
An official website of the United States government
The .gov means it’s official. Federal government websites often end in .gov or .mil. Before sharing sensitive information, make sure you’re on a federal government site.
The site is secure. The https:// ensures that you are connecting to the official website and that any information you provide is encrypted and transmitted securely.
- Publications
- Account settings
Preview improvements coming to the PMC website in October 2024. Learn More or Try it out now .
- Advanced Search
- Journal List
- HCA Healthc J Med
- v.1(2); 2020
- PMC10324782


Introduction to Research Statistical Analysis: An Overview of the Basics
Christian vandever.
1 HCA Healthcare Graduate Medical Education
Description
This article covers many statistical ideas essential to research statistical analysis. Sample size is explained through the concepts of statistical significance level and power. Variable types and definitions are included to clarify necessities for how the analysis will be interpreted. Categorical and quantitative variable types are defined, as well as response and predictor variables. Statistical tests described include t-tests, ANOVA and chi-square tests. Multiple regression is also explored for both logistic and linear regression. Finally, the most common statistics produced by these methods are explored.
Introduction
Statistical analysis is necessary for any research project seeking to make quantitative conclusions. The following is a primer for research-based statistical analysis. It is intended to be a high-level overview of appropriate statistical testing, while not diving too deep into any specific methodology. Some of the information is more applicable to retrospective projects, where analysis is performed on data that has already been collected, but most of it will be suitable to any type of research. This primer will help the reader understand research results in coordination with a statistician, not to perform the actual analysis. Analysis is commonly performed using statistical programming software such as R, SAS or SPSS. These allow for analysis to be replicated while minimizing the risk for an error. Resources are listed later for those working on analysis without a statistician.
After coming up with a hypothesis for a study, including any variables to be used, one of the first steps is to think about the patient population to apply the question. Results are only relevant to the population that the underlying data represents. Since it is impractical to include everyone with a certain condition, a subset of the population of interest should be taken. This subset should be large enough to have power, which means there is enough data to deliver significant results and accurately reflect the study’s population.
The first statistics of interest are related to significance level and power, alpha and beta. Alpha (α) is the significance level and probability of a type I error, the rejection of the null hypothesis when it is true. The null hypothesis is generally that there is no difference between the groups compared. A type I error is also known as a false positive. An example would be an analysis that finds one medication statistically better than another, when in reality there is no difference in efficacy between the two. Beta (β) is the probability of a type II error, the failure to reject the null hypothesis when it is actually false. A type II error is also known as a false negative. This occurs when the analysis finds there is no difference in two medications when in reality one works better than the other. Power is defined as 1-β and should be calculated prior to running any sort of statistical testing. Ideally, alpha should be as small as possible while power should be as large as possible. Power generally increases with a larger sample size, but so does cost and the effect of any bias in the study design. Additionally, as the sample size gets bigger, the chance for a statistically significant result goes up even though these results can be small differences that do not matter practically. Power calculators include the magnitude of the effect in order to combat the potential for exaggeration and only give significant results that have an actual impact. The calculators take inputs like the mean, effect size and desired power, and output the required minimum sample size for analysis. Effect size is calculated using statistical information on the variables of interest. If that information is not available, most tests have commonly used values for small, medium or large effect sizes.
When the desired patient population is decided, the next step is to define the variables previously chosen to be included. Variables come in different types that determine which statistical methods are appropriate and useful. One way variables can be split is into categorical and quantitative variables. ( Table 1 ) Categorical variables place patients into groups, such as gender, race and smoking status. Quantitative variables measure or count some quantity of interest. Common quantitative variables in research include age and weight. An important note is that there can often be a choice for whether to treat a variable as quantitative or categorical. For example, in a study looking at body mass index (BMI), BMI could be defined as a quantitative variable or as a categorical variable, with each patient’s BMI listed as a category (underweight, normal, overweight, and obese) rather than the discrete value. The decision whether a variable is quantitative or categorical will affect what conclusions can be made when interpreting results from statistical tests. Keep in mind that since quantitative variables are treated on a continuous scale it would be inappropriate to transform a variable like which medication was given into a quantitative variable with values 1, 2 and 3.
Categorical vs. Quantitative Variables
Both of these types of variables can also be split into response and predictor variables. ( Table 2 ) Predictor variables are explanatory, or independent, variables that help explain changes in a response variable. Conversely, response variables are outcome, or dependent, variables whose changes can be partially explained by the predictor variables.
Response vs. Predictor Variables
Choosing the correct statistical test depends on the types of variables defined and the question being answered. The appropriate test is determined by the variables being compared. Some common statistical tests include t-tests, ANOVA and chi-square tests.
T-tests compare whether there are differences in a quantitative variable between two values of a categorical variable. For example, a t-test could be useful to compare the length of stay for knee replacement surgery patients between those that took apixaban and those that took rivaroxaban. A t-test could examine whether there is a statistically significant difference in the length of stay between the two groups. The t-test will output a p-value, a number between zero and one, which represents the probability that the two groups could be as different as they are in the data, if they were actually the same. A value closer to zero suggests that the difference, in this case for length of stay, is more statistically significant than a number closer to one. Prior to collecting the data, set a significance level, the previously defined alpha. Alpha is typically set at 0.05, but is commonly reduced in order to limit the chance of a type I error, or false positive. Going back to the example above, if alpha is set at 0.05 and the analysis gives a p-value of 0.039, then a statistically significant difference in length of stay is observed between apixaban and rivaroxaban patients. If the analysis gives a p-value of 0.91, then there was no statistical evidence of a difference in length of stay between the two medications. Other statistical summaries or methods examine how big of a difference that might be. These other summaries are known as post-hoc analysis since they are performed after the original test to provide additional context to the results.
Analysis of variance, or ANOVA, tests can observe mean differences in a quantitative variable between values of a categorical variable, typically with three or more values to distinguish from a t-test. ANOVA could add patients given dabigatran to the previous population and evaluate whether the length of stay was significantly different across the three medications. If the p-value is lower than the designated significance level then the hypothesis that length of stay was the same across the three medications is rejected. Summaries and post-hoc tests also could be performed to look at the differences between length of stay and which individual medications may have observed statistically significant differences in length of stay from the other medications. A chi-square test examines the association between two categorical variables. An example would be to consider whether the rate of having a post-operative bleed is the same across patients provided with apixaban, rivaroxaban and dabigatran. A chi-square test can compute a p-value determining whether the bleeding rates were significantly different or not. Post-hoc tests could then give the bleeding rate for each medication, as well as a breakdown as to which specific medications may have a significantly different bleeding rate from each other.
A slightly more advanced way of examining a question can come through multiple regression. Regression allows more predictor variables to be analyzed and can act as a control when looking at associations between variables. Common control variables are age, sex and any comorbidities likely to affect the outcome variable that are not closely related to the other explanatory variables. Control variables can be especially important in reducing the effect of bias in a retrospective population. Since retrospective data was not built with the research question in mind, it is important to eliminate threats to the validity of the analysis. Testing that controls for confounding variables, such as regression, is often more valuable with retrospective data because it can ease these concerns. The two main types of regression are linear and logistic. Linear regression is used to predict differences in a quantitative, continuous response variable, such as length of stay. Logistic regression predicts differences in a dichotomous, categorical response variable, such as 90-day readmission. So whether the outcome variable is categorical or quantitative, regression can be appropriate. An example for each of these types could be found in two similar cases. For both examples define the predictor variables as age, gender and anticoagulant usage. In the first, use the predictor variables in a linear regression to evaluate their individual effects on length of stay, a quantitative variable. For the second, use the same predictor variables in a logistic regression to evaluate their individual effects on whether the patient had a 90-day readmission, a dichotomous categorical variable. Analysis can compute a p-value for each included predictor variable to determine whether they are significantly associated. The statistical tests in this article generate an associated test statistic which determines the probability the results could be acquired given that there is no association between the compared variables. These results often come with coefficients which can give the degree of the association and the degree to which one variable changes with another. Most tests, including all listed in this article, also have confidence intervals, which give a range for the correlation with a specified level of confidence. Even if these tests do not give statistically significant results, the results are still important. Not reporting statistically insignificant findings creates a bias in research. Ideas can be repeated enough times that eventually statistically significant results are reached, even though there is no true significance. In some cases with very large sample sizes, p-values will almost always be significant. In this case the effect size is critical as even the smallest, meaningless differences can be found to be statistically significant.
These variables and tests are just some things to keep in mind before, during and after the analysis process in order to make sure that the statistical reports are supporting the questions being answered. The patient population, types of variables and statistical tests are all important things to consider in the process of statistical analysis. Any results are only as useful as the process used to obtain them. This primer can be used as a reference to help ensure appropriate statistical analysis.
Funding Statement
This research was supported (in whole or in part) by HCA Healthcare and/or an HCA Healthcare affiliated entity.
Conflicts of Interest
The author declares he has no conflicts of interest.
Christian Vandever is an employee of HCA Healthcare Graduate Medical Education, an organization affiliated with the journal’s publisher.
This research was supported (in whole or in part) by HCA Healthcare and/or an HCA Healthcare affiliated entity. The views expressed in this publication represent those of the author(s) and do not necessarily represent the official views of HCA Healthcare or any of its affiliated entities.
Data Science: the impact of statistics
- Regular Paper
- Open access
- Published: 16 February 2018
- Volume 6 , pages 189–194, ( 2018 )
Cite this article
You have full access to this open access article
- Claus Weihs 1 &
- Katja Ickstadt 2
40k Accesses
47 Citations
17 Altmetric
Explore all metrics
In this paper, we substantiate our premise that statistics is one of the most important disciplines to provide tools and methods to find structure in and to give deeper insight into data, and the most important discipline to analyze and quantify uncertainty. We give an overview over different proposed structures of Data Science and address the impact of statistics on such steps as data acquisition and enrichment, data exploration, data analysis and modeling, validation and representation and reporting. Also, we indicate fallacies when neglecting statistical reasoning.
Similar content being viewed by others
Data Analysis
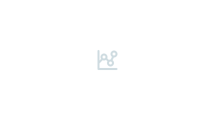
Data science vs. statistics: two cultures?
Data Science: An Introduction
Avoid common mistakes on your manuscript.
1 Introduction and premise
Data Science as a scientific discipline is influenced by informatics, computer science, mathematics, operations research, and statistics as well as the applied sciences.
In 1996, for the first time, the term Data Science was included in the title of a statistical conference (International Federation of Classification Societies (IFCS) “Data Science, classification, and related methods”) [ 37 ]. Even though the term was founded by statisticians, in the public image of Data Science, the importance of computer science and business applications is often much more stressed, in particular in the era of Big Data.
Already in the 1970s, the ideas of John Tukey [ 43 ] changed the viewpoint of statistics from a purely mathematical setting , e.g., statistical testing, to deriving hypotheses from data ( exploratory setting ), i.e., trying to understand the data before hypothesizing.
Another root of Data Science is Knowledge Discovery in Databases (KDD) [ 36 ] with its sub-topic Data Mining . KDD already brings together many different approaches to knowledge discovery, including inductive learning, (Bayesian) statistics, query optimization, expert systems, information theory, and fuzzy sets. Thus, KDD is a big building block for fostering interaction between different fields for the overall goal of identifying knowledge in data.
Nowadays, these ideas are combined in the notion of Data Science, leading to different definitions. One of the most comprehensive definitions of Data Science was recently given by Cao as the formula [ 12 ]:
data science = (statistics + informatics + computing + communication + sociology + management) | (data + environment + thinking) .
In this formula, sociology stands for the social aspects and | (data + environment + thinking) means that all the mentioned sciences act on the basis of data, the environment and the so-called data-to-knowledge-to-wisdom thinking.
A recent, comprehensive overview of Data Science provided by Donoho in 2015 [ 16 ] focuses on the evolution of Data Science from statistics. Indeed, as early as 1997, there was an even more radical view suggesting to rename statistics to Data Science [ 50 ]. And in 2015, a number of ASA leaders [ 17 ] released a statement about the role of statistics in Data Science, saying that “statistics and machine learning play a central role in data science.”
In our view, statistical methods are crucial in most fundamental steps of Data Science. Hence, the premise of our contribution is:
Statistics is one of the most important disciplines to provide tools and methods to find structure in and to give deeper insight into data, and the most important discipline to analyze and quantify uncertainty.
This paper aims at addressing the major impact of statistics on the most important steps in Data Science.
2 Steps in data science
One of forerunners of Data Science from a structural perspective is the famous CRISP-DM (Cross Industry Standard Process for Data Mining) which is organized in six main steps: Business Understanding, Data Understanding, Data Preparation, Modeling, Evaluation, and Deployment [ 10 ], see Table 1 , left column. Ideas like CRISP-DM are now fundamental for applied statistics.
In our view, the main steps in Data Science have been inspired by CRISP-DM and have evolved, leading to, e.g., our definition of Data Science as a sequence of the following steps: Data Acquisition and Enrichment, Data Storage and Access , Data Exploration, Data Analysis and Modeling, Optimization of Algorithms , Model Validation and Selection, Representation and Reporting of Results, and Business Deployment of Results . Note that topics in small capitals indicate steps where statistics is less involved, cp. Table 1 , right column.
Usually, these steps are not just conducted once but are iterated in a cyclic loop. In addition, it is common to alternate between two or more steps. This holds especially for the steps Data Acquisition and Enrichment , Data Exploration , and Statistical Data Analysis , as well as for Statistical Data Analysis and Modeling and Model Validation and Selection .
Table 1 compares different definitions of steps in Data Science. The relationship of terms is indicated by horizontal blocks. The missing step Data Acquisition and Enrichment in CRISP-DM indicates that that scheme deals with observational data only. Moreover, in our proposal, the steps Data Storage and Access and Optimization of Algorithms are added to CRISP-DM, where statistics is less involved.
The list of steps for Data Science may even be enlarged, see, e.g., Cao in [ 12 ], Figure 6, cp. also Table 1 , middle column, for the following recent list: Domain-specific Data Applications and Problems, Data Storage and Management, Data Quality Enhancement, Data Modeling and Representation, Deep Analytics, Learning and Discovery, Simulation and Experiment Design, High-performance Processing and Analytics, Networking, Communication, Data-to-Decision and Actions.
In principle, Cao’s and our proposal cover the same main steps. However, in parts, Cao’s formulation is more detailed; e.g., our step Data Analysis and Modeling corresponds to Data Modeling and Representation, Deep Analytics, Learning and Discovery . Also, the vocabularies differ slightly, depending on whether the respective background is computer science or statistics. In that respect note that Experiment Design in Cao’s definition means the design of the simulation experiments.
In what follows, we will highlight the role of statistics discussing all the steps, where it is heavily involved, in Sects. 2.1 – 2.6 . These coincide with all steps in our proposal in Table 1 except steps in small capitals. The corresponding entries Data Storage and Access and Optimization of Algorithms are mainly covered by informatics and computer science , whereas Business Deployment of Results is covered by Business Management .
2.1 Data acquisition and enrichment
Design of experiments (DOE) is essential for a systematic generation of data when the effect of noisy factors has to be identified. Controlled experiments are fundamental for robust process engineering to produce reliable products despite variation in the process variables. On the one hand, even controllable factors contain a certain amount of uncontrollable variation that affects the response. On the other hand, some factors, like environmental factors, cannot be controlled at all. Nevertheless, at least the effect of such noisy influencing factors should be controlled by, e.g., DOE.
DOE can be utilized, e.g.,
to systematically generate new data ( data acquisition ) [ 33 ],
for systematically reducing data bases [ 41 ], and
for tuning (i.e., optimizing) parameters of algorithms [ 1 ], i.e., for improving the data analysis methods (see Sect. 2.3 ) themselves.
Simulations [ 7 ] may also be used to generate new data. A tool for the enrichment of data bases to fill data gaps is the imputation of missing data [ 31 ].
Such statistical methods for data generation and enrichment need to be part of the backbone of Data Science. The exclusive use of observational data without any noise control distinctly diminishes the quality of data analysis results and may even lead to wrong result interpretation. The hope for “The End of Theory: The Data Deluge Makes the Scientific Method Obsolete” [ 4 ] appears to be wrong due to noise in the data.
Thus, experimental design is crucial for the reliability, validity, and replicability of our results.
2.2 Data exploration
Exploratory statistics is essential for data preprocessing to learn about the contents of a data base. Exploration and visualization of observed data was, in a way, initiated by John Tukey [ 43 ]. Since that time, the most laborious part of data analysis, namely data understanding and transformation, became an important part in statistical science.
Data exploration or data mining is fundamental for the proper usage of analytical methods in Data Science. The most important contribution of statistics is the notion of distribution . It allows us to represent variability in the data as well as (a-priori) knowledge of parameters, the concept underlying Bayesian statistics. Distributions also enable us to choose adequate subsequent analytic models and methods.
2.3 Statistical data analysis
Finding structure in data and making predictions are the most important steps in Data Science. Here, in particular, statistical methods are essential since they are able to handle many different analytical tasks. Important examples of statistical data analysis methods are the following.
Hypothesis testing is one of the pillars of statistical analysis. Questions arising in data driven problems can often be translated to hypotheses. Also, hypotheses are the natural links between underlying theory and statistics. Since statistical hypotheses are related to statistical tests, questions and theory can be tested for the available data. Multiple usage of the same data in different tests often leads to the necessity to correct significance levels. In applied statistics, correct multiple testing is one of the most important problems, e.g., in pharmaceutical studies [ 15 ]. Ignoring such techniques would lead to many more significant results than justified.
Classification methods are basic for finding and predicting subpopulations from data. In the so-called unsupervised case, such subpopulations are to be found from a data set without a-priori knowledge of any cases of such subpopulations. This is often called clustering.
In the so-called supervised case, classification rules should be found from a labeled data set for the prediction of unknown labels when only influential factors are available.
Nowadays, there is a plethora of methods for the unsupervised [ 22 ] as well for the supervised case [ 2 ].
In the age of Big Data, a new look at the classical methods appears to be necessary, though, since most of the time the calculation effort of complex analysis methods grows stronger than linear with the number of observations n or the number of features p . In the case of Big Data, i.e., if n or p is large, this leads to too high calculation times and to numerical problems. This results both, in the comeback of simpler optimization algorithms with low time-complexity [ 9 ] and in re-examining the traditional methods in statistics and machine learning for Big Data [ 46 ].
Regression methods are the main tool to find global and local relationships between features when the target variable is measured. Depending on the distributional assumption for the underlying data, different approaches may be applied. Under the normality assumption, linear regression is the most common method, while generalized linear regression is usually employed for other distributions from the exponential family [ 18 ]. More advanced methods comprise functional regression for functional data [ 38 ], quantile regression [ 25 ], and regression based on loss functions other than squared error loss like, e.g., Lasso regression [ 11 , 21 ]. In the context of Big Data, the challenges are similar to those for classification methods given large numbers of observations n (e.g., in data streams) and / or large numbers of features p . For the reduction of n , data reduction techniques like compressed sensing, random projection methods [ 20 ] or sampling-based procedures [ 28 ] enable faster computations. For decreasing the number p to the most influential features, variable selection or shrinkage approaches like the Lasso [ 21 ] can be employed, keeping the interpretability of the features. (Sparse) principal component analysis [ 21 ] may also be used.
Time series analysis aims at understanding and predicting temporal structure [ 42 ]. Time series are very common in studies of observational data, and prediction is the most important challenge for such data. Typical application areas are the behavioral sciences and economics as well as the natural sciences and engineering. As an example, let us have a look at signal analysis, e.g., speech or music data analysis. Here, statistical methods comprise the analysis of models in the time and frequency domains. The main aim is the prediction of future values of the time series itself or of its properties. For example, the vibrato of an audio time series might be modeled in order to realistically predict the tone in the future [ 24 ] and the fundamental frequency of a musical tone might be predicted by rules learned from elapsed time periods [ 29 ].
In econometrics, multiple time series and their co-integration are often analyzed [ 27 ]. In technical applications, process control is a common aim of time series analysis [ 34 ].
2.4 Statistical modeling
Complex interactions between factors can be modeled by graphs or networks . Here, an interaction between two factors is modeled by a connection in the graph or network [ 26 , 35 ]. The graphs can be undirected as, e.g., in Gaussian graphical models, or directed as, e.g., in Bayesian networks. The main goal in network analysis is deriving the network structure. Sometimes, it is necessary to separate (unmix) subpopulation specific network topologies [ 49 ].
Stochastic differential and difference equations can represent models from the natural and engineering sciences [ 3 , 39 ]. The finding of approximate statistical models solving such equations can lead to valuable insights for, e.g., the statistical control of such processes, e.g., in mechanical engineering [ 48 ]. Such methods can build a bridge between the applied sciences and Data Science.
Local models and globalization Typically, statistical models are only valid in sub-regions of the domain of the involved variables. Then, local models can be used [ 8 ]. The analysis of structural breaks can be basic to identify the regions for local modeling in time series [ 5 ]. Also, the analysis of concept drifts can be used to investigate model changes over time [ 30 ].
In time series, there are often hierarchies of more and more global structures. For example, in music, a basic local structure is given by the notes and more and more global ones by bars, motifs, phrases, parts etc. In order to find global properties of a time series, properties of the local models can be combined to more global characteristics [ 47 ].
Mixture models can also be used for the generalization of local to global models [ 19 , 23 ]. Model combination is essential for the characterization of real relationships since standard mathematical models are often much too simple to be valid for heterogeneous data or bigger regions of interest.
2.5 Model validation and model selection
In cases where more than one model is proposed for, e.g., prediction, statistical tests for comparing models are helpful to structure the models, e.g., concerning their predictive power [ 45 ].
Predictive power is typically assessed by means of so-called resampling methods where the distribution of power characteristics is studied by artificially varying the subpopulation used to learn the model. Characteristics of such distributions can be used for model selection [ 7 ].
Perturbation experiments offer another possibility to evaluate the performance of models. In this way, the stability of the different models against noise is assessed [ 32 , 44 ].
Meta-analysis as well as model averaging are methods to evaluate combined models [ 13 , 14 ].
Model selection became more and more important in the last years since the number of classification and regression models proposed in the literature increased with higher and higher speed.
2.6 Representation and reporting
Visualization to interpret found structures and storing of models in an easy-to-update form are very important tasks in statistical analyses to communicate the results and safeguard data analysis deployment. Deployment is decisive for obtaining interpretable results in Data Science. It is the last step in CRISP-DM [ 10 ] and underlying the data-to-decision and action step in Cao [ 12 ].
Besides visualization and adequate model storing, for statistics, the main task is reporting of uncertainties and review [ 6 ].
3 Fallacies
The statistical methods described in Sect. 2 are fundamental for finding structure in data and for obtaining deeper insight into data, and thus, for a successful data analysis. Ignoring modern statistical thinking or using simplistic data analytics/statistical methods may lead to avoidable fallacies. This holds, in particular, for the analysis of big and/or complex data.
As mentioned at the end of Sect. 2.2 , the notion of distribution is the key contribution of statistics. Not taking into account distributions in data exploration and in modeling restricts us to report values and parameter estimates without their corresponding variability. Only the notion of distributions enables us to predict with corresponding error bands.
Moreover, distributions are the key to model-based data analytics. For example, unsupervised learning can be employed to find clusters in data. If additional structure like dependency on space or time is present, it is often important to infer parameters like cluster radii and their spatio-temporal evolution. Such model-based analysis heavily depends on the notion of distributions (see [ 40 ] for an application to protein clusters).
If more than one parameter is of interest, it is advisable to compare univariate hypothesis testing approaches to multiple procedures, e.g., in multiple regression, and choose the most adequate model by variable selection. Restricting oneself to univariate testing, would ignore relationships between variables.
Deeper insight into data might require more complex models, like, e.g., mixture models for detecting heterogeneous groups in data. When ignoring the mixture, the result often represents a meaningless average, and learning the subgroups by unmixing the components might be needed. In a Bayesian framework, this is enabled by, e.g., latent allocation variables in a Dirichlet mixture model. For an application of decomposing a mixture of different networks in a heterogeneous cell population in molecular biology see [ 49 ].
A mixture model might represent mixtures of components of very unequal sizes, with small components (outliers) being of particular importance. In the context of Big Data, naïve sampling procedures are often employed for model estimation. However, these have the risk of missing small mixture components. Hence, model validation or sampling according to a more suitable distribution as well as resampling methods for predictive power are important.
4 Conclusion
Following the above assessment of the capabilities and impacts of statistics our conclusion is:
The role of statistics in Data Science is under-estimated as, e.g., compared to computer science. This yields, in particular, for the areas of data acquisition and enrichment as well as for advanced modeling needed for prediction.
Stimulated by this conclusion, statisticians are well-advised to more offensively play their role in this modern and well accepted field of Data Science.
Only complementing and/or combining mathematical methods and computational algorithms with statistical reasoning, particularly for Big Data, will lead to scientific results based on suitable approaches. Ultimately, only a balanced interplay of all sciences involved will lead to successful solutions in Data Science.
Adenso-Diaz, B., Laguna, M.: Fine-tuning of algorithms using fractional experimental designs and local search. Oper. Res. 54 (1), 99–114 (2006)
Article Google Scholar
Aggarwal, C.C. (ed.): Data Classification: Algorithms and Applications. CRC Press, Boca Raton (2014)
Google Scholar
Allen, E., Allen, L., Arciniega, A., Greenwood, P.: Construction of equivalent stochastic differential equation models. Stoch. Anal. Appl. 26 , 274–297 (2008)
Article MathSciNet Google Scholar
Anderson, C.: The End of Theory: The Data Deluge Makes the Scientific Method Obsolete. Wired Magazine https://www.wired.com/2008/06/pb-theory/ (2008)
Aue, A., Horváth, L.: Structural breaks in time series. J. Time Ser. Anal. 34 (1), 1–16 (2013)
Berger, R.E.: A scientific approach to writing for engineers and scientists. IEEE PCS Professional Engineering Communication Series IEEE Press, Wiley (2014)
Book Google Scholar
Bischl, B., Mersmann, O., Trautmann, H., Weihs, C.: Resampling methods for meta-model validation with recommendations for evolutionary computation. Evol. Comput. 20 (2), 249–275 (2012)
Bischl, B., Schiffner, J., Weihs, C.: Benchmarking local classification methods. Comput. Stat. 28 (6), 2599–2619 (2013)
Bottou, L., Curtis, F.E., Nocedal, J.: Optimization methods for large-scale machine learning. arXiv preprint arXiv:1606.04838 (2016)
Brown, M.S.: Data Mining for Dummies. Wiley, London (2014)
Bühlmann, P., Van De Geer, S.: Statistics for High-Dimensional Data: Methods, Theory and Applications. Springer, Berlin (2011)
Cao, L.: Data science: a comprehensive overview. ACM Comput. Surv. (2017). https://doi.org/10.1145/3076253
Claeskens, G., Hjort, N.L.: Model Selection and Model Averaging. Cambridge University Press, Cambridge (2008)
Cooper, H., Hedges, L.V., Valentine, J.C.: The Handbook of Research Synthesis and Meta-analysis. Russell Sage Foundation, New York City (2009)
Dmitrienko, A., Tamhane, A.C., Bretz, F.: Multiple Testing Problems in Pharmaceutical Statistics. Chapman and Hall/CRC, London (2009)
Donoho, D.: 50 Years of Data Science. http://courses.csail.mit.edu/18.337/2015/docs/50YearsDataScience.pdf (2015)
Dyk, D.V., Fuentes, M., Jordan, M.I., Newton, M., Ray, B.K., Lang, D.T., Wickham, H.: ASA Statement on the Role of Statistics in Data Science. http://magazine.amstat.org/blog/2015/10/01/asa-statement-on-the-role-of-statistics-in-data-science/ (2015)
Fahrmeir, L., Kneib, T., Lang, S., Marx, B.: Regression: Models, Methods and Applications. Springer, Berlin (2013)
Frühwirth-Schnatter, S.: Finite Mixture and Markov Switching Models. Springer, Berlin (2006)
MATH Google Scholar
Geppert, L., Ickstadt, K., Munteanu, A., Quedenfeld, J., Sohler, C.: Random projections for Bayesian regression. Stat. Comput. 27 (1), 79–101 (2017). https://doi.org/10.1007/s11222-015-9608-z
Article MathSciNet MATH Google Scholar
Hastie, T., Tibshirani, R., Wainwright, M.: Statistical Learning with Sparsity: The Lasso and Generalizations. CRC Press, Boca Raton (2015)
Hennig, C., Meila, M., Murtagh, F., Rocci, R.: Handbook of Cluster Analysis. Chapman & Hall, London (2015)
Klein, H.U., Schäfer, M., Porse, B.T., Hasemann, M.S., Ickstadt, K., Dugas, M.: Integrative analysis of histone chip-seq and transcription data using Bayesian mixture models. Bioinformatics 30 (8), 1154–1162 (2014)
Knoche, S., Ebeling, M.: The musical signal: physically and psychologically, chap 2. In: Weihs, C., Jannach, D., Vatolkin, I., Rudolph, G. (eds.) Music Data Analysis—Foundations and Applications, pp. 15–68. CRC Press, Boca Raton (2017)
Koenker, R.: Quantile Regression. Econometric Society Monographs, vol. 38 (2010)
Koller, D., Friedman, N.: Probabilistic Graphical Models: Principles and Techniques. MIT press, Cambridge (2009)
Lütkepohl, H.: New Introduction to Multiple Time Series Analysis. Springer, Berlin (2010)
Ma, P., Mahoney, M.W., Yu, B.: A statistical perspective on algorithmic leveraging. In: Proceedings of the 31th International Conference on Machine Learning, ICML 2014, Beijing, China, 21–26 June 2014, pp 91–99. http://jmlr.org/proceedings/papers/v32/ma14.html (2014)
Martin, R., Nagathil, A.: Digital filters and spectral analysis, chap 4. In: Weihs, C., Jannach, D., Vatolkin, I., Rudolph, G. (eds.) Music Data Analysis—Foundations and Applications, pp. 111–143. CRC Press, Boca Raton (2017)
Mejri, D., Limam, M., Weihs, C.: A new dynamic weighted majority control chart for data streams. Soft Comput. 22(2), 511–522. https://doi.org/10.1007/s00500-016-2351-3
Molenberghs, G., Fitzmaurice, G., Kenward, M.G., Tsiatis, A., Verbeke, G.: Handbook of Missing Data Methodology. CRC Press, Boca Raton (2014)
Molinelli, E.J., Korkut, A., Wang, W.Q., Miller, M.L., Gauthier, N.P., Jing, X., Kaushik, P., He, Q., Mills, G., Solit, D.B., Pratilas, C.A., Weigt, M., Braunstein, A., Pagnani, A., Zecchina, R., Sander, C.: Perturbation Biology: Inferring Signaling Networks in Cellular Systems. arXiv preprint arXiv:1308.5193 (2013)
Montgomery, D.C.: Design and Analysis of Experiments, 8th edn. Wiley, London (2013)
Oakland, J.: Statistical Process Control. Routledge, London (2007)
Pearl, J.: Probabilistic Reasoning in Intelligent Systems: Networks of Plausible Inference. Morgan Kaufmann, Los Altos (1988)
Chapter Google Scholar
Piateski, G., Frawley, W.: Knowledge Discovery in Databases. MIT Press, Cambridge (1991)
Press, G.: A Very Short History of Data Science. https://www.forbescom/sites/gilpress/2013/05/28/a-very-short-history-of-data-science/#5c515ed055cf (2013). [last visit: March 19, 2017]
Ramsay, J., Silverman, B.W.: Functional Data Analysis. Springer, Berlin (2005)
Särkkä, S.: Applied Stochastic Differential Equations. https://users.aalto.fi/~ssarkka/course_s2012/pdf/sde_course_booklet_2012.pdf (2012). [last visit: March 6, 2017]
Schäfer, M., Radon, Y., Klein, T., Herrmann, S., Schwender, H., Verveer, P.J., Ickstadt, K.: A Bayesian mixture model to quantify parameters of spatial clustering. Comput. Stat. Data Anal. 92 , 163–176 (2015). https://doi.org/10.1016/j.csda.2015.07.004
Schiffner, J., Weihs, C.: D-optimal plans for variable selection in data bases. Technical Report, 14/09, SFB 475 (2009)
Shumway, R.H., Stoffer, D.S.: Time Series Analysis and Its Applications: With R Examples. Springer, Berlin (2010)
Tukey, J.W.: Exploratory Data Analysis. Pearson, London (1977)
Vatcheva, I., de Jong, H., Mars, N.: Selection of perturbation experiments for model discrimination. In: Horn, W. (ed.) Proceedings of the 14th European Conference on Artificial Intelligence, ECAI-2000, IOS Press, pp 191–195 (2000)
Vatolkin, I., Weihs, C.: Evaluation, chap 13. In: Weihs, C., Jannach, D., Vatolkin, I., Rudolph, G. (eds.) Music Data Analysis—Foundations and Applications, pp. 329–363. CRC Press, Boca Raton (2017)
Weihs, C.: Big data classification — aspects on many features. In: Michaelis, S., Piatkowski, N., Stolpe, M. (eds.) Solving Large Scale Learning Tasks: Challenges and Algorithms, Springer Lecture Notes in Artificial Intelligence, vol. 9580, pp. 139–147 (2016)
Weihs, C., Ligges, U.: From local to global analysis of music time series. In: Morik, K., Siebes, A., Boulicault, J.F. (eds.) Detecting Local Patterns, Springer Lecture Notes in Artificial Intelligence, vol. 3539, pp. 233–245 (2005)
Weihs, C., Messaoud, A., Raabe, N.: Control charts based on models derived from differential equations. Qual. Reliab. Eng. Int. 26 (8), 807–816 (2010)
Wieczorek, J., Malik-Sheriff, R.S., Fermin, Y., Grecco, H.E., Zamir, E., Ickstadt, K.: Uncovering distinct protein-network topologies in heterogeneous cell populations. BMC Syst. Biol. 9 (1), 24 (2015)
Wu, J.: Statistics = data science? http://www2.isye.gatech.edu/~jeffwu/presentations/datascience.pdf (1997)
Download references
Acknowledgements
The authors would like to thank the editor, the guest editors and all reviewers for valuable comments on an earlier version of the manuscript. They also thank Leo Geppert for fruitful discussions.
Author information
Authors and affiliations.
Computational Statistics, TU Dortmund University, 44221, Dortmund, Germany
Claus Weihs
Mathematical Statistics and Biometric Applications, TU Dortmund University, 44221, Dortmund, Germany
Katja Ickstadt
You can also search for this author in PubMed Google Scholar
Corresponding author
Correspondence to Claus Weihs .
Rights and permissions
Open Access This article is distributed under the terms of the Creative Commons Attribution 4.0 International License ( http://creativecommons.org/licenses/by/4.0 /), which permits unrestricted use, distribution, and reproduction in any medium, provided you give appropriate credit to the original author(s) and the source, provide a link to the Creative Commons license, and indicate if changes were made.
Reprints and permissions
About this article
Weihs, C., Ickstadt, K. Data Science: the impact of statistics. Int J Data Sci Anal 6 , 189–194 (2018). https://doi.org/10.1007/s41060-018-0102-5
Download citation
Received : 20 March 2017
Accepted : 25 January 2018
Published : 16 February 2018
Issue Date : November 2018
DOI : https://doi.org/10.1007/s41060-018-0102-5
Share this article
Anyone you share the following link with will be able to read this content:
Sorry, a shareable link is not currently available for this article.
Provided by the Springer Nature SharedIt content-sharing initiative
- Structures of data science
- Impact of statistics on data science
- Fallacies in data science
- Find a journal
- Publish with us
- Track your research
Academia.edu no longer supports Internet Explorer.
To browse Academia.edu and the wider internet faster and more securely, please take a few seconds to upgrade your browser .
Enter the email address you signed up with and we'll email you a reset link.
- We're Hiring!
- Help Center
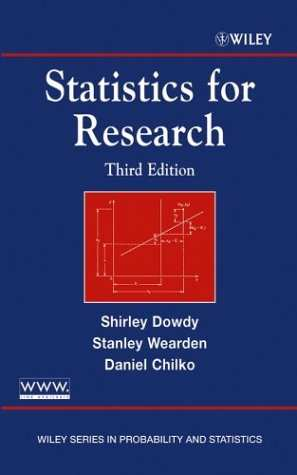
Wiley (2004) Statistics for Research (third edition)

Related Papers
Putra Mulia Siahaan
The journal
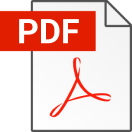
Tortuga Aire
Eric Silverman
Manfred Borovcnik
Mathematical concepts enable us to structure our thinking, corresponding models help us to structure reality. They supply us with tools to recognize and solve problems. Stochastic models are not mere images of reality that fit more or less. Right from the basics they have more the character of scenarios to explore reality. This circumstance and only indirect feedback about their success impede understanding of concepts and reasonable application of the like models. Accordingly, mis-conceptions are abundant and recipe application is ubiquitous. Stochastic thinking seems to be quite different from other types of thinking like causal thinking, or logical thinking. The educational discussion until the 90’s coined the notion of ‘probabilistic thinking’, from the 80’s the discussion shifted to the notion of ‘numeracy’ and ‘statistical thinking’. By examples and figurative deliberations a multi-faceted image of probabilistic and statistical thinking will be given.
Daniel Courgeau
This work examines in depth the methodological relationships that probability and statistics have maintained with the social sciences, particularly demography. It tells the history of their paradigms, from their emergence in the seventeenth century up to the most recent developments. The narrative runs in two directions: from probability to the social sciences, and from the latter—specifically demography—to probability. We show that, while the ties may have seemed loose at times, they have more often been very close: some advances in probability were driven by the search for answers to questions raised by the social sciences; conversely, the latter—in particular, demography—have made progress thanks to advances in probability. This dual approach sheds new light on the historical development of the social sciences and probability, and on the enduring relevance of their links.
Mathematical Structures in Computer Science
Giuseppe Longo
Under a variety of names, and in a more or less explicit form, the concept that we now call ‘probability’ must have taken shape in the mind of human beings since the dawn of thought, as a nuance added to the idea of chance (randomness) or unpredictability, though chance may not be exactly the right word. Some time later, the concepts of what we now describe as ‘statistics’ and ‘statistically stable’, moved away from the idea of ‘chance’ and came closer to something else, which was called ‘probability’ and has been fuzzily conceived as being, in some sense, abstract and ‘ideal’. Throughout history it has been felt that unpredictability can have degrees, and that it can be measured using probabilities.
Statistics Education Research Journal
Dionysia Bakogianni
Bridging the Gap: Empowering and Educating Today’s Learners in Statistics. Proceedings of the Eleventh International Conference on Teaching Statistics
Dani Ben-Zvi
University of Warwick, and the School of Education, University of Leicester. Twenty-four researchers in statistics education from seven countries shared their work, discussed important issues, and initiated collaborative projects in a stimulating and enriching environment. Sessions were held in an informal style, with a high level of interaction. With emphasis on reasoning about informal inference, a wide range of research projects were presented spanning learners of all ages, as well as teachers and practitioners in the workplace. These demonstrated an interesting diversity in research methods, theoretical approaches, and points of view. As a result of the success of this gathering, plans are already underway for the next gathering (SRTL-6) in 2009. The research forum proved to be very productive in many ways. Progress was made towards identifying the key elements of statistical inference and in locating the range of resources that might be brought to bear in supporting engagement ...
Elart Von Collani
RELATED PAPERS
A Golden Age of Science and Philosophy
Afifi al-Akiti
Devina David
JURNAL PEMBELAJARAN DAN BIOLOGI NUKLEUS
Rusdi Machrizal
Rob Hovsapian
Heike Knicker
lutfi chasanah
Clinical & medical biochemistry
Muneyuki Matsuo
2015 IEEE 12th International Symposium on Biomedical Imaging (ISBI)
Lin-ching Chang
Open Journal of Thoracic Surgery
satoshi makino
Waste Management
Dimitris Komilis
Ana Luisa Alonso Mariño
Eileen Thomas
European Journal of Endocrinology
Josef Köhrle
Gillian Jein
Journal of Radioanalytical and Nuclear Chemistry
JAn Szymanowski
Tecnologia em Metalurgia e Materiais
Dagoberto Santos
The Indonesian Green Technology Journal
soemarno S O E marno
The Journal of pharmacology and experimental therapeutics
Jamal Zweit
- We're Hiring!
- Help Center
- Find new research papers in:
- Health Sciences
- Earth Sciences
- Cognitive Science
- Mathematics
- Computer Science
- Academia ©2024
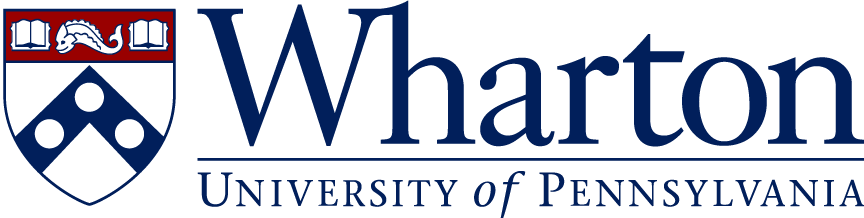
- Youth Program
- Wharton Online
Research Papers / Publications
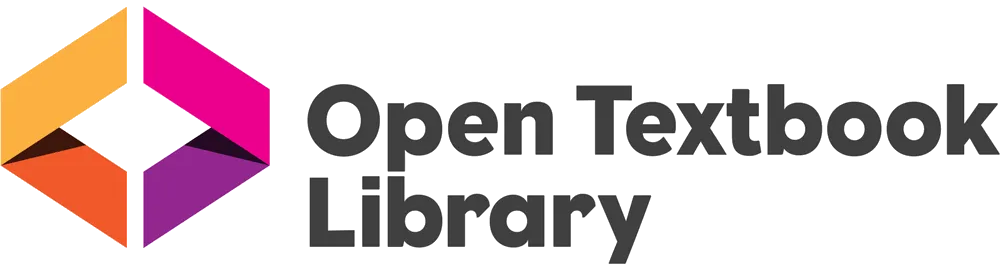
Introduction to Statistics
(15 reviews)

David Lane, Rice University
Copyright Year: 2003
Publisher: David Lane
Language: English
Formats Available
Conditions of use.

Learn more about reviews.
Reviewed by Terri Torres, professor, Oregon Institute of Technology on 8/17/23
This author covers all the topics that would be covered in an introductory statistics course plus some. I could imagine using it for two courses at my university, which is on the quarter system. I would rather have the problem of too many topics... read more
Comprehensiveness rating: 5 see less
This author covers all the topics that would be covered in an introductory statistics course plus some. I could imagine using it for two courses at my university, which is on the quarter system. I would rather have the problem of too many topics rather than too few.
Content Accuracy rating: 5
Yes, Lane is both thorough and accurate.
Relevance/Longevity rating: 5
What is covered is what is usually covered in an introductory statistics book. The only topic I may, given sufficient time, cover is bootstrapping.
Clarity rating: 5
The book is clear and well-written. For the trickier topics, simulations are included to help with understanding.
Consistency rating: 5
All is organized in a way that is consistent with the previous topic.
Modularity rating: 5
The text is organized in a way that easily enables navigation.
Organization/Structure/Flow rating: 5
The text is organized like most statistics texts.
Interface rating: 5
Easy navigation.
Grammatical Errors rating: 5
I didn't see any grammatical errors.
Cultural Relevance rating: 5
Nothing is included that is culturally insensitive.
The videos that accompany this text are short and easy to watch and understand. Videos should be short enough to teach, but not so long that they are tiresome. This text includes almost everything: videos, simulations, case studies---all nicely organized in one spot. In addition, Lane has promised to send an instructor's manual and slide deck.
Reviewed by Professor Sandberg, Professor, Framingham State University on 6/29/21
This text covers all the usual topics in an Introduction to Statistics for college students. In addition, it has some additional topics that are useful. read more
This text covers all the usual topics in an Introduction to Statistics for college students. In addition, it has some additional topics that are useful.
I did not find any errors.
Some of the examples are dated. And the frequent use of male/female examples need updating in terms of current gender splits.
I found it was easy to read and understand and I expect that students would also find the writing clear and the explanations accessible.
Even with different authors of chapter, the writing is consistent.
The text is well organized into sections making it easy to assign individual topics and sections.
The topics are presented in the usual order. Regression comes later in the text but there is a difference of opinions about whether to present it early with descriptive statistics for bivariate data or later with inferential statistics.
I had no problem navigating the text online.
The writing is grammatical correct.
I saw no issues that would be offensive.
I did like this text. It seems like it would be a good choice for most introductory statistics courses. I liked that the Monty Hall problem was included in the probability section. The author offers to provide an instructor's manual, PowerPoint slides and additional questions. These additional resources are very helpful and not always available with online OER texts.
Reviewed by Emilio Vazquez, Associate Professor, Trine University on 4/23/21
This appears to be an excellent textbook for an Introductory Course in Statistics. It covers subjects in enough depth to fulfill the needs of a beginner in Statistics work yet is not so complex as to be overwhelming. read more
This appears to be an excellent textbook for an Introductory Course in Statistics. It covers subjects in enough depth to fulfill the needs of a beginner in Statistics work yet is not so complex as to be overwhelming.
I found no errors in their discussions. Did not work out all of the questions and answers but my sampling did not reveal any errors.
Some of the examples may need updating depending on the times but the examples are still relevant at this time.
This is a Statistics text so a little dry. I found that the derivation of some of the formulas was not explained. However the background is there to allow the instructor to derive these in class if desired.
The text is consistent throughout using the same verbiage in various sections.
The text dose lend itself to reasonable reading assignments. For example the chapter (Chapter 3) on Summarizing Distributions covers Central Tendency and its associated components in an easy 20 pages with Measures of Variability making up most of the rest of the chapter and covering approximately another 20 pages. Exercises are available at the end of each chapter making it easy for the instructor to assign reading and exercises to be discussed in class.
The textbook flows easily from Descriptive to Inferential Statistics with chapters on Sampling and Estimation preceding chapters on hypothesis testing
I had no problems with navigation
All textbooks have a few errors but certainly nothing glaring or making text difficult
I saw no issues and I am part of a cultural minority in the US
Overall I found this to be a excellent in-depth overview of Statistical Theory, Concepts and Analysis. The length of the textbook appears to be more than adequate for a one-semester course in Introduction to Statistics. As I no longer teach a full statistics course but simply a few lectures as part of our Research Curriculum, I am recommending this book to my students as a good reference. Especially as it is available on-line and in Open Access.
Reviewed by Audrey Hickert, Assistant Professor, Southern Illinois University Carbondale on 3/29/21
All of the major topics of an introductory level statistics course for social science are covered. Background areas include levels of measurement and research design basics. Descriptive statistics include all major measures of central tendency and... read more
All of the major topics of an introductory level statistics course for social science are covered. Background areas include levels of measurement and research design basics. Descriptive statistics include all major measures of central tendency and dispersion/variation. Building blocks for inferential statistics include sampling distributions, the standard normal curve (z scores), and hypothesis testing sections. Inferential statistics include how to calculate confidence intervals, as well as conduct tests of one-sample tests of the population mean (Z- and t-tests), two-sample tests of the difference in population means (Z- and t-tests), chi square test of independence, correlation, and regression. Doesn’t include full probability distribution tables (e.g., t or Z), but those can be easily found online in many places.
I did not find any errors or issues of inaccuracy. When a particular method or practice is debated in the field, the authors acknowledge it (and provide citations in some circumstances).
Relevance/Longevity rating: 4
Basic statistics are standard, so the core information will remain relevant in perpetuity. Some of the examples are dated (e.g., salaries from 1999), but not problematic.
Clarity rating: 4
All of the key terms, formulas, and logic for statistical tests are clearly explained. The book sometimes uses different notation than other entry-level books. For example, the variance formula uses "M" for mean, rather than x-bar.
The explanations are consistent and build from and relate to corresponding sections that are listed in each unit.
Modularity is a strength of this text in both the PDF and interactive online format. Students can easily navigate to the necessary sections and each starts with a “Prerequisites” list of other sections in the book for those who need the additional background material. Instructors could easily compile concise sub-sections of the book for readings.
The presentation of topics differs somewhat from the standard introductory social science statistics textbooks I have used before. However, the modularity allows the instructor and student to work through the discrete sections in the desired order.
Interface rating: 4
For the most part the display of all images/charts is good and navigation is straightforward. One concern is that the organization of the Table of Contents does not exactly match the organizational outline at the start of each chapter in the PDF version. For example, sometimes there are more detailed sub-headings at the start of chapter and occasionally slightly different section headings/titles. There are also inconsistencies in section listings at start of chapters vs. start of sub-sections.
The text is easy to read and free from any obvious grammatical errors.
Although some of the examples are outdated, I did not review any that were offensive. One example of an outdated reference is using descriptive data on “Men per 100 Women” in U.S. cities as “useful if we are looking for an opposite-sex partner”.
This is a good introduction level statistics text book if you have a course with students who may be intimated by longer texts with more detailed information. Just the core basics are provided here and it is easy to select the sections you need. It is a good text if you plan to supplement with an array of your own materials (lectures, practice, etc.) that are specifically tailored to your discipline (e.g., criminal justice and criminology). Be advised that some formulas use different notation than other standard texts, so you will need to point that out to students if they differ from your lectures or assessment materials.
Reviewed by Shahar Boneh, Professor, Metropolitan State University of Denver on 3/26/21, updated 4/22/21
The textbook is indeed quite comprehensive. It can accommodate any style of introductory statistics course. read more
The textbook is indeed quite comprehensive. It can accommodate any style of introductory statistics course.
The text seems to be statistically accurate.
It is a little too extensive, which requires instructors to cover it selectively, and has a potential to confuse the students.
It is written clearly.
Consistency rating: 4
The terminology is fairly consistent. There is room for some improvement.
By the nature of the subject, the topics have to be presented in a sequential and coherent order. However, the book breaks things down quite effectively.
Organization/Structure/Flow rating: 3
Some of the topics are interleaved and not presented in the order I would like to cover them.
Good interface.
The grammar is ok.
The book seems to be culturally neutral, and not offensive in any way.
I really liked the simulations that go with the book. Parts of the book are a little too advanced for students who are learning statistics for the first time.
Reviewed by Julie Gray, Adjunct Assistant Professor, University of Texas at Arlington on 2/26/21
The textbook is for beginner-level students. The concept development is appropriate--there is always room to grow to high higher level, but for an introduction, the basics are what is needed. This is a well-thought-through OER textbook project by... read more
The textbook is for beginner-level students. The concept development is appropriate--there is always room to grow to high higher level, but for an introduction, the basics are what is needed. This is a well-thought-through OER textbook project by Dr. Lane and colleagues. It is obvious that several iterations have only made it better.
I found all the material accurate.
Essentially, statistical concepts at the introductory level are accepted as universal. This suggests that the relevance of this textbook will continue for a long time.
The book is well written for introducing beginners to statistical concepts. The figures, tables, and animated examples reinforce the clarity of the written text.
Yes, the information is consistent; when it is introduced in early chapters it ties in well in later chapters that build on and add more understanding for the topic.
Modularity rating: 4
The book is well-written with attention to modularity where possible. Due to the nature of statistics, that is not always possible. The content is presented in the order that I usually teach these concepts.
The organization of the book is good, I particularly like the sample lecture slide presentations and the problem set with solutions for use in quizzes and exams. These are available by writing to the author. It is wonderful to have access to these helpful resources for instructors to use in preparation.
I did not find any interface issues.
The book is well written. In my reading I did not notice grammatical errors.
For this subject and in the examples given, I did not notice any cultural issues.
For the field of social work where qualitative data is as common as quantitative, the importance of giving students the rationale or the motivation to learn the quantitative side is understated. To use this text as an introductory statistics OER textbook in a social work curriculum, the instructor will want to bring in field-relevant examples to engage and motivate students. The field needs data-driven decision making and evidence-based practices to become more ubiquitous than not. Preparing future social workers by teaching introductory statistics is essential to meet that goal.
Reviewed by Mamata Marme, Assistant Professor, Augustana College on 6/25/19
This textbook offers a fairly comprehensive summary of what should be discussed in an introductory course in Statistics. The statistical literacy exercises are particularly interesting. It would be helpful to have the statistical tables... read more
Comprehensiveness rating: 4 see less
This textbook offers a fairly comprehensive summary of what should be discussed in an introductory course in Statistics. The statistical literacy exercises are particularly interesting. It would be helpful to have the statistical tables attached in the same package, even though they are available online.
The terminology and notation used in the textbook is pretty standard. The content is accurate.
The statistical literacy example are up to date but will need to be updated fairly regularly to keep the textbook fresh. The applications within the chapter are accessible and can be used fairly easily over a couple of editions.
The textbook does not necessarily explain the derivation of some of the formulae and this will need to be augmented by the instructor in class discussion. What is beneficial is that there are multiple ways that a topic is discussed using graphs, calculations and explanations of the results. Statistics textbooks have to cover a wide variety of topics with a fair amount of depth. To do this concisely is difficult. There is a fine line between being concise and clear, which this textbook does well, and being somewhat dry. It may be up to the instructor to bring case studies into the readings we are going through the topics rather than wait until the end of the chapter.
The textbook uses standard notation and terminology. The heading section of each chapter is closely tied to topics that are covered. The end of chapter problems and the statistical literacy applications are closely tied to the material covered.
The authors have done a good job treating each chapter as if they stand alone. The lack of connection to a past reference may create a sense of disconnect between the topics discussed
The text's "modularity" does make the flow of the material a little disconnected. If would be better if there was accountability of what a student should already have learnt in a different section. The earlier material is easy to find but not consistently referred to in the text.
I had no problem with the interface. The online version is more visually interesting than the pdf version.
I did not see any grammatical errors.
Cultural Relevance rating: 4
I am not sure how to evaluate this. The examples are mostly based on the American experience and the data alluded to mostly domestic. However, I am not sure if that creates a problem in understanding the methodology.
Overall, this textbook will cover most of the topics in a survey of statistics course.
Reviewed by Alexandra Verkhovtseva, Professor, Anoka-Ramsey Community College on 6/3/19
This is a comprehensive enough text, considering that it is not easy to create a comprehensive statistics textbook. It is suitable for an introductory statistics course for non-math majors. It contains twenty-one chapters, covering the wide range... read more
This is a comprehensive enough text, considering that it is not easy to create a comprehensive statistics textbook. It is suitable for an introductory statistics course for non-math majors. It contains twenty-one chapters, covering the wide range of intro stats topics (and some more), plus the case studies and the glossary.
The content is pretty accurate, I did not find any biases or errors.
The book contains fairly recent data presented in the form of exercises, examples and applications. The topics are up-to-date, and appropriate technology is used for examples, applications, and case studies.
The language is simple and clear, which is a good thing, since students are usually scared of this class, and instructors are looking for something to put them at ease. I would, however, try to make it a little more interesting, exciting, or may be even funny.
Consistency is good, the book has a great structure. I like how each chapter has prerequisites and learner outcomes, this gives students a good idea of what to expect. Material in this book is covered in good detail.
The text can be easily divided into sub-sections, some of which can be omitted if needed. The chapter on regression is covered towards the end (chapter 14), but part of it can be covered sooner in the course.
The book contains well organized chapters that makes reading through easy and understandable. The order of chapters and sections is clear and logical.
The online version has many functions and is easy to navigate. This book also comes with a PDF version. There is no distortion of images or charts. The text is clean and clear, the examples provided contain appropriate format of data presentation.
No grammatical errors found.
The text uses simple and clear language, which is helpful for non-native speakers. I would include more culturally-relevant examples and case studies. Overall, good text.
In all, this book is a good learning experience. It contains tools and techniques that free and easy to use and also easy to modify for both, students and instructors. I very much appreciate this opportunity to use this textbook at no cost for our students.
Reviewed by Dabrina Dutcher, Assistant Professor, Bucknell University on 3/4/19
This is a reasonably thorough first-semester statistics book for most classes. It would have worked well for the general statistics courses I have taught in the past but is not as suitable for specialized introductory statistics courses for... read more
This is a reasonably thorough first-semester statistics book for most classes. It would have worked well for the general statistics courses I have taught in the past but is not as suitable for specialized introductory statistics courses for engineers or business applications. That is OK, they have separate texts for that! The only sections that feel somewhat light in terms of content are the confidence intervals and ANOVA sections. Given that these topics are often sort of crammed in at the end of many introductory classes, that might not be problematic for many instructors. It should also be pointed out that while there are a couple of chapters on probability, this book spends presents most formulas as "black boxes" rather than worry about the derivation or origin of the formulas. The probability sections do not include any significant combinatorics work, which is sometimes included at this level.
I did not find any errors in the formulas presented but I did not work many end-of-chapter problems to gauge the accuracy of their answers.
There isn't much changing in the introductory stats world, so I have no concerns about the book becoming outdated rapidly. The examples and problems still feel relevant and reasonably modern. My only concern is that the statistical tool most often referenced in the book are TI-83/84 type calculators. As students increasingly buy TI-89s or Inspires, these sections of the book may lose relevance faster than other parts.
Solid. The book gives a list of key terms and their definitions at the end of each chapter which is a nice feature. It also has a formula review at the end of each chapter. I can imagine that these are heavily used by students when studying! Formulas are easy to find and read and are well defined. There are a few areas that I might have found frustrating as a student. For example, the explanation for the difference in formulas for a population vs sample standard deviation is quite weak. Again, this is a book that focuses on sort of a "black-box" approach but you may have to supplement such sections for some students.
I did not detect any problems with inconsistent symbol use or switches in terminology.
Modularity rating: 3
This low rating should not be taken as an indicator of an issue with this book but would be true of virtually any statistics book. Different books still use different variable symbols even for basic calculated statistics. So trying to use a chapter of this book without some sort of symbol/variable cheat-sheet would likely be frustrating to the students.
However, I think it would be possible to skip some chapters or use the chapters in a different order without any loss of functionality.
This book uses a very standard order for the material. The chapter on regressions comes later than it does in some texts but it doesn't really matter since that chapter never seems to fit smoothly anywhere.
There are numerous end of chapter problems, some with answers, available in this book. I'm vacillating on whether these problems would be more useful if they were distributed after each relevant section or are better clumped at the end of the whole chapter. That might be a matter of individual preference.
I did not detect any problems.
I found no errors. However, there were several sections where the punctuation seemed non-ideal. This did not affect the over-all useability of the book though
I'm not sure how well this book would work internationally as many of the examples contain domestic (American) references. However, I did not see anything offensive or biased in the book.
Reviewed by Ilgin Sager, Assistant Professor, University of Missouri - St. Louis on 1/14/19
As the title implies, this is a brief introduction textbook. It covers the fundamental of the introductory statistics, however not a comprehensive text on the subject. A teacher can use this book as the sole text of an introductory statistics.... read more
As the title implies, this is a brief introduction textbook. It covers the fundamental of the introductory statistics, however not a comprehensive text on the subject. A teacher can use this book as the sole text of an introductory statistics. The prose format of definitions and theorems make theoretical concepts accessible to non-math major students. The textbook covers all chapters required in this level course.
It is accurate; the subject matter in the examples to be up to date, is timeless and wouldn't need to be revised in future editions; there is no error except a few typographical errors. There are no logic errors or incorrect explanations.
This text will remain up to date for a long time since it has timeless examples and exercises, it wouldn't be outdated. The information is presented clearly with a simple way and the exercises are beneficial to follow the information.
The material is presented in a clear, concise manner. The text is easy readable for the first time statistics student.
The structure of the text is very consistent. Topics are presented with examples, followed by exercises. Problem sets are appropriate for the level of learner.
When the earlier matters need to be referenced, it is easy to find; no trouble reading the book and finding results, it has a consistent scheme. This book is set very well in sections.
The text presents the information in a logical order.
The learner can easily follow up the material; there is no interface problem.
There is no logic errors and incorrect explanations, a few typographical errors is just to be ignored.
Not applicable for this textbook.
Reviewed by Suhwon Lee, Associate Teaching Professor, University of Missouri on 6/19/18
This book is pretty comprehensive for being a brief introductory book. This book covers all necessary content areas for an introduction to Statistics course for non-math majors. The text book provides an effective index, plenty of exercises,... read more
This book is pretty comprehensive for being a brief introductory book. This book covers all necessary content areas for an introduction to Statistics course for non-math majors. The text book provides an effective index, plenty of exercises, review questions, and practice tests. It provides references and case studies. The glossary and index section is very helpful for students and can be used as a great resource.
Content appears to be accurate throughout. Being an introductory book, the book is unbiased and straight to the point. The terminology is standard.
The content in textbook is up to date. It will be very easy to update it or make changes at any point in time because of the well-structured contents in the textbook.
The author does a great job of explaining nearly every new term or concept. The book is easy to follow, clear and concise. The graphics are good to follow. The language in the book is easily understandable. I found most instructions in the book to be very detailed and clear for students to follow.
Overall consistency is good. It is consistent in terms of terminology and framework. The writing is straightforward and standardized throughout the text and it makes reading easier.
The authors do a great job of partitioning the text and labeling sections with appropriate headings. The table of contents is well organized and easily divisible into reading sections and it can be assigned at different points within the course.
Organization/Structure/Flow rating: 4
Overall, the topics are arranged in an order that follows natural progression in a statistics course with some exception. They are addressed logically and given adequate coverage.
The text is free of any issues. There are no navigation problems nor any display issues.
The text contains no grammatical errors.
The text is not culturally insensitive or offensive in any way most of time. Some examples might need to consider citing the sources or use differently to reflect current inclusive teaching strategies.
Overall, it's well-written and good recourse to be an introduction to statistical methods. Some materials may not need to be covered in an one-semester course. Various examples and quizzes can be a great recourse for instructor.
Reviewed by Jenna Kowalski, Mathematics Instructor, Anoka-Ramsey Community College on 3/27/18
The text includes the introductory statistics topics covered in a college-level semester course. An effective index and glossary are included, with functional hyperlinks. read more
The text includes the introductory statistics topics covered in a college-level semester course. An effective index and glossary are included, with functional hyperlinks.
Content Accuracy rating: 3
The content of this text is accurate and error-free, based on a random sampling of various pages throughout the text. Several examples included information without formal citation, leading the reader to potential bias and discrimination. These examples should be corrected to reflect current values of inclusive teaching.
The text contains relevant information that is current and will not become outdated in the near future. The statistical formulas and calculations have been used for centuries. The examples are direct applications of the formulas and accurately assess the conceptual knowledge of the reader.
The text is very clear and direct with the language used. The jargon does require a basic mathematical and/or statistical foundation to interpret, but this foundational requirement should be met with course prerequisites and placement testing. Graphs, tables, and visual displays are clearly labeled.
The terminology and framework of the text is consistent. The hyperlinks are working effectively, and the glossary is valuable. Each chapter contains modules that begin with prerequisite information and upcoming learning objectives for mastery.
The modules are clearly defined and can be used in conjunction with other modules, or individually to exemplify a choice topic. With the prerequisite information stated, the reader understands what prior mathematical understanding is required to successfully use the module.
The topics are presented well, but I recommend placing Sampling Distributions, Advanced Graphs, and Research Design ahead of Probability in the text. I think this rearranged version of the index would better align with current Introductory Statistics texts. The structure is very organized with the prerequisite information stated and upcoming learner outcomes highlighted. Each module is well-defined.
Adding an option of returning to the previous page would be of great value to the reader. While progressing through the text systematically, this is not an issue, but when the reader chooses to skip modules and read select pages then returning to the previous state of information is not easily accessible.
No grammatical errors were found while reviewing select pages of this text at random.
Cultural Relevance rating: 3
Several examples contained data that were not formally cited. These examples need to be corrected to reflect current inclusive teaching strategies. For example, one question stated that “while men are XX times more likely to commit murder than women, …” This data should be cited, otherwise the information can be interpreted as biased and offensive.
An included solutions manual for the exercises would be valuable to educators who choose to use this text.
Reviewed by Zaki Kuruppalil, Associate Professor, Ohio University on 2/1/18
This is a comprehensive book on statistical methods, its settings and most importantly the interpretation of the results. With the advent of computers and software’s, complex statistical analysis can be done very easily. But the challenge is the... read more
This is a comprehensive book on statistical methods, its settings and most importantly the interpretation of the results. With the advent of computers and software’s, complex statistical analysis can be done very easily. But the challenge is the knowledge of how to set the case, setting parameters (for example confidence intervals) and knowing its implication on the interpretation of the results. If not done properly this could lead to deceptive inferences, inadvertently or purposely. This book does a great job in explaining the above using many examples and real world case studies. If you are looking for a book to learn and apply statistical methods, this is a great one. I think the author could consider revising the title of the book to reflect the above, as it is more than just an introduction to statistics, may be include the word such as practical guide.
The contents of the book seems accurate. Some plots and calculations were randomly selected and checked for accuracy.
The book topics are up to date and in my opinion, will not be obsolete in the near future. I think the smartest thing the author has done is, not tied the book with any particular software such as minitab or spss . No matter what the software is, standard deviation is calculated the same way as it is always. The only noticeable exception in this case was using the Java Applet for calculating Z values in page 261 and in page 416 an excerpt of SPSS analysis is provided for ANOVA calculations.
The contents and examples cited are clear and explained in simple language. Data analysis and presentation of the results including mathematical calculations, graphical explanation using charts, tables, figures etc are presented with clarity.
Terminology is consistant. Framework for each chapter seems consistent with each chapter beginning with a set of defined topics, and each of the topic divided into modules with each module having a set of learning objectives and prerequisite chapters.
The text book is divided into chapters with each chapter further divided into modules. Each of the modules have detailed learning objectives and prerequisite required. So you can extract a portion of the book and use it as a standalone to teach certain topics or as a learning guide to apply a relevant topic.
Presentation of the topics are well thought and are presented in a logical fashion as if it would be introduced to someone who is learning the contents. However, there are some issues with table of contents and page numbers, for example chapter 17 starts in page 597 not 598. Also some tables and figures does not have a number, for instance the graph shown in page 114 does not have a number. Also it would have been better if the chapter number was included in table and figure identification, for example Figure 4-5 . Also in some cases, for instance page 109, the figures and titles are in two different pages.
No major issues. Only suggestion would be, since each chapter has several modules, any means such as a header to trace back where you are currently, would certainly help.
Grammatical Errors rating: 4
Easy to read and phrased correctly in most cases. Minor grammatical errors such as missing prepositions etc. In some cases the author seems to have the habbit of using a period after the decimal. For instance page 464, 467 etc. For X = 1, Y' = (0.425)(1) + 0.785 = 1.21. For X = 2, Y' = (0.425)(2) + 0.785 = 1.64.
However it contains some statements (even though given as examples) that could be perceived as subjective, which the author could consider citing the sources. For example from page 11: Statistics include numerical facts and figures. For instance: • The largest earthquake measured 9.2 on the Richter scale. • Men are at least 10 times more likely than women to commit murder. • One in every 8 South Africans is HIV positive. • By the year 2020, there will be 15 people aged 65 and over for every new baby born.
Solutions for the exercises would be a great teaching resource to have
Reviewed by Randy Vander Wal, Professor, The Pennsylvania State University on 2/1/18
As a text for an introductory course, standard topics are covered. It was nice to see some topics such as power, sampling, research design and distribution free methods covered, as these are often omitted in abbreviated texts. Each module... read more
As a text for an introductory course, standard topics are covered. It was nice to see some topics such as power, sampling, research design and distribution free methods covered, as these are often omitted in abbreviated texts. Each module introduces the topic, has appropriate graphics, illustration or worked example(s) as appropriate and concluding with many exercises. An instructor’s manual is available by contacting the author. A comprehensive glossary provides definitions for all the major terms and concepts. The case studies give examples of practical applications of statistical analyses. Many of the case studies contain the actual raw data. To note is that the on-line e-book provides several calculators for the essential distributions and tests. These are provided in lieu of printed tables which are not included in the pdf. (Such tables are readily available on the web.)
The content is accurate and error free. Notation is standard and terminology is used accurately, as are the videos and verbal explanations therein. Online links work properly as do all the calculators. The text appears neutral and unbiased in subject and content.
The text achieves contemporary relevance by ending each section with a Statistical Literacy example, drawn from contemporary headlines and issues. Of course, the core topics are time proven. There is no obvious material that may become “dated”.
The text is very readable. While the pdf text may appear “sparse” by absence varied colored and inset boxes, pictures etc., the essential illustrations and descriptions are provided. Meanwhile for this same content the on-line version appears streamlined, uncluttered, enhancing the value of the active links. Moreover, the videos provide nice short segments of “active” instruction that are clear and concise. Despite being a mathematical text, the text is not overly burdened by formulas and numbers but rather has “readable feel”.
This terminology and symbol use are consistent throughout the text and with common use in the field. The pdf text and online version are also consistent by content, but with the online e-book offering much greater functionality.
The chapters and topics may be used in a selective manner. Certain chapters have no pre-requisite chapter and in all cases, those required are listed at the beginning of each module. It would be straightforward to select portions of the text and reorganize as needed. The online version is highly modular offering students both ease of navigation and selection of topics.
Chapter topics are arranged appropriately. In an introductory statistics course, there is a logical flow given the buildup to the normal distribution, concept of sampling distributions, confidence intervals, hypothesis testing, regression and additional parametric and non-parametric tests. The normal distribution is central to an introductory course. Necessary precursor topics are covered in this text, while its use in significance and hypothesis testing follow, and thereafter more advanced topics, including multi-factor ANOVA.
Each chapter is structured with several modules, each beginning with pre-requisite chapter(s), learning objectives and concluding with Statistical Literacy sections providing a self-check question addressing the core concept, along with answer, followed by an extensive problem set. The clear and concise learning objectives will be of benefit to students and the course instructor. No solutions or answer key is provided to students. An instructor’s manual is available by request.
The on-line interface works well. In fact, I was pleasantly surprised by its options and functionality. The pdf appears somewhat sparse by comparison to publisher texts, lacking pictures, colored boxes, etc. But the on-line version has many active links providing definitions and graphic illustrations for key terms and topics. This can really facilitate learning as making such “refreshers” integral to the new material. Most sections also have short videos that are professionally done, with narration and smooth graphics. In this way, the text is interactive and flexible, offering varied tools for students. To note is that the interactive e-book works for both IOS and OS X.
The text in pdf form appeared to free of grammatical errors, as did the on-line version, text, graphics and videos.
This text contains no culturally insensitive or offensive content. The focus of the text is on concepts and explanation.
The text would be a great resource for students. The full content would be ambitious for a 1-semester course, such use would be unlikely. The text is clearly geared towards students with no statistics background nor calculus. The text could be used in two styles of course. For 1st year students early chapters on graphs and distributions would be the starting point, omitting later chapters on Chi-square, transformations, distribution-free and size effect chapters. Alternatively, for upper level students the introductory chapters could be bypassed with the latter chapters then covered to completion.
This text adopts a descriptive style of presentation with topics well and fully explained, much like the “Dummy series”. For this, it may seem a bit “wordy”, but this can well serve students and notably it complements powerpoint slides that are generally sparse on written content. This text could be used as the primary text, for regular lectures, or as reference for a “flipped” class. The e-book videos are an enabling tool if this approach is adopted.
Reviewed by David jabon, Associate Professor, DePaul University on 8/15/17
This text covers all the standard topics in a semester long introductory course in statistics. It is particularly well indexed and very easy to navigate. There is comprehensive hyperlinked glossary. read more
This text covers all the standard topics in a semester long introductory course in statistics. It is particularly well indexed and very easy to navigate. There is comprehensive hyperlinked glossary.
The material is completely accurate. There are no errors. The terminology is standard with one exception: the book calls what most people call the interquartile range, the H-spread in a number of places. Ideally, the term "interquartile range" would be used in place of every reference to "H-spread." "Interquartile range" is simply a better, more descriptive term of the concept that it describes. It is also more commonly used nowadays.
This book came out a number of years ago, but the material is still up to date. Some more recent case studies have been added.
The writing is very clear. There are also videos for almost every section. The section on boxplots uses a lot of technical terms that I don't find are very helpful for my students (hinge, H-spread, upper adjacent value).
The text is internally consistent with one exception that I noted (the use of the synonymous words "H-spread" and "interquartile range").
The text book is brokenly into very short sections, almost to a fault. Each section is at most two pages long. However at the end of each of these sections there are a few multiple choice questions to test yourself. These questions are a very appealing feature of the text.
The organization, in particular the ordering of the topics, is rather standard with a few exceptions. Boxplots are introduced in Chapter II before the discussion of measures of center and dispersion. Most books introduce them as part of discussion of summaries of data using measure of center and dispersion. Some statistics instructors may not like the way the text lumps all of the sampling distributions in a single chapter (sampling distribution of mean, sampling distribution for the difference of means, sampling distribution of a proportion, sampling distribution of r). I have tried this approach, and I now like this approach. But it is a very challenging chapter for students.
The book's interface has no features that distracted me. Overall the text is very clean and spare, with no additional distracting visual elements.
The book contains no grammatical errors.
The book's cultural relevance comes out in the case studies. As of this writing there are 33 such case studies, and they cover a wide range of issues from health to racial, ethnic, and gender disparity.
Each chapter as a nice set of exercises with selected answers. The thirty three case studies are excellent and can be supplement with some other online case studies. An instructor's manual and PowerPoint slides can be obtained by emailing the author. There are direct links to online simulations within the text. This text is very high quality textbook in every way.
Table of Contents
- 1. Introduction
- 2. Graphing Distributions
- 3. Summarizing Distributions
- 4. Describing Bivariate Data
- 5. Probability
- 6. Research Design
- 7. Normal Distributions
- 8. Advanced Graphs
- 9. Sampling Distributions
- 10. Estimation
- 11. Logic of Hypothesis Testing
- 12. Testing Means
- 14. Regression
- 15. Analysis of Variance
- 16. Transformations
- 17. Chi Square
- 18. Distribution-Free Tests
- 19. Effect Size
- 20. Case Studies
- 21. Glossary
Ancillary Material
- Ancillary materials are available by contacting the author or publisher .
About the Book
Introduction to Statistics is a resource for learning and teaching introductory statistics. This work is in the public domain. Therefore, it can be copied and reproduced without limitation. However, we would appreciate a citation where possible. Please cite as: Online Statistics Education: A Multimedia Course of Study (http://onlinestatbook.com/). Project Leader: David M. Lane, Rice University. Instructor's manual, PowerPoint Slides, and additional questions are available.
About the Contributors
David Lane is an Associate Professor in the Departments of Psychology, Statistics, and Management at the Rice University. Lane is the principal developer of this resource although many others have made substantial contributions. This site was developed at Rice University, University of Houston-Clear Lake, and Tufts University.
Contribute to this Page

Innovative Statistics Project Ideas for Insightful Analysis

Table of contents
- 1.1 AP Statistics Topics for Project
- 1.2 Statistics Project Topics for High School Students
- 1.3 Statistical Survey Topics
- 1.4 Statistical Experiment Ideas
- 1.5 Easy Stats Project Ideas
- 1.6 Business Ideas for Statistics Project
- 1.7 Socio-Economic Easy Statistics Project Ideas
- 1.8 Experiment Ideas for Statistics and Analysis
- 2 Conclusion: Navigating the World of Data Through Statistics
Diving into the world of data, statistics presents a unique blend of challenges and opportunities to uncover patterns, test hypotheses, and make informed decisions. It is a fascinating field that offers many opportunities for exploration and discovery. This article is designed to inspire students, educators, and statistics enthusiasts with various project ideas. We will cover:
- Challenging concepts suitable for advanced placement courses.
- Accessible ideas that are engaging and educational for younger students.
- Ideas for conducting surveys and analyzing the results.
- Topics that explore the application of statistics in business and socio-economic areas.
Each category of topics for the statistics project provides unique insights into the world of statistics, offering opportunities for learning and application. Let’s dive into these ideas and explore the exciting world of statistical analysis.
Top Statistics Project Ideas for High School
Statistics is not only about numbers and data; it’s a unique lens for interpreting the world. Ideal for students, educators, or anyone with a curiosity about statistical analysis, these project ideas offer an interactive, hands-on approach to learning. These projects range from fundamental concepts suitable for beginners to more intricate studies for advanced learners. They are designed to ignite interest in statistics by demonstrating its real-world applications, making it accessible and enjoyable for people of all skill levels.
Need help with statistics project? Get your paper written by a professional writer Get Help Reviews.io 4.9/5
AP Statistics Topics for Project
- Analyzing Variance in Climate Data Over Decades.
- The Correlation Between Economic Indicators and Standard of Living.
- Statistical Analysis of Voter Behavior Patterns.
- Probability Models in Sports: Predicting Outcomes.
- The Effectiveness of Different Teaching Methods: A Statistical Study.
- Analysis of Demographic Data in Public Health.
- Time Series Analysis of Stock Market Trends.
- Investigating the Impact of Social Media on Academic Performance.
- Survival Analysis in Clinical Trial Data.
- Regression Analysis on Housing Prices and Market Factors.
Statistics Project Topics for High School Students
- The Mathematics of Personal Finance: Budgeting and Spending Habits.
- Analysis of Class Performance: Test Scores and Study Habits.
- A Statistical Comparison of Local Public Transportation Options.
- Survey on Dietary Habits and Physical Health Among Teenagers.
- Analyzing the Popularity of Various Music Genres in School.
- The Impact of Sleep on Academic Performance: A Statistical Approach.
- Statistical Study on the Use of Technology in Education.
- Comparing Athletic Performance Across Different Sports.
- Trends in Social Media Usage Among High School Students.
- The Effect of Part-Time Jobs on Student Academic Achievement.
Statistical Survey Topics
- Public Opinion on Environmental Conservation Efforts.
- Consumer Preferences in the Fast Food Industry.
- Attitudes Towards Online Learning vs. Traditional Classroom Learning.
- Survey on Workplace Satisfaction and Productivity.
- Public Health: Attitudes Towards Vaccination.
- Trends in Mobile Phone Usage and Preferences.
- Community Response to Local Government Policies.
- Consumer Behavior in Online vs. Offline Shopping.
- Perceptions of Public Safety and Law Enforcement.
- Social Media Influence on Political Opinions.
Statistical Experiment Ideas
- The Effect of Light on Plant Growth.
- Memory Retention: Visual vs. Auditory Information.
- Caffeine Consumption and Cognitive Performance.
- The Impact of Exercise on Stress Levels.
- Testing the Efficacy of Natural vs. Chemical Fertilizers.
- The Influence of Color on Mood and Perception.
- Sleep Patterns: Analyzing Factors Affecting Sleep Quality.
- The Effectiveness of Different Types of Water Filters.
- Analyzing the Impact of Room Temperature on Concentration.
- Testing the Strength of Different Brands of Batteries.
Easy Stats Project Ideas
- Average Daily Screen Time Among Students.
- Analyzing the Most Common Birth Months.
- Favorite School Subjects Among Peers.
- Average Time Spent on Homework Weekly.
- Frequency of Public Transport Usage.
- Comparison of Pet Ownership in the Community.
- Favorite Types of Movies or TV Shows.
- Daily Water Consumption Habits.
- Common Breakfast Choices and Their Nutritional Value.
- Steps Count: A Week-Long Study.
Business Ideas for Statistics Project
- Analyzing Customer Satisfaction in Retail Stores.
- Market Analysis of a New Product Launch.
- Employee Performance Metrics and Organizational Success.
- Sales Data Analysis for E-commerce Websites.
- Impact of Advertising on Consumer Buying Behavior.
- Analysis of Supply Chain Efficiency.
- Customer Loyalty and Retention Strategies.
- Trend Analysis in Social Media Marketing.
- Financial Risk Assessment in Investment Decisions.
- Market Segmentation and Targeting Strategies.
Socio-Economic Easy Statistics Project Ideas
- Income Inequality and Its Impact on Education.
- The Correlation Between Unemployment Rates and Crime Levels.
- Analyzing the Effects of Minimum Wage Changes.
- The Relationship Between Public Health Expenditure and Population Health.
- Demographic Analysis of Housing Affordability.
- The Impact of Immigration on Local Economies.
- Analysis of Gender Pay Gap in Different Industries.
- Statistical Study of Homelessness Causes and Solutions.
- Education Levels and Their Impact on Job Opportunities.
- Analyzing Trends in Government Social Spending.
Experiment Ideas for Statistics and Analysis
- Multivariate Analysis of Global Climate Change Data.
- Time-Series Analysis in Predicting Economic Recessions.
- Logistic Regression in Medical Outcome Prediction.
- Machine Learning Applications in Statistical Modeling.
- Network Analysis in Social Media Data.
- Bayesian Analysis of Scientific Research Data.
- The Use of Factor Analysis in Psychology Studies.
- Spatial Data Analysis in Geographic Information Systems (GIS).
- Predictive Analysis in Customer Relationship Management (CRM).
- Cluster Analysis in Market Research.
Conclusion: Navigating the World of Data Through Statistics
In this exploration of good statistics project ideas, we’ve ventured through various topics, from the straightforward to the complex, from personal finance to global climate change. These ideas are gateways to understanding the world of data and statistics, and platforms for cultivating critical thinking and analytical skills. Whether you’re a high school student, a college student, or a professional, engaging in these projects can deepen your appreciation of how statistics shapes our understanding of the world around us. These projects encourage exploration, inquiry, and a deeper engagement with the world of numbers, trends, and patterns – the essence of statistics.
Readers also enjoyed
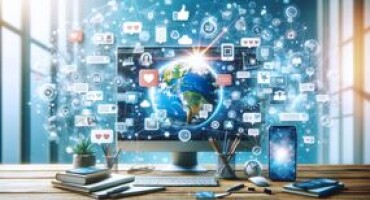
WHY WAIT? PLACE AN ORDER RIGHT NOW!
Just fill out the form, press the button, and have no worries!
We use cookies to give you the best experience possible. By continuing we’ll assume you board with our cookie policy.
Thank you for visiting nature.com. You are using a browser version with limited support for CSS. To obtain the best experience, we recommend you use a more up to date browser (or turn off compatibility mode in Internet Explorer). In the meantime, to ensure continued support, we are displaying the site without styles and JavaScript.
- View all journals
- My Account Login
- Explore content
- About the journal
- Publish with us
- Sign up for alerts
- Open access
- Published: 16 May 2024
The Egyptian pyramid chain was built along the now abandoned Ahramat Nile Branch
- Eman Ghoneim ORCID: orcid.org/0000-0003-3988-0335 1 ,
- Timothy J. Ralph ORCID: orcid.org/0000-0002-4956-606X 2 ,
- Suzanne Onstine 3 ,
- Raghda El-Behaedi 4 ,
- Gad El-Qady 5 ,
- Amr S. Fahil 6 ,
- Mahfooz Hafez 5 ,
- Magdy Atya 5 ,
- Mohamed Ebrahim ORCID: orcid.org/0000-0002-4068-5628 5 ,
- Ashraf Khozym 5 &
- Mohamed S. Fathy 6
Communications Earth & Environment volume 5 , Article number: 233 ( 2024 ) Cite this article
61k Accesses
1725 Altmetric
Metrics details
- Archaeology
- Geomorphology
- Hydrogeology
- Sedimentology
The largest pyramid field in Egypt is clustered along a narrow desert strip, yet no convincing explanation as to why these pyramids are concentrated in this specific locality has been given so far. Here we use radar satellite imagery, in conjunction with geophysical data and deep soil coring, to investigate the subsurface structure and sedimentology in the Nile Valley next to these pyramids. We identify segments of a major extinct Nile branch, which we name The Ahramat Branch, running at the foothills of the Western Desert Plateau, where the majority of the pyramids lie. Many of the pyramids, dating to the Old and Middle Kingdoms, have causeways that lead to the branch and terminate with Valley Temples which may have acted as river harbors along it in the past. We suggest that The Ahramat Branch played a role in the monuments’ construction and that it was simultaneously active and used as a transportation waterway for workmen and building materials to the pyramids’ sites.
Similar content being viewed by others
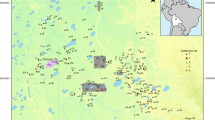
Lidar reveals pre-Hispanic low-density urbanism in the Bolivian Amazon
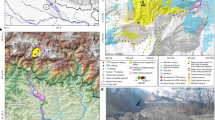
Medieval demise of a Himalayan giant summit induced by mega-landslide
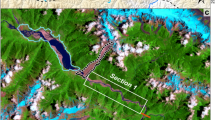
Quantitative assessment of the erosion and deposition effects of landslide-dam outburst flood, Eastern Himalaya
Introduction.
The landscape of the northern Nile Valley in Egypt, between Lisht in the south and the Giza Plateau in the north, was subject to a number of environmental and hydrological changes during the past few millennia 1 , 2 . In the Early Holocene (~12,000 years before present), the Sahara of North Africa transformed from a hyper-arid desert to a savannah-like environment, with large river systems and lake basins 3 , 4 due to an increase in global sea level at the end of the Last Glacial Maximum (LGM). The wet conditions of the Sahara provided a suitable habitat for people and wildlife, unlike in the Nile Valley, which was virtually inhospitable to humans because of the constantly higher river levels and swampy environment 5 . At this time, Nile River discharge was high, which is evident from the extensive deposition of organic-rich fluvial sediment in the Eastern Mediterranean basin 6 . Based on the interpretation of archeological material and pollen records, this period, known as the African Humid Period (AHP) (ca. 14,500–5000 years ago), was the most significant and persistent wet period from the early to mid-Holocene in the eastern Sahara region 7 , with an annual rainfall rate of 300–920 mm yr −1 8 . During this time the Nile would have had several secondary channels branching across the floodplain, similar to those described by early historians (e.g., Herodotus).
During the mid-Holocene (~10,000–6000 years ago), freshwater marshes were common within the Nile floodplain causing habitation to be more nucleated along the desert margins of the Nile Valley 9 . The desert margins provided a haven from the high Nile water. With the ending of the AHP and the beginning of the Late Holocene (~5500 years ago to present), rainfall greatly declined, and the region’s humid phase gradually came to an end with punctuated short wet episodes 10 . Due to increased aridity in the Sahara, more people moved out of the desert towards the Nile Valley and settled along the edge of the Nile floodplain. With the reduced precipitation, sedimentation increased in and around the Nile River channels causing the proximal floodplain to rise in height and adjacent marshland to decrease in the area 11 , 12 estimated the Nile flood levels to have ranged from 1 to 4 m above the baseline (~5000 BP). Inhabitants moved downhill to the Nile Valley and settled in the elevated areas on the floodplain, including the raised natural levees of the river and jeziras (islands). This was the beginning of the Old Kingdom Period (ca. 2686 BCE) and the time when early pyramid complexes, including the Step Pyramid of Djoser, were constructed at the margins of the floodplain. During this time the Nile discharge was still considerably higher than its present level. The high flow of the river, particularly during the short-wet intervals, enabled the Nile to maintain multiple branches, which meandered through its floodplain. Although the landscape of the Nile floodplain has greatly transformed due to river regulation associated with the construction of the Aswan High Dam in the 1960s, this region still retains some clear hydro-geomorphological traces of the abandoned river channels.
Since the beginning of the Pharaonic era, the Nile River has played a fundamental role in the rapid growth and expansion of the Egyptian civilization. Serving as their lifeline in a largely arid landscape, the Nile provided sustenance and functioned as the main water corridor that allowed for the transportation of goods and building materials. For this reason, most of the key cities and monuments were in close proximity to the banks of the Nile and its peripheral branches. Over time, however, the main course of the Nile River laterally migrated, and its peripheral branches silted up, leaving behind many ancient Egyptian sites distant from the present-day river course 9 , 13 , 14 , 15 . Yet, it is still unclear as to where exactly the ancient Nile courses were situated 16 , and whether different reaches of the Nile had single or multiple branches that were simultaneously active in the past. Given the lack of consensus amongst scholars regarding this subject, it is imperative to develop a comprehensive understanding of the Nile during the time of the ancient Egyptian civilization. Such a poor understanding of Nile River morphodynamics, particularly in the region that hosts the largest pyramid fields of Egypt, from Lisht to Giza, limits our understanding of how changes in the landscape influenced human activities and settlement patterns in this region, and significantly restricts our ability to understand the daily lives and stories of the ancient Egyptians.
Currently, much of the original surface of the ancient Nile floodplain is masked by either anthropogenic activity or broad silt and sand sheets. For this reason, singular approaches such as on-ground searches for the remains of hidden former Nile branches are both increasingly difficult and inauspicious. A number of studies have already been carried out in Egypt to locate segments of the ancient Nile course. For instance 9 , proposed that the axis of the Nile River ran far west of its modern course past ancient cities such as el-Ashmunein (Hermopolis) 13 . mapped the ancient hydrological landscape in the Luxor area and estimated both an eastward and westward Nile migration rate of 2–3 km per 1000 years. In the Nile Delta region 17 , detected several segments of buried Nile distributaries and elevated mounds using geoelectrical resistivity surveys. Similarly, a study by Bunbury and Lutley 14 identified a segment of an ancient Nile channel, about 5000 years old, near the ancient town of Memphis ( men-nefer ). More recently 15 , used cores taken around Memphis to reveal a section of a lateral ancient Nile branch that was dated to the Neolithic and Predynastic times (ca. 7000–5000 BCE). On the bank of this branch, Memphis, the first capital of unified Egypt, was founded in early Pharaonic times. Over the Dynastic period, this lateral branch then significantly migrated eastwards 15 . A study by Toonen et al. 18 , using borehole data and electrical resistivity tomography, further revealed a segment of an ancient Nile branch, dating to the New Kingdom Period, situated near the desert edge west of Luxor. This river branch would have connected important localities and thus played a significant role in the cultural landscape of this area. More recent research conducted further north by Sheisha et al. 2 , near the Giza Plateau, indicated the presence of a former river and marsh-like environment in the floodplain east of the three great Pyramids of Giza.
Even though the largest concentration of pyramids in Egypt are located along a narrow desert strip from south Lisht to Giza, no explanation has been offered as to why these pyramid fields were condensed in this particular area. Monumental structures, such as pyramids and temples, would logically be built near major waterways to facilitate the transportation of their construction materials and workers. Yet, no waterway has been found near the largest pyramid field in Egypt, with the Nile River lying several kilometers away. Even though many efforts to reconstruct the ancient Nile waterways have been conducted, they have largely been confined to small sites, which has led to the mapping of only fragmented sections of the ancient Nile channel systems.
In this work, we present remote sensing, geomorphological, soil coring and geophysical evidence to support the existence of a long-lost ancient river branch, the Ahramat Branch, and provide the first map of the paleohydrological setting in the Lisht-Giza area. The finding of the Ahramat Branch is not only crucial to our understanding of why the pyramids were built in these specific geographical areas, but also for understanding how the pyramids were accessed and constructed by the ancient population. It has been speculated by many scholars that the ancient Egyptians used the Nile River for help transporting construction materials to pyramid building sites, but until now, this ancient Nile branch was not fully uncovered or mapped. This work can help us better understand the former hydrological setting of this region, which would in turn help us learn more about the environmental parameters that may have influenced the decision to build these pyramids in their current locations during the time of Pharaonic Egypt.
Position and morphology of the Ahramat Branch
Synthetic Aperture Radar (SAR) imagery and radar high-resolution elevation data for the Nile floodplain and its desert margins, between south Lisht and the Giza Plateau area, provide evidence for the existence of segments of a major ancient river branch bordering 31 pyramids dating from the Old Kingdom to Second Intermediate Period (2686−1649 BCE) and spanning between Dynasties 3–13 (Fig. 1a ). This extinct branch is referred to hereafter as the Ahramat Branch, meaning the “Pyramids Branch” in Arabic. Although masked by the cultivated fields of the Nile floodplain, subtle topographic expressions of this former branch, now invisible in optical satellite data, can be traced on the ground surface by TanDEM-X (TDX) radar data and the Topographic Position Index (TPI). Data analysis indicates that this lateral distributary channel lies between 2.5 and 10.25 km west from the modern Nile River. The branch appears to have a surface channel depth between 2 and 8 m, a channel length of about 64 km and a channel width of 200–700 m, which is similar to the width of the contemporary neighboring Nile course. The size and longitudinal continuity of the Ahramat Branch and its proximity to all the pyramids in the study area implies a functional waterway of great significance.
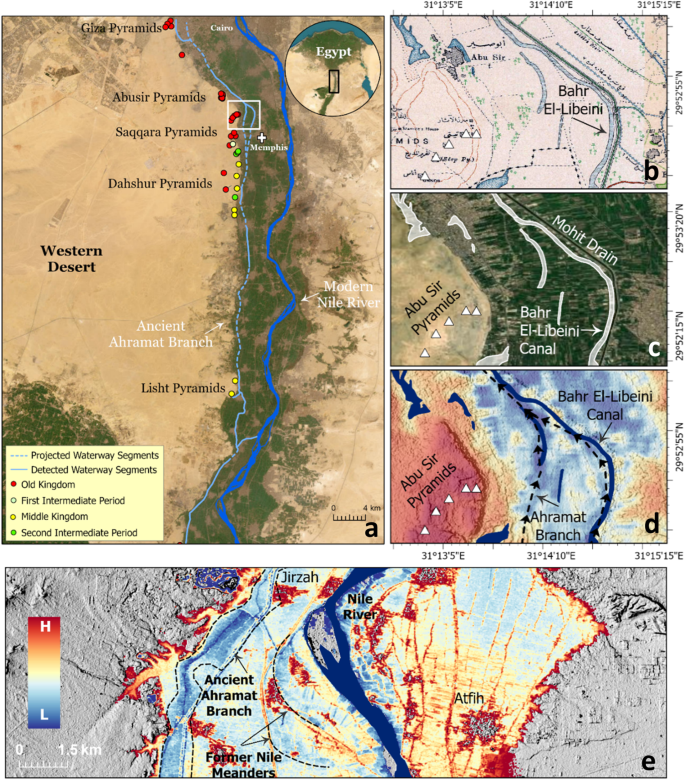
a Shows the Ahramat Branch borders a large number of pyramids dating from the Old Kingdom to the 2 nd Intermediate Period and spanning between Dynasties 3 and 13. b Shows Bahr el-Libeini canal and remnant of abandoned channel visible in the 1911 historical map (Egyptian Survey Department scale 1:50,000). c Bahr el-Libeini canal and the abandoned channel are overlain on satellite basemap. Bahr el-Libeini is possibly the last remnant of the Ahramat Branch before it migrated eastward. d A visible segment of the Ahramat Branch in TDX is now partially occupied by the modern Bahr el-Libeini canal. e A major segment of the Ahramat Branch, approximately 20 km long and 0.5 km wide, can be traced in the floodplain along the Western Desert Plateau south of the town of Jirza. Location of e is marked in white a box in a . (ESRI World Image Basemap, source: Esri, Maxar, Earthstar Geographics).
A trace of a 3 km river segment of the Ahramat Branch, with a width of about 260 m, is observable in the floodplain west of the Abu Sir pyramids field (Fig. 1b–d ). Another major segment of the Ahramat Branch, approximately 20 km long and 0.5 km wide can be traced in the floodplain along the Western Desert Plateau south of the town of Jirza (Fig. 1e ). The visible segments of the Ahramat Branch in TDX are now partially occupied by the modern Bahr el-Libeini canal. Such partial overlap between the courses of this canal, traced in the1911 historical maps (Egyptian Survey Department scale 1:50,000), and the Ahramat Branch is clear in areas where the Nile floodplain is narrower (Fig. 1b–d ), while in areas where the floodplain gets wider, the two water courses are about 2 km apart. In light of that, Bahr el-Libeini canal is possibly the last remnant of the Ahramat Branch before it migrated eastward, silted up, and vanished. In the course of the eastward migration over the Nile floodplain, the meandering Ahramat Branch would have left behind traces of abandoned channels (narrow oxbow lakes) which formed as a result of the river erosion through the neck of its meanders. A number of these abandoned channels can be traced in the 1911 historical maps near the foothill of the Western Desert plateau proving the eastward shifting of the branch at this locality (Fig. 1b–d ). The Dahshur Lake, southwest of the city of Dahshur, is most likely the last existing trace of the course of the Ahramat Branch.
Subsurface structure and sedimentology of the Ahramat Branch
Geophysical surveys using Ground Penetrating Radar (GPR) and Electromagnetic Tomography (EMT) along a 1.2 km long profile revealed a hidden river channel lying 1–1.5 m below the cultivated Nile floodplain (Fig. 2 ). The position and shape of this river channel is in an excellent match with those derived from radar satellite imagery for the Ahramat Branch. The EMT profile shows a distinct unconformity in the middle, which in this case indicates sediments that have a different texture than the overlying recent floodplain silt deposits and the sandy sediments that are adjacent to this former branch (Fig. 2 ). GPR overlapping the EMT profile from 600–1100 m on the transect confirms this. Here, we see evidence of an abandoned riverbed approximately 400 m wide and at least 25 m deep (width:depth ratio ~16) at this location. This branch has a symmetrical channel shape and has been infilled with sandy Neonile sediment different to other surrounding Neonile deposits and the underlying Eocene bedrock. The geophysical profile interpretation for the Ahramat Branch at this locality was validated using two sediment cores of depths 20 m (Core A) and 13 m (Core B) (Fig. 3 ). In Core A between the center and left bank of the former branch we found brown sandy mud at the floodplain surface and down to ~2.7 m with some limestone and chert fragments, a reddish sandy mud layer with gravel and handmade material inclusions at ~2.8 m, a gray sandy mud layer from ~3–5.8 m, another reddish sandy mud layer with gravel and freshwater mussel shells at ~6 m, black sandy mud from ~6–8 m, and sandy silt grading into clean, well-sorted medium sand dominated the profile from ~8 to >13 m. In Core B on the right bank of the former branch we found recently deposited brown sandy mud at the floodplain surface and down to ~1.5 m, alternating brown and gray layers of silty and sandy mud down to ~4 m (some reddish layers with gravel and handmade material inclusions), a black sandy mud layer from ~4–4.9 m, and another reddish sandy mud layer with gravel and freshwater mussel shells at ~5 m, before clean, well-sorted medium sand dominated the profile from 5 to >20 m. Shallow groundwater was encountered in both cores concurrently with the sand layers, indicating that the buried sedimentary structure of the abandoned Ahramat Branch acts as a conduit for subsurface water flow beneath the distal floodplain of the modern Nile River.
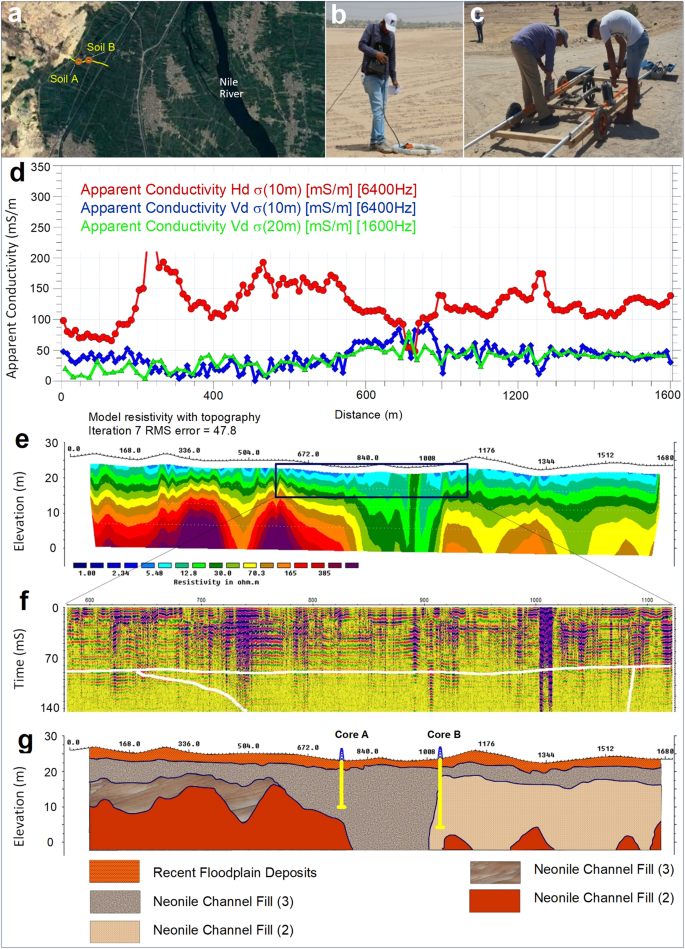
a Locations of geophysical profile and soil drilling (ESRI World Image Basemap, source: Esri, Maxar, Earthstar Geographics). Photos taken from the field while using the b Electromagnetic Tomography (EMT) and c Ground Penetrating Radar (GPR). d Showing the apparent conductivity profile, e showing EMT profile, and f showing GPR profiles with overlain sketch of the channel boundary on the GPR graph. g Simplified interpretation of the buried channel with the location of the two-soil coring of A and B.
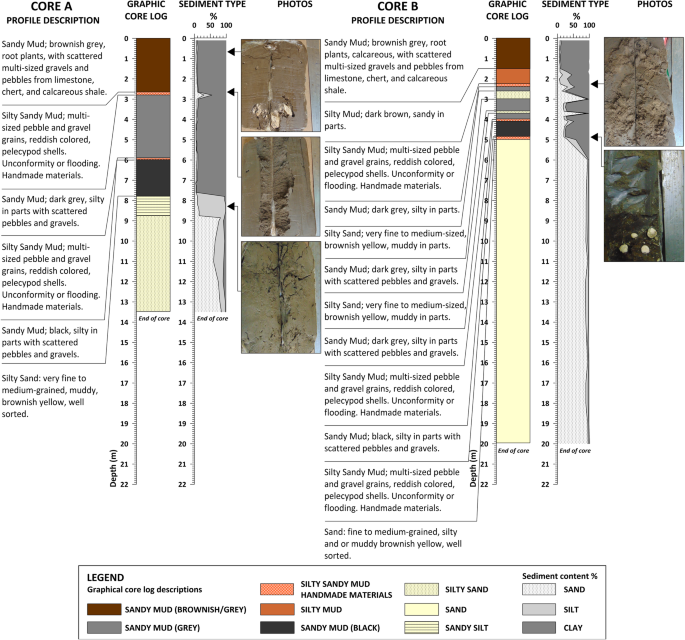
It shows two-soil cores, A and core B, with soil profile descriptions, graphic core logs, sediment grain size charts, and example photographs.
Alignment of old and middle kingdom pyramids to the Ahramat Branch
The royal pyramids in ancient Egypt are not isolated monuments, but rather joined with several other structures to form complexes. Besides the pyramid itself, the pyramid complex includes the mortuary temple next to the pyramid, a valley temple farther away from the pyramid on the edge of a waterbody, and a long sloping causeway that connects the two temples. A causeway is a ceremonial raised walkway, which provides access to the pyramid site and was part of the religious aspects of the pyramid itself 19 . In the study area, it was found that many of the causeways of the pyramids run perpendicular to the course of the Ahramat Branch and terminate directly on its riverbank.
In Egyptian pyramid complexes, the valley temples at the end of causeways acted as river harbors. These harbors served as an entry point for the river borne visitors and ceremonial roads to the pyramid. Countless valley temples in Egypt have not yet been found and, therefore, might still be buried beneath the agricultural fields and desert sands along the riverbank of the Ahramat Branch. Five of these valley temples, however, partially survived and still exist in the study area. These temples include the valley temples of the Bent Pyramid, the Pyramid of Khafre, and the Pyramid of Menkaure from Dynasty 4; the valley temple of the Pyramid of Sahure from Dynasty 5, and the valley temple of the Pyramid of Pepi II from Dynasty 6. All the aforementioned temples are dated to the Old Kingdom. These five surviving temples were found to be positioned adjacent to the riverbank of the Ahramat Branch, which strongly implies that this river branch was contemporaneously functioning during the Old Kingdom, at the time of pyramid construction.
Analysis of the ground elevation of the 31 pyramids and their proximity to the floodplain, within the study area, helped explain the position and relative water level of the Ahramat Branch during the time between the Old Kingdom and Second Intermediate Period (ca. 2649–1540 BCE). Based on Fig. ( 4) , the Ahramat Branch had a high-water level during the first part of the Old Kingdom, especially during Dynasty 4. This is evident from the high ground elevation and long distance from the floodplain of the pyramids dated to that period. For instance, the remote position of the Bent and Red Pyramids in the desert, very far from the Nile floodplain, is a testament to the branch’s high-water level. On the contrary, during the Old Kingdom, our data demonstrated that the Ahramat Branch would have reached its lowest level during Dynasty 5. This is evident from the low altitudes and close proximity to the floodplain of most Dynasty 5 pyramids. The orientation of the Sahure Pyramid’s causeway (Dynasty 5) and the location of its valley temple in the low-lying floodplain provide compelling evidence for the relatively low water level proposition of the Ahramat Branch during this stage. The water level of the Ahramat Branch would have been slightly raised by the end of Dynasty 5 (the last 15–30 years), during the reign of King Unas and continued to rise during Dynasty 6. The position of Pepi II and Merenre Pyramids (Dynasty 6) deep in the desert, west of the Djedkare Isesi Pyramid (Dynasty 5), supports this notion.
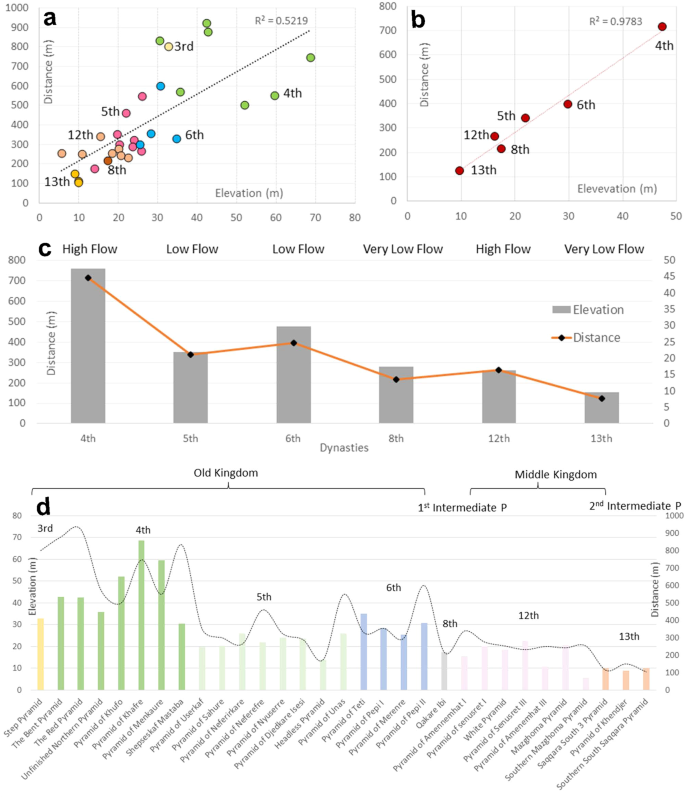
It explains the position and relative water level of the Ahramat Branch during the time between the Old Kingdom and Second Intermediate Period. a Shows positive correlation between the ground elevation of the pyramids and their proximity to the floodplain. b Shows positive correlation between the average ground elevation of the pyramids and their average proximity to the floodplain in each Dynasty. c Illustrates the water level interpretation by Hassan (1986) in Faiyum Lake in correlation to the average pyramids ground elevation and average distances to the floodplain in each Dynasty. d The data indicates that the Ahramat Branch had a high-water level during the first period of the Old Kingdom, especially during Dynasty 4. The water level reduced afterwards but was raised slightly in Dynasty 6. The position of the Middle Kingdom’s pyramids, which was at lower altitudes and in close proximity to the floodplain as compared to those of the Old Kingdom might be explained by the slight eastward migration of the Ahramat Branch.
In addition, our analysis in Fig. ( 4) , shows that the Qakare Ibi Pyramid of Dynasty 8 was constructed very close to the floodplain on very low elevation, which implies that the Nile water levels were very low at this time of the First Intermediate Period (2181–2055 BCE). This finding is in agreement with previous work conducted by Kitchen 20 which implies that the sudden collapse of the Old Kingdom in Egypt (after 4160 BCE) was largely caused by catastrophic failure of the annual flood of the Nile River for a period of 30–40 years. Data from soil cores near Memphis indicated that the Old Kingdom settlement is covered by about 3 m of sand 11 . Accordingly, the Ahramat Branch was initially positioned further west during the Old Kingdom and then shifted east during the Middle Kingdom due to the drought-induced sand encroachments of the First Intermediate Period, “a period of decentralization and weak pharaonic rule” in ancient Egypt, spanning about 125 years (2181–2055 BCE) post Old Kingdom era. Soil cores from the drilling program at Memphis show dominant dry conditions during the First Intermediate Period with massive eolian sand sheets extended over a distance of at least 0.5 km from the edge of the western desert escarpment 21 . The Ahramat Branch continued to move east during the Second Intermediate Period until it had gradually lost most of its water supply by the New Kingdom.
The western tributaries of the Ahramat Branch
Sentinal-1 radar data unveiled several wide channels (inlets) in the Western Desert Plateau connected to the Ahramat Branch. These inlets are currently covered by a layer of sand, thus partially invisible in multispectral satellite imagery. In Sentinal-1 radar imagery, the valley floors of these inlets appear darker than the surrounding surfaces, indicating subsurface fluvial deposits. These smooth deposits appear dark owing to the specular reflection of the radar signals away from the receiving antenna (Fig. 5a, b ) 22 . Considering that Sentinel-1’s C-Band has a penetration capability of approximately 50 cm in dry sand surface 23 , this would suggest that the riverbed of these channels is covered by at least half a meter of desert sand. Unlike these former inlets, the course of the Ahramat Branch is invisible in SAR data due in large part to the presence of dense farmlands in the floodplain, which limits radar penetration and the detection of underlying fluvial deposition. Moreover, the radar topographic data from TDX revealed the areal extent of these inlets. Their river courses were extracted from TDX data using the Topographic Position Index (TPI), an algorithm which is used to compute the topographic slope positions and to automate landform classifications (Fig. 5c, d ). Negative TPI values show the former riverbeds of the inlets, while positive TPI signify the riverbanks bordering them.
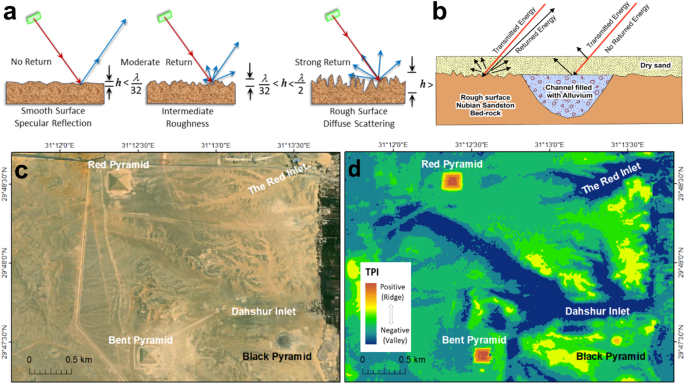
a Conceptual sketch of the dependence of surface roughness on the sensor wavelength λ (modified after 48 ). b Expected backscatter characteristics in sandy desert areas with buried dry riverbeds. c Dry channels/inlets masked by desert sand in the Dahshur area. d The channels’ courses were extracted using TPI. Negative TPI values highlight the courses of the channels while positive TPI signify their banks.
Analysis indicated that several of the pyramid’s causeways, from Dynasties 4 and 6, lead to the inlet’s riverbanks (Fig. 6 ). Among these pyramids, are the Bent Pyramid, the first pyramid built by King Snefru in Dynasty 4 and among the oldest, largest, and best preserved ancient Egyptian pyramids that predates the Giza Pyramids. This pyramid is situated at the royal necropolis of Dahshur. The position of the Bent Pyramid, deep in the desert, far from the modern Nile floodplain, remained unexplained by researchers. This pyramid has a long causeway (~700 m) that is paved in the desert with limestone blocks and is attached to a large valley temple. Although all the pyramids’ valley temples in Egypt are connected to a water body and served as the landing point of all the river-borne visitors, the valley temple of the Bent Pyramid is oddly located deep in the desert, very distant from any waterways and more than 1 km away from the western edge of the modern Nile floodplain. Radar data revealed that this temple overlooked the bank of one of these extinct channels (called Wadi al-Taflah in historical maps). This extinct channel (referred to hereafter as the Dahshur Inlet due to its geographical location) is more than 200 m wide on average (Fig. 6 ). In light of this finding, the Dahshur Inlet, and the Ahramat Branch, are thus strongly argued to have been active during Dynasty 4 and must have played an important role in transporting building materials to the Bent Pyramid site. The Dahshur Inlet could have also served the adjacent Red Pyramid, the second pyramid built by the same king (King Snefru) in the Dahshur area. Yet, no traces of a causeway nor of a valley temple has been found thus far for the Red Pyramid. Interestingly, pyramids in this site dated to the Middle Kingdom, including the Amenemhat III pyramid, also known as the Black Pyramid, White Pyramid, and Pyramid of Senusret III, are all located at least 1 km far to the east of the Dynasty 4 pyramids (Bent and Red) near the floodplain (Fig. 6 ), which once again supports the notion of the eastward shift of the Ahramat Branch after the Old Kingdom.
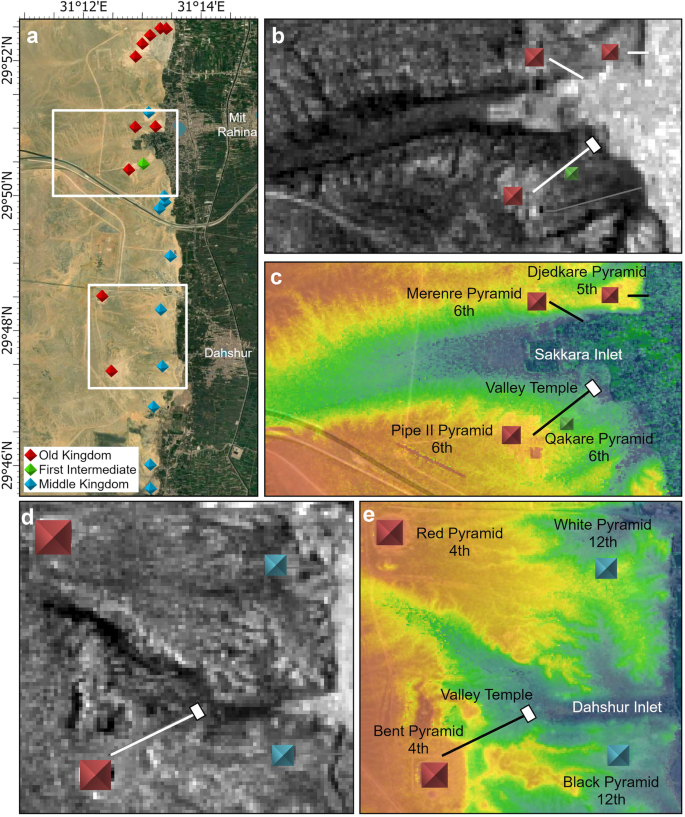
a The two inlets are presently covered by sand, thus invisible in optical satellite imagery. b Radar data, and c TDX topographic data reveal the riverbed of the Sakkara Inlet due to radar signals penetration capability in dry sand. b and c show the causeways of Pepi II and Merenre Pyramids, from Dynasty 6, leading to the Saqqara Inlet. The Valley Temple of Pepi II Pyramid overlooks the inlet riverbank, which indicates that the inlet, and thus Ahramat Branch, were active during Dynasty 6. d Radar data, and e TDX topographic data, reveal the riverbed of the Dahshur Inlet with the Bent Pyramid’s causeway of Dynasty 4 leading to the Inlet. The Valley Temple of the Bent Pyramid overlooks the riverbank of the Dahshur Inlet, which indicates that the inlet and the Ahramat Branch were active during Dynasty 4 of the Old Kingdom.
Radar satellite data revealed yet another sandy buried channel (tributary), about 6 km north of the Dahshur Inlet, to the west of the ancient city of Memphis. This former fluvial channel (referred to hereafter as the Saqqara Inlet due to its geographical location) connects to the Ahramat Branch with a broad river course of more than 600 m wide. Data shows that the causeways of the two pyramids of Pepi II and Merenre, situated at the royal necropolis of Saqqara and dated to Dynasty 6, lead directly to the banks of the Saqqara Inlet (see Fig. 6 ). The 400 m long causeway of Pepi II pyramid runs northeast over the southern Saqqara plateau and connects to the riverbank of the Saqqara Inlet from the south. The causeway terminates with a valley temple that lies on the inlet’s riverbank. The 250 long causeway of the Pyramid of Merenre runs southeast over the northern Saqqara plateau and connects to the riverbank of the Saqqara Inlet from the north. Since both pyramids dated to Dynasty 6, it can be argued that the water level of the Ahramat Branch was higher during this period, which would have flooded at least the entrance of its western inlets. This indicates that the downstream segment of the Saqqara Inlet was active during Dynasty 6 and played a vital role in transporting construction materials and workers to the two pyramids sites. The fact that none of the Dynasty 5 pyramids in this area (e.g., the Djedkare Isesi Pyramid) were positioned on the Saqqara Inlet suggests that the water level in the Ahramat Branch was not high enough to enter and submerge its inlets during this period.
In addition, our data analysis clearly shows that the causeways of the Khafre, Menkaure, and Khentkaus pyramids, in the Giza Plateau, lead to a smaller but equally important river bay associated with the Ahramat Branch. This lagoon-like river arm is referred to here as the Giza Inlet (Fig. 7 ). The Khufu Pyramid, the largest pyramid in Egypt, seems to be connected directly to the river course of the Ahramat Branch (Fig. 7 ). This finding proves once again that the Ahramat Branch and its western inlets were hydrologically active during Dynasty 4 of the Old Kingdom. Our ancient river inlet hypothesis is also in accordance with earlier research, conducted on the Giza Plateau, which indicates the presence of a river and marsh-like environment in the floodplain east of the Giza pyramids 2 .
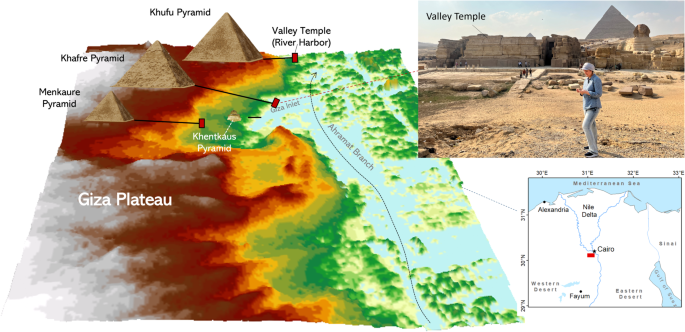
The causeways of the four Pyramids lead to an inlet, which we named the Giza Inlet, that connects from the west with the Ahramat Branch. These causeways connect the pyramids with valley temples which acted as river harbors in antiquity. These river segments are invisible in optical satellite imagery since they are masked by the cultivated lands of the Nile floodplain. The photo shows the valley temple of Khafre Pyramid (Photo source: Author Eman Ghoneim).
During the Old Kingdom Period, our analysis suggests that the Ahramat Branch had a high-water level during the first part, especially during Dynasty 4 whereas this water level was significantly decreased during Dynasty 5. This finding is in agreement with previous studies which indicate a high Nile discharge during Dynasty 4 (e.g., ref. 24 ). Sediment isotopic analysis of the Nile Delta indicated that Nile flows decrease more rapidly by the end of Dynasty 4 25 , in addition 26 reported that during Dynasties 5 and 6 the Nile flows were the lowest of the entire Dynastic period. This long-lost Ahramat Branch (possibly a former Yazoo tributary to the Nile) was large enough to carry a large volume of the Nile discharge in the past. The ancient channel segment uncovered by 1 , 15 west of the city of Memphis through borehole logs is most likely a small section of the large Ahramat Branch detected in this study. In the Middle Kingdom, although previous studies implied that the Nile witnessed abundant flood with occasional failures (e.g., ref. 27 ), our analysis shows that all the pyramids from the Middle Kingdom were built far east of their Old Kingdom counterparts, on lower altitudes and in close proximity to the floodplain as compared to those of the Old Kingdom. This paradox might be explained by the fact that the Ahramat Branch migrated eastward, slightly away from the Western Desert escarpment, prior to the construction of the Middle Kingdom pyramids, resulting in the pyramids being built eastward so that they could be near the waterway.
The eastward migration and abandonment of the Ahramat Branch could be attributed to gradual tilting of the Nile delta and floodplain in lower Egypt towards the northeast due to tectonic activity 28 . A topographic tilt such as this would have accelerated river movement eastward due to the river being located in the west at a relatively higher elevation of the floodplain. While near-channel floodplain deposition would naturally lead to alluvial ridge development around the active Ahramat Branch, and therefore to lower-lying tracts of adjacent floodplain to the east, regional tilting may explain the wholesale lateral migration of the river in that direction. The eastward migration and abandonment of the branch could also be ascribed to sand incursion due to the branch’s proximity to the Western Desert Plateau, where windblown sand is abundant. This would have increased sand deposition along the riverbanks and caused the river to silt up, particularly during periods of low flow. The region experienced drought during the First Intermediate Period, prior to the Middle Kingdom. In the area of Abu Rawash north 29 and Dahshur site 11 , settlements from the Early Dynastic and Old Kingdom were found to be covered by more than 3 m of desert sands. During this time, windblown sand engulfed the Old Kingdom settlements and desert sands extended eastward downhill over a distance of at least 0.5 km 21 . The abandonment of sites at Abusir (5 th Dynasty), where the early pottery-rich deposits are covered by wind-blown sand and then mud without sherds, can be used as evidence that the Ahramat Branch migrated eastward after the Old Kingdom. The increased sand deposition activity, during the end of the Old Kingdom, and throughout the First Intermediate Period, was most likely linked to the period of drought and desertification of the Sahara 30 . In addition, the reduced river discharge caused by decreased rainfall and increased aridity in the region would have gradually reduced the river course’s capacity, leading to silting and abandonment of the Ahramat Branch as the river migrated to the east.
The Dahshur, Saqqara, and Giza inlets, which were connected to the Ahramat Branch from the west, were remnants of past active drainage systems dated to the late Tertiary or the Pleistocene when rainwater was plentiful 31 . It is proposed that the downstream reaches of these former channels (wadis) were submerged during times of high-water levels of the Ahramat Branch, forming long narrow water arms (inlets) that gave a wedge-like shape to the western flank of the Ahramat Branch. During the Old Kingdom, the waters of these inlets would have flowed westward from the Ahramat Branch rather than from their headwaters. As the drought intensified during the First Intermediate Period, the water level of the Ahramat Branch was lowered and withdrew from its western inlets, causing them to silt up and eventually dry out. The Dahshur, Saqqara, and Giza inlets would have provided a bay environment where the water would have been calm enough for vessels and boats to dock far from the busy, open water of the Ahramat Branch.
Sediments from the Ahramat Branch riverbed, which were collected from the two deep soil cores (cores A and B), show an abrupt shift from well-sorted medium sands at depth to overlying finer materials with layers including gravel, shell, and handmade materials. This indicates a step-change from a relatively consistent higher-energy depositional regime to a generally lower-energy depositional regime with periodic flash floods at these sites. So, the Ahramat Branch in this region carried and deposited well-sorted medium sand during its last active phase, and over time became inactive, infilling with sand and mud until an abrupt change led the (by then) shallow depression fill with finer distal floodplain sediment (possibly in a wetland) that was utilized by people and experienced periodic flash flooding. Validation of the paleo-channel position and sediment type using these cores shows that the Ahramat Branch has similar morphological features and an upward-fining depositional sequence as that reported near Giza, where two cores were previously used to reconstruct late Holocene Nile floodplain paleo-environments 2 . Further deep soil coring could determine how consistent the geomorphological features are along the length of the Ahramat branch, and to help explain anomalies in areas where the branch has less surface expression and where remote sensing and geophysical techniques have limitations. Considering more core logs can give a better understanding of the floodplain and the buried paleo-channels.
The position of the Ahramat Branch along the western edge of the Nile floodplain suggests it to be the downstream extension of Bahr Yusef. In fact, Bahr Yusef’s course may have initially flowed north following the natural surface gradient of the floodplain before being forced to turn west to flow into the Fayum Depression. This assumption could be supported by the sharp westward bend of Bahr Yusef’s course at the entrance to the Fayum Depression, which could be a man-made attempt to change the waterflow direction of this branch. According to Römer 32 , during the Middle Kingdom, the Gadallah Dam located at the entrance of the Fayum, and a possible continuation running eastwards, blocked the flow of Bahr Yusef towards the north. However, a sluice, probably located near the village of el-Lahun, was created in order to better control the flow of water into the Fayum. When the sluice was locked, the water from Bahr Yusef was directed to the west and into the depression, and when the sluice was open, the water would flow towards the north via the course of the Ahramat Branch. Today, the abandoned Ahramat Branch north of Fayum appears to support subsurface water flow in the buried coarse sand bed layers, however these shallow groundwater levels are likely to be quite variable due to proximity of the bed layers to canals and other waterways that artificially maintain shallow groundwater. Groundwater levels in the region are known to be variable 33 , but data on shallow groundwater could be used to further validate the delineated paleo-channel of the Ahramat Branch.
The present work enabled the detection of segments of a major former Nile branch running at the foothills of the Western Desert Plateau, where the vast majority of the Ancient Egyptian pyramids lie. The enormity of this branch and its proximity to the pyramid complexes, in addition to the fact that the pyramids’ causeways terminate at its riverbank, all imply that this branch was active and operational during the construction phase of these pyramids. This waterway would have connected important locations in ancient Egypt, including cities and towns, and therefore, played an important role in the cultural landscape of the region. The eastward migration and abandonment of the Ahramat Branch could be attributed to gradual movement of the river to the lower-lying adjacent floodplain or tilting of the Nile floodplain toward the northeast as a result of tectonic activity, as well as windblown sand incursion due to the branch’s proximity to the Western Desert Plateau. The increased sand deposition was most likely related to periods of desertification of the Great Sahara in North Africa. In addition, the branch eastward movement and diminishing could be explained by the reduction of the river discharge and channel capacity caused by the decreased precipitation and increased aridity in the region, particularly during the end of the Old Kingdom.
The integration of radar satellite data with geophysical surveying and soil coring, which we utilized in this study, is a highly adaptable approach in locating similar former buried river systems in arid regions worldwide. Mapping the hidden course of the Ahramat Branch, allowed us to piece together a more complete picture of ancient Egypt’s former landscape and a possible water transportation route in Lower Egypt, in the area between Lisht and the Giza Plateau.
Revealing this extinct Nile branch can provide a more refined idea of where ancient settlements were possibly located in relation to it and prevent them from being lost to rapid urbanization. This could improve the protection measures of Egyptian cultural heritage. It is the hope that our findings can improve conservation measures and raise awareness of these sites for modern development planning. By understanding the landscape of the Nile floodplain and its environmental history, archeologists will be better equipped to prioritize locations for fieldwork investigation and, consequently, raise awareness of these sites for conservation purposes and modern development planning. Our finding has filled a much-needed knowledge gap related to the dominant waterscape in ancient Egypt, which could help inform and educate a wide array of global audiences about how earlier inhabitants were living and in what ways shifts in their landscape drove human activity in such an iconic region.
Materials and methods
The work comprised of two main elements: satellite remote sensing and historical maps and geophysical survey and sediment coring, complemented by archeological resources. Using this suite of investigative techniques provided insights into the nature and relationship of the former Ahramat Branch with the geographical location of the pyramid complexes in Egypt.
Satellite remote sensing and historical maps
Unlike optical sensors that image the land surface, radar sensors image the subsurface due to their unique ability to penetrate the ground and produce images of hidden paleo-rivers and structures. In this context, radar waves strip away the surface sand layer and expose previously unidentified buried channels. The penetration capability of radar waves in the hyper-arid regions of North Africa is well documented 4 , 34 , 35 , 36 , 37 . The penetration depth varies according to the radar wavelength used at the time of imaging. Radar signal penetration becomes possible without significant attenuation if the surface cover material is extremely dry (<1% moisture content), fine grained (<1/5 of the imaging wavelength) and physically homogeneous 23 . When penetrating desert sand, radar signals have the ability to detect subsurface soil roughness, texture, compactness, and dielectric properties 38 . We used the European Space Agency (ESA) Sentinel-1 data, a radar satellite constellation consisting of a C-Band synthetic aperture radar (SAR) sensor, operating at 5.405 GHz. The Sentinel-1 SAR image used here was acquired in a descending orbit with an interferometric wide swath mode (IW) at ground resolutions of 5 m × 20 m, and dual polarizations of VV + VH. Since Sentinal-1 is operated in the C-Band, it has an estimated penetration depth of 50 cm in very dry, sandy, loose soils 39 . We used ENVI v. 5.7 SARscape software for processing radar imagery. The used SAR processing sequences have generated geo-coded, orthorectified, terrain-corrected, noise free, radiometrically calibrated, and normalized Sentinel-1 images with a pixel size of 12.5 m. In SAR imagery subsurface fluvial deposits appear dark owing to specular reflection of the radar signals away from the receiving antenna, whereas buried coarse and compacted material, such as archeological remains appear bright due to diffuse reflection of radar signals 40 .
Other previous studies have shown that combining radar topographic imagery (e.g., Shuttle Radar Topography Mission-SRTM) with SAR images improves the extraction and delineation of mega paleo-drainage systems and lake basins concealed under present-day topographic signatures 3 , 4 , 22 , 41 . Topographic data represents a primary tool in investigating surface landforms and geomorphological change both spatially and temporally. This data is vital in mapping past river systems due to its ability to show subtle variations in landform morphology 37 . In low lying areas, such as the Nile floodplain, detailed elevation data can detect abandoned channels, fossilized natural levees, river meander scars and former islands, which are all crucial elements for reconstructing the ancient Nile hydrological network. In fact, the modern topography in many parts of the study area is still a good analog of the past landscape. In the present study, TanDEM-X (TDX) topographic data, from the German Aerospace Centre (DLR), has been utilized in ArcGIS Pro v. 3.1 software due to its fine spatial resolution of 0.4 arc-second ( ∼ 12 m). TDX is based on high frequency X-Band Synthetic Aperture Radar (SAR) (9.65 GHz) and has a relative vertical accuracy of 2 m for areas with a slope of ≤20% 42 . This data was found to be superior to other topographic DEMs (e.g., Shuttle Radar Topography Mission and ASTER Global Digital Elevation Map) in displaying fine topographic features even in the cultivated Nile floodplain, thus making it particularly well suited for this study. Similar archeological investigations using TDX elevation data in the flat terrains of the Seyhan River in Turkey and the Nile Delta 43 , 44 allowed for the detection of levees and other geomorphologic features in unprecedented spatial resolution. We used the Topographic Position Index (TPI) module of 45 with the TDX data by applying varying neighboring radiuses (20–100 m) to compute the difference between a cell elevation value and the average elevation of the neighborhood around that cell. TPI values of zero are either flat surfaces with minimal slope, or surfaces with a constant gradient. The TPI can be computed using the following expression 46 .
Where the scaleFactor is the outer radius in map units and Irad and Orad are the inner and outer radius of annulus in cells. Negative TPI values highlight abandoned riverbeds and meander scars, while positive TPI signify the riverbanks and natural levees bordering them.
The course of the Ahramat Branch was mapped from multiple data sources and used different approaches. For instance, some segments of the river course were derived automatically using the TPI approach, particularly in the cultivated floodplain, whereas others were mapped using radar roughness signatures specially in sandy desert areas. Moreover, a number of abandoned channel segments were digitized on screen from rectified historical maps (Egyptian Survey Department scale 1:50,000 collected on years 1910–1911) near the foothill of the Western Desert Plateau. These channel segments together with the former river course segments delineated from radar and topographic data were aggregated to generate the former Ahramat Branch. In addition to this and to ensure that none of the channel segments of the Ahramat Branch were left unmapped during the automated process, a systematic grid-based survey (through expert’s visual observation) was performed on the satellite data. Here, Landsat 8 and Sentinal-2 multispectral images, Sentinal-1 radar images and TDX topographic data were used as base layers, which were thoroughly examined, grid-square by grid-square (2*2 km per a square) at a full resolution, in order to identify small-scale fluvial landforms, anomalous agricultural field patterns and irregular ditches, and determine their spatial distributions. Here, ancient fluvial channels were identified using two key aspects: First, the sinuous geometry of natural and manmade features and, second the color tone variations in the satellite imagery. For example, clusters of contiguous pixels with darker tones and sinuous shapes may signify areas of a higher moisture content in optical imagery, and hence the possible existence of a buried riverbed. Stretching and edge detection were applied to enhance contrasts in satellite images brightness to enable the visualization of traces of buried river segments that would otherwise go unobserved. Lastly, all the pyramids and causeways in the study site, along with ancient harbors and valley temples, as indicators of preexisting river channels, were digitized from satellite data and available archeological resources and overlaid onto the delineated Ahramat Branch for geospatial analysis.
Geophysical survey and sediment coring
Geophysical measurements using Ground Penetrating Radar (GPR) and Electromagnetic Tomography (EMT) were utilized to map subsurface fluvial features and validate the satellite remote sensing findings. GPR is effective in detecting changes of dielectric constant properties of sediment layers, and its signal responses can be directly related to changes in relative porosity, material composition, and moisture content. Therefore, GPR can help in identifying transitional boundaries in subsurface layers. EMT, on the other hand, shows the variations and thickness of large-scale sedimentary deposits and is more useful in clay-rich soil than GPR. In summer 2022, a geophysical profile was measured using GPR and EMT units with a total length of approximately 1.2 km. The GPR survey was conducted with a central frequency antenna of 35 MHz and a trigger interval of 5 cm. The EMT survey was performed using the multi-frequency terrain conductivity (EM–34–3) measuring system with a spacing of 10–11 meters between stations. To validate the remote sensing and geophysical data, two sediment cores with depths of 20 m (Core A) and 13 m (Core B) were collected using a deep soil driller. These cores were collected from along the geophysical profile in the floodplain. Sieving and organic analysis were performed on the sediment samples at Tanta University sediment lab to extract information about grain size for soil texture and total organic carbon. In soil texture analysis medium to coarse sediment, such as sands, are typical for river channel sediments, loamy sand and sandy loam deposits can be interpreted as levees and crevasse splays, whereas fine texture deposits, such as silt loam, silty clay loam, and clay deposits, are representative of the more distal parts of the river floodplain 47 .
Data availability
Data for replicating the results of this study are available as supplementary files at: https://figshare.com/articles/journal_contribution/Pyramids_Elevations_and_Distances_xlsx/25216259 .
Bunbury, J., Tavares, A., Pennington, B. & Gonçalves, P. Development of the Memphite Floodplain: Landscape and Settlement Symbiosis in the Egyptian Capital Zone. In The Nile: Natural and Cultural Landscape in Egypt (eds. Willems, H. & Dahms, J.-M.) 71–96 (Transcript Verlag, 2017). https://doi.org/10.1515/9783839436158-003 .
Sheisha, H. et al. Nile waterscapes facilitated the construction of the Giza pyramids during the 3rd millennium BCE. Proc. Natl. Acad. Sci. 119 , e2202530119 (2022).
Article CAS Google Scholar
Ghoneim, E. & El-Baz, F. K. DEM‐optical‐radar data integration for palaeohydrological mapping in the northern Darfur, Sudan: implication for groundwater exploration. Int. J. Remote Sens. 28 , 5001–5018 (2007).
Article Google Scholar
Ghoneim, E., Benedetti, M. M. & El-Baz, F. K. An integrated remote sensing and GIS analysis of the Kufrah Paleoriver, Eastern Sahara. Geomorphology 139 , 242–257 (2012).
Zaki, A. S. et al. Did increased flooding during the African Humid Period force migration of modern humans from the Nile Valley? Quat. Sci. Rev. 272 , 107200 (2021).
Rohling, E. J., Marino, G. & Grant, K. M. Mediterranean climate and oceanography, and the periodic development of anoxic events (sapropels). Earth Sci. Rev. 143 , 62–97 (2015).
DeMenocal, P. et al. Abrupt onset and termination of the African Humid Period: rapid climate responses to gradual insolation forcing. Quat. Sci. Rev. 19 , 347–361 (2000).
Ritchie, J. C. & Haynes, C. V. Holocene vegetation zonation in the eastern Sahara. Nature 330 , 645–647 (1987).
Butzer, K. W. Early Hydraulic Civilization in Egypt: A Study in Cultural Ecology (The University of Chicago press, Chicago [Ill.] London, 1976).
Kröpelin, S. et al. Climate-Driven Ecosystem Succession in the Sahara: The Past 6000 Years. Science 320 , 765–768 (2008).
Bunbury, J. & Jeffreys, D. Real and Literary Landscapes in Ancient Egypt. Camb. Archaeol. J. 21 , 65–76 (2011).
Sterling, S. Mortality Profiles as Indicators of Slowed Reproductive Rates: Evidence from Ancient Egypt. J. Anthropol. Archaeol. 18 , 319–343 (1999).
Hillier, J. K., Bunbury, J. M. & Graham, A. Monuments on a migrating Nile. J. Archaeol. Sci. 34 , 1011–1015 (2007).
Bunbury, J. & Lutley, K. The Nile on the move. https://api.semanticscholar.org/CorpusID:131474399 (2008).
Hassan, F. A., Hamdan, M. A., Flower, R. J., Shallaly, N. A. & Ebrahem, E. Holocene alluvial history and archaeological significance of the Nile floodplain in the Saqqara-Memphis region, Egypt. Quat. Sci. Rev. 176 , 51–70 (2017).
Bietak, M., Czerny, E. & Forstner-Müller, I. Cities and urbanism in ancient Egypt . Papers from a workshop in November 2006 at the Austrian Academy of Sciences (Austrian Academy of Sciences, 2010).
El-Qady, G., Shaaban, H., El-Said, A. A., Ghazala, H. & El-Shahat, A. Tracing of the defunct Canopic Nile branch using geoelectrical resistivity data around Itay El-Baroud area, Nile Delta, Egypt. J. Geophys. Eng. 8 , 83–91 (2011).
Toonen, W. H. J. et al. Holocene fluvial history of the Nile’s west bank at ancient Thebes, Luxor, Egypt, and its relation with cultural dynamics and basin-wide hydroclimatic variability. Geoarchaeology 33 , 273–290 (2018).
Lehner, M. The Complete Pyramids (Thames and Hudson, New York, 1997).
Kitchen, K. A. The chronology of ancient Egypt. World Archaeol. 23 , 201–208 (1991).
Giddy, L. & Jeffreys, D. Memphis, 1991. J. Egypt. Archaeol. 78 , 1–11 (1992).
Ghoneim, E., Robinson, C. & El‐Baz, F. Radar topography data reveal drainage relics in the eastern Sahara. Int. J. Remote Sens. 28 , 1759–1772 (2007).
Roth, L. & Elachi, C. Coherent electromagnetic losses by scattering from volume inhomogeneities. IEEE Trans. Antennas Propag. 23 , 674–675 (1975).
Hassan, F. A. Holocene lakes and prehistoric settlements of the Western Faiyum, Egypt. J. Archaeol. Sci. 13 , 483–501 (1986).
Woodward, J. C., Macklin, M. G., Krom, M. D. & Williams, M. A. J. The Nile: Evolution, Quaternary River Environments and Material Fluxes. In Large Rivers (ed. Gupta, A.) 261–292 (John Wiley & Sons, Ltd, Chichester, UK, 2007). https://doi.org/10.1002/9780470723722.ch13 .
Krom, M. D., Stanley, J. D., Cliff, R. A. & Woodward, J. C. Nile River sediment fluctuations over the past 7000 yr and their key role in sapropel development. Geology 30 , 71–74 (2002).
Stanley, J.-D., Krom, M. D., Cliff, R. A. & Woodward, J. C. Short contribution: Nile flow failure at the end of the Old Kingdom, Egypt: Strontium isotopic and petrologic evidence. Geoarchaeology 18 , 395–402 (2003).
Stanley, D. J. & Warne, A. G. Nile Delta: Recent Geological Evolution and Human Impact. Science 260 , 628–634 (1993).
Jones, M. A new old Kingdom settlement near Ausim: report of the archaeological discoveries made in the Barakat drain improvements project, https://api.semanticscholar.org/CorpusID:194486461 (1995).
Bunbury, J. M. The development of the River Nile and the Egyptian Civilization: A Water Historical Perspective with Focus on the First Intermediate Period. In A History of Water: Rivers and Society — From the Birth of Agriculture to Modern Times , Vol. 2 (eds. Tvedt, T. & Coopey, R) 50–69 (I.B. Tauris, 2010).
Bubenzer, O. & Riemer, H. Holocene climatic change and human settlement between the central Sahara and the Nile Valley: Archaeological and geomorphological results. Geoarchaeology 22 , 607–620 (2007).
Römer, C. The Nile in the Fayum: Strategies of Dominating and Using the Water Resources of the River in the Oasis in the Middle Kingdom and the Graeco-Roman Period. In The Nile: Natural and Cultural Landscape in Egypt (eds. Willems, H. & Dahms, J.-M.) 171–192 (transcript Verlag, 2017). https://doi.org/10.1515/9783839436158-006 .
Mansour, K. et al. Investigation of Groundwater Occurrences Along the Nile Valley Between South Cairo and Beni Suef, Egypt, Using Geophysical and Geodetic Techniques. Pure Appl. Geophys. 180 , 3071–3088 (2023).
McCauley, J. F. et al. Subsurface Valleys and Geoarcheology of the Eastern Sahara Revealed by Shuttle Radar. Science 218 , 1004–1020 (1982).
El-Baz, F. & Robinson, C. A. Paleo-channels revealed by SIR-C data in the Western Desert of Egypt: Implications to sand dune accumulations. In Proceedings of the 12th International Conference on Applied Geologic Remote Sensing , Vol. 1, I–469 (Environmental Research Institute of Michigan, Ann Arbor, 1997).
Robinson, C. A., El-Baz, F., Al-Saud, T. S. M. & Jeon, S. B. Use of radar data to delineate palaeodrainage leading to the Kufra Oasis in the eastern Sahara. J. Afr. Earth Sci. 44 , 229–240 (2006).
Ghoneim, E. Rimaal: A Sand Buried Structure of Possible Impact Origin in the Sahara: Optical and Radar Remote Sensing Investigation. Remote Sens. 10 , 880 (2018).
Ghoneim, E. M. Ibn-Batutah: A possible simple impact structure in southeastern Libya, a remote sensing study. Geomorphology 103 , 341–350 (2009).
Schaber, G. G., Kirk, R. L. & Strom, R. Data base of impact craters on Venus based on analysis of Magellan radar images and altimetry data. U.S. Geological Survey, Open-File Report, https://doi.org/10.3133/ofr98104 , https://pubs.usgs.gov/of/1998/0104/report.pdf (1998).
Ghoneim, E. & El-Baz, F. K. Satellite Image Data Integration for Groundwater Exploration in Egypt, https://api.semanticscholar.org/CorpusID:216495993 (2020).
Skonieczny, C. et al. African humid periods triggered the reactivation of a large river system in Western Sahara. Nat. Commun. 6 , 8751 (2015).
Wessel, B. et al. Accuracy assessment of the global TanDEM-X Digital Elevation Model with GPS data. ISPRS J. Photogramm. Remote Sens. 139 , 171–182 (2018).
Erasmi, S., Rosenbauer, R., Buchbach, R., Busche, T. & Rutishauser, S. Evaluating the Quality and Accuracy of TanDEM-X Digital Elevation Models at Archaeological Sites in the Cilician Plain, Turkey. Remote Sens. 6 , 9475–9493 (2014).
Ginau, A., Schiestl, R. & Wunderlich, J. Integrative geoarchaeological research on settlement patterns in the dynamic landscape of the northwestern Nile delta. Quat. Int. 511 , 51–67 (2019).
JENNESS, J. Topographic position index (tpi_jen.avx_extension for Arcview 3.x, v.1.3a, Jenness Enterprises [EB/OL], http://www.jennessent.com/arcview/tpi.htm (2006).
Weiss, A. D. Topographic position and landforms analysis, https://api.semanticscholar.org/CorpusID:131349144 (2001).
Verstraeten, G., Mohamed, I., Notebaert, B. & Willems, H. The Dynamic Nature of the Transition from the Nile Floodplain to the Desert in Central Egypt since the Mid-Holocene. In The Nile: Natural and Cultural Landscape in Egypt (eds. Willems, H. & Dahms, J.-M.) 239–254 (transcript Verlag, 2017). https://doi.org/10.1515/9783839436158-009 .
Meyer, F. Spaceborne Synthetic Aperture Radar: Principles, data access, and basic processing techniques. In Synthetic Aperture Radar the SAR Handbook: Comprehensive Methodologies for Forest Monitoring and Biomass Estimation. 21–64 (2019). https://doi.org/10.25966/nr2c-s697 , https://gis1.servirglobal.net/TrainingMaterials/SAR/SARHB_FullRes.pdf .
Download references
Acknowledgements
This work was funded by NSF grant # 2114295 awarded to E.G., S.O. and T.R. and partially supported by Research Momentum Fund, UNCW, to E.G. TanDEM-X data was awarded to E.G. and R.E by the German Aerospace Centre (DLR) (contract # DEM_OTHER2886). Permissions for collecting soil coring and sampling were obtained from the Faculty of Science, Tanta University, Egypt by coauthors Dr. Amr Fhail and Dr. Mohamed Fathy. Bradley Graves at Macquarie University assisted with preparation of the sedimentological figures. Hamada Salama at NRIAG assisted with the GPR field data collection.
Author information
Authors and affiliations.
Department of Earth and Ocean Sciences, University of North Carolina Wilmington, Wilmington, NC, 28403-5944, USA
Eman Ghoneim
School of Natural Sciences, Macquarie University, Macquarie, NSW, 2109, Australia
Timothy J. Ralph
Department of History, The University of Memphis, Memphis, TN, 38152-3450, USA
Suzanne Onstine
Near Eastern Languages and Civilizations, University of Chicago, Chicago, IL, 60637, USA
Raghda El-Behaedi
National Research Institute of Astronomy and Geophysics (NRIAG), Helwan, Cairo, 11421, Egypt
Gad El-Qady, Mahfooz Hafez, Magdy Atya, Mohamed Ebrahim & Ashraf Khozym
Geology Department, Faculty of Science, Tanta University, Tanta, 31527, Egypt
Amr S. Fahil & Mohamed S. Fathy
You can also search for this author in PubMed Google Scholar
Contributions
Eman Ghoneim conceived the ideas, lead the research project, and conducted the data processing and interpretations. The manuscript was written and prepared by Eman Ghoneim. Timothy J. Ralph co-supervised the project, contributed to the geomorphological and sedimentological interpretations, edited the manuscript and the figures. Suzanne Onstine co-supervised the project, contributed to the archeological and historical interpretations, and edited the manuscript. Raghda El-Behaedi contributed to the remote sensing data processing and methodology and edited the manuscript. Gad El-Qady supervised the geophysical survey. Mahfooz Hafez, Magdy Atya, Mohamed Ebrahim, Ashraf Khozym designed, collected, and interpreted the GPR and EMT data. Amr S. Fahil and Mohamed S. Fathy supervised the soil coring, sediment analysis, drafted sedimentological figures and contributed to the interpretations. All authors reviewed the manuscript and participated in the fieldwork.
Corresponding author
Correspondence to Eman Ghoneim .
Ethics declarations
Competing interests.
The authors declare no competing interests.
Peer review
Peer review information.
Communications Earth & Environment thanks Ritambhara Upadhyay and Judith Bunbury for their contribution to the peer review of this work. Primary Handling Editors: Patricia Spellman and Joe Aslin. A peer review file is available.
Additional information
Publisher’s note Springer Nature remains neutral with regard to jurisdictional claims in published maps and institutional affiliations.
Supplementary information
Peer review file, supplementary information file, rights and permissions.
Open Access This article is licensed under a Creative Commons Attribution 4.0 International License, which permits use, sharing, adaptation, distribution and reproduction in any medium or format, as long as you give appropriate credit to the original author(s) and the source, provide a link to the Creative Commons licence, and indicate if changes were made. The images or other third party material in this article are included in the article’s Creative Commons licence, unless indicated otherwise in a credit line to the material. If material is not included in the article’s Creative Commons licence and your intended use is not permitted by statutory regulation or exceeds the permitted use, you will need to obtain permission directly from the copyright holder. To view a copy of this licence, visit http://creativecommons.org/licenses/by/4.0/ .
Reprints and permissions
About this article
Cite this article.
Ghoneim, E., Ralph, T.J., Onstine, S. et al. The Egyptian pyramid chain was built along the now abandoned Ahramat Nile Branch. Commun Earth Environ 5 , 233 (2024). https://doi.org/10.1038/s43247-024-01379-7
Download citation
Received : 06 December 2023
Accepted : 10 April 2024
Published : 16 May 2024
DOI : https://doi.org/10.1038/s43247-024-01379-7
Share this article
Anyone you share the following link with will be able to read this content:
Sorry, a shareable link is not currently available for this article.
Provided by the Springer Nature SharedIt content-sharing initiative
By submitting a comment you agree to abide by our Terms and Community Guidelines . If you find something abusive or that does not comply with our terms or guidelines please flag it as inappropriate.
Quick links
- Explore articles by subject
- Guide to authors
- Editorial policies
Sign up for the Nature Briefing newsletter — what matters in science, free to your inbox daily.


COMMENTS
1 Introduction. Statistics is a set of methods used to analyze data. The statistic is present in all areas of science involving the. collection, handling and sorting of data, given the insight of ...
Introduction. Statistical analysis is necessary for any research project seeking to make quantitative conclusions. The following is a primer for research-based statistical analysis. It is intended to be a high-level overview of appropriate statistical testing, while not diving too deep into any specific methodology.
In this paper, we substantiate our premise that statistics is one of the most important disciplines to provide tools and methods. to find structure in and to give deeper insight into data, and ...
Overview. Statistical Papers is a forum for presentation and critical assessment of statistical methods encouraging the discussion of methodological foundations and potential applications. The Journal stresses statistical methods that have broad applications, giving special attention to those relevant to the economic and social sciences.
Similarly, De scriptive statistics are used to summarize and analyze data in. a variety of academic areas, including psychology, sociology, economics, education, and epidemiology [3 ]. Descriptive ...
Statistics is a branch of mathematics used to summarize, analyze, and interpret a group of numbers or observations. We begin by introducing two general types of statistics: •• Descriptive statistics: statistics that summarize observations. •• Inferential statistics: statistics used to interpret the meaning of descriptive statistics.
In this paper, we substantiate our premise that statistics is one of the most important disciplines to provide tools and methods to find structure in and to give deeper insight into data, and the most important discipline to analyze and quantify uncertainty. We give an overview over different proposed structures of Data Science and address the impact of statistics on such steps as data ...
I. Chapter One - Exploring Your Data. II. Chapter Two - Test Statistics, p Values, Confidence Intervals and Effect Sizes. III. Chapter Three- Comparing Two Group Means. IV. Chapter Four - Comparing Associations Between Two Variables. V. Chapter Five- Comparing Associations Between Multiple Variables. VI.
Read the latest Research articles in Statistics from Scientific Reports
are found. The paper discusses implications for the specification of the skills needed for accessing, filtering, comprehending, and critically evaluating information in these products. Directions for future research and educational practice are outlined. Keywords: Statistics education research; Statistical literacy; Official statistics;
usability and reach of social media platforms. For instance, a report by 2015 Pew research informs that there was a 7% rise in the usage of social media from 2005 to 2015. The report informs that 65% adults use social media (Perrin, 2015). As social media evolves into a more
Bureau of Labor Statistics (BLS) to track unemployment rates, and have been used in previous research to study determinants of business ownership (e.g. recently, Levine and Rubenstein 2017, Wang 2019, Fairlie and Fossen 2019). The data allow for an analysis of recent trends in
Table of contents. Step 1: Write your hypotheses and plan your research design. Step 2: Collect data from a sample. Step 3: Summarize your data with descriptive statistics. Step 4: Test hypotheses or make estimates with inferential statistics.
These demonstrated an interesting diversity in research methods, theoretical approaches, and points of view. As a result of the success of this gathering, plans are already underway for the next gathering (SRTL-6) in 2009. The research forum proved to be very productive in many ways.
Research Papers / Publications. Xinmeng Huang, Shuo Li, Mengxin Yu, Matteo Sesia, Seyed Hamed Hassani, Insup Lee, Osbert Bastani, Edgar Dobriban, Uncertainty in Language Models: Assessment through Rank-Calibration. Patrick Chao, Edoardo Debenedetti, Alexander Robey, Maksym Andriushchenko, Francesco Croce, Vikash Sehwag, Edgar Dobriban, Nicolas ...
important writing you will do for the paper. IMHO your reader will either be interested and continuing on with your paper, or... A scholarly introduction is respectful of the literature. In my experience, the introduction is part of a paper that I will outline relatively early in the process, but will nish and repeatedly edit at the end of the ...
Only a few of the most influential papers on the field of statistics are included on our list. through papers in statistics'. Four of our most cited papers, Duncan (1955), Kramer. (1956), and ...
The length of the textbook appears to be more than adequate for a one-semester course in Introduction to Statistics. As I no longer teach a full statistics course but simply a few lectures as part of our Research Curriculum, I am recommending this book to my students as a good reference. Especially as it is available on-line and in Open Access.
Research Methods and Statistics in Psychology Second edition Research Methods and Statistics in Psychology provides a seamless introduction to statistics and research in psychology, identifying various research areas and analyzing how one can approach them statistically. The text provides a solid empirical foundation for undergraduate Psychology
in research methods and statistics during his PhD program at Ohio State University. He currently teaches four courses in research methods and statistics. His research involves leadership, occupational health, and motivation, as well as issues related to research methods such as the following article: "Safeguarding Access and Safeguarding
The Statistical Research Report Series (RR) covers research in statistical methodology and estimation. Facebook. X (Twitter) Page Last Revised - October 8, 2021. View Statistical Research reports by their topics.
Here, we combine observed and reconstructed June-August (JJA) surface air temperatures to show that 2023 was the warmest NH extra-tropical summer over the past 2000 years exceeding the 95% ...
1.2 Statistics Project Topics for High School Students. 1.3 Statistical Survey Topics. 1.4 Statistical Experiment Ideas. 1.5 Easy Stats Project Ideas. 1.6 Business Ideas for Statistics Project. 1.7 Socio-Economic Easy Statistics Project Ideas. 1.8 Experiment Ideas for Statistics and Analysis. 2 Conclusion: Navigating the World of Data Through ...
The paper shows that the receipt of in-kind benefits, primarily education, is what drives which groups that receive the largest net benefits from the fiscal system. The results also show that the fiscal system in Jordan is reducing within-group inequalities, which represent over 80 percent of total inequality for both fiscal and care groups.
the reader will understand the way a research project is carried out both. practically and theoretically. Therefore, this book is a clear and simpli ed. valuable document for the nal year students ...
A white paper is a document used by business professionals to share in-depth information about a specific topic. For example, you can use a white paper to share marketing statistics, compare different campaigns, present a complex analysis of an industry trend, or share an in-depth explanation of a specific process carried out by a team or company.
Eman Ghoneim conceived the ideas, lead the research project, and conducted the data processing and interpretations. The manuscript was written and prepared by Eman Ghoneim.
In this paper, we present OneLLM, an MLLM that aligns eight modalities to language using a unified framework. We achieve this through a unified multimodal encoder and a progressive multimodal alignment pipeline. In detail, we first train an image projection module to connect a vi-sion encoder with LLM. Then, we build a universal pro-
The function of statistics in research is to purpose as a tool in conniving research, analyzing its data and portrayal of conclusions. there from. Most research studies result in a extensive ...
of Houston to bring you the White Paper Series. This series is a collaboration of research reports examining pertinent topics throughout the energy sector and aims to provide leaders from industry, nonprofits and regulatory agencies with information they need to navigate the changing energy landscape.