Thank you for visiting nature.com. You are using a browser version with limited support for CSS. To obtain the best experience, we recommend you use a more up to date browser (or turn off compatibility mode in Internet Explorer). In the meantime, to ensure continued support, we are displaying the site without styles and JavaScript.
- View all journals
- Explore content
- About the journal
- Publish with us
- Sign up for alerts
- Review Article
- Published: 12 March 2024

Deep learning for water quality
- Wei Zhi 1 , 2 ,
- Alison P. Appling ORCID: orcid.org/0000-0003-3638-8572 3 ,
- Heather E. Golden ORCID: orcid.org/0000-0001-5501-9444 4 ,
- Joel Podgorski ORCID: orcid.org/0000-0003-2522-1021 5 &
- Li Li ORCID: orcid.org/0000-0002-1641-3710 2
Nature Water volume 2 , pages 228–241 ( 2024 ) Cite this article
5391 Accesses
50 Altmetric
Metrics details
- Environmental sciences
Understanding and predicting the quality of inland waters are challenging, particularly in the context of intensifying climate extremes expected in the future. These challenges arise partly due to complex processes that regulate water quality, and arduous and expensive data collection that exacerbate the issue of data scarcity. Traditional process-based and statistical models often fall short in predicting water quality. In this Review, we posit that deep learning represents an underutilized yet promising approach that can unravel intricate structures and relationships in high-dimensional data. We demonstrate that deep learning methods can help address data scarcity by filling temporal and spatial gaps and aid in formulating and testing hypotheses via identifying influential drivers of water quality. This Review highlights the strengths and limitations of deep learning methods relative to traditional approaches, and underscores its potential as an emerging and indispensable approach in overcoming challenges and discovering new knowledge in water-quality sciences.
This is a preview of subscription content, access via your institution
Access options
Subscribe to this journal
Receive 12 digital issues and online access to articles
92,52 € per year
only 7,71 € per issue
Buy this article
- Purchase on Springer Link
- Instant access to full article PDF
Prices may be subject to local taxes which are calculated during checkout
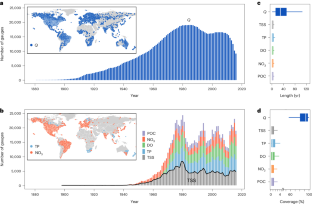
Similar content being viewed by others
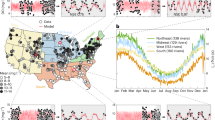
Temperature outweighs light and flow as the predominant driver of dissolved oxygen in US rivers
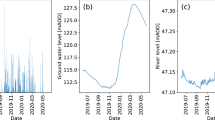
Machine learning approach towards explaining water quality dynamics in an urbanised river
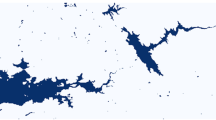
ReaLSAT, a global dataset of reservoir and lake surface area variations
Data availability.
Streamflow data (Fig. 1a ) from the Global Streamflow Indices and Metadata Archive (GSIM) were compiled from repositories at https://doi.org/10.1594/PANGAEA.887477 and https://doi.org/10.1594/PANGAEA.887470 . Water-quality data (Fig. 1b ) from the Global River Water Quality Archive (GRQA) were downloaded from https://doi.org/10.5281/zenodo.7056647 .
Turing, A. M. Computing machinery and intelligence. Mind 59 , 433–460 (1950).
Article Google Scholar
Turing, A. M. On computable numbers, with an application to the Entscheidungsproblem. Proc. Lond. Math. Soc. s2-42 , 230–265 (1937).
Muggleton, S. Alan Turing and the development of artificial intelligence. AI Commun. 27 , 3–10 (2014).
Dechter, R. Learning while searching in constraint-satisfaction problems. In Proceedings of the AAAI Conference on Artificial Intelligence Vol. 5, 178–183 (AAAI, 1986).
Hinton, G. E. & Salakhutdinov, R. R. Reducing the dimensionality of data with neural networks. Science 313 , 504–507 (2006).
Article CAS PubMed Google Scholar
Hinton, G. E., Osindero, S. & Teh, Y.-W. A fast learning algorithm for deep belief nets. Neural Comput. 18 , 1527–1554 (2006).
Article PubMed Google Scholar
Lecun, Y., Bengio, Y. & Hinton, G. Deep learning. Nature 521 , 436–444 (2015).
Reichstein, M. et al. Deep learning and process understanding for data-driven Earth system science. Nature 566 , 195–204 (2019).
Shen, C. A transdisciplinary review of deep learning research and its relevance for water resources scientists. Water Resour. Res. 54 , 8558–8593 (2018).
Shen, C. P. et al. HESS opinions: incubating deep-learning-powered hydrologic science advances as a community. Hydrol. Earth Syst. Sci. 22 , 5639–5656 (2018).
Xu, T. & Liang, F. Machine learning for hydrologic sciences: an introductory overview. Wiley Interdiscip. Rev. Water 8 , e1533 (2021).
Zhi, W. et al. From hydrometeorology to river water quality: can a deep learning model predict dissolved oxygen at the continental scale? Environ. Sci. Technol. 55 , 2357–2368 (2021).
Varadharajan, C. et al. Can machine learning accelerate process understanding and decision‐relevant predictions of river water quality? Hydrol. Process. https://doi.org/10.1002/hyp.14565 (2022).
Tripathy, K. P. & Mishra, A. K. Deep learning in hydrology and water resources disciplines: concepts, methods, applications, and research directions. J. Hydrol. https://doi.org/10.1016/j.jhydrol.2023.130458 (2023).
Perry, G. L. W., Seidl, R., Bellvé, A. M. & Rammer, W. An outlook for deep learning in ecosystem science. Ecosystems 25 , 1700–1718 (2022).
Song, T. et al. A review of artificial intelligence in marine science. Front. Earth Sci. https://doi.org/10.3389/feart.2023.1090185 (2023).
Sun, A. Y. & Scanlon, B. R. How can big data and machine learning benefit environment and water management: a survey of methods, applications, and future directions. Environ. Res. Lett. 14 , 073001 (2019).
Zhu, J.-J., Yang, M. & Ren, Z. J. Machine learning in environmental research: common pitfalls and best practices. Environ. Sci. Technol. 57 , 17671–17689 (2023).
van Klompenburg, T., Kassahun, A. & Catal, C. Crop yield prediction using machine learning: a systematic literature review. Comput. Electron. Agric. 177 , 105709 (2020).
Zhong, S. et al. Machine learning: new ideas and tools in environmental science and engineering. Environ. Sci. Technol. https://doi.org/10.1021/acs.est.1c01339 (2021).
Appling, A. P., Oliver, S. K., Read, J. S., Sadler, J. M. & Zwart, J. A. in Encyclopedia of Inland Waters 2nd edn (eds Mehner, T. & Tockner, K.) 585–606 (Elsevier, 2022).
Shen, C. et al. Differentiable modelling to unify machine learning and physical models for geosciences. Nat. Rev. Earth Environ. 4 , 552–567 (2023).
Diamond, J. S. et al. Hypoxia is common in temperate headwaters and driven by hydrological extremes. Ecol. Indic. 147 , 109987 (2023).
Article CAS Google Scholar
Creed, I. F. et al. Enhancing protection for vulnerable waters. Nat. Geosci. 10 , 809–815 (2017).
Article CAS PubMed PubMed Central Google Scholar
Nazari-Sharabian, M., Ahmad, S. & Karakouzian, M. Climate change and eutrophication: a short review. Eng. Technol. Appl. Sci. Res. 8 , 3668 (2018).
Paerl, H. W., Otten, T. G. & Kudela, R. Mitigating the expansion of harmful algal blooms across the freshwater-to-marine continuum. Environ. Sci. Technol. 52 , 5519–5529 (2018).
McCabe, M. F. et al. The future of Earth observation in hydrology. Hydrol. Earth Syst. Sci. 21 , 3879–3914 (2017).
Article PubMed PubMed Central Google Scholar
Abbott, B. W. et al. Using multi-tracer inference to move beyond single-catchment ecohydrology. Earth Sci. Rev. 160 , 19–42 (2016).
Milly, P. C. D. et al. Stationarity is dead: whither water management? Science 319 , 573–574 (2008).
Runkel, R. L., Crawford, C. G. & Cohn, T. A. Load Estimator (LOADEST): A FORTRAN Program for Estimating Constituent Loads in Streams and Rivers Report no. 4-A5 (USGS, 2004).
Hirsch, R. M., Moyer, D. L. & Archfield, S. A. Weighted regressions on time, discharge, and season (WRTDS), with an application to Chesapeake Bay River inputs. J. Am. Water Resour. Assoc. 46 , 857–880 (2010).
Zhang, Q., Blomquist, J. D., Moyer, D. L. & Chanat, J. G. Estimation bias in water-quality constituent concentrations and fluxes: a synthesis for Chesapeake Bay rivers and streams. Front. Ecol. Evol. https://doi.org/10.3389/fevo.2019.00109 (2019).
Zhi, W. et al. Distinct source water chemistry shapes contrasting concentration–discharge patterns. Water Resour. Res. 55 , 4233–4251 (2019).
Archfield, S. A. et al. Accelerating advances in continental domain hydrologic modeling. Water Resour. Res. 51 , 10078–10091 (2015).
Zhi, W. et al. BioRT-Flux-PIHM v1.0: a watershed biogeochemical reactive transport model. Geosci. Model Dev. 15 , 19 (2022).
Rahmani, F. et al. Exploring the exceptional performance of a deep learning stream temperature model and the value of streamflow data. Environ. Res. Lett. https://doi.org/10.1088/1748-9326/abd501 (2021).
Bolton, T. & Zanna, L. Applications of deep learning to ocean data inference and subgrid parameterization. J. Adv. Model. Earth Syst. 11 , 376–399 (2019).
Rasp, S., Pritchard, M. S. & Gentine, P. Deep learning to represent subgrid processes in climate models. Proc. Natl Acad. Sci. USA 115 , 9684–9689 (2018).
Irrgang, C. et al. Towards neural Earth system modelling by integrating artificial intelligence in Earth system science. Nat. Mach. Intell. 3 , 667–674 (2021).
Zhi, W., Ouyang, W., Shen, C. & Li, L. Temperature outweighs light and flow as the predominant driver of dissolved oxygen in US rivers. Nat. Water 1 , 249–260 (2023).
Willard, J. D. et al. Predicting water temperature dynamics of unmonitored lakes with meta-transfer learning. Water Resour. Res. 57 , e2021WR029579 (2021).
He, Y. et al. Water clarity mapping of global lakes using a novel hybrid deep-learning-based recurrent model with Landsat OLI images. Water Res. 215 , 118241 (2022).
Jia, X. et al. Physics-guided recurrent graph model for predicting flow and temperature in river networks. In Proc. 2021 SIAM International Conference on Data Mining (SDM) 612–620 (SIAM, 2021); https://doi.org/10.1137/1.9781611976700.69
Bao, T. et al. Partial differential equation driven dynamic graph networks for predicting stream water temperature. In 2021 IEEE International Conference on Data Mining (ICDM) 11–20 (IEEE, 2021); https://doi.org/10.1109/ICDM51629.2021.00011
Bindas, T. et al. Improving river routing using a differentiable Muskingum‐Cunge model and physics‐informed machine learning. Water Resour. Res. 60 , e2023WR035337 (2024).
Chen, S. et al. Heterogeneous stream-reservoir graph networks with data assimilation. In 2021 IEEE International Conference on Data Mining (ICDM) 1024–1029 (IEEE, 2021).
Chen, S., Zwart, J. A. & Jia, X. Physics-guided graph meta learning for predicting water temperature and streamflow in stream networks. In Proc. 28th ACM SIGKDD Conference on Knowledge Discovery and Data Mining 2752–2761 (Association for Computing Machinery, 2022).
Saha, G. K., Rahmani, F., Shen, C., Li, L. & Cibin, R. A deep learning-based novel approach to generate continuous daily stream nitrate concentration for nitrate data-sparse watersheds. Sci. Total Environ. 878 , 162930 (2023).
Kratzert, F. et al. Towards learning universal, regional, and local hydrological behaviors via machine learning applied to large-sample datasets. Hydrol. Earth Syst. Sci. 23 , 5089–5110 (2019).
Willard, J. D., Read, J. S., Topp, S., Hansen, G. J. A. & Kumar, V. Daily surface temperatures for 185,549 lakes in the conterminous United States estimated using deep learning (1980–2020). Limnol. Oceanogr. Lett. https://doi.org/10.1002/lol2.10249 (2022).
Ren, H., Cromwell, E., Kravitz, B. & Chen, X. Using long short-term memory models to fill data gaps in hydrological monitoring networks. Hydrol. Earth Syst. Sci. 26 , 1727–1743 (2022).
Latif, S. D. et al. Sediment load prediction in Johor River: deep learning versus machine learning models. Appl. Water Sci. https://doi.org/10.1007/s13201-023-01874-w (2023).
Jamei, M. et al. Designing a decomposition-based multi-phase pre-processing strategy coupled with EDBi-LSTM deep learning approach for sediment load forecasting. Ecol. Indic. 153 , 110478 (2023).
Hill, P. R., Kumar, A., Temimi, M. & Bull, D. R. HABNet: machine learning, remote sensing-based detection of harmful algal blooms. IEEE J. Sel. Top. Appl. Earth Obs. Remote Sens. 13 , 3229–3239 (2020).
D’Alimonte, D., Zibordi, G. & Berthon, J. F. Determination of CDOM and NPPM absorption coefficient spectra from coastal water remote sensing reflectance. IEEE Trans. Geosci. Remote Sens. 42 , 1770–1777 (2004).
Zhang, Y. et al. Improving remote sensing estimation of Secchi disk depth for global lakes and reservoirs using machine learning methods. GIsci. Remote Sens. 59 , 1367–1383 (2022).
Li, L. et al. River water quality shaped by land–river connectivity in a changing climate. Nat. Clim. Change https://doi.org/10.1038/s41558-023-01923-x (2024).
Rasmussen, P. P., Gray, J. R., Glysson, G. D. & Ziegler, A. C. Guidelines and Procedures for Computing Time-Series Suspended-Sediment Concentrations and Loads from In-Stream Turbidity-Sensor and Streamflow Report No. 3-C4 (USGS, 2009).
Covert, S. A., Bunch, A. R., Crawford, C. G. & Oelsner, G. P. Comparison of Surrogate Models to Estimate Pesticide Concentrations at Six U.S. Geological Survey National Water Quality Network Sites during Water Years 2013–18 Report No. 2022-5109 (USGS, 2023).
Schilling, K. E., Kim, S.-W. & Jones, C. S. Use of water quality surrogates to estimate total phosphorus concentrations in Iowa rivers. J. Hydrol. Reg. Stud. 12 , 111–121 (2017).
Wang, D. et al. Satellite retrieval of surface water nutrients in the coastal regions of the East China Sea. Remote Sens. 10 , 1896 (2018).
Guo, H. et al. Performance of deep learning in mapping water quality of Lake Simcoe with long-term Landsat archive. ISPRS J. Photogramm. Remote Sens. 183 , 451–469 (2022).
Kerins, D. & Li, L. High dissolved carbon concentration in arid rocky mountain streams. Environ. Sci. Technol. 57 , 4656–4667 (2023).
Zhang, Y. et al. A hybrid model combining mode decomposition and deep learning algorithms for detecting TP in urban sewer networks. Appl. Energy 333 , 120600 (2023).
Jiang, Y., Li, C., Song, H. & Wang, W. Deep learning model based on urban multi-source data for predicting heavy metals (Cu, Zn, Ni, Cr) in industrial sewer networks. J. Hazard. Mater. https://doi.org/10.1016/j.jhazmat.2022.128732 (2022).
Li, L. et al. Expanding the role of reactive transport models in critical zone processes. Earth Sci. Rev. 165 , 280–301 (2017).
Li, L. et al. Toward catchment hydro-biogeochemical theories. WIREs Water 8 , e1495 (2021).
Bergen, K. J., Johnson, P. A., de Hoop, M. V. & Beroza, G. C. Machine learning for data-driven discovery in solid Earth geoscience. Science 363 , eaau0323 (2019).
Kolbe, T. et al. Stratification of reactivity determines nitrate removal in groundwater. Proc. Natl Acad. Sci. USA 116 , 2494–2499 (2019).
Sun, A. Y. Discovering state‐parameter mappings in subsurface models using generative adversarial networks. Geophys. Res. Lett. https://doi.org/10.1029/2018gl080404 (2018).
Jiang, P., Shuai, P., Sun, A., Mudunuru, M. K. & Chen, X. Knowledge-informed deep learning for hydrological model calibration: an application to Coal Creek Watershed in Colorado. Hydrol. Earth Syst. Sci. Discuss. 2022 , 1–31 (2022).
Google Scholar
Jiang, Z. et al. Sub3DNet1.0: a deep-learning model for regional-scale 3D subsurface structure mapping. Geosci. Model Dev. 14 , 3421–3435 (2021).
Cromwell, E. et al. Estimating watershed subsurface permeability from stream discharge data using deep neural networks. Front. Earth Sci. https://doi.org/10.3389/feart.2021.613011 (2021).
Podgorski, J. & Berg, M. Global threat of arsenic in groundwater. Science 368 , 845–850 (2020).
Podgorski, J. & Berg, M. Global analysis and prediction of fluoride in groundwater. Nat. Commun. https://doi.org/10.1038/s41467-022-31940-x (2022).
Ransom, K. M., Nolan, B. T., Stackelberg, P. E., Belitz, K. & Fram, M. S. Machine learning predictions of nitrate in groundwater used for drinking supply in the conterminous United States. Sci. Total Environ. 807 , 151065 (2022).
Nolan, B. T., Green, C. T., Juckem, P. F., Liao, L. & Reddy, J. E. Metamodeling and mapping of nitrate flux in the unsaturated zone and groundwater, Wisconsin, USA. J. Hydrol. 559 , 428–441 (2018).
Wen, T., Liu, M., Woda, J., Zheng, G. & Brantley, S. L. Detecting anomalous methane in groundwater within hydrocarbon production areas across the United States. Water Res. 200 , 117236 (2021).
Willard, J., Jia, X., Xu, S., Steinbach, M. & Kumar, V. Integrating scientific knowledge with machine learning for engineering and environmental systems. ACM Comput. Surv. 55 , 1–37 (2023).
Feng, D., Beck, H., Lawson, K. & Shen, C. The suitability of differentiable, learnable hydrologic models for ungauged regions and climate change impact assessment. Hydrol. Earth Syst. Sci. Discuss. 2022 , 1–28 (2022).
Sun, A. Y., Yoon, H., Shih, C.-Y. & Zhong, Z. Applications of physics-informed scientific machine learning in subsurface science: A survey. In Knowledge Guided Machine Learning (eds Karpatne, A. et al.) 111–132 (Chapman and Hall/CRC, 2022).
Read, J. S. et al. Process‐guided deep learning predictions of lake water temperature. Water Resour. Res. 55 , 9173–9190 (2019).
Beucler, T., Rasp, S., Pritchard, M. & Gentine, P. Achieving conservation of energy in neural network emulators for climate modeling. Preprint at https://arxiv.org/abs/1906.06622 (2019).
Daw, A. et al. Physics-Guided Architecture (PGA) of Neural Networks for Quantifying Uncertainty in Lake Temperature Modeling. In Proc. 2020 SIAM International Conference on Data Mining (SDM) 532–540 (SIAM, 2020).
Shen, C. et al. Differentiable modelling to unify machine learning and physical models for geosciences. Nat. Rev. Earth Environ. https://doi.org/10.1038/s43017-023-00450-9 (2023).
Jia, X. et al. Physics Guided RNNs for Modeling Dynamical Systems: A Case Study in Simulating Lake Temperature Profiles. In Proceedings of the 2019 SIAM International Conference on Data Mining 558–566 (SIAM, 2019).
Sadler, J. M. et al. Multi-task deep learning of daily streamflow and water temperature. Water Resour. Res. 58 , e2021WR030138 (2022).
He, Q., Barajas-Solano, D., Tartakovsky, G. & Tartakovsky, A. M. Physics-informed neural networks for multiphysics data assimilation with application to subsurface transport. Adv. Water Res. 141 , 103610 (2020).
Jiang, S., Zheng, Y. & Solomatine, D. Improving AI system awareness of geoscience knowledge: symbiotic integration of physical approaches and deep learning. Geophys. Res. Lett. https://doi.org/10.1029/2020gl088229 (2020).
Höge, M., Scheidegger, A., Baity-Jesi, M., Albert, C. & Fenicia, F. Improving hydrologic models for predictions and process understanding using neural ODEs. Hydrol. Earth Syst. Sci. 26 , 5085–5102 (2022).
Molnar, C. Interpretable Machine Learning (Lulu.com, 2020).
Samek, W., Montavon, G., Lapuschkin, S., Anders, C. J. & Muller, K.-R. Explaining deep neural networks and beyond: a review of methods and applications. Proc. IEEE 109 , 247–278 (2021).
Sundararajan, M., Taly, A. & Yan, Q. Axiomatic attribution for deep networks. In International Conference on Machine Learning 3319–3328 (ICML, 2017).
Erion, G. et al. Improving performance of deep learning models with axiomatic attribution priors and expected gradients. Nat. Mach. Intell. 3 , 620–631 (2021).
Lundberg, S. M. & Lee, S.-I. A unified approach to interpreting model predictions. Adv. Neural Inf. Process. Syst. 30 , 1–10 (2017).
Ribeiro, M. T., Singh, S. & Guestrin, C. " Why should i trust you?" Explaining the predictions of any classifier. In Proc. 22nd ACM SIGKDD International Conference on Knowledge Discovery and Data Mining 1135–1144 (ACM, 2016).
Xie, W. et al. Interpretable framework of physics‐guided neural network with attention mechanism: simulating paddy field water temperature variations. Water Resour. Res. 58 , e2021WR030493 (2022).
Liu, Y., Duffy, K., Dy, J. G. & Ganguly, A. R. Explainable deep learning for insights in El Niño and river flows. Nat. Commun. https://doi.org/10.1038/s41467-023-35968-5 (2023).
Sadayappan, K., Kerins, D., Shen, C. & Li, L. Nitrate concentrations predominantly driven by human, climate, and soil properties in US rivers. Water Res. 226 , 119295 (2022).
Topp, S. N. et al. Stream temperature prediction in a shifting environment: explaining the influence of deep learning architecture. Water Resour. Res. https://doi.org/10.1029/2022wr033880 (2023).
Lee, D. et al. Integrated explainable deep learning prediction of harmful algal blooms. Technol. Forecast. Soc. Change 185 , 122046 (2022).
Zheng, H., Liu, Y., Wan, W., Zhao, J. & Xie, G. Large-scale prediction of stream water quality using an interpretable deep learning approach. J. Environ. Manage. 331 , 117309 (2023).
Hanson, P. C. et al. Predicting lake surface water phosphorus dynamics using process-guided machine learning. Ecol. Modell. 430 , 109136 (2020).
Carpenter, S. R., Booth, E. G. & Kucharik, C. J. Extreme precipitation and phosphorus loads from two agricultural watersheds. Limnol. Oceanogr. 63 , 1221–1233 (2018).
Robinne, F.-N. et al. Scientists’ warning on extreme wildfire risks to water supply. Hydrol. Process. 35 , e14086 (2021).
Whitehead, P. G., Wilby, R. L., Battarbee, R. W., Kernan, M. & Wade, A. J. A review of the potential impacts of climate change on surface water quality. Hydrol. Sci. J. 54 , 101–123 (2009).
Wang, P. et al. Exploring the application of artificial intelligence technology for identification of water pollution characteristics and tracing the source of water quality pollutants. Sci. Total Environ. 693 , 133440 (2019).
Kontos, Y. N., Kassandros, T., Katsifarakis, K. L. & Karatzas, K. Deep Learning Modeling of Groundwater Pollution Sources. In International Conference on Engineering Applications of Neural Networks 165–177 (Springer, 2021).
Zwart, J. A. et al. Near-term forecasts of stream temperature using deep learning and data assimilation in support of management decisions. J. Am. Water Res. Assoc. 59 , 317–337 (2023).
van Lieshout, C., van Oeveren, K., van Emmerik, T. & Postma, E. Automated river plastic monitoring using deep learning and cameras. Earth Space Sci. 7 , e2019EA000960 (2020).
Beria, H., Larsen, J. R., Michelon, A., Ceperley, N. C. & Schaefli, B. HydroMix v1.0: a new Bayesian mixing framework for attributing uncertain hydrological sources. Geosci. Model Dev. 13 , 2433–2450 (2020).
Tang, Y., Reed, P. & Wagener, T. How effective and efficient are multiobjective evolutionary algorithms at hydrologic model calibration? Hydrol. Earth Syst. Sci. 10 , 289–307 (2006).
Iman, M., Arabnia, H. R. & Rasheed, K. A review of deep transfer learning and recent advancements. Technologies 11 , 40 (2023).
Qian, K., Jiang, J., Ding, Y. & Yang, S.-H. DLGEA: a deep learning guided evolutionary algorithm for water contamination source identification. Neural Comput. Appl. 33 , 11889–11903 (2021).
Chen, Z. et al. A transfer learning-based LSTM strategy for imputing large-scale consecutive missing data and its application in a water quality prediction system. J. Hydrol. 602 , 126573 (2021).
Xiang, Z., Yan, J. & Demir, I. A rainfall-runoff model with LSTM-based sequence-to-sequence learning. Water Resour. Res. 56 , e2019WR025326 (2020).
Nearing, G. S. et al. What role does hydrological science play in the age of machine learning? Water Resour. Res . https://doi.org/10.1029/2020WR028091 (2021).
Guo, D. et al. A data-based predictive model for spatiotemporal variability in stream water quality. Hydrol. Earth Syst. Sci. 24 , 827–847 (2020).
Zimmer, M. A. et al. Zero or not? Causes and consequences of zero-flow stream gage readings. WIREs Water 7 , e1436 (2020).
Chaudhary, P., D’Aronco, S., Moy de Vitry, M., Leitão, J. P. & Wegner, J. D. Flood-water level estimation from social media images. ISPRS Ann. Photogramm. Remote Sens. Spatial Inf. Sci . https://doi.org/10.5194/isprs-annals-IV-2-W5-5-2019 (2019).
Kanth, A. K., Chitra, P. & Sowmya, G. G. Deep learning-based assessment of flood severity using social media streams. Stoch. Environ. Res. Risk Assess. 36 , 473–493 (2022).
Hanif, M., Khawar, A., Tahir, M. A. & Rafi, M. Deep learning based framework for classification of water quality in social media data. In Proc. MediaEval 2021 Workshop ( MediaEval 2021).
Njue, N. et al. Citizen science in hydrological monitoring and ecosystem services management: state of the art and future prospects. Sci. Total Environ. 693 , 133531 (2019).
Yevenes, M. A., Pereira, H. & Bermudez, R. Citizen science as a co-creative measure to water quality: chemical data and local participation in a rural territory. Front. Environ. Sci. 10 , 940778 (2022).
Nardi, F. et al. Citizens and Hydrology (CANDHY): conceptualizing a transdisciplinary framework for citizen science addressing hydrological challenges. Hydrol. Sci. J. 67 , 2534–2551 (2022).
Dyer, F. et al. Waterwatch data quality: an opportunity to augment professionally collected data sets. In Proc. 7th Australian Stream Management Conference 27–30 (ASM, 2014).
Rose, L. A., Karwan, D. L. & Godsey, S. E. Concentration–discharge relationships describe solute and sediment mobilization, reaction, and transport at event and longer timescales. Hydrol. Processes 32 , 2829–2844 (2018).
Hare, D. K., Helton, A. M., Johnson, Z. C., Lane, J. W. & Briggs, M. A. Continental-scale analysis of shallow and deep groundwater contributions to streams. Nat. Commun. https://doi.org/10.1038/s41467-021-21651-0 (2021).
Li, L. et al. Toward catchment hydro‐biogeochemical theories. WIREs Water https://doi.org/10.1002/wat2.1495 (2021).
Zhi, W. & Li, L. The shallow and deep hypothesis: subsurface vertical chemical contrasts shape nitrate export patterns from different land uses. Environ. Sci. Technol. 54 , 11915–11928 (2020).
Zhi, W., Klingler, C., Liu, J. & Li, L. Widespread deoxygenation in warming rivers. Nat. Clim. Change 13 , 1105–1113 (2023).
Li, L. et al. Climate controls on river chemistry. Earths Future 10 , e2021EF002603 (2022).
Harari, Y. N. Sapiens: A Brief History of Humankind (Random House, 2014).
Popper, K. The Logic of Scientific Discovery (Basic Books, 1959).
Hodges, A. Alan Turing: The Enigma: The Centenary Edition (Princeton Univ. Press, 2012).
Do, H. X., Gudmundsson, L., Leonard, M. & Westra, S. The Global Streamflow Indices and Metadata Archive (GSIM)—Part 1: the production of a daily streamflow archive and metadata. Earth Syst. Sci. Data 10 , 765–785 (2018).
Virro, H., Amatulli, G., Kmoch, A., Shen, L. & Uuemaa, E. GRQA: global river water quality archive. Earth System Sci. Data 13 , 5483–5507 (2021).
Gunn, M. A., Matherne, A. M. & Mason, J. R. R. The USGS at Embudo, New Mexico: 125 Years of Systematic Streamgaging in the United States Report No. 2014-30344 (USGS, 2014).
Burt, T. P. & McDonnell, J. J. Whither field hydrology? The need for discovery science and outrageous hydrological hypotheses. Water Resour. Res. 51 , 5919–5928 (2015).
Read, E. K. et al. Water quality data for national‐scale aquatic research: the Water Quality Portal. Water Resour. Res. 53 , 1735–1745 (2017).
Council, N. R. Confronting the Nation’s Water Problems: The Role of Research (National Academies Press, 2004).
Li, Z., Liu, H., Zhang, C. & Fu, G. Generative adversarial networks for detecting contamination events in water distribution systems using multi-parameter, multi-site water quality monitoring. Environ. Sci. Ecotechnol. 14 , 100231 (2023).
Qu, H. & Yuan, W. Water quality Anomaly detection based on optimally reconfigured convolutional autoencoder. In 2022 International Conference on Wearables, Sports and Lifestyle Management (WSLM) 137–141 (IEEE, 2022).
Shen, C., Chen, X. & Laloy, E. Broadening the use of machine learning in hydrology. Front. Water https://doi.org/10.3389/frwa.2021.681023 (2021).
Schmidhuber, J. Annotated history of modern AI and deep learning. Preprint at https://arxiv.org/abs/2212.11279 (2022).
McCulloch, W. S. & Pitts, W. A logical calculus of the ideas immanent in nervous activity. Bull. Math. Biophys. 5 , 115–133 (1943).
Rosenblatt, F. The perceptron: a probabilistic model for information storage and organization in the brain. Psychol. Rev. 65 , 386 (1958).
Amari, S.-I. Learning patterns and pattern sequences by self-organizing nets of threshold elements. IEEE Trans. Comput. 100 , 1197–1206 (1972).
Maier, H. R. & Dandy, G. C. The use of artificial neural networks for the prediction of water quality parameters. Water Resour. Res. 32 , 1013–1022 (1996).
Maier, H. R. & Dandy, G. C. Neural networks for the prediction and forecasting of water resources variables: a review of modelling issues and applications. Environ. Modell. Softw. 15 , 101–124 (2000).
Chang, F. J. & Hwang, Y. Y. A self-organization algorithm for real-time flood forecast. Hydrol. Process. 13 , 123–138 (1999).
Dawson, C. W. & Wilby, R. L. A comparison of artificial neural networks used for river flow forecasting. Hydrol. Earth Syst. Sci. 3 , 529–540 (1999).
Cigizoglu, H. K. Estimation and forecasting of daily suspended sediment data by multi-layer perceptrons. Adv. Water Res. 27 , 185–195 (2004).
Dransfeld, S., Tatnall, A. R., Robinson, I. S. & Mobley, C. D. A comparison of multi-layer perceptron and multilinear regression algorithms for the inversion of synthetic ocean colour spectra. Int. J. Remote Sens. 25 , 4829–4834 (2004).
Pankiewicz, G. S. Neural network classification of convective airmasses for a flood forecasting system. Int. J. Remote Sens. 18 , 887–898 (1997).
Addor, N., Newman, A. J., Mizukami, N. & Clark, M. P. The CAMELS data set: catchment attributes and meteorology for large-sample studies. Hydrol. Earth Syst. Sci. 21 , 5293–5313 (2017).
Newman, A. et al. Development of a large-sample watershed-scale hydrometeorological data set for the contiguous USA: data set characteristics and assessment of regional variability in hydrologic model performance. Hydrol. Earth Syst. Sci. 19 , 209 (2015).
Kratzert, F. et al. Caravan—a global community dataset for large-sample hydrology. Sci. Data https://doi.org/10.1038/s41597-023-01975-w (2023).
GEMStat Database of the Global Environment Monitoring System for Freshwater (GEMS/Water) Programme (UN Environment Programme, 2018).
Hartmann, J., Lauerwald, R. & Moosdorf, N. GLORICH—global river chemistry database. Pangaea 902360 , 520 (2019).
Rotteveel, L., Heubach, F. & Sterling, S. M. The Surface Water Chemistry (SWatCh) database: a standardized global database of water chemistry to facilitate large-sample hydrological research. Earth Syst. Sci. Data 14 , 4667–4680 (2022).
Sterle, G. et al. CAMELS-Chem: augmenting CAMELS (Catchment Attributes and Meteorology for Large-sample Studies) with atmospheric and stream water chemistry data. Hydrol. Earth Syst. Sci. Discuss. 2022 , 1–23 (2022).
D’Alimonte, D. & Zibordi, G. Phytoplankton determination in an optically complex coastal region using a multilayer perceptron neural network. IEEE Trans. Geosci. Remote Sens. 41 , 2861–2868 (2003).
Download references
Acknowledgements
W.Z. was supported by the National Natural Science Foundation of China (52121006) and by the Barry and Shirley Isett Professorship (to L.L.) at Penn State University. L.L. was supported by the US National Science Foundation via the Critical Zone Collaborative Network (EAR-2012123 and EAR-2012669), Frontier Research in Earth Sciences (EAR-2121621), Signals in Soils (EAR-2034214), and US Department of Energy Environmental System Science (DE-SC0020146). J.P. was supported by Swiss Agency for Development and Cooperation (SDC) (WABES project, 7F-09963.02.01). This paper has been reviewed in accordance with the US Environmental Protection Agency’s peer and administrative review policies and approved for publication. Mention of trade names or commercial products does not constitute endorsement or recommendation for use. Any use of trade, firm, or product names is for descriptive purposes only and does not imply endorsement or recommendation for use by the US Government. Statements in this publication reflect the authors’ professional views and opinions and should not be construed to represent any determination or policy of the US Environmental Protection Agency.
Author information
Authors and affiliations.
The National Key Laboratory of Water Disaster Prevention, Yangtze Institute for Conservation and Development, Key Laboratory of Hydrologic-Cycle and Hydrodynamic-System of Ministry of Water Resources, Hohai University, Nanjing, China
Department of Civil and Environmental Engineering, The Pennsylvania State University, University Park, PA, USA
Wei Zhi & Li Li
US Geological Survey, Reston, VA, USA
Alison P. Appling
Office of Research and Development, US Environmental Protection Agency, Cincinnati, OH, USA
Heather E. Golden
Department of Water Resources and Drinking Water, Swiss Federal Institute of Aquatic Science and Technology (EAWAG), Dübendorf, Switzerland
Joel Podgorski
You can also search for this author in PubMed Google Scholar
Contributions
W.Z. and L.L. conceived the idea for the review paper and wrote the first draft. A.P.A., H.E.G. and J.P. provided content for multiple sections and edited multiple versions of the paper. L.L. finalized the paper.
Corresponding author
Correspondence to Li Li .
Ethics declarations
Competing interests.
The authors declare no competing interests.
Peer review
Peer review information.
Nature Water thanks Danlu Guo and the other, anonymous, reviewer(s) for their contribution to the peer review of this work.
Additional information
Publisher’s note Springer Nature remains neutral with regard to jurisdictional claims in published maps and institutional affiliations.
Supplementary information
Supplementary information.
Supplementary text, Figs. 1 and 2, and Table 1.
Rights and permissions
Springer Nature or its licensor (e.g. a society or other partner) holds exclusive rights to this article under a publishing agreement with the author(s) or other rightsholder(s); author self-archiving of the accepted manuscript version of this article is solely governed by the terms of such publishing agreement and applicable law.
Reprints and permissions
About this article
Cite this article.
Zhi, W., Appling, A.P., Golden, H.E. et al. Deep learning for water quality. Nat Water 2 , 228–241 (2024). https://doi.org/10.1038/s44221-024-00202-z
Download citation
Received : 13 April 2023
Accepted : 10 January 2024
Published : 12 March 2024
Issue Date : March 2024
DOI : https://doi.org/10.1038/s44221-024-00202-z
Share this article
Anyone you share the following link with will be able to read this content:
Sorry, a shareable link is not currently available for this article.
Provided by the Springer Nature SharedIt content-sharing initiative
Quick links
- Explore articles by subject
- Guide to authors
- Editorial policies
Sign up for the Nature Briefing newsletter — what matters in science, free to your inbox daily.


An official website of the United States government
Here’s how you know
Official websites use .gov A .gov website belongs to an official government organization in the United States.
Secure .gov websites use HTTPS A lock ( Lock A locked padlock ) or https:// means you’ve safely connected to the .gov website. Share sensitive information only on official, secure websites.
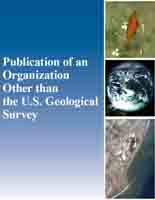
Modeling water quality in watersheds: From here to the next generation
- More information: Publisher Index Page (via DOI)
- Open Access Version: Publisher Index Page
- Download citation as: RIS | Dublin Core
In this synthesis, we assess present research and anticipate future development needs in modeling water quality in watersheds. We first discuss areas of potential improvement in the representation of freshwater systems pertaining to water quality, including representation of environmental interfaces, in‐stream water quality and process interactions, soil health and land management, and (peri‐)urban areas. In addition, we provide insights into the contemporary challenges in the practices of watershed water quality modeling, including quality control of monitoring data, model parameterization and calibration, uncertainty management, scale mismatches, and provisioning of modeling tools. Finally, we make three recommendations to provide a path forward for improving watershed water quality modeling science, infrastructure, and practices. These include building stronger collaborations between experimentalists and modelers, bridging gaps between modelers and stakeholders, and cultivating and applying procedural knowledge to better govern and support water quality modeling processes within organizations.
Publication type | Article |
---|---|
Publication Subtype | Journal Article |
Title | Modeling water quality in watersheds: From here to the next generation |
Series title | Water Resources Research |
DOI | 10.1029/2020WR027721 |
Volume | 56 |
Issue | 11 |
Year Published | 2020 |
Language | English |
Publisher | American Geophysical Union |
Contributing office(s) | Upper Midwest Water Science Center |
Description | e2020WR027721, 28 p. |
Google Analytic Metrics |
This site uses cookies to optimize functionality and give you the best possible experience. If you continue to navigate this website beyond this page, cookies will be placed on your browser. To learn more about cookies, click here .

An official website of the United States government
Here’s how you know
Official websites use .gov A .gov website belongs to an official government organization in the United States.
Secure .gov websites use HTTPS A lock ( Lock A locked padlock ) or https:// means you’ve safely connected to the .gov website. Share sensitive information only on official, secure websites.
JavaScript appears to be disabled on this computer. Please click here to see any active alerts .
Surface Water Quality Modeling
Surface water quality models are critically important tools for managing our nation's surface waters. Quantitative models help local communities and environmental managers better understand how surface waters change in response to pollution and how to protect them.
Water quality specialists use models for many purposes:
- Assessing water quality conditions and causes of impairment
- Predicting how surface waters will respond to changes in their watersheds and the environment (e.g., future growth, climate change)
- Developing Total Maximum Daily Loads (TMDLs) and National Pollutant Discharge Elimination Systems (NPDES) permits
- Forecasting quantitative benefits of new surface water protection policies
EPA's Water Modeling Workgroup (WMW) works to facilitate the use of surface water quality models through collaboration, information-sharing, and training on models and modeling resources.
- Assessment of Surface Water Model Maintenance and Support Status
- Surface Water Quality Modeling Training
- Integrated Water Analysis
- Ambient Water Quality
- Community Financing
- Drinking Water
- Water Restoration
- Water Quality Models
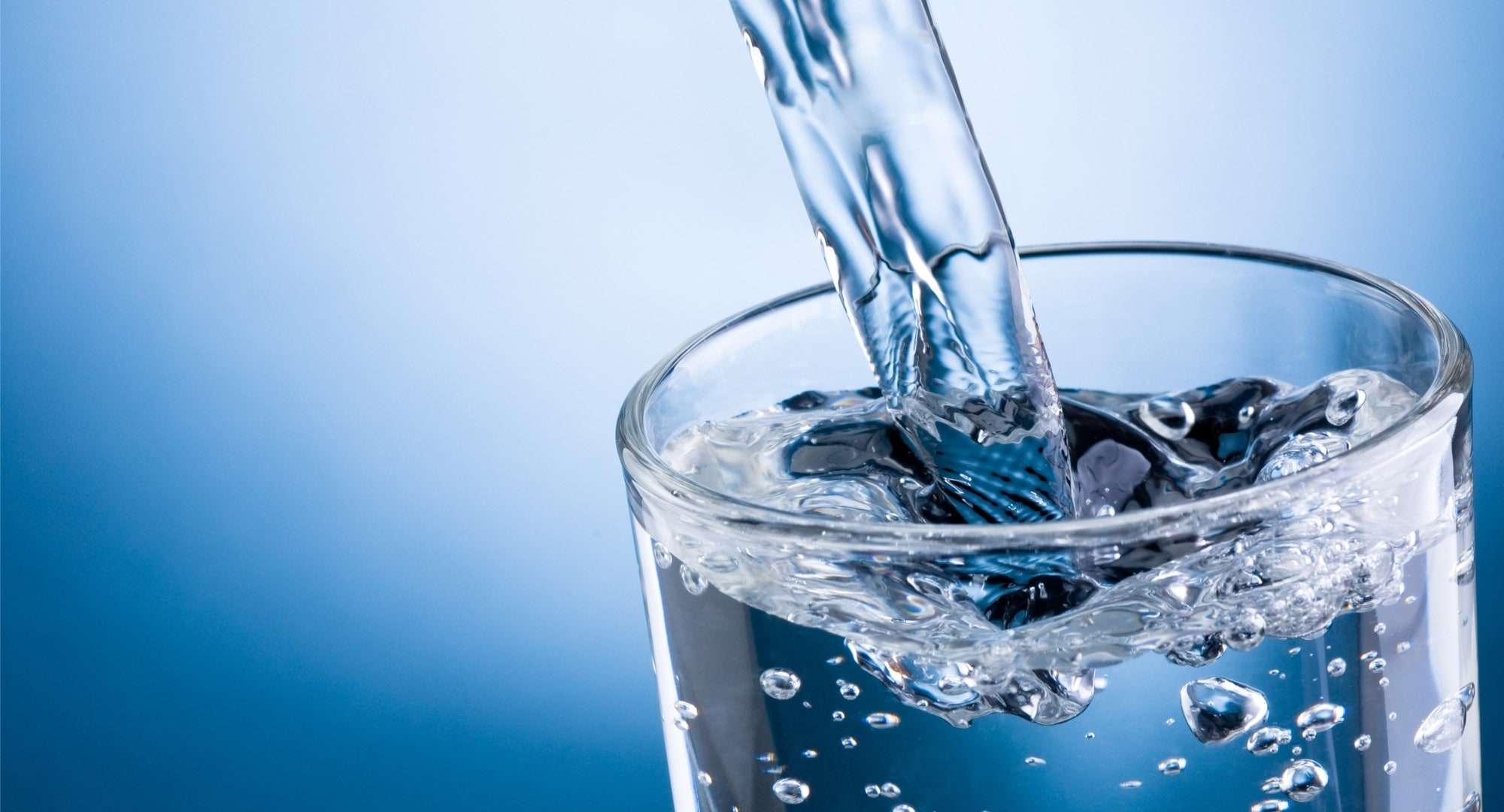
Water Quality Models: A Survey and Assessment
Related projects.
This project:
- Presents a review and summary of approximately 130 runoff, hydrodynamic, receiving water, and/or groundwater models available in either the public or private domain.
- Provides guidance on selecting the appropriate model for screening-level versus detailed planning-level applications.
- Describes the use of a Model Selection Tool, developed as part of this project. The Model Selection Tool guides the user to identify the appropriate model(s) available suitable for addressing the environmental problem being considered.
As of February 2020, the web tool is no longer available.
Originally funded as WERF project 99-WSM-5.
Roadmap Workshop on Prioritizing Permitting and Linkages Research in Water Quality
This project held a roadmap workshop to identify and foster research priorities exploring the linkages between receiving waterbody water quality and inputs such as wastewater discharges, nonpoint sources...
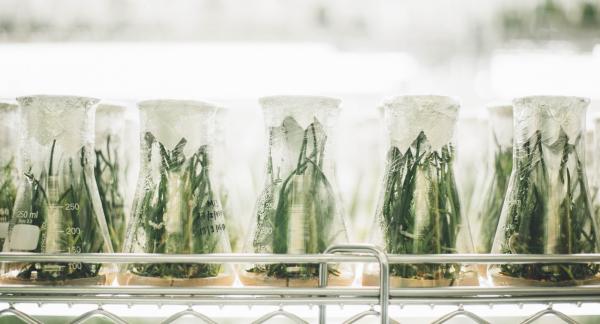
Modeling Guidance for Developing Site-Specific Nutrient Goals
This research report presents guidance and tools for the use of models to set waterbody-specific nutrient goals, including Numeric Nutrient Criteria (NNC) and allowable nutrient loadings. The developed...
Technical Approaches for Setting Site Specific Nutrient Criteria
This project developed a methodology for deriving site-specific nutrient criteria (SSNC) for surface waters, including streams and rivers, lakes and reservoirs, and coastal estuaries. The methodology was developed...
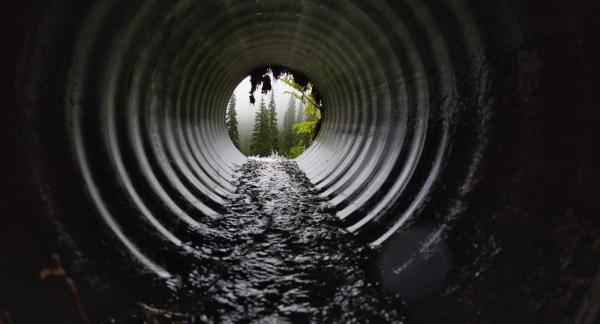
Linking Nutrient Reductions to Receiving Water Responses
While the water sector has made significant investments in nutrient control, there is a need for increased availability of data and information on effective practices, expanded engagement with...
Navigating One Water Planning through Municipal Water Programs: Meeting Multiple Objectives and Regulatory Challenges
Today’s water utilities are facing unprecedented challenges to their primary mission—providing reliable and accessible water service, protecting human health and the environment, and making wise infrastructure investments—all while...
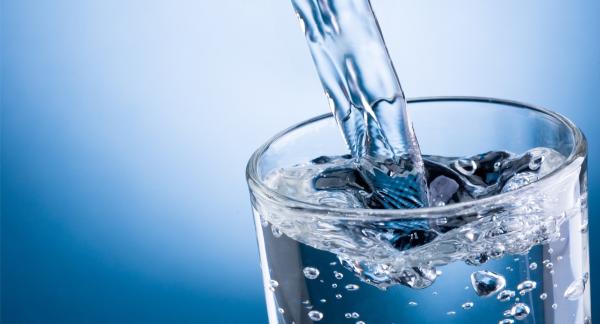

Satellite and Drone Remote Sensing Models and Tools for Water Quality Monitoring and Ecological Assessment of Fresh Water Resources
Lakes, reservoirs, and rivers are invaluable inland freshwater resources. Freshwater resources are increasingly experiencing widespread degradation and deterioration, manifested as poor water clarity and high turbidity, eutrophication, and...
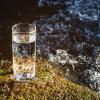
Water Quality Modeling (WQM)
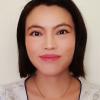
Research Scholar (WAT)
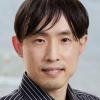
Yoshihide Wada
Principal Research Scholar (WAT)
Water quality modeling for water availability/scarcity assessment, water-energy-land-environment nexus analysis and identification of cost-effective solutions under long-term changes.
Water quality issues pose increasing risks to human health, water security and ecosystem functioning worldwide. Water quality is an important consideration in both water supply and environmental quality.
In this context, water quality modeling is added as a new component into IIASA’s Water Program to assess the long-term impacts of future changing socio-economic and climatic conditions on water quality and water resources, and to identify potential solution options.
- The nutrient export model MARINA is soft-linked to other IIASA models to explore basin-scale nexus solutions.
- A global gridded water quality model is being developed (currently for nutrients, next steps including sediment transport and salinity).
- The model is intended to be open source, modular and will be coupled with existing IIASA models, including CWATM and ECHO
Two main lines of ongoing activities:
1. Water quality modeling using the MARINA model (Strokal et al., 2016), mainly in collaboration with the Water Systems and Global Change Group, Wageningen University & Research, Netherlands.
MARINA was originally developed for China to quantify nutrient export to seas (Strokal et al., 2016) and recently up-scaled to the world for multiple pollutants in rivers from point sources. As part of on-going IIASA projects, the MARINA model is used to quantify current and future nutrient export to coastal waters for selected large river basins (e.g., Zambezi, Indus, Yangtze) under different socioeconomic development and climate change pathways. To this end, the model is soft-linked to other IIASA models ( CWATM , ECHO , GLOBIOM , EPIC , etc.) to explore basin-scale nexus solutions. The model linkages ( Figure 1 ) and some example outputs ( Figure 2 ) for the Zambezi river basin are shown below.
2. Development of a global gridded water quality model (currently for nutrients, next steps including sediment transport and salinity) The model is intended to be open source and will be coupled with CWATM and ECHO . To facilitate the coupling with these models, it is designed as a flexible modular process-based parsimonious model with a mixture of empirical or mechanistic process descriptions. This is part of our efforts to develop a next-generation global hydro-economic modeling framework that can explore the economic trade-offs among different water management options, encompassing both water infrastructure and management of water demand and water resources.
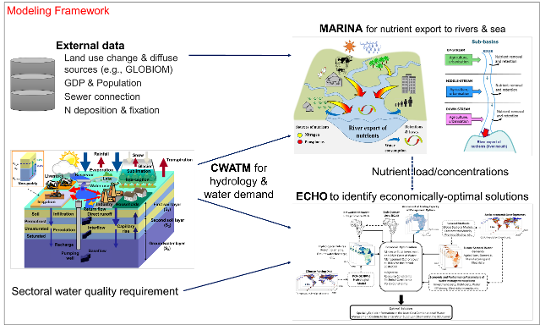
Figure 1 The MARINA model is soft-linked to CWATM and ECHO at IIASA’s Water Program to explore economically-optimal water management solutions for selected river basins.
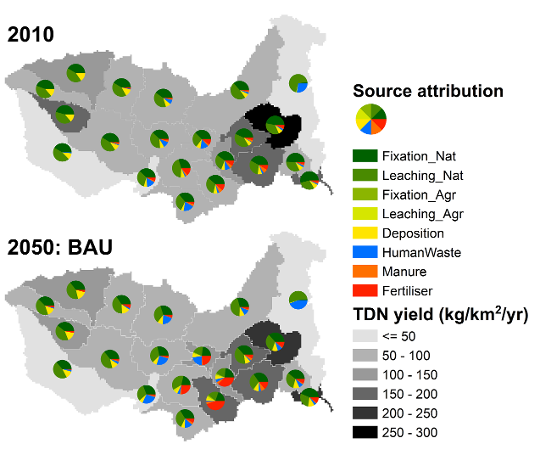
Figure 2 Illustrative example of the MARINA output for annual river export of total dissolved nitrogen by source (TDN, kg/km2/yr) for the Zambezi river basin. The figure illustrates the increase in river export of TDN to sea between 2010 and 2050.
Relevant publications:
Strokal M, Kroeze C, Wang M, Bai Z, Ma L, (2016). The MARINA model (Model to Assess River Inputs of Nutrients to seAs): Model description and results for China. Sci. Total Environ . 562, 869–888. DOI: 10.1016/j.scitotenv.2016.04.071
Tang T , Strokal M, van Vliet MTH, Seuntjens P, Burek P , Kroeze C, Langan S , & Wada Y (2019). Bridging global, basin and local-scale water quality modeling towards enhancing water quality management worldwide. Current Opinion in Environmental Sustainability 36: 39-48. DOI: 10.1016/j.cosust.2018.10.004 .
Tang T , Strokal M, Wada Y , Burek P , Kroeze C , van Vliet M , & Langan S (2018). Sources and export of nutrients in the Zambezi River basin: status and future trend. In: International Conference Water Science for Impact , 16-18 October 2018, Wageningen, Netherlands.
- Water Quality
- Water Analysis
- Water Science
- Agricultural Science
- Water Quality Modeling
Surface Water Quality Modelling
- February 2023
- Water 15(4):828

- University of Saskatchewan
Discover the world's research
- 25+ million members
- 160+ million publication pages
- 2.3+ billion citations

- Maciej Zdralewicz
- Xiaoyan Chen

- Yanming Yao
- Jinxiong Yuan

- John-Mark Davies

- Jingchen Yin
- Haitao Chen
- Puzhou Wang

- Recruit researchers
- Join for free
- Login Email Tip: Most researchers use their institutional email address as their ResearchGate login Password Forgot password? Keep me logged in Log in or Continue with Google Welcome back! Please log in. Email · Hint Tip: Most researchers use their institutional email address as their ResearchGate login Password Forgot password? Keep me logged in Log in or Continue with Google No account? Sign up
Information
- Author Services
Initiatives
You are accessing a machine-readable page. In order to be human-readable, please install an RSS reader.
All articles published by MDPI are made immediately available worldwide under an open access license. No special permission is required to reuse all or part of the article published by MDPI, including figures and tables. For articles published under an open access Creative Common CC BY license, any part of the article may be reused without permission provided that the original article is clearly cited. For more information, please refer to https://www.mdpi.com/openaccess .
Feature papers represent the most advanced research with significant potential for high impact in the field. A Feature Paper should be a substantial original Article that involves several techniques or approaches, provides an outlook for future research directions and describes possible research applications.
Feature papers are submitted upon individual invitation or recommendation by the scientific editors and must receive positive feedback from the reviewers.
Editor’s Choice articles are based on recommendations by the scientific editors of MDPI journals from around the world. Editors select a small number of articles recently published in the journal that they believe will be particularly interesting to readers, or important in the respective research area. The aim is to provide a snapshot of some of the most exciting work published in the various research areas of the journal.
Original Submission Date Received: .
- Active Journals
- Find a Journal
- Proceedings Series
- For Authors
- For Reviewers
- For Editors
- For Librarians
- For Publishers
- For Societies
- For Conference Organizers
- Open Access Policy
- Institutional Open Access Program
- Special Issues Guidelines
- Editorial Process
- Research and Publication Ethics
- Article Processing Charges
- Testimonials
- Preprints.org
- SciProfiles
- Encyclopedia
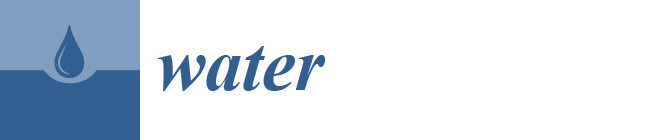
Article Menu

- Subscribe SciFeed
- Recommended Articles
- Google Scholar
- on Google Scholar
- Table of Contents
Find support for a specific problem in the support section of our website.
Please let us know what you think of our products and services.
Visit our dedicated information section to learn more about MDPI.
JSmol Viewer
Interactive changes in climatic and hydrological droughts, water quality, and land use/cover of tajan watershed, northern iran.

Share and Cite
Avand, M.; Moradi, H.R.; Hazbavi, Z. Interactive Changes in Climatic and Hydrological Droughts, Water Quality, and Land Use/Cover of Tajan Watershed, Northern Iran. Water 2024 , 16 , 1784. https://doi.org/10.3390/w16131784
Avand M, Moradi HR, Hazbavi Z. Interactive Changes in Climatic and Hydrological Droughts, Water Quality, and Land Use/Cover of Tajan Watershed, Northern Iran. Water . 2024; 16(13):1784. https://doi.org/10.3390/w16131784
Avand, Mohammadtaghi, Hamid Reza Moradi, and Zeinab Hazbavi. 2024. "Interactive Changes in Climatic and Hydrological Droughts, Water Quality, and Land Use/Cover of Tajan Watershed, Northern Iran" Water 16, no. 13: 1784. https://doi.org/10.3390/w16131784
Article Metrics
Article access statistics, further information, mdpi initiatives, follow mdpi.
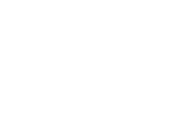
Subscribe to receive issue release notifications and newsletters from MDPI journals

An official website of the United States government
Here's how you know
Official websites use .gov A .gov website belongs to an official government organization in the United States.
Secure .gov websites use HTTPS A lock ( ) or https:// means you’ve safely connected to the .gov website. Share sensitive information only on official, secure websites.
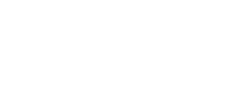
- Digg
Latest Earthquakes | Chat Share Social Media
Bibliography of water-quality studies in Gateway National Recreation Area, New York and New Jersey
The U.S. Geological Survey (USGS) provided technical assistance to the National Park Service (NPS) as part of the USGS-NPS Water-Quality Partnership, by gathering references related to water-quality research conducted in the three units of Gateway National Recreation Area (GATE): Jamaica Bay and Staten Island in New York, and Sandy Hook in New Jersey. As part of this effort, a literature search was performed to compile previous water-quality research conducted within the boundaries of GATE. The resulting bibliography is meant to assist GATE resource managers in understanding the extent of available data and developing plans to close data gaps.
Citation Information
Publication Year | 2024 |
---|---|
Title | Bibliography of water-quality studies in Gateway National Recreation Area, New York and New Jersey |
DOI | |
Authors | Philip Savoy, Maria Marionkova, Christopher Schubert |
Publication Type | Report |
Publication Subtype | USGS Numbered Series |
Series Title | Open-File Report |
Series Number | 2024-1035 |
Index ID | |
Record Source | |
USGS Organization | New York Water Science Center; WMA - Earth System Processes Division |
Related Content
Christopher e schubert, program development specialist.
Enhancing the reliability of hydrological simulations through global weather data assimilation in watersheds with limited data
- Original Paper
- Published: 25 June 2024
Cite this article
- Mahalingam Jayaprathiga 1 ,
- A. N. Rohith 2 ,
- Raj Cibin 2 , 5 &
- K. P. Sudheer 1 , 3 , 4
Hydrological models are critical for water resources planning and management. The precision and reliability of the simulations hinge greatly on the accessibility and quality of available input data. Particularly in developing nations, the major challenge in modeling is the scarcity of fine-scale spatiotemporal input data, specifically precipitation. Remotely sensed weather data has been increasingly used in recent years. However, they possess bias compared to ground observations due to the nature of indirect measurement and may affect the simulated water balance. To address these limitations, we explored data assimilation techniques to improve the Global Precipitation Measurement product (IMERG) precipitation using limited ground observations. Multiple assimilation methods are applied by incorporating Linear scaling Correction Factor (CF) and Power Transformation Function methods (PF). The assimilated IMERG precipitation from the most effective method identified, is utilized in an eco-hydrological model, and the resulting stream flow simulations are validated against observed flow data. The findings indicate that assimilated precipitation enhances the monthly flow statistics in both CF and PF methods and also in conditional merged precipitation. An ensemble of hydrological simulations, outperformed those based on raw IMERG precipitation. Additionally, the hydrological simulations are compared with observed gauge precipitation data and the widely used Climate Forecast System Reanalysis (CFSR) dataset in data-limited watersheds. The simulations utilizing the assimilated IMERG dataset (NSE = 0.52) are comparable to gauge precipitation-based simulations (NSE = 0.61) and significantly superior to CFSR-based simulations (NSE=-0.2). These results highlight the potential of utilizing assimilated remote sensing data for hydrological modeling in data-limited watersheds, leading to improved simulation accuracy and reliability.
This is a preview of subscription content, log in via an institution to check access.
Access this article
Price includes VAT (Russian Federation)
Instant access to the full article PDF.
Rent this article via DeepDyve
Institutional subscriptions

Data availability
No datasets were generated or analysed during the current study.
Adjei KA, Ren L, Appiah-Adjei EK, Odai SN (2015) Application of satellite-derived rainfall for hydrological modelling in the data-scarce black volta trans-boundary basin. Hydrol Res 46(5):777–791. https://doi.org/10.2166/nh.2014.111
Article Google Scholar
Ahmed E, Al Janabi F, Yang W, Ali A, Saddique N, Krebs P (2022) Comparison of flow simulations with sub-daily and daily GPM IMERG products over a transboundary Chenab River catchment. J Water Clim Change 13(3):1204–1224. https://doi.org/10.2166/wcc.2022.420
Alnahit AO, Mishra AK, Khan AA (2020) Evaluation of high-resolution satellite products for streamflow and water quality assessment in a Southeastern US watershed. J Hydrology: Reg Stud 27:100660. https://doi.org/10.1016/j.ejrh.2019.100660
Amorim JDS, Viola MR, Junqueira R, Oliveira VAD, Mello CRD (2020) Evaluation of satellite precipitation products for hydrological modeling in the Brazilian cerrado biome. Water 12(9):2571. https://doi.org/10.3390/w12092571
Ang R, Kinouchi T, Zhao W (2022) Evaluation of daily gridded meteorological datasets for hydrological modeling in data-sparse basins of the largest lake in Southeast Asia. J Hydrology: Reg Stud 42:101135. https://doi.org/10.1016/j.ejrh.2022.101135
Anthanahalli Nanjegowda R, Kulamulla Parambath S (2022) A novel bias correction method for extreme rainfall events based on L-moments. Int J Climatol 42(1):250–264. https://doi.org/10.1002/joc.7242
Arnold JG, Srinivasan R, Muttiah RS, Williams JR (1998) Large area hydrologic modeling and assessment part I: model development 1. JAWRA J Am Water Resour Association 34(1):73–89. https://doi.org/10.1111/j.1752-1688.1998.tb05961.x
Article CAS Google Scholar
Auerbach DA, Easton ZM, Walter MT, Flecker AS, Fuka DR (2016) Evaluating weather observations and the Climate Forecast System Reanalysis as inputs for hydrologic modelling in the tropics. Hydrol Process 30(19):3466–3477. https://doi.org/10.1002/hyp.10860
Beven K (2001) How far can we go in distributed hydrological modelling? Hydrol Earth Syst Sci 5(1):1–12. https://doi.org/10.5194/hess-5-1-2001
Bhattacharyya S, Sanyal J (2019) Impact of different types of meteorological data inputs on predicted hydrological and erosive responses to projected land use changes. J Earth Syst Sci 128:1–15. https://doi.org/10.1007/s12040-019-1076-y
Chen X, Huang G (2020) Applicability and hydrologic substitutability of TMPA satellite precipitation product in the feilaixia catchment. China Water 12(6):1803. https://doi.org/10.3390/w12061803
Cuceloglu G, Ozturk I (2019) Assessing the impact of CFSR and local climate datasets on hydrological modeling performance in the mountainous black sea catchment. Water 11(11):2277. https://doi.org/10.3390/w11112277
Daly C, Halbleib M, Smith JI, Gibson WP, Doggett MK, Taylor GH, Pasteris PP (2008) Physiographically sensitive mapping of climatological temperature and precipitation across the conterminous United States. Int J Climatology: J Royal Meteorological Soc 28(15):2031–2064. https://doi.org/10.1002/joc.1688
Dile YT, Srinivasan R (2014) Evaluation of CFSR climate data for hydrologic prediction in data-scarce watersheds: an application in the Blue Nile River Basin. JAWRA J Am Water Resour Association 50(5):1226–1241. https://doi.org/10.1111/jawr.12182
Duan Z, Tuo Y, Liu J, Gao H, Song X, Zhang Z, Mekonnen DF (2019) Hydrological evaluation of open-access precipitation and air temperature datasets using SWAT in a poorly gauged basin in Ethiopia. J Hydrol 569:612–626. https://doi.org/10.1016/j.jhydrol.2018.12.026
Ehret U (2002) Rainfall and flood nowcasting in small catchments usingweather radar. PhD thesis, University of Stuttgart
Eini MR, Javadi S, Delavar M, Monteiro JA, Darand M (2019) High accuracy of precipitation reanalyses resulted in good river discharge simulations in a semi-arid basin. Ecol Eng 131:107–119. https://doi.org/10.1016/j.ecoleng.2019.03.005
Fuka DR, Walter MT, MacAlister C, Degaetano AT, Steenhuis TS, Easton ZM (2014) Using the Climate Forecast System Reanalysis as weather input data for watershed models. Hydrol Process 28(22):5613–5623. https://doi.org/10.1002/hyp.10073
Gaona MR, Overeem A, Leijnse H, Uijlenhoet R (2016) First-year evaluation of GPM rainfall over the Netherlands: IMERG day 1 final run (V03D). J Hydrometeorol 17(11):2799–2814. https://doi.org/10.1175/JHM-D-16-0087.1
Gebregiorgis AS, Kirstetter PE, Hong YE, Gourley JJ, Huffman GJ, Petersen WA, Schwaller MR (2018) To what extent is the day 1 GPM IMERG satellite precipitation estimate improved as compared to TRMM TMPA-RT? J Geophys Research: Atmos 123(3):1694–1707. https://doi.org/10.1002/2017JD027606
Ghimire U, Akhtar T, Shrestha NK, Paul PK, Schürz C, Srinivasan R, Daggupati P (2022) A long-term global comparison of IMERG and CFSR with Surface Precipitation stations. Water Resour Manage 36(14):5695–5709. https://doi.org/10.1007/s11269-022-03328-5
Green WH, Ampt GA (1911) The flow of air and water through soils. J Agric Sci 4:1–24
Google Scholar
Hashemi H, Nordin M, Lakshmi V, Huffman GJ, Knight R (2017) Bias correction of long-term satellite monthly precipitation product (TRMM 3B43) over the conterminous United States. J Hydrometeorol 18(9):2491–2509. https://doi.org/10.1175/JHM-D-17-0025.1
Himanshu SK, Pandey A, Patil A (2018) Hydrologic evaluation of the TMPA-3B42V7 precipitation data set over an agricultural watershed using the SWAT model. J Hydrol Eng 23(4):05018003. https://doi.org/10.1061/(ASCE)HE.1943-5584.0001629
Huffman GJ, Bolvin DT, Nelkin EJ, Wolff DB, Adler RF, Gu G, Stocker EF (2007) The TRMM multisatellite precipitation analysis (TMPA): quasi-global, multiyear, combined-sensor precipitation estimates at fine scales. J Hydrometeorol 8(1):38–55. https://doi.org/10.1175/JHM560.1
Huffman GJ, Bolvin DT, Braithwaite D, Hsu K-L, Joyce RJ, Kidd C, Nelkin EJ et al (2020) Integrated multi-satellite retrievals for the global precipitation measurement (GPM) mission (IMERG). Satellite precipitation measurement: 1. 343–353. https://doi.org/10.1007/978-3-030-24568-9_19
Jajarmizadeh M, Sidek LM, Mirzai M, Alaghmand S, Harun S, Majid MR (2016) Prediction of surface flow by forcing of climate forecast system reanalysis data. Water Resour Manage 30:2627–2640. https://doi.org/10.1007/s11269-016-1303-0
Jayaprathiga M, Cibin R, Sudheer KP (2022) Reliability of Hydrology and Water Quality simulations using global scale datasets. JAWRA J Am Water Resour Association 58(3):453–470. https://doi.org/10.1111/1752-1688.13006
Jongjin B, Jongmin P, Dongryeol R, Minha C (2016) Geospatial blending to improve spatial mapping of precipitation with high spatial resolution by merging satellite-based and ground‐based data. Hydrol Process 30(16):2789–2803. https://doi.org/10.1002/hyp.10786
Kidd C, Becker A, Huffman GJ, Muller CL, Joe P, Skofronick-Jackson G, Kirschbaum DB (2017) So, how much of the Earth’s surface is covered by rain gauges? Bull Am Meteorol Soc 98(1):69–78. https://doi.org/10.1175/BAMS-D-14-00283.1
Leander R, Buishand TA (2007) Resampling of regional climate model output for the simulation of extreme river flows. J Hydrol 332(3–4):487–496. https://doi.org/10.1016/j.jhydrol.2006.08.006
Leander R, Buishand TA, van den Hurk BJ, de Wit MJ (2008) Estimated changes in flood quantiles of the river Meuse from resampling of regional climate model output. J Hydrol 351(3–4):331–343. https://doi.org/10.1016/j.jhydrol.2007.12.020
Li D, Christakos G, Ding X, Wu J (2018) Adequacy of TRMM satellite rainfall data in driving the SWAT modeling of Tiaoxi catchment (Taihu lake basin, China). J Hydrol 556:1139–1152. https://doi.org/10.1016/j.jhydrol.2017.01.006
Li X, Chen Y, Deng X, Zhang Y, Chen L (2021) Evaluation and hydrological utility of the GPM IMERG precipitation products over the Xinfengjiang River Reservoir basin, China. Remote Sens 13(5):866. https://doi.org/10.3390/rs13050866
Lu C, Ye J, Fang G, Huang X, Yan M (2021) Assessment of GPM IMERG satellite precipitation estimation under complex climatic and topographic conditions. Atmosphere 12(6):780
Mararakanye N, Le Roux JJ, Franke AC (2020) Using satellite-based weather data as input to SWAT in a data poor catchment. Phys Chem Earth Parts A/B/C 117:102871. https://doi.org/10.1016/j.pce.2020.102871
Moges DM, Kmoch A, Uuemaa E (2022) Application of satellite and reanalysis precipitation products for hydrological modeling in the data-scarce Porijõgi catchment, Estonia. J Hydrology: Reg Stud 41:101070. https://doi.org/10.1016/j.ejrh.2022.101070
Monteiro JA, Strauch M, Srinivasan R, Abbaspour K, Gücker B (2016) Accuracy of grid precipitation data for Brazil: application in river discharge modelling of the Tocantins catchment. Hydrol Process 30(9):1419–1430. https://doi.org/10.1002/hyp.10708
Mulligan M (2013) WaterWorld: a self-parameterising, physically based model for application in data-poor but problem-rich environments globally. Hydrol Res 44(5):748–769. https://doi.org/10.2166/nh.2012.217
Musie M, Sen S, Srivastava P (2019) Comparison and evaluation of gridded precipitation datasets for streamflow simulation in data scarce watersheds of Ethiopia. J Hydrol 579:124168. https://doi.org/10.1016/j.jhydrol.2019.124168
Neitsch SL, Arnold JG, Kiniry JR, Williams JR (2011) Soil and water assessment tool theoretical documentation version 2009. Texas Water Resources Institute
Palomino-Ángel S, Anaya-Acevedo JA, Botero BA (2019) Evaluation of 3B42V7 and IMERG daily-precipitation products for a very high-precipitation region in northwestern South America. Atmos Res 217:37–48. https://doi.org/10.1016/j.atmosres.2018.10.012
Pradhan RK, Markonis Y, Godoy MRV, Villalba-Pradas A, Andreadis KM, Nikolopoulos EI, Hanel M (2022) Review of GPM IMERG performance: a global perspective. Remote Sens Environ 268:112754. https://doi.org/10.1016/j.rse.2021.112754
Radcliffe DE, Mukundan R (2017) PRISM vs. CFSR precipitation data effects on calibration and validation of SWAT models. JAWRA J Am Water Resour Association 53(1):89–100. https://doi.org/10.1111/1752-1688.12484
Romagnoli M, Portapila M, Rigalli A, Maydana G, Burgués M, García CM (2017) Assessment of the SWAT model to simulate a watershed with limited available data in the Pampas region, Argentina. Sci Total Environ 596:437–450. https://doi.org/10.1016/j.scitotenv.2017.01.041
Roth V, Lemann T (2016) Comparing CFSR and conventional weather data for discharge and soil loss modelling with SWAT in small catchments in the Ethiopian Highlands. Hydrology and Earth System Sciences, 20(2), 921–934. https://doi.org/10.5194/hess-20-921-2016 , 2016
Rozante JR, Vila DA, Chiquetto B, Fernandes J, A. D. A., Alvim S, D (2018) Evaluation of TRMM/GPM blended daily products over Brazil. Remote Sens 10(6):882. https://doi.org/10.3390/rs10060882
Saha S, Moorthi S, Pan HL, Wu X, Wang J, Nadiga S, Goldberg M (2010) The NCEP climate forecast system reanalysis. Bull Am Meteorol Soc 91(8):1015–1058. https://doi.org/10.1175/2010BAMS3001.1
Setti S, Maheswaran R, Sridhar V, Barik KK, Merz B, Agarwal A (2020) Inter-comparison of gauge-based gridded data, reanalysis and satellite precipitation product with an emphasis on hydrological modeling. Atmosphere 11(11):1252. https://doi.org/10.3390/atmos11111252
Sharannya TM, Al-Ansari N, Barma D, S., Mahesha A (2020) Evaluation of satellite precipitation products in simulating streamflow in a humid tropical catchment of India using a semi-distributed hydrological model. Water 12(9):2400. https://doi.org/10.3390/w12092400
Shortridge J, Guikema S, Zaitchik B (2017) Clim Change 140:323–337. https://doi.org/10.1007/s10584-016-1845-4 . Robust decision making in data scarce contexts: addressing data and model limitations for infrastructure planning under transient climate change
Sinclair S, Pegram G (2005) Combining radar and rain gauge rainfall estimates using conditional merging. Atmospheric Sci Lett 6(1):19–22. https://doi.org/10.1002/asl.85
Singh L, Saravanan S (2020) Simulation of monthly streamflow using the SWAT model of the Ib River watershed, India. HydroResearch 3:95–105. https://doi.org/10.1016/j.hydres.2020.09.001
Singh AK, Tripathi JN, Singh KK, Singh V, Sateesh M (2019) Comparison of different satellite-derived rainfall products with IMD gridded data over Indian meteorological subdivisions during Indian summer Monsoon (ISM) 2016 at weekly temporal resolution. J Hydrol 575:1371–1379. https://doi.org/10.1016/j.jhydrol.2019.02.016
Strauch M, Kumar R, Eisner S, Mulligan M, Reinhardt J, Santini W, Friesen J (2017) Adjustment of global precipitation data for enhanced hydrologic modeling of tropical Andean watersheds. Clim Change 141:547–560. https://doi.org/10.1007/s10584-016-1706-1
Sun Q, Miao C, Duan Q, Ashouri H, Sorooshian S, Hsu KL (2018) Rev Geophys 56(1):79–107. https://doi.org/10.1002/2017RG000574 . A review of global precipitation data sets: Data sources, estimation, and intercomparisons
Swain S, Verma MK, Verma MK (2018) Streamflow estimation using SWAT model over Seonath river basin, Chhattisgarh, India. In Hydrologic Modeling: Select Proceedings of ICWEES-2016 (pp. 659–665). Springer Singapore
Tan ML, Gassman PW, Liang J, Haywood JM (2021) A review of alternative climate products for SWAT modelling: sources, assessment and future directions. Sci Total Environ 795:148915. https://doi.org/10.1016/j.scitotenv.2021.148915
Tang G, Ma Y, Long D, Zhong L, Hong Y (2016) Evaluation of GPM Day-1 IMERG and TMPA Version-7 legacy products over Mainland China at multiple spatiotemporal scales. J Hydrol 533:152–167. https://doi.org/10.1016/j.jhydrol.2015.12.008
Teutschbein C, Seibert J (2012) Bias correction of regional climate model simulations for hydrological climate-change impact studies: review and evaluation of different methods. J Hydrol 456:12–29. https://doi.org/10.1016/j.jhydrol.2012.05.052
Tobin KJ, Bennett ME (2014) Impact of model complexity and precipitation data products on modeled streamflow. J Hydroinformatics 16(3):588–599. https://doi.org/10.2166/hydro.2013.056
Tolera MB, Chung IM, Chang SW (2018) Evaluation of the climate forecast system reanalysis weather data for watershed modeling in Upper Awash basin, Ethiopia. Water 10(6):725. https://doi.org/10.3390/w10060725
Tuo Y, Chiogna G, Disse M (2015) A multi-criteria model selection protocol for practical applications to nutrient transport at the catchment scale. Water 7(6):2851–2880. https://doi.org/10.3390/w7062851
USDA-SCS (1972) National engineering handbook. USDA, Washington, DC
Valdés-Pineda R, Demaría E, Valdés JB, Wi S, Serrat-Capdevilla A (2016) Bias correction of daily satellite-based rainfall estimates for hydrologic forecasting in the Upper Zambezi, Africa. Hydrology and earth system sciences discussions. 1–28. https://doi.org/10.5194/hess-2016-473
Wang X, Ding Y, Zhao C, Wang J (2019) Similarities and improvements of GPM IMERG upon TRMM 3B42 precipitation product under complex topographic and climatic conditions over Hexi region. Northeastern Tibetan Plateau Atmospheric Res 218:347–363. https://doi.org/10.1016/j.atmosres.2018.12.011
Wang J, Liu G, Zhu C (2020) Evaluating precipitation products for hydrologic modeling over a large river basin in the Midwestern USA. Hydrol Sci J 65(7):1221–1238. https://doi.org/10.1080/02626667.2020.1737868
Yaswanth P, Kannan BAM, Bindhu VM et al (2023) Evaluation of remote sensing Rainfall products, Bias correction and temporal disaggregation approaches, for Improved Accuracy in Hydrologic simulations. Water Resour Manage 37:3069–3092. https://doi.org/10.1007/s11269-023-03486-0
Yu L, Ma L, Li H, Zhang Y, Kong F, Yang Y (2020) Assessment of high-resolution satellite rainfall products over a gradually elevating mountainous terrain based on a high-density rain gauge network. Int J Remote Sens 41(14):5620–5644. https://doi.org/10.1080/01431161.2020.1734255
Yuan F, Zhang L, Win KWW, Ren L, Zhao C, Zhu Y, Liu Y (2017) Assessment of GPM and TRMM multi-satellite precipitation products in streamflow simulations in a data-sparse mountainous watershed in Myanmar. Remote Sens 9(3):302. https://doi.org/10.3390/rs9030302
Zhang X, Anagnostou EN, Schwartz CS (2018) NWP-based adjustment of IMERG precipitation for flood-inducing complex terrain storms: evaluation over CONUS. Remote Sens 10(4):642. https://doi.org/10.3390/rs10040642
Zhang Y, Sun A, Sun H, Gui D, Xue J, Liao W, Zeng X (2019) Error adjustment of TMPA satellite precipitation estimates and assessment of their hydrological utility in the middle and upper Yangtze River Basin, China. Atmos Res 216:52–64. https://doi.org/10.1016/j.atmosres.2018.09.021
Zhu Q, Xuan W, Liu L, Xu YP (2016) Evaluation and hydrological application of precipitation estimates derived from PERSIANN-CDR, TRMM 3B42V7, and NCEP‐CFSR over humid regions in China. Hydrol Process 30(17):3061–3083. https://doi.org/10.1002/hyp.10846
Download references
Author information
Authors and affiliations.
Department of Civil Engineering, Indian Institute of Technology Madras, Chennai, India
Mahalingam Jayaprathiga & K. P. Sudheer
Department of Agricultural and Biological Engineering, The Pennsylvania State University, University Park, PA, USA
A. N. Rohith & Raj Cibin
Kerala State Council for Science, Technology, and Environment, Thiruvananthapuram, Kerala, India
K. P. Sudheer
Department of Agricultural and Biological Engineering, Purdue University, West Lafayette, IN, USA
Department of Civil and Environmental Engineering, The Pennsylvania State University, University Park, PA, USA
You can also search for this author in PubMed Google Scholar
Contributions
All authors contributed to the study conceptualization and design. Material preparation, data collection were performed by JM. Analysis was performed by JM and RAN. The first draft of the manuscript was prepared by JM. Supervision, reviewing and editing of the manuscript were performed by RAN, CR, and KPS. All authors have read and approved the final manuscript.
Corresponding author
Correspondence to K. P. Sudheer .
Ethics declarations
Competing interests.
The authors declare no competing interests.
Additional information
Publisher’s note.
Springer Nature remains neutral with regard to jurisdictional claims in published maps and institutional affiliations.
Rights and permissions
Springer Nature or its licensor (e.g. a society or other partner) holds exclusive rights to this article under a publishing agreement with the author(s) or other rightsholder(s); author self-archiving of the accepted manuscript version of this article is solely governed by the terms of such publishing agreement and applicable law.
Reprints and permissions
About this article
Jayaprathiga, M., Rohith, A., Cibin, R. et al. Enhancing the reliability of hydrological simulations through global weather data assimilation in watersheds with limited data. Stoch Environ Res Risk Assess (2024). https://doi.org/10.1007/s00477-024-02758-4
Download citation
Accepted : 11 June 2024
Published : 25 June 2024
DOI : https://doi.org/10.1007/s00477-024-02758-4
Share this article
Anyone you share the following link with will be able to read this content:
Sorry, a shareable link is not currently available for this article.
Provided by the Springer Nature SharedIt content-sharing initiative
- Data assimilation
- Find a journal
- Publish with us
- Track your research
Funding and Research Opportunities
Funding opportunities.
The South Dakota Water Resources Institute offers, in collaboration with the United States Geological Survey (USGS) several competitive research grant programs.
Grant Title | Grant Description | Application deadline | Who can apply? | # of awards annually |
---|---|---|---|---|
The 104b grants are awarded to research projects relating to water resources science and engineering. | March 15, 2024 | Faculty or affiliates from South Dakota public universities and colleges | Two to four | |
The 104g grants focuses on water problems and issues of regional or interstate nature. | May 13, 2024 | Any investigator at an institution of higher learning in the United States | Five to 10 nationwide |
Student Research Opportunities
The South Dakota Water Resources Institute frequently has student research opportunities available at both undergraduate and graduate level. The research topics typically relate to agricultural and environmental water management, including hydrology, water management, water quality and water use.
We routinely use remote sensing technologies, computer-based ecosystem or hydrological models and state-of-the-art field monitoring equipment as part of our research programs.
Please give us a call or send us an email if you are interested in working with us or are looking for research opportunities.

IMAGES
VIDEO
COMMENTS
2. Significance of water quality modeling. Water quality management is an essential component of overall integrated water resources management (UNESCO, Citation 2005).The output of the model for different pollution scenarios with water quality models is an imperative component of environmental impact assessment (Q. Wang et al., Citation 2013).Sound water quality is very limited in the world ...
In this synthesis, we assess present research and anticipate future development needs in modeling water quality in watersheds. We first discuss areas of potential improvement in the representation of freshwater systems pertaining to water quality, including representation of environmental interfaces, in-stream water quality and process interactions, soil health and land management, and (peri ...
In 1973, the Scottish Research Development Department (SRDD) developed their SRDD-WQI which was also somewhat based on Brown's model and used it for assessment of river water quality (reference). ... The model proposed 26 water quality parameter representing different groups of water quality characteristics (Abrahãoet al., 2007, Pesce and ...
Water quality monitoring and modeling are vital in improving the aquatic ecosystem's health and surroundings. The advancements in computer science and its integration with mathematics have resulted in the development of divergent algorithms and models for evaluating/predicting water quality and simulating the fate/transport of environmental contaminants. In this paper, four widely used ...
Citizen science has also become increasingly useful in hydrological and water-quality research 123 ... important for predicting water quality, DL models can potentially overcome long-standing data ...
water quality models is an imperative component of environmental impact assessment (Q. Wang et al., 2013 ). Sound water quality is very limited in the world and more care to water quality ...
Several models have been developed to help understand biogeochemical processes involving surface water for water quality and pollution assessment. In this paper, we review research studies that employed six common surface water quality models, namely, AQUATOX, CE-QUAL-W2, Delft3D, EFDC, MIKE, and WASP, that focus on the transport and fate of ...
Water quality modelling is a complex process of capturing the dynamic interactions between river, lake, and groundwater hydrology, chemistry and ecology, and trying to quantify these relationships to better achieve an understanding of the underlying science.
In this synthesis, we assess present research and anticipate future development needs in modeling water quality in watersheds. We first discuss areas of potential improvement in the representation of freshwater systems pertaining to water quality, including representation of environmental interfaces, in‐stream water quality and process interactions, soil health and land management, and (peri ...
The data indicate that research on water quality modeling in China became increasingly frequent and it had an evident influence throughout the world. 5.2. Trends in surface water quality modeling. Integrated models were developed to provide a better understanding of complex problems. Model coupling is an important development in surface water ...
Many computer models have been created and are effectively used in many countries today [1]. Review research studies from the Web of Science focused on the fate and transport of water quality ...
Books. Water Quality Modeling. This report serves as a guide to the utility and relevance of water quality prediction modeling. It draws upon examples from recent World Bank water resources and wastewater management projects. The goal of the guide is to provide a broad-based understanding of the water quality prediction process and to evaluate ...
Surface water quality modelling has become an important means of better understanding aquatic and riparian ecosystem processes at all scales, from the micro-scale (e.g., bottom sediment dynamics), to the meso-scale (e.g., algal bloom growth) and the macro-scale (e.g., the role of cascading reservoirs on sediment transport).
This report serves as a guide to the utility and relevance of water quality prediction modeling. It draws upon examples from recent World Bank water resources and . ... Data and research help us understand these challenges and set priorities, share knowledge of what works, and measure progress. Data & Research. Open Data;
Surface water quality models are critically important tools for managing our nation's surface waters. Quantitative models help local communities and environmental managers better understand how surface waters change in response to pollution and how to protect them. Water quality specialists use models for many purposes: EPA's Water Modeling ...
3. Modeling can be used to assess (predict) future water quality situations resulting from different management strategies. For example, assessing the improvement in water quality after a new wastewater treatment plant begins operating, or the effect of increased industrial growth and effluent discharges.
Water Quality Models: A Survey and Assessment. This project: Presents a review and summary of approximately 130 runoff, hydrodynamic, receiving water, and/or groundwater models available in either the public or private domain. Provides guidance on selecting the appropriate model for screening-level versus detailed planning-level applications.
Water Resources Research is an AGU hydrology journal publishing original research articles and commentaries on hydrology, water resources, and the social sciences of water. This paper reviews the role of uncertainty in the identification of mathematical models of water quality and in the application of these models to problems of prediction.
Two main lines of ongoing activities: 1. Water quality modeling using the MARINA model (Strokal et al., 2016), mainly in collaboration with the Water Systems and Global Change Group, Wageningen University & Research, Netherlands.. MARINA was originally developed for China to quantify nutrient export to seas (Strokal et al., 2016) and recently up-scaled to the world for multiple pollutants in ...
Water quality modeling involves water quality based data using mathematical simulation techniques. Water quality modeling helps people understand the eminence of water quality issues and models provide evidence for policy makers to make decisions in order to properly mitigate water. Water quality modeling also helps determine correlations to constituent sources and water quality along with ...
Research demonstrates that compared to other methods, the SVM model for evaluating water quality classification (WQC) has demonstrated higher accuracy . In conclusion, the SVM algorithm will significantly enhance and expedite information-driven decision-making in the management of the quality of water by making information more readily ...
watershed water quality modeling, including quality control of monitoring data, model parameterization. and calibration, uncertainty management, scale mismatches, and provisioning of modeling ...
Surface W ater Quality Modelling. Karl-Erich Lindenschmidt. Global Institute for Water Security, School of Environment and Sustainability, University of Saskatchewan, 11 Innovation Boulevard ...
Accurate long-term water demand forecasting is beneficial to the sustainable development and management of cities. However, the randomness and nonlinear nature of water demand bring great challenges to accurate long-term water demand forecasting. For accurate long-term water demand forecasting, the models currently in use demand the input of extensive datasets, leading to increased costs for ...
In response to novel and complex uncertainties, the present research is conducted to characterize the most significant indicators of watershed health including drought, water quality, and vegetation for the Tajan watershed, Mazandaran, Iran. The Standardized Precipitation Index (SPI) and Streamflow Drought Index (SDI) are, respectively, used to quantify the meteorological and hydrological ...
1. Introduction. Water quality monitoring is considered crucial for fish farming. Several studies have found that measuring dissolved oxygen is crucial to sidestep high values of water quality, which may result in serious harm to fish such as anoxia, hyperoxia, as well as hypoxia [].The term "water quality monitoring" refers to the process of collecting samples of water and analysing them.
Water quality modeling needs information and data to predict existing and future water quality situations in the ecosystem as a function of the baseline conditions and pollutant loads (Grimsrud et al., 1976). To model water quality, several parameters and data are required as input (Table 2).
The U.S. Geological Survey (USGS) provided technical assistance to the National Park Service (NPS) as part of the USGS-NPS Water-Quality Partnership, by gathering references related to water-quality research conducted in the three units of Gateway National Recreation Area (GATE): Jamaica Bay and Staten Island in New York, and Sandy Hook in New Jersey.
2.2 The hydrologic model: soil and water assessment tool. The Soil and Water Assessment Tool (SWAT) is a widely used semi-distributed, process-based watershed scale model developed by the Agricultural Research Service of the United States Department of Agriculture (USDA)-Agricultural Research Service (Arnold et al. 1998).SWAT facilitates the modeling of hydrological processes and water quality ...
The research topics typically relate to agricultural and environmental water management, including hydrology, water management, water quality and water use. We routinely use remote sensing technologies, computer-based ecosystem or hydrological models and state-of-the-art field monitoring equipment as part of our research programs.