trends
So, What’s the Difference Between Data Collecting and Data Processing?
Data collection is the first step in the data processing process. Data collection involves gathering information (raw data) from various sources such as interviews, surveys, questionnaires, etc. Data processing describes the steps taken to organize, manipulate and transform the collected data into a useful and meaningful resource. This process may include tasks such as cleaning and validating data, analyzing and summarizing data, and creating visualizations or reports.
So, data collection is just one step in the overall data processing chain of events.
Do You Want to Become a Data Scientist?
If this discussion about data collection and the professionals who conduct it has sparked your enthusiasm for a new career, why not check out this online data science program ?
The Glassdoor.com jobs website shows that data scientists in the United States typically make an average yearly salary of $129,127 plus additional bonuses and cash incentives. So, if you’re interested in a new career or are already in the field but want to upskill or refresh your current skill set, sign up for this bootcamp and prepare to tackle the challenges of today’s big data.
You might also like to read:
Navigating Data Scientist Roles and Responsibilities in Today’s Market
Differences Between Data Scientist and Data Analyst: Complete Explanation
What Is Data Collection? A Guide for Aspiring Data Scientists
A Data Scientist Job Description: The Roles and Responsibilities in 2024
Top Data Science Projects With Source Code to Try
Data Science Bootcamp
- Learning Format:
Online Bootcamp
Leave a comment cancel reply.
Your email address will not be published. Required fields are marked *
Save my name, email, and website in this browser for the next time I comment.
Recommended Articles
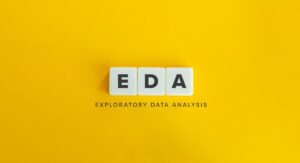
What is Exploratory Data Analysis? Types, Tools, Importance, etc.
This article highlights exploratory data analysis, including its definition, role in data science, types, and overall importance.
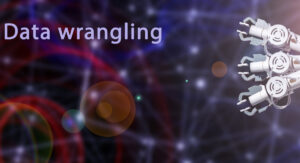
What is Data Wrangling? Importance, Tools, and More
This article explores data wrangling, including its definition, importance, steps, benefits, and tools.
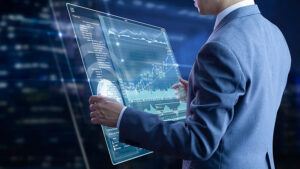
What is Spatial Data Science? Definition, Applications, Careers & More
Do you want to know what spatial data science is? Read this guide to learn its basics, real-world applications, and the exciting career options in this field.
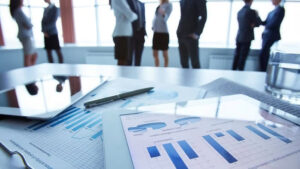
Data Science and Marketing: Transforming Strategies and Enhancing Engagement
Employing data science in marketing is critical for any organization today. This blog explores this intersection of the two disciplines and how professionals and businesses can ensure they have the skills to drive successful digital marketing strategies.
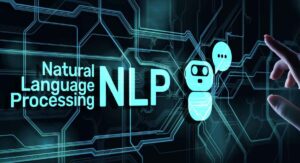
An Introduction to Natural Language Processing in Data Science
Natural language processing may seem straightforward, but there’s a lot going on behind the scenes. This blog explores NLP in data science.
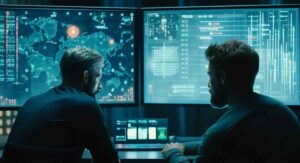
Why Use Python for Data Science?
This article explains why you should use Python for data science tasks, including how it’s done and the benefits.
Learning Format
Program Benefits
- 12+ tools covered, 25+ hands-on projects
- Masterclasses by distinguished Caltech CTME instructors
- Caltech CTME Circle Membership
- Industry-specific training from global experts
- Call us on : 1800-212-7688
Step-by-Step Guide: Data Gathering in Research Projects
- by Willie Wilson
- October 22, 2023
Welcome to our ultimate guide on data gathering in research projects! Whether you’re an aspiring researcher or a seasoned professional, this blog post will equip you with the essential steps to effectively gather data. In this ever-evolving digital age, data has become the cornerstone of decision-making and problem-solving in various fields. So, understanding the process of data gathering is crucial to ensure accurate and reliable results.
In this article, we will delve into the ten key steps involved in data gathering. From formulating research questions to selecting the right data collection methods , we’ll cover everything you need to know to conduct a successful research project. So, grab your notebook and get ready to embark on an exciting journey of data exploration!
Let’s dive right in and discover the step-by-step process of data gathering, enabling you to enhance your research skills and deliver impactful results.
10 Steps to Master Data Gathering
Data gathering is a crucial step in any research or analysis process. It provides the foundation for informed decision-making , insightful analysis, and meaningful insights. Whether you’re a data scientist, a market researcher, or just someone curious about a specific topic, understanding the steps involved in data gathering is essential. So, let’s dive into the 10 steps you need to master to become a data gathering wizard!
Step 1: Define Your Objective
First things first, clearly define your objective. Ask yourself what you’re trying to achieve with the data you gather. Are you looking for trends, patterns, or correlations? Do you want to support a hypothesis or disprove it? Having a clear goal in mind will help you stay focused and ensure that your data gathering efforts are purposeful.
Step 2: Determine Your Data Sources
Once you know what you’re after, it’s time to identify your data sources. Will you be collecting primary data through surveys, interviews, or experiments? Or will you rely on secondary sources like databases, research papers, or official reports? Consider the pros and cons of each source and choose the ones that align best with your objective.
Step 3: Create a Data Collection Plan
Planning is key! Before you start gathering data, create a detailed data collection plan. Outline the key variables you want to measure, determine the sampling technique, and devise a timeline. This plan will serve as your roadmap throughout the data gathering process and ensure that you don’t miss any important steps or variables.
Step 4: Design Your Data Collection Tools
Now that your plan is in place, it’s time to design the tools you’ll use to collect the data. This could be a survey questionnaire, an interview script, or an observation checklist . Remember to keep your questions clear, concise, and unbiased to ensure high-quality data.
Step 5: Pretest Your Tools
Before you launch into full-scale data collection, it’s wise to pretest your tools. This involves trying out your survey questionnaire, interview script, or observation checklist on a small sample of respondents. This step allows you to identify any issues or ambiguities in your tools and make necessary revisions.
Step 6: Collect Your Data
Now comes the exciting part—collecting the actual data! Deploy your data collection tools on your chosen sample and gather the information you need. Be organized, diligent, and ethical in your data collection, ensuring that you respect respondents’ privacy and confidentiality.
Step 7: Clean and Validate Your Data
Raw data can be messy. Before you start analyzing it, you need to clean and validate it. Remove any duplicate entries, correct any errors or inconsistencies, and check for data integrity. This step is critical to ensure the accuracy and reliability of your findings.
Step 8: Analyze Your Data
With clean and validated data in hand, it’s time to analyze! Use statistical techniques , visualization tools, or any other relevant methods to uncover patterns, relationships, and insights within your data. This step is where the true magic happens, so put on your analytical hat and dig deep!
Step 9: Interpret Your Findings
Analyzing data is just the first step; interpreting the findings is where the real value lies. Look for meaningful patterns, draw connections, and uncover insights that align with your objective. Remember to consider the limitations of your data and acknowledge any potential biases.
Step 10: Communicate Your Results
Last but not least, share your findings with the world! Prepare visualizations, reports, or presentations that effectively communicate your results. Make sure your audience understands the key takeaways and implications of your findings. Remember, knowledge is power, but only if it’s effectively shared.
And voila! You’ve now familiarized yourself with the 10 steps to master data gathering. Whether you’re a data enthusiast or a professional in the field, following these steps will set you on the path to success. So go forth, embrace the data, and uncover the hidden treasures within!
FAQ: What are the 10 Steps in Data Gathering
In the world of data-driven decision-making, gathering accurate and reliable data is crucial. Whether you’re conducting market research, academic studies, or simply exploring a topic of interest, the process of data gathering involves various steps. In this FAQ-style guide, we’ll explore the 10 steps of data gathering that will help you collect and analyze data effectively.
What are the Steps in Data Gathering
Identify your research objective: Before diving into data gathering, it’s essential to define the purpose of your research. Determine what information you need to collect and how it will contribute to your overall goal.
Create a research plan: Develop a detailed plan outlining the methods and strategies you’ll use to gather data. Consider factors such as time constraints, available resources, and potential obstacles.
Choose your data collection method: There are various methods to collect data, including surveys, interviews, observations, and experiments. Select a method or combination of methods that align with your research objective and provide the most accurate and relevant data.
Design your data collection tool: Once you’ve chosen your data collection method, design the tools you’ll use to gather information. This may include developing survey questionnaires, interview guides, or observation protocols.
Collect your data: Now it’s time to put your plan into action and start gathering data. Ensure proper training for data collectors, maintain accurate records, and adhere to ethical guidelines if applicable.
Clean and organize your data: After collecting the data, it’s essential to clean and organize it to ensure accuracy and ease of analysis. Remove any inconsistencies, irrelevant information, or duplicate entries. Use software tools such as spreadsheets or statistical software to manage your data effectively.
Analyze your data: With the cleaned and organized data, begin analyzing it to uncover patterns, trends, and insights. Utilize statistical techniques and visualizations to make sense of your data and draw meaningful conclusions .
Interpret your findings: Once you’ve analyzed the data, interpret the results in the context of your research objective. Look for connections, relationships, and implications that can inform your decision-making process.
Draw conclusions and make recommendations: Based on your analysis and interpretation, draw conclusions about your research question and provide recommendations for further action or future studies.
Communicate your findings: Finally, present your findings in a clear and concise manner. This could be through a research report, presentation, or infographic. Consider the appropriate format for your audience and ensure your communication is engaging and accessible.
Data gathering may seem like a daunting process, but by following these 10 steps, you can navigate it successfully. Remember to stay focused on your research objective, choose the right methods and tools, and analyze your data thoroughly. With proper planning and execution, you’ll gather valuable insights that can inform decision-making and drive meaningful outcomes.
- data gathering
- data sources
- decision-making
- essential steps
- impactful results
- informed decision-making
- insightful analysis
- observation checklist
- research projects
- right data collection methods
Willie Wilson
Which actor has a lazy eye, is light pink ok to wear to a wedding, you may also like, how long does it take for turmeric to lighten skin.
- by Mr. Gilbert Preston
- November 1, 2023
The Ultimate Guide to Understanding Your Sleeping Habits in 2023
- by Brandon Thompson
- October 29, 2023
Are most psychopaths Capricorns?
- by Travis Heath
- October 23, 2023
What is the Oldest Area Code? Unraveling the Telephone Number Mysteries of the Past
- by Donna Gonzalez
- October 27, 2023
Is Dune Set in Our Universe?
- October 21, 2023
What is a Caboose Baby: The Unspoken Challenges of Being the Last Born in the Family
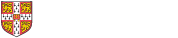
Study at Cambridge
About the university, research at cambridge.
- Undergraduate courses
- Events and open days
- Fees and finance
- Postgraduate courses
- How to apply
- Postgraduate events
- Fees and funding
- International students
- Continuing education
- Executive and professional education
- Courses in education
- How the University and Colleges work
- Term dates and calendars
- Visiting the University
- Annual reports
- Equality and diversity
- A global university
- Public engagement
- Give to Cambridge
- For Cambridge students
- For our researchers
- Business and enterprise
- Colleges & departments
- Email & phone search
- Museums & collections
- Data Management Guide

- Data Management Guide overview
- Creating your data overview
Organising your data
- Accessing your data overview
- Looking after your data
- Sharing your data
- Choosing a software licence
- Electronic Research Notebooks overview
- Support overview
- Resources and support at Cambridge overview
- Data Management Plan Support Service
- DMP Pilot for PhDs
- External support
- Data Repository overview
- Upload your data
- Depositor's checklist
- Guidance on the data submission process
- Data Policies overview
- University of Cambridge Research Data Management Policy Framework overview
- Cambridge data-related policies
- Funders Policies overview
- News overview
- Data Champions overview
- Data Champion list
- Data Champion Community
- Data Champions Cartoons
- Alumni Data Champions
- 2024 Call for Data Champions
- Events overview
- Love Data Week 2024
- Past Events
- Contact Us overview
- Our Governance
- Request a Meeting
- Research Data Management
- Creating your data
- Accessing your data
- Electronic Research Notebooks
- Data Repository
- Data Policies
- Data Champions

Once you create, gather, or start manipulating data and files, they can quickly become disorganised. To save time and prevent errors later on, you and your colleagues should decide how you will name and structure files and folders. Including documentation (or 'metadata') will allow you to add context to your data so that you and others can understand it in the short, medium, and long-term.
Below you can find some guidance on:
- Naming and Organising Files
Documentation and Metadata
Managing references.
- Organising E-mail
Naming and Organising files
Choosing a logical and consistent way to name and organise your files allows you and others to easily locate and use them. Ideally, the best time to think how to name and structure the documents and directories you create is at the start of a project.
Agreeing on a naming convention will help to provide consistency, which will make it easier to find and correctly identify your files, prevent version control problems when working on files collaboratively. Organising your files carefully will save you time and frustration by helping you and your colleagues find what you need when you need it.
How should I organise my files?
Whether you are working on a stand alone computer, or on a networked drive, the need to establish a system that allows you to access your files, avoid duplication, and ensure that your data can be backed up, takes a little planning. A good place to start is to develop a logical folder structure. The following tips should help you develop such a system:
- Use folders - group files within folders so information on a particular topic is located in one place
- Adhere to existing procedures - check for established approaches in your team or department which you can adopt
- Name folders appropriately - name folders after the areas of work to which they relate and not after individual researchers or students. This avoids confusion in shared workspaces if a member of staff leaves, and makes the file system easier to navigate for new people joining the workspace
- Be consistent – when developing a naming scheme for your folders it is important that once you have decided on a method, you stick to it. If you can, try to agree on a naming scheme from the outset of your research project
- Structure folders hierarchically - start with a limited number of folders for the broader topics, and then create more specific folders within these
- Separate ongoing and completed work - as you start to create lots of folders and files, it is a good idea to start thinking about separating your older documents from those you are currently working on
- Try to keep your ‘My Documents’ folder for files you are actively working on , and every month or so, move the files you are no longer working on to a different folder or location, such as a folder on your desktop, a special archive folder or an external hard drive
- Backup – ensure that your files, whether they are on your local drive, or on a network drive, are backed up
- Review records - assess materials regularly or at the end of a project to ensure files are not kept needlessly. Put a reminder in your calendar so you do not forget!
What do I need to consider when creating a file name?
Decide on a file naming convention at the start of your project.
Useful file names are:
- meaningful to you and your colleagues
- allow you to find the file easily.
It is useful if your department/project agrees on the following elements of a file name:
- Vocabulary – choose a standard vocabulary for file names, so that everyone uses a common language
- Punctuation – decide on conventions for if and when to use punctuation symbols, capitals, hyphens and spaces
- Dates – agree on a logical use of dates so that they display chronologically i.e. YYYY-MM-DD
- Order - confirm which element should go first, so that files on the same theme are listed together and can therefore be found easily
- Numbers – specify the amount of digits that will be used in numbering so that files are listed numerically e.g. 01, 002, etc.
How should I name my files, so that I know which document is the most recent version?
Very few documents are drafted by one person in one sitting. More often there will be several people involved in the process and it will occur over an extended period of time. Without proper controls this can quickly lead to confusion as to which version is the most recent. Here is a suggestion of one way to avoid this:
- Use a 'revision' numbering system. Any major changes to a file can be indicated by whole numbers, for example, v01 would be the first version, v02 the second version. Minor changes can be indicated by increasing the decimal figure for example, v01_01 indicates a minor change has been made to the first version, and v03_01 a minor change has been made to the third version.
- When draft documents are sent out for amendments, upon return they should carry additional information to identify the individual who has made the amendments . Example: a file with the name datav01_20130816_SJ indicates that a colleague (SJ) has made amendments to the first version on the 16th August 2013. The lead author would then add those amendments to version v01 and rename the file following the revision numbering system.
- Include a ' version control table ' for each important document, noting changes and their dates alongside the appropriate version number of the document. If helpful, you can include the file names themselves along with (or instead of) the version number.
- Agree who will finish finals and mark them as 'final.'
There are also numerous external resources that will offer you guidance on the best file naming conventions and you can find more information about them here .
To ensure that you understand your own data and that others may find, use and properly cite your data, it helps to add documentation and metadata (data about data) to the documents and datasets you create.

What are 'documentation' and 'metadata'?
The term 'documentation' encompasses all the information necessary to interpret, understand and use a given dataset or set of documents. On this website, we use 'documentation' and 'metadata' (data about data - usually embedded in the data files/documents themselves) interchangeably.
When and how do I include documentation/metadata?
It is a good practice to begin to document your data at the very beginning of your research project and continue to add information as the project progresses. Include procedures for documentation in your data planning.
There are a number of ways you can add documentation to your data:
Embedded documentation
Information about a file or dataset can be included within the data or document itself. For digital datasets, this means that the documentation can sit in separate files (for example text files) or be integrated into the data file(s), as a header or at specified locations in the file. Examples of embedded documentation include:
- code, field and label descriptions
- descriptive headers or summaries
- recording information in the Document Properties function of a file (Microsoft)
You may wish to consider creating a README file which documents the contents, naming convention and structure of files and folders and the project they relate to, as well as a description of file formats and software needed to use the files. You can also document details of licence or any restrictions placed on the data.
Supporting documentation;
This is information in separate files that accompanies data in order to provide context, explanation, or instructions on confidentiality and data use or reuse. Examples of supporting documentation include:
- Working papers or laboratory books
- Questionnaires or interview guides
- Final project reports and publications
- Catalogue metadata
Supporting documentation should be structured, so that it can be used to identify and locate the data via a web browser or web based catalogue. Catalogue metadata is usually structured according to an international standard and associated with the data by repositories or data centres when materials are deposited. Examples of catalogue data are:
- Description
- Affiliation
Digital Curation Centre provides examples of disciplinary-specific metadata, which can be viewed here .
Tools for metadata tracking and data standards
- ISA Tools - metadata tracking tools for life sciences
The open source ISA metadata tracking tools help to manage an increasingly diverse set of life science, environmental and biomedical experiments that employing one or a combination of technologies.
Built around the ‘Investigation’ (the project context), ‘Study’ (a unit of research) and ‘Assay’ (analytical measurement) general-purpose Tabular format, the ISA tools helps you to provide rich description of the experimental metadata (i.e. sample characteristics, technology and measurement types, sample-to-data relationships) so that the resulting data and discoveries are reproducible and reusable.
FAIRsharing - searchable portal of inter-related data standards, databases, and policies for life sciences
FAIRsharing is a curated, searchable portal of inter-related data standards, databases, and policies in the life, environmental, and biomedical sciences.
Projects can last for months or years, and it is easy to lose track of which piece of information came from which source. It can be a challenge to have to reconstruct half of your citations in the scramble at the end of the project! Your future self may not remember everything that seems obvious in the present, so it is important to take clear notes about your sources.
What is 'reference management software'?
Reference management software helps you keep track of your citations as you work, and partially automates the process of constructing bibliographies when it is time to publish. The University of Cambridge also offers support and training on several referencing systems.
Who can help me with reference conventions and formats for my academic discipline or particular project?
Your departmental librarian will be able to help you pick the right format for references and will probably know about some useful search and management tools that you have not used before. Feel free to ask him/her for advice. Additionally, your college librarian is also a very good resource and is there to help. Find your departmental and college librarian on the University's Libraries Directory .
Organising e-mail
Most people now routinely send and receive lots of messages every day and as a result, their inbox can get very quickly overloaded with hundreds of personal and work-related email. Setting aside some time to organise your emails will ensure information can be found quickly and easily, and is stored securely.
Why should I organise my email?
Apart from the obvious frustration and time wasted looking for that email you remember sending to someone last month, email is increasingly used to store important documents and data, often with information related to the attachments within the email itself. Without the proper controls in place they can often be deleted by mistake. It is also important to remember that your work email comes under The Data Protection Act 2018 and the Freedom of Information Act 2000 , so your emails are potentially open to scrutiny.
What are the first steps to organising my email?
If your emails have got out of control there are a number of immediate steps you can take to control the problem:
- Archive your old emails . If you have hundreds of emails hanging around from more than a month ago, move them into a new folder called something like "Archive". You can always come back to these at a later date.
- Now go through your remaining inbox email by email. If an email is useless, delete it. If not, ask yourself: is it "active" - is there a specific action you, or someone else, need to take, or do you just vaguely think it is worth keeping? If the latter, move it to the archive.
How can I ensure my emails remain organised?
Here are some general tips to ensure your email remains organised in the long term:
- Delete emails you do not need. Remove any trivial or old messages from your inbox and sent items on a regular (ideally daily) basis.
- Use folders to store messages. Establish a structured file directory by subject, activity or project.
- Separate personal emails. Set up a separate folder for these. Ideally, you should not receive any personal emails to your work email account.
- Limit the use of attachments. Use alternative and more secure methods to exchange data where possible (see ‘data sharing’ for options). If attachments are used, exercise version control and save important attachments to other places, such as a network drive.
Related links
- Cambridge Libraries directory
- Discipline-specific metadata
- BioSharing - searchable portal of inter-related data standards, databases, and policies for life sciences
About this site :
This site is managed by the Research Data Team.
If you have any questions about this site, please e-mail us directly
The project is a joint initiative of Cambridge University Library and the Research Operations Office .
Privacy policy
© 2024 University of Cambridge
- Contact the University
- Accessibility
- Freedom of information
- Privacy policy and cookies
- Statement on Modern Slavery
- Terms and conditions
- University A-Z
- Undergraduate
- Postgraduate
- Research news
- About research at Cambridge
- Spotlight on...
Have a language expert improve your writing
Run a free plagiarism check in 10 minutes, automatically generate references for free.
- Knowledge Base
- Methodology
- Data Collection Methods | Step-by-Step Guide & Examples
Data Collection Methods | Step-by-Step Guide & Examples
Published on 4 May 2022 by Pritha Bhandari .
Data collection is a systematic process of gathering observations or measurements. Whether you are performing research for business, governmental, or academic purposes, data collection allows you to gain first-hand knowledge and original insights into your research problem .
While methods and aims may differ between fields, the overall process of data collection remains largely the same. Before you begin collecting data, you need to consider:
- The aim of the research
- The type of data that you will collect
- The methods and procedures you will use to collect, store, and process the data
To collect high-quality data that is relevant to your purposes, follow these four steps.
Table of contents
Step 1: define the aim of your research, step 2: choose your data collection method, step 3: plan your data collection procedures, step 4: collect the data, frequently asked questions about data collection.
Before you start the process of data collection, you need to identify exactly what you want to achieve. You can start by writing a problem statement : what is the practical or scientific issue that you want to address, and why does it matter?
Next, formulate one or more research questions that precisely define what you want to find out. Depending on your research questions, you might need to collect quantitative or qualitative data :
- Quantitative data is expressed in numbers and graphs and is analysed through statistical methods .
- Qualitative data is expressed in words and analysed through interpretations and categorisations.
If your aim is to test a hypothesis , measure something precisely, or gain large-scale statistical insights, collect quantitative data. If your aim is to explore ideas, understand experiences, or gain detailed insights into a specific context, collect qualitative data.
If you have several aims, you can use a mixed methods approach that collects both types of data.
- Your first aim is to assess whether there are significant differences in perceptions of managers across different departments and office locations.
- Your second aim is to gather meaningful feedback from employees to explore new ideas for how managers can improve.
Prevent plagiarism, run a free check.
Based on the data you want to collect, decide which method is best suited for your research.
- Experimental research is primarily a quantitative method.
- Interviews , focus groups , and ethnographies are qualitative methods.
- Surveys , observations, archival research, and secondary data collection can be quantitative or qualitative methods.
Carefully consider what method you will use to gather data that helps you directly answer your research questions.
When you know which method(s) you are using, you need to plan exactly how you will implement them. What procedures will you follow to make accurate observations or measurements of the variables you are interested in?
For instance, if you’re conducting surveys or interviews, decide what form the questions will take; if you’re conducting an experiment, make decisions about your experimental design .
Operationalisation
Sometimes your variables can be measured directly: for example, you can collect data on the average age of employees simply by asking for dates of birth. However, often you’ll be interested in collecting data on more abstract concepts or variables that can’t be directly observed.
Operationalisation means turning abstract conceptual ideas into measurable observations. When planning how you will collect data, you need to translate the conceptual definition of what you want to study into the operational definition of what you will actually measure.
- You ask managers to rate their own leadership skills on 5-point scales assessing the ability to delegate, decisiveness, and dependability.
- You ask their direct employees to provide anonymous feedback on the managers regarding the same topics.
You may need to develop a sampling plan to obtain data systematically. This involves defining a population , the group you want to draw conclusions about, and a sample, the group you will actually collect data from.
Your sampling method will determine how you recruit participants or obtain measurements for your study. To decide on a sampling method you will need to consider factors like the required sample size, accessibility of the sample, and time frame of the data collection.
Standardising procedures
If multiple researchers are involved, write a detailed manual to standardise data collection procedures in your study.
This means laying out specific step-by-step instructions so that everyone in your research team collects data in a consistent way – for example, by conducting experiments under the same conditions and using objective criteria to record and categorise observations.
This helps ensure the reliability of your data, and you can also use it to replicate the study in the future.
Creating a data management plan
Before beginning data collection, you should also decide how you will organise and store your data.
- If you are collecting data from people, you will likely need to anonymise and safeguard the data to prevent leaks of sensitive information (e.g. names or identity numbers).
- If you are collecting data via interviews or pencil-and-paper formats, you will need to perform transcriptions or data entry in systematic ways to minimise distortion.
- You can prevent loss of data by having an organisation system that is routinely backed up.
Finally, you can implement your chosen methods to measure or observe the variables you are interested in.
The closed-ended questions ask participants to rate their manager’s leadership skills on scales from 1 to 5. The data produced is numerical and can be statistically analysed for averages and patterns.
To ensure that high-quality data is recorded in a systematic way, here are some best practices:
- Record all relevant information as and when you obtain data. For example, note down whether or how lab equipment is recalibrated during an experimental study.
- Double-check manual data entry for errors.
- If you collect quantitative data, you can assess the reliability and validity to get an indication of your data quality.
Data collection is the systematic process by which observations or measurements are gathered in research. It is used in many different contexts by academics, governments, businesses, and other organisations.
When conducting research, collecting original data has significant advantages:
- You can tailor data collection to your specific research aims (e.g., understanding the needs of your consumers or user testing your website).
- You can control and standardise the process for high reliability and validity (e.g., choosing appropriate measurements and sampling methods ).
However, there are also some drawbacks: data collection can be time-consuming, labour-intensive, and expensive. In some cases, it’s more efficient to use secondary data that has already been collected by someone else, but the data might be less reliable.
Quantitative research deals with numbers and statistics, while qualitative research deals with words and meanings.
Quantitative methods allow you to test a hypothesis by systematically collecting and analysing data, while qualitative methods allow you to explore ideas and experiences in depth.
Reliability and validity are both about how well a method measures something:
- Reliability refers to the consistency of a measure (whether the results can be reproduced under the same conditions).
- Validity refers to the accuracy of a measure (whether the results really do represent what they are supposed to measure).
If you are doing experimental research , you also have to consider the internal and external validity of your experiment.
In mixed methods research , you use both qualitative and quantitative data collection and analysis methods to answer your research question .
Operationalisation means turning abstract conceptual ideas into measurable observations.
For example, the concept of social anxiety isn’t directly observable, but it can be operationally defined in terms of self-rating scores, behavioural avoidance of crowded places, or physical anxiety symptoms in social situations.
Before collecting data , it’s important to consider how you will operationalise the variables that you want to measure.
Cite this Scribbr article
If you want to cite this source, you can copy and paste the citation or click the ‘Cite this Scribbr article’ button to automatically add the citation to our free Reference Generator.
Bhandari, P. (2022, May 04). Data Collection Methods | Step-by-Step Guide & Examples. Scribbr. Retrieved 31 May 2024, from https://www.scribbr.co.uk/research-methods/data-collection-guide/
Is this article helpful?
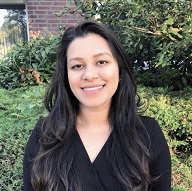
Pritha Bhandari
Other students also liked, qualitative vs quantitative research | examples & methods, triangulation in research | guide, types, examples, what is a conceptual framework | tips & examples.
Put your best data forward
Organizing data.
Organizing data involves ensuring that you can find your data and other research materials (including documentation, code, and physical samples) when you need to, and ensuring that data and materials that go together are connected in a meaningful way.
ORGANIZING: HOW DO YOU ORGANIZE YOUR RESEARCH DATA?
I don’t follow a consistent approach for keeping my data organized, so it often takes time to find things.
I have an approach for organizing my data, but I only put it into action after my project is complete.
I have an approach for organizing my data that I implement prospectively, but it not necessarily standardized.
I organize my data to the so that others can navigate, understand, and use it without me being present.
What does it mean to organize data?
Organizing data means arranging your data and other research materials so they can be found—by yourself and by others—as needed. Here are four factors to consider when organizing data. Remember: you can’t use data you can’t find.
Data should be labeled using a consistent and descriptive file naming system. Your system should allow you to immediately and uniquely identify the contents of your files.
Data should be organized with a consistent and easy to navigate file structure. Maintaining such a structure can help reduce the risk of data loss and unnecessary replication.
CONNECTIONS
Connections give context. Data and other materials should be organized in a manner that emphasizes the links between them. This may refer to different versions of the same file or different files related to the same aim or project.
DOCUMENTATION
You should document how you organize your data and other research materials and refer back to and update your documentation often. When thinking through how to organize your files, make sure you also consider how you include all of the related description and documentation (e.g. notes, data dictionaries, metadata).
Requirements and how to meet then
There are specific requirements about how certain types of data should be organized. Under most circumstances, data containing sensitive or potentially identifying information should be stored separately from data that does not. However, whenever possible, you should apply the same organizational principles to both.
Things to think about
- You should document your file naming and structuring schemes. Such documentation may take the form of a data dictionary or ReadMe file and should enable somebody other than you to understand how your research materials are organized.
- The size and content of your data will determine the degree of flexibility you have about keeping it organized. It is very likely that your organizational scheme will not be perfect. There may be times when you’ll need to rearrange your files.
- Versioning your data may be a good way to keep it organized, as long as it is done in a consistent and descriptive manner. Data_v2.csv may be informative, Data_NewEdits is less so.
- These principles (naming, hierarchies, linking, and documentation) also apply within data files. For example, variable names within a file should be consistent and descriptive. You should maintain documentation about what they refer to.
DOWNLOAD PDF VERSIONS
American Society for Microbiology
- Sparking Joy with Research: Organizing Your Data
Sparking Joy With Research: Organizing Your Data
Feb. 6, 2019
- Start with having different folders for different projects such as “Greenhouse Phytoremediation Study” and “Arid Land Soil Microbiome Project.”
- Divide these major project folders into specific folders for different data types, for example, “Soil Chemical Analyses” and “Microbial Molecular Data.”
- When naming your folders and data files, use names that are human-readable, descriptive and include details about the data set, even if it’s in abbreviated manner. Titles should not be too long and should be easily identified and imported into data analytics tools. Also, ensure that there are no blanks in the file names. Instead, use underscores to separate elements in the title. For example, “Year1_Site1_PlantData_121118”, this file contains plant metrics data for Site 1 from Year 1 and the survey was conducted on December 11, 2018.
- Include a .txt file in each folder describing the naming scheme for that folder and try to adhere to the scheme for all the files in the folder.
- One of the easiest ways of keeping your field and lab data is in spreadsheets. Ensure that the first row is never empty and reserve it for descriptive headers. Each column should contain data in the same format (either numerical or text). Each row should be a record of a sample type or an individual response.
- Spreadsheets should be saved in nonproprietary software formats such as csv or txt. Keeping data in proprietary software formats can result in incompatible files when the software is updated. As a result, data can be lost due to inaccessibility.

- Undergraduate Student
- Graduate Student
- Management Skills
- Professional Development
Author: ASM Careers

ASM Microbe 2024 Registration Now Open!
Discover asm membership, get published in an asm journal.

- Numeracy Skills
7 Things That Can Help You Improve Your Data Collection Skills
Search SkillsYouNeed:
Digital Skills:
- Glossary of Digital Terms
- Getting Online
- Apps, Website and Widgets
- Online Search Tips | How to Search
- Protecting Yourself in the Digital World
- Internet Browsing and Privacy
- Back-up and Storage Solutions
- Online Shopping and Payments
- Understanding and Interpreting Online Product Reviews
- Online Banking and Other Financial Services
- Understanding Digital Currencies and Cryptocurrencies
- Travel and Buying Holidays Online
- Online Education and Learning
- Assessing Internet Information
- Understanding Large Language Models (LLMs)
- Managing Your Online Presence
- Managing Your Digital Legacy
- Working with Others Remotely: Building and Maintaining Relationships
- The Importance of Digital Skills in the Modern Workplace
- Essential Digital Skills for Students
Subscribe to our FREE newsletter and start improving your life in just 5 minutes a day.
You'll get our 5 free 'One Minute Life Skills' and our weekly newsletter.
We'll never share your email address and you can unsubscribe at any time.
Analytics and data science are becoming extremely relevant in government and corporate decision-making.
It is critical to understand the fundamentals of research and information in order to know how companies make important business decisions.
An important part of data science is data collection. And let’s see why collecting the right data has become more important than ever in the current era.
The quantity of data available to businesses will rise significantly as they incorporate more technology. However, not all of your available data is meaningful and beneficial.
As you gather more information, it will become more difficult to process and analyze. To avoid problems, make sure you incorporate a comprehensive data collection framework.
The secret to successfully using the statistics available to any company is a firm base – in this scenario, the method you use to collect data.
By having the right structure in order, you'll recognise what stats to gather and quantify as well as how essential it is. You can then more efficiently interpret and analyze it to make better decisions.
Whether you aim to roll out a fresh data collection framework or enhance the manner in which you presently collect data, here are seven pointers to consider:
7 Points to consider if you aim to boost your data collection prowess
Here's an in-depth overview of the top seven tips for data collection skills improvement so that your company is able to compete and stay afloat in this tough data-driven market.
1. Strategize, filter, and set objectives

The first suggestion for improvising your data collection abilities is to develop a methodological approach.
Have precise strategies for data collection, analysis, categorization, and management. It has to be a consistent framework that addresses all phases of the data collected.
Again, firms need a large amount of information, and you must be clear as to which statistics you require in your research. Hence, filter out the channels from where you receive data.
The information — which can come via customer responses and input or through your customer relationship management team — can all serve as relevant data points according to your end business goals.
Finally, for your data to serve its purpose, you should have clear objectives in terms of what you wish to learn from the data analysis.
Different companies and brands may have different goals. You should establish a defined set of goals so that you gather the appropriate data and conduct the most favorable analysis for your firm.
2. Consider the importance of customer and behavior-related data
Frequently, the most critical data you require relates to your consumers. Hence, identify key communications with customers.
For instance, if you have an ecommerce website, you'll wish to know from where your clients visit your site, what they browse, what they include in their basket, and what they eventually buy.
You can search for metrics plus data collection methodologies pertaining to these actions after first defining key interactions to monitor.
This helps make it simpler for you to keep track of the most important information.
Again, don't just concentrate on consumers who have made purchases or abided by your entire business chain.
Consider what behavior could result in information that is critical to the organization.
To continue with the ecommerce website example, this data could also include how much further down the webpage individuals navigate, the number of pages they check while searching for particular product categories, the time they spend on your site, and at what point they leave.
This type of data collection and analysis can become a great indicator of what processes are functioning well and which areas need improvement.
Once you've decided what information you would like to obtain, think about how much it will cost to gather that data as every extra data point, as well as survey questions, will increase the cost.
3. Use effective data collection tools

Forms and questionnaires are among the most popular data gathering methods, owing to how simple, customizable and effective they are.
For example, medical forms enable you to easily and securely collect data from a massive user base — in this case, patients in a hospital. Since the information is organized by default, this sampling technique significantly enhances data accuracy.
If properly designed, such a framework can be used to obtain answers to closed-ended questions — generating a considerable amount of data and leads.
Another method of collecting data that is used to collect critical info is the interview. It can be done in person, over the phone, or via web chat.
In interview sessions, you can pose open-ended questions. Even here, you should be conscious about the questions being asked and must not compromise on the efficiency.
Records and document-based data collection gathers information by using existing information. This technique is used to identify monetary and attendance numbers, for example.
Finally, survey is a data collection approach whose end goal is to obtain collective answers instead of individual opinions.
You can pose open-ended queries such as "What was your preferred thing about this software?" or similar ones. A shared viewpoint lends an overall understanding of how customers perceive your offerings in the market.
4. Master the art of mobile data collection
While documented data gathering is a time-honored method, mobile-based techniques cannot be ignored in the present era. They are quick and efficient, and they enable you to obtain a wide range of data at a minimal price.
With today's flood of low-cost smartphones, there hardly exists any reason not to opt for mobile-based information collection.
There are three kinds of mobile data gathering.
The first one is IVRS (interactive voice response technology), that will place a call to your interviewee and ask him or her pre-recorded queries.
Second is SMS collection of data that will share a text with your respondent, after which he or she will be capable of answering text queries on his or her phone.
Third, using mobile applications, ground surveyors can effectively enter data into an immersive questionnaire while speaking with each participant.
Also, every tool has its own benefits and drawbacks, so choose the best one for your questionnaire and people surveyed.
5. Identify key metrics and data sources
The method you use to gather information may differ depending on how you intend to quantify it.
This encompasses numerous metrics you employ to specify the successes and failures of promotional campaigns, sales efforts, and also how visitors are tracked.
Make a note of which metrics your company currently uses, because those will frequently steer you in the direction of the relevant information you'll need to gather.
Again, there are several redundancies in collection of data in many businesses. A CMS (content management system), for instance, will frequently also have multiple identical data sets as Web analytics.
A POS (point of sale) might very well have similar data sets as your inventory system. As a result, determine which systems will provide you which data -- to avoid repetitions and wasting resources.
Many companies use data from across multiple channel to calculate a single key metric. To make sure that you obtain the correct data, you must classify these sources and verify that these are compliant with your data capturing system.
If they're not, you might face issues and even make poor decisions because of flawed data, which can also cost your company money.
6. Identify who will review your data
When you incorporate data gathering processes, as well as tools and data analysis methods, you will almost certainly begin to generate reports based on this information.
As a result, it's a smart option to decide who will analyze these findings and what will be the most valuable knowledge for them.
These details will differ depending on context, so be sure to specify the data they believe are essential.
For best results, take into account who would be reviewing your data reports and which categories of relevant data must be captured so as to generate them.
7. Finalize a frequency for data capture and analysis
This step can be difficult to master, particularly if you operate in a volatile market or if your company is constantly changing.
Your best option is to consider when you anticipate requiring data.
For instance, if you are in charge of submitting a quarterly sales forecast, it may be a smart option to obtain data at least each week so that you have enough material to create a final report toward the conclusion of the quarter.
You must also consider who will receive the findings and how lengthy various campaigns or commercial transactions will be.
Each company will have a different frequency, so choose something that truly works for you.
We hope you have gathered a deeper insight regarding the different techniques and skills, vital to improve your data collection skills.
We are sure that employing these tips will aid you in your business. You have also learned about various methods used in sampling procedures, which can assist you if you concentrate on and strengthen your weak spots.
Continue to: Surveys and Survey Design Quantitative and Qualitative Research Methods
See also: Navigating the Data Deluge - Tools That Transform Analysis into Insight Statistical Analysis: Understanding Statistical Distributions 10 Data Analyst Skills You Need to Get Hired
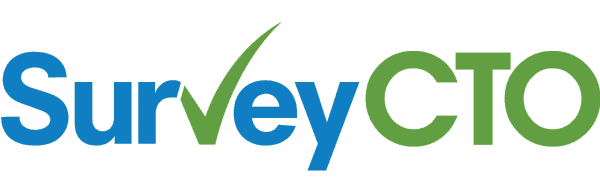
A Guide to Data Collection: Methods, Process, and Tools
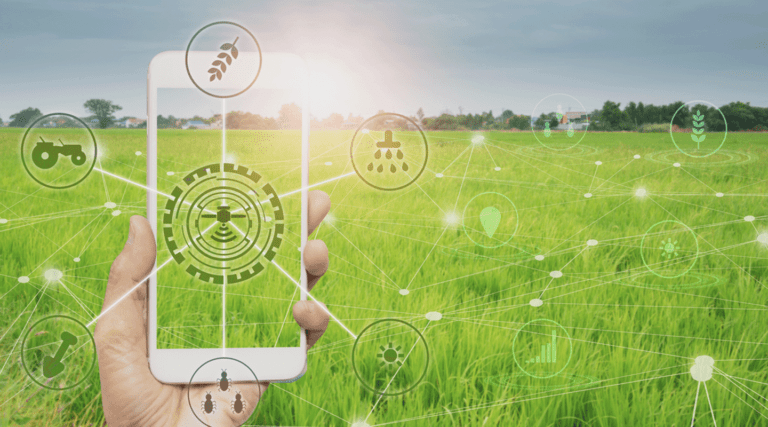
Whether your field is development economics, international development, the nonprofit sector, or myriad other industries, effective data collection is essential. It informs decision-making and increases your organization’s impact. However, the process of data collection can be complex and challenging. If you’re in the beginning stages of creating a data collection process, this guide is for you. It outlines tested methods, efficient procedures, and effective tools to help you improve your data collection activities and outcomes. At SurveyCTO, we’ve used our years of experience and expertise to build a robust, secure, and scalable mobile data collection platform. It’s trusted by respected institutions like The World Bank, J-PAL, Oxfam, and the Gates Foundation, and it’s changed the way many organizations collect and use data. With this guide, we want to share what we know and help you get ready to take the first step in your data collection journey.
Main takeaways from this guide
- Before starting the data collection process, define your goals and identify data sources, which can be primary (first-hand research) or secondary (existing resources).
- Your data collection method should align with your goals, resources, and the nature of the data needed. Surveys, interviews, observations, focus groups, and forms are common data collection methods.
- Sampling involves selecting a representative group from a larger population. Choosing the right sampling method to gather representative and relevant data is crucial.
- Crafting effective data collection instruments like surveys and questionnaires is key. Instruments should undergo rigorous testing for reliability and accuracy.
- Data collection is an ongoing, iterative process that demands real-time monitoring and adjustments to ensure high-quality, reliable results.
- After data collection, data should be cleaned to eliminate errors and organized for efficient analysis. The data collection journey further extends into data analysis, where patterns and useful information that can inform decision-making are discovered.
- Common challenges in data collection include data quality and consistency issues, data security concerns, and limitations with offline surveys . Employing robust data validation processes, implementing strong security protocols, and using offline-enabled data collection tools can help overcome these challenges.
- Data collection, entry, and management tools and data analysis, visualization, reporting, and workflow tools can streamline the data collection process, improve data quality, and facilitate data analysis.
What is data collection?
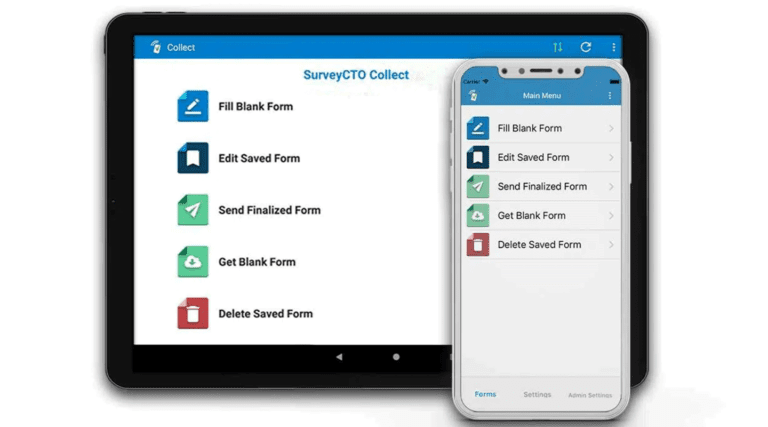
The traditional definition of data collection might lead us to think of gathering information through surveys, observations, or interviews. However, the modern-age definition of data collection extends beyond conducting surveys and observations. It encompasses the systematic gathering and recording of any kind of information through digital or manual methods. Data collection can be as routine as a doctor logging a patient’s information into an electronic medical record system during each clinic visit, or as specific as keeping a record of mosquito nets delivered to a rural household.
Getting started with data collection
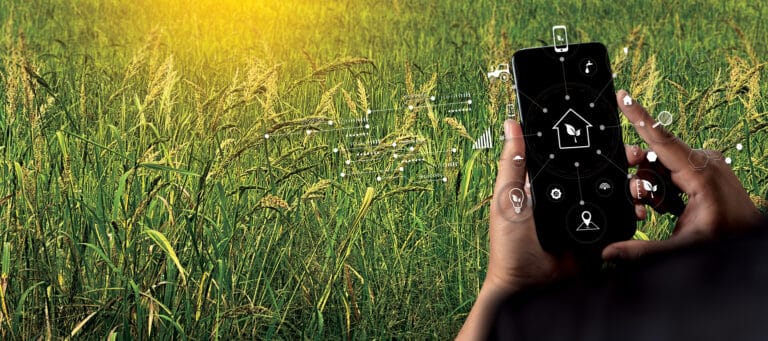
Before starting your data collection process, you must clearly understand what you aim to achieve and how you’ll get there. Below are some actionable steps to help you get started.
1. Define your goals
Defining your goals is a crucial first step. Engage relevant stakeholders and team members in an iterative and collaborative process to establish clear goals. It’s important that projects start with the identification of key questions and desired outcomes to ensure you focus your efforts on gathering the right information.
Start by understanding the purpose of your project– what problem are you trying to solve, or what change do you want to bring about? Think about your project’s potential outcomes and obstacles and try to anticipate what kind of data would be useful in these scenarios. Consider who will be using the data you collect and what data would be the most valuable to them. Think about the long-term effects of your project and how you will measure these over time. Lastly, leverage any historical data from previous projects to help you refine key questions that may have been overlooked previously.
Once questions and outcomes are established, your data collection goals may still vary based on the context of your work. To demonstrate, let’s use the example of an international organization working on a healthcare project in a remote area.
- If you’re a researcher , your goal will revolve around collecting primary data to answer specific questions. This could involve designing a survey or conducting interviews to collect first-hand data on patient improvement, disease or illness prevalence, and behavior changes (such as an increase in patients seeking healthcare).
- If you’re part of the monitoring and evaluation ( M&E) team , your goal will revolve around measuring the success of your healthcare project. This could involve collecting primary data through surveys or observations and developing a dashboard to display real-time metrics like the number of patients treated, percentage of reduction in incidences of disease,, and average patient wait times. Your focus would be using this data to implement any needed program changes and ensure your project meets its objectives.
- If you’re part of a field team , your goal will center around the efficient and accurate execution of project plans. You might be responsible for using data collection tools to capture pertinent information in different settings, such as in interviews takendirectly from the sample community or over the phone. The data you collect and manage will directly influence the operational efficiency of the project and assist in achieving the project’s overarching objectives.
2. Identify your data sources
The crucial next step in your research process is determining your data source. Essentially, there are two main data types to choose from: primary and secondary.
- Primary data is the information you collect directly from first-hand engagements. It’s gathered specifically for your research and tailored to your research question. Primary data collection methods can range from surveys and interviews to focus groups and observations. Because you design the data collection process, primary data can offer precise, context-specific information directly related to your research objectives. For example, suppose you are investigating the impact of a new education policy. In that case, primary data might be collected through surveys distributed to teachers or interviews with school administrators dealing directly with the policy’s implementation.
- Secondary data, on the other hand, is derived from resources that already exist. This can include information gathered for other research projects, administrative records, historical documents, statistical databases, and more. While not originally collected for your specific study, secondary data can offer valuable insights and background information that complement your primary data. For instance, continuing with the education policy example, secondary data might involve academic articles about similar policies, government reports on education or previous survey data about teachers’ opinions on educational reforms.
While both types of data have their strengths, this guide will predominantly focus on primary data and the methods to collect it. Primary data is often emphasized in research because it provides fresh, first-hand insights that directly address your research questions. Primary data also allows for more control over the data collection process, ensuring data is relevant, accurate, and up-to-date.
However, secondary data can offer critical context, allow for longitudinal analysis, save time and resources, and provide a comparative framework for interpreting your primary data. It can be a crucial backdrop against which your primary data can be understood and analyzed. While we focus on primary data collection methods in this guide, we encourage you not to overlook the value of incorporating secondary data into your research design where appropriate.
3. Choose your data collection method
When choosing your data collection method, there are many options at your disposal. Data collection is not limited to methods like surveys and interviews. In fact, many of the processes in our daily lives serve the goal of collecting data, from intake forms to automated endpoints, such as payment terminals and mass transit card readers. Let us dive into some common types of data collection methods:
Surveys and Questionnaires
Surveys and questionnaires are tools for gathering information about a group of individuals, typically by asking them predefined questions. They can be used to collect quantitative and qualitative data and be administered in various ways, including online, over the phone, in person (offline), or by mail.
- Advantages : They allow researchers to reach many participants quickly and cost-effectively, making them ideal for large-scale studies. The structured format of questions makes analysis easier.
- Disadvantages : They may not capture complex or nuanced information as participants are limited to predefined response choices. Also, there can be issues with response bias, where participants might provide socially desirable answers rather than honest ones.
Interviews involve a one-on-one conversation between the researcher and the participant. The interviewer asks open-ended questions to gain detailed information about the participant’s thoughts, feelings, experiences, and behaviors.
- Advantages : They allow for an in-depth understanding of the topic at hand. The researcher can adapt the questioning in real time based on the participant’s responses, allowing for more flexibility.
- Disadvantages : They can be time-consuming and resource-intensive, as they require trained interviewers and a significant amount of time for both conducting and analyzing responses. They may also introduce interviewer bias if not conducted carefully, due to how an interviewer presents questions and perceives the respondent, and how the respondent perceives the interviewer.
Observations
Observations involve directly observing and recording behavior or other phenomena as they occur in their natural settings.
- Advantages : Observations can provide valuable contextual information, as researchers can study behavior in the environment where it naturally occurs, reducing the risk of artificiality associated with laboratory settings or self-reported measures.
- Disadvantages : Observational studies may suffer from observer bias, where the observer’s expectations or biases could influence their interpretation of the data. Also, some behaviors might be altered if subjects are aware they are being observed.
Focus Groups
Focus groups are guided discussions among selected individuals to gain information about their views and experiences.
- Advantages : Focus groups allow for interaction among participants, which can generate a diverse range of opinions and ideas. They are good for exploring new topics where there is little pre-existing knowledge.
- Disadvantages : Dominant voices in the group can sway the discussion, potentially silencing less assertive participants. They also require skilled facilitators to moderate the discussion effectively.
Forms are standardized documents with blank fields for collecting data in a systematic manner. They are often used in fields like Customer Relationship Management (CRM) or Electronic Medical Records (EMR) data entry. Surveys may also be referred to as forms.
- Advantages : Forms are versatile, easy to use, and efficient for data collection. They can streamline workflows by standardizing the data entry process.
- Disadvantages : They may not provide in-depth insights as the responses are typically structured and limited. There is also potential for errors in data entry, especially when done manually.
Selecting the right data collection method should be an intentional process, taking into consideration the unique requirements of your project. The method selected should align with your goals, available resources, and the nature of the data you need to collect.
If you aim to collect quantitative data, surveys, questionnaires, and forms can be excellent tools, particularly for large-scale studies. These methods are suited to providing structured responses that can be analyzed statistically, delivering solid numerical data.
However, if you’re looking to uncover a deeper understanding of a subject, qualitative data might be more suitable. In such cases, interviews, observations, and focus groups can provide richer, more nuanced insights. These methods allow you to explore experiences, opinions, and behaviors deeply. Some surveys can also include open-ended questions that provide qualitative data.
The cost of data collection is also an important consideration. If you have budget constraints, in-depth, in-person conversations with every member of your target population may not be practical. In such cases, distributing questionnaires or forms can be a cost-saving approach.
Additional considerations include language barriers and connectivity issues. If your respondents speak different languages, consider translation services or multilingual data collection tools . If your target population resides in areas with limited connectivity and your method will be to collect data using mobile devices, ensure your tool provides offline data collection , which will allow you to carry out your data collection plan without internet connectivity.
4. Determine your sampling method
Now that you’ve established your data collection goals and how you’ll collect your data, the next step is deciding whom to collect your data from. Sampling involves carefully selecting a representative group from a larger population. Choosing the right sampling method is crucial for gathering representative and relevant data that aligns with your data collection goal.
Consider the following guidelines to choose the appropriate sampling method for your research goal and data collection method:
- Understand Your Target Population: Start by conducting thorough research of your target population. Understand who they are, their characteristics, and subgroups within the population.
- Anticipate and Minimize Biases: Anticipate and address potential biases within the target population to help minimize their impact on the data. For example, will your sampling method accurately reflect all ages, gender, cultures, etc., of your target population? Are there barriers to participation for any subgroups? Your sampling method should allow you to capture the most accurate representation of your target population.
- Maintain Cost-Effective Practices: Consider the cost implications of your chosen sampling methods. Some sampling methods will require more resources, time, and effort. Your chosen sampling method should balance the cost factors with the ability to collect your data effectively and accurately.
- Consider Your Project’s Objectives: Tailor the sampling method to meet your specific objectives and constraints, such as M&E teams requiring real-time impact data and researchers needing representative samples for statistical analysis.
By adhering to these guidelines, you can make informed choices when selecting a sampling method, maximizing the quality and relevance of your data collection efforts.
5. Identify and train collectors
Not every data collection use case requires data collectors, but training individuals responsible for data collection becomes crucial in scenarios involving field presence.
The SurveyCTO platform supports both self-response survey modes and surveys that require a human field worker to do in-person interviews. Whether you’re hiring and training data collectors, utilizing an existing team, or training existing field staff, we offer comprehensive guidance and the right tools to ensure effective data collection practices.
Here are some common training approaches for data collectors:
- In-Class Training: Comprehensive sessions covering protocols, survey instruments, and best practices empower data collectors with skills and knowledge.
- Tests and Assessments: Assessments evaluate collectors’ understanding and competence, highlighting areas where additional support is needed.
- Mock Interviews: Simulated interviews refine collectors’ techniques and communication skills.
- Pre-Recorded Training Sessions: Accessible reinforcement and self-paced learning to refresh and stay updated.
Training data collectors is vital for successful data collection techniques. Your training should focus on proper instrument usage and effective interaction with respondents, including communication skills, cultural literacy, and ethical considerations.
Remember, training is an ongoing process. Knowledge gaps and issues may arise in the field, necessitating further training.
Moving Ahead: Iterative Steps in Data Collection
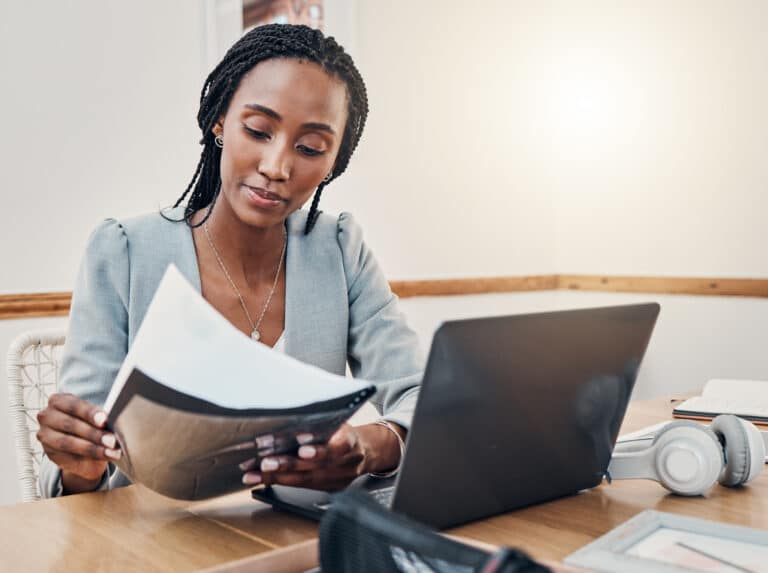
Once you’ve established the preliminary elements of your data collection process, you’re ready to start your data collection journey. In this section, we’ll delve into the specifics of designing and testing your instruments, collecting data, and organizing data while embracing the iterative nature of the data collection process, which requires diligent monitoring and making adjustments when needed.
6. Design and test your instruments
Designing effective data collection instruments like surveys and questionnaires is key. It’s crucial to prioritize respondent consent and privacy to ensure the integrity of your research. Thoughtful design and careful testing of survey questions are essential for optimizing research insights. Other critical considerations are:
- Clear and Unbiased Question Wording: Craft unambiguous, neutral questions free from bias to gather accurate and meaningful data. For example, instead of asking, “Shouldn’t we invest more into renewable energy that will combat the effects of climate change?” ask your question in a neutral way that allows the respondent to voice their thoughts. For example: “What are your thoughts on investing more in renewable energy?”
- Logical Ordering and Appropriate Response Format: Arrange questions logically and choose response formats (such as multiple-choice, Likert scale, or open-ended) that suit the nature of the data you aim to collect.
- Coverage of Relevant Topics: Ensure that your instrument covers all topics pertinent to your data collection goals while respecting cultural and social sensitivities. Make sure your instrument avoids assumptions, stereotypes, and languages or topics that could be considered offensive or taboo in certain contexts. The goal is to avoid marginalizing or offending respondents based on their social or cultural background.
- Collect Only Necessary Data: Design survey instruments that focus solely on gathering the data required for your research objectives, avoiding unnecessary information.
- Language(s) of the Respondent Population: Tailor your instruments to accommodate the languages your target respondents speak, offering translated versions if needed. Similarly, take into account accessibility for respondents who can’t read by offering alternative formats like images in place of text.
- Desired Length of Time for Completion: Respect respondents’ time by designing instruments that can be completed within a reasonable timeframe, balancing thoroughness with engagement. Having a general timeframe for the amount of time needed to complete a response will also help you weed out bad responses. For example, a response that was rushed and completed outside of your response timeframe could indicate a response that needs to be excluded.
- Collecting and Documenting Respondents’ Consent and Privacy: Ensure a robust consent process, transparent data usage communication, and privacy protection throughout data collection.
Perform Cognitive Interviewing
Cognitive interviewing is a method used to refine survey instruments and improve the accuracy of survey responses by evaluating how respondents understand, process, and respond to the instrument’s questions. In practice, cognitive interviewing involves an interview with the respondent, asking them to verbalize their thoughts as they interact with the instrument. By actively probing and observing their responses, you can identify and address ambiguities, ensuring accurate data collection.
Thoughtful question wording, well-organized response options, and logical sequencing enhance comprehension, minimize biases, and ensure accurate data collection. Iterative testing and refinement based on respondent feedback improve the validity, reliability, and actionability of insights obtained.
Put Your Instrument to the Test
Through rigorous testing, you can uncover flaws, ensure reliability, maximize accuracy, and validate your instrument’s performance. This can be achieved by:
- Conducting pilot testing to enhance the reliability and effectiveness of data collection. Administer the instrument, identify difficulties, gather feedback, and assess performance in real-world conditions.
- Making revisions based on pilot testing to enhance clarity, accuracy, usability, and participant satisfaction. Refine questions, instructions, and format for effective data collection.
- Continuously iterating and refining your instrument based on feedback and real-world testing. This ensures reliable, accurate, and audience-aligned methods of data collection. Additionally, this ensures your instrument adapts to changes, incorporates insights, and maintains ongoing effectiveness.
7. Collect your data
Now that you have your well-designed survey, interview questions, observation plan, or form, it’s time to implement it and gather the needed data. Data collection is not a one-and-done deal; it’s an ongoing process that demands attention to detail. Imagine spending weeks collecting data, only to discover later that a significant portion is unusable due to incomplete responses, improper collection methods, or falsified responses. To avoid such setbacks, adopt an iterative approach.
Leverage data collection tools with real-time monitoring to proactively identify outliers and issues. Take immediate action by fine-tuning your instruments, optimizing the data collection process, addressing concerns like additional training, or reevaluating personnel responsible for inaccurate data (for example, a field worker who sits in a coffee shop entering fake responses rather than doing the work of knocking on doors).
SurveyCTO’s Data Explorer was specifically designed to fulfill this requirement, empowering you to monitor incoming data, gain valuable insights, and know where changes may be needed. Embracing this iterative approach ensures ongoing improvement in data collection, resulting in more reliable and precise results.
8. Clean and organize your data
After data collection, the next step is to clean and organize the data to ensure its integrity and usability.
- Data Cleaning: This stage involves sifting through your data to identify and rectify any errors, inconsistencies, or missing values. It’s essential to maintain the accuracy of your data and ensure that it’s reliable for further analysis. Data cleaning can uncover duplicates, outliers, and gaps that could skew your results if left unchecked. With real-time data monitoring , this continuous cleaning process keeps your data precise and current throughout the data collection period. Similarly, review and corrections workflows allow you to monitor the quality of your incoming data.
- Organizing Your Data: Post-cleaning, it’s time to organize your data for efficient analysis and interpretation. Labeling your data using appropriate codes or categorizations can simplify navigation and streamline the extraction of insights. When you use a survey or form, labeling your data is often not necessary because you can design the instrument to collect in the right categories or return the right codes. An organized dataset is easier to manage, analyze, and interpret, ensuring that your collection efforts are not wasted but lead to valuable, actionable insights.
Remember, each stage of the data collection process, from design to cleaning, is iterative and interconnected. By diligently cleaning and organizing your data, you are setting the stage for robust, meaningful analysis that can inform your data-driven decisions and actions.
What happens after data collection?
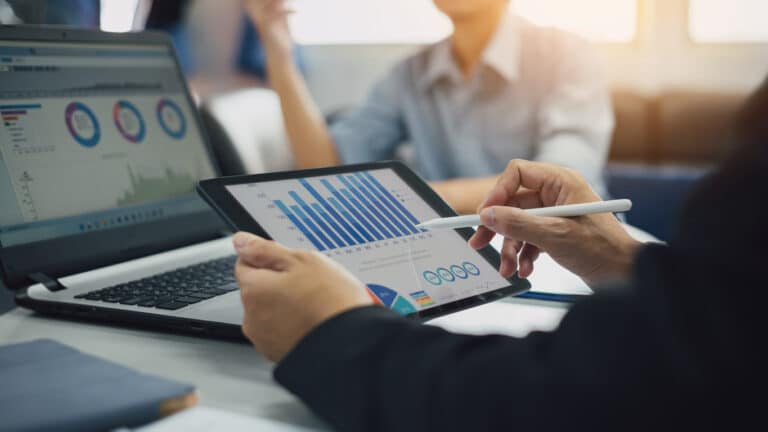
The data collection journey takes us next into data analysis, where you’ll uncover patterns, empowering informed decision-making for researchers, evaluation teams, and field personnel.
Process and Analyze Your Data
Explore data through statistical and qualitative techniques to discover patterns, correlations, and insights during this pivotal stage. It’s about extracting the essence of your data and translating numbers into knowledge. Whether applying descriptive statistics, conducting regression analysis, or using thematic coding for qualitative data, this process drives decision-making and charts the path toward actionable outcomes.
Interpret and Report Your Results
Interpreting and reporting your data brings meaning and context to the numbers. Translating raw data into digestible insights for informed decision-making and effective stakeholder communication is critical.
The approach to interpretation and reporting varies depending on the perspective and role:
- Researchers often lean heavily on statistical methods to identify trends, extract meaningful conclusions, and share their findings in academic circles, contributing to their knowledge pool.
- M&E teams typically produce comprehensive reports, shedding light on the effectiveness and impact of programs. These reports guide internal and sometimes external stakeholders, supporting informed decisions and driving program improvements.
Field teams provide a first-hand perspective. Since they are often the first to see the results of the practical implementation of data, field teams are instrumental in providing immediate feedback loops on project initiatives. Field teams do the work that provides context to help research and M&E teams understand external factors like the local environment, cultural nuances, and logistical challenges that impact data results.
Safely store and handle data
Throughout the data collection process, and after it has been collected, it is vital to follow best practices for storing and handling data to ensure the integrity of your research. While the specifics of how to best store and handle data will depend on your project, here are some important guidelines to keep in mind:
- Use cloud storage to hold your data if possible, since this is safer than storing data on hard drives and keeps it more accessible,
- Periodically back up and purge old data from your system, since it’s safer to not retain data longer than necessary,
- If you use mobile devices to collect and store data, use options for private, internal apps-specific storage if and when possible,
- Restrict access to stored data to only those who need to work with that data.
Further considerations for data safety are discussed below in the section on data security .
Remember to uphold ethical standards in interpreting and reporting your data, regardless of your role. Clear communication, respectful handling of sensitive information, and adhering to confidentiality and privacy rights are all essential to fostering trust, promoting transparency, and bolstering your work’s credibility.
Common Data Collection Challenges
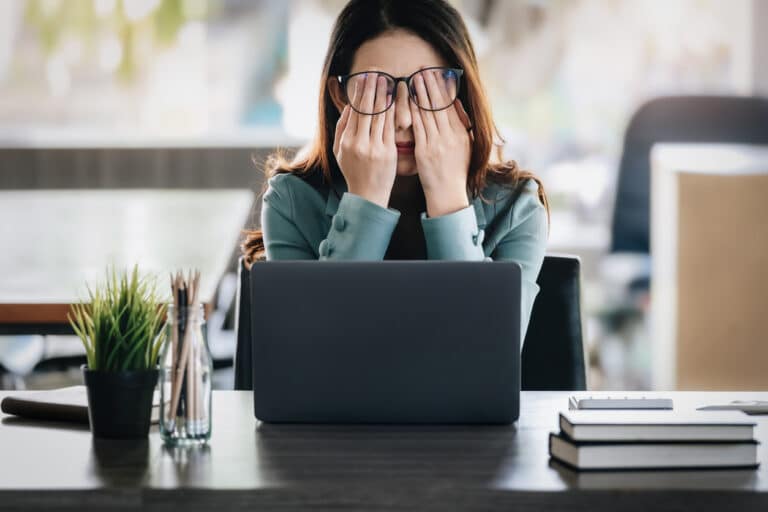
Data collection is vital to data-driven initiatives, but it comes with challenges. Addressing common challenges such as poor data quality, privacy concerns, inadequate sample sizes, and bias is essential to ensure the collected data is reliable, trustworthy, and secure.
In this section, we’ll explore three major challenges: data quality and consistency issues, data security concerns, and limitations with offline data collection , along with strategies to overcome them.
Data Quality and Consistency
Data quality and consistency refer to data accuracy and reliability throughout the collection and analysis process.
Challenges such as incomplete or missing data, data entry errors, measurement errors, and data coding/categorization errors can impact the integrity and usefulness of the data.
To navigate these complexities and maintain high standards, consistency, and integrity in the dataset:
- Implement robust data validation processes,
- Ensure proper training for data entry personnel,
- Employ automated data validation techniques, and
- Conduct regular data quality audits.
Data security
Data security encompasses safeguarding data through ensuring data privacy and confidentiality, securing storage and backup, and controlling data sharing and access.
Challenges include the risk of potential breaches, unauthorized access, and the need to comply with data protection regulations.
To address these setbacks and maintain privacy, trust, and confidence during the data collection process:
- Use encryption and authentication methods,
- Implement robust security protocols,
- Update security measures regularly,
- Provide employee training on data security, and
- Adopt secure cloud storage solutions.
Offline Data Collection
Offline data collection refers to the process of gathering data using modes like mobile device-based computer-assisted personal interviewing (CAPI) when t here is an inconsistent or unreliable internet connection, and the data collection tool being used for CAPI has the functionality to work offline.
Challenges associated with offline data collection include synchronization issues, difficulty transferring data, and compatibility problems between devices, and data collection tools.
To overcome these challenges and enable efficient and reliable offline data collection processes, employ the following strategies:
- Leverage offline-enabled data collection apps or tools that enable you to survey respondents even when there’s no internet connection, and upload data to a central repository at a later time.
- Your data collection plan should include times for periodic data synchronization when connectivity is available,
- Use offline, device-based storage for seamless data transfer and compatibility, and
- Provide clear instructions to field personnel on handling offline data collection scenarios.
Utilizing Technology in Data Collection
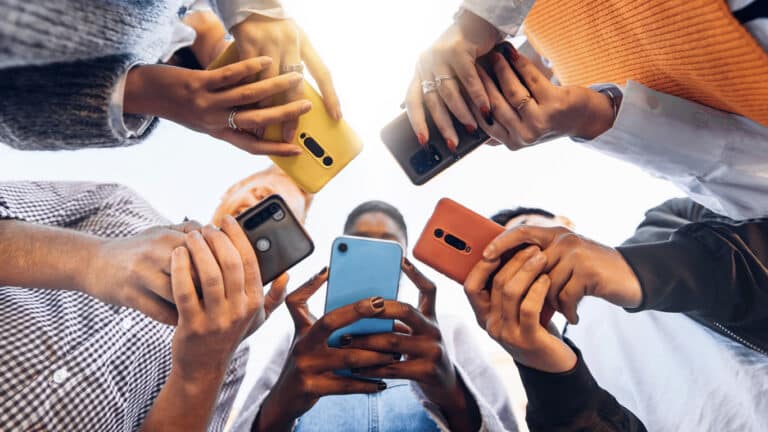
Embracing technology throughout your data collection process can help you overcome many challenges described in the previous section. Data collection tools can streamline your data collection, improve the quality and security of your data, and facilitate the analysis of your data. Let’s look at two broad categories of tools that are essential for data collection:
Data Collection, Entry, & Management Tools
These tools help with data collection, input, and organization. They can range from digital survey platforms to comprehensive database systems, allowing you to gather, enter, and manage your data effectively. They can significantly simplify the data collection process, minimize human error, and offer practical ways to organize and manage large volumes of data. Some of these tools are:
- Microsoft Office
- Google Docs
- SurveyMonkey
- Google Forms
Data Analysis, Visualization, Reporting, & Workflow Tools
These tools assist in processing and interpreting the collected data. They provide a way to visualize data in a user-friendly format, making it easier to identify trends and patterns. These tools can also generate comprehensive reports to share your findings with stakeholders and help manage your workflow efficiently. By automating complex tasks, they can help ensure accuracy and save time. Tools for these purposes include:
- Google sheets
Data collection tools like SurveyCTO often have integrations to help users seamlessly transition from data collection to data analysis, visualization, reporting, and managing workflows.
Master Your Data Collection Process With SurveyCTO
As we bring this guide to a close, you now possess a wealth of knowledge to develop your data collection process. From understanding the significance of setting clear goals to the crucial process of selecting your data collection methods and addressing common challenges, you are equipped to handle the intricate details of this dynamic process.
Remember, you’re not venturing into this complex process alone. At SurveyCTO, we offer not just a tool but an entire support system committed to your success. Beyond troubleshooting support, our success team serves as research advisors and expert partners, ready to provide guidance at every stage of your data collection journey.
With SurveyCTO , you can design flexible surveys in Microsoft Excel or Google Sheets, collect data online and offline with above-industry-standard security, monitor your data in real time, and effortlessly export it for further analysis in any tool of your choice. You also get access to our Data Explorer, which allows you to visualize incoming data at both individual survey and aggregate levels instantly.
In the iterative data collection process, our users tell us that SurveyCTO stands out with its capacity to establish review and correction workflows. It enables you to monitor incoming data and configure automated quality checks to flag error-prone submissions.
Finally, data security is of paramount importance to us. We ensure best-in-class security measures like SOC 2 compliance, end-to-end encryption, single sign-on (SSO), GDPR-compliant setups, customizable user roles, and self-hosting options to keep your data safe.
As you embark on your data collection journey, you can count on SurveyCTO’s experience and expertise to be by your side every step of the way. Our team would be excited and honored to be a part of your research project, offering you the tools and processes to gain informative insights and make effective decisions. Partner with us today and revolutionize the way you collect data.
Better data, better decision making, better world.
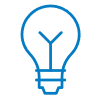
INTEGRATIONS
- Business Essentials
- Leadership & Management
- Credential of Leadership, Impact, and Management in Business (CLIMB)
- Entrepreneurship & Innovation
- Digital Transformation
- Finance & Accounting
- Business in Society
- For Organizations
- Support Portal
- Media Coverage
- Founding Donors
- Leadership Team
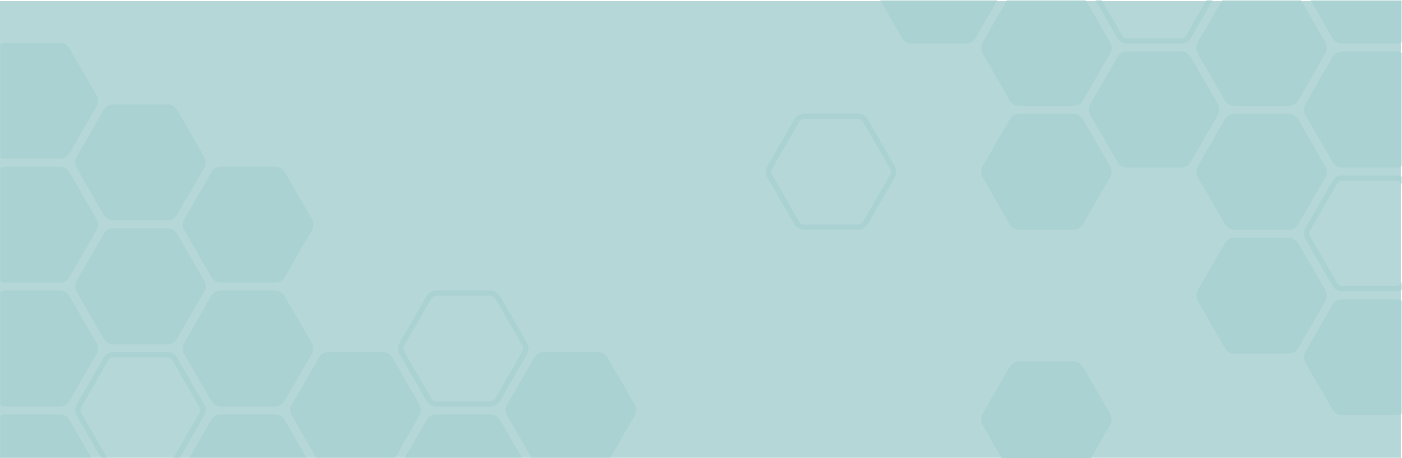
- Harvard Business School →
- HBS Online →
- Business Insights →
Business Insights
Harvard Business School Online's Business Insights Blog provides the career insights you need to achieve your goals and gain confidence in your business skills.
- Career Development
- Communication
- Decision-Making
- Earning Your MBA
- Negotiation
- News & Events
- Productivity
- Staff Spotlight
- Student Profiles
- Work-Life Balance
- AI Essentials for Business
- Alternative Investments
- Business Analytics
- Business Strategy
- Business and Climate Change
- Design Thinking and Innovation
- Digital Marketing Strategy
- Disruptive Strategy
- Economics for Managers
- Entrepreneurship Essentials
- Financial Accounting
- Global Business
- Launching Tech Ventures
- Leadership Principles
- Leadership, Ethics, and Corporate Accountability
- Leading Change and Organizational Renewal
- Leading with Finance
- Management Essentials
- Negotiation Mastery
- Organizational Leadership
- Power and Influence for Positive Impact
- Strategy Execution
- Sustainable Business Strategy
- Sustainable Investing
- Winning with Digital Platforms
7 Data Collection Methods in Business Analytics
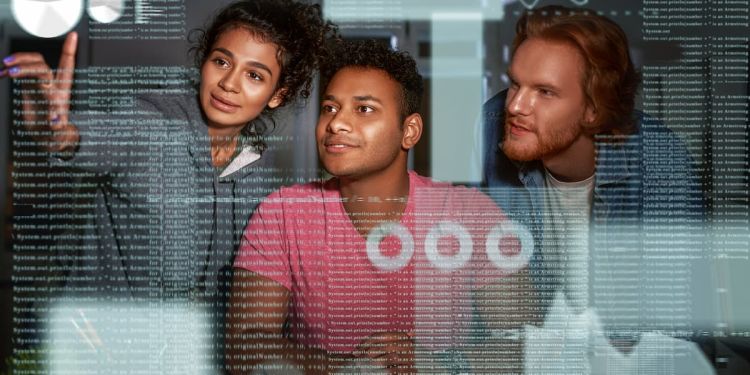
- 02 Dec 2021
Data is being generated at an ever-increasing pace. According to Statista , the total volume of data was 64.2 zettabytes in 2020; it’s predicted to reach 181 zettabytes by 2025. This abundance of data can be overwhelming if you aren’t sure where to start.
So, how do you ensure the data you use is relevant and important to the business problems you aim to solve? After all, a data-driven decision is only as strong as the data it’s based on. One way is to collect data yourself.
Here’s a breakdown of data types, why data collection is important, what to know before you begin collecting, and seven data collection methods to leverage.
Access your free e-book today.
What Is Data Collection?
Data collection is the methodological process of gathering information about a specific subject. It’s crucial to ensure your data is complete during the collection phase and that it’s collected legally and ethically . If not, your analysis won’t be accurate and could have far-reaching consequences.
In general, there are three types of consumer data:
- First-party data , which is collected directly from users by your organization
- Second-party data , which is data shared by another organization about its customers (or its first-party data)
- Third-party data , which is data that’s been aggregated and rented or sold by organizations that don’t have a connection to your company or users
Although there are use cases for second- and third-party data, first-party data (data you’ve collected yourself) is more valuable because you receive information about how your audience behaves, thinks, and feels—all from a trusted source.
Data can be qualitative (meaning contextual in nature) or quantitative (meaning numeric in nature). Many data collection methods apply to either type, but some are better suited to one over the other.
In the data life cycle , data collection is the second step. After data is generated, it must be collected to be of use to your team. After that, it can be processed, stored, managed, analyzed, and visualized to aid in your organization’s decision-making.

Before collecting data, there are several factors you need to define:
- The question you aim to answer
- The data subject(s) you need to collect data from
- The collection timeframe
- The data collection method(s) best suited to your needs
The data collection method you select should be based on the question you want to answer, the type of data you need, your timeframe, and your company’s budget.
The Importance of Data Collection
Collecting data is an integral part of a business’s success; it can enable you to ensure the data’s accuracy, completeness, and relevance to your organization and the issue at hand. The information gathered allows organizations to analyze past strategies and stay informed on what needs to change.
The insights gleaned from data can make you hyperaware of your organization’s efforts and give you actionable steps to improve various strategies—from altering marketing strategies to assessing customer complaints.
Basing decisions on inaccurate data can have far-reaching negative consequences, so it’s important to be able to trust your own data collection procedures and abilities. By ensuring accurate data collection, business professionals can feel secure in their business decisions.
Explore the options in the next section to see which data collection method is the best fit for your company.
7 Data Collection Methods Used in Business Analytics
Surveys are physical or digital questionnaires that gather both qualitative and quantitative data from subjects. One situation in which you might conduct a survey is gathering attendee feedback after an event. This can provide a sense of what attendees enjoyed, what they wish was different, and areas in which you can improve or save money during your next event for a similar audience.
While physical copies of surveys can be sent out to participants, online surveys present the opportunity for distribution at scale. They can also be inexpensive; running a survey can cost nothing if you use a free tool. If you wish to target a specific group of people, partnering with a market research firm to get the survey in front of that demographic may be worth the money.
Something to watch out for when crafting and running surveys is the effect of bias, including:
- Collection bias : It can be easy to accidentally write survey questions with a biased lean. Watch out for this when creating questions to ensure your subjects answer honestly and aren’t swayed by your wording.
- Subject bias : Because your subjects know their responses will be read by you, their answers may be biased toward what seems socially acceptable. For this reason, consider pairing survey data with behavioral data from other collection methods to get the full picture.
Related: 3 Examples of Bad Survey Questions & How to Fix Them
2. Transactional Tracking
Each time your customers make a purchase, tracking that data can allow you to make decisions about targeted marketing efforts and understand your customer base better.
Often, e-commerce and point-of-sale platforms allow you to store data as soon as it’s generated, making this a seamless data collection method that can pay off in the form of customer insights.
3. Interviews and Focus Groups
Interviews and focus groups consist of talking to subjects face-to-face about a specific topic or issue. Interviews tend to be one-on-one, and focus groups are typically made up of several people. You can use both to gather qualitative and quantitative data.
Through interviews and focus groups, you can gather feedback from people in your target audience about new product features. Seeing them interact with your product in real-time and recording their reactions and responses to questions can provide valuable data about which product features to pursue.
As is the case with surveys, these collection methods allow you to ask subjects anything you want about their opinions, motivations, and feelings regarding your product or brand. It also introduces the potential for bias. Aim to craft questions that don’t lead them in one particular direction.
One downside of interviewing and conducting focus groups is they can be time-consuming and expensive. If you plan to conduct them yourself, it can be a lengthy process. To avoid this, you can hire a market research facilitator to organize and conduct interviews on your behalf.
4. Observation
Observing people interacting with your website or product can be useful for data collection because of the candor it offers. If your user experience is confusing or difficult, you can witness it in real-time.
Yet, setting up observation sessions can be difficult. You can use a third-party tool to record users’ journeys through your site or observe a user’s interaction with a beta version of your site or product.
While less accessible than other data collection methods, observations enable you to see firsthand how users interact with your product or site. You can leverage the qualitative and quantitative data gleaned from this to make improvements and double down on points of success.

5. Online Tracking
To gather behavioral data, you can implement pixels and cookies. These are both tools that track users’ online behavior across websites and provide insight into what content they’re interested in and typically engage with.
You can also track users’ behavior on your company’s website, including which parts are of the highest interest, whether users are confused when using it, and how long they spend on product pages. This can enable you to improve the website’s design and help users navigate to their destination.
Inserting a pixel is often free and relatively easy to set up. Implementing cookies may come with a fee but could be worth it for the quality of data you’ll receive. Once pixels and cookies are set, they gather data on their own and don’t need much maintenance, if any.
It’s important to note: Tracking online behavior can have legal and ethical privacy implications. Before tracking users’ online behavior, ensure you’re in compliance with local and industry data privacy standards .
Online forms are beneficial for gathering qualitative data about users, specifically demographic data or contact information. They’re relatively inexpensive and simple to set up, and you can use them to gate content or registrations, such as webinars and email newsletters.
You can then use this data to contact people who may be interested in your product, build out demographic profiles of existing customers, and in remarketing efforts, such as email workflows and content recommendations.
Related: What Is Marketing Analytics?
7. Social Media Monitoring
Monitoring your company’s social media channels for follower engagement is an accessible way to track data about your audience’s interests and motivations. Many social media platforms have analytics built in, but there are also third-party social platforms that give more detailed, organized insights pulled from multiple channels.
You can use data collected from social media to determine which issues are most important to your followers. For instance, you may notice that the number of engagements dramatically increases when your company posts about its sustainability efforts.

Building Your Data Capabilities
Understanding the variety of data collection methods available can help you decide which is best for your timeline, budget, and the question you’re aiming to answer. When stored together and combined, multiple data types collected through different methods can give an informed picture of your subjects and help you make better business decisions.
Do you want to become a data-driven professional? Explore our eight-week Business Analytics course and our three-course Credential of Readiness (CORe) program to deepen your analytical skills and apply them to real-world business problems. Not sure which course is right for you? Download our free flowchart .
This post was updated on October 17, 2022. It was originally published on December 2, 2021.

About the Author
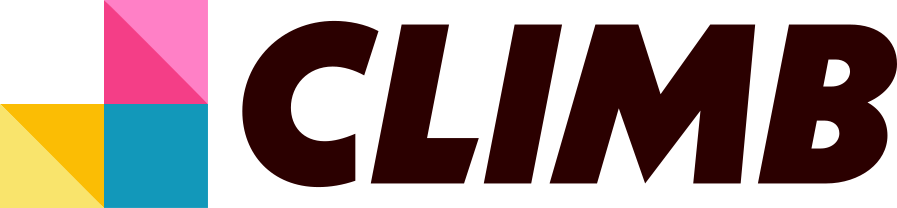
11 Data Collector Skills for Your Career and Resume
Learn about the most important Data Collector skills, how you can utilize them in the workplace, and what to list on your resume.

Data collectors are responsible for gathering information from a variety of sources. This data is then used to help organizations make informed decisions. Data collectors must have strong research and analytical skills to be successful in their role. If you’re interested in becoming a data collector, learning more about the skills required for this position can help you determine if it’s the right career for you.
Time Management
Problem solving, attention to detail, adaptability, organization, communication, independent thinking, flexibility, critical thinking.
Time management is the ability to plan and execute tasks in a timely manner. Data collectors often have multiple responsibilities, so it’s important for them to manage their time well. They may need to track down information from different sources or complete other tasks before they can meet their daily quota of data. Having good time management skills ensures that data collectors are able to complete all of their duties on time.
Problem solving is the ability to identify and resolve issues. Data collectors often use problem-solving skills when they encounter challenges while collecting data, such as if a machine breaks or if they can’t find the right information. It’s also important for data collectors to solve problems that arise during analysis, like if they accidentally input incorrect data or if their results don’t make sense.
Data collectors must be able to accurately record the information they receive from their sources. This skill is important because it ensures that the data you collect will be accurate and useful for your organization’s purposes. Data collectors should also be attentive when entering data into a database so that they can ensure its accuracy.
Data collectors often work with different types of data, in different locations and for different companies. Adaptability is the ability to change your approach based on the situation at hand. For example, if you’re collecting demographic information from a group of people who speak a language other than English, adaptability would be the ability to quickly learn that language so you can continue working.
Data entry is the process of inputting data into a computer system. Data collectors often use software to record and store information, so it’s important for them to know how to enter that data correctly. This skill also helps them ensure they have all the necessary information when entering their data into an analysis program.
Organization is the ability to keep track of your work and files. Data collectors often use organizational skills when creating spreadsheets or other types of data storage. They also need organization when entering their collected data into a computer system, as they may have to create folders for each type of information. Finally, data collectors who are working in teams can benefit from organizational skills because it allows them to delegate tasks effectively and ensure that everyone has what they need to complete their job.
Data collectors must be able to communicate clearly with their supervisors and colleagues. This includes being able to explain the data they’ve collected, how they obtained it and any challenges they faced during the collection process. It also means that they can listen to instructions from their supervisors and respond to questions about their work.
Communication skills are important in all aspects of a data collector’s job, including when interacting with survey respondents or customers. Data collectors should be friendly and respectful when speaking with others so they can get accurate information and maintain good working relationships.
Independent thinking is the ability to make decisions and solve problems on your own. Data collectors often work independently, so it’s important for them to think through their actions and find solutions when challenges arise. For example, if a data collector needs to enter information into an online form but can’t access the internet, they might need to use independent thinking skills to figure out how to complete the task.
Flexibility is the ability to adapt to changing circumstances. Data collectors often need flexibility because their work schedules and locations change frequently. They also may have to adjust their methods of data collection if they find that certain ways aren’t effective or efficient. For example, a data collector who normally uses paper forms might switch to an online survey if it’s more convenient for respondents.
Critical thinking is the ability to analyze a situation and make decisions that lead to positive outcomes. Data collectors use critical thinking skills when they evaluate their work, determine what data is most important and decide how to proceed if they encounter challenges. For example, if a data collector realizes they need more information from an interviewee, they can think critically about how to ask follow-up questions in order to get the needed data.
Data collectors must be precise when recording data. They should ensure that they record the correct information and enter it into a database correctly. This ensures that the data is accurate and can help companies make better business decisions. Data collectors may also need to verify their own work, so accuracy skills are important for ensuring that the data is reliable.
How Can I Learn These Data Collector Skills?
There are a few ways that you can learn the necessary skills to become a data collector. One way is to take a course or class that covers the topics that you need to know. Another way is to read books or articles that cover the topics that you need to know. Finally, you can also learn by doing, by working with a data collector and observing how they do their job.
16 Fitter Skills for Your Career and Resume
16 chief accountant skills for your career and resume, you may also be interested in..., what does a waffle house cook do, 16 internal medicine doctor skills for your career and resume, what does a contract coordinator do, what does a head coach do.

IMAGES
VIDEO
COMMENTS
Data collection requires you to define your research question, choose your data sources, design your data collection methods, and ensure the quality and ethics of your data. Data collection skills ...
Revised on June 21, 2023. Data collection is a systematic process of gathering observations or measurements. Whether you are performing research for business, governmental or academic purposes, data collection allows you to gain first-hand knowledge and original insights into your research problem. While methods and aims may differ between ...
Research Data Management. Welcome to this module, where we will cover all the main aspects of looking after your research data, including: how to store and backup up data. how to organise data. what to do with protected data (personal or commercially sensitive) why sharing data is important and how to do it. writing Data Management Plans.
Considering a test period or a pilot phase to allow you to improve and modify data collection methods, as may be needed. Step 5: Analyze and interpret data. Step 5 involves analyzing and interpreting the data collected. Whether quantitative and/or qualitative methods of gathering data are used, the analysis can be complex, or less so, depending ...
Qualitative research is a method of exploring and understanding human experiences, behaviors, and meanings. It involves collecting and analyzing data from various sources, such as interviews ...
Organizing data means arranging your data and other research materials so they can be found - by yourself and by others - as needed. Here are four factors to consider when organizing data. Remember, you can't use data you can't find. Names. Data should be labelled using a consistent and descriptive file naming system.
If you want to become a data scientist or just collect those skills, check out this 44-week data science bootcamp. You will learn the essential data science, machine learning, and analytical skills needed for a solid career in the field. Glassdoor.com shows that data scientists in the United States make an average yearly salary of $129,127. So ...
Your choice of data collection method (or alternately called a data gathering procedure) depends on the research questions you're working on, the type of data required, and the available time and resources and time. You can categorize data-gathering procedures into two main methods: Primary data collection. Primary data is collected via first ...
One of the main stages in a research study is data collection that enables the researcher to find answers to research questions. Data collection is the process of collecting data aiming to gain insights regarding the research topic. There are different types of data and different data collection methods accordingly.
Research skills are the ability to find out accurate information on a topic. They include being able to determine the data you need, find and interpret those findings, and then explain that to others. Being able to do effective research is a beneficial skill in any profession, as data and research inform how businesses operate. …
Step 4: Design Your Data Collection Tools. Now that your plan is in place, it's time to design the tools you'll use to collect the data. This could be a survey questionnaire, an interview script, or an observation checklist. Remember to keep your questions clear, concise, and unbiased to ensure high-quality data.
Organising your data. Once you create, gather, or start manipulating data and files, they can quickly become disorganised. To save time and prevent errors later on, you and your colleagues should decide how you will name and structure files and folders. Including documentation (or 'metadata') will allow you to add context to your data so that ...
Table of contents. Step 1: Define the aim of your research. Step 2: Choose your data collection method. Step 3: Plan your data collection procedures. Step 4: Collect the data. Frequently asked questions about data collection.
What does it mean to organize data? Organizing data means arranging your data and other research materials so they can be found—by yourself and by others—as needed. Here are four factors to consider when organizing data. Remember: you can't use data you can't find. NAMES. Data should be labeled using a consistent and descriptive file ...
For starters, add dates in your file names and organize by project folders. Start with having different folders for different projects such as "Greenhouse Phytoremediation Study" and "Arid Land Soil Microbiome Project.". Divide these major project folders into specific folders for different data types, for example, "Soil Chemical ...
Here's an in-depth overview of the top seven tips for data collection skills improvement so that your company is able to compete and stay afloat in this tough data-driven market. 1. Strategize, filter, and set objectives. The first suggestion for improvising your data collection abilities is to develop a methodological approach.
While we focus on primary data collection methods in this guide, we encourage you not to overlook the value of incorporating secondary data into your research design where appropriate. 3. Choose your data collection method. When choosing your data collection method, there are many options at your disposal.
Use data entry software. There are many data entry software programs available that can help you improve your data entry skills. Data entry software is designed to streamline the process of entering data by providing an interface that is easy to use and understand. 2. Use Excel for data analysis.
7 Data Collection Methods Used in Business Analytics. 1. Surveys. Surveys are physical or digital questionnaires that gather both qualitative and quantitative data from subjects. One situation in which you might conduct a survey is gathering attendee feedback after an event.
If you're interested in becoming a data collector, learning more about the skills required for this position can help you determine if it's the right career for you. Data Collector Skills. Time Management. Problem Solving. Attention to Detail. Adaptability. Data Entry.
Kristen Briney provides a useful guidebook, although the title may mislead those looking for a broad-ranging view of data management. The book addresses data management relatively narrowly and targets a fairly specific kind of researcher - those the author encounters in her work as a research scientist turned librarian, specialising in advising scientists on data management.
This chapter provides guidance on using existing data in INAs. Existing data can be obtained from a wide variety of sources and can take many different forms. In some INAs the gathering of new information might not be required because enough data already exist. In many INAs the investigators will use existing information to establish the ...
According to the National Association of Colleges and Employers (page 9-10 from the textbook), the following are the top five personal qualities or skills potential employers seek: 1. Communication skills (verbal and written) 2. Strong work ethic. 3. Teamwork skills (works well with others, group communication) 4. Initiative. 5. Analytical skills