What Is Scope And Limitation In Research?
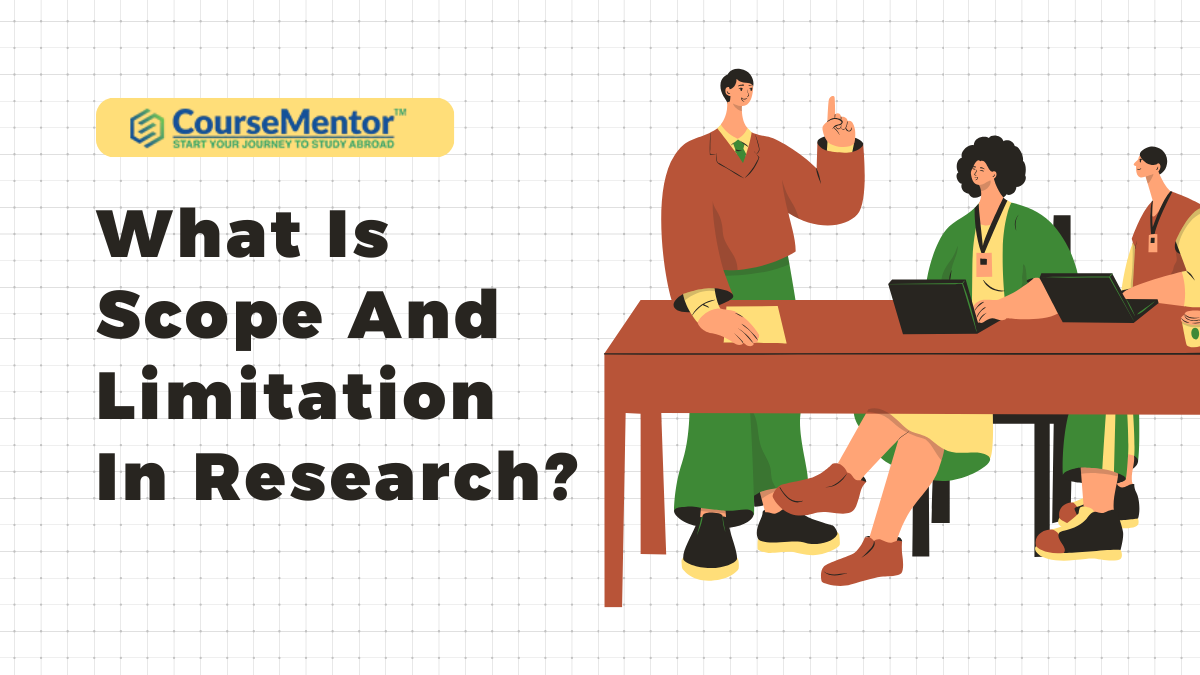
- Post author By admin
- April 16, 2024
Research is the cornerstone of progress in any field, be it science, social sciences, or humanities. But behind every research endeavor lies a crucial aspect that often goes unnoticed by many: the scope and limitation. In this blog, we’ll delve into what is scope and limitation in research, why they matter, and how they influence the outcome of a study.
Table of Contents

What Are The Main 10 Stages Of Research Process?
The research process typically involves several stages, each crucial for the successful completion of a study. Here are the main 10 stages of the research process:
- Identifying the Research Problem: This stage involves identifying a topic or issue that warrants investigation. Researchers should ensure the problem is significant, relevant, and feasible for study.
- Reviewing the Literature: Conducting a thorough review of existing literature on the chosen topic is essential. This helps researchers figure out what we already know, spot where there are holes in our knowledge, and make their research questions clearer.
- Formulating Research Questions or Hypotheses: Based on the identified research problem and literature review, researchers develop specific research questions or hypotheses that they aim to address through their study.
- Designing the Research Methodology: This stage involves selecting the appropriate research design, methods, and techniques for data collection and analysis. Researchers must consider factors such as the research objectives, the nature of the data, and ethical considerations.
- Sampling: Researchers need to determine the sampling strategy and select participants or samples that represent the population of interest. Sampling methods may vary depending on the research design and objectives.
- Data Collection: This stage involves collecting data using the chosen methods and techniques. Data collection may involve surveys, interviews, observations, experiments, or analysis of existing data, among other methods.
- Data Analysis: After collecting data, researchers look at it carefully using the right tools to figure out what it means. They want to find important ideas, check if their guesses are right, and answer the questions they’re studying.
- Interpreting Results: Researchers analyze the results of their data to understand how they relate to the questions or guesses they had at the beginning of their study. They assess the significance of the results, identify patterns or trends, and draw conclusions.
- Drawing Conclusions and Implications: Based on the interpretation of results, researchers draw conclusions regarding the research questions or hypotheses. They discuss the implications of their findings, their relevance to theory or practice, and any recommendations for future research or applications.
- Writing and Presenting the Research Report: Finally, researchers write a comprehensive research report detailing the entire research process, from problem identification to conclusions. The report typically includes an introduction, literature review, methodology, results, discussion, and conclusion. Researchers may also present their findings at conferences or publish them in academic journals.
Scope in Research
- Definition: The scope of research outlines the boundaries and extent of the study.
- Components: It includes various elements such as research objectives, questions, methodology, timeframe, and geographic coverage.
- Guiding Factor: Scope guides researchers in determining what aspects of the topic will be included in the study and what will be excluded.
- Clarity: Defining the scope ensures clarity and focus, preventing researchers from straying off-topic.
- Relevance: It helps in ensuring that the research addresses pertinent issues and contributes meaningfully to the existing body of knowledge.
Limitation in Research
- Definition: Limitations refer to constraints or weaknesses within the research study that may impact its validity or generalizability.
- Types: Limitations can arise due to various factors such as methodological constraints, resource limitations, scope constraints, ethical considerations, and time constraints.
- Acknowledgement: Researchers should openly acknowledge limitations to maintain transparency and credibility.
- Mitigation: While some limitations may be unavoidable, researchers can mitigate their impact through careful planning, rigorous methodology, and transparent reporting.
- Future Implications: Identifying and addressing limitations can provide valuable insights for future research, guiding researchers in overcoming similar challenges in subsequent studies.
What Is Scope And Limitation In Research Example?
Scope in research example.
Let’s consider a research study investigating the impact of social media usage on teenagers’ mental health in urban areas of a particular city over the past three years. The scope of this study would include:
- Research Goals: We want to understand how using social media affects how teenagers feel.
- Research Questions: How much do teenagers in cities use social media, and for how long? Does using social media relate to how teenagers feel, like if they feel sad or anxious?
- Research Methodology: Utilizing surveys and interviews to gather data on social media usage patterns and mental health indicators.
- Timeframe: The study will focus on data collected over the past three years to capture recent trends and changes.
- Geographic Coverage: The study will concentrate on urban areas within a specific city, ensuring relevance and context specificity.
Limitation in Research Example
In the same study, limitations may arise due to various factors:
- Methodological Limitation: The reliance on self-reported data from surveys and interviews may introduce response bias and inaccuracies.
- Resource Limitation: Limited funding and time constraints may restrict the sample size and the depth of data collection, potentially affecting the study’s comprehensiveness.
- Scope Limitation: Focusing solely on urban areas may limit the generalizability of the findings to rural or suburban populations.
- Ethical Limitation: Ensuring informed consent and protecting the privacy of participants may pose ethical challenges, especially when dealing with sensitive topics like mental health.
- Time Limitation: The study’s timeframe of three years may not capture long-term effects or trends in social media usage and mental health outcomes.
In this example, the scope defines the parameters and objectives of the study, while the limitations highlight potential constraints and challenges that may impact the research process and findings.
How Do You Write Scope And Limitations In Research?
Writing the scope and limitations section in a research paper involves clearly defining the parameters of your study and acknowledging any constraints or weaknesses that may impact its validity or generalizability. Here’s a step-by-step guide on how to write the scope and limitations in research:
- Begin with the Scope
- Start by defining the scope of your research. This involves outlining the boundaries and extent of your study.
- Clearly state the objectives of your research and the specific aspects you will investigate.
- Identify the research questions or hypotheses that you aim to address.
- Describe the methodology you will use, including data collection and analysis techniques.
- Specify the timeframe and geographic coverage of your study.
- Be Concise and Specific
- Avoid ambiguity by being concise and specific in your description of the scope. Clearly define what will be included in your study and what will be excluded.
- Use clear and precise language to convey the scope of your research to your readers.
- Acknowledge Limitations
- After defining the scope, acknowledge any limitations or constraints that may impact your study.
- Identify potential methodological limitations, such as sample size, data collection methods, or measurement tools.
- Consider resource limitations, including funding, time, and access to data or participants.
- Discuss any scope limitations, such as geographic or demographic restrictions.
- Address ethical considerations and any potential biases or confounding factors.
- Provide Justification
- Explain why these limitations are relevant to your study and how they may affect the interpretation of your results.
- Justify your choices and decisions regarding the scope and limitations of your research.
- Demonstrate awareness of potential challenges and demonstrate transparency in your reporting.
- Offer Recommendations
- Despite limitations, suggest ways to mitigate their impact or address them in future research.
- Provide recommendations for researchers who may encounter similar constraints in their own studies.
- Highlight the implications of your research findings in light of the acknowledged limitations.
- Review and Revise
- Review your scope and limitations section to ensure clarity, coherence, and accuracy.
- Revise as needed to ensure that your description accurately reflects the parameters of your study and acknowledges any potential constraints.
In conclusion (of what is scope and limitation in research), scope and limitation are integral components of any research project. Understanding the scope helps researchers define the boundaries and parameters of their study, while acknowledging limitations ensures transparency and credibility.
By carefully considering scope and limitation, researchers can conduct more rigorous and meaningful studies that contribute to the advancement of knowledge in their respective fields.
- australia (2)
- duolingo (13)
- Education (284)
- General (77)
- How To (18)
- IELTS (127)
- Latest Updates (162)
- Malta Visa (6)
- Permanent residency (1)
- Programming (31)
- Scholarship (1)
- Sponsored (4)
- Study Abroad (187)
- Technology (12)
- work permit (8)
Recent Posts
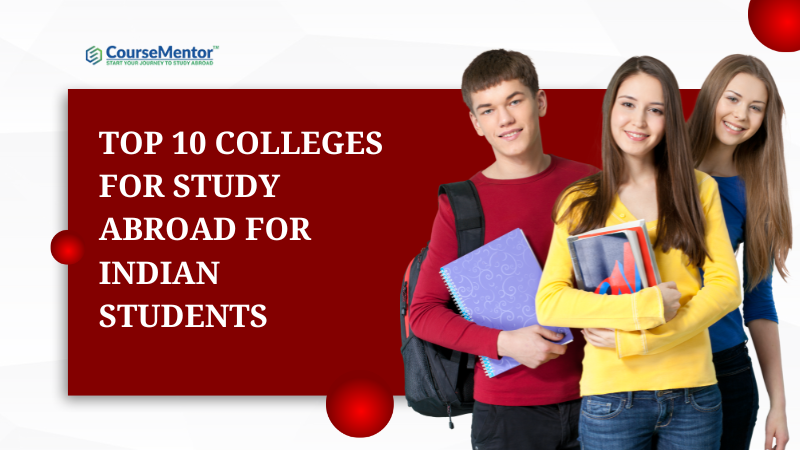
Scope and Delimitations in Research
Delimitations are the boundaries that the researcher sets in a research study, deciding what to include and what to exclude. They help to narrow down the study and make it more manageable and relevant to the research goal.
Updated on October 19, 2022

All scientific research has boundaries, whether or not the authors clearly explain them. Your study's scope and delimitations are the sections where you define the broader parameters and boundaries of your research.
The scope details what your study will explore, such as the target population, extent, or study duration. Delimitations are factors and variables not included in the study.
Scope and delimitations are not methodological shortcomings; they're always under your control. Discussing these is essential because doing so shows that your project is manageable and scientifically sound.
This article covers:
- What's meant by “scope” and “delimitations”
- Why these are integral components of every study
- How and where to actually write about scope and delimitations in your manuscript
- Examples of scope and delimitations from published studies
What is the scope in a research paper?
Simply put, the scope is the domain of your research. It describes the extent to which the research question will be explored in your study.
Articulating your study's scope early on helps you make your research question focused and realistic.
It also helps decide what data you need to collect (and, therefore, what data collection tools you need to design). Getting this right is vital for both academic articles and funding applications.
What are delimitations in a research paper?
Delimitations are those factors or aspects of the research area that you'll exclude from your research. The scope and delimitations of the study are intimately linked.
Essentially, delimitations form a more detailed and narrowed-down formulation of the scope in terms of exclusion. The delimitations explain what was (intentionally) not considered within the given piece of research.
Scope and delimitations examples
Use the following examples provided by our expert PhD editors as a reference when coming up with your own scope and delimitations.
Scope example
Your research question is, “What is the impact of bullying on the mental health of adolescents?” This topic, on its own, doesn't say much about what's being investigated.
The scope, for example, could encompass:
- Variables: “bullying” (dependent variable), “mental health” (independent variable), and ways of defining or measuring them
- Bullying type: Both face-to-face and cyberbullying
- Target population: Adolescents aged 12–17
- Geographical coverage: France or only one specific town in France
Delimitations example
Look back at the previous example.
Exploring the adverse effects of bullying on adolescents' mental health is a preliminary delimitation. This one was chosen from among many possible research questions (e.g., the impact of bullying on suicide rates, or children or adults).
Delimiting factors could include:
- Research design : Mixed-methods research, including thematic analysis of semi-structured interviews and statistical analysis of a survey
- Timeframe : Data collection to run for 3 months
- Population size : 100 survey participants; 15 interviewees
- Recruitment of participants : Quota sampling (aiming for specific portions of men, women, ethnic minority students etc.)
We can see that every choice you make in planning and conducting your research inevitably excludes other possible options.
What's the difference between limitations and delimitations?
Delimitations and limitations are entirely different, although they often get mixed up. These are the main differences:
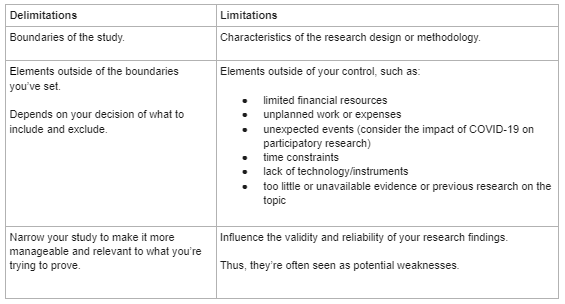
This chart explains the difference between delimitations and limitations. Delimitations are the boundaries of the study while the limitations are the characteristics of the research design or methodology.
Delimitations encompass the elements outside of the boundaries you've set and depends on your decision of what yo include and exclude. On the flip side, limitations are the elements outside of your control, such as:
- limited financial resources
- unplanned work or expenses
- unexpected events (for example, the COVID-19 pandemic)
- time constraints
- lack of technology/instruments
- unavailable evidence or previous research on the topic
Delimitations involve narrowing your study to make it more manageable and relevant to what you're trying to prove. Limitations influence the validity and reliability of your research findings. Limitations are seen as potential weaknesses in your research.
Example of the differences
To clarify these differences, go back to the limitations of the earlier example.
Limitations could comprise:
- Sample size : Not large enough to provide generalizable conclusions.
- Sampling approach : Non-probability sampling has increased bias risk. For instance, the researchers might not manage to capture the experiences of ethnic minority students.
- Methodological pitfalls : Research participants from an urban area (Paris) are likely to be more advantaged than students in rural areas. A study exploring the latter's experiences will probably yield very different findings.
Where do you write the scope and delimitations, and why?
It can be surprisingly empowering to realize you're restricted when conducting scholarly research. But this realization also makes writing up your research easier to grasp and makes it easier to see its limits and the expectations placed on it. Properly revealing this information serves your field and the greater scientific community.
Openly (but briefly) acknowledge the scope and delimitations of your study early on. The Abstract and Introduction sections are good places to set the parameters of your paper.
Next, discuss the scope and delimitations in greater detail in the Methods section. You'll need to do this to justify your methodological approach and data collection instruments, as well as analyses
At this point, spell out why these delimitations were set. What alternative options did you consider? Why did you reject alternatives? What could your study not address?
Let's say you're gathering data that can be derived from different but related experiments. You must convince the reader that the one you selected best suits your research question.
Finally, a solid paper will return to the scope and delimitations in the Findings or Discussion section. Doing so helps readers contextualize and interpret findings because the study's scope and methods influence the results.
For instance, agricultural field experiments carried out under irrigated conditions yield different results from experiments carried out without irrigation.
Being transparent about the scope and any outstanding issues increases your research's credibility and objectivity. It helps other researchers replicate your study and advance scientific understanding of the same topic (e.g., by adopting a different approach).
How do you write the scope and delimitations?
Define the scope and delimitations of your study before collecting data. This is critical. This step should be part of your research project planning.
Answering the following questions will help you address your scope and delimitations clearly and convincingly.
- What are your study's aims and objectives?
- Why did you carry out the study?
- What was the exact topic under investigation?
- Which factors and variables were included? And state why specific variables were omitted from the research scope.
- Who or what did the study explore? What was the target population?
- What was the study's location (geographical area) or setting (e.g., laboratory)?
- What was the timeframe within which you collected your data ?
- Consider a study exploring the differences between identical twins who were raised together versus identical twins who weren't. The data collection might span 5, 10, or more years.
- A study exploring a new immigration policy will cover the period since the policy came into effect and the present moment.
- How was the research conducted (research design)?
- Experimental research, qualitative, quantitative, or mixed-methods research, literature review, etc.
- What data collection tools and analysis techniques were used? e.g., If you chose quantitative methods, which statistical analysis techniques and software did you use?
- What did you find?
- What did you conclude?
Useful vocabulary for scope and delimitations
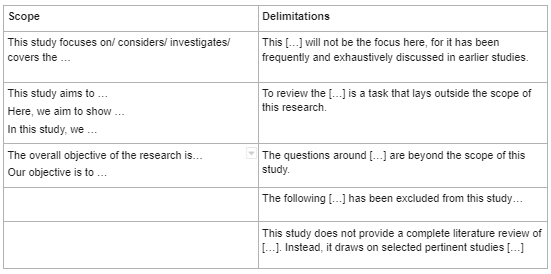
When explaining both the scope and delimitations, it's important to use the proper language to clearly state each.
For the scope , use the following language:
- This study focuses on/considers/investigates/covers the following:
- This study aims to . . . / Here, we aim to show . . . / In this study, we . . .
- The overall objective of the research is . . . / Our objective is to . . .
When stating the delimitations, use the following language:
- This [ . . . ] will not be the focus, for it has been frequently and exhaustively discusses in earlier studies.
- To review the [ . . . ] is a task that lies outside the scope of this study.
- The following [ . . . ] has been excluded from this study . . .
- This study does not provide a complete literature review of [ . . . ]. Instead, it draws on selected pertinent studies [ . . . ]
Analysis of a published scope
In one example, Simione and Gnagnarella (2020) compared the psychological and behavioral impact of COVID-19 on Italy's health workers and general population.
Here's a breakdown of the study's scope into smaller chunks and discussion of what works and why.
Also notable is that this study's delimitations include references to:
- Recruitment of participants: Convenience sampling
- Demographic characteristics of study participants: Age, sex, etc.
- Measurements methods: E.g., the death anxiety scale of the Existential Concerns Questionnaire (ECQ; van Bruggen et al., 2017) etc.
- Data analysis tool: The statistical software R
Analysis of published scope and delimitations
Scope of the study : Johnsson et al. (2019) explored the effect of in-hospital physiotherapy on postoperative physical capacity, physical activity, and lung function in patients who underwent lung cancer surgery.
The delimitations narrowed down the scope as follows:
Refine your scope, delimitations, and scientific English
English ability shouldn't limit how clear and impactful your research can be. Expert AJE editors are available to assess your science and polish your academic writing. See AJE services here .

The AJE Team
See our "Privacy Policy"
Educational resources and simple solutions for your research journey
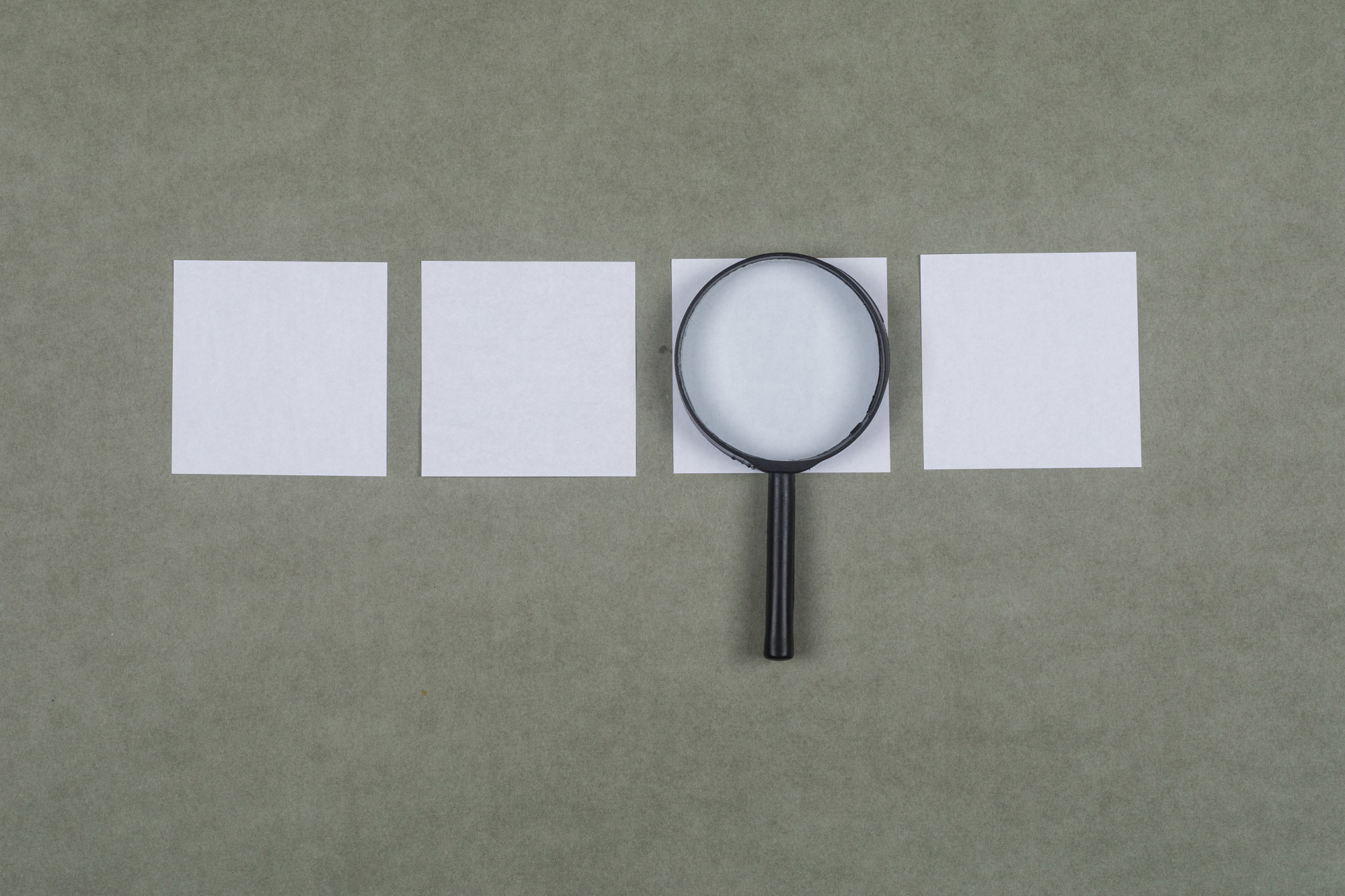
Decoding the Scope and Delimitations of the Study in Research
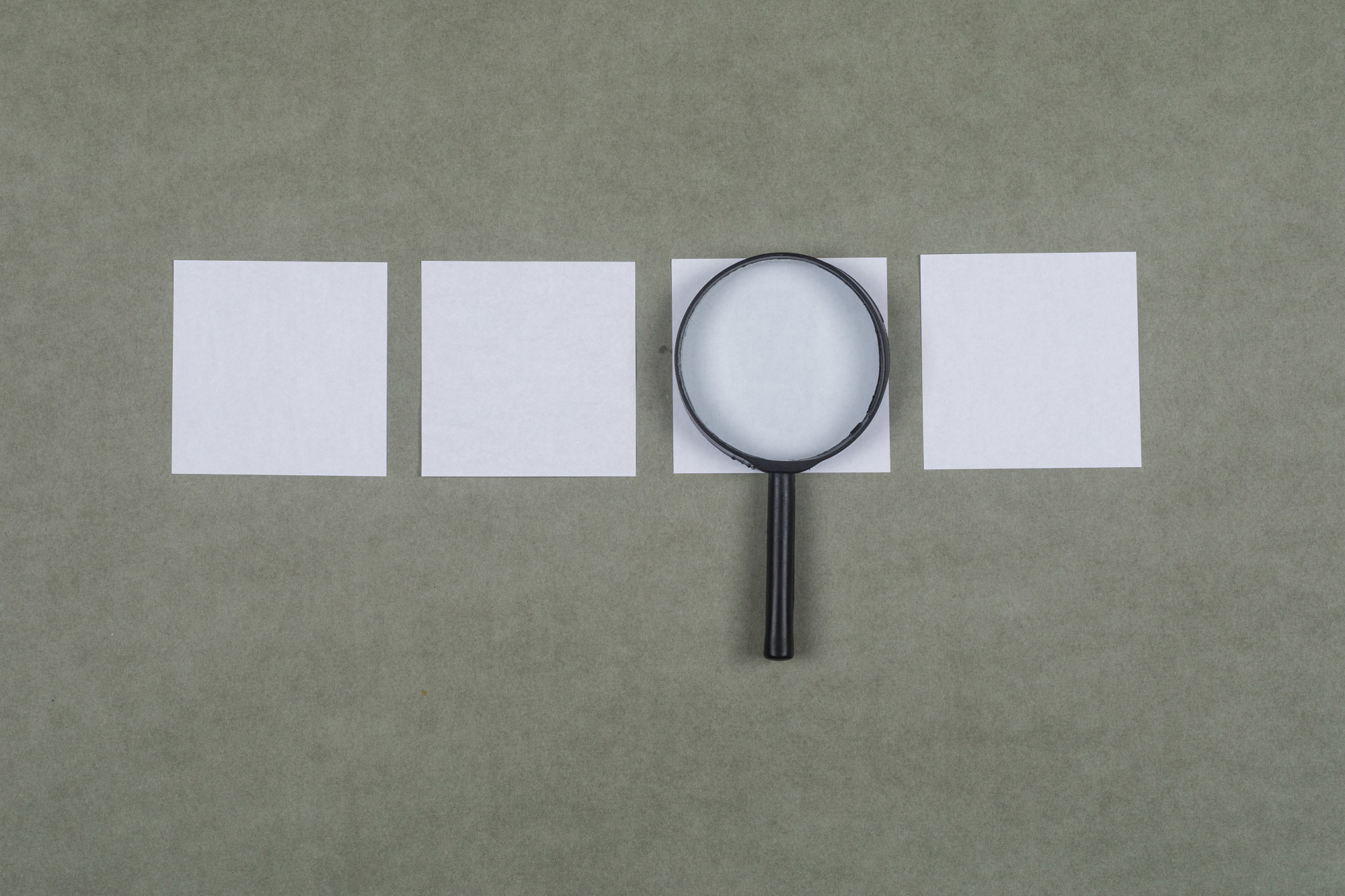
Scope and delimitations of the study are two essential elements of a research paper or thesis that help to contextualize and convey the focus and boundaries of a research study. This allows readers to understand the research focus and the kind of information to expect. For researchers, especially students and early career researchers, understanding the meaning and purpose of the scope and delimitation of a study is crucial to craft a well-defined and impactful research project. In this article, we delve into the core concepts of scope and delimitation in a study, providing insightful examples, and practical tips on how to effectively incorporate them into your research endeavors.
Table of Contents
What is scope and delimitation in research
The scope of a research paper explains the context and framework for the study, outlines the extent, variables, or dimensions that will be investigated, and provides details of the parameters within which the study is conducted. Delimitations in research , on the other hand, refer to the limitations imposed on the study. It identifies aspects of the topic that will not be covered in the research, conveys why these choices were made, and how this will affect the outcome of the research. By narrowing down the scope and defining delimitations, researchers can ensure focused research and avoid pitfalls, which ensures the study remains feasible and attainable.
Example of scope and delimitation of a study
A researcher might want to study the effects of regular physical exercise on the health of senior citizens. This would be the broad scope of the study, after which the researcher would refine the scope by excluding specific groups of senior citizens, perhaps based on their age, gender, geographical location, cultural influences, and sample sizes. These then, would form the delimitations of the study; in other words, elements that describe the boundaries of the research.
The purpose of scope and delimitation in a study
The purpose of scope and delimitation in a study is to establish clear boundaries and focus for the research. This allows researchers to avoid ambiguity, set achievable objectives, and manage their project efficiently, ultimately leading to more credible and meaningful findings in their study. The scope and delimitation of a study serve several important purposes, including:
- Establishing clarity: Clearly defining the scope and delimitation of a study helps researchers and readers alike understand the boundaries of the investigation and what to expect from it.
- Focus and relevance: By setting the scope, researchers can concentrate on specific research questions, preventing the study from becoming too broad or irrelevant.
- Feasibility: Delimitations of the study prevent researchers from taking on too unrealistic or unmanageable tasks, making the research more achievable.
- Avoiding ambiguity: A well-defined scope and delimitation of the study minimizes any confusion or misinterpretation regarding the research objectives and methods.
Given the importance of both the scope and delimitations of a study, it is imperative to ensure that they are mentioned early on in the research manuscript. Most experts agree that the scope of research should be mentioned as part of the introduction and the delimitations must be mentioned as part of the methods section. Now that we’ve covered the scope and delimitation meaning and purpose, we look at how to write each of these sections.
How to write the scope of the study in research
When writing the scope of the study, remain focused on what you hope to achieve. Broadening the scope too much might make it too generic while narrowing it down too much may affect the way it would be interpreted. Ensure the scope of the study is clear, concise and accurate. Conduct a thorough literature review to understand existing literature, which will help identify gaps and refine the scope of your study.
It is helpful if you structure the scope in a way that answers the Six Ws – questions whose answers are considered basic in information-gathering.
Why: State the purpose of the research by articulating the research objectives and questions you aim to address in your study.
What: Outline the specific topic to be studied, while mentioning the variables, concepts, or aspects central to your research; these will define the extent of your study.
Where: Provide the setting or geographical location where the research study will be conducted.
When : Mention the specific timeframe within which the research data will be collected.
Who : Specify the sample size for the study and the profile of the population they will be drawn from.
How : Explain the research methodology, research design, and tools and analysis techniques.
How to write the delimitations of a study in research
When writing the delimitations of the study, researchers must provide all the details clearly and precisely. Writing the delimitations of the study requires a systematic approach to narrow down the research’s focus and establish boundaries. Follow these steps to craft delimitations effectively:
- Clearly understand the research objectives and questions you intend to address in your study.
- Conduct a comprehensive literature review to identify gaps and areas that have already been extensively covered. This helps to avoid redundancies and home in on a unique issue.
- Clearly state what aspects, variables, or factors you will be excluding in your research; mention available alternatives, if any, and why these alternatives were rejected.
- Explain how you the delimitations were set, and they contribute to the feasibility and relevance of your study, and how they align with the research objectives.
- Be sure to acknowledge limitations in your research, such as constraints related to time, resources, or data availability.
Being transparent ensures credibility, while explaining why the delimitations of your study could not be overcome with standard research methods backed up by scientific evidence can help readers understand the context better.
Differentiating between delimitations and limitations
Most early career researchers get confused and often use these two terms interchangeably which is wrong. Delimitations of a study refer to the set boundaries and specific parameters within which the research is carried out. They help narrow down your focus and makes it more relevant to what you are trying to prove.
Meanwhile, limitations in a study refer to the validity and reliability of the research being conducted. They are those elements of your study that are usually out of your immediate control but are still able to affect your findings in some way. In other words, limitation are potential weaknesses of your research.
In conclusion, scope and delimitation of a study are vital elements that shape the trajectory of your research study. The above explanations will have hopefully helped you better understand the scope and delimitations meaning, purpose, and importance in crafting focused, feasible, and impactful research studies. Be sure to follow the simple techniques to write the scope and delimitations of the study to embark on your research journey with clarity and confidence. Happy researching!
Editage All Access is a subscription-based platform that unifies the best AI tools and services designed to speed up, simplify, and streamline every step of a researcher’s journey. The Editage All Access Pack is a one-of-a-kind subscription that unlocks full access to an AI writing assistant, literature recommender, journal finder, scientific illustration tool, and exclusive discounts on professional publication services from Editage.
Based on 22+ years of experience in academia, Editage All Access empowers researchers to put their best research forward and move closer to success. Explore our top AI Tools pack, AI Tools + Publication Services pack, or Build Your Own Plan. Find everything a researcher needs to succeed, all in one place – Get All Access now starting at just $14 a month !
Related Posts

Conference Paper vs. Journal Paper: What’s the Difference

What does Ibid. mean? Citation Examples

Setting Limits and Focusing Your Study: Exploring scope and delimitation
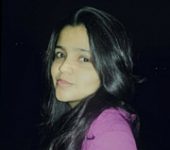
As a researcher, it can be easy to get lost in the vast expanse of information and data available. Thus, when starting a research project, one of the most important things to consider is the scope and delimitation of the study. Setting limits and focusing your study is essential to ensure that the research project is manageable, relevant, and able to produce useful results. In this article, we will explore the importance of setting limits and focusing your study through an in-depth analysis of scope and delimitation.
Company Name 123
Lorem ipsum dolor sit amet, cu usu cibo vituperata, id ius probo maiestatis inciderint, sit eu vide volutpat.
Sign Up for More Insights
Table of Contents
Scope and Delimitation – Definition and difference
Scope refers to the range of the research project and the study limitations set in place to define the boundaries of the project and delimitation refers to the specific aspects of the research project that the study will focus on.
In simpler words, scope is the breadth of your study, while delimitation is the depth of your study.
Scope and delimitation are both essential components of a research project, and they are often confused with one another. The scope defines the parameters of the study, while delimitation sets the boundaries within those parameters. The scope and delimitation of a study are usually established early on in the research process and guide the rest of the project.
Types of Scope and Delimitation
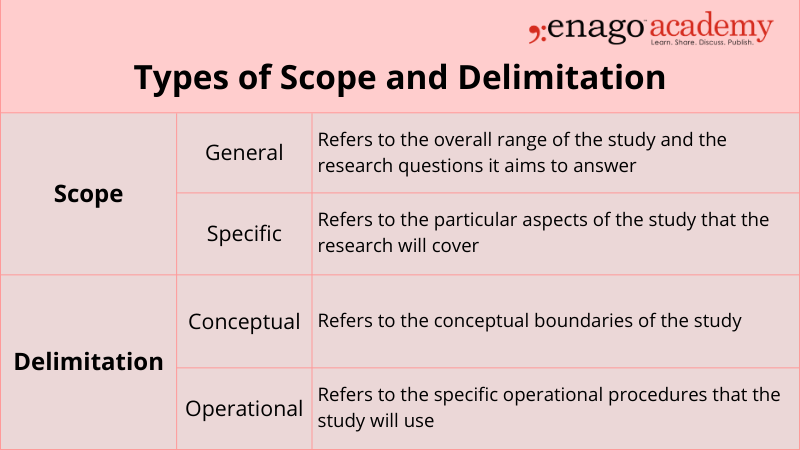
Significance of Scope and Delimitation
Setting limits and focusing your study through scope and delimitation is crucial for the following reasons:
- It allows researchers to define the research project’s boundaries, enabling them to focus on specific aspects of the project. This focus makes it easier to gather relevant data and avoid unnecessary information that might complicate the study’s results.
- Setting limits and focusing your study through scope and delimitation enables the researcher to stay within the parameters of the project’s resources.
- A well-defined scope and delimitation ensure that the research project can be completed within the available resources, such as time and budget, while still achieving the project’s objectives.
5 Steps to Setting Limits and Defining the Scope and Delimitation of Your Study
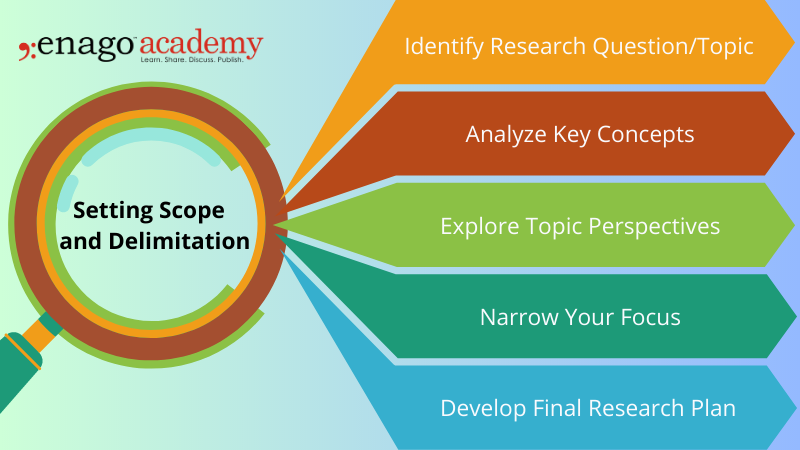
There are a few steps that you can take to set limits and focus your study.
1. Identify your research question or topic
The first step is to identify what you are interested in learning about. The research question should be specific, measurable, achievable, relevant, and time-bound (SMART). Once you have a research question or topic, you can start to narrow your focus.
2. Consider the key terms or concepts related to your topic
What are the important terms or concepts that you need to understand in order to answer your research question? Consider all available resources, such as time, budget, and data availability, when setting scope and delimitation.
The scope and delimitation should be established within the parameters of the available resources. Once you have identified the key terms or concepts, you can start to develop a glossary or list of definitions.
3. Consider the different perspectives on your topic
There are often different perspectives on any given topic. Get feedback on the proposed scope and delimitation. Advisors can provide guidance on the feasibility of the study and offer suggestions for improvement.
It is important to consider all of the different perspectives in order to get a well-rounded understanding of your topic.
4. Narrow your focus
Be specific and concise when setting scope and delimitation. The parameters of the study should be clearly defined to avoid ambiguity and ensure that the study is focused on relevant aspects of the research question.
This means deciding which aspects of your topic you will focus on and which aspects you will eliminate.
5. Develop the final research plan
Revisit and revise the scope and delimitation as needed. As the research project progresses, the scope and delimitation may need to be adjusted to ensure that the study remains focused on the research question and can produce useful results. This plan should include your research goals, methods, and timeline.
Examples of Scope and Delimitation
To better understand scope and delimitation, let us consider two examples of research questions and how scope and delimitation would apply to them.
Research question: What are the effects of social media on mental health?
Scope: The scope of the study will focus on the impact of social media on the mental health of young adults aged 18-24 in the United States.
Delimitation: The study will specifically examine the following aspects of social media: frequency of use, types of social media platforms used, and the impact of social media on self-esteem and body image.
Research question: What are the factors that influence employee job satisfaction in the healthcare industry?
Scope: The scope of the study will focus on employee job satisfaction in the healthcare industry in the United States.
Delimitation: The study will specifically examine the following factors that influence employee job satisfaction: salary, work-life balance, job security, and opportunities for career growth.
Setting limits and defining the scope and delimitation of a research study is essential to conducting effective research. By doing so, researchers can ensure that their study is focused, manageable, and feasible within the given time frame and resources. It can also help to identify areas that require further study, providing a foundation for future research.
So, the next time you embark on a research project, don’t forget to set clear limits and define the scope and delimitation of your study. It may seem like a tedious task, but it can ultimately lead to more meaningful and impactful research. And if you still can’t find a solution, reach out to Enago Academy using #AskEnago and tag @EnagoAcademy on Twitter , Facebook , and Quora .
Frequently Asked Questions
The scope in research refers to the boundaries and extent of a study, defining its specific objectives, target population, variables, methods, and limitations, which helps researchers focus and provide a clear understanding of what will be investigated.
Delimitation in research defines the specific boundaries and limitations of a study, such as geographical, temporal, or conceptual constraints, outlining what will be excluded or not within the scope of investigation, providing clarity and ensuring the study remains focused and manageable.
To write a scope; 1. Clearly define research objectives. 2. Identify specific research questions. 3. Determine the target population for the study. 4. Outline the variables to be investigated. 5. Establish limitations and constraints. 6. Set boundaries and extent of the investigation. 7. Ensure focus, clarity, and manageability. 8. Provide context for the research project.
To write delimitations; 1. Identify geographical boundaries or constraints. 2. Define the specific time period or timeframe of the study. 3. Specify the sample size or selection criteria. 4. Clarify any demographic limitations (e.g., age, gender, occupation). 5. Address any limitations related to data collection methods. 6. Consider limitations regarding the availability of resources or data. 7. Exclude specific variables or factors from the scope of the study. 8. Clearly state any conceptual boundaries or theoretical frameworks. 9. Acknowledge any potential biases or constraints in the research design. 10. Ensure that the delimitations provide a clear focus and scope for the study.

What is an example of delimitation of the study?
Thank you 💕
Thank You very simplified🩷
Thanks, I find this article very helpful
Rate this article Cancel Reply
Your email address will not be published.
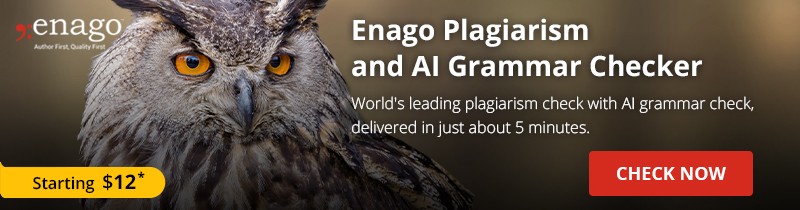
Enago Academy's Most Popular Articles
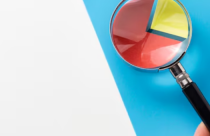
- Promoting Research
Graphical Abstracts Vs. Infographics: Best practices for using visual illustrations for increased research impact
Dr. Sarah Chen stared at her computer screen, her eyes staring at her recently published…
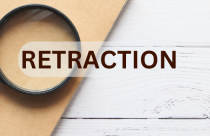
- Publishing Research
10 Tips to Prevent Research Papers From Being Retracted
Research paper retractions represent a critical event in the scientific community. When a published article…
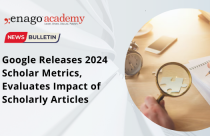
- Industry News
Google Releases 2024 Scholar Metrics, Evaluates Impact of Scholarly Articles
Google has released its 2024 Scholar Metrics, assessing scholarly articles from 2019 to 2023. This…
![scope and limitation of the study in research What is Academic Integrity and How to Uphold it [FREE CHECKLIST]](https://www.enago.com/academy/wp-content/uploads/2024/05/FeatureImages-59-210x136.png)
Ensuring Academic Integrity and Transparency in Academic Research: A comprehensive checklist for researchers
Academic integrity is the foundation upon which the credibility and value of scientific findings are…
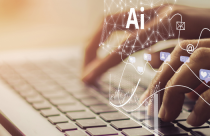
- Old Webinars
- Webinar Mobile App
Improving Research Manuscripts Using AI-Powered Insights: Enago reports for effective research communication
Language Quality Importance in Academia AI in Evaluating Language Quality Enago Language Reports Live Demo…
How to Optimize Your Research Process: A step-by-step guide
Choosing the Right Analytical Approach: Thematic analysis vs. content analysis for…
Research Recommendations – Guiding policy-makers for evidence-based decision making
Demystifying the Role of Confounding Variables in Research

Sign-up to read more
Subscribe for free to get unrestricted access to all our resources on research writing and academic publishing including:
- 2000+ blog articles
- 50+ Webinars
- 10+ Expert podcasts
- 50+ Infographics
- 10+ Checklists
- Research Guides
We hate spam too. We promise to protect your privacy and never spam you.
- Reporting Research
- AI in Academia
- Career Corner
- Diversity and Inclusion
- Infographics
- Expert Video Library
- Other Resources
- Enago Learn
- Upcoming & On-Demand Webinars
- Peer Review Week 2024
- Open Access Week 2023
- Conference Videos
- Enago Report
- Journal Finder
- Enago Plagiarism & AI Grammar Check
- Editing Services
- Publication Support Services
- Research Impact
- Translation Services
- Publication solutions
- AI-Based Solutions
- Thought Leadership
- Call for Articles
- Call for Speakers
- Author Training
- Edit Profile
I am looking for Editing/ Proofreading services for my manuscript Tentative date of next journal submission:
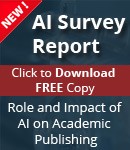
Which among these features would you prefer the most in a peer review assistant?

Community Blog
Keep up-to-date on postgraduate related issues with our quick reads written by students, postdocs, professors and industry leaders.
Scope and Delimitations – Explained & Example

- By DiscoverPhDs
- October 2, 2020
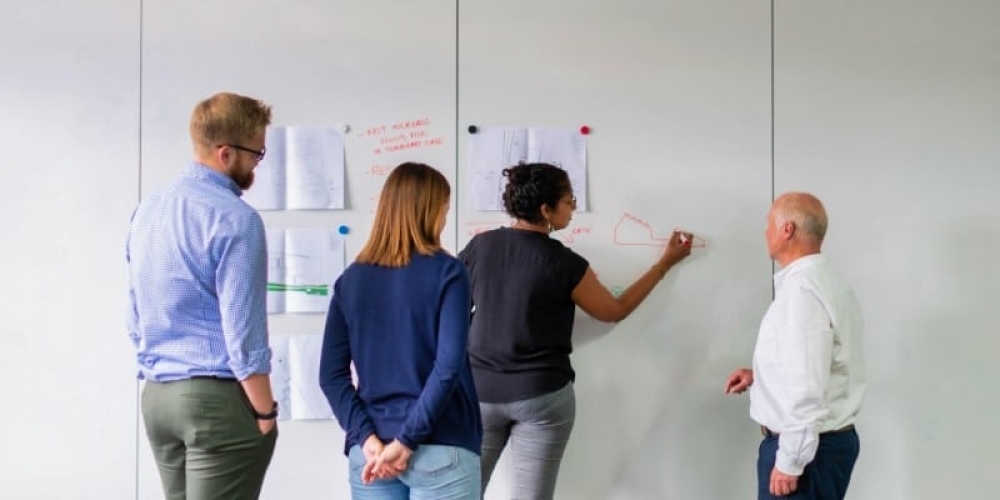
What Is Scope and Delimitation in Research?
The scope and delimitations of a thesis, dissertation or research paper define the topic and boundaries of the research problem to be investigated.
The scope details how in-depth your study is to explore the research question and the parameters in which it will operate in relation to the population and timeframe.
The delimitations of a study are the factors and variables not to be included in the investigation. In other words, they are the boundaries the researcher sets in terms of study duration, population size and type of participants, etc.
Difference Between Delimitations and Limitations
Delimitations refer to the boundaries of the research study, based on the researcher’s decision of what to include and what to exclude. They narrow your study to make it more manageable and relevant to what you are trying to prove.
Limitations relate to the validity and reliability of the study. They are characteristics of the research design or methodology that are out of your control but influence your research findings. Because of this, they determine the internal and external validity of your study and are considered potential weaknesses.
In other words, limitations are what the researcher cannot do (elements outside of their control) and delimitations are what the researcher will not do (elements outside of the boundaries they have set). Both are important because they help to put the research findings into context, and although they explain how the study is limited, they increase the credibility and validity of a research project.
Guidelines on How to Write a Scope
A good scope statement will answer the following six questions:

- Why – the general aims and objectives (purpose) of the research.
- What – the subject to be investigated, and the included variables.
- Where – the location or setting of the study, i.e. where the data will be gathered and to which entity the data will belong.
- When – the timeframe within which the data is to be collected.
- Who – the subject matter of the study and the population from which they will be selected. This population needs to be large enough to be able to make generalisations.
- How – how the research is to be conducted, including a description of the research design (e.g. whether it is experimental research, qualitative research or a case study), methodology, research tools and analysis techniques.
To make things as clear as possible, you should also state why specific variables were omitted from the research scope, and whether this was because it was a delimitation or a limitation. You should also explain why they could not be overcome with standard research methods backed up by scientific evidence.
How to Start Writing Your Study Scope
Use the below prompts as an effective way to start writing your scope:
- This study is to focus on…
- This study covers the…
- This study aims to…
Guidelines on How to Write Delimitations
Since the delimitation parameters are within the researcher’s control, readers need to know why they were set, what alternative options were available, and why these alternatives were rejected. For example, if you are collecting data that can be derived from three different but similar experiments, the reader needs to understand how and why you decided to select the one you have.
Your reasons should always be linked back to your research question, as all delimitations should result from trying to make your study more relevant to your scope. Therefore, the scope and delimitations are usually considered together when writing a paper.
How to Start Writing Your Study Delimitations
Use the below prompts as an effective way to start writing your study delimitations:
- This study does not cover…
- This study is limited to…
- The following has been excluded from this study…
Examples of Delimitation in Research
Examples of delimitations include:
- research objectives,
- research questions,
- research variables,
- target populations,
- statistical analysis techniques .
Examples of Limitations in Research
Examples of limitations include:
- Issues with sample and selection,
- Insufficient sample size, population traits or specific participants for statistical significance,
- Lack of previous research studies on the topic which has allowed for further analysis,
- Limitations in the technology/instruments used to collect your data,
- Limited financial resources and/or funding constraints.

An abstract and introduction are the first two sections of your paper or thesis. This guide explains the differences between them and how to write them.
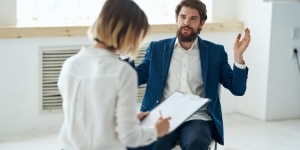
You’ve impressed the supervisor with your PhD application, now it’s time to ace your interview with these powerful body language tips.
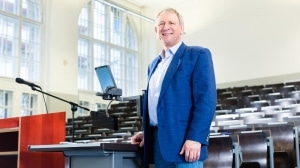
Tenure is a permanent position awarded to professors showing excellence in research and teaching. Find out more about the competitive position!
Join thousands of other students and stay up to date with the latest PhD programmes, funding opportunities and advice.
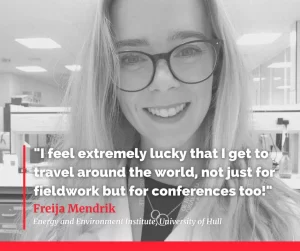
Browse PhDs Now

Scientific misconduct can be described as a deviation from the accepted standards of scientific research, study and publication ethics.
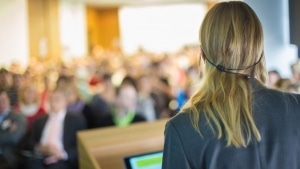
Academic conferences are expensive and it can be tough finding the funds to go; this naturally leads to the question of are academic conferences worth it?

Annabel is a third-year PhD student at the University of Glasgow, looking at the effects of online self-diagnosis and health information seeking on the patient-healthcare professional relationship.
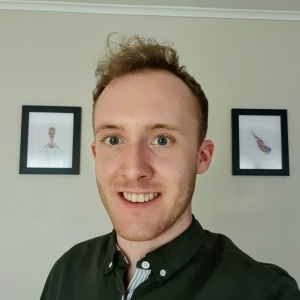
Dr Norman gained his PhD in Biochemistry and Molecular Biology from the University of East Anglia in 2018. He is now the Public Engagement Officer at the Babraham Institute.
Join Thousands of Students
- Privacy Policy

Home » Limitations in Research – Types, Examples and Writing Guide
Limitations in Research – Types, Examples and Writing Guide
Table of Contents

Limitations in Research
Limitations in research refer to the factors that may affect the results, conclusions , and generalizability of a study. These limitations can arise from various sources, such as the design of the study, the sampling methods used, the measurement tools employed, and the limitations of the data analysis techniques.
Types of Limitations in Research
Types of Limitations in Research are as follows:
Sample Size Limitations
This refers to the size of the group of people or subjects that are being studied. If the sample size is too small, then the results may not be representative of the population being studied. This can lead to a lack of generalizability of the results.
Time Limitations
Time limitations can be a constraint on the research process . This could mean that the study is unable to be conducted for a long enough period of time to observe the long-term effects of an intervention, or to collect enough data to draw accurate conclusions.
Selection Bias
This refers to a type of bias that can occur when the selection of participants in a study is not random. This can lead to a biased sample that is not representative of the population being studied.
Confounding Variables
Confounding variables are factors that can influence the outcome of a study, but are not being measured or controlled for. These can lead to inaccurate conclusions or a lack of clarity in the results.
Measurement Error
This refers to inaccuracies in the measurement of variables, such as using a faulty instrument or scale. This can lead to inaccurate results or a lack of validity in the study.
Ethical Limitations
Ethical limitations refer to the ethical constraints placed on research studies. For example, certain studies may not be allowed to be conducted due to ethical concerns, such as studies that involve harm to participants.
Examples of Limitations in Research
Some Examples of Limitations in Research are as follows:
Research Title: “The Effectiveness of Machine Learning Algorithms in Predicting Customer Behavior”
Limitations:
- The study only considered a limited number of machine learning algorithms and did not explore the effectiveness of other algorithms.
- The study used a specific dataset, which may not be representative of all customer behaviors or demographics.
- The study did not consider the potential ethical implications of using machine learning algorithms in predicting customer behavior.
Research Title: “The Impact of Online Learning on Student Performance in Computer Science Courses”
- The study was conducted during the COVID-19 pandemic, which may have affected the results due to the unique circumstances of remote learning.
- The study only included students from a single university, which may limit the generalizability of the findings to other institutions.
- The study did not consider the impact of individual differences, such as prior knowledge or motivation, on student performance in online learning environments.
Research Title: “The Effect of Gamification on User Engagement in Mobile Health Applications”
- The study only tested a specific gamification strategy and did not explore the effectiveness of other gamification techniques.
- The study relied on self-reported measures of user engagement, which may be subject to social desirability bias or measurement errors.
- The study only included a specific demographic group (e.g., young adults) and may not be generalizable to other populations with different preferences or needs.
How to Write Limitations in Research
When writing about the limitations of a research study, it is important to be honest and clear about the potential weaknesses of your work. Here are some tips for writing about limitations in research:
- Identify the limitations: Start by identifying the potential limitations of your research. These may include sample size, selection bias, measurement error, or other issues that could affect the validity and reliability of your findings.
- Be honest and objective: When describing the limitations of your research, be honest and objective. Do not try to minimize or downplay the limitations, but also do not exaggerate them. Be clear and concise in your description of the limitations.
- Provide context: It is important to provide context for the limitations of your research. For example, if your sample size was small, explain why this was the case and how it may have affected your results. Providing context can help readers understand the limitations in a broader context.
- Discuss implications : Discuss the implications of the limitations for your research findings. For example, if there was a selection bias in your sample, explain how this may have affected the generalizability of your findings. This can help readers understand the limitations in terms of their impact on the overall validity of your research.
- Provide suggestions for future research : Finally, provide suggestions for future research that can address the limitations of your study. This can help readers understand how your research fits into the broader field and can provide a roadmap for future studies.
Purpose of Limitations in Research
There are several purposes of limitations in research. Here are some of the most important ones:
- To acknowledge the boundaries of the study : Limitations help to define the scope of the research project and set realistic expectations for the findings. They can help to clarify what the study is not intended to address.
- To identify potential sources of bias: Limitations can help researchers identify potential sources of bias in their research design, data collection, or analysis. This can help to improve the validity and reliability of the findings.
- To provide opportunities for future research: Limitations can highlight areas for future research and suggest avenues for further exploration. This can help to advance knowledge in a particular field.
- To demonstrate transparency and accountability: By acknowledging the limitations of their research, researchers can demonstrate transparency and accountability to their readers, peers, and funders. This can help to build trust and credibility in the research community.
- To encourage critical thinking: Limitations can encourage readers to critically evaluate the study’s findings and consider alternative explanations or interpretations. This can help to promote a more nuanced and sophisticated understanding of the topic under investigation.
When to Write Limitations in Research
Limitations should be included in research when they help to provide a more complete understanding of the study’s results and implications. A limitation is any factor that could potentially impact the accuracy, reliability, or generalizability of the study’s findings.
It is important to identify and discuss limitations in research because doing so helps to ensure that the results are interpreted appropriately and that any conclusions drawn are supported by the available evidence. Limitations can also suggest areas for future research, highlight potential biases or confounding factors that may have affected the results, and provide context for the study’s findings.
Generally, limitations should be discussed in the conclusion section of a research paper or thesis, although they may also be mentioned in other sections, such as the introduction or methods. The specific limitations that are discussed will depend on the nature of the study, the research question being investigated, and the data that was collected.
Examples of limitations that might be discussed in research include sample size limitations, data collection methods, the validity and reliability of measures used, and potential biases or confounding factors that could have affected the results. It is important to note that limitations should not be used as a justification for poor research design or methodology, but rather as a way to enhance the understanding and interpretation of the study’s findings.

Importance of Limitations in Research
Here are some reasons why limitations are important in research:
- Enhances the credibility of research: Limitations highlight the potential weaknesses and threats to validity, which helps readers to understand the scope and boundaries of the study. This improves the credibility of research by acknowledging its limitations and providing a clear picture of what can and cannot be concluded from the study.
- Facilitates replication: By highlighting the limitations, researchers can provide detailed information about the study’s methodology, data collection, and analysis. This information helps other researchers to replicate the study and test the validity of the findings, which enhances the reliability of research.
- Guides future research : Limitations provide insights into areas for future research by identifying gaps or areas that require further investigation. This can help researchers to design more comprehensive and effective studies that build on existing knowledge.
- Provides a balanced view: Limitations help to provide a balanced view of the research by highlighting both strengths and weaknesses. This ensures that readers have a clear understanding of the study’s limitations and can make informed decisions about the generalizability and applicability of the findings.
Advantages of Limitations in Research
Here are some potential advantages of limitations in research:
- Focus : Limitations can help researchers focus their study on a specific area or population, which can make the research more relevant and useful.
- Realism : Limitations can make a study more realistic by reflecting the practical constraints and challenges of conducting research in the real world.
- Innovation : Limitations can spur researchers to be more innovative and creative in their research design and methodology, as they search for ways to work around the limitations.
- Rigor : Limitations can actually increase the rigor and credibility of a study, as researchers are forced to carefully consider the potential sources of bias and error, and address them to the best of their abilities.
- Generalizability : Limitations can actually improve the generalizability of a study by ensuring that it is not overly focused on a specific sample or situation, and that the results can be applied more broadly.
About the author
Muhammad Hassan
Researcher, Academic Writer, Web developer
You may also like

Research Report – Example, Writing Guide and...

Evaluating Research – Process, Examples and...

Research Paper Outline – Types, Example, Template

Research Paper Title Page – Example and Making...

Research Paper Format – Types, Examples and...

Research Findings – Types Examples and Writing...
- USC Libraries
- Research Guides
Organizing Your Social Sciences Research Paper
- Limitations of the Study
- Purpose of Guide
- Design Flaws to Avoid
- Independent and Dependent Variables
- Glossary of Research Terms
- Reading Research Effectively
- Narrowing a Topic Idea
- Broadening a Topic Idea
- Extending the Timeliness of a Topic Idea
- Academic Writing Style
- Applying Critical Thinking
- Choosing a Title
- Making an Outline
- Paragraph Development
- Research Process Video Series
- Executive Summary
- The C.A.R.S. Model
- Background Information
- The Research Problem/Question
- Theoretical Framework
- Citation Tracking
- Content Alert Services
- Evaluating Sources
- Primary Sources
- Secondary Sources
- Tiertiary Sources
- Scholarly vs. Popular Publications
- Qualitative Methods
- Quantitative Methods
- Insiderness
- Using Non-Textual Elements
- Common Grammar Mistakes
- Writing Concisely
- Avoiding Plagiarism
- Footnotes or Endnotes?
- Further Readings
- Generative AI and Writing
- USC Libraries Tutorials and Other Guides
- Bibliography
The limitations of the study are those characteristics of design or methodology that impacted or influenced the interpretation of the findings from your research. Study limitations are the constraints placed on the ability to generalize from the results, to further describe applications to practice, and/or related to the utility of findings that are the result of the ways in which you initially chose to design the study or the method used to establish internal and external validity or the result of unanticipated challenges that emerged during the study.
Price, James H. and Judy Murnan. “Research Limitations and the Necessity of Reporting Them.” American Journal of Health Education 35 (2004): 66-67; Theofanidis, Dimitrios and Antigoni Fountouki. "Limitations and Delimitations in the Research Process." Perioperative Nursing 7 (September-December 2018): 155-163. .
Importance of...
Always acknowledge a study's limitations. It is far better that you identify and acknowledge your study’s limitations than to have them pointed out by your professor and have your grade lowered because you appeared to have ignored them or didn't realize they existed.
Keep in mind that acknowledgment of a study's limitations is an opportunity to make suggestions for further research. If you do connect your study's limitations to suggestions for further research, be sure to explain the ways in which these unanswered questions may become more focused because of your study.
Acknowledgment of a study's limitations also provides you with opportunities to demonstrate that you have thought critically about the research problem, understood the relevant literature published about it, and correctly assessed the methods chosen for studying the problem. A key objective of the research process is not only discovering new knowledge but also to confront assumptions and explore what we don't know.
Claiming limitations is a subjective process because you must evaluate the impact of those limitations . Don't just list key weaknesses and the magnitude of a study's limitations. To do so diminishes the validity of your research because it leaves the reader wondering whether, or in what ways, limitation(s) in your study may have impacted the results and conclusions. Limitations require a critical, overall appraisal and interpretation of their impact. You should answer the question: do these problems with errors, methods, validity, etc. eventually matter and, if so, to what extent?
Price, James H. and Judy Murnan. “Research Limitations and the Necessity of Reporting Them.” American Journal of Health Education 35 (2004): 66-67; Structure: How to Structure the Research Limitations Section of Your Dissertation. Dissertations and Theses: An Online Textbook. Laerd.com.
Descriptions of Possible Limitations
All studies have limitations . However, it is important that you restrict your discussion to limitations related to the research problem under investigation. For example, if a meta-analysis of existing literature is not a stated purpose of your research, it should not be discussed as a limitation. Do not apologize for not addressing issues that you did not promise to investigate in the introduction of your paper.
Here are examples of limitations related to methodology and the research process you may need to describe and discuss how they possibly impacted your results. Note that descriptions of limitations should be stated in the past tense because they were discovered after you completed your research.
Possible Methodological Limitations
- Sample size -- the number of the units of analysis you use in your study is dictated by the type of research problem you are investigating. Note that, if your sample size is too small, it will be difficult to find significant relationships from the data, as statistical tests normally require a larger sample size to ensure a representative distribution of the population and to be considered representative of groups of people to whom results will be generalized or transferred. Note that sample size is generally less relevant in qualitative research if explained in the context of the research problem.
- Lack of available and/or reliable data -- a lack of data or of reliable data will likely require you to limit the scope of your analysis, the size of your sample, or it can be a significant obstacle in finding a trend and a meaningful relationship. You need to not only describe these limitations but provide cogent reasons why you believe data is missing or is unreliable. However, don’t just throw up your hands in frustration; use this as an opportunity to describe a need for future research based on designing a different method for gathering data.
- Lack of prior research studies on the topic -- citing prior research studies forms the basis of your literature review and helps lay a foundation for understanding the research problem you are investigating. Depending on the currency or scope of your research topic, there may be little, if any, prior research on your topic. Before assuming this to be true, though, consult with a librarian! In cases when a librarian has confirmed that there is little or no prior research, you may be required to develop an entirely new research typology [for example, using an exploratory rather than an explanatory research design ]. Note again that discovering a limitation can serve as an important opportunity to identify new gaps in the literature and to describe the need for further research.
- Measure used to collect the data -- sometimes it is the case that, after completing your interpretation of the findings, you discover that the way in which you gathered data inhibited your ability to conduct a thorough analysis of the results. For example, you regret not including a specific question in a survey that, in retrospect, could have helped address a particular issue that emerged later in the study. Acknowledge the deficiency by stating a need for future researchers to revise the specific method for gathering data.
- Self-reported data -- whether you are relying on pre-existing data or you are conducting a qualitative research study and gathering the data yourself, self-reported data is limited by the fact that it rarely can be independently verified. In other words, you have to the accuracy of what people say, whether in interviews, focus groups, or on questionnaires, at face value. However, self-reported data can contain several potential sources of bias that you should be alert to and note as limitations. These biases become apparent if they are incongruent with data from other sources. These are: (1) selective memory [remembering or not remembering experiences or events that occurred at some point in the past]; (2) telescoping [recalling events that occurred at one time as if they occurred at another time]; (3) attribution [the act of attributing positive events and outcomes to one's own agency, but attributing negative events and outcomes to external forces]; and, (4) exaggeration [the act of representing outcomes or embellishing events as more significant than is actually suggested from other data].
Possible Limitations of the Researcher
- Access -- if your study depends on having access to people, organizations, data, or documents and, for whatever reason, access is denied or limited in some way, the reasons for this needs to be described. Also, include an explanation why being denied or limited access did not prevent you from following through on your study.
- Longitudinal effects -- unlike your professor, who can literally devote years [even a lifetime] to studying a single topic, the time available to investigate a research problem and to measure change or stability over time is constrained by the due date of your assignment. Be sure to choose a research problem that does not require an excessive amount of time to complete the literature review, apply the methodology, and gather and interpret the results. If you're unsure whether you can complete your research within the confines of the assignment's due date, talk to your professor.
- Cultural and other type of bias -- we all have biases, whether we are conscience of them or not. Bias is when a person, place, event, or thing is viewed or shown in a consistently inaccurate way. Bias is usually negative, though one can have a positive bias as well, especially if that bias reflects your reliance on research that only support your hypothesis. When proof-reading your paper, be especially critical in reviewing how you have stated a problem, selected the data to be studied, what may have been omitted, the manner in which you have ordered events, people, or places, how you have chosen to represent a person, place, or thing, to name a phenomenon, or to use possible words with a positive or negative connotation. NOTE : If you detect bias in prior research, it must be acknowledged and you should explain what measures were taken to avoid perpetuating that bias. For example, if a previous study only used boys to examine how music education supports effective math skills, describe how your research expands the study to include girls.
- Fluency in a language -- if your research focuses , for example, on measuring the perceived value of after-school tutoring among Mexican-American ESL [English as a Second Language] students and you are not fluent in Spanish, you are limited in being able to read and interpret Spanish language research studies on the topic or to speak with these students in their primary language. This deficiency should be acknowledged.
Aguinis, Hermam and Jeffrey R. Edwards. “Methodological Wishes for the Next Decade and How to Make Wishes Come True.” Journal of Management Studies 51 (January 2014): 143-174; Brutus, Stéphane et al. "Self-Reported Limitations and Future Directions in Scholarly Reports: Analysis and Recommendations." Journal of Management 39 (January 2013): 48-75; Senunyeme, Emmanuel K. Business Research Methods. Powerpoint Presentation. Regent University of Science and Technology; ter Riet, Gerben et al. “All That Glitters Isn't Gold: A Survey on Acknowledgment of Limitations in Biomedical Studies.” PLOS One 8 (November 2013): 1-6.
Structure and Writing Style
Information about the limitations of your study are generally placed either at the beginning of the discussion section of your paper so the reader knows and understands the limitations before reading the rest of your analysis of the findings, or, the limitations are outlined at the conclusion of the discussion section as an acknowledgement of the need for further study. Statements about a study's limitations should not be buried in the body [middle] of the discussion section unless a limitation is specific to something covered in that part of the paper. If this is the case, though, the limitation should be reiterated at the conclusion of the section.
If you determine that your study is seriously flawed due to important limitations , such as, an inability to acquire critical data, consider reframing it as an exploratory study intended to lay the groundwork for a more complete research study in the future. Be sure, though, to specifically explain the ways that these flaws can be successfully overcome in a new study.
But, do not use this as an excuse for not developing a thorough research paper! Review the tab in this guide for developing a research topic . If serious limitations exist, it generally indicates a likelihood that your research problem is too narrowly defined or that the issue or event under study is too recent and, thus, very little research has been written about it. If serious limitations do emerge, consult with your professor about possible ways to overcome them or how to revise your study.
When discussing the limitations of your research, be sure to:
- Describe each limitation in detailed but concise terms;
- Explain why each limitation exists;
- Provide the reasons why each limitation could not be overcome using the method(s) chosen to acquire or gather the data [cite to other studies that had similar problems when possible];
- Assess the impact of each limitation in relation to the overall findings and conclusions of your study; and,
- If appropriate, describe how these limitations could point to the need for further research.
Remember that the method you chose may be the source of a significant limitation that has emerged during your interpretation of the results [for example, you didn't interview a group of people that you later wish you had]. If this is the case, don't panic. Acknowledge it, and explain how applying a different or more robust methodology might address the research problem more effectively in a future study. A underlying goal of scholarly research is not only to show what works, but to demonstrate what doesn't work or what needs further clarification.
Aguinis, Hermam and Jeffrey R. Edwards. “Methodological Wishes for the Next Decade and How to Make Wishes Come True.” Journal of Management Studies 51 (January 2014): 143-174; Brutus, Stéphane et al. "Self-Reported Limitations and Future Directions in Scholarly Reports: Analysis and Recommendations." Journal of Management 39 (January 2013): 48-75; Ioannidis, John P.A. "Limitations are not Properly Acknowledged in the Scientific Literature." Journal of Clinical Epidemiology 60 (2007): 324-329; Pasek, Josh. Writing the Empirical Social Science Research Paper: A Guide for the Perplexed. January 24, 2012. Academia.edu; Structure: How to Structure the Research Limitations Section of Your Dissertation. Dissertations and Theses: An Online Textbook. Laerd.com; What Is an Academic Paper? Institute for Writing Rhetoric. Dartmouth College; Writing the Experimental Report: Methods, Results, and Discussion. The Writing Lab and The OWL. Purdue University.
Writing Tip
Don't Inflate the Importance of Your Findings!
After all the hard work and long hours devoted to writing your research paper, it is easy to get carried away with attributing unwarranted importance to what you’ve done. We all want our academic work to be viewed as excellent and worthy of a good grade, but it is important that you understand and openly acknowledge the limitations of your study. Inflating the importance of your study's findings could be perceived by your readers as an attempt hide its flaws or encourage a biased interpretation of the results. A small measure of humility goes a long way!
Another Writing Tip
Negative Results are Not a Limitation!
Negative evidence refers to findings that unexpectedly challenge rather than support your hypothesis. If you didn't get the results you anticipated, it may mean your hypothesis was incorrect and needs to be reformulated. Or, perhaps you have stumbled onto something unexpected that warrants further study. Moreover, the absence of an effect may be very telling in many situations, particularly in experimental research designs. In any case, your results may very well be of importance to others even though they did not support your hypothesis. Do not fall into the trap of thinking that results contrary to what you expected is a limitation to your study. If you carried out the research well, they are simply your results and only require additional interpretation.
Lewis, George H. and Jonathan F. Lewis. “The Dog in the Night-Time: Negative Evidence in Social Research.” The British Journal of Sociology 31 (December 1980): 544-558.
Yet Another Writing Tip
Sample Size Limitations in Qualitative Research
Sample sizes are typically smaller in qualitative research because, as the study goes on, acquiring more data does not necessarily lead to more information. This is because one occurrence of a piece of data, or a code, is all that is necessary to ensure that it becomes part of the analysis framework. However, it remains true that sample sizes that are too small cannot adequately support claims of having achieved valid conclusions and sample sizes that are too large do not permit the deep, naturalistic, and inductive analysis that defines qualitative inquiry. Determining adequate sample size in qualitative research is ultimately a matter of judgment and experience in evaluating the quality of the information collected against the uses to which it will be applied and the particular research method and purposeful sampling strategy employed. If the sample size is found to be a limitation, it may reflect your judgment about the methodological technique chosen [e.g., single life history study versus focus group interviews] rather than the number of respondents used.
Boddy, Clive Roland. "Sample Size for Qualitative Research." Qualitative Market Research: An International Journal 19 (2016): 426-432; Huberman, A. Michael and Matthew B. Miles. "Data Management and Analysis Methods." In Handbook of Qualitative Research . Norman K. Denzin and Yvonna S. Lincoln, eds. (Thousand Oaks, CA: Sage, 1994), pp. 428-444; Blaikie, Norman. "Confounding Issues Related to Determining Sample Size in Qualitative Research." International Journal of Social Research Methodology 21 (2018): 635-641; Oppong, Steward Harrison. "The Problem of Sampling in qualitative Research." Asian Journal of Management Sciences and Education 2 (2013): 202-210.
- << Previous: 8. The Discussion
- Next: 9. The Conclusion >>
- Last Updated: Sep 27, 2024 1:09 PM
- URL: https://libguides.usc.edu/writingguide
A Roadmap to Defining Research Scope and Limitations: A Step-by-Step Guide for Education Professionals
Defining the scope and limitations of a research project is essential for ensuring that education professionals can design focused and practical studies. The ability to properly delineate the boundaries of a study ensures that researchers maintain a clear vision of the project’s goals and lays the groundwork for a successful conclusion. In this article, we will outline a step-by-step process for defining the scope and limitations of a research project to guide education professionals toward constructing valid, reliable, and meaningful results.
Table of Contents
Step 1: Identify the Research Topic and Problem Statement
The first step entails pinpointing the research topic and developing a concise problem statement that addresses a knowledge gap in education. To achieve this, researchers need to:
- Conduct a thorough literature review to identify current research and existing gaps.
- Develop a problem statement that should be concise, specific, and easily understood by those within the field.
The problem statement will be used as a basis for the entire research process, providing a clear focus for the study.
Step 2: Determine the Research Objectives and Questions
Once the problem statement has been crafted, the next step is to outline the research objectives and questions to guide the investigation. These objectives should be:
- Aligned with the problem statement
- Measurable and attainable
- Specific to the given research topic
Having well-defined research objectives and questions will assist in creating a well-structured study that seeks to answer specific queries and make a significant academic contribution.
Step 3: Establish the Research Scope
Researchers must then establish the scope of the study. Scope delineation is vital in determining the study’s extent, depth, and boundaries. Several factors influence the research scope, including time, resources, and goals. To define the research scope, consider the following aspects:
- Timeframe: Determine the specific period the research should be conducted and completed.
- Target Population: Identify the particular group or population for which the research will be relevant.
- Geographical Location: Specify the area or region where the research will be carried out.
- Conceptual Coverage: Identify the main concepts, theories, or variables under investigation.
By outlining the research scope, researchers can maintain a focus on the most critical aspects of their study, ensuring that the conclusions answer the initial problem statement effectively.
Step 4: Define the Research Limitations
After establishing the research scope, researchers should identify potential limitations impacting the study’s validity, reliability, or generalizability. Limitations are typically outside the researcher’s control but must be acknowledged to provide an appropriate context for the research findings. Some common research limitations include the following:
- Sample Size: A small sample size may limit the generalizability of the study findings to the broader population.
- Time Constraints: Limited data collection or analysis time may influence the findings’ comprehensiveness.
- Financial Constraints: Insufficient funds for conducting a large-scale study may result in narrow or inadequate findings.
- Accessibility of Participants: The availability of participants for data collection may not be representative of the broader population.
- Methodological Constraints: The chosen research design may only be suitable for answering some aspects of the research question.
By identifying and acknowledging these limitations, researchers ensure that their findings can be evaluated in the proper context.
Step 5: Revise Research Objectives and Questions Based on Scope and Limitations
Once the scope and limitations have been identified, researchers must revisit their research objectives and questions to guarantee alignment. Any changes resulting from this process should be documented and justified by referring to the identified scope and limitations. Researchers must ensure that the revised objectives and questions are:
- Aligned with the revised scope
- Specific and focused
- Attainable within the project’s constraints
Step 6: Seek Feedback from Peers and Experts
As a final step, researchers should seek feedback from their colleagues and experts in their field to validate their scope and limitations. These consultations can offer valuable insights and suggestions for refining the research boundaries and identifying potential pitfalls related to the project’s scope and limitations.
Defining research scope and limitations is crucial for education professionals to ensure that their studies make meaningful contributions to their field. By following the step-by-step process presented in this article, researchers can develop a well-structured study design that encompasses a clear focus, attainable objectives, and defined boundaries. Acknowledging and addressing limitations further enhances the study’s validity and reliability, positively impacting the research’s quality and relevance.
Mark Anthony Llego
Mark Anthony Llego, a visionary from the Philippines, founded TeacherPH in October 2014 with a mission to transform the educational landscape. His platform has empowered thousands of Filipino teachers, providing them with crucial resources and a space for meaningful idea exchange, ultimately enhancing their instructional and supervisory capabilities. TeacherPH's influence extends far beyond its origins. Mark's insightful articles on education have garnered international attention, featuring on respected U.S. educational websites. Moreover, his work has become a valuable reference for researchers, contributing to the academic discourse on education.
Leave a Comment Cancel reply
Can't find what you're looking for.
We are here to help - please use the search box below.
Frequently asked questions
How do i determine scope of research.
Scope of research is determined at the beginning of your research process , prior to the data collection stage. Sometimes called “scope of study,” your scope delineates what will and will not be covered in your project. It helps you focus your work and your time, ensuring that you’ll be able to achieve your goals and outcomes.
Defining a scope can be very useful in any research project, from a research proposal to a thesis or dissertation . A scope is needed for all types of research: quantitative , qualitative , and mixed methods .
To define your scope of research, consider the following:
- Budget constraints or any specifics of grant funding
- Your proposed timeline and duration
- Specifics about your population of study, your proposed sample size , and the research methodology you’ll pursue
- Any inclusion and exclusion criteria
- Any anticipated control , extraneous , or confounding variables that could bias your research if not accounted for properly.
Ask our team
Want to contact us directly? No problem. We are always here for you.
- Email [email protected]
- Start live chat
- Call +1 (510) 822-8066
- WhatsApp +31 20 261 6040
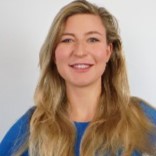
Our team helps students graduate by offering:
- A world-class citation generator
- Plagiarism Checker software powered by Turnitin
- Innovative Citation Checker software
- Professional proofreading services
- Over 300 helpful articles about academic writing, citing sources, plagiarism, and more
Scribbr specializes in editing study-related documents . We proofread:
- PhD dissertations
- Research proposals
- Personal statements
- Admission essays
- Motivation letters
- Reflection papers
- Journal articles
- Capstone projects
Scribbr’s Plagiarism Checker is powered by elements of Turnitin’s Similarity Checker , namely the plagiarism detection software and the Internet Archive and Premium Scholarly Publications content databases .
The add-on AI detector is powered by Scribbr’s proprietary software.
The Scribbr Citation Generator is developed using the open-source Citation Style Language (CSL) project and Frank Bennett’s citeproc-js . It’s the same technology used by dozens of other popular citation tools, including Mendeley and Zotero.
You can find all the citation styles and locales used in the Scribbr Citation Generator in our publicly accessible repository on Github .

Stating the Obvious: Writing Assumptions, Limitations, and Delimitations

During the process of writing your thesis or dissertation, you might suddenly realize that your research has inherent flaws. Don’t worry! Virtually all projects contain restrictions to your research. However, being able to recognize and accurately describe these problems is the difference between a true researcher and a grade-school kid with a science-fair project. Concerns with truthful responding, access to participants, and survey instruments are just a few of examples of restrictions on your research. In the following sections, the differences among delimitations, limitations, and assumptions of a dissertation will be clarified.
Delimitations
Delimitations are the definitions you set as the boundaries of your own thesis or dissertation, so delimitations are in your control. Delimitations are set so that your goals do not become impossibly large to complete. Examples of delimitations include objectives, research questions, variables, theoretical objectives that you have adopted, and populations chosen as targets to study. When you are stating your delimitations, clearly inform readers why you chose this course of study. The answer might simply be that you were curious about the topic and/or wanted to improve standards of a professional field by revealing certain findings. In any case, you should clearly list the other options available and the reasons why you did not choose these options immediately after you list your delimitations. You might have avoided these options for reasons of practicality, interest, or relativity to the study at hand. For example, you might have only studied Hispanic mothers because they have the highest rate of obese babies. Delimitations are often strongly related to your theory and research questions. If you were researching whether there are different parenting styles between unmarried Asian, Caucasian, African American, and Hispanic women, then a delimitation of your study would be the inclusion of only participants with those demographics and the exclusion of participants from other demographics such as men, married women, and all other ethnicities of single women (inclusion and exclusion criteria). A further delimitation might be that you only included closed-ended Likert scale responses in the survey, rather than including additional open-ended responses, which might make some people more willing to take and complete your survey. Remember that delimitations are not good or bad. They are simply a detailed description of the scope of interest for your study as it relates to the research design. Don’t forget to describe the philosophical framework you used throughout your study, which also delimits your study.
Limitations
Limitations of a dissertation are potential weaknesses in your study that are mostly out of your control, given limited funding, choice of research design, statistical model constraints, or other factors. In addition, a limitation is a restriction on your study that cannot be reasonably dismissed and can affect your design and results. Do not worry about limitations because limitations affect virtually all research projects, as well as most things in life. Even when you are going to your favorite restaurant, you are limited by the menu choices. If you went to a restaurant that had a menu that you were craving, you might not receive the service, price, or location that makes you enjoy your favorite restaurant. If you studied participants’ responses to a survey, you might be limited in your abilities to gain the exact type or geographic scope of participants you wanted. The people whom you managed to get to take your survey may not truly be a random sample, which is also a limitation. If you used a common test for data findings, your results are limited by the reliability of the test. If your study was limited to a certain amount of time, your results are affected by the operations of society during that time period (e.g., economy, social trends). It is important for you to remember that limitations of a dissertation are often not something that can be solved by the researcher. Also, remember that whatever limits you also limits other researchers, whether they are the largest medical research companies or consumer habits corporations. Certain kinds of limitations are often associated with the analytical approach you take in your research, too. For example, some qualitative methods like heuristics or phenomenology do not lend themselves well to replicability. Also, most of the commonly used quantitative statistical models can only determine correlation, but not causation.
Assumptions
Assumptions are things that are accepted as true, or at least plausible, by researchers and peers who will read your dissertation or thesis. In other words, any scholar reading your paper will assume that certain aspects of your study is true given your population, statistical test, research design, or other delimitations. For example, if you tell your friend that your favorite restaurant is an Italian place, your friend will assume that you don’t go there for the sushi. It’s assumed that you go there to eat Italian food. Because most assumptions are not discussed in-text, assumptions that are discussed in-text are discussed in the context of the limitations of your study, which is typically in the discussion section. This is important, because both assumptions and limitations affect the inferences you can draw from your study. One of the more common assumptions made in survey research is the assumption of honesty and truthful responses. However, for certain sensitive questions this assumption may be more difficult to accept, in which case it would be described as a limitation of the study. For example, asking people to report their criminal behavior in a survey may not be as reliable as asking people to report their eating habits. It is important to remember that your limitations and assumptions should not contradict one another. For instance, if you state that generalizability is a limitation of your study given that your sample was limited to one city in the United States, then you should not claim generalizability to the United States population as an assumption of your study. Statistical models in quantitative research designs are accompanied with assumptions as well, some more strict than others. These assumptions generally refer to the characteristics of the data, such as distributions, correlational trends, and variable type, just to name a few. Violating these assumptions can lead to drastically invalid results, though this often depends on sample size and other considerations.
Click here to cancel reply.
You must be logged in to post a comment.
Copyright © 2024 PhDStudent.com. All rights reserved. Designed by Divergent Web Solutions, LLC .
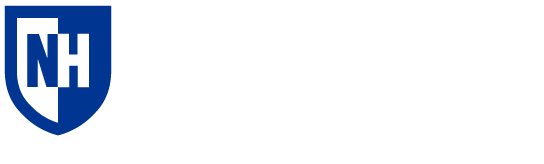
CPS Online Graduate Studies Research Paper (UNH Manchester Library): Limitations of the Study
- Overview of the Research Process for Capstone Projects
- Types of Research Design
- Selecting a Research Problem
- The Title of Your Research Paper
- Before You Begin Writing
- 7 Parts of the Research Paper
- Background Information
- Quanitative and Qualitative Methods
- Qualitative Methods
- Quanitative Methods
- Resources to Help You With the Literature Review
- Non-Textual Elements
Limitations of the Study
- Format of Capstone Research Projects at GSC
- Editing and Proofreading Your Paper
- Acknowledgements
- UNH Scholar's Repository
The limitations of the study are those characteristics of design or methodology that impacted or influenced the interpretation of the findings from your research. They are the constraints on generalizability, applications to practice, and/or utility of findings that are the result of the ways in which you initially chose to design the study and/or the method used to establish internal and external validity.
Price, James H. and Judy Murnan. “Research Limitations and the Necessity of Reporting Them.” American Journal of Health Education 35 (2004): 66-67.
Always acknowledge a study's limitations. It is far better that you identify and acknowledge your study’s limitations than to have them pointed out by your professor and be graded down because you appear to have ignored them.
Keep in mind that acknowledgement of a study's limitations is an opportunity to make suggestions for further research. If you do connect your study's limitations to suggestions for further research, be sure to explain the ways in which these unanswered questions may become more focused because of your study.
Acknowledgement of a study's limitations also provides you with an opportunity to demonstrate that you have thought critically about the research problem, understood the relevant literature published about it, and correctly assessed the methods chosen for studying the problem. A key objective of the research process is not only discovering new knowledge but to also confront assumptions and explore what we don't know.
Claiming limitations is a subjective process because you must evaluate the impact of those limitations . Don't just list key weaknesses and the magnitude of a study's limitations. To do so diminishes the validity of your research because it leaves the reader wondering whether, or in what ways, limitation(s) in your study may have impacted the results and conclusions. Limitations require a critical, overall appraisal and interpretation of their impact. You should answer the question: do these problems with errors, methods, validity, etc. eventually matter and, if so, to what extent?
Price, James H. and Judy Murnan. “Research Limitations and the Necessity of Reporting Them.” American Journal of Health Education 35 (2004): 66-67; Structure: How to Structure the Research Limitations Section of Your Dissertation . Dissertations and Theses: An Online Textbook. Laerd.com.
Descriptions of Possible Limitations
All studies have limitations . However, it is important that you restrict your discussion to limitations related to the research problem under investigation. For example, if a meta-analysis of existing literature is not a stated purpose of your research, it should not be discussed as a limitation. Do not apologize for not addressing issues that you did not promise to investigate in the introduction of your paper.
Here are examples of limitations related to methodology and the research process you may need to describe and to discuss how they possibly impacted your results. Descriptions of limitations should be stated in the past tense because they were discovered after you completed your research.
Possible Methodological Limitations
- Sample size -- the number of the units of analysis you use in your study is dictated by the type of research problem you are investigating. Note that, if your sample size is too small, it will be difficult to find significant relationships from the data, as statistical tests normally require a larger sample size to ensure a representative distribution of the population and to be considered representative of groups of people to whom results will be generalized or transferred. Note that sample size is less relevant in qualitative research.
- Lack of available and/or reliable data -- a lack of data or of reliable data will likely require you to limit the scope of your analysis, the size of your sample, or it can be a significant obstacle in finding a trend and a meaningful relationship. You need to not only describe these limitations but to offer reasons why you believe data is missing or is unreliable. However, don’t just throw up your hands in frustration; use this as an opportunity to describe the need for future research.
- Lack of prior research studies on the topic -- citing prior research studies forms the basis of your literature review and helps lay a foundation for understanding the research problem you are investigating. Depending on the currency or scope of your research topic, there may be little, if any, prior research on your topic. Before assuming this to be true, though, consult with a librarian. In cases when a librarian has confirmed that there is no prior research, you may be required to develop an entirely new research typology [for example, using an exploratory rather than an explanatory research design]. Note again that discovering a limitation can serve as an important opportunity to identify new gaps in the literature and to describe the need for further research.
- Measure used to collect the data -- sometimes it is the case that, after completing your interpretation of the findings, you discover that the way in which you gathered data inhibited your ability to conduct a thorough analysis of the results. For example, you regret not including a specific question in a survey that, in retrospect, could have helped address a particular issue that emerged later in the study. Acknowledge the deficiency by stating a need for future researchers to revise the specific method for gathering data.
- Self-reported data -- whether you are relying on pre-existing data or you are conducting a qualitative research study and gathering the data yourself, self-reported data is limited by the fact that it rarely can be independently verified. In other words, you have to take what people say, whether in interviews, focus groups, or on questionnaires, at face value. However, self-reported data can contain several potential sources of bias that you should be alert to and note as limitations. These biases become apparent if they are incongruent with data from other sources. These are: (1) selective memory [remembering or not remembering experiences or events that occurred at some point in the past]; (2) telescoping [recalling events that occurred at one time as if they occurred at another time]; (3) attribution [the act of attributing positive events and outcomes to one's own agency but attributing negative events and outcomes to external forces]; and, (4) exaggeration [the act of representing outcomes or embellishing events as more significant than is actually suggested from other data].
Possible Limitations of the Researcher
- Access -- if your study depends on having access to people, organizations, or documents and, for whatever reason, access is denied or limited in some way, the reasons for this need to be described.
- Longitudinal effects -- unlike your professor, who can literally devote years [even a lifetime] to studying a single topic, the time available to investigate a research problem and to measure change or stability over time is pretty much constrained by the due date of your assignment. Be sure to choose a research problem that does not require an excessive amount of time to complete the literature review, apply the methodology, and gather and interpret the results. If you're unsure whether you can complete your research within the confines of the assignment's due date, talk to your professor.
- Cultural and other type of bias -- we all have biases, whether we are conscience of them or not. Bias is when a person, place, or thing is viewed or shown in a consistently inaccurate way. Bias is usually negative, though one can have a positive bias as well, especially if that bias reflects your reliance on research that only support for your hypothesis. When proof-reading your paper, be especially critical in reviewing how you have stated a problem, selected the data to be studied, what may have been omitted, the manner in which you have ordered events, people, or places, how you have chosen to represent a person, place, or thing, to name a phenomenon, or to use possible words with a positive or negative connotation.
NOTE: If you detect bias in prior research, it must be acknowledged and you should explain what measures were taken to avoid perpetuating that bias.
- Fluency in a language -- if your research focuses on measuring the perceived value of after-school tutoring among Mexican-American ESL [English as a Second Language] students, for example, and you are not fluent in Spanish, you are limited in being able to read and interpret Spanish language research studies on the topic. This deficiency should be acknowledged.
Aguinis, Hermam and Jeffrey R. Edwards. “Methodological Wishes for the Next Decade and How to Make Wishes Come True.” Journal of Management Studies 51 (January 2014): 143-174; Brutus, Stéphane et al. "Self-Reported Limitations and Future Directions in Scholarly Reports: Analysis and Recommendations." Journal of Management 39 (January 2013): 48-75; Senunyeme, Emmanuel K. Business Research Methods . Powerpoint Presentation. Regent University of Science and Technology; ter Riet, Gerben et al. “All That Glitters Isn't Gold: A Survey on Acknowledgment of Limitations in Biomedical Studies.” PLOS One 8 (November 2013): 1-6.
Structure and Writing Style
Information about the limitations of your study are generally placed either at the beginning of the discussion section of your paper so the reader knows and understands the limitations before reading the rest of your analysis of the findings, or, the limitations are outlined at the conclusion of the discussion section as an acknowledgement of the need for further study. Statements about a study's limitations should not be buried in the body [middle] of the discussion section unless a limitation is specific to something covered in that part of the paper. If this is the case, though, the limitation should be reiterated at the conclusion of the section. If you determine that your study is seriously flawed due to important limitations, such as, an inability to acquire critical data, consider reframing it as an exploratory study intended to lay the groundwork for a more complete research study in the future. Be sure, though, to specifically explain the ways that these flaws can be successfully overcome in a new study. But, do not use this as an excuse for not developing a thorough research paper! Review the tab in this guide for developing a research topic. If serious limitations exist, it generally indicates a likelihood that your research problem is too narrowly defined or that the issue or event under study is too recent and, thus, very little research has been written about it. If serious limitations do emerge, consult with your professor about possible ways to overcome them or how to revise your study. When discussing the limitations of your research, be sure to: Describe each limitation in detailed but concise terms; Explain why each limitation exists; Provide the reasons why each limitation could not be overcome using the method(s) chosen to acquire or gather the data [cite to other studies that had similar problems when possible]; Assess the impact of each limitation in relation to the overall findings and conclusions of your study; and, If appropriate, describe how these limitations could point to the need for further research. Remember that the method you chose may be the source of a significant limitation that has emerged during your interpretation of the results [for example, you didn't interview a group of people that you later wish you had]. If this is the case, don't panic. Acknowledge it, and explain how applying a different or more robust methodology might address the research problem more effectively in a future study. A underlying goal of scholarly research is not only to show what works, but to demonstrate what doesn't work or what needs further clarification. Aguinis, Hermam and Jeffrey R. Edwards. “Methodological Wishes for the Next Decade and How to Make Wishes Come True.” Journal of Management Studies 51 (January 2014): 143-174; Brutus, Stéphane et al. "Self-Reported Limitations and Future Directions in Scholarly Reports: Analysis and Recommendations." Journal of Management 39 (January 2013): 48-75; Ioannidis, John P.A. "Limitations are not Properly Acknowledged in the Scientific Literature." Journal of Clinical Epidemiology 60 (2007): 324-329; Pasek, Josh. Writing the Empirical Social Science Research Paper: A Guide for the Perplexed. January 24, 2012. Academia.edu; Structure: How to Structure the Research Limitations Section of Your Dissertation. Dissertations and Theses: An Online Textbook. Laerd.com; What Is an Academic Paper? Institute for Writing Rhetoric. Dartmouth College; Writing the Experimental Report: Methods, Results, and Discussion. The Writing Lab and The OWL. Purdue University.
Information about the limitations of your study are generally placed either at the beginning of the discussion section of your paper so the reader knows and understands the limitations before reading the rest of your analysis of the findings, or, the limitations are outlined at the conclusion of the discussion section as an acknowledgement of the need for further study. Statements about a study's limitations should not be buried in the body [middle] of the discussion section unless a limitation is specific to something covered in that part of the paper. If this is the case, though, the limitation should be reiterated at the conclusion of the section.
If you determine that your study is seriously flawed due to important limitations , such as, an inability to acquire critical data, consider reframing it as an exploratory study intended to lay the groundwork for a more complete research study in the future. Be sure, though, to specifically explain the ways that these flaws can be successfully overcome in a new study.
But, do not use this as an excuse for not developing a thorough research paper! Review the tab in this guide for developing a research topic . If serious limitations exist, it generally indicates a likelihood that your research problem is too narrowly defined or that the issue or event under study is too recent and, thus, very little research has been written about it. If serious limitations do emerge, consult with your professor about possible ways to overcome them or how to revise your study.
When discussing the limitations of your research, be sure to:
- Describe each limitation in detailed but concise terms;
- Explain why each limitation exists;
- Provide the reasons why each limitation could not be overcome using the method(s) chosen to acquire or gather the data [cite to other studies that had similar problems when possible];
- Assess the impact of each limitation in relation to the overall findings and conclusions of your study; and,
- If appropriate, describe how these limitations could point to the need for further research.
Remember that the method you chose may be the source of a significant limitation that has emerged during your interpretation of the results [for example, you didn't interview a group of people that you later wish you had]. If this is the case, don't panic. Acknowledge it, and explain how applying a different or more robust methodology might address the research problem more effectively in a future study. A underlying goal of scholarly research is not only to show what works, but to demonstrate what doesn't work or what needs further clarification.
Aguinis, Hermam and Jeffrey R. Edwards. “Methodological Wishes for the Next Decade and How to Make Wishes Come True.” Journal of Management Studies 51 (January 2014): 143-174; Brutus, Stéphane et al. "Self-Reported Limitations and Future Directions in Scholarly Reports: Analysis and Recommendations." Journal of Management 39 (January 2013): 48-75; Ioannidis, John P.A. "Limitations are not Properly Acknowledged in the Scientific Literature." Journal of Clinical Epidemiology 60 (2007): 324-329; Pasek, Josh. Writing the Empirical Social Science Research Paper: A Guide for the Perplexed . January 24, 2012. Academia.edu; Structure: How to Structure the Research Limitations Section of Your Dissertation . Dissertations and Theses: An Online Textbook. Laerd.com; What Is an Academic Paper? Institute for Writing Rhetoric. Dartmouth College; Writing the Experimental Report: Methods, Results, and Discussion . The Writing Lab and The OWL. Purdue University.
- << Previous: The Discussion
- Next: Conclusion >>
- Last Updated: Nov 6, 2023 1:43 PM
- URL: https://libraryguides.unh.edu/cpsonlinegradpaper
Exploring user privacy awareness on GitHub: an empirical study
- Open access
- Published: 27 September 2024
- Volume 29 , article number 156 , ( 2024 )
Cite this article
You have full access to this open access article
- Costanza Alfieri ORCID: orcid.org/0000-0002-5082-3844 1 ,
- Juri Di Rocco ORCID: orcid.org/0000-0002-7909-3902 1 ,
- Paola Inverardi ORCID: orcid.org/0000-0001-6734-1318 2 &
- Phuong T. Nguyen ORCID: orcid.org/0000-0002-3666-4162 1
GitHub provides developers with a practical way to distribute source code and collaboratively work on common projects. To enhance account security and privacy, GitHub allows its users to manage access permissions, review audit logs, and enable two-factor authentication. However, despite the endless effort, the platform still faces various issues related to the privacy of its users. This paper presents an empirical study delving into the GitHub ecosystem. Our focus is on investigating the utilization of privacy settings on the platform and identifying various types of sensitive information disclosed by users. Leveraging a dataset comprising 6,132 developers, we report and analyze their activities by means of comments on pull requests. Our findings indicate an active engagement by users with the available privacy settings on GitHub. Notably, we observe the disclosure of different forms of private information within pull request comments. This observation has prompted our exploration into sensitivity detection using a large language model and BERT, to pave the way for a personalized privacy assistant. Our work provides insights into the utilization of existing privacy protection tools, such as privacy settings, along with their inherent limitations. Essentially, we aim to advance research in this field by providing both the motivation for creating such privacy protection tools and a proposed methodology for personalizing them.
Explore related subjects
- Artificial Intelligence
Avoid common mistakes on your manuscript.
1 Introduction
In recent years, privacy in the digital world has become a major concern. Regulations like the EU General Data Protection Regulation (GDPR), the California Consumer Privacy Act (CCPA), or the UK’s Data Protection Act have been ratified to regulate the (ab-)use of sensitive data (Voigt and Von dem Bussche 2017 ; Pardau 2018 ; Jay 2000 ). While being undeniably valuable, it has become clear that regulations alone may not suffice to ensure robust protection of user privacy. Indeed, software platforms provide mechanisms that allow users to set their privacy preferences and that, together with regulation should offer a wider shield to user privacy.
GitHub Footnote 1 is a platform for software developers where a significant amount of professional collaboration and coding takes place. As a matter of fact, it holds vast amounts of data that can be privacy sensitive for its users. GitHub users are first class inhabitants of the digital world and may be considered experts. However, ensuring that they are aware of the sensitivity of personal information that they leave on the platform and that they have control over who can see this data and how it’s used, is crucial for maintaining trust in the platform and protecting users’ interests and safety. Indeed, GitHub offers to users means to declare which pieces of personal information they are willing to make public setting this way their privacy preferences. However, this is not enough provided that in their daily activity users may leave intentionally or unintentionally breadcrumbs of personal information open to the public.
In this paper, we show that diverse pieces of personal information about a specific user can be uncovered on GitHub, and this information may disclose data that are hidden in the user’s privacy settings. In the study, we extend upon concepts introduced in previous research. In particular, we take privacy settings provided on GitHub as a declaration of intent of the user that defines their privacy profile (Inverardi et al. 2023 ; Migliarini et al. 2020 ). Our exploration focuses on determining whether these privacy settings are actively utilized by users and how. We leverage this information to compare it with the actual behaviors of users, particularly what they disclose in their comments. Our objective is to enhance our understanding of the effectiveness of privacy settings and explore any potential correlation with the users’ observable behaviors. Our research offers insights into the utilization of existing privacy protection tools, such as privacy settings, along with their inherent limitations. Given the interest in developing privacy assistants (e.g., Liu et al. ( 2016 )), our research supports this field by providing both the motivation for creating such privacy protection tools and a proposed methodology for personalizing them.
To achieve this, we conducted an analysis of pull_request comments within a subset of GitHub users, namely the Active users (see Section 4.1 ), intending to identify what type of private information can be found on the platform. The choice of restricting to pull_request is motivated by previous studies in Sajadi et al. ( 2023 ); Iyer et al. ( 2019 ) (see Section 2 ). Our examination yielded insights into users’ family info, moral values, workplace details, travel habits, time zones, and more. In conjunction with these findings, we present a dataset comprising both sensitive and non-sensitive comments, each manually labeled into distinct sensitive classes.
In the analysis process, we curated a labeled dataset of pull_requests comments made by the Active users. The novelty of this labeled dataset consists in having labeled “digital behaviour”, that can be linked to certain privacy profiles. The analysis discovered several discrepancies between the user privacy settings and pieces of information disclosed by the user in some comments. These discrepancies may represent instances of the so-called “privacy paradox,” i.e., inconsistencies between users declared preferences and their actual behavior (Barth and de Jong 2017 ; Kokolakis 2017 ), where users appear to be very attentive about their privacy, but eventually in their actual behavior miss to defend their personal data. Alternatively, they may simply indicate a lack of attention/importance of the user for the privacy settings regarding her actual behavior. In both cases, the results show that the way personal privacy is dealt with in GitHub needs to be improved.
To address similar problems, many researchers claim the importance of having automated tools to protect users’ privacy in the digital world (Liu et al. 2016 ; Fukuyama et al. 2021 ; Autili et al. 2019 ).
Our work represents a step forward in this direction allowing for the realization of an awareness tool that can assist the users in composing their textual comments in a privacy consistent way with the user profile. A proof of concepts of such a tool is presented in Section 5 .
To summarize, in this work we answer the following research questions that help understanding the privacy dynamics on GitHub:
RQ \(_1\) : Are the privacy settings provided on GitHub used/adopted by the users? In other terms, do we observe different combinations of these settings, or is there a dominant configuration? We investigate a large set of developers to find out if there exist differences in their privacy preferences. The use of privacy settings and the potential differences between users’ selection indicate a certain attention to their privacy.
RQ \(_2\) : What types of private information are disclosed on GitHub by users? Although designed as a platform for technical purposes, GitHub inherently possesses social media characteristics. Consequently, our study investigates the information that developers disclose, whether intentionally or unintentionally, in their pull_requests comments.
RQ \(_3\) : After users choose their privacy settings, do they adhere to what they have declared? In other words, can we observe a discrepancy between their stated privacy preferences and their actual behavior, such as their textual activity? It is relevant to understand whether there is a mismatch to assess the effectiveness of these privacy settings and to facilitate the development of personalized privacy assistant for users.
RQ \(_4\) : To which extent is it possible to automate the detection of sensitive comments with the use of BERT or Llama2? We explored if the sensitivity detection of textual comments on this platform can be conducted by leveraging models as BERT or Llama2.
The main contributions of our work are summarized below:
We conducted an empirical study on a set of 6,132 developers to investigate the privacy dynamics in the GitHub ecosystem.
Our investigation reveals that users adopt different configurations of privacy settings, showing different privacy concerns.
The empirical study shows that users reveal different types of information about themselves or other developers through pull_request comments. This triggers the need to overhaul the privacy management from the designers of GitHub.
Our ultimate goal is to encourage the development of innovative applications for detecting privacy data leakage. Specifically, we present a proof of concept where we train BERT and we fine-tuned Llama–a large language model (Touvron et al. 2023 ) (LLM)–to automatically assess whether a text discloses sensitive information.
The dataset curated through this paper has been published Footnote 2 to facilitate future research (see Section 8 ).
The paper is structured as follows. In Section 2 , we provide a background on privacy settings on GitHub, a set of vulnerabilities that show the need for techniques and tools to manage privacy settings in the platform, and a real case scenario. Section 3 reviews related work. Section 4 presents the methodology to perform the empirical study on the GitHub ecosystem. Section 5 presents the proof of concepts with BERT and Llama2. The results are reported and analyzed in Section 6 . We discuss the results in Section 7 . Section 8 sketches future work, and concludes the paper.
2 Motivations and Background
In this section, we illustrate the different motivations behind this study and provide background on privacy settings on GitHub and on GitHub privacy vulnerabilities.
2.1 GitHub and its Privacy Settings
We chose to investigate GitHub for different reasons: (i) GitHub is a platform for technical purposes and may appear neutral or devoid of any privacy or ethical concerns. However, “[open source software] is as much social as it is technical” (Vasilescu et al. 2015c ) and there is already evidence from the literature that proves privacy infringements and gender discrimination on the platform (Terrell et al. 2017 ; Meli et al. 2019 ; Ford et al. 2019 ; Niu et al. 2023 ); (ii) On this platform, there is a large availability of data that can be downloaded as well as dataset already processed (Gousios 2013 ); (iii) Furthermore, this platform allows us to access users’ privacy settings thus permitting to compare users’ stated privacy preferences with their actual behaviors. This approach represents a novelty compared to previous studies on the utilization of privacy settings on platforms, which relied solely on user surveys rather than an analysis of actual preference selections (Kanampiu and Anwar 2019 ; Chen et al. 2019 ). The analysis of privacy preferences offers a deeper understanding of user behaviors and the limitations associated with the available privacy settings.
In this study, we concentrated on examining users’ selection of privacy preferences and their self-disclosure of personal information in pull_request comments. Our objective is to analyze the privacy dynamics on GitHub, i.e., whether users effectively used the privacy settings provided, if these settings present any limitations, and if any personal information, intentionally concealed or not, can be inferred from a user’s textual activity on GitHub. Textual activities vary from making a commit, sending a pull_request, commenting on all these different activities. In this study, we focused on the task of commenting on a pull_request. This decision was guided by previous studies highlighting a greater probability of encountering significant user interactions and consequently more sensitive information in pull_request contexts (Sajadi et al. 2023 ; Iyer et al. 2019 ).
According to the GitHub Privacy Statement (see also Fig. 1 ), Footnote 3 a user who does not wish to show all the information available on her GitHub profile, can adjust the privacy settings provided by the platform in order to hide pieces of information.
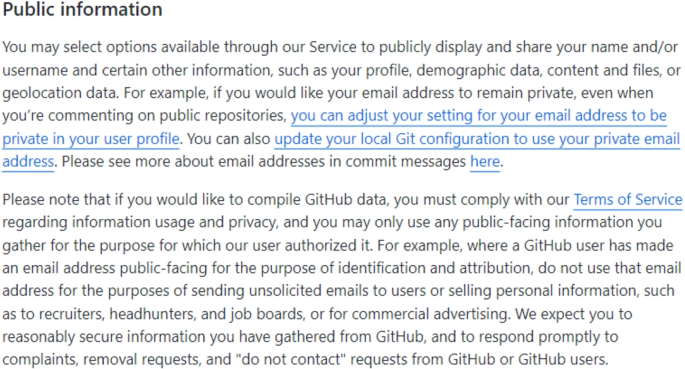
GitHub Privacy Statement on settings
When referring to users’ privacy, we talk about personal users’ privacy, i.e., what they aim to share about their life. On GitHub, these desiderata can be expressed through the privacy settings of the profile. An example of what can be shown or hidden on GitHub is illustrated in Fig. 2 . We consider the totality of the privacy settings selected by the users as their privacy desiderata .
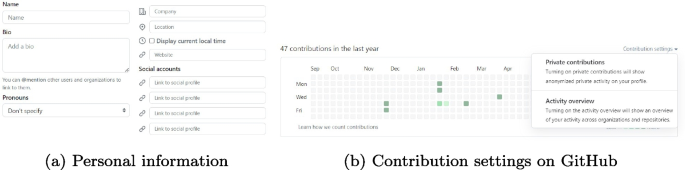
Privacy settings on GitHub
2.2 Privacy Vulnerability in GitHub
Privacy vulnerability arises in several places. Personal information such as email addresses, location, and potentially even real names are often part of a user’s profile. Even if GitHub provides tailored settings for privacy (see Fig. 2 ), the activities of users, such as the repositories they star, the issues they comment on, or the projects they follow, can reveal a lot about their interests, expertise, and professional activities. Due to the social nature of GitHub, user collaborations can be used to identify user’s professional network, which might include sensitive information, especially for those working on confidential or competitive projects.
Privacy vulnerabilities can arise as follows:
The activities of a user who has chosen to set her event settings to private can potentially be discovered by examining the history of repositories to which she has made contributions.
When users make links that expose personal or sensitive information, their privacy desiderata may be compromised.
Public visibility occurs when users fork or star each other’s repositories. These actions provide information about users’ hobbies, projects, or collaborations. Additionally, issue and pull request discussions are public by default, revealing complex collaboration networks. A user frequently addressing user-initiated issues or pull requests, may suggest a relationship beyond GitHub, such as coworkers or acquaintances. Many organizations and teams are open to the public. This could reveal confidential professional relationships or collaborations. The public exposure of the user’s contribution graph, which shows their work patterns and hours, might reveal work habits and inactivity. This information may be linked to job absence or personal activities.
Textual contents such as commit messages, Pull requests, and issue comments may disclose privacy information.
The above-listed privacy vulnerabilities are well-known to researchers who publish under double-blind peer review contexts (Bacchelli and Beller 2017 ). In many research environments, double-blind peer review is pivotal to the integrity of knowledge dissemination. This requires to ensure the anonymity of submission materials, including associated replication packages. On GitHub, users need to anonymize sensitive information to protect privacy while still allowing for the replication of their results. Although GitHub allows users to specify privacy settings for their repositories reducing the likelihood of disclosing user identities, it is necessary to thoroughly analyze repository content, metadata, and textual comments (e.g., commit, issue, and pull request comments) to delete any trace that can reveal identities or affiliations. In this context, external tools are developed to remove user data from repositories, e.g., Anonymous GitHub . Footnote 4 However, the risk of revealing the user identity still exists as those tools mainly apply rewriting rules, where the user should provide the complete list of terms that will be anonymized. Moreover, to the best of our knowledge, no tool is provided for sanitizing textual comments which represent a threat to privacy, as we show in this paper. Ideally, any automated means should take in input the user’s privacy desiderata and then act to support the user in maintaining her privacy desiderata throughout her interactions with the system.
2.3 The Recruiters Problem
It is widely acknowledged that recruiters leverage social networks to gather insights into their candidates’ backgrounds (Becton et al. 2019 ; El Ouirdi et al. 2016 ). The information sought varies, including personality traits, communication skills, the presence of provocative or inappropriate photographs, or any other factors that might dissuade the hiring of a candidate (Henderson 2019 ).
A survey conducted by CareerBuilder Footnote 5 reveals that 70% of employers utilize social media as a screening tool for potential hires. Despite the potential utility or innocuous intentions perceived by recruiters, this practice raises concerns about potential discrimination against candidates and the disclosure of information forbidden during the interview process. For example, Acquisti and Fong ( 2020 ) showed how the disclosure of certain personal information online can influence the hiring decisions of some U.S. employers, confirming the problematic nature of this practice.
Currently, it is a common practice for companies to request a candidate’s GitHub profile as part of the hiring process. Figure 3 displays a screenshot of the online application procedures for two renowned companies: Anthropic (Fig. 3 a) and Meta (Fig. 3 b). In addition to the conventional inclusion of LinkedIn profiles and links to Google Scholar publications, both companies demand the inclusion of the GitHub URL.
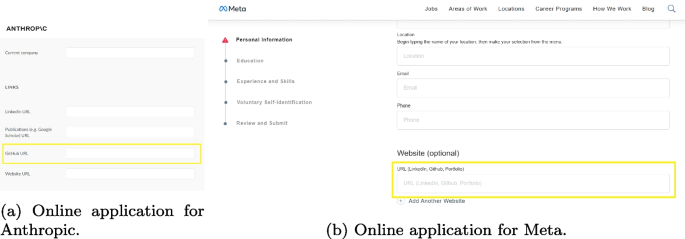
Examples of companies requiring the GitHub profile
The companies under consideration are technology giants, and requesting the GitHub profile could constitute a component of the verification process for assessing technical skills. However, on GitHub, numerous interactions among users have been scrutinized by various researchers to tackle and alleviate social issues such as toxicity or stress (Miller et al. 2022a ; Raman et al. 2020 ), showing that the usage of this platform goes beyond the sharing of technical knowledge.
Understanding the nature of information accessible on GitHub is not only essential for the evidence we gathered regarding recruiter interest in this platform, but also from a broader perspective as it contributes to the overall “digital breadcrumbs” left by users (Stretton and Aaron 2015 ). The significance lies not solely in the context of a single platform; rather, it pertains to the aggregation of information within the digital realm that can be associated with a user and exploited for different purposes, such as surveillance or psychological targeting (Lustgarten et al. 2020 ; Matz et al. 2020 ; Miller 2010 ).
3 Related Work
This section reviews empirical studies on GitHub and findings on privacy issues on this platform. Moreover, we review works on the analysis of user-generated content and users’ privacy. We focus on works that have produced labeled corpora as well as privacy profiling.
3.1 GitHub Studies
GitHub is a widely used platform, and numerous researchers have conducted empirical studies on this platform. In their exploratory study, Henning et al. ( 2023 ) examined reported issues related to data protection on GitHub, shedding light on the influence of data protection regulations throughout the entire software development process. Acar et al. ( 2017 ) conducted a study aimed at enhancing security decision-making for IT professionals. The researchers conducted an experiment with 307 active GitHub users to assess their performance on security-related programming tasks. They found differences in performance related to experience levels, but no significant disparities based on student or professional status. Khalajzadeh et al. ( 2022a ) conducted an analysis of comments on GitHub in order to uncover issues that are centred around human concerns. The analysis revealed a diverse array of issues, encompassing topics such as privacy and security.
Many researchers have focused on investigating a more social aspect of GitHub. Sajadi et al. ( 2023 ) explored the dimension of interpersonal trust in Open Source Software (OSS) teams and how it is exhibited. The study analyzes 100 GitHub pull requests from Apache Software Foundation projects to understand how trust is expressed in these interactions. Guzman et al. ( 2014 ) investigated the impact of emotions on productivity, task quality, creativity, group relationship, and job satisfaction through sentiment analysis of commit comments in various open-source projects. The results indicate that Java projects tend to receive more negative commit comments, while projects with widely distributed teams tend to have more positive emotional content. Raman et al. ( 2020 ) studied the problem of stress and burnout in open-source environments due to toxic discussions on GitHub issues. They demonstrated that a combination of pre-trained detectors for negative sentiment can effectively identify these issues. Furthermore, they established that classification accuracy is enhanced through domain adaptation. In their study, Blincoe et al. ( 2016 ) defined popular users as individuals who provide guidance to OSS developers when they join new projects. The authors observed that those users did not possess the highest contribution rate. Miller et al. ( 2022b ) curated a sample of 100 toxic GitHub issue discussions to gain an understanding of the characteristics of open-source toxicity. They found that some of the most prevalent forms of toxicity are entitled, demanding, and arrogant comments from project users as well as insults arising from technical disagreements.
Different authors have investigated more specifically solely the topic of privacy leakages and self-disclosure on GitHub. According to Vasilescu et al. ( 2015c ), their user survey revealed that platform users are aware of certain demographic details about other developers, including gender, real names, and countries of residence. Additionally, Ford et al. ( 2019 ) demonstrated that GitHub developers explore a much wider array of information while scrutinizing pull requests, particularly information tied to the identity of the person submitting the pull request. This implies that GitHub not only contains identity information but also that such information is exploited during the evaluation of pull requests. In a similar vein, Meli et al. ( 2019 ) discovered that hundreds of thousands of API and cryptographic keys are leaked on GitHub at a rate of thousands per day. Meanwhile, Niu et al. ( 2023 ) demonstrated that it is possible to extract sensitive personal information from the Codex model used in GitHub Copilot.
From these studies, it is evident that GitHub has garnered attention from the software engineering community for several years. The primary findings indicate that various user information, including gender, real names, and countries, can be extracted from GitHub. Furthermore, the platform faces general privacy threats, such as the leakage of crypto-related secrets and private information on Copilot. Additionally, GitHub exhibits characteristics akin to other social networks, such as language toxicity, and is subject to study in the context of team working and cooperation. It is worth noting that none of the mentioned studies investigated the adoption of privacy settings provided by the platform. Previous research has explored the adoption of privacy settings on other platforms such as Facebook (Fiesler et al. 2017 ; Chen et al. 2019 ; Kanampiu and Anwar 2019 ), primarily through user surveys. To the best of our knowledge, this is the first study on GitHub privacy settings that is conducted based on the actual selections made by users.
3.2 Analysis of User-Generated Content
User-generated content plays a significant and ubiquitous role on various platforms, attracting extensive attention from researchers for diverse purposes such as sentiment analysis, risk detection of depression (e.g., on Reddit), marketing analysis, and self-disclosure (Alaei et al. 2023 ; Tadesse et al. 2019 ; Timoshenko and Hauser 2019 ; Umar et al. 2019 ). In this section, we present an overview of studies focused on the analysis of user-generated content, with a specific emphasis on the detection of self-disclosure.
Bioglio and Pensa ( 2022 ) conducted a study aimed at automatically detecting the sensitivity of Facebook posts. Their research involved analyzing a dataset comprising 9,917 Facebook posts, each one annotated by three experts as either sensitive, non-sensitive, or of unknown sensitivity. To enhance their investigation, two additional datasets were incorporated for comparative analysis. The first dataset consisted of posts extracted from Reddit, manually labeled to align with the Facebook corpus. The second dataset comprised anonymous posts from Whisper, considered sensitive, alongside non-sensitive tweets sourced from Twitter (now renamed as X ).
In their experiment, the researchers employed four distinct classifiers on the datasets: Convolutional Neural Network (CNN), Recurrent Neural Network (RNN) with gated recurrent unit, RNN with long short-term memory, and BERT. Notably, they highlighted the limitations of existing corpora for sensitivity detection, emphasizing that many are often derived from specific topics, and thus incapable of detecting sensitivity on wider topics. Furthermore, their findings demonstrated that, on their datasets, RNNs and BERT exhibited significant performance in classification, and that lexical features are not sufficient for discriminating between sensitive and non-sensitive texts.
In their examination of social media safety, DiSalvo et al. ( 2022 ) introduced a methodology for categorizing social media posts by utilizing a pre-established corpus of violent phrases. Specifically focusing on Twitter, the researchers collected posts that were then organized using the labelTweets function. This function evaluates a tweet based on whether it contains a word from a predefined array of ’violent’ terms, either accepting or rejecting the tweet accordingly. Subsequently, the authors manually assigned labels (negative, positive, or neutral) to the output generated by the labelTweets function, guided the corresponding corpora. The resulting corpus comprised nearly 600 tweets. The authors proceeded with a classification task to differentiate between negative and positive tweets, employing three classifiers: Naive Bayes Classifier, Support Vector Machine, and Logistic Regression. Their analysis concluded that the Naive Bayes Classifier performed the least effectively among the three.
Blose et al. ( 2020 ) conducted a study on self-disclosure patterns on social media, specifically focusing on tweets posted during the Coronavirus pandemic. The authors utilized a dataset comprising Tweet IDs, which was updated through the Amazon Web Service. Their methodology involved a pre-processing of the dataset, for filtering only content posted by individual users. To automate the detection of self-disclosure, the researchers employed a dictionary obtained from Umar et al. ( 2019 ) and compared their dataset against an already annotated Twitter dataset. This comparison yielded satisfactory results, demonstrating the effectiveness of their approach. To better understand disclosure trends, the authors conducted a topic-modeling analysis on the described corpus. Furthermore, they conducted a comparative analysis, juxtaposing their observations of self-disclosure behaviors during the Coronavirus pandemic with those observed during Hurricane Harvey in 2017. The findings of the study revealed an increase in self-disclosure behaviors during the pandemic. In their conclusion, the authors encourage further research engagement to “better understand more subtle, voluntary self-privacy violations.” This underscores the importance of ongoing investigations into evolving patterns of online self-disclosure, especially during critical events such as the Coronavirus pandemic.
Despite their distinct objectives, all these studies analyzing user-generated content share a common procedure, which can be summarized as follows: selecting a specific platform or online social network, retrieving textual data from this platform for preprocessing, and utilizing domain-specific resources like the Privacy Dictionary (Gill et al. 2011 ; Vasalou et al. 2011 ) or the self-disclosure dictionary (Umar et al. 2019 ) to support the preprocessing phase. Ultimately, classifiers are employed to automate the detection process.
In our research, we adhered to the same methodology described above for self-disclosure detection in pull_requests comments. We focused on creating a multi-label corpus, where each comment is annotated for every sensitive information shared in the same text.
3.3 User’s Privacy Studies
With the increasing attention to the issue of privacy in the digital world, different researchers have investigated how to capture users’ privacy desiderata and users’ behaviours in online platforms.
Brandäo et al. ( 2022 ) analyzed users’ privacy profiles for what concerns mobile settings and exploited this information to predict the users’ answers to a permission request, while preserving user privacy by applying a federated learning approach.
Tahaei et al. ( 2020 ) examined Stack Overflow for privacy-related challenges faced by developers. They used topic modelling on 1,733 questions, identifying key topics. The results indicated that developers do seek support on privacy issues on Stack Overflow.
In an attempt to study privacy issues in Twitter (now X ) (Keküllüoglu et al. 2020 ), the authors collected a dataset of 635k tweets containing the expression “happy for you.” By employing LDA topic modeling, the tweets were categorized into 12 distinct clusters representing different life events. They found out that around 8% of the tweets mentioned protected users, with varying rates across different topics.
4 Methodology
A primary goal of this study is to investigate the use of privacy settings on GitHub, that is, users’ privacy desiderata expressed on the platform, and their potential limitations (RQ1). To this end, we started by exploring the largest existing dataset of GitHub activities, the GHTorrent dataset (Gousios 2013 ; Gill et al. 2011 ). In this dataset, the Users table provides the set of privacy settings selected by each GitHub user as their privacy preferences on the platform. We adopted this information as being users’ privacy desiderata. Furthermore, from the GHTorrent dataset, we defined and selected a subset of users being more “active” on GitHub, in order to conduct a more fine-grained analysis and to have more textual data to analyze. We called this dataset the Active users . We updated the Active users dataset due to the absence of certain privacy settings that were missing from the original GHTorrent dataset, such as email addresses and social media channels. This process is detailed in Section 4.1 . By strategically retrieving additional data from the current database, it was possible to effectively address the gaps in users’ metadata and ensure a thorough understanding of user data. This was achieved through the use of GitHub APIs Footnote 6 and the users’ login information, which allowed for the seamless integration and enrichment of GHTorrent existing data with detailed and updated user-specific insights. On both datasets ( Users and Active users ), we conducted a cluster analysis to define users’ privacy profiles and observe differences between users selection of settings. All these steps are detailed in Sections 4.1 , 4.2 , and represented in Fig. 4 .
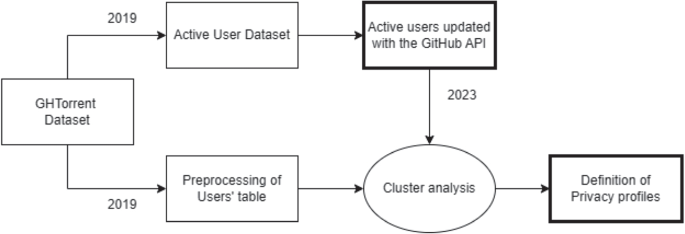
Workflow of the study
To investigate RQ2 and RQ3, that is, the information disclosed on the platform and the analysis of users’ behavior, we considered textual comments provided by the GHTorrent dataset. In particular, we analyzed the pull_request comments to find privacy-sensitive information disclosed intentionally or unintentionally by the users. The methodology for this analysis is described in Section 4.3 . In addition, it should be noted that GHTorrent truncates textual comments, specifically those found in commits, issues, and pull_requests. In order to facilitate the empirical investigation and analysis of privacy awareness in pull_requests, we acquired the original text through the use of GitHub APIs.
4.1 Data Curation
When talking about users’ private information, we refer to the personal information they do not want to share. On GitHub, these privacy desiderata can be expressed through the profile settings where the user chooses to reveal or hide certain information (company, location, bio, social accounts, and so on). In the GHTorrent dataset (Gousios 2013 ; Gill et al. 2011 ), this information was collected in the User’s table (see Table 1 ). For each user in the GHTorrent dataset, we were able to retrieve information regarding whether the user chose to share the name of their company on their profile, along with details about their location, including city and state. These choices reflect the decisions made by users at the time of the last GHTorrent updates, which occurred in 2019. To overcome these limitations, we updated the privacy choices of the Active users dataset. The data curation process is summarized in Table 2 .
Step 1: GHTorrent preprocessing Users We used the GHTorrent dataset to conduct a cluster analysis on a broader population of GitHub users.
The Users’ table from the GHTorrent dataset contains 32,411,628 accounts, from which we eliminated those marked as organization , fake and deleted . This resulted in a set of 22,525,012 users. Figure 5 shows the correlation matrix of the variables in Users table, obtained using the Python library Scikit-learn. Based on this correlation matrix, we excluded the state , country code , lat , and location variables because of the value of correlation being very high (above 0.7), following the methodology outlined by King ( 2015 ).
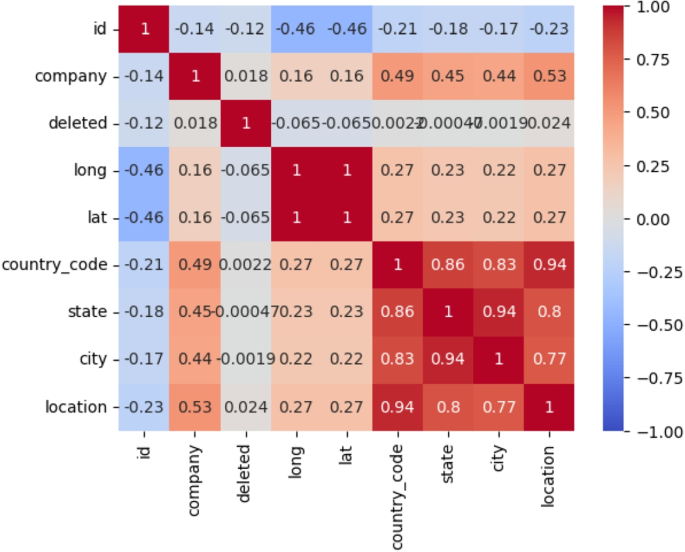
Correlation matrix of the variables in the Users dataset

Step 2: Active users To have a more updated version of users’ privacy preferences, we restricted our research to those users considered more “active” on GitHub, those who may be identified by quantifying the number of actions they have performed on the platform. Therefore, we constructed a new dataset that contained information about the number of commits , commit_comments , followers , pull_requests comments and issue_comments executed by each user (see Table 4 ). This achievement was made possible through the GHTorrent dataset since this dataset allows the extraction of the precise count for each action executed by the users. Indeed, we retrieved this information from the corresponding tables from the GHTorrent dataset. After the due preprocessing step, we performed a cluster analysis on the dataset described in Table 4 . Primarily, we scaled this dataset, and performed a K-means cluster analysis on the dataset with a number of clusters K=4 (found with the Elbow method).
Secondly, we discretized the variables into three distinct bins. The “low” label was assigned to instances where the number of actions ranged from the minimum value to the 65th percentile. The “medium” label was applied when the number of actions fell between the 65th percentile and the mean value. Finally, the “high” label was assigned to cases where the number of actions varied from the mean value to the maximum value. The choice of the 65th percentile as a threshold was deliberate, as it consistently yielded values lower than the mean across all variables in the dataset, as evidenced by the statistics in Table 5 .
Figure 6 a shows the different clusters of users, according to the number of actions performed. Figure 6 b, c, d, and e illustrate the percentage of each variable per cluster. For instance, examining Fig. 6 b reveals that users in Cluster 0 tend to make few commit comments (the variable ’low’ is predominant in this cluster). The analysis of the variables per cluster in Fig. 6 allowed us to clearly identify the least active users, being cluster 0. Consequently, we excluded this cluster of Non-Active users from the overall dataset, resulting in what we refer to as the Active users dataset (6,329 users in total). The number of Active users decreased to 6,132 with the updates of users’ privacy settings that we have performed through the GitHub API, as explained in the next step. This is attributed to users departing the platform by 2023.
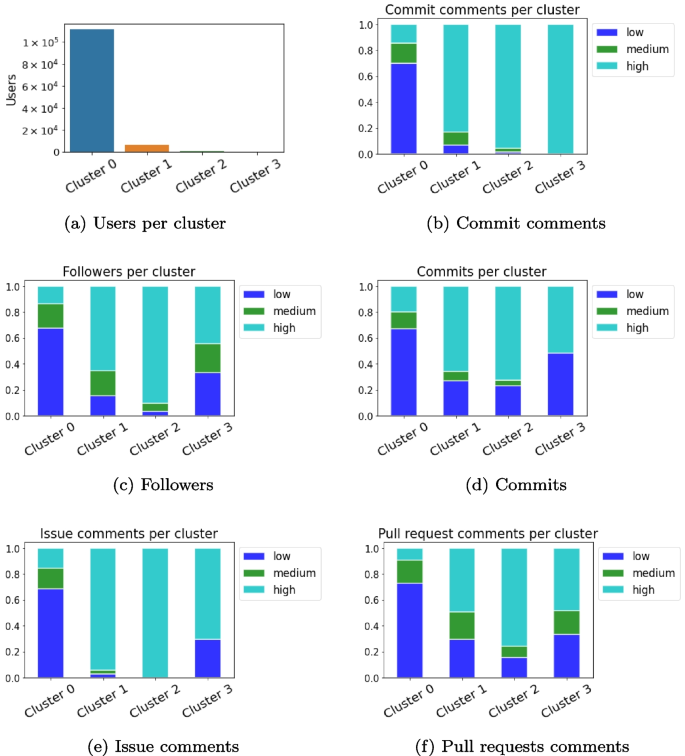
Cluster analysis on the dataset of the actions performed by each user. Figure 6 a shows the number of users per cluster, Fig. 6 b, c, d, and e show the distribution of variables per cluster
Step 3: Updating active users privacy settings Once we selected the Active users , we updated their privacy settings choices using the GitHub API with their login. This action was taken due to the incomplete nature of the GHTorrent dataset, which lacked information such as users displaying their email addresses, events, and their Twitter accounts. The updated dataset is illustrated in Table 6 . Compared with the GHTorrent dataset, the columns added were email , events and twitter . Some of the users in the Active users dataset were no longer present on GitHub at the time of the update so they were eliminated. The final number of updated Active users is 6,132 users. The methodology detailed in this section is represented in Fig. 4 .
After the data curation described in Section 4.1 , we conducted a cluster analysis on the two datasets: Users and Active users . The goal was to understand whether users present variability in terms of privacy settings on GitHub, i.e., if there are different privacy profiles that can be associated with the users of this platform (Di Ruscio et al. 2024 ) meaning that users adopt different privacy settings as a tool for their privacy. The clustering techniques adopted were K-means and hierarchical clustering, as suggested by different authors (Sanchez et al. 2020 ; Brandäo et al. 2022 ).
4.2 Cluster Analysis
To define the privacy profiles of GitHub users, we performed cluster analysis on the two datasets, Users and Active users . The variables considered are the privacy settings chosen by each user, however, there are variations between the two sets due to recent additions of privacy features by GitHub, such as events and twitter . This novelty is reflected in the updated dataset of Active users . In the Active users dataset, we have added the email field, which was removed from the Users dataset by the author of the GHTorrent dataset.
For the Users dataset preprocessed as described in Section 4.1 Step 1 , we performed a K-means cluster analysis (Hartigan and Wong 1979 ) with Euclidean distance. The number of clusters K=3 was chosen with the Elbow method (Syakur et al. 2018 ).
For the Active users dataset updated as described in Section 4.1 Step 3 , we removed the variables location , long , lat , country code , and state from the Active users dataset due to their correlations as shown by the correlation matrix in Fig. 7 . We employed both the Elbow method (Fig. 8 ) and an analysis of the dendrogram obtained using Ward’s method (Fig. 9 ) to determine an appropriate number of clusters. We identified K=4 as a reasonable number of clusters for this dataset, therefore a K-means cluster analysis was performed with this value.
Both cluster analyses for the datasets Users and Active users were conducted in order to study users’ privacy profiles and to verify whether these settings were actively adopted by the users. The results are discussed in Section 6 .
4.3 Construction of the Corpus
To address RQ2 and RQ3, we started exploring users’ privacy behaviour for what concerns textual data, e.g., their comments on GitHub. While there’s a wealth of textual data in the GHTorrent dataset, we focused our research on pull_requests comments exclusively (86,000 comments overall).This decision was guided by previous studies highlighting a greater probability of encountering significant user interactions and consequently more sensitive information in pull_request contexts (Sajadi et al. 2023 ; Iyer et al. 2019 ).To collect the more privacy-sensitive data and prepare the dataset for subsequent manual labeling, we automatically labeled each comment using the Privacy Dictionary created by Vasalou et al. ( 2011 ); Gill et al. ( 2011 ), exploiting libraries provided by existing work (Casillo et al. 2022 ).This dictionary was constructed and validated by its authors through interviews and focus groups from different privacy-sensitive (offline and online) contexts, leading to identify eight different privacy categories, as illustrated in Table 7 . Each category represents a distinct privacy realm, potentially encompassing different types of private information. Previous authors have successfully adopted this dictionary to detect privacy language patterns within a given text, such as Bioglio and Pensa ( 2022 ) and D’Acunto et al. ( 2021 ). The final goal of this process is to gather evidence of self-disclosure on GitHub. An example from the corpus labeled with the Privacy Dictionary is shown in Table 8 . The first column indicates the user who made the comment in the “body” column. The “Categories” column shows the privacy category assigned to each comment, identified through the “Keywords” in the corresponding column.
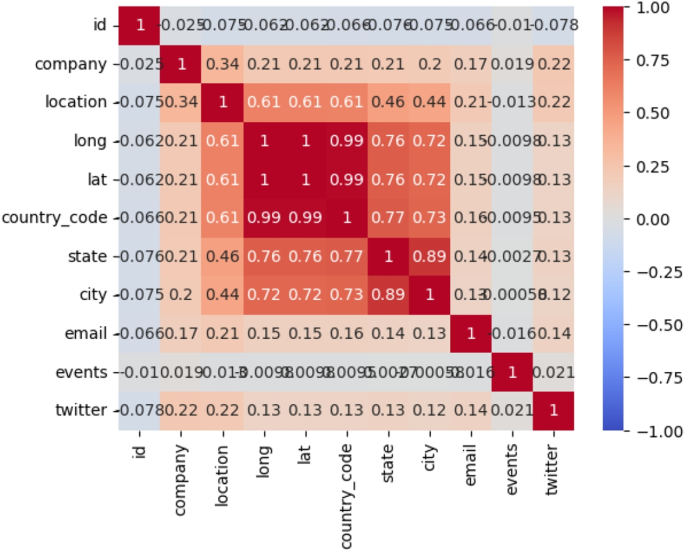
Correlation matrix of the variables present in the Active users dataset
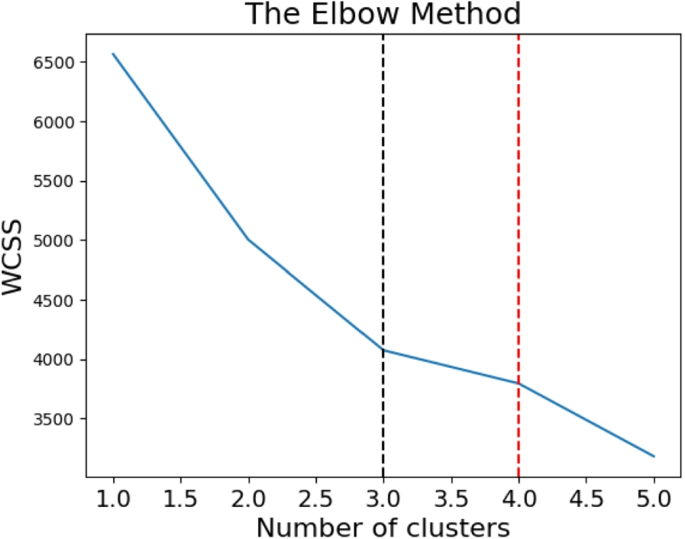
Elbow method on the Active users dataset to establish the more appropriate number of clusters
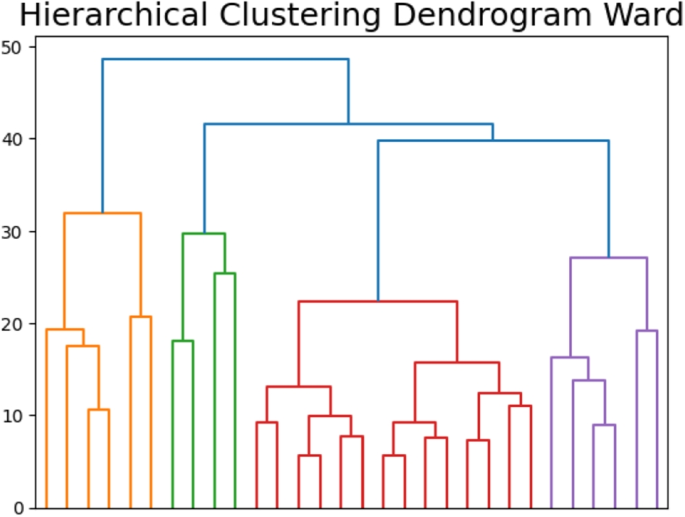
Dendrogram with Ward’s method on the Active users dataset to have an overview of the dataset and to confirm the appropriate number of clusters
Given that GitHub pull_request comments often involve technical details, we aimed to enhance the efficiency of identifying comments with private information. To achieve this, we specifically focus on comments that carry more than one label from the categories within the Privacy Dictionary. Indeed, due to the broad scope of the dictionary, we have empirically noted that most comments were assigned at least one label. Therefore, we chose to select comments with multiple labels that potentially included information from various privacy domains represented by each privacy category of the dictionary. This approach increases the likelihood of discovering more sensitive information, potentially different from what was discovered by previous authors (Vasilescu et al. 2015b ). This selection resulted in a final corpus of 15,672 comments. However, since these comments in the GHTorrent dataset were truncated, we updated all of them through the GitHub API. This process in represented in Fig. 10 . This corpus, developed with the aid of the Privacy Dictionary, was manually labeled as described in the following Section 4.4 to classify sensitive comments and the type of information disclosed.
4.4 Manual Labeling Process and Protocol

Process for constructing the corpus
After skimming the pull_requests comments corpus, as outlined in Section 4.3 , we curated a set of 2,000 comments, representing nearly 10% of the extracted comments. To ensure a sufficient level of informativeness, we specifically chose comments with a minimum of 2,000 characters. The aim was to explore the nature of information that users potentially disclose in their pull_request comments. The selection of longer comments, coupled with their prior labeling using the Privacy Dictionary, aimed to enhance the likelihood of filtering out irrelevant or purely technical comments. These comments were subjected to manual labeling by all the authors of this paper.
The annotation team consisted of four members, ensuring a gender balance. All annotators had STEM backgrounds and held various academic positions, ranging from PhD student to full professor. Three of the annotators were from the same country, while the fourth was from a different one. Each annotator was given a file of roughly 1,000 comments; every file was assigned to at least two different annotators, following existing guidelines about how to conduct a user study (Di Rocco et al. 2021 ; Robillard et al. 2010 ). A value of 1 was assigned to comments that revealed personal information about the user, 0 otherwise, irrespective of our perception of sensitivity. Sensitivity is a subjective concept influenced by social and cultural factors; thus, what one deems sensitive may differ from person to person. Indeed, for the manual labeling process, we refer to the definition provided by Bioglio and Pensa ( 2022 ) about Privacy-sensitive content:
A generic user-generated content is privacy-sensitive if it discloses, explicitly or implicitly, any kind of personal information about its author or other identifiable persons.
For comments deemed as disclosing information, annotators had to select a label from the Possible category column corresponding to the type of information disclosed. The proposed labels were Personal name , Workplace , Email , Location , and Gender . The labels regarding personal name, job’s information, email and location were derived from a preliminary corpus analysis conducted by one of the authors. Furthermore, these labels aligned with the privacy settings available on the GitHub profile, where users have the option to conceal specific private information. The inclusion of the gender label was prompted by findings from various studies on GitHub that highlight instances of gender discrimination or non-inclusive behaviors (Imtiaz et al. 2019 ; Garcia et al. 2023 ). Annotators were allowed to choose multiple labels for each comment and could use the Other column to indicate information not covered by provided labels. Participants were given an annotation guide to enhance consistency throughout the process.
After completion, the four annotators met together via Teams in two different days to discuss the comments marked as privacy sensitive and to reach an agreement in case a comment was marked only once. Each comment expressing disagreement underwent thorough discussion between the two assigned annotators, while the remaining two played a moderating role, facilitating the conversation and aiding in reaching a consensus. During these discussions, one annotator was prompted to explain why a comment was considered sensitive or not sensitive to the other annotator of the same comment. Agreement would result in either flagging the comment as sensitive or deleting it. Moreover, we discovered during these meetings that several sensitive comments disclosed information not covered by the existing labels. This information was added by the annotators in the column Other . We unanimously decided to introduce additional labels, such as Moral values , Community etiquette , Personal info , Language , and Relation with a user , to account for instances where comments disclosed such information. Ultimately, 147 comments were unanimously identified as disclosing private user information. Table 9 provides an excerpt from the fully annotated corpus, displaying comments with specific labels and brief descriptions of the label meanings.
On the labeled corpus, we conducted an analysis to calculate the level of agreement. Each comment was annotated by two annotators, resulting in two raters per comment. Across the entire dataset, we computed Cohen’s kappa coefficient, a metric utilized to assess inter-rater reliability (Cohen 1960 ). The Kappa score of 0.49 was computed for the binary sensitivity column before any discussion occurred, indicating a moderate level of agreement among the raters (Warrens 2015 ). This is not surprising considering the novelty of the analyzed data and the nuances present in the dataset. It could also be attributed to the observation that, out of 2,000 comments, 1846 received identical binary labels from both evaluators.
5 Sensitivity Detection in Textual Comments
Pre-trained generic language models (Devlin et al. 2018 ; Inan et al. 2023 ; Howard and Ruder 2018 ) have achieved great results on different NLP tasks. To illustrate the potential of these models in enhancing user privacy awareness, our study concentrates on demonstrating their ability to detect possible privacy leaks within textual comments. The primary aim is not to develop high-performance tools or methodologies but rather to explore the feasibility of autonomously detecting self-disclosure across various contexts.
To achieve this, we fine-tuned the Llama2 model (Touvron et al. 2023 ) (Section 5.1 ), using the labeled corpus of comments obtained through the process explained in Sections 4.3 and 4.4 . This fine-tuning process aimed to enhance the model’s performance in identifying privacy data leakage, leveraging the targeted information in the curated dataset.
Additionally, we investigated the performance of BERT (Bidirectional Encoder Representations from Transformers) (Devlin et al. 2018 ) for sensitivity detection (Section 5.2 ). BERT, a widely used pretrained model, was fine-tuned for binary classification of self-disclosure in comments. We utilized the Hugging Face Transformers library and trained BERT on our labeled dataset curated in Section 4.4 to adapt it to the sensitivity detection task. The task consists of a binary classification of sensitive or insensitive comments.
5.1 Large Language Models for Sensitivity Detection
Research in the domain of content sensitivity detection and privacy leakage in text spans various disciplines, including machine learning (ML), natural language processing (NLP), philosophy, psychology, and the social sciences. The overarching goal is to enhance privacy awareness and conduct risk assessments, primarily within specific platforms, with the ultimate aim of developing technology for empowering users in protecting their privacy. Despite the considerable success of these approaches, they generally do not conform to a one-size-fits-all model (Nguyen et al. 2023 ; Tang et al. 2023 ). Performance disparities exist across datasets, with models excelling in specific contexts while underperforming in others, as demonstrated in experiments by Peiretti and Pensa ( 2023 ). Language, dataset balance, and text length are among the factors influencing model effectiveness. Moreover, achieving satisfactory performance necessitates a harmonious combination of feature extraction and classifier design. Researchers are required to explore numerous combinations to optimize synchronization (Nguyen et al. 2023 ; Tang et al. 2023 ). This comprehensive approach is crucial for content sensitivity detection in text. Recent advancements in LLMs have significantly enhanced the performance of diverse natural language processing (NLP) tasks (Min et al. 2023 ), opening up new possibilities for automating functions traditionally executed by humans. These models consist of large neural networks pretrained on vast corpora of text data in multiple languages, offering potential solutions to the challenges associated with conventional text classification methods. This is the reason why we chose to use LLMs to for this detection sensitivity task. In order to demonstrate the potential of Llama, we have asked the model to provide only Yes or No as an output. In particular, Listing 1 shows this instruction.

Several successful research endeavors have utilized fine-tuning of large language models (LLMs) (Lu et al. 2023 ; Behnia et al. 2022 ; Yao et al. 2024 ). In order to enhance the accuracy of Llama’s predictions, we have chosen to utilize the Parameter-Efficient Fine-Tuning (PEFT) method. It allows for the effective customization of pre-trained language models (PLMs) for different downstream applications without the need to fine-tune all of the model’s parameters. Optimizing extensive pre-trained language models (PLMs) is frequently too expensive. PEFT approaches specifically focus on fine-tuning a limited number of additional model parameters, resulting in a significant reduction in both computational and storage expenses. The fine-tuning process involves providing a cue to the model and directing it to provide an appropriate binary classification, specifically distinguishing between privacy-sensitive and privacy-non-sensitive. The target provided corresponded to the projected classification. This was done to enable the model to provide a direct response using binary classification. The prompt employed during the process of fine-tuning is illustrated in Listing 2. It consists of three main parts: (i) Instruction , where we are asking for a binary classification; (ii) Input is the comment to be classified; and (iii) Response is the expected answer. The results of this experiment are illustrated in Section 6 .
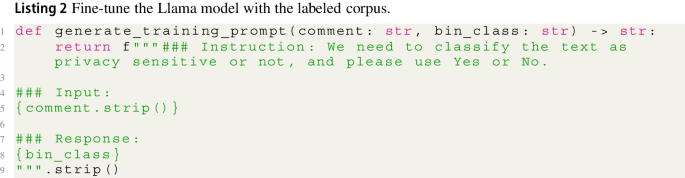
5.2 BERT for Sensitivity Detection
In our study, we explore the capability of BERT to classify user privacy disclosures effectively. Its architecture is based on a transformer encoder and the basic BERT architecture consists of different attention-based layers. The tokens are represented as input vectors which include the tokens they-self, their positions, and their context sentence. For each token, the attention-based layers produce a representation. Each token representation is based on the representations of all tokens. The output of one attention-based layer is provided as input for the next one. Finally, the last attention layer provides the model output. The BERT model is unsupervisedly trained on large amounts of text and may later be applied to potentially any task. This allows us to train the bert-base-uncased model Footnote 7 using the labeled set of comments defined in Section 4.4 . We have chosen this model because previous authors have successfully employed BERT in the context of empowering users ( Adhikari et al. 2022 ; Khalajzadeh et al. 2022a ; Wang et al. 2022 ). The applications span from automated labeling of GitHub issues to privacy policy classification.
5.3 Metrics and Methodology
Our study evaluates the effectiveness of pre-trained models in identifying private information within text by conducting three distinct configurations.
The first configuration, zero-shot Llama ( Llama \(_{zs}\) ), employs the LLaMA model utilizing predefined queries as outlined in Listing 1. This approach tests the baseline ability of LLaMA to recognize privacy-related information without any model customization. In the second configuration, fine-tuning Llama ( Llama \(_{ft}\) ), we enhance the LLaMA model’s capability by fine-tuning it on 80% of our curated dataset as outlined in Listing 2. The remaining 20% of the data serves as a test set to evaluate the model’s prediction accuracy. The third configuration, fine-tuning BERT ( BERT \(_{ft}\) ), involves a BERT model that we trained specifically for the task. Similar to the Llama \(_{ft}\) configuration, we fine-tuned the model on 80% of the manually curated comments, reserving the remaining 20% for performance evaluation.
To asses the performance of the model, we used state-of-the art metrics: accuracy, precision, recall, and false positive rate (FPR). In what follows, TP is the number of true positive predictions, TN is the number of true negative predictions, FP is the number of false positive predictions, and FN is the number of false negative predictions. The metrics are calculated as follows (Hossin ans Sulaiman 2015 ):
Accuracy refers to the ratio of right predictions, including both true positives (TP) and true negatives (TN), to the total number of cases analyzed:
Precision measures the fraction of the number of correctly classified comments as yes (TP) to the total number of classified comments as yes (TP + FP):
Recall measures the proportion of actual positive cases that are correctly identified by the model. It is defined as the ratio of true positives (TP) to the sum of true positives and false negatives (FN):
F1 represents the harmonic mean between recall and precision values, and it is particularly high when true negatives ( TN ) are high:
Section 6.4 shows the results of the different experiments and compare the performances of each configurations.
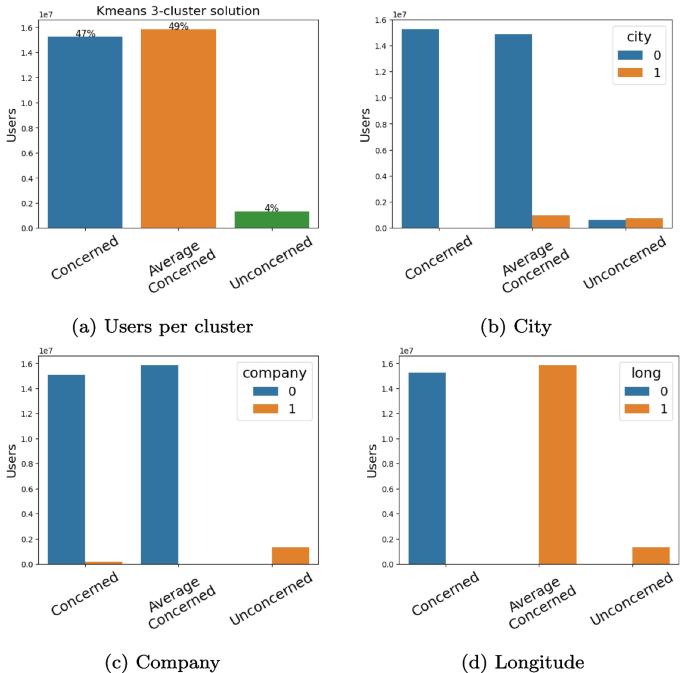
Cluster analysis on the Users’ dataset with K-means. Number of users per cluster (Fig. 11 a) and distribution of variables per cluster (Fig. ( 11 b, c and d)
6 Empirical Results
In this section, we report and analyze the experimental results by answering the four research questions introduced in Section 1 .
6.1 RQ \(_1\)
Are the privacy settings provided on GitHub used/adopted by the users? In other terms, do we observe different combinations of these settings, or is there a dominant configuration?
Users’ dataset cluster analysis The cluster analysis conducted on the Users’ dataset led to three unbalanced clusters, shown in Fig. 11 a. Figure 11 b, c and d show the number of users that set (1) or hide (0) the corresponding setting being City , Company , and Longitude . From these plots, we can observe that the clusters are distinguished according to each variable considered. For example, users in the first cluster, named “Concerned”, exhibit a reluctance to share any information on GitHub. Conversely, those in the second cluster, denoted as “Average Concerned” are willing to share information only regarding their location ( Longitude ). On the other hand, users in the third cluster, namely “Unconcerned”, have a tendency to share comprehensive information through their privacy settings. Indeed, the majority of the users in this cluster have set the option to disclose both their City , their Company , and their Longitude (location). This solution suggests that the privacy desiderata of GitHub users can vary even on a small set of privacy options and it demonstrates that GitHub users actively utilize privacy settings. It is interesting to observe the population distribution depicted in Fig. 11 a, as the cluster “Unconcerned” stands out as the less populated cluster (4% of the entire population). This observation potentially implies that only a limited percentage of GitHub users are willing to disclose comprehensive information in their profiles. This finding further motivates our study.
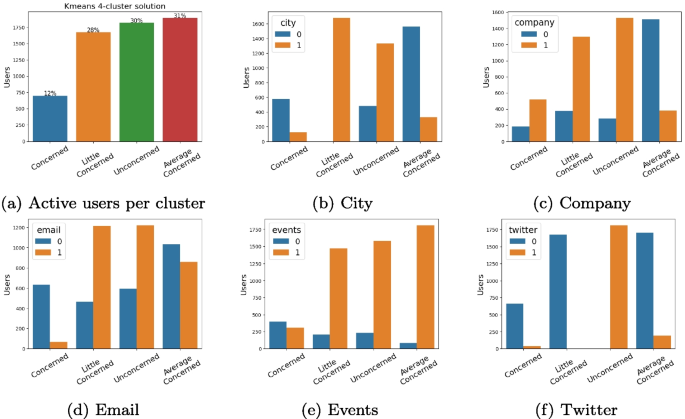
Cluster analysis on the Active users dataset with K-means. Number of active users per cluster (Fig. 12 a) and distribution of variables per cluster (Fig. 12 b, c, d, e and f)
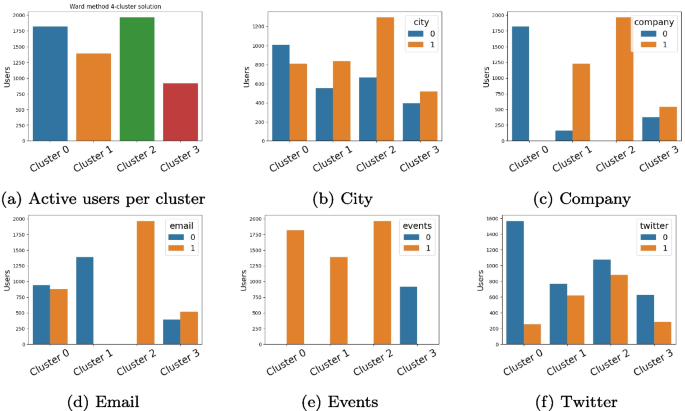
Cluster analysis on the Active users dataset through the hierarchical clustering methods. Number of active users per cluster (Fig. 13 a) and distribution of variables per cluster (Fig. 13 b, c, d, e and f)
Active users analysis with K-means On the Active users dataset, we performed a K-means cluster analysis with K=4 chosen with the Elbow method. Figure 12 shows an overview of privacy settings choices made by the active users. The privacy profiles are rather balanced, as illustrated in Fig. 12 a by the cardinality of each cluster. Figure 12 b, c, d, e, and f depict users’ privacy settings choices per cluster. We used this analysis to qualitatively define each profile as follows: “Concerned” about hiding their information from the GitHub profile, “Little concerned”, “Unconcerned” and “Average concerned”. For instance, Fig. 12 b reveals that in the first cluster, namely “Concerned”, a significant majority of users opted to conceal their City information on their profiles, similarly to users in cluster “Average concerned”. On the contrary, users from clusters “Little concerned” and “Unconcerned” exhibit a significant number of users willing to share information about their City. Analogous considerations can be applied to the other variables: Company , Email , Events and Twitter .
These results are significant as they illustrate that users’ privacy concerns, as expressed through the privacy settings of GitHub, vary. Thus, users exhibit distinct privacy profiles. This is visible in Fig. 12 a, where the profiles are rather balanced in terms of the number of users, showing that the choices of privacy settings do not converge towards one main combination of settings. These privacy profiles helped us categorize active users according to their privacy desiderata. We exploited this categorization to address RQ \(_3\) .
Active users analysis with hierarchical clustering As many authors suggest (Brandäo et al. 2022 ; Sanchez et al. 2020 ), another way to generate users’ profiles is through a hierarchical clustering algorithm. We used this method to further analyze the Active users dataset. We applied this technique using Ward’s method on the Active users dataset, with the number of clusters equal to 4. As previously explained, this number was chosen by analyzing the dendrogram (Fig. 9 ). We report the bar charts regarding the distribution of variables per cluster and the cardinality of each cluster (see Fig. 13 ). The clusters are less balanced than the one obtained with K-means (Fig. 13 a). By observing the distribution of variables per cluster, the situation is unclear compared to the clusters with K-means. Indeed, the variable City seems irrelevant in discriminating between the profiles (Fig. 13 b).
Answer to RQ \(_1\) . GitHub users manifest diverse privacy preferences, as reflected in their selection of privacy settings, both on a broad scale -exemplified by the analysis conducted on the entire Users dataset- and on a more granular level, as seen in the examination of the Active user dataset. The latter is interesting, given that these users share a high level of activity on the platform, yet their privacy preferences can vary considerably. According to our analysis of the Users dataset, it emerges that only a small percentage of users are willing to disclose all their information in their GitHub profiles. Overall, privacy settings are a tool used by users to safeguard their privacy and should accurately reflect their privacy preferences.
6.2 RQ \(_2\)
What types of private information are disclosed on GitHub by users?
In order to address RQ \(_2\) , we began with a dataset of 2,000 texts of Active users , selected using the Privacy Dictionary, as described in Section 4.3 . From this corpus, we manually labeled 147 comments as privacy sensitive.
This corpus provides examples of different types of private information that is disclosed by the GitHub users. Sometimes this information is more explicit, as visible in comment 1) from Table 9 . In this case, the user reveals his/her personal email. Sometimes, a piece of private information is more implicit, as in comment 8), where the user speaks of a tornado in the area of his/her interlocutor. This reveals a close relationship between the users and the information of the tornado can lead to the location of the interlocutor. Similarly, comment 5) reveals a close relationship between the two users, having a conversation on the phone. Figure 14 shows the percentage distribution of each label in the corpus. As it is evident, “Personal name” represents a significant portion of the pie chart. However, it is noteworthy that nearly 40% of the sensitive comments contain a wide range of sensitive information, from “Moral values” to work-related details.

Percentage of each label in the corpus
This finding reaffirms the observations made by previous researchers, that while GitHub primarily serves as a platform for sharing technical knowledge, an examination of users’ textual comments expose instances of disclosing private information. Such information may originate from the commenter, either pertaining to themselves or involving another user.
Answer to RQ \(_2\) . Even if GitHub is considered a platform used for technical purposes only, different types of private information are consciously or unconsciously disclosed by the users. The categories of information revealed in pull_request comments exhibit diversity. To date, our investigations have uncovered instances of Personal Name , Workplace , Email , Location , Moral Values , Community Etiquette , Personal Info , Language , and Relation with a User .
6.3 RQ \(_3\)
After users choose their privacy settings, do they adhere to what they have declared? In other words, can we observe a discrepancy between their stated privacy preferences and their actual behavior, such as their textual activity?
During the manual-labeling process described in Section 4.4 , we selected comments that were particularly meaningful from a privacy perspective to analyze the profile of the author. Table 10 presents examples of sensitive comments and the profiles of their authors, as identified in Section 6.1 .
Interestingly, many of these comments fall into the profiles of “Average Concerned” and “Concerned” users. This suggests that users who are presumed to be concerned about their privacy do not necessarily demonstrate this concern through their behaviors. Figure 15 shows the distribution of each label per privacy profile, i.e., how many comments disclosing that information were found in each cluster. The bar plot in Fig. 15 a represents the distribution of sensitive comments across different privacy profiles. Each privacy profile is indicated on the x-axis, categorized into the four groups: “Concerned”, “Little Concerned”, “Unconcerned”, and “Average Concerned”. The bar plot in Fig. 15 b presents the same data with the y-axis logarithmically scaled, allowing for a more compact and interpretable visualization. Consistent with previous findings, the label “Personal name” is the most commonly shared across all privacy profiles. As shown in the plot, the profile of “Concerned” users displays six out of nine labels, which is expected as these users are likely more attentive to their privacy. However, evidence of self-disclosure is still found among users in this cluster. Conversely, the “Average Concerned” user profile contains all the different labels, suggesting a discrepancy between their stated privacy preferences and their actual behaviors.
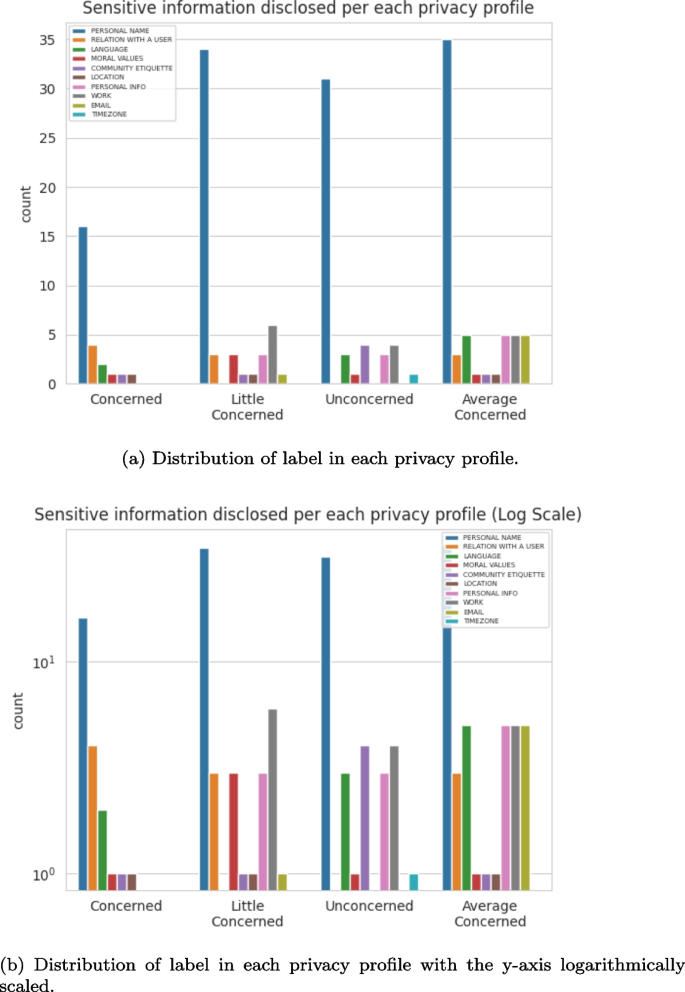
Analysis of the disclosure of each label per privacy profile
This phenomenon can be interpreted in various ways. One possible explanation is that GitHub privacy settings may not comprehensively capture users’ privacy preferences. Alternatively, developers might believe that disclosing certain information could be advantageous in specific situations, potentially overlooking the fact that this information is openly available. Another consideration is the so-called “privacy paradox” (Acquisti and Grossklags 2005 ; Gerber et al. 2018 ). For example, users in the profile “Average Concerned” express a desire to keep information private through their privacy choices but exhibit a disclosing behavior in their writing/commenting activities. However, the validity of the privacy paradox is highly debated in the literature, and its existence is questioned (Solove 2021 ). Lastly, this finding can be also explained with the concept of “privacy fatigue”, which refers to the increasing difficulty individuals face in managing their online personal data, leading to weariness about having to constantly consider online privacy (Choi et al. 2018 ).
Answer to RQ \(_3\) . The privacy preferences selected by the users on GitHub might not be entirely representative of their privacy desiderata. Indeed, we found a discrepancy between what they declared as privacy settings, and how they behaved. This finding can be attributed to various factors, spanning from a perceived advantage in sharing specific information, to the so-called “privacy fatigue”, to a potential lack of awareness, often referred to as the “privacy paradox”.
6.4 RQ \(_4\)
To which extent is it possible to automate the detection of sensitive comments with the use of BERT or Llama2?
This section compares the configurations described in Section 5.3 , each fine-tuned or used directly to classify privacy disclosures in textual comments. Our analysis focuses on several key performance metrics and explores the models’ practical effectiveness in real-world privacy identification tasks.
In Table 11 , we present the results of configurations in which comments were classified as either privacy-sensitive (PS) or non-sensitive (PNS). In particular, the table compares the three configurations described in Section 5 , i.e., Llama \(_{zs}\) , Llama \(_{ft}\) , BERT \(_{ft}\) , across four metrics described in Section 5.3 . Evidently, by the Llama \(_{ft}\) and BERT \(_{ft}\) configurations, the prediction performance is better than that of Llama \(_{zs}\) . The accuracy metrics stand at 0.94, 0.94 and 0.417, respectively, signifying that the fine-tuned configurations outperform the zero-shot in predicting the correct class. Across all the configurations, it is noticeable that the values for predicting non-sensitive instances consistently outweigh those for sensitive ones. This imbalance can be attributed to the fact that the dataset used is heavily skewed towards non-sensitive texts. Further improvement can be achieved with a more balanced dataset.
Even though we have asked the model to provide only “Yes” or “No” as acceptable answers (see Listing 1), we observed that the output of the Llama \(_{zs}\) was frequently uninterpretable. Examples such as “I’m not sure what you mean by ’privacy-sensitive,’ I’m not sure what you mean by ’not”’ and “This is a simple yes or no” were generated by the model. On the contrary, the Llama \(_{ft}\) always generated interpretable output, with a clear answer to the prompt given which consisted in either “Yes” or “No”. Further studies should delve into prompt-engineering techniques to assess whether the performance in predicting sensitive text can be significantly enhanced. It is worth noting that by Llama \(_{ft}\) and BERT \(_{ft}\) their prediction performance is somehow comparable, two indicating that both models can be exploited for building a tool of sensitivity detection. The curated dataset together with the python scripts to preprocess and fine-tuning pre-trained models are available on the supporting GitHub repositories. Footnote 8
Answer to RQ \(_4\) . Pre-trained models can be employed to identify sensitive information in textual data. The availability of a curated corpus of user comments enables the fine-tuning of pre-trained models. Both Llama \(_{ft}\) and BERT \(_{ft}\) configurations exhibit superior performance compared to the Llama \(_{zs}\) . Nevertheless, to ascertain whether the low performance on sensitive data is attributed to the skewed dataset or the model itself, it is recommended that more examples of sensitive texts be introduced for evaluation.
7 Discussion
Our empirical analysis addressed the study of the privacy dynamics on GitHub, with particular attention to users’ privacy settings and behaviours on the activities related to pull_requests comments. Users privacy preferences were deduced from the privacy settings they chose in their profiles, while the analysis of their behaviours was conducted on their textual activity (pull_requests comments). Primarily, we observed that users from both Users and Active users dataset exhibit significantly distinct privacy preferences. This finding indicates that users actually adopt privacy settings and that they express different privacy concerns (RQ1). Consequently, there is a clear indication for a more thorough investigation into the dynamics of privacy on this platform.
Additionally, despite GitHub being primarily used for sharing technical knowledge, there are instances of unintentional or deliberate leakage of users’ private information in their textual activity (RQ2). The disclosed information ranged from real names to personal values, surpassing what could be concealed by the privacy settings provided on GitHub. This is in contrast with the GitHub privacy statement, which asserts that it is sufficient to “adjust your setting for your email address to be private in your user profile” (see Fig. 1 ). Indeed, along with previous studies (Vasilescu et al. 2015a ; Terrell et al. 2017 ; Meli et al. 2019 ; Niu et al. 2023 ), we realized that this statement does not hold true. This suggests that privacy settings alone do not guarantee users’ privacy and that a more sophisticated tool for privacy protection and awareness is needed.
The analysis of user behaviors (pull_request comments) has enabled us to identify diverse types of sensitive information disclosed on GitHub and compare them with the privacy preferences expressed by the users (privacy profile). We observed that users assigned to a privacy-concerned profile were authors of privacy-sensitive comments (RQ3). Our findings indicate that although users do engage with privacy settings using various configurations, their behaviors may inadvertently expose certain private information. This can be attributed to users’ lack of awareness, convenience, or privacy fatigue. In any case, more sophisticated privacy settings on GitHub would allow users to more accurately reflect their preferences. Due to the limitations of privacy settings, we explored using Llama2 and BERT to detect sensitive comments on GitHub (RQ4). This preliminary study suggests that BERT outperforms Llama2 in this task. Further investigation with more prompt engineering should be conducted to confirm this result. The implementation of finer-grained privacy settings could serve as a foundation for developing a privacy awareness tool on GitHub, which could notify users when their behavior, identified with the help of models like Llama2 or BERT, deviates from what is specified in their profile. In this context, a privacy awareness tool could be useful for alerting users when such deviations occur or for suggesting less sensitive rephrasings of text.
7.1 Threats to Validity
External validity. By using the GHTorrent dataset, we were able to get a large sample of privacy settings on GitHub, as well as the number of actions performed by each user. This allowed us to establish a definition of Active users on the platform. It is worth noting that the GHTorrent version we used was from 2019, which means our selection of Active users was based on data available up to that time. So, no new users were added to the original GHTorrent data and the analysis was done on users who were part of the 2019 dump. To tackle this potential limitation, we updated users’ privacy preferences and their comments using the GitHub API. Moreover, our results mainly concern the population of Active users and might not apply to other GitHub users. Future studies could address this limitation by directly collecting data from the platform and verifying whether the results still hold. We have excluded comments without privacy-related labels from the fine-tuning and evaluation datasets. While this does not seem to compromise the model’s ability to distinguish between sensitive and non-sensitive comments, the lack of these comments may limit the generalizability and robustness of the findings. A more diverse dataset could help address this concern. The use of truncated comments from GHTorrent may have led to the omission of privacy-related terms in the truncated sections. While this does not affect the accuracy of the automated labeling, it may have resulted in an underestimation of the total number of privacy-sensitive comments. The textual data comprised only pull_requests comments. We chose this as “pull request comments are likely to contain valuable insight into the relationships of developers interacting with one another” (Sajadi et al. 2023 ), thus we expected to find more sensitive information in this type of data. Future work may consider also issues and commits.
Internal validity. We adopted privacy settings chosen by the users as a declaration of their privacy desiderata. Even if this can be the case for some of them, for others their choice of privacy settings might be arbitrary or not necessarily aligned with their actual preferences. This discrepancy could stem from limitations in the options available on GitHub or from a lack of awareness regarding privacy implications. Moreover, some users might be unemployed and therefore not showing information related to their job, or not have a Twitter account. In our study, these cases are considered as hiding information.
Construct validity. While there were four annotators in total, each comment was evaluated by only two individuals. People may perceive the same comment in different ways, and this can pose a threat to the corpus validity. To mitigate this bias, we organized the discussion phase, where any discrepancy was discussed and resolved by two involved participants. The agreement process took place in two different sessions, during which the raters could explain their choices. For a more nuanced understanding of user privacy, a method like the Experience Sampling Method could offer valuable insights (Zhang et al. 2020 , 2021 ).
8 Conclusion and Future Work
With the aim of gaining a better understanding of users’ privacy on GitHub, we conducted an empirical study on this platform regarding users’ privacy preferences and behaviors. Our findings demonstrate that users actively engage with the platform’s privacy settings, leading to the identification of four distinct privacy profiles that emerged from a cluster analysis on the privacy settings (RQ1). For what concerns privacy behavior, we found that users share different personal information on GitHub, including family details, location, and company-related information, among other things (RQ2). This indicates that on GitHub there is a wide range of private information that can be associated with a user, beyond technical matters and surpassing information that can be hidden using privacy settings.
The synthesis of these results revealed a discrepancy between the chosen privacy settings and the actual behaviors of users (RQ3). Despite the possible explanations for interpreting this phenomenon, it is evident that privacy settings alone are insufficient to ensure users’ privacy. The last result underscores the necessity for more nuanced privacy settings on these platforms and the development of automated tools to assist users in consistently managing their actions on the platform. Given the limitations of privacy settings in protecting users’ privacy, we explored adopting models like Llama2 and BERT to detect sensitive comments and pave the way for a privacy awareness tool (RQ4).
Indeed, our work enables the creation of a privacy awareness tool on GitHub that may advice users when they are entering sensitive information in their comments. In this direction, our corpus can be exploited for prediction on sensitive comments. At a more general level, this study lays the foundation for a methodology to observe similar privacy vulnerabilities also on other platforms.
In our future research, we endeavor to enhance our understanding of the necessity for automated privacy tools on GitHub. This will be achieved through the implementation of a user survey, akin to the one made by Vasilescu et al. ( 2015a ). In this respect, it would be valuable to acquire updated data on privacy choices made by the same users in 2024. This could serve as a future work to explore the evolution of privacy attitudes and its potential impact on the use of privacy settings. Furthermore, our objective is to augment the size of the labeled corpus by leveraging Llama2, as fine-tuned in this study, or BERT. We plan to utilize this corpus to create a multi-label tool capable of predicting specific sensitive information disclosed in comments. An expanded corpus should also include issue and commit comments to ensure a more diverse range of textual data is represented in the dataset. The various types of sensitive information disclosed on this platform can have varying consequences on an individual’s life. In our future research, it is crucial to study the impact that each type of self-disclosed information may have on users’ lives. This could also enhance the effectiveness of privacy awareness tools.
Ultimately, our overarching goal is to develop a tool that suggests sanitized comments upon identifying sensitive information thus empowering the users in managing their privacy concerns. The authors are actively progressing along this trajectory.
Data Availability Statements
The experimental data and the simulation results that support the findings of this study are available in GitHub in the following address: https://github.com/MDEGroup/EMSE-CHASE-Privacy .
https://github.com/
https://github.com/MDEGroup/EMSE-CHASE-Privacy
https://bit.ly/461tL9i
https://anonymous.4open.science/
https://prn.to/3NugUWa
https://docs.github.com/en/rest?apiVersion=2022-11-28
https://huggingface.co/google-bert/bert-base-uncased
Acar Y, Stransky C, Wermke D, Mazurek ML, Fahl S (2017) Security developer studies with GitHub users: Exploring a convenience sample. In: 13th Symposium on Usable Privacy and Security (SOUPS 2017), pp 81–95
Acquisti A Fong C (2020) An Experiment in Hiring Discrimination via Online Social Networks. Manag Sci 66(3):1005–1024. https://doi.org/10.1287/mnsc.2018.3269 , https://pubsonline.informs.org/doi/abs/10.1287/mnsc.2018.3269
Acquisti A, Grossklags J (2005) Privacy and rationality in individual decision making. IEEE Secur Priv 3(1):26–33. https://doi.org/10.1109/MSP.2005.22 , https://ieeexplore.ieee.org/abstractdocument/13926 96casa_token=rS6wHgIPjCQAAAAA:WAbt9Gq1MRK7TidTwlvgnrbn3MIftH6LzTnn8NiLPfW0pqP y8IuOQk8EEtZLD-sX30_agg
Adhikari A, Das S, Dewri R (2022) Privacy policy analysis with sentence classification. In: 2022 19th Annual International Conference on Privacy, Security Trust (PST), IEEE, pp 1–10
Alaei AR, Becken S, Stantic B (2019) Sentiment analysis in tourism: capitalizing on big data. J. Travel Res. 58(2):175–191
Article Google Scholar
Autili M, Di Ruscio D, Inverardi P, Pelliccione P, Tivoli M (2019) A software exoskeleton to protect and support citizen’s ethics and privacy in the digital world. IEEE Access 7:62011–62021. https://doi.org/10.1109/ACCESS.2019.2916203 , https://doi.org/10.1109/ACCESS.2019.2916203
Bacchelli A, Beller M (2017) Double-blind review in software engineering venues: The community’s perspective. In: 2017 IEEE/ACM 39th International Conference on Software Engineering Companion (ICSE-C), pp 385–396 . https://doi.org/10.1109/ICSE-C.2017.49
Barth S (2017) de Jong MD (2017) The privacy paradox – investigating discrepancies between expressed privacy concerns nd actual online behavior – a systematic literature review. Telematics Inf. 34(7):1038–1058. https://doi.org/10.1016/j.tele.2017.04.013 , https://www.sciencedirect.com/science/article/pii/S0736585317302022
Becton JB, Walker HJ, Gilstrap JB, Schwager PH (2019) Social media snooping on job applicants: The effects of unprofessional social media information on recruiter perceptions. Pers Rev 48(5):1261–1280 . https://doi.org/10.1108/PR-09-2017-0278
Behnia R, Ebrahimi MR, Pacheco J, Padmanabhan B (2022) Ew-tune: A framework for privately fine-tuning large language models with differential privacy. In: 2022 IEEE International Conference on Data Mining Workshops (ICDMW), pp 560–566. https://doi.org/10.1109/ICDMW58026.2022.00078
Bioglio L, Pensa RG (2022) Analysis and classification of privacy-sensitive content in social media posts. EPJ Data Sci 11(1):12
Blincoe K, Sheoran J, Goggins S, Petakovic E, Damian D (2016) Understanding the popular users: Following, affiliation influence and leadership on github. Inf Softw Technol 70:30–39
Blose T, Umar P, Squicciarini A, Rajtmajer S (2020) Privacy in Crisis: A study of self-disclosure during the Coronavirus pandemic. https://doi.org/10.48550/arXiv.2004.09717
Brandäo A, Mendes R, Vilela JP (2022) Prediction of mobile app privacy preferences with user profiles via federated learning. In: Proceedings of the Twelfth ACM Conference on Data and Application Security and Privacy, pp 89–100
Casillo F, Deufemia V, Gravino C (2022) Detecting privacy requirements from user stories with nlp transfer learning models. Inf Softw Technol 146:106853
Chen Y, Zha M, Zhang N, Xu D, Zhao Q, Feng X, Yuan K, Suya F, Tian Y, Chen K et al (2019) Demystifying hidden privacy settings in mobile apps. In: 2019 IEEE Symposium on Security and Privacy (SP), IEEE, pp 570–586
Choi H, Park J, Jung Y (2018) The role of privacy fatigue in online privacy behavior. Comput Hum Behav 81:42–51
Cohen J (1960) A coefficient of agreement for nominal scales. Educ Psychol Meas 20(1):37–46
D’Acunto D, Volo S, Filieri R (2021) “most americans like their privacy." exploring privacy concerns through us guests’ reviews. Int J Contemp Hosp Manag 33(8):2773–2798
Devlin J, Chang MW, Lee K, Toutanova K (2018) Bert: Pre-training of deep bidirectional transformers for language understanding. arXiv preprint arXiv:1810.04805
Di Rocco J, Di Ruscio D, Di Sipio C, Nguyen PT, Rubei R (2021) Development of recommendation systems for software engineering: the CROSSMINER experience. Empir Softw Eng 26(4):69
Di Ruscio D, Inverardi P, Migliarini P, Nguyen PT (2024) Leveraging privacy profiles to empower users in the digital society. Autom Softw Eng 31(1):16
DiSalvo LM, Saenz GV, Wong WE, Li D (2022) Social Media Safety Practices and Flagging Sensitive Posts. In: 2022 IEEE 22nd International Conference on Software Quality, Reliability, and Security Companion (QRS-C), IEEE, pp 8–15. https://doi.org/10.1109/QRS-C57518.2022.00012 , https://ieeexplore.ieee.org/document/10076960/
El Ouirdi M, Pais I, Segers J, El Ouirdi A (2016) The relationship between recruiter characteristics and applicant assessment on social media. Comput. Hum. Behav. 62:415–422. https://doi.org/10.1016/j.chb.2016.04.012 , https://www.sciencedirect.com/science/article/pii/ S0747563216302771
Fiesler C, Dye M, Feuston JL, Hiruncharoenvate C, Hutto CJ, Morrison S, Khanipour Roshan P, Pavalanathan U, Bruckman AS, De Choudhury M et al (2017) What (or who) is public? privacy settings and social media content sharing. In: Proceedings of the 2017 ACM conference on computer supported cooperative work and social computing, pp 567–580
Ford D, Behroozi M, Serebrenik A, Parnin C (2019) Beyond the Code Itself: How Programmers Really Look at Pull Requests. In: 2019 IEEE/ACM 41st International Conference on Software Engineering: Software Engineering in Society (ICSE-SEIS), IEEE, pp 51–60. https://doi.org/10.1109/ICSE-SEIS.2019.00014 , https://ieeexplore.ieee.org/document/8797633/
Fukuyama F, Richman B, Goel A (2021) How to save democracy from technology: ending big tech’s information monopoly. Foreign Aff 100:98
Google Scholar
Garcia R, Treude C, La W (2023) Towards Understanding the Open Source Interest in Gender-Related GitHub Projects. http://arxiv.org/abs/2303.09727 ,
Gerber N, Gerber P, Volkamer M (2018) Explaining the privacy paradox: A systematic review of literature investigating privacy attitude and behavior. Comput. Secur. 77:226–261. https://doi.org/10.1016/j.cose.2018.04.002 , https://www.sciencedirect.com/science/article/pii/S0167404818303031
Gill AJ, Vasalou A, Papoutsi C, Joinson AN (2011) Privacy dictionary: a linguistic taxonomy of privacy for content analysis. In: Proceedings of the SIGCHI conference on human factors in computing systems, pp 3227–3236
Gousios G (2013) The GHTorent dataset and tool suite. In: 2013 10th Working Conference on Mining Software Repositories (MSR), pp. 233–236. https://doi.org/10.1109/MSR.2013.6624034
Guzman E, Azócar D, Li Y (2014) Sentiment analysis of commit comments in github: an empirical study. In: Proceedings of the 11th working conference on mining software repositories, pp 352–355
Hartigan JA, Wong MA (1979) Algorithm as 136: A k-means clustering algorithm. J Royal Stat Soc Ser C (Appl Stat) 28(1):100–108
Henderson KE (2019) They posted what? Recruiter use of social media for selection 48(4). https://doi.org/10.1016/j.orgdyn.2018.05.005
Henning A, Schulte L, Herbold S, Kulyk O, Mayer P (2023) Understanding issues related to personal data and data protection in open source projects on github. arXiv e-prints pp arXiv–2304
Hossin M, Sulaiman MN (2015) A review on evaluation metrics for data classification evaluations. Int J Data Min Knowl Manag Proc 5(2):1
Howard J, Ruder S (2018) Universal language model fine-tuning for text classification. arXiv preprint arXiv:1801.06146
Imtiaz N, Middleton J, Chakraborty J, Robson N, Bai G, Murphy-Hill E (2019) Investigating the Effects of Gender Bias on GitHub. In: 2019 IEEE/ACM 41st International Conference on Software Engineering (ICSE), pp 700–711. https://doi.org/10.1109/ICSE.2019.00079 , https://ieeexplore.ieee.org/abstract/document/8812110?casa_token=LUfYyiYCTGMAAAAA:mDF9o-uDnunu01ee2s5rBcSUUdhApw4mNl6K92dbHz7CXvNuZolV0P4I-ZhqPrwa3nkQdsM
Inan H, Upasani K, Chi J, Rungta R, Iyer K, Mao Y, Tontchev M, Hu Q, Fuller B, Testuggine D, Khabsa M (2023) Llama guard: Llm-based input-output safeguard for human-ai conversations. 2312:06674
Inverardi P, Migliarini P, Palmiero M (2023) Systematic review on privacy categorisation. Comput Sci Rev 49
Iyer RN, Yun SA, Nagappan M, Hoey J (2019) Effects of Personality Traits on Pull Request Acceptance 47(11):2632–2643
Jay R (2000) Uk data protection act 1998 - the human rights context. Int Rev Law Comput Technol 14(3):385–395. https://doi.org/10.1080/713673366 , https://doi.org/10.1080/713673366
Kanampiu M, Anwar M (2019) Privacy preferences vs. privacy settings: An exploratory facebook study. In: Advances in Human Factors in Cybersecurity: Proceedings of the AHFE 2018 International Conference on Human Factors in Cybersecurity, July 21-25, 2018, Loews Sapphire Falls Resort at Universal Studios, Orlando, Florida, USA 9, Springer, pp 116–126
Keküllüoglu D, Magdy W, Vaniea K (2020) Analysing privacy leakage of life events on twitter. In: Proceedings of the 12th ACM Conference on Web Science, pp 287–294
Khalajzadeh H, Shahin M, Obie HO, Grundy J (2022a) How are diverse end-user humancentric issues discussed on github? Association for Computing Machinery, New York, NY, USA, ICSE-SEIS ’22, p 79–89. https://doi.org/10.1145/3510458.3513014
Khalajzadeh H, Shahin M, Obie HO, Grundy J (2022b) How are diverse end-user humancentric issues discussed on github? In: Proceedings of the 2022 ACM/IEEE 44th International Conference on Software Engineering: Software Engineering in Society, pp 79–89
King RS (2015) Cluster analysis and data mining: An introduction. Mercury Learn Inf
Kokolakis S (2017) Privacy attitudes and privacy behaviour: A review of current research on the privacy paradox phenomenon. Comput Secur 64:122–134
Liu B, Andersen MS, Schaub F, Almuhimedi H, Zhang S, Sadeh NM, Agarwal Y, Acquisti A (2016) Follow my recommendations: A personalized privacy assistant for mobile app permissions. In: Twelfth Symposium on Usable Privacy and Security, SOUPS 2016, Denver, CO, USA, June 22-24, 2016, USENIX Association, pp 27–41. https://www.usenix.org/conference/soups2016/technical-sessions/presentation/liu
Lustgarten SD, Garrison YL, Sinnard MT, Flynn AW (2020) Digit Priv Ment Healthc Curr Issues Recomm Technol Use 36:25–31. https://doi.org/10.1016/j.copsyc.2020.03.012 , https://www.sciencedirect.com/science/article/pii/S2352250X20300415
Lu J, Yu L, Li X, Yang L, Zuo C (2023) Llama-reviewer: Advancing code review automation with large language models through parameter-efficient fine-tuning. In: 2023 IEEE 34th International Symposium on Software Reliability Engineering (ISSRE), pp 647–658. https://doi.org/10.1109/ISSRE59848.2023.00026
Matz SC, Appel RE, Kosinski M (2020) Priv Age Psychol Target 31:116–121. https://doi.org/10.1016/j.copsyc.2019.08.010 , https://www.sciencedirect.com/science/article/pii/S2352250X19301332
Meli M, McNiece MR, Reaves B (2019) How Bad Can It Git? Characterizing Secret Leakage in Public GitHub Repositories. In: Proceedings 2019 Network and Distributed System Security Symposium, Internet Society. https://doi.org/10.14722/ndss.2019.23418 , https://www.ndss-symposium.org/wp-content/uploads/2019/02/ndss2019_04B-3_Meli_paper.pdf
Migliarini P, Scoccia GL, Autili M, Inverardi P (2020) On the elicitation of privacy and ethics preferences of mobile users. In: Proceedings of the IEEE/ACM 7th International Conference on Mobile Software Engineering and Systems, pp 132–136
Miller T (2010) Surveillance: The “Digital Trail of Breadcrumbs" 2(1):9–14. https://doi.org/10.3384/cu.2000.1525.10219 , https://cultureunbound.ep.liu.se/article/view/1913
Miller C, Cohen S, Klug D, Vasilescu B, Kästner C (2022b) “did you miss my comment or what?": Understanding toxicity in open source discussions. In: Proceedings of the 44th International Conference on Software Engineering, Association for Computing Machinery, New York, NY, USA, ICSE ’22, pp 710–722. https://doi.org/10.1145/3510003.3510111 , https://doi.org/10.1145/3510003.3510111
Miller C, Cohen S, Klug D, Vasilescu B, KaUstner C (2022a) "Did you miss my comment or what?": Understanding toxicity in open source discussions. In: Proceedings of the 44th International Conference on Software Engineering, Association for Computing Machinery, ICSE ’22, pp 710–722. https://doi.org/10.1145/3510003.3510111 , https://dl.acm.org/doi/10.1145/3510003.3510111
Min B, Ross H, Sulem E, Veyseh APB, Nguyen TH, Sainz O, Agirre E, Heintz I, Roth D (2023) Recent advances in natural language processing via large pre-trained language models: A survey. ACM Comput Surv 56(2). https://doi.org/10.1145/3605943 , https://doi.org/10.1145/3605943
Nguyen TT, Wilson C, Dalins J (2023) Fine-Tuning Llama 2 Large Language Models for Detecting Online Sexual Predatory Chats and Abusive Texts. http://arxiv.org/abs/2308.14683 ,
Niu L, Mirza S, Maradni Z, Pöpper C (2023) CodexLeaks: Privacy leaks from code generation language models in GitHub copilot. In: 32nd USENIX Security Symposium (USENIX Security 23), pp 2133–2150
Pardau SL (2018) The california consumer privacy act: Towards a european-style privacy regime in the united states. J Tech L & Pol’y 23:68
Peiretti F, Pensa RG (2023) Detection of Privacy-Harming Social Media Posts in Italian. In: Arief B, Monreale A, Sirivianos M, Li S (eds) Security and Privacy in Social Networks and Big Data, Springer Nature, Lecture Notes in Computer Science, pp 203–223. https://doi.org/10.1007/978-981-99-5177-2_12
Raman N, Cao M, Tsvetkov Y, Kästner C, Vasilescu B (2020) Stress and burnout in open source: Toward finding, understanding, and mitigating unhealthy interactions. In: Proceedings of the ACM/IEEE 42nd International Conference on Software Engineering: New Ideas and Emerging Results, Association for Computing Machinery, ICSE-NIER ’20, pp 57–60. https://doi.org/10.1145/3377816.3381732 , https://dl.acm.org/doi/10.1145/3377816.3381732
Robillard MP, Walker RJ, Zimmermann T (2010) Recommendation systems for software engineering. IEEE Softw 27(4):80–86. https://doi.org/10.1109/MS.2009.161 , https://doi.org/10.1109/MS.2009.161
Sajadi A, Damevski K, Chatterjee P (2023) Interpersonal Trust in OSS: Exploring Dimensions of Trust in GitHub Pull Requests. In: 2023 IEEE/ACM 45th International Conference on Software Engineering: New Ideas and Emerging Results (ICSE-NIER), pp 19–24. https://doi.org/10.1109/ICSE-NIER58687.2023.00010 , https://ieeexplore.ieee.org/abstract/document/10173872?casa_token=hBC8Zu0afC0AAAAA:-ozSXKFFvF78i5L9Srsw6nNuHzZYi5qzPKtg5MyrZsEPiKKnVEFtgnhUiP9Tulnkh-EapOo-OmE
Sanchez OR, Torre I, He Y, Knijnenburg BP (2020) A recommendation approach for user privacy preferences in the fitness domain. User Model User-Adap Inter 30:513–565
Solove DJ (2021) The myth of the privacy paradox. Geo Wash L Rev 89:1
Stretton T, Aaron L (2015) Dangers Our Trail Digit Breadcrumbs 2015(1):13–15. https://doi.org/10.1016/S1361-3723(15)70006-0 , https://www.sciencedirect.com/science/article/pii/S1361372315700060
Syakur M, Khotimah B, Rochman E, Satoto BD (2018) Integration k-means clustering method and elbow method for identification of the best customer profile cluster. In: IOP conference series: materials science and engineering, vol 336, p 012017. IOP Publishing
Tadesse MM, Lin H, Xu B, Yang L (2019) Detection of Depression-Related Posts in Reddit Social Media. Forum 7:44883–44893. https://doi.org/10.1109/ACCESS.2019.2909180 , https://ieeexplore.ieee.org/abstract/document/8681445
Tahaei M, Vaniea K, Saphra N (2020) Understanding privacy-related questions on stack overflow. In: Proceedings of the 2020 CHI conference on human factors in computing systems, pp 1–14
Tang R, Han X, Jiang X, Hu X (2023) Does Synthetic Data Generation of LLMs Help Clinical Text Mining?
Terrell J, Kofink A, Middleton J, Rainear C, Murphy-Hill E, Parnin C, Stallings J (2017) Gender differences and bias in open source: Pull request acceptance of women versus men. PeerJ Comput Sci 3
Timoshenko A, Hauser JR (2019) Identifying Customer Needs from User-Generated Content 38(1):1–20. https://doi.org/10.1287/mksc.2018.1123 , https://pubsonline.informs.org/doi/abs/10.1287/mksc.2018.1123
Touvron H, Lavril T, Izacard G, Martinet X, Lachaux MA, Lacroix T, Roziére B, Goyal N, Hambro E, Azhar F et al (2023) Llama: Open and efficient foundation language models. arXiv preprint arXiv:2302.13971
Umar P, Squicciarini A, Rajtmajer S (2019) Detection and Analysis of Self-Disclosure in Online News Commentaries. In: The World Wide Web Conference, ACM, pp 3272–3278. https://doi.org/10.1145/3308558.3313669 , https://dl.acm.org/doi/10.1145/3308558.3313669
Vasalou A, Gill A, Mazanderani F, Papoutsi C, Joinson A (2011) Privacy dictionary: A new resource for the automated content analysis of privacy 62(11):2095–2105. https://doi.org/10.1002/asi.21610
Vasilescu B, Filkov V, Serebrenik A (2015a) Perceptions of Diversity on Git Hub: A User Survey. In: 2015 IEEE/ACM 8th International Workshop on Cooperative and Human Aspects of Software Engineering, pp 50–56. https://doi.org/10.1109/CHASE.2015.14 , https://ieeexplore.ieee.org/abstract/document/7166088
Vasilescu B, Posnett D, Ray B, van den Brand MG, Serebrenik A, Devanbu P, Filkov V (2015b) Gender and tenure diversity in github teams. In: Proceedings of the 33rd annual ACM conference on human factors in computing systems, pp 3789–3798
Vasilescu B, Serebrenik A, Filkov V (2015c) A Data Set for Socia Diversity Studies of GitHub Teams. In: 2015 IEEE/ACM 12th Working Conference on Mining Software Repositories, pp 514–517. https://doi.org/10.1109/MSR.2015.77
Voigt P, Von dem Bussche A (2017) The eu general data protection regulation (gdpr). A Practical Guide, 1st Ed, Cham: Springer International Publishing 10(3152676):10–5555
Wang J, Zhang X, Chen L, Xie X (2022) Personalizing label prediction for github issues. Inf Softw Technol 145:106845
Warrens MJ (2015) Five ways to look at cohen’s kappa. J Psychol & Psychother 5
Yao Y, Duan J, Xu K, Cai Y, Sun Z, Zhang Y (2024) A survey on large language model (llm) security and privacy: The good, the bad, and the ugly. High-Confidence Comput. 4(2):100211. https://doi.org/10.1016/j.hcc.2024.100211 , https://www.sciencedirect.com/science/article/pii/S266729522400014X
Zhang S, Feng Y, Bauer L, Cranor LF, Das A, Sadeh N (2021) “did you know this camera tracks your mood?": Understanding privacy expectations and preferences in the age of video analytics. Proc Priv Enhancing Technol 2021(2)
Zhang S, Feng Y, Das A, Bauer L, Cranor LF, Sadeh N (2020) Understanding people’s privacy attitudes towards video analytics technologies. Proceedings of the FTC PrivacyCon, pp 1–18
Download references
Acknowledgements
This work has been partially supported by: MUR project 2020 “EMELIOT” grant n. 2020W3A5FY; MUR projects PRIN 2022 PNRR: “FRINGE: context-aware FaiRness engineerING in complex software systEms” grant n. P2022553SL, “TRex-SE: Trustworthy Recommenders for Software Engineers” grant n. 2022LKJWHC, and “HALO: etHical-aware AdjustabLe autOnomous systems” grant n. 2022JKA4SL. We also acknowledge the MUR Department of Excellence 2023 - 2027 program. We thank the anonymous reviewers for their useful comments and suggestions that helped us improve our manuscript.
Open access funding provided by Università degli Studi dell’Aquila within the CRUI-CARE Agreement.
Author information
Authors and affiliations.
DISIM - University of L’Aquila, L’Aquila, Italy
Costanza Alfieri, Juri Di Rocco & Phuong T. Nguyen
Gran Sasso Science Institute, L’Aquila, Italy
Paola Inverardi
You can also search for this author in PubMed Google Scholar
Corresponding author
Correspondence to Costanza Alfieri .
Ethics declarations
Conflict of interests.
The authors have no relevant financial or non-financial interests to declare.
Additional information
Communicated by: Fabio Calefato, Hourieh Khalajzadeh and Igor Steinmacher
Publisher's Note
Springer Nature remains neutral with regard to jurisdictional claims in published maps and institutional affiliations.
This article belongs to the Topical Collection: Special Issue on CHASE 2023 .
Rights and permissions
Open Access This article is licensed under a Creative Commons Attribution 4.0 International License, which permits use, sharing, adaptation, distribution and reproduction in any medium or format, as long as you give appropriate credit to the original author(s) and the source, provide a link to the Creative Commons licence, and indicate if changes were made. The images or other third party material in this article are included in the article's Creative Commons licence, unless indicated otherwise in a credit line to the material. If material is not included in the article's Creative Commons licence and your intended use is not permitted by statutory regulation or exceeds the permitted use, you will need to obtain permission directly from the copyright holder. To view a copy of this licence, visit http://creativecommons.org/licenses/by/4.0/ .
Reprints and permissions
About this article
Alfieri, C., Di Rocco, J., Inverardi, P. et al. Exploring user privacy awareness on GitHub: an empirical study. Empir Software Eng 29 , 156 (2024). https://doi.org/10.1007/s10664-024-10544-7
Download citation
Accepted : 05 September 2024
Published : 27 September 2024
DOI : https://doi.org/10.1007/s10664-024-10544-7
Share this article
Anyone you share the following link with will be able to read this content:
Sorry, a shareable link is not currently available for this article.
Provided by the Springer Nature SharedIt content-sharing initiative
- Empirical study
- Experience report
- Sensitivity detection
- Large language models
- Privacy profile
Advertisement
- Find a journal
- Publish with us
- Track your research

IMAGES
VIDEO
COMMENTS
Learn the importance and types of limitations in research and how to write them effectively. This article provides guidelines, examples, and tips for acknowledging and addressing the practical or theoretical shortcomings of a study.
Scope of social research is to make research manageable, handy, researchable, optimal. and SMART. Researchers need to determine the scope very early enough in the research cycle. Aside from the ...
In conclusion (of what is scope and limitation in research), scope and limitation are integral components of any research project. Understanding the scope helps researchers define the boundaries and parameters of their study, while acknowledging limitations ensures transparency and credibility. By carefully considering scope and limitation ...
Learn how to write the scope of the research, which is the range of topics, areas, and subjects that a research project intends to cover. See examples of scope of the research for different types of studies and learn when and why to write it.
Your study's scope and delimitations are the sections where you define the broader parameters and boundaries of your research. The scope details what your study will explore, such as the target population, extent, or study duration. Delimitations are factors and variables not included in the study. Scope and delimitations are not methodological ...
How to Write the Scope of the Study. Take home message. The scope of the study is defined at the start of the research project before data collection begins. It is used by researchers to set the boundaries and limitations within which the study will be performed. In this post you will learn exactly what the scope of the study means, why it is ...
Learn how to define and write the scope and delimitations of a study, which help to contextualize and convey the focus and boundaries of a research project. Find examples, tips, and differentiation between delimitations and limitations.
Delimitations refer to the specific boundaries or limitations that are set in a research study in order to narrow its scope and focus. Delimitations may be related to a variety of factors, including the population being studied, the geographical location, the time period, the research design, and the methods or tools being used to collect data.
Example 1. Research question: What are the effects of social media on mental health? Scope: The scope of the study will focus on the impact of social media on the mental health of young adults aged 18-24 in the United States. Delimitation: The study will specifically examine the following aspects of social media: frequency of use, types of social media platforms used, and the impact of social ...
Learn how to define the scope and delimitations of your research topic, and how to write them clearly and effectively. Find out the difference between delimitations and limitations, and see examples of both.
Purpose of Limitations in Research. There are several purposes of limitations in research. Here are some of the most important ones: To acknowledge the boundaries of the study: Limitations help to define the scope of the research project and set realistic expectations for the findings. They can help to clarify what the study is not intended to ...
The scope of the study refers to the parameters under which the study will be operating -- what the study covers -- and is closely connected to the framing of the problem. The problem you seek to resolve will fit within certain parameters. Think of the scope as the domain of your research—what's in the study domain, and what is not.
Possible Limitations of the Researcher. Access-- if your study depends on having access to people, organizations, data, or documents and, for whatever reason, access is denied or limited in some way, the reasons for this needs to be described.Also, include an explanation why being denied or limited access did not prevent you from following through on your study.
Learn how to identify the research topic, problem statement, objectives, questions, scope, and limitations for your education project. Follow a six-step process to design a valid, reliable, and meaningful study that addresses a knowledge gap in your field.
Scope of research is the part of your project that defines what will and will not be covered. Learn how to consider budget, timeline, population, sample, methodology, and variables when defining your scope.
Limitations of a dissertation are potential weaknesses in your study that are mostly out of your control, given limited funding, choice of research design, statistical model constraints, or other factors. In addition, a limitation is a restriction on your study that cannot be reasonably dismissed and can affect your design and results.
Every study, no matter how well it is conducted and constructed, has limitations. This is one of the reasons why we do not use the words "prove" and "disprove" with respect to research findings. It is always possible that future research may cast doubt on the validity of any hypothesis or conclusion from a study.
sentence tha. signals what you're about to discu. s. For example:"Our study had some limitations."Then, provide a concise sentence or two identifying each limitation and explaining how the limitation may have affected the quality. of the study. s findings and/or their applicability. For example:"First, owing to the rarity of the ...
Scope and delimitations are two elements of a research paper or thesis. The scope of a study explains the extent to which the research area will be explored in the work and specifies the parameters within which the study will be operating. For example, let's say a researcher wants to study the impact of mobile phones on behavior patterns of ...
The scope of a study explains the extent to which the research area will be explored in the work and specifies the parameters within the study will be operating. Basically, this means that you will have to define what the study is going to cover and what it is focusing on. Similarly, you also have to define what the study is not going to cover.
Don't just list key weaknesses and the magnitude of a study's limitations. To do so diminishes the validity of your research because it leaves the reader wondering whether, or in what ways, limitation(s) in your study may have impacted the results and conclusions. Limitations require a critical, overall appraisal and interpretation of their impact.
Researchers may focus on specific research issues and avoid potential biases or inaccuracies by considering the study's scope and limitations (Akanle et al., 2020). In this study, the behavior and ...
Answer: In simple words, the scope of a study refers to all the aspects that it will cover or the boundaries within which it will be performed. This means that researchers have to define what the study is focusing on. Similarly, they have to define what the study is not going to cover (these often comprise the limitations of the study).
Our research offers insights into the utilization of existing privacy protection tools, such as privacy settings, along with their inherent limitations. Given the interest in developing privacy assistants (e.g., Liu et al. ( 2016 )), our research supports this field by providing both the motivation for creating such privacy protection tools and ...