Thank you for visiting nature.com. You are using a browser version with limited support for CSS. To obtain the best experience, we recommend you use a more up to date browser (or turn off compatibility mode in Internet Explorer). In the meantime, to ensure continued support, we are displaying the site without styles and JavaScript.
- View all journals
- My Account Login
- Explore content
- About the journal
- Publish with us
- Sign up for alerts
- Review Article
- Open access
- Published: 11 January 2022

Effect of sleep and mood on academic performance—at interface of physiology, psychology, and education
- Kosha J. Mehta ORCID: orcid.org/0000-0002-0716-5081 1
Humanities and Social Sciences Communications volume 9 , Article number: 16 ( 2022 ) Cite this article
67k Accesses
11 Citations
39 Altmetric
Metrics details
Academic achievement and cognitive functions are influenced by sleep and mood/emotion. In addition, several other factors affect learning. A coherent overview of the resultant interrelationships is essential but has not been presented till date. This unique and interdisciplinary review sits at the interface of physiology, psychology, and education. It compiles and critically examines the effects of sleep and mood on cognition and academic performance while including relevant conflicting observations. Moreover, it discusses the impact of several regulatory factors on learning, namely, age, gender, diet, hydration level, obesity, sex hormones, daytime nap, circadian rhythm, and genetics. Core physiological mechanisms that mediate the effects of these factors are described briefly and simplistically. The bidirectional relationship between sleep and mood is addressed. Contextual pictorial models that hypothesise learning on an emotion scale and emotion on a learning scale have been proposed. Essentially, convoluted associations between physiological and psychological factors, including sleep and mood that determine academic performance are recognised and affirmed. The emerged picture reveals far more complexity than perceived. It questions the currently adopted ‘one-size fits all’ approach in education and urges to envisage formulating bespoke strategies to optimise teaching-learning approaches while retaining uniformity in education. The information presented here can help improvise education strategies and provide better academic and pastoral support to students during their academic journey.
Similar content being viewed by others
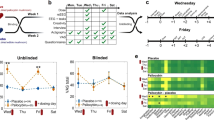
Microdosing with psilocybin mushrooms: a double-blind placebo-controlled study
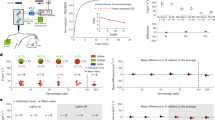
Brain clearance is reduced during sleep and anesthesia
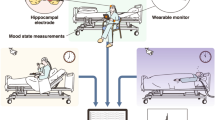
Hippocampal sharp-wave ripples correlate with periods of naturally occurring self-generated thoughts in humans
Introduction.
Academic performance and cognitive activities like learning are influenced by sleep and mood or emotion. This review discusses the roles of sleep and mood/emotion in learning and academic performance.
Sleep, mood, and emotion: definitions and descriptions
Sleep duration refers to “total amount of sleep obtained, either during the nocturnal sleep episode or across the 24-hour period” (Kline, 2013a ). Sleep quality is defined as “one’s satisfaction of the sleep experience, integrating aspects of sleep initiation, sleep maintenance, sleep quantity, and refreshment upon awakening” (Kline, 2013b ). Along similar lines, it is thought to be “one’s perception that they fall asleep easily, get sufficient duration so as to wake up feeling rested, and can make it through their day without experiencing excessive daytime sleepiness” (Štefan et al., 2018 ). Sleep disturbance includes disorders of initiating and maintaining sleep (insomnias) and sleep–wake schedule, as well as dysfunctions associated with either sleep or stages of sleep or partial arousals (Cormier, 1990 ). Sleep deprivation is a term used loosely to describe a lack of appropriate/sufficient amount of sleep (Levesque, 2018 ). It is “abnormal sleep that can be described in measures of deficient sleep quantity, structure and/or sleep quality” (Banfi et al., 2019 ). In a study, sleep deprivation was defined as a sleep duration of 6 h or less (Roberts and Duong, 2014 ). Sleep disorder overarches disorders related to sleep. It has many classifications (B. Zhu et al., 2018 ). Sleep disorders or sleep-related problems include insomnia, hypersomnia, obstructive sleep apnoea, restless legs and periodic limb movement disorders, and circadian rhythm sleep disorders (Hershner and Chervin, 2014 ).
Mood is a pervasive and sustained feeling that is felt internally and affects all aspects of an individual’s behaviour (Sekhon and Gupta, 2021 ). However, by another definition, it is believed to be transient. It is low-intensity, nonspecific, and an affective state. Affective state is an overarching term that includes both emotions and moods. In addition to transient affective states of daily life, mood includes low-energy/activation states like fatigue or serenity (Kleinstäuber, 2013 ). Yet another definition of mood refers to mood as feelings that vary in intensity and duration, and that usually involves more than one emotion (Quartiroli et al., 2017 ). According to the American Psychological Association, mood is “any short-lived emotional state, usually of low intensity” and which lacks stimuli, whereas emotion is a “complex reaction pattern, involving experiential, behavioural and physiological elements”. Emotion is a certain level of pleasure or displeasure (X. Liu et al., 2018 ). It is “a response to external stimuli and internal mental representations” (L. Zhang et al., 2021 ). It is “a conscious mental reaction (such as anger or fear) which is subjectively experienced as a strong feeling usually deriving from one’s circumstances, mood, or relationships with others”. “This feeling is typically accompanied by physiological and behavioural changes in the body”. “This mental state is an instinctive or intuitive feeling which arises spontaneously as distinguished from reasoning or knowledge” (Thibaut, 2015 ).
Since there is some overlap between the descriptions of mood and emotion, in the context of the core content of this review, here, mood and emotion have not been differentiated based on their theoretical/psychological definitions. This is because the aim of the review is not to distinguish between the effects of mood and emotion on learning. Thus, these have been referred to as general affective states; essentially specific states of mind that affect learning. Also, these have been addressed in the context of the study being discussed and cited in that specific place in the review.
Rationale for the topic
Sleep is essential for normal physiological functionality. The panel of National Sleep Foundation suggests sleep durations for various age groups and agrees that the appropriate sleep duration for young adults and adults would be 7–9 hours, and for older adults would be 7–8 hours (Hirshkowitz et al., 2015 ). Today, people sleep for 1–2 hours less than that around 50–100 years ago (Roenneberg, 2013 ). Millions of adults frequently get insufficient sleep (Vecsey et al., 2009 ), including college and university students who often report poor and/or insufficient sleep (Bahammam et al., 2012 ; Curcio et al., 2006 ; Hershner and Chervin, 2014 ). During the COVID-19 pandemic, sleep problems have been highly prevalent in the general population (Gualano et al., 2020 ; Jahrami et al., 2021 ; Janati Idrissi et al., 2020 ) and the student community (Marelli et al., 2020 ). Poor and insufficient sleep is a public health issue because it increases the risk of developing chronic pathologies, and imparts negative social and economic outcomes (Hafner et al., 2017 ).
Like sleep, mood and emotions determine our physical and mental health. Depressive disorders have prevailed as one of the leading causes of health loss for nearly 30 years (James et al., 2018 ). Increased incidence of mood disorders amongst the general population has been observed (Walker et al., 2020 ), and there is an increase in such disorders amongst students (Auerbach et al., 2018 ). These have further risen during the COVID-19 pandemic (Son et al., 2020 ; Wang et al., 2020 ).
The relationship between sleep, mood and cognition/learning is far more complex than perceived. Therefore, this review aims to recognise the interrelationships between the aforementioned trio. It critically examines the effects of sleep and mood on cognition, learning and academic performance (Fig. 1 ). Furthermore, it discusses how various regulatory factors can directly or indirectly influence cognition and learning. Factors discussed here are age, gender, diet, hydration level, obesity, sex hormones, daytime nap, circadian rhythm, and genetics (Fig. 1 ). The effect of sleep and mood on each other is also addressed. Pictorial models that hypothesise learning on an emotion scale and vice-versa have been proposed.
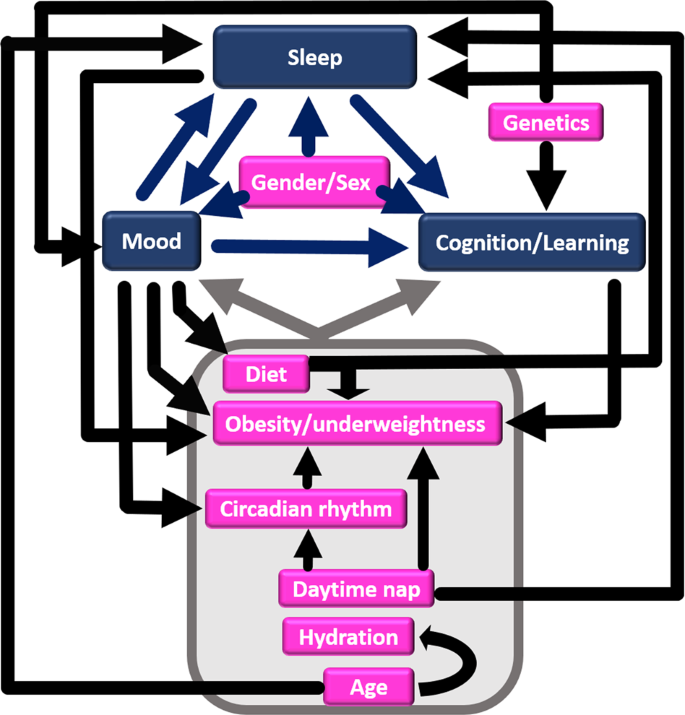
Sleep and mood/emotion affect cognition and academic achievement. Their effects can be additionally influenced by other factors like diet, metabolic disorders (e.g., obesity), circadian rhythm, daytime nap, hydration level, age, gender, and genetics. The figure presents the interrelationships and highlights the complexity emerging from the interdependence between factors, action of multiple factors on a single factor or vice-versa and the bidirectional nature of some associations. These associations collectively determine learning and thereby, academic achievement. Direction of the arrow represents effect of a factor on another.
Effect of sleep on cognition and academic performance
Adequate sleep positively affects memory, learning, acquisition of skills and knowledge extraction (Fenn et al., 2003 ; Friedrich et al., 2020 ; Huber et al., 2004 ; Schönauer et al., 2017 ; Wagner et al., 2004 ). It allows the recall of previously gained knowledge despite the acquisition of new information and memories (Norman, 2006 ). Sleeping after learning acquisition regardless of the time of the day is thought to be beneficial for memory consolidation and performance (Hagewoud et al., 2010 ). Therefore, unperturbed sleep is essential for maintaining learning efficiency (Fattinger et al., 2017 ).
Sleep quality and quantity are strongly associated with academic achievement in college students (Curcio et al., 2006 ; Okano et al., 2019 ). Sufficient sleep positively affects grade point average, which is an indicator of academic performance (Abdulghani et al., 2012 ; Hershner and Chervin, 2014 ) and supports cognitive functionality in school-aged children (Gruber et al., 2010 ). As expected, insufficient sleep is associated with poor performance in school, college and university students (Bahammam et al., 2012 ; Hayley et al., 2017 ; Hedin et al., 2020 ; Kayaba et al., 2020 ; Perez-Chada et al., 2007 ; Shochat et al., 2014 ; Suardiaz-Muro et al., 2020 ; Taras and Potts-Datema, 2005 ). In adolescents aged 14–18 years, not only did sleep quality affect academic performance (Adelantado-Renau, Jiménez-Pavón, et al., 2019 ) but one night of total sleep deprivation negatively affected neurobehavioral performance-attention, reaction time and speed of cognitive processing, thereby putting them at risk of poor academic performance (Louca and Short, 2014 ). In university students aged 18–25 years, poor sleep quality has been strongly associated with daytime dysfunctionality (Assaad et al., 2014 ). Medical students tend to show poor sleep quality and quantity. In these students, not sleep duration but sleep quality has been shown to correlate with academic scores (Seoane et al., 2020 ; Toscano-Hermoso et al., 2020 ). Students may go through repeated cycles wherein the poor quality of sleep could lead to poor performance, which in turn may again lead to poor quality of sleep (Ahrberg et al., 2012 ). Sleep deprivation in surgical residents tends to decrease procedural skills, while in non-surgical residents it diminishes interpretational ability and performance (Veasey et al., 2002 ).
Such effects of sleep deprivation are obvious because it can impair procedural and declarative learning (Curcio et al., 2006 ; Kurniawan et al., 2016 ), decrease alertness (Alexandre et al., 2017 ), and impair memory consolidation (Hagewoud et al., 2010 ), attention and decision making (Alhola and Polo-Kantola, 2007 ). It can increase low-grade systemic inflammation and hinder cognitive functionality (Choshen-Hillel et al., 2020 ). Hippocampus is the region in the brain that plays the main role in learning, memory, social cognition, and emotion regulation (Y. Zhu et al., 2019 ). cAMP signalling plays an important role in several neural processes such as learning and memory, cellular excitability, motor function and pain (Lee, 2015 ). A brief 5-hour period of sleep deprivation interferes with cAMP signalling in the hippocampus and impairs its function (Vecsey et al., 2009 ). Thus, optimal academic performance is hindered, if there is a sleep disorder (Hershner and Chervin, 2014 ).
Caveats to affirming the impact of sleep on cognition and academic performance
Despite the clear significance of appropriate sleep quality and quantity in cognitive processes, there are some caveats to drawing definitive conclusions in certain areas. First, there are uncertainties around how much sleep is optimal and how to measure sleep quality. This is further confounded by the dependence of sleep quality and quantity on various genetic and environmental factors (Roenneberg, 2013 ). Moreover, although sleep enhances emotional memory, during laboratory investigations, this effect has been observed only under specific experimental conditions. Also, the experiments conducted have differed in the methods used and in considering parameters like timing and duration of sleep, age, gender and outcome measure (Lipinska et al., 2019 ). This orientates conclusions to be specific to those experimental conditions and prevents the formation of generic opinions that would be applicable to all circumstances.
Furthermore, some studies on the effects of sleep on learning and cognitive functions have shown either inconclusive or apparently unexpected results. For example, in a study, although college students at risk for sleeping disorders were thought to be at risk for academic failure, this association remained unclear (Gaultney, 2010 ). Other studies showed that the effect of sleep quality and duration on academic performance was trivial (Dewald et al., 2010 ) and did not significantly correlate with academic performance (Johnston et al., 2010 ; Sweileh et al., 2011 ). In yet another example, despite the reduction in sleep hours during stressful periods, pharmacy students did not show adversely affected academic performance (Mnatzaganian et al., 2020 ). Also, the premise underlining the significance of sleep hours in enhancing the performance of clinical duties was challenged when the average daily sleep did not affect burnout in clinical residents, where the optimal sleep hours that would maximise learning and improve performance remained unknown (Mendelsohn et al., 2019 ). In some other examples, poor sleep quality was associated with stress but not with academic performance that was measured as grade point average (Alotaibi et al., 2020 ), showed no significant impact on academic scores (Javaid et al., 2020 ) and there was no significant difference between high-grade and low-grade achievers based on sleep quality (Jalali et al., 2020 ). Insomnia reflects regularly experienced sleeping problems. Strangely, in adults aged 40–69 years, those with frequent insomnia showed slightly better cognitive performance than others (Kyle et al., 2017 ).
The reason for such inconclusive and unanticipated results could be that sleep is not the sole determinant of learning. Learning is affected by various other factors that may alter, exacerbate, or surpass the influence of sleep on learning (Fig. 1 ). These factors have been discussed in the subsequent sections.
Effect of mood/emotion on cognition and learning
Emotions reflect a certain level of pleasure or displeasure (X. Liu et al., 2018 ). Panksepp described seven basic types of emotions, whereby lust, seeking, play and care are positive emotions whereas anger, fear and sadness are negative emotions (Davis and Montag, 2019 ). Emotions influence all cognitive functions including memory, focus, problem-solving and reasoning (Tyng et al., 2017 ). Positive emotions such as hope, joy and pride positively correlate with students’ academic interest, effort and achievement (Valiente et al., 2012 ) and portend a flexible brain network that facilitates cognitive flexibility and learning (Betzel et al., 2017 ).
Mood deficit often precedes learning impairment (LeGates et al., 2012 ). In a study by Miller et al. ( 2018 ), the negative mood is referred to as negative emotional induction, as was achieved by watching six horror films by the subjects in that study. Other examples of negative emotions given by the authors were anxiety and shame. Negative mood can unfavourably affect the learning of an unfamiliar language by suppressing the processing of native language that would otherwise help make connections, thereby reiterating the link between emotions and cognitive processing (Miller et al., 2018 ). Likewise, worry and anxiety affect decision-making. High level of worry is associated with poor task performance and decreased foresight during decision-making (Worthy et al., 2014 ). State anxiety reflects a current mood state and trait anxiety reflects a stable personality trait. Both are associated with an increased tendency of “more negative or more threatening interpretation of ambiguous information”, as can be the case in clinically depressed individuals (Bisson and Sears, 2007 ). This could explain why some people who show the symptoms of depression and anxiety may complain of confusion and show an inability to focus and use cognition skills to appraise contextual clues. Patients with major depressive disorder have scored lower on working and verbal memory, motor speed and attention than healthy participants (Hidese et al., 2018 ). Similarly, apathy, anxiety, depression, and mood disorders in stroke patients can adversely affect the functional recovery of patients’ cognitive functions (Hama et al., 2020 ). These examples collectively present a positive correlation between good mood and cognitive processes.
Caveats to affirming the impact of mood/emotion on cognition and academic performance
Based on the examples and discussion so far, a direct relationship between emotions and learning could be hypothesised, whereby positive emotions would promote creative learning strategies and academic success, whereas negative emotions would lead to cognitive impairment (Fig. 2a ). However, this relationship is far more complex and different than perceived.
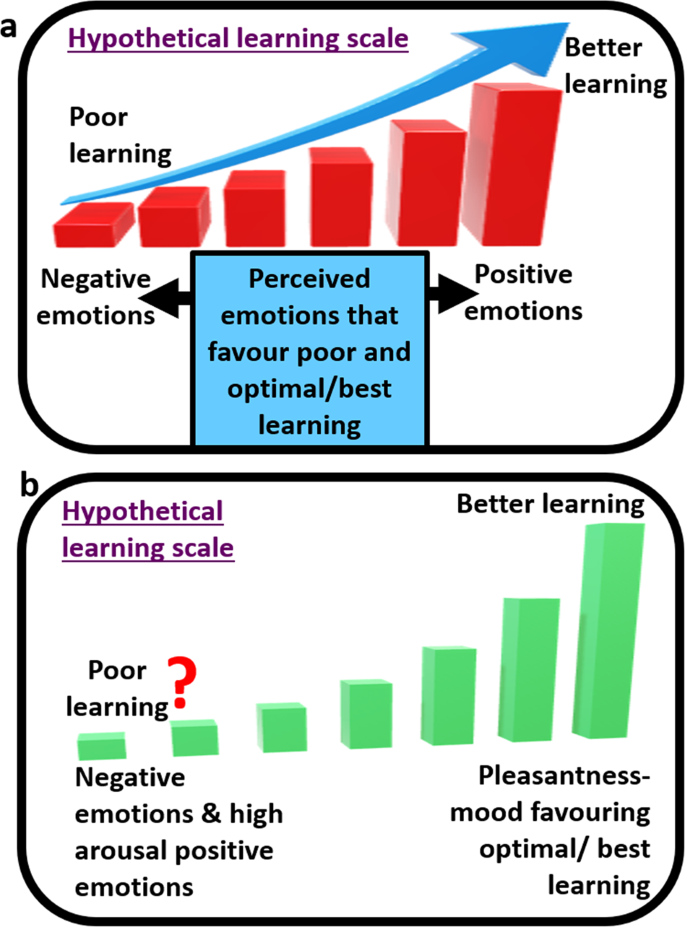
Emotions have been shown on a hypothetical learning scale. a Usually, positive and negative emotions are perceived to match with optimal and poor learning, respectively. b Emotions that lead to sub-optimal/poor and optimal/better learning have been shown on the hypothetical learning scale. Here, distinct from ( a ), both negative emotions and high arousal positive emotions have been implicated in poorer learning compared with low-intensity positive emotion like pleasantness; the latter is believed to lead to optimal learning. The question mark reflects that some negative emotions like shame might stimulate learning, but the exact intensity of such emotions and whether these would facilitate better or worse learning than high arousal positive emotions or pleasantness need to be investigated.
Although positive mood favours the recall of learnt words, it correlates with increased distraction and poor planning (Martin and Kerns, 2011 ). High levels of positive emotions like excitedness and elatedness may decrease achievement (Fig. 2b ) (Valiente et al., 2012 ). It may be surprising to know that negative emotions such as shame and anxiety can arouse cognitive activity (Miller et al., 2018 ). Along similar lines, it has been observed that participants exposed to sad and neutral moods performed similarly in visual statistical (learning) tasks but those who experienced sad stimuli showed high conscious access to the acquired statistical knowledge (Bertels et al., 2013 ). Dysphoria is a state of dissatisfaction that may be accompanied by anxiety and depression. Participants with dysphoria have shown more sensitivity to temporal shifts in outcome contingencies than those without dysphoria (Msetfi et al., 2012 ), and these participants reiterated the depressive realism effect and were quicker in endorsing the connection between negative words and ambiguous statements, demonstrating a negative bias (Hindash and Amir, 2012 ). Likewise, not the positive emotion but negative emotion has been shown to influence the learning outcomes, and it increased the efficiency and precision of learning morphosyntactic instructions involving morphology and syntax of a foreign language (X. Liu et al., 2018 ). Thus, negative emotions can allow, and at times, stimulate or facilitate learning (Figs. 2 and 3 ). Further investigation is needed on the intensity of these emotions, whether these would facilitate better or worse learning than high-intensity positive emotions and whether the results would be task specific.
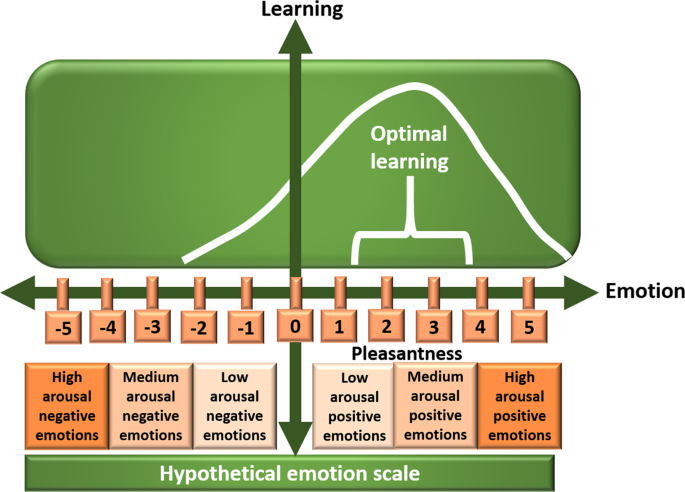
The figure depicts that low-to-medium intensity positive emotion like pleasantness leads to optimal learning, whereas high-intensity emotions, either positive or negative, may lead to suboptimal or comparatively poorer learning. The model considers the apparently unexpected data that negative emotions may stimulate learning. However, which negative emotions these would be, their intensities and their corresponding level of learning are not known, and so these are not shown in the figure. Also, the figure shows bias towards positive emotions in mediating optimal learning. This information is based on the literature so far. Note that the figure represents concepts only and is not prescriptive. It shows inequality and differences between the impacts of high arousal positive and high arousal negative emotions. This concept needs to be investigated. Therefore, the figure may/may not be an accurate mathematical representation of learning with regards to the intensities of positive and negative emotions. In actuality, the scaling and intensities of emotions on the negative and positive sides of the scale may not be equal, particularly in reference to the position of optimal learning on the scale. Furthermore, upon plotting the 3rd dimension, which could be one or more of the regulatory factors discussed here might alter the position and shape of the optimal learning peak.
Moreover, the intensity of positive emotions does not show direct mathematical proportionality to learning/achievement. In other words, the concept of ‘higher the intensity of positive emotions, higher the achievement’ is not applicable. Low-intensity positive emotions such as satisfaction and relaxedness may be potentially dysregulating and high-intensity positive emotions may hamper achievement (Figs. 2b and 3 ). Optimal achievement is likely to be associated with low to medium level intensity of positive emotions like pleasantness (Valiente et al., 2012 ) (Fig. 3 ). Therefore, it has been proposed that both positive and negative high arousal emotions impair cognitive ability (Figs. 2b and 3 ) whereas low-arousal emotions could enhance behavioural performance (Miller et al., 2018 ).
Interestingly, some studies have indicated that emotions do not play a significant role in context. For example, a study showed that there was no evidence that negative emotions in depressed participants showed negative interpretations of ambiguous information (Bisson and Sears, 2007 ). In another study, improvements in visuomotor skills happened regardless of perturbation or mood states (Kaida et al., 2017 ). Thus, mood can either impair, enhance or have no effect on cognition. The effect of mood on cognition and learning can be variable and depend on the complexity of the task (Martin and Kerns, 2011 ) and/or other factors. Some of these factors have been discussed in the following section. The discrepancies in the data on the effects of mood on cognition and learning may be partly attributed to the influence of these factors on cognitive functions.
Factors affecting cognition and its relationships with sleep and mood/emotion
The relationship of cognition with sleep and mood is confounded by the influence of various factors (Tyng et al., 2017 ) such as diet, hydration level, metabolic disorders (e.g., obesity), sex hormones and gender, sleep, circadian rhythm, age and genetics (Fig. 1 ). These factors and their relationships with learning are discussed in this section.
A healthy diet is defined as eating many servings per day of fruits and vegetables, while maintaining a critical view of the consumption of saturated fat, sugar and salt (Healthy Diet—an Overview|ScienceDirect Topics, n.d.). It is also about adhering to two or more of the three healthy attributes with regards to food intake, namely, sufficiently low meat, high fish and high fruits and vegetables (Sarris et al., 2020 ). Another definition of a healthy diet is the total score of the healthy eating index >51 (Zhao et al., 2021 ).
The association between an unhealthy diet and the development of metabolic disorders has been long established. In addition, food affects both cognition and emotion (Fig. 1 ) (Spencer et al., 2017 ). Food and mood show a bidirectional relation whereby food affects mood and mood affects the choice of food made by the individual. Alongside, poor diet can lead to depression while a healthy diet reduces the risk of depression (Francis et al., 2019 ). A high-fat diet stimulates the hippocampus to initiate neuroinflammatory responses to minor immune challenges and this causes memory loss. Likewise, low intake of omega-3 polyunsaturated fatty acids can affect endocannabinoid and inflammatory pathways in the brain causing microglial phagocytosis, i.e., engulfment of synapses by the brain microglia in the hippocampus, eventually leading to memory deficits and depression. On the other hand, vegetables and fruits rich in polyphenolics can lower oxidative stress and inflammation, and thereby avert and/or reverse age-related cognitive dysfunctionality (Spencer et al., 2017 ). Fruits and vegetables, fish, eggs, nuts, and dairy products found in the Mediterranean diet can reduce the risk of developing depression and promote better mental health than sugar-sweetened beverages and high-fat food found in Western diets. Consumption of dietary antioxidants such as the polyphenols in green tea has shown a negative correlation with depression-like symptoms (Firth et al., 2020 ; Huang et al., 2019 ; Knüppel et al., 2017 ). Likewise, chocolate or its components have been found to reduce negative mood or enhance mood, and also enhance or alter cognitive functions temporarily (Scholey and Owen, 2013 ). Alcohol consumption is prevalent amongst university students including those who report feelings of sadness and hopelessness (Htet et al., 2020 ). It can lead to poor academic performance, hamper tasks that require a high degree of cognitive control, dampen emotional responsiveness, impair emotional processing, and generally cause emotional dysregulation (Euser and Franken, 2012 ). Further details on the effects of diet on mood have been discussed elsewhere (Singh, 2014 ). Diet also affects sleep (Binks et al., 2020 ), which in turn affects learning and academic performance. Thus, diet is linked with sleep, mood, and brain functionality (Fig. 1 ).
Water is a critical nutrient accounting for about 3/4th of the brain mass (N. Zhang et al., 2019 ). Unlike the previously thought deficit of 2% or more in body water levels, loss of about 1–2% can be detrimental and hinder normal cognitive functionality (Riebl and Davy, 2013 ). Thus, mild dehydration can disrupt cognitive functions and mood; particularly applicable to the very old, the very young and those living in hot climatic conditions or those exercising rigorously. Dehydration diminishes alertness, concentration, short-term memory, arithmetic ability, psychomotor skills and visuomotor tracking. This is possibly due to the dehydration-induced physiological stress which competes with cognitive processes. In children, voluntary water intake has been shown to improve visual attention, enhance memory performance (Popkin et al., 2010 ) and generally improve memory and attention (Benton, 2011 ). In adults, dehydration can elevate anger, fatigue and depression and impair short-term memory and attention, while rehydration can alleviate or significantly improve these parameters (Popkin et al., 2010 ; N. Zhang et al., 2019 ). Thus, dehydration causes alterations in cognition and emotions, thereby showcasing the impact of hydration levels on both learning and emotional status (Fig. 1 ).
Interestingly, when older persons are deprived of water, they are less thirsty and less likely to drink water than water-deprived younger persons. This can be due to the defective functionality of baroreceptors, osmoreceptors and opioid receptors that alter thirst regulation with aging (Popkin et al., 2010 ). Since water is essential for the maintenance of memory and cognitive performance, the decline of cognitive functionality in the elderly could be partly attributed to their lack of sufficient fluid/water intake when dehydrated.
Obesity and underweightness
Normal weight is defined as a body mass index between 18.5 and 25 kg/m 2 (McGee and Diverse Populations Collaboration, 2005 ) or between 22 and 26.99 kg/m 2 (Nösslinger et al., 2021 ). Being underweight reflects rapid weight loss or an inability to increase body mass and is defined through grades (1–3) of thinness. In children, these are associated with poor academic performance in reading and writing skills, and mathematics (Haywood and Pienaar, 2021 ). Basically, underweight children may have health issues and this could affect their academic abilities (Zavodny, 2013 ). Also, malnourished children tend to show low school attendance and may show poor concentration and impaired motor functioning and problem-solving skills that could collectively lead to poor academic performance at school (Haywood and Pienaar, 2021 ). Malnourished children can show poor performance on cognitive tasks that require executive function. Executive functions could be impaired in overweight children too and this may lead to poor academic performance (Ishihara et al., 2020 ). The negative relation between overweightness and academic performance also implies that the reverse may be true. Poor academic outcome may cause children to overeat and reduce exercise or play and this could lead to them being overweight (Zavodny, 2013 ).
The influence of weight on academic performance is reiterated in observations that in children independent of socioeconomic and other factors, weight loss in overweight/obese children and weight gain in underweight children positively influenced their academic performance (Ishihara et al., 2020 ). Interestingly, independent of the BMI classification, perceptions of underweight and overweight can predict poorer academic performance. In youth, not only larger body sizes but perceptions about deviating from the “correct weight” can impede academic success. This clearly indicates an impact of overweight and underweight perceptions on the emotional and physical health of adolescents (Fig. 1 ) (Livermore et al., 2020 ).
Cognitive and mood disorders are common co-morbidities associated with obesity. Compared to people with normal weight, obese individuals frequently show some dysfunction in learning, memory, and other executive functions. This has been partly attributed to an unhealthy diet, which causes a drift in the gut microbiota. In turn, the obesity-associated microbiota contributes to obesity-related complications including neurochemical, endocrine and inflammatory changes underlying obesity and its comorbidities (Agustí et al., 2018 ). The exacerbated inflammation in obesity may impair the functionality of the region in the brain that is associated with learning, memory, and mood regulation (Castanon et al., 2015 ).
Obesity and mood appear to have a reciprocal relationship whereby obesity is highly prevalent amongst individuals with major depressive disorder and obese individuals are at a high risk of developing anxiety, depression and cognitive malfunction (Restivo et al., 2017 ). In patients with major depressive disorder, obesity has been associated with reduced cognitive functions, likely due to the reduction in grey matter and impaired integrity of white matter in the brain, particularly in areas related to cognition (Hidese et al., 2018 ). Obesity has been shown to be a predictor of depression and the two are linked via psychobiological mechanisms (LaGrotte et al., 2016 ). Notably, sleep deprivation increases the risk of obesity (Beccuti and Pannain, 2011 ) and sleep helps evade obesity (Pearson, 2006 ). Collectively, this links cognition and academic achievement with sleep, obesity, and mood.
Sex hormones and gender
According to the Office of National Statistics, the UK government defines sex as that assigned at birth and which is generally male or female, whereas gender is where an individual may see themselves as having no gender or non-binary gender or on a spectrum between man and woman. The following section discusses both sex and gender in context, as addressed within the cited studies.
Studies show that females outperform males in most academic subjects (Okano et al., 2019 ) and show more sustained performance in tests than male peers (Balart and Oosterveen, 2019 ). This indicates that biological sex may play a role in academic performance. The hormone oestrogen helps develop and maintain female characteristics and the reproductive system. Oestrogen also affects hippocampal neurogenesis, which involves neural stem cells proliferation and survival, and this contributes to memory retention and cognitive processing. Generally, on average, females show higher levels of oestrogen than males. This may partly explain the observed sex-based differences in academic achievement. Administration of oestrogen in females has been proposed to positively affect cognitive behaviour as well as depressive-like and anxiety-like behaviours (Hiroi et al., 2016 ). Clinical trials can establish whether there are any sex-based differences in cognition following oestrogen administration in males and females.
Progesterone, the hormone released by ovaries in females is also produced by males to synthesise testosterone. It affects some non-reproduction functions in the central nervous system in both males and females such as neural circuits formation, and regulates memory, learning and mood (González-Orozco and Camacho-Arroyo, 2019 ). The menstrual cycle in females shows alterations in oestrogen and progesterone levels and is broadly divided into early follicular, mid ovulation and late luteal phase. It is believed that the low-oestrogen-low-progesterone early follicular phase relates to better spatial abilities and “male favouring” cognitive abilities, whereas the high-oestrogen-high-progesterone late follicular or mid-luteal phases relate to verbal fluency, memory and other “female favouring” cognitive abilities (Sundström Poromaa and Gingnell, 2014 ). Thus, sex-hormone derivatives (salivary oestrogen and salivary progesterone) can be used as predictors of cognitive behaviour (McNamara et al., 2014 ). These ovarian hormones decline with menopause, which may affect cognitive and somatosensory functions. However, ovariectomy of rats, which depleted ovarian hormones, caused depression-like behaviour in rats but did not affect spatial performance (Li et al., 2014 ). While this suggests a positive effect of these hormones on mood, it questions their function in cognition and proposes activity-specific functions, which need to be investigated.
Serotonin is a neurotransmitter. Reduced serotonin is correlated with cognitive dysfunctions. Tryptophan hydroxylase-2 is the rate-limiting enzyme in serotonin synthesis. Polymorphisms of this enzyme have been implicated in cognitive disorders. Women have a lower rate of serotonin synthesis and are more susceptible to such dysfunctions than men (Hiroi et al., 2016 ; Nishizawa et al., 1997 ), implying a greater impact of serotonin reduction on cognitive functions in women than in men. Central serotonin also helps to maintain the feeling of happiness and wellbeing, regulates behaviour, and suppresses appetite, thereby modulating nutrient intake. Additionally, it has the ability to promote the wake state and inhibit rapid eye movement sleep (Arnaldi et al., 2015 ; Yabut et al., 2019 ). Thus, any sex-based differences in serotonin levels may affect cognitive functions directly or indirectly via the aforementioned parameters.
Interestingly, data on the relationship between sex and sleep have been ambiguous. While in one study, female students at a university showed less sleep difficulties than male peers (Assaad et al., 2014 ), other studies showed that female students were at a higher risk of presenting sleep disorders related to nightmares (Toscano-Hermoso et al., 2020 ) and insomnia was significantly associated with the risk of poor academic performance only in females (Marta et al., 2020 ). Collectively, sex and gender may influence learning directly, or indirectly by affecting sleep and mood; the other two factors that affect cognitive functions (Fig. 1 ).
Circadian rhythm
Circadian rhythm is a biological phenomenon that lasts for ~24 hours and regulates various physiological processes in the body including the sleep–wake cycles. Circadian rhythm is linked with memory formation, learning (Gerstner and Yin, 2010 ), light, mood and brain circuits (Bedrosian and Nelson, 2017 ). We use light to distinguish between day and night. Interestingly, light stimulates the expression of microRNA-132, which is the sole known microRNA involved in photic regulation of circadian clock in mammals (Teodori and Albertini, 2019 ). The photosensitive retinal ganglions that express melanopsin in eyes not only orchestrate the circadian rhythm with the external light-dark cycle but also influence the impact of light on mood, learning and overall health (Patterson et al., 2020 ). For example, we frequently experience depression-like feelings during the dark winter months and pleasantness during bright summer months. This can be attributed to the circadian regulation of neural systems such as the limbic system, hypothalamic–pituitary–adrenal axis, and monoamine neurotransmitters. Mistimed light in the night disturbs our biological judgement leading to a negative impact on health and mood. Thus, increased incidence of mood disorders correlates with disruption of the circadian rhythm (Walker et al., 2020 ). Interestingly, a study involving university students showed the significance of short-wavelength light, specifically, blue-enriched LED light in reducing melatonin levels [best circadian marker rhythm (Arendt, 2019 )], and improved the perception of mood and alertness (Choi et al., 2019 ). While these examples depict the effect of circadian rhythm on mood, the reverse is also true. Individuals who demonstrate depression show altered circadian rhythm and disturbances in sleep (Fig. 1 ) (Germain and Kupfer, 2008 ). Also, since circadian rhythm regulates physiological and metabolic processes, disruption in circadian rhythm can cause metabolic dysfunctions like diabetes and obesity (Shimizu et al., 2016 ), eventually affecting cognition and learning (Fig. 1 ).
Delayed circadian preference including a tendency to sleep later in the night is common amongst young adults and university students (Hershner and Chervin, 2014 ). This delayed sleep phase disorder, often seen in adolescents, negatively impacts academic achievement and is frequently accompanied by depression (Bartlett et al., 2013 ; Sivertsen et al., 2015 ). Alongside, there is a positive correlation between sleep regularity and academic grades, implying that irregularity in sleep–wake cycles is associated with poor academic performance, delayed circadian rhythm and sleep and wake timings (Phillips et al., 2017 ). Even weekday-to-weekend discrepancy in sleeping patterns has been associated with impaired academic performance in adolescents (Sun et al., 2019 ). Further connection between sleep pattern, circadian rhythm, alertness, and the mood was observed in adolescents aged 13–18 where evening chronotypes showed poor sleep quality and low alertness. In turn, sleep quality was associated with poor outcomes including low daytime alertness and depressed mood. Evening chronotypes and those with poor sleep quality were more likely to report poor academic performance via association with depression. Strangely, sleep duration did not directly affect their functionality (Short et al., 2013 ). Contrastingly, in adults aged 40–69 years, the evening and morning chronotypes were associated with superior and poor cognitive performance, respectively, relative to intermediate chronotype (Kyle et al., 2017 ). In addition to this age-specific effect, the effect of chronotype can be subject-specific. For example, in subjects involving fluid cognition for example science, there was a significant correlation between grades and chronotype, implying that late chronotypes would be disadvantaged in exams of scientific subjects if examined early in the day. This was distinct from humanistic/linguistic subjects in which no correlation with chronotype was observed (Zerbini et al., 2017 ). These observations question the “one size fits all” approach of assessment strategies.
Daytime nap
The benefits of daytime napping in healthy adults have been discussed in detail elsewhere (Milner and Cote, 2009 ). In children, daytime nap facilitates generalisation of word meanings (Horváth et al., 2016 ) and explicit memory consolidation but not implicit perceptual learning (Giganti et al., 2014 ). A 90-min nap increases hippocampal activation, restores its function and improves declarative memory encoding (Ong et al., 2020 ). Generally, daytime napping has been found to be beneficial for memory, alertness, and abstraction of general concepts, i.e. creating relational memory networks (Lau et al., 2011 ). Delayed nap following a learning activity helps in the retention of declarative memory (Alger et al., 2010 ) and exercising before the daytime nap is thought to benefit memory more than napping or exercising alone (Mograss et al., 2020 ). Also, napping for 0.1–1 hour has been associated with a reduced prevalence of overweightness (Chen et al., 2019 ).
Contrastingly, in some studies, napping has been found to impart no substantial benefits to cognition. For example, despite the daytime nap of 1 hour, procedural performance remained impaired after total deprivation of night sleep (Kurniawan et al., 2016 ), indicating that daytime nap may not always be reparative. In other studies, 4 weeks of 90-minute nap intervention (napping or restriction) did not alter behavioural performance or brain activity during sleep in healthy adults aged 18–35 (McDevitt et al., 2018 ) and enhancements in visuomotor skills occurred regardless of daytime nap (Kaida et al., 2017 ). Age is a factor in relishing the benefits of napping. A 90-min nap can benefit episodic memory retention in young adults but these benefits decrease with age (Scullin et al., 2017 ) and may be harmful in the older population, particularly in those getting more than 9 hours of sleep (Mantua and Spencer, 2017 ; Mehra and Patel, 2012 ).
Napping can increase the risk for depression (Foley et al., 2007 ) and show a positive association with depression, i.e., napping is associated with greater likelihood of depression (Y. Liu et al., 2018 ). Cardiovascular diseases, cirrhosis and kidney disease have been linked with both daytime napping and depression (Abdel-Kader et al., 2009 ; Hare et al., 2014 ; Ko et al., 2013 ). While a previous study indicated that the time of nap, morning or afternoon, made no difference to its effect on mood (Gillin et al., 1989 ), a subsequent study suggested that the timing of nap influenced relapses into depression. Specifically, in depressed individuals, morning naps caused a higher propensity of relapse into depression than afternoon naps, thereby proposing the involvement of circadian rhythm in this process. Apart from depression, studies have struggled to identify the direct effect of nap on mood (Gillin et al., 1989 ; Wiegand et al., 1993 ). As daytime napping has been associated with poor sleep quality (Alotaibi et al., 2020 ), it may lead to irregular sleep–wake patterns and thereby alter circadian rhythm (Phillips et al., 2017 ). Also, nap duration was found to be important. In patients with affirmed depression, shorter naps were found to be more detrimental than longer naps (Wiegand et al., 1993 ), whereas, in the elderly, more and longer naps were associated with increased risk of mortality amongst the cognitively impaired individuals (Hays et al., 1996 ). Thus, daytime napping can affect cognitive processes directly or indirectly via its association with circadian rhythm, metabolic dysfunctions, mood, or sleep (Fig. 1 ).
Aging is associated with decreased neurogenesis and structural changes in the hippocampus amongst other neurophysiological effects. This in turn is associated with age-related mood and memory impairments (Kodali et al., 2015 ). Study on the effect of age on mood and emotion regulation in adults aged 20–70 years showed that older participants had a higher tendency to use cognitive reappraisal while reducing negative mood and enhancing positive mood. Interestingly, while women did not show correlations between age and reappraisal, men showed an increment in cognitive reappraisal with age. This indicates gender-based differences in the effect of aging on emotion regulation (Masumoto et al., 2016 ). The influence of age on sleep is well known. Older people that have sleep patterns like the young demonstrate stronger cognitive functions and lesser health issues than those whose sleep patterns match their age (Djonlagic et al., 2021 ). Collectively, this interlinks age, cognition, mood, and sleep.
Apparently, there is a genetic influence on learning and emotions. Approximately 148 independent genetic loci have been identified that influence and support the notion of heritability of general cognitive functions (Davies et al., 2018 ). This indicates the role of genetics in cognition (Fig. 1 ). The α-7 nicotinic acetylcholine receptor (encoded by the gene CHRNA7 ) is expressed in the central and peripheral nervous systems and other peripheral tissues. It has been implicated in various behavioural and psychiatric disorders (Yin et al., 2017 ) and recognised as an important receptor of the cholinergic anti-inflammatory pathway that exhibits a neuroprotective role. Its activation has been shown to improve learning, working memory and cognition (Ren et al., 2017 ). However, there have been some contrasting results related to this receptor. While its deletion has been linked with cognitive impairments, aggressive behaviours, decreased attention span and epilepsy, Chrna7 deficient mice have shown normal learning and memory, and the gene was not deemed essential for the control of emotions and behaviour in mice. Thus, the role of α-7 nicotinic acetylcholine receptor in maintaining mood and cognitive functions, although indicative, is yet to be fully deciphered in humans (Yin et al., 2017 ). Similarly, the gene Slitrk6 , which plays a role in the development of neural circuits in the inner ear may also play a role in some cognitive functions, but it does not appear to play a clear role in mood or memory (Matsumoto et al., 2011 ). Notably, inborn errors of metabolism, i.e., rare inherited disorders may show psychiatric manifestations even in the absence of obvious neurological symptoms. These manifestations could involve impairments in cognitive functions, and/or in the regulation of learning, mood and behaviour (Bonnot et al., 2015 ).
Other factors and associations
Indeed, optimal learning is additionally influenced by factors beyond those discussed here. These factors could be adequate meal frequency, physical activity and low screen time (Adelantado-Renau, Jiménez-Pavón, et al., 2019 ; Burns et al., 2018 ). In adolescents, the time of internet usage was identified as a factor that mediated the association between sleep quality (but not duration) and academic performance (Adelantado-Renau, Diez-Fernandez, et al., 2019 ; Evers et al., 2020 ). Self-perception is another determinant of performance. The American Psychological Association defines self-perception as “person’s view of his or herself or of any of the mental or physical attributes that constitute the self. Such a view may involve genuine self-knowledge or varying degrees of distortion”. Compared to other residents, surgery residents indicated the less perceived impact of sleep-loss on their performance (Woodrow et al., 2008 ). This may be related to specific work culture or profession where there is the reluctance of acceptance of natural human limitations posed by sleep deprivation. Whether there is real resistance to sleep deprivation amongst such professional groups or a misconception requires investigation. Exercise affects both sleep and mood; the latter probably affects in a sex-dependent manner. Thus, moderate exercise has been proposed as a therapy for treating mood disorders (Lalanza et al., 2015 ).
Sleep and mood: a bidirectional but unequal relationship
While the cause of the relationship between sleep and mood is not fully understood, adequate quality and quantity of sleep has shown physiological benefits and may enhance mood (Scully, 2013 ). Sleep encourages insightful behaviour (Wagner et al., 2004 ) and regulates mood (Vandekerckhove and Wang, 2017 ). Sleeping and dreaming activate emotional and reward systems that help process information, and consolidate memory “with high emotional or motivational value”. Inevitably, sleep disturbances can dysregulate these motivational and emotional processes and cause predisposition to mood disorders (Perogamvros et al., 2013 ). Sleep loss can reinforce negative emotions, reduce positive emotions, and increase the risk for psychiatric disorders. In children and adolescents, it can increase anger, depression, confusion and aggression (Vandekerckhove and Wang, 2017 ). Thus, sleep disorder has been associated with depression, where the former can predict the latter (LaGrotte et al., 2016 ). Sleep deprivation and daytime sleepiness amongst adolescents and college students cause mood deficits, negatively affect their mood and learning, and lead to poor academic performance (Hershner and Chervin, 2014 ; Short and Louca, 2015 ). Thus, disrupted sleep acts as a diagnostic factor for mood disorders, including post-traumatic stress disorder, major depression and anxiety (Walker et al., 2020 ).
In turn, mood affects sleep quality. Emotional events and stress during the daytime can affect sleep physiology. Negative states such as sadness, loneliness, and grief are related to sleep impairments, whereas positive states like love can be associated with lessened sleep duration but better sleep quality; the latter needs further evidence. Although dysregulation of emotion relates to poor sleep quality (Vandekerckhove and Wang, 2017 ), the effect of mood on sleep can be modulated by our approach of coping with our emotions (Vandekerckhove and Wang, 2017 ). Therefore, this effect is significantly smaller than the reverse (Triantafillou et al., 2019 ).
Summary and future direction
Sleep and mood influence cognitive functions and thereby affect academic performance. In turn, these are influenced by a network of regulatory factors that directly or indirectly affect learning. The compilation of observations clearly demonstrates the complexity and multifactorial dependence of academic achievement on students’ lifestyle and physiology, as discussed in the form of effectors like age, gender, diet, hydration level, obesity, sex hormones, circadian rhythm, and genetics (Fig. 1 ).
The emerged picture brings forth two points. First, it partly explains the ambiguous and conflicting data on the effects of sleep and mood on academic performance. Second, these revelations collectively question the ‘one-size fits all’ approach in implementing education strategies. It urges to explore formulating bespoke group-specific or subject-specific strategies to optimise teaching–learning approaches. Knowledge of these factors and their associations with each other can aid in forming these groups and improving educational strategies to better support students. However, it is essential to retain parity in education, and this would be the biggest challenge while formulating bespoke approaches.
In the context of sleep, studies could be conducted that first establish standardised means of measuring sleep quality and then measure sleep quality and quantity simultaneously in individuals of different ages groups, sex, and professions. This could then be related to their performance in their respective fields/professions; academic or otherwise. Such studies will help to better understand these interrelationships and address some discrepancies in the data.
Limitations
One limitation of this review is that it addresses only academic performance. Performance should be viewed broadly and be inclusive of all types, for example, athletic performance, dance performance or performance at work on a desk job that may include creative work or financial/mathematical calculations. It would be interesting to investigate the effect of alterations in sleep and mood on various types of performances and those results will be able to provide us with a much broader picture than the one depicted here. Notably, while learning can be assessed, it is difficult to quantify emotions (Ayaz‐Alkaya, 2018 ; Nieh et al., 2013 ). As such, it is believed that qualitative research is a better approach for studying emotional responses than quantitative research (Ayaz‐Alkaya, 2018 ).
Another point of limitation is related to the proposed models in Figs. 2 and 3 . These show hypothetical mathematical scales of learning and emotion where emotions are placed on a scale of learning, and learning is placed on the scale of emotions, respectively. While these models certainly help to better visualise and understand the interrelationships, these scales show only 2-dimensions. There could be a 3rd dimension, and this could be either one of the factors or a combination of the several factors discussed here (and beyond) that determine the effect of mood/emotion on learning/cognition. Additionally, the depicted scales and their interpretations may vary between individuals because the intensity of the same emotion felt by different individuals may differ. Figure 3 depicts emotions and learning. Based on the studies so far, here, negative emotions have been shown to stimulate learning, but which negative emotions these would be (for e.g., shame or anxiety), at what intensities these would stimulate optimal learning if at all, and how this compares with optimal learning induced by positive emotions remains to be investigated. Therefore, the extent to which these scales can be applied in real-life needs to be verified.
Abdel-Kader K, Unruh ML, Weisbord SD (2009) Symptom burden, depression, and quality of life in chronic and end-stage kidney disease. Clin J Am Soc Nephrol 4(6):1057–1064. https://doi.org/10.2215/CJN.00430109
Article PubMed PubMed Central Google Scholar
Abdulghani HM, Alrowais NA, Bin-Saad NS, Al-Subaie NM, Haji AMA, Alhaqwi AI (2012) Sleep disorder among medical students: relationship to their academic performance. Med Teacher 34(Suppl 1):S37–S41. https://doi.org/10.3109/0142159X.2012.656749
Article Google Scholar
Adelantado-Renau M, Diez-Fernandez A, Beltran-Valls MR, Soriano-Maldonado A, Moliner-Urdiales D (2019) The effect of sleep quality on academic performance is mediated by Internet use time: DADOS study. J Pediatr 95(4):410–418. https://doi.org/10.1016/j.jped.2018.03.006
Adelantado-Renau M, Jiménez-Pavón D, Beltran-Valls MR, Moliner-Urdiales D (2019) Independent and combined influence of healthy lifestyle factors on academic performance in adolescents: DADOS Study. Pediatr Res 85(4):456–462. https://doi.org/10.1038/s41390-019-0285-z
Article PubMed Google Scholar
Agustí A, García-Pardo MP, López-Almela I, Campillo I, Maes M, Romaní-Pérez M, Sanz Y (2018) Interplay between the gut–brain axis, obesity and cognitive function. Front Neurosci 12:155. https://doi.org/10.3389/fnins.2018.00155
Ahrberg K, Dresler M, Niedermaier S, Steiger A, Genzel L (2012) The interaction between sleep quality and academic performance. J Psychiatr Res 46(12):1618–1622. https://doi.org/10.1016/j.jpsychires.2012.09.008
Article CAS PubMed Google Scholar
Alexandre C, Latremoliere A, Ferreira A, Miracca G, Yamamoto M, Scammell TE, Woolf CJ (2017) Decreased alertness due to sleep loss increases pain sensitivity in mice. Nat Med 23(6):768–774. https://doi.org/10.1038/nm.4329
Article CAS PubMed PubMed Central Google Scholar
Alger SE, Lau H, Fishbein W (2010) Delayed onset of a daytime nap facilitates retention of declarative memory. PLoS ONE 5(8):e12131. https://doi.org/10.1371/journal.pone.0012131
Article ADS CAS PubMed PubMed Central Google Scholar
Alhola P, Polo-Kantola P (2007) Sleep deprivation: impact on cognitive performance Neuropsychiatr Disease Treat 3(5):553–567. https://www.ncbi.nlm.nih.gov/pmc/articles/PMC2656292/
Alotaibi AD, Alosaimi FM, Alajlan AA, Bin Abdulrahman KA (2020) The relationship between sleep quality, stress, and academic performance among medical students. J Fam Community Med 27(1):23–28. https://doi.org/10.4103/jfcm.JFCM_132_19
Arendt J (2019). Melatonin: countering chaotic time cues. Front Endocrinol 10. https://doi.org/10.3389/fendo.2019.00391
Arnaldi D, Famà F, De Carli F, Morbelli S, Ferrara M, Picco A, Accardo J, Primavera A, Sambuceti G, Nobili F (2015) The role of the serotonergic system in REM sleep behavior disorder. Sleep 38(9):1505–1509. https://doi.org/10.5665/sleep.5000
Assaad S, Costanian C, Haddad G, Tannous F (2014) Sleep patterns and disorders among university students in Lebanon. J Res Health Sci 14(3):198–204
PubMed Google Scholar
Auerbach RP, Mortier P, Bruffaerts R, Alonso J, Benjet C, Cuijpers P, Demyttenaere K, Ebert DD, Green JG, Hasking P, Murray E, Nock MK, Pinder-Amaker S, Sampson NA, Stein DJ, Vilagut G, Zaslavsky AM, Kessler RC (2018) The WHO World Mental Health Surveys International College Student Project: prevalence and distribution of mental disorders. J Abnormal Psychol 127(7):623–638. https://doi.org/10.1037/abn0000362
Ayaz‐Alkaya S (2018) Overview of psychosocial problems in individuals with stoma: a review of literature. Int Wound J 16(1):243–249. https://doi.org/10.1111/iwj.13018
Bahammam AS, Alaseem AM, Alzakri AA, Almeneessier AS, Sharif MM (2012) The relationship between sleep and wake habits and academic performance in medical students: a cross-sectional study. BMC Med Educ 12:61. https://doi.org/10.1186/1472-6920-12-61
Balart P, Oosterveen M (2019) Females show more sustained performance during test-taking than males. Nat Commun 10(1):3798. https://doi.org/10.1038/s41467-019-11691-y
Banfi T, Coletto E, d’Ascanio P, Dario P, Menciassi A, Faraguna U, Ciuti G (2019) Effects of sleep deprivation on surgeons dexterity. Front Neurol 10:595. https://doi.org/10.3389/fneur.2019.00595
Bartlett DJ, Biggs SN, Armstrong SM (2013) Circadian rhythm disorders among adolescents: assessment and treatment options. Med J Aust 199(8):S16–S20. https://doi.org/10.5694/mja13.10912
Beccuti G, Pannain S (2011) Sleep and obesity. Curr Opin Clin Nutr Metab Care 14(4):402–412. https://doi.org/10.1097/MCO.0b013e3283479109
Bedrosian TA, Nelson RJ (2017) Timing of light exposure affects mood and brain circuits. Transl Psychiatry 7(1):e1017. https://doi.org/10.1038/tp.2016.262
Benton D (2011) Dehydration influences mood and cognition: a plausible hypothesis? Nutrients 3(5):555–573. https://doi.org/10.3390/nu3050555
Bertels J, Demoulin C, Franco A, Destrebecqz A (2013) Side effects of being blue: influence of sad mood on visual statistical learning. PLoS ONE 8(3):e59832. https://doi.org/10.1371/journal.pone.0059832
Betzel RF, Satterthwaite TD, Gold JI, Bassett DS (2017) Positive affect, surprise, and fatigue are correlates of network flexibility. Sci Rep 7(1):520. https://doi.org/10.1038/s41598-017-00425-z
Binks H, Vincent E, Gupta G, Irwin C, Khalesi S (2020) Effects of diet on sleep: a narrative review. Nutrients 12(4). https://doi.org/10.3390/nu12040936
Bisson MAS, Sears CR (2007) The effect of depressed mood on the interpretation of ambiguity, with and without negative mood induction. Cogn Emotion 21(3):614–645. https://doi.org/10.1080/02699930600750715
Bonnot O, Herrera PM, Tordjman S, Walterfang M (2015) Secondary psychosis induced by metabolic disorders. Front Neurosci 9:177. https://doi.org/10.3389/fnins.2015.00177
Burns RD, Fu Y, Brusseau TA, Clements-Nolle K, Yang W (2018) Relationships among physical activity, sleep duration, diet, and academic achievement in a sample of adolescents. Prev Med Rep 12:71–74. https://doi.org/10.1016/j.pmedr.2018.08.014
Castanon N, Luheshi G, Layé S (2015) Role of neuroinflammation in the emotional and cognitive alterations displayed by animal models of obesity. Front Neurosci 9:229. https://doi.org/10.3389/fnins.2015.00229
Chen M, Zhang X, Liang Y, Xue H, Gong Y, Xiong J, He F, Yang Y, Cheng G (2019) Associations between nocturnal sleep duration, midday nap duration and body composition among adults in Southwest China. PLoS ONE 14(10):e0223665. https://doi.org/10.1371/journal.pone.0223665
Choi K, Shin C, Kim T, Chung HJ, Suk H-J (2019) Awakening effects of blue-enriched morning light exposure on university students’ physiological and subjective responses. Sci Rep 9(1):345. https://doi.org/10.1038/s41598-018-36791-5
Choshen-Hillel S, Ishqer A, Mahameed F, Reiter J, Gozal D, Gileles-Hillel A, Berger I (2020) Acute and chronic sleep deprivation in residents: cognition and stress biomarkers. Med Educ. https://doi.org/10.1111/medu.14296
Cormier RE (1990) Sleep disturbances. In: Walker HK, Hall WD, Hurst JW (eds) Clinical methods: the history, physical, and laboratory examinations, 3rd edn. Butterworths.
Curcio G, Ferrara M, De Gennaro L (2006) Sleep loss, learning capacity and academic performance. Sleep Med Rev 10(5):323–337. https://doi.org/10.1016/j.smrv.2005.11.001
Davies G, Lam M, Harris SE, Trampush JW, Luciano M, Hill WD, Hagenaars SP, Ritchie SJ, Marioni RE, Fawns-Ritchie C, Liewald DCM, Okely JA, Ahola-Olli AV, Barnes CLK, Bertram L, Bis JC, Burdick KE, Christoforou A, DeRosse P, Deary IJ (2018) Study of 300,486 individuals identifies 148 independent genetic loci influencing general cognitive function. Nat Commun 9(1):2098. https://doi.org/10.1038/s41467-018-04362-x
Davis KL, Montag C (2019) Selected principles of pankseppian affective neuroscience. Front Neurosci 12. https://doi.org/10.3389/fnins.2018.01025
Dewald JF, Meijer AM, Oort FJ, Kerkhof GA, Bögels SM (2010) The influence of sleep quality, sleep duration and sleepiness on school performance in children and adolescents: a meta-analytic review. Sleep Med Rev 14(3):179–189. https://doi.org/10.1016/j.smrv.2009.10.004
Djonlagic I, Mariani S, Fitzpatrick AL, Van Der Klei V.M.G.T.H, Johnson DA, Wood AC, Seeman T, Nguyen HT, Prerau MJ, Luchsinger JA, Dzierzewski JM, Rapp SR, Tranah GJ, Yaffe K, Burdick KE, Stone KL, Redline S, Purcell SM (2021) Macro and micro sleep architecture and cognitive performance in older adults. Nat Hum Behav 5, 123–145. https://doi.org/10.1038/s41562-020-00964-y
Euser AS, Franken IHA (2012) Alcohol affects the emotional modulation of cognitive control: an event-related brain potential study. Psychopharmacology 222(3):459–476. https://doi.org/10.1007/s00213-012-2664-6
Evers K, Chen S, Rothmann S, Dhir A, Pallesen S (2020) Investigating the relation among disturbed sleep due to social media use, school burnout, and academic performance. J Adolesc 84:156–164. https://doi.org/10.1016/j.adolescence.2020.08.011
Fattinger S, de Beukelaar TT, Ruddy KL, Volk C, Heyse NC, Herbst JA, Hahnloser RHR, Wenderoth N, Huber R (2017) Deep sleep maintains learning efficiency of the human brain. Nat Commun 8:15405. https://doi.org/10.1038/ncomms15405
Fenn KM, Nusbaum HC, Margoliash D (2003) Consolidation during sleep of perceptual learning of spoken language. Nature 425(6958):614–616. https://doi.org/10.1038/nature01951
Article ADS CAS PubMed Google Scholar
Firth, J, Gangwisch, JE, Borsini, A, Wootton, RE, & Mayer, EA (2020). Food and mood: how do diet and nutrition affect mental wellbeing? The BMJ 369. https://doi.org/10.1136/bmj.m2382
Foley DJ, Vitiello MV, Bliwise DL, Ancoli-Israel S, Monjan AA, Walsh JK (2007) Frequent napping is associated with excessive daytime sleepiness, depression, pain, and nocturia in older adults: findings from the National Sleep Foundation ‘2003 Sleep in America’ Poll. Am J Geriatr Psychiatry 15(4):344–350. https://doi.org/10.1097/01.JGP.0000249385.50101.67
Francis HM, Stevenson RJ, Chambers JR, Gupta D, Newey B, Lim CK (2019) A brief diet intervention can reduce symptoms of depression in young adults—a randomised controlled trial. PLoS ONE 14(10):e0222768. https://doi.org/10.1371/journal.pone.0222768
Friedrich M, Mölle M, Friederici AD, Born J (2020) Sleep-dependent memory consolidation in infants protects new episodic memories from existing semantic memories. Nat Commun 11(1):1298. https://doi.org/10.1038/s41467-020-14850-8
Gaultney JF (2010) The prevalence of sleep disorders in college students: Impact on academic performance. J Am College Health 59(2):91–97. https://doi.org/10.1080/07448481.2010.483708
Germain A, Kupfer DJ (2008) Circadian rhythm disturbances in depression. Hum Psychopharmacol 23(7):571–585. https://doi.org/10.1002/hup.964
Gerstner JR, Yin JCP (2010) Circadian rhythms and memory formation. Nat Rev Neurosci 11(8):577–588. https://doi.org/10.1038/nrn2881
Giganti F, Arzilli C, Conte F, Toselli M, Viggiano MP, Ficca G (2014) The effect of a daytime nap on priming and recognition tasks in preschool children. Sleep 37(6):1087–1093. https://doi.org/10.5665/sleep.3766
Gillin JC, Kripke DF, Janowsky DS, Risch SC (1989) Effects of brief naps on mood and sleep in sleep-deprived depressed patients. Psychiatry Res 27(3):253–265. https://doi.org/10.1016/0165-1781(89)90141-8
González-Orozco JC, Camacho-Arroyo I (2019) Progesterone actions during central nervous system development. Front Neurosci 13:503. https://doi.org/10.3389/fnins.2019.00503
Gruber R, Laviolette R, Deluca P, Monson E, Cornish K, Carrier J (2010) Short sleep duration is associated with poor performance on IQ measures in healthy school-age children. Sleep Med 11(3):289–294. https://doi.org/10.1016/j.sleep.2009.09.007
Gualano MR, Lo Moro G, Voglino G, Bert F, Siliquini R (2020) Effects of Covid-19 lockdown on mental health and sleep disturbances in Italy. Int J Environ Res Public Health 17(13). https://doi.org/10.3390/ijerph17134779
Hafner M, Stepanek M, Taylor J, Troxel WM, van Stolk C (2017) Why sleep matters-the economic costs of insufficient sleep: a Cross-Country Comparative Analysis. Rand Health Q 6(4):11
PubMed PubMed Central Google Scholar
Hagewoud R, Whitcomb SN, Heeringa AN, Havekes R, Koolhaas JM, Meerlo P (2010) A time for learning and a time for sleep: the effect of sleep deprivation on contextual fear conditioning at different times of the day Sleep 33(10):1315–1322. https://www.ncbi.nlm.nih.gov/pmc/articles/PMC2941417/
Hama S, Yoshimura K, Yanagawa A, Shimonaga K, Furui A, Soh Z, Nishino S, Hirano H, Yamawaki S, Tsuji T (2020) Relationships between motor and cognitive functions and subsequent post-stroke mood disorders revealed by machine learning analysis. Sci Rep 10(1):19571. https://doi.org/10.1038/s41598-020-76429-z
Hare DL, Toukhsati SR, Johansson P, Jaarsma T (2014) Depression and cardiovascular disease: a clinical review. Eur Heart J 35(21):1365–1372. https://doi.org/10.1093/eurheartj/eht462
Hayley AC, Sivertsen B, Hysing M, Vedaa Ø, Øverland S (2017) Sleep difficulties and academic performance in Norwegian higher education students. Br J Educ Psychol 87(4):722–737. https://doi.org/10.1111/bjep.12180
Hays JC, Blazer DG, Foley DJ (1996) Risk of napping: excessive daytime sleepiness and mortality in an older community population. J Am Geriatr Soc 44(6):693–698. https://doi.org/10.1111/j.1532-5415.1996.tb01834.x
Haywood X, Pienaar AE (2021) Long-term influences of stunting, being underweight, and thinness on the academic performance of primary school girls: the NW-CHILD Study. Int J Environ Res Public Health 18(17):8973. https://doi.org/10.3390/ijerph18178973
Healthy Diet—an overview|ScienceDirect Topics (n.d.) https://www.sciencedirect.com/topics/agricultural-and-biological-sciences/healthy-diet . Accessed 4 Dec 2021.
Hedin G, Norell-Clarke A, Hagell P, Tønnesen H, Westergren A, Garmy P (2020). Insomnia in relation to academic performance, self-reported health, physical activity, and substance use among adolescents. Int J Environ Res Public Health 17(17). https://doi.org/10.3390/ijerph17176433
Hershner SD, Chervin RD (2014) Causes and consequences of sleepiness among college students. Nat Sci Sleep 6:73–84. https://doi.org/10.2147/NSS.S62907
Hidese S, Ota M, Matsuo J, Ishida I, Hiraishi M, Yoshida S, Noda T, Sato N, Teraishi T, Hattori K, Kunugi H (2018) Association of obesity with cognitive function and brain structure in patients with major depressive disorder. J Affect Disord 225:188–194. https://doi.org/10.1016/j.jad.2017.08.028
Hindash AHC, Amir N (2012) Negative interpretation bias in individuals with depressive symptoms. Cogn Ther Res 36(5):502–511. https://doi.org/10.1007/s10608-011-9397-4
Hiroi R, Weyrich G, Koebele SV, Mennenga SE, Talboom JS, Hewitt LT, Lavery CN, Mendoza P, Jordan A, Bimonte-Nelson HA (2016) Benefits of hormone therapy estrogens depend on estrogen type: 17β-estradiol and conjugated equine estrogens have differential effects on cognitive, anxiety-like, and depressive-like behaviors and increase tryptophan hydroxylase-2 mRNA levels in dorsal raphe nucleus subregions. Front Neurosci 10:517. https://doi.org/10.3389/fnins.2016.00517
Hirshkowitz M, Whiton K, Albert SM, Alessi C, Bruni O, DonCarlos L, Hazen N, Herman J, Katz ES, Kheirandish-Gozal L, Neubauer DN, O’Donnell AE, Ohayon M, Peever J, Rawding R, Sachdeva RC, Setters B, Vitiello MV, Ware JC, Adams Hillard PJ (2015) National Sleep Foundation’s sleep time duration recommendations: methodology and results summary. Sleep Health 1(1):40–43. https://doi.org/10.1016/j.sleh.2014.12.010
Horváth K, Liu S, Plunkett K (2016) A daytime nap facilitates generalization of word meanings in young toddlers. Sleep 39(1):203–207. https://doi.org/10.5665/sleep.5348
Htet H, Saw YM, Saw TN, Htun NMM, Mon KL, Cho SM, Thike T, Khine AT, Kariya T, Yamamoto E, Hamajima N (2020) Prevalence of alcohol consumption and its risk factors among university students: a cross-sectional study across six universities in Myanmar. PLoS ONE 15(2):e0229329. https://doi.org/10.1371/journal.pone.0229329
Huang Q, Liu H, Suzuki K, Ma S, Liu C (2019) Linking what we eat to our mood: a review of diet, dietary antioxidants, and depression. Antioxidants 8(9). https://doi.org/10.3390/antiox8090376
Huber R, Ghilardi MF, Massimini M, Tononi G (2004) Local sleep and learning. Nature 430(6995):78–81. https://doi.org/10.1038/nature02663
Ishihara T, Nakajima T, Yamatsu K, Okita K, Sagawa M, Morita N (2020) Longitudinal relationship of favorable weight change to academic performance in children. npj Sci Learn 5(1):1–8. https://doi.org/10.1038/s41539-020-0063-z
Jahrami H, BaHammam AS, Bragazzi NL, Saif Z, Faris M, Vitiello MV (2021) Sleep problems during the COVID-19 pandemic by population: a systematic review and meta-analysis. J Clin Sleep Med 17(2):299–313. https://doi.org/10.5664/jcsm.8930
Jalali R, Khazaei H, Paveh BK, Hayrani Z, Menati L (2020) The effect of sleep quality on students’ academic achievement. Adv Med Educ Pract 11:497–502. https://doi.org/10.2147/AMEP.S261525
James SL, Abate D, Abate KH, Abay SM, Abbafati C, Abbasi N, Abbastabar H, Abd-Allah F, Abdela J, Abdelalim A, Abdollahpour I, Abdulkader RS, Abebe Z, Abera SF, Abil OZ, Abraha HN, Abu-Raddad LJ, Abu-Rmeileh NME, Accrombessi MMK, Murray CJL (2018) Global, regional, and national incidence, prevalence, and years lived with disability for 354 diseases and injuries for 195 countries and territories, 1990–2017: a systematic analysis for the Global Burden of Disease Study 2017. The Lancet 392(10159):1789–1858. https://doi.org/10.1016/S0140-6736(18)32279-7
Janati Idrissi A, Lamkaddem A, Benouajjit A, Ben El Bouaazzaoui M, El Houari F, Alami M, Labyad S, Chahidi A, Benjelloun M, Rabhi S, Kissani N, Zarhbouch B, Ouazzani R, Kadiri F, Alouane R, Elbiaze M, Boujraf S, El Fakir S, Souirti Z (2020) Sleep quality and mental health in the context of COVID-19 pandemic and lockdown in Morocco. Sleep Med 74:248–253. https://doi.org/10.1016/j.sleep.2020.07.045
Javaid R, Momina AU, Sarwar MZ, Naqi SA (2020) Quality of sleep and academic performance among medical university students. J College Physicians Surg-Pakistan 30(8):844–848. https://doi.org/10.29271/jcpsp.2020.08.844
Johnston A, Gradisar M, Dohnt H, Billows M, Mccappin S (2010) Adolescent sleep and fluid intelligence performance. Sleep Biol Rhythm 8(3):180–186. https://doi.org/10.1111/j.1479-8425.2010.00442.x
Kaida K, Itaguchi Y, Iwaki S (2017) Interactive effects of visuomotor perturbation and an afternoon nap on performance and the flow experience. PLoS ONE 12(2):e0171907. https://doi.org/10.1371/journal.pone.0171907
Kayaba M, Matsushita T, Enomoto M, Kanai C, Katayama N, Inoue Y, Sasai-Sakuma T (2020) Impact of sleep problems on daytime function in school life: a cross-sectional study involving Japanese university students. BMC Public Health 20(1):371. https://doi.org/10.1186/s12889-020-08483-1
Kleinstäuber M (2013) Mood. In: Gellman MD, Turner JR (eds) Encyclopedia of behavioral medicine. Springer, pp. 1259–1261
Kline C (2013a) Sleep duration. In: Gellman MD, Turner JR (eds) Encyclopedia of behavioral medicine. Springer, pp. 1808–1810
Kline C (2013b) Sleep quality. In: Gellman MD, Turner JR (eds) Encyclopedia of behavioral medicine. Springer, pp. 1811–1813
Knüppel A, Shipley MJ, Llewellyn CH, Brunner EJ (2017) Sugar intake from sweet food and beverages, common mental disorder and depression: prospective findings from the Whitehall II study. Sci Rep 7. https://doi.org/10.1038/s41598-017-05649-7
Ko F-Y, Yang AC, Tsai S-J, Zhou Y, Xu L-M (2013) Physiologic and laboratory correlates of depression, anxiety, and poor sleep in liver cirrhosis. BMC Gastroenterol 13:18. https://doi.org/10.1186/1471-230X-13-18
Kodali M, Parihar VK, Hattiangady B, Mishra V, Shuai B, Shetty AK (2015) Resveratrol prevents age-related memory and mood dysfunction with increased hippocampal neurogenesis and microvasculature, and reduced glial activation. Sci Rep 5:8075. https://doi.org/10.1038/srep08075
Kurniawan IT, Cousins JN, Chong PLH, Chee MWL (2016) Procedural performance following sleep deprivation remains impaired despite extended practice and an afternoon nap. Sci Rep 6:36001. https://doi.org/10.1038/srep36001
Kyle SD, Sexton CE, Feige B, Luik AI, Lane J, Saxena R, Anderson SG, Bechtold DA, Dixon W, Little MA, Ray D, Riemann D, Espie CA, Rutter MK, Spiegelhalder K (2017) Sleep and cognitive performance: cross-sectional associations in the UK Biobank. Sleep Med 38:85–91. https://doi.org/10.1016/j.sleep.2017.07.001
LaGrotte C, Fernandez-Mendoza J, Calhoun SL, Liao D, Bixler EO, Vgontzas AN (2005) (2016). The relative association of obstructive sleep apnea, obesity and excessive daytime sleepiness with incident depression: a longitudinal, population-based study. Int J Obes 40(9):1397–1404. https://doi.org/10.1038/ijo.2016.87
Article CAS Google Scholar
Lalanza JF, Sanchez-Roige S, Cigarroa I, Gagliano H, Fuentes S, Armario A, Capdevila L, Escorihuela RM (2015) Long-term moderate treadmill exercise promotes stress-coping strategies in male and female rats. Sci Rep 5:16166. https://doi.org/10.1038/srep16166
Lau H, Alger SE, Fishbein W (2011) Relational memory: a daytime nap facilitates the abstraction of general concepts. PLoS ONE 6(11):e27139. https://doi.org/10.1371/journal.pone.0027139
Lee D (2015) Global and local missions of cAMP signaling in neural plasticity, learning, and memory. Front Pharmacol 6:161. https://doi.org/10.3389/fphar.2015.00161
LeGates TA, Altimus CM, Wang H, Lee H-K, Yang S, Zhao H, Kirkwood A, Weber ET, Hattar S (2012) Aberrant light directly impairs mood and learning through melanopsin-expressing neurons. Nature 491(7425):594–598. https://doi.org/10.1038/nature11673
Levesque RJR (2018) Sleep deprivation. In: Levesque RJR (ed) Encyclopedia of adolescence. Springer International Publishing, pp. 3606–3607
Li L-H, Wang Z-C, Yu J, Zhang Y-Q (2014) Ovariectomy results in variable changes in nociception, mood and depression in adult female rats. PLoS ONE 9(4):e94312. https://doi.org/10.1371/journal.pone.0094312
Lipinska G, Stuart B, Thomas KGF, Baldwin DS, Bolinger E (2019) Preferential consolidation of emotional memory during sleep: a meta-analysis. Front Psychol 10:1014. https://doi.org/10.3389/fpsyg.2019.01014
Liu X, Xu X, Wang H (2018) The effect of emotion on morphosyntactic learning in foreign language learners. PLoS ONE 13(11):e0207592. https://doi.org/10.1371/journal.pone.0207592
Liu Y, Peng T, Zhang S, Tang K (2018) The relationship between depression, daytime napping, daytime dysfunction, and snoring in 0.5 million Chinese populations: exploring the effects of socio-economic status and age. BMC Public Health 18(1):759. https://doi.org/10.1186/s12889-018-5629-9
Livermore M, Duncan MJ, Leatherdale ST, Patte KA (2020) Are weight status and weight perception associated with academic performance among youth? J Eat Disord 8:52. https://doi.org/10.1186/s40337-020-00329-w
Louca M, Short MA (2014) The effect of one night’s sleep deprivation on adolescent neurobehavioral performance. Sleep 37(11):1799–1807. https://doi.org/10.5665/sleep.4174
Mantua J, Spencer RMC (2017) Exploring the nap paradox: are mid-day sleep bouts a friend or foe? Sleep Med 37:88–97. https://doi.org/10.1016/j.sleep.2017.01.019
Marelli S, Castelnuovo A, Somma A, Castronovo V, Mombelli S, Bottoni D, Leitner C, Fossati A, Ferini-Strambi L (2020) Impact of COVID-19 lockdown on sleep quality in university students and administration staff. J Neurol 1–8. https://doi.org/10.1007/s00415-020-10056-6
Marta OFD, Kuo S-Y, Bloomfield J, Lee H-C, Ruhyanudin F, Poynor MY, Brahmadhi A, Pratiwi ID, Aini N, Mashfufa EW, Hasan F, Chiu H-Y (2020) Gender differences in the relationships between sleep disturbances and academic performance among nursing students: a cross-sectional study. Nurse Educ Today 85:104270. https://doi.org/10.1016/j.nedt.2019.104270
Martin EA, Kerns JG (2011) The influence of positive mood on different aspects of cognitive control. Cogn Emotion 25(2):265–279. https://doi.org/10.1080/02699931.2010.491652
Masumoto K, Taishi N, Shiozaki M (2016) Age and gender differences in relationships among emotion regulation, mood, and mental health. Gerontol Geriatr Med 2. https://doi.org/10.1177/2333721416637022
Matsumoto Y, Katayama K, Okamoto T, Yamada K, Takashima N, Nagao S, Aruga J (2011) Impaired auditory-vestibular functions and behavioral abnormalities of Slitrk6-deficient mice. PLoS ONE 6(1):e16497. https://doi.org/10.1371/journal.pone.0016497
McDevitt EA, Sattari N, Duggan KA, Cellini N, Whitehurst LN, Perera C, Reihanabad N, Granados S, Hernandez L, Mednick SC (2018) The impact of frequent napping and nap practice on sleep-dependent memory in humans. Sci Rep 8(1):15053. https://doi.org/10.1038/s41598-018-33209-0
McGee DL, Diverse Populations Collaboration (2005) Body mass index and mortality: a meta-analysis based on person-level data from twenty-six observational studies. Ann Epidemiol 15(2):87–97. https://doi.org/10.1016/j.annepidem.2004.05.012
McNamara A, Moakes K, Aston P, Gavin C, Sterr A (2014) The importance of the derivative in sex-hormone cycles: a reason why behavioural measures in sex-hormone studies are so mercurial. PLoS ONE 9(11):e111891. https://doi.org/10.1371/journal.pone.0111891
Mehra R, Patel SR (2012) To nap or not to nap: that is the question. Sleep 35(7):903–904. https://doi.org/10.5665/Sleep.1946
Mendelsohn D, Despot I, Gooderham PA, Singhal A, Redekop GJ, Toyota BD (2019) Impact of work hours and sleep on well-being and burnout for physicians-in-training: the Resident Activity Tracker Evaluation Study. Med Educ 53(3):306–315. https://doi.org/10.1111/medu.13757
Miller ZF, Fox JK, Moser JS, Godfroid A (2018) Playing with fire: effects of negative mood induction and working memory on vocabulary acquisition. Cogn Emotion 32(5):1105–1113. https://doi.org/10.1080/02699931.2017.1362374
Milner CE, Cote KA (2009) Benefits of napping in healthy adults: impact of nap length, time of day, age, and experience with napping. J Sleep Res 18(2):272–281. https://doi.org/10.1111/j.1365-2869.2008.00718.x
Mnatzaganian CL, Atayee RS, Namba JM, Brandl K, Lee KC (2020) The effect of sleep quality, sleep components, and environmental sleep factors on core curriculum exam scores among pharmacy students. Curr Pharm Teach Learn 12(2):119–126. https://doi.org/10.1016/j.cptl.2019.11.004
Mograss M, Crosetta M, Abi-Jaoude J, Frolova E, Robertson EM, Pepin V, Dang-Vu TT (2020) Exercising before a nap benefits memory better than napping or exercising alone. Sleep 43(9). https://doi.org/10.1093/sleep/zsaa062
Msetfi RM, Murphy RA, Kornbrot DE (2012) Dysphoric mood states are related to sensitivity to temporal changes in contingency. Front Psychol 3:368. https://doi.org/10.3389/fpsyg.2012.00368
Nieh EH, Kim S-Y, Namburi P, Tye KM (2013) Optogenetic dissection of neural circuits underlying emotional valence and motivated behaviors. Brain Res 1511:73–92. https://doi.org/10.1016/j.brainres.2012.11.001
Nishizawa S, Benkelfat C, Young SN, Leyton M, Mzengeza S, de Montigny C, Blier P, Diksic M(1997) Differences between males and females in rates of serotonin synthesis in human brain Proc Natl Acad Sci USA 94(10):5308–5313. https://www.ncbi.nlm.nih.gov/pmc/articles/PMC24674/
Norman KA (2006) Declarative memory: sleep protects new memories from interference. Curr Biol 16(15):R596–R597. https://doi.org/10.1016/j.cub.2006.07.008
Nösslinger H, Mair E, Toplak H, Hörmann-Wallner M (2021) Underestimation of resting metabolic rate using equations compared to indirect calorimetry in normal-weight subjects: consideration of resting metabolic rate as a function of body composition. Clin Nutr Open Sci 35:48–66. https://doi.org/10.1016/j.nutos.2021.01.003
Okano K, Kaczmarzyk JR, Dave N, Gabrieli JDE, Grossman JC (2019) Sleep quality, duration, and consistency are associated with better academic performance in college students. npj Sci Learn 4(1):1–5. https://doi.org/10.1038/s41539-019-0055-z
Ong JL, Lau TY, Lee XK, van Rijn E, Chee MWL (2020) A daytime nap restores hippocampal function and improves declarative learning. Sleep 43(9). https://doi.org/10.1093/sleep/zsaa058
Patterson SS, Kuchenbecker JA, Anderson JR, Neitz M, Neitz J (2020) A color vision circuit for non-image-forming vision in the primate retina. Curr Biol 30(7):1269–1274.e2. https://doi.org/10.1016/j.cub.2020.01.040
Pearson H (2006) Medicine: sleep it off. Nature 443(7109):261–263. https://doi.org/10.1038/443261a
Perez-Chada D, Perez-Lloret S, Videla AJ, Cardinali D, Bergna MA, Fernández-Acquier M, Larrateguy L, Zabert GE, Drake C (2007) Sleep disordered breathing and daytime sleepiness are associated with poor academic performance in teenagers. a study using the Pediatric Daytime Sleepiness Scale (PDSS) Sleep 30(12):1698–1703. https://www.ncbi.nlm.nih.gov/pmc/articles/PMC2276125/
Perogamvros L, Dang-Vu TT, Desseilles M, Schwartz S (2013) Sleep and dreaming are for important matters. Front Psychol 4:474. https://doi.org/10.3389/fpsyg.2013.00474
Phillips AJK, Clerx WM, O’Brien CS, Sano A, Barger LK, Picard RW, Lockley SW, Klerman EB, Czeisler CA (2017) Irregular sleep/wake patterns are associated with poorer academic performance and delayed circadian and sleep/wake timing. Sci Rep 7(1):3216. https://doi.org/10.1038/s41598-017-03171-4
Popkin BM, D’Anci KE, Rosenberg IH (2010) Water, hydration and health. Nutr Rev 68(8):439–458. https://doi.org/10.1111/j.1753-4887.2010.00304.x
Quartiroli A, Terry PC, Fogarty GJ (2017) Development and initial validation of the Italian Mood Scale (ITAMS) for use in sport and exercise contexts. Front Psychol 8:1483. https://doi.org/10.3389/fpsyg.2017.01483
Ren C, Tong YL, Li JC, Lu ZQ, Yao YM (2017) The protective effect of alpha 7 nicotinic acetylcholine receptor activation on critical illness and its mechanism. Int J Biol Sci 13(1):46–56. https://doi.org/10.7150/ijbs.16404
Restivo MR, McKinnon MC, Frey BN, Hall GB, Syed W, Taylor VH (2017) The impact of obesity on neuropsychological functioning in adults with and without major depressive disorder. PLoS ONE 12(5):e0176898. https://doi.org/10.1371/journal.pone.0176898
Riebl SK, Davy BM (2013) The hydration equation: update on water balance and cognitive performance. ACSM’s Health Fit J 17(6):21–28. https://doi.org/10.1249/FIT.0b013e3182a9570f
Roberts RE, Duong HT (2014) The prospective association between sleep deprivation and depression among adolescents. Sleep 37(2):239–244. https://doi.org/10.5665/sleep.3388
Roenneberg T (2013) Chronobiology: the human sleep project. Nature 498(7455):427–428. https://doi.org/10.1038/498427a
Sarris J, Thomson R, Hargraves F, Eaton M, de Manincor M, Veronese N, Solmi M, Stubbs B, Yung AR, Firth J (2020) Multiple lifestyle factors and depressed mood: a cross-sectional and longitudinal analysis of the UK Biobank ( N = 84,860). BMC Med 18:354. https://doi.org/10.1186/s12916-020-01813-5
Scholey A, Owen L (2013) Effects of chocolate on cognitive function and mood: a systematic review. Nutr Rev 71(10):665–681. https://doi.org/10.1111/nure.12065
Schönauer M, Alizadeh S, Jamalabadi H, Abraham A, Pawlizki A, Gais S (2017) Decoding material-specific memory reprocessing during sleep in humans. Nat Commun 8:15404. https://doi.org/10.1038/ncomms15404
Scullin MK, Fairley J, Decker MJ, Bliwise DL (2017) The effects of an afternoon nap on episodic memory in young and older adults. Sleep 40(5). https://doi.org/10.1093/sleep/zsx035
Scully T (2013) Sleep. Nature 497(7450):S1–S3. https://doi.org/10.1038/497S1a
Sekhon S, Gupta V (2021) Mood disorder. StatPearls Publishing.
Seoane HA, Moschetto L, Orliacq F, Orliacq J, Serrano E, Cazenave MI, Vigo DE, Perez-Lloret S (2020) Sleep disruption in medicine students and its relationship with impaired academic performance: a systematic review and meta-analysis. Sleep Med Rev 53:101333. https://doi.org/10.1016/j.smrv.2020.101333
Shimizu I, Yoshida Y, Minamino T (2016) A role for circadian clock in metabolic disease. Hypertens Res 39(7):483–491. https://doi.org/10.1038/hr.2016.12
Shochat T, Cohen-Zion M, Tzischinsky O (2014) Functional consequences of inadequate sleep in adolescents: a systematic review. Sleep Med Rev 18(1):75–87. https://doi.org/10.1016/j.smrv.2013.03.005
Short MA, Gradisar M, Lack LC, Wright HR (2013) The impact of sleep on adolescent depressed mood, alertness and academic performance. J Adolesc 36(6):1025–1033. https://doi.org/10.1016/j.adolescence.2013.08.007
Short MA, Louca M (2015) Sleep deprivation leads to mood deficits in healthy adolescents. Sleep Med 16(8):987–993. https://doi.org/10.1016/j.sleep.2015.03.007
Singh M (2014) Mood, food, and obesity. Front Psychol 5. https://doi.org/10.3389/fpsyg.2014.00925
Sivertsen B, Glozier N, Harvey AG, Hysing M (2015) Academic performance in adolescents with delayed sleep phase. Sleep Med 16(9):1084–1090. https://doi.org/10.1016/j.sleep.2015.04.011
Son C, Hegde S, Smith A, Wang X, Sasangohar F (2020) Effects of COVID-19 on college students’ mental health in the United States: Interview Survey Study. J Med Internet Res 22(9):e21279. https://doi.org/10.2196/21279
Spencer SJ, Korosi A, Layé S, Shukitt-Hale B, Barrientos RM (2017) Food for thought: how nutrition impacts cognition and emotion. NPJ Sci Food 1. https://doi.org/10.1038/s41538-017-0008-y
Štefan L, Sporiš G, Krističević T, Knjaz D (2018) Associations between sleep quality and its domains and insufficient physical activity in a large sample of Croatian young adults: a cross-sectional study. BMJ Open 8(7):e021902. https://doi.org/10.1136/bmjopen-2018-021902
Suardiaz-Muro M, Morante-Ruiz M, Ortega-Moreno M, Ruiz MA, Martín-Plasencia P, Vela-Bueno A (2020) [Sleep and academic performance in university students: a systematic review]. Rev Neurol 71(2):43–53. https://doi.org/10.33588/rn.7102.2020015
Sun W, Ling J, Zhu X, Lee TM-C, Li SX (2019) Associations of weekday-to-weekend sleep differences with academic performance and health-related outcomes in school-age children and youths. Sleep Med Rev 46:27–53. https://doi.org/10.1016/j.smrv.2019.04.003
Sundström Poromaa I, Gingnell M (2014) Menstrual cycle influence on cognitive function and emotion processing-from a reproductive perspective. Front Neurosci 8:380. https://doi.org/10.3389/fnins.2014.00380
Sweileh WM, Ali IA, Sawalha AF, Abu-Taha AS, Zyoud SH, Al-Jabi SW (2011) Sleep habits and sleep problems among Palestinian students. Child Adolesc Psychiatry Mental Health 5(1):25. https://doi.org/10.1186/1753-2000-5-25
Taras H, Potts-Datema W (2005) Sleep and student performance at school. J School Health 75(7):248–254. https://doi.org/10.1111/j.1746-1561.2005.00033.x
Teodori L, Albertini MC (2019) Shedding light into memories under circadian rhythm system control. Proc Natl Acad Sci USA 116(17):8099–8101. https://doi.org/10.1073/pnas.1903413116
Thibaut F (2015) Emotional processing needs further study in major psychiatric diseases Dialogues Clin Neurosci 17(4):359. https://www.ncbi.nlm.nih.gov/pmc/articles/PMC4734874/
Toscano-Hermoso MD, Arbinaga F, Fernández-Ozcorta EJ, Gómez-Salgado J, Ruiz-Frutos C (2020) Influence of sleeping patterns in health and academic performance among University Students. Int J Environ Res Public Health 17(8). https://doi.org/10.3390/ijerph17082760
Triantafillou S, Saeb S, Lattie EG, Mohr DC, Kording KP (2019) Relationship between sleep quality and mood: Ecological Momentary Assessment Study. JMIR Mental Health 6(3). https://doi.org/10.2196/12613
Tyng CM, Amin HU, Saad MNM, Malik AS (2017) The influences of emotion on learning and memory. Front Psychol 8. https://doi.org/10.3389/fpsyg.2017.01454
Valiente C, Swanson J, Eisenberg N (2012) Linking students’ emotions and academic achievement: when and why emotions matter. Child Dev Perspect 6(2):129–135. https://doi.org/10.1111/j.1750-8606.2011.00192.x
Vandekerckhove M, Wang Y (2017) Emotion, emotion regulation and sleep: an intimate relationship. AIMS Neurosci 5(1):1–17. https://doi.org/10.3934/Neuroscience.2018.1.1
Veasey S, Rosen R, Barzansky B, Rosen I, Owens J (2002) Sleep loss and fatigue in residency training: a reappraisal. JAMA 288(9):1116–1124. https://doi.org/10.1001/jama.288.9.1116
Vecsey CG, Baillie GS, Jaganath D, Havekes R, Daniels A, Wimmer M, Huang T, Brown KM, Li X-Y, Descalzi G, Kim SS, Chen T, Shang Y-Z, Zhuo M, Houslay MD, Abel T (2009) Sleep deprivation impairs cAMP signalling in the hippocampus. Nature 461(7267):1122–1125. https://doi.org/10.1038/nature08488
Wagner U, Gais S, Haider H, Verleger R, Born J (2004) Sleep inspires insight. Nature 427(6972):352–355. https://doi.org/10.1038/nature02223
Walker WH, Walton JC, DeVries AC, Nelson RJ (2020) Circadian rhythm disruption and mental health. Transl Psychiatry 10(1):1–13. https://doi.org/10.1038/s41398-020-0694-0
Wang X, Chen H, Liu L, Liu Y, Zhang N, Sun Z, Lou Q, Ge W, Hu B, Li M (2020) Anxiety and sleep problems of college students during the outbreak of COVID-19. Front Psychiatry 11. https://doi.org/10.3389/fpsyt.2020.588693
Wiegand M, Riemann D, Schreiber W, Lauer CJ, Berger M (1993) Effect of morning and afternoon naps on mood after total sleep deprivation in patients with major depression. Biol Psychiatry 33(6):467–476. https://doi.org/10.1016/0006-3223(93)90175-d
Woodrow SI, Park J, Murray BJ, Wang C, Bernstein M, Reznick RK, Hamstra SJ (2008) Differences in the perceived impact of sleep deprivation among surgical and non-surgical residents. Med Educ 42(5):459–467. https://doi.org/10.1111/j.1365-2923.2007.02963.x
Worthy DA, Byrne KA, Fields S (2014) Effects of emotion on prospection during decision-making. Front Psychol 5:591. https://doi.org/10.3389/fpsyg.2014.00591
Yabut JM, Crane JD, Green AE, Keating DJ, Khan WI, Steinberg GR (2019) Emerging roles for serotonin in regulating metabolism: new implications for an ancient molecule. Endocr Rev 40(4):1092–1107. https://doi.org/10.1210/er.2018-00283
Yin J, Chen W, Yang H, Xue M, Schaaf CP (2017) Chrna7 deficient mice manifest no consistent neuropsychiatric and behavioral phenotypes. Sci Rep 7:39941. https://doi.org/10.1038/srep39941
Zavodny M (2013) Does weight affect children’s test scores and teacher assessments differently? Econ Educ Rev 34:135–145. https://doi.org/10.1016/j.econedurev.2013.02.003
Zerbini G, van der Vinne V, Otto LKM, Kantermann T, Krijnen WP, Roenneberg T, Merrow M (2017) Lower school performance in late chronotypes: underlying factors and mechanisms. Sci Rep 7(1):4385. https://doi.org/10.1038/s41598-017-04076-y
Zhang L, Liu S, Liu X, Zhang B, An X, Ming D (2021) Emotional arousal and valence jointly modulate the auditory response: a 40-Hz ASSR study. IEEE Trans Neural Syst Rehabil Eng 29:1150–1157. https://doi.org/10.1109/TNSRE.2021.3088257
Zhang N, Du SM, Zhang JF, Ma GS (2019) Effects of dehydration and rehydration on cognitive performance and mood among male college students in Cangzhou, China: a self-controlled trial. Int J Environ Res Public Health 16(11) https://doi.org/10.3390/ijerph16111891
Zhao H, Zhang X, Xu Y, Gao L, Ma Z, Sun Y, Wang W (2021) Predicting the risk of hypertension based on several easy-to-collect risk factors: a machine learning method. Front Public Health 9:619429. https://doi.org/10.3389/fpubh.2021.619429
Zhu B, Vincent C, Kapella MC, Quinn L, Collins EG, Ruggiero L, Park C, Fritschi C (2018) Sleep disturbance in people with diabetes: a concept analysis. J Clin Nurs 27(1–2):e50–e60. https://doi.org/10.1111/jocn.14010
Zhu Y, Gao H, Tong L, Li Z, Wang L, Zhang C, Yang Q, Yan B (2019) Emotion regulation of hippocampus using real-time fMRI neurofeedback in healthy human. Front Hum Neurosci 13. https://doi.org/10.3389/fnhum.2019.00242
Download references
Author information
Authors and affiliations.
Centre for Education, Faculty of Life Sciences and Medicine, King’s College London, London, UK
Kosha J. Mehta
You can also search for this author in PubMed Google Scholar
Contributions
Conceptualisation, composition, and writing: KJM.
Corresponding author
Correspondence to Kosha J. Mehta .
Ethics declarations
Competing interests.
The author declares no competing interests.
Informed consent
Not applicable.
Ethical approval
This article does not contain any studies with human participants or animals performed by any of the authors.
Additional information
Publisher’s note Springer Nature remains neutral with regard to jurisdictional claims in published maps and institutional affiliations.
Rights and permissions
Open Access This article is licensed under a Creative Commons Attribution 4.0 International License, which permits use, sharing, adaptation, distribution and reproduction in any medium or format, as long as you give appropriate credit to the original author(s) and the source, provide a link to the Creative Commons license, and indicate if changes were made. The images or other third party material in this article are included in the article’s Creative Commons license, unless indicated otherwise in a credit line to the material. If material is not included in the article’s Creative Commons license and your intended use is not permitted by statutory regulation or exceeds the permitted use, you will need to obtain permission directly from the copyright holder. To view a copy of this license, visit http://creativecommons.org/licenses/by/4.0/ .
Reprints and permissions
About this article
Cite this article.
Mehta, K.J. Effect of sleep and mood on academic performance—at interface of physiology, psychology, and education. Humanit Soc Sci Commun 9 , 16 (2022). https://doi.org/10.1057/s41599-021-01031-1
Download citation
Received : 24 June 2021
Accepted : 31 December 2021
Published : 11 January 2022
DOI : https://doi.org/10.1057/s41599-021-01031-1
Share this article
Anyone you share the following link with will be able to read this content:
Sorry, a shareable link is not currently available for this article.
Provided by the Springer Nature SharedIt content-sharing initiative
This article is cited by
The role of school connectedness and friend contact in adolescent loneliness, and implications for physical health.
- Yixuan Zheng
- Margarita Panayiotou
- Joanna Inchley
Child Psychiatry & Human Development (2024)
Neurocognitive and mental health outcomes in children with tungiasis: a cross-sectional study in rural Kenya and Uganda
- Berrick Otieno
- Lynne Elson
- Amina Abubakar
Infectious Diseases of Poverty (2023)
Quick links
- Explore articles by subject
- Guide to authors
- Editorial policies


An official website of the United States government
The .gov means it’s official. Federal government websites often end in .gov or .mil. Before sharing sensitive information, make sure you’re on a federal government site.
The site is secure. The https:// ensures that you are connecting to the official website and that any information you provide is encrypted and transmitted securely.
- Publications
- Account settings
Preview improvements coming to the PMC website in October 2024. Learn More or Try it out now .
- Advanced Search
- Journal List
- Front Psychol
The influence of achievement motivation on college students’ employability: A chain mediation analysis of self-efficacy and academic performance
1 Rattanakosin International College of Creative Entrepreneurship, Rajamangala University of Technology Rattanakosin, Nakhon Pathom, Thailand
2 School of Foreign Languages and Cultures, Panzhihua University, Panzhihua, Sichuan, China
3 Faculty of Economics, Srinakharinwirot University, Bangkok, Thailand
Nutteera Phakdeephirot
Associated data.
The raw data supporting the conclusions of this article will be made available by the authors, without undue reservation.
Employability of college students has been attached great importance by higher education institutions, employers, and governments because college graduates are the strategic human resource for the sustainable growth of universities, organizations, and countries across the world. It is also receiving growing attention from academic community. This study aimed to examine the psychological mechanism that impacts college students’ employability. It adopted an empirical approach by collecting data from 646 final-year students from 9 universities in the mainland of China. SPSS 25.0 was used for description, correlation, and regression analysis. AMOS 24.0 was utilized for path analysis. Model 6 Bootstrap method of PROCESS Version 3.5 was adopted for mediation analysis. The results showed that achievement motivation positively predicted self-efficacy, academic performance, and employability among undergraduates. Participants’ self-efficacy did not significantly impact their employability or play a mediating role in the relationship between achievement motivation and employability, while academic performance was a significant mediator of this association. Self-efficacy and academic performance served as chain mediators in the prediction of achievement motivation on college students’ employability. After controlling gender and family residence, achievement motivation still had significant and positive impact on employability of college students. This research made several noteworthy contributions to the existing studies on college students’ employability and provided insight for practitioners in strengthening their employability through these psychological constructs.
Introduction
A growing number of students are graduating from higher education institutions across the world in recent years. On the one hand, converting these potential human resources into available talents to satisfy social needs is a significant way to promote economic development and social progress. These newly graduated talents can ensure the competitive advantage of organizations and innovation-driven development of nations. On the other hand, mounting pressure is laid on the shoulders of employers and governments to provide sufficient job positions for them. The rage of COVID-19 pandemic worsened the worrying situation due to economic slowdown ( Leslie and Wilson, 2020 ) and financial uncertainties ( Volenec et al., 2021 ). As a result, it became increasingly important for college students to enhance their employability to secure a job after graduation and contribute to sustainable economic growth.
Employability of college students has become a hot research field in academia in the past few years in various disciplines such as human resource management ( Donald et al., 2019 ; Nimmi and Donald, 2022 ), educational management ( Peng et al., 2021 ), psychology ( Wang et al., 2021 ), sociology ( Ingram and Allen, 2019 ), etc. Higher education institutions are striving to integrate employability developing programs into their educational system to ensure that the college students can be equipped with necessary knowledge, skills, attributes, and behaviors for a successful transition from campus to workplace ( Yawson and Yamoah, 2020 ). Scholars have been making continuous academic efforts from the perspectives of definition ( Harvey, 2001 ), influencing factors ( Nimmi and Donald, 2022 ), models ( Dacre Pool and Sewell, 2007 ), and other aspects of undergraduates’ employability to help tertiary institutions improve the performance of talent cultivation.
Among these existing studies, achievement motivation was tested to be positively associated with employability ( Diao, 2015 ). Achievement motivation is an intrinsic desire that motivates people to develop their capabilities to successfully achieve the dreams they pursue in their daily lives and in a variety of activities ( Suny and Suardiman, 2019 ). Motivation theories in contemporary education-related studies embody a variety of properties, such as goals, needs, desires, emotions, values, and interests ( Wang et al., 2020 ). Motivation has a significant correlation with individuals’ behavior, to be specific, positive motivation is able to stimulate good behavior, while negative motivation holds back such behavior ( Chiang et al., 2018 ). Achievement motivation has an impact on students’ success and excellence in the process of academic study ( Nofrizal et al., 2020 ), which, in turn, influences their employment prospects upon graduation ( Kamaliah et al., 2018 ). Prior studies have confirmed that achievement motivation significantly impacts the employability of college students, but only a few studies investigated the underlying mechanism of such influence. More specifically, no studies have been found to examine the path from achievement motivation to undergraduates’ employability through the chain mediating effect of self-efficacy and academic performance.
According to the social cognitive theory that interprets how the mechanisms of self-influence and self-regulation stimulate and govern human behavior ( Owusu-Agyeman and Fourie-Malherbe, 2019 ), self-efficacy is identified as a dominant behavior determinant ( Lee et al., 2018 ). It reflects an individual’ beliefs about his or her ability to take actions, accomplish tasks, and reach goals under different situations ( Bandura, 1986 ). Previous research revealed that achievement motivation and self-efficacy were significantly correlated ( Rui et al., 2011 ; Sabti et al., 2019 ), and self-efficacy was tested to have significant and positive influence on employability ( Lian et al., 2021 ). However, the majority of these studies concentrated on exploring the impact of self-efficacy on employability through the mediation of achievement motivation ( Wang et al., 2022 ). Less attention was paid to examine the influence of achievement motivation on employability through the mediation of self-efficacy among college students.
Additionally, achievement motivation from social cognitive perspective comprises of expectancy and value components in a broad sense, and previous studies based on such social cognitive model found that students’ motivational beliefs had significant and positive predicting effect on their academic performance ( Muenks et al., 2018 ; Steinmayr et al., 2019 ). In the meanwhile, several studies showed a significant positive relationship between students’ self-efficacy and their academic performance ( Shoval et al., 2021 ; Macakova and Wood, 2022 ). In terms of the research objects of the current study, students’ academic performance at the university was tested to have a significant positive contribution to their employment outcomes ( Chhinzer and Russo, 2018 ; Tentama and Abdillah, 2019 ). As a result, it could be of great theoretical and practical importance to explore the functioning mechanism through which achievement motivation, self-efficacy, and academic performance influence undergraduates’ employability, which has not yet been empirically investigated.
By conducting a questionnaire survey among undergraduates in the mainland of China, this study aims to examine how achievement motivation impacts self-efficacy and subsequently influences academic performance and employability under the psychological mechanism of social cognitive theory. The findings could provide theoretical insight and empirical evidence for the research on the correlation between crucial psychological constructs and undergraduates’ employability. The results would also offer feasible positive interference for higher education institutions to enhance the employability of their students.
Literature review and hypothesis development
Employability.
Employability is a combination of knowledge, skills, thought, and personal traits that improve an individual’s prospects of finding and maintaining jobs that are both satisfying and profitable to them ( Dacre Pool and Sewell, 2007 ). It refers to the integration of traits such as capability, personality, desire, and social resources to ensure employment, including the knowledge and skills a person possesses in the course of career development, and a variety of extensive adjustments to the work environment ( Yorke, 2006 ). It enables people to effectively accomplish work assignments by giving full play to their career-related abilities with proactive occupational behaviors ( Schreuder and Coetzee, 2011 ).
Since the subjects of the current research are students in higher education institutions, the specific definition of college students’ employability can be viewed as a set of capabilities that assist students to find jobs upon graduation and attain success in the process of their career development, such as occupational knowledge, skills, self-regulation, communication skills, and interpersonal relationship ( Harvey, 2001 ). It reflects the integrative abilities to accomplish satisfying work performance derived from learning ability and has turned into a critical factor for the career success of college graduates. Cultivating students’ career-related competencies and traits has become a core strategy for the transformation and development of higher education in many countries ( White et al., 2021 ). Strong employability skills can empower college students with competitive advantages in the job market and ensure their success in the workplace of the future ( Millican and Bourner, 2011 ).
Achievement motivation
Achievement motivation refers to the intrinsic drive within individuals that motivates them to accomplish important and meaningful tasks and leads them to excel at what they do ( Ye and Hagtvet, 1992 ). It is a complicated construct that includes several components such as self-perceived competency, task values, goals, and motives ( Steinmayr et al., 2019 ), which refers to the kind of motivation that drives individuals for excellent performance, competitive advantage, perseverance, and growing efforts in fulfilling activities or tasks ( Smith et al., 2019 ). As an internalized competency, it allows individuals to mobilize and manage their social and physical resources in a manner that improves performance and builds personal skills for success ( Werdhiastutie et al., 2020 ). Student achievement motivation theory holds that students with this psychological trait are fully motivated to achieve goals successfully, develop their own abilities more confidently, and avoid failure in a variety of changing situations ( Ishihara et al., 2018 ). Prior research found that achievement motivation had significant and positive correlation with the attainment of employability skills ( Diao, 2015 ) because it can implant a stronger desire into individuals to strive for success and alleviate fear of failure ( Tanjung and Musa, 2021 ). Individuals with strong achievement motivation have higher possibility to be accepted by job vacancies in the social contexts ( Huang et al., 2022 ). Hence, the following hypothesis was put forward:
H1 : Achievement motivation can positively predict employability of college students.
Self-efficacy
Self-efficacy refers to individuals’ beliefs that they can perform a specific behavior and achieve anticipated outcomes ( Bandura, 1977 ). It plays an important part in influencing people’s confidence in their ability to take actions and persevere under adversity ( Yang et al., 2021 ). Self-efficacy leads individuals to believe that they have the capability to make productive use of motivational and cognitive resources to achieve the ultimate effect of the expected actions ( Luthans, 2002 ). It is an important behavior-oriented factor that can affect students’ determination of learning objectives, choice of learning tasks, persistence of learning activities, and attribution of learning results.
Achievement motivation and self-efficacy
Individuals with strong intrinsic motivation to finish tasks and achieve goals tend to have self-confidence in taking specific actions and reaching desired objectives. For example, Wei et al. (2013) conducted a quantitative study on 336 Chinese students in higher education institutions and found that achievement motivation was positively correlated to self-efficacy in career decision. Damrongpanit (2019) suggested that achievement motivation was significantly and positively related with students’ self-efficacy in mathematics performance according to the research on 2,205 students and 117 teachers in Thailand. The study of 100 undergraduate students from two Iraqi public universities revealed that achievement motivation exhibited a significant positive correlation with self-efficacy in English writing ( Sabti et al., 2019 ). Therefore, H2 was proposed as follows:
H2: Achievement motivation can positively predict self-efficacy among college undergraduates.

Self-efficacy and employability
Self-efficacy has been widely studied in a variety of research fields because it was positively related to learning strategies ( Mornar et al., 2022 ), job performance ( Downes et al., 2021 ), occupational success ( Chughtai, 2018 ), self-esteem ( Ouyang et al., 2020 ), and commitment ( Yang et al., 2021 ). It is positively correlated with job search behavior and plays a crucial role in the employment of college graduates. Wang et al. (2022) found that self-efficacy was positively related to the levels of employability among college students in a quantitative study across six provinces in the Chinese mainland. A higher level of self-efficacy tends to make students more confident in seeking jobs when they graduate ( Lian et al., 2021 ) and more employable in the job market ( Chow et al., 2019 ). Thus, Hypothesis 3 was proposed:
H3 : There is a positive prediction of self-efficacy on college students’ employability.
Achievement motivation, self-efficacy, and employability
The previous studies revealed that achievement motivation had a positive impact on students’ self-efficacy, and higher level of self-efficacy led to stronger sense of employability of students in colleges and universities. It implied that self-efficacy may have a mediating effect in the relationship between achievement motivation and employability. As a result, the following hypothesis was proposed:
H4 : Self-efficacy played a mediating role in the correlation between achievement motivation and employability among college students.
Academic performance
Currently, academic performance has no universally agreed-upon definition in the research field of higher education. Many researchers believe that college students’ academic achievements are the sum of their learning results, behaviors, and attitudes during the period of higher education, mainly including college students’ behavioral performance and objective achievements ( Choi, 2005 ; Poropat, 2009 ; Stajkovic et al., 2018 ). Chamorro-Premuzic and Furnham (2003) measured academic performance by overall exam marks and final-year project performance. O’Connor and Paunonen (2007) argued that academic performance includes the grades of exams, essays, and courses as well as grade point average (GPA) and classroom performance. In general, the majority of the studies used GPA as a measure and indicator of students’ academic performance ( Endalamaw Yigermal, 2017 ; Eakman et al., 2019 ; Lou, 2021 ). For example, Gatzka and Hell (2018) conducted a meta-analysis on the correlation between six facets of openness and postsecondary academic performance with students’ official GPA from higher education institutions as the criterion for their academic performance. Jan et al. (2020) made correlation analysis between emotional intelligence, library anxiety, and academic achievement among college undergraduates with GPA as the indicator for academic performance. Based on the previous research, the current study takes GPA as the measurement for academic performance.
Achievement motivation and academic performance
Many researchers have found that students’ achievement motivation is closely and positively correlated with academic performance ( She et al., 2019 ; Gamazo and Martínez-Abad, 2020 ). Various constructs of motivation such as perceived control, values, and self-perception can be used as a supplement to intelligence tests to positively predict the academic performance ( Daniels and Dueck, 2022 ). Achievement motivation of students influences their psychological and behavioral characteristics such as hope of success, coping with failure, persistence in adversity, and willingness to take more challenging courses ( Yeager et al., 2019 ; Kapasi and Pei, 2022 ), which ultimately have an impact on their academic performance. A study on 4, 290 medical students from 10 Latin American countries indicated that their motivation was closely associated with achieving good academic performance during their college years ( Torres-Roman et al., 2018 ). Hence, the following hypothesis was proposed:
H5 : Achievement motivation plays a positive role in academic performance for college students.
Academic performance and employability
Several studies confirmed that academic performance is linked to college students’ employability ( Dong et al., 2019 ; Tentama and Abdillah, 2019 ). There is a statistically significant relationship between academic achievement and employability and a positive correlation between employers’ perceptions of graduates’ employability and academic achievement ( Chhinzer and Russo, 2018 ). Pinto and He (2017) found that higher academic performance led to greater job suitability and employability skills among business graduates from Peking University in China. A study on the graduate recruiters and employers who are looking for job applicants in the United Kingdom context revealed that academic achievements significantly affected the perceived employability of college graduates ( Byrne, 2022 ). Hence, Hypothesis 6 was put forward as follows:
H6: Academic performance has a positive impact on college students’ employability.
Achievement motivation, academic performance, and employability
Based on the above arguments, achievement motivation can positively predict students’ academic performance, and better academic performance result in higher level of employability, which indicated that academic performance may mediate the effect of achievement motivation on employability. Therefore, this study proposed the following hypothesis:
H7 : Academic performance played a mediating role in the correlation between achievement motivation and undergraduates’ employability.
Chain mediating effect of self-efficacy and academic performance
It has been found that students’ self-efficacy is significantly correlated with their academic performance ( Shoval et al., 2021 ; Macakova and Wood, 2022 ). A meta-analysis showed that self-efficacy was an influential factor to improve academic performance in online learning environment ( Yokoyama, 2019 ). College students’ self-efficacy was found to have a positive effect on their academic performance in online English classes in China in the midst of the COVID-19 pandemic ( Chang and Tsai, 2022 ). A systematic literature review on 27 articles revealed that self-efficacy and academic performance had significant and positive relationship among Latino students in America across all levels of education and with different measuring instruments ( Manzano-Sanchez et al., 2018 ). Thus, the following hypothesis was proposed:
H8 : College students’ self-efficacy has a positive impact on their academic performance.
In the light of the social cognitive theory, human functioning relies on the interaction between personal behavior (actions, choices, and verbal statements), internal personal factors (beliefs, expectations, attitudes, and knowledge), and environmental factors (resources, family, other people; Bandura, 1986 , 1997 ). Students who are highly motivated to strive for excellence would have higher level of self-efficacy ( Li and Li, 2018 ), generate better academic outcomes ( Liu et al., 2019 ), and become more employable in the labor market ( Dong et al., 2019 ). As a result, Hypothesis 9 was put forward:
H9 : Self-efficacy and academic performance play chain mediating roles in the relation between college students’ achievement motivation and their employability.
Control variables
Previous studies on employability have taken gender as a control variable ( De Cuyper and De Witte, 2011 ; Misra and Mishra, 2016 ; González-Romá et al., 2018 ). Cifre et al. (2018) highlighted the necessity to delve into employability from the perspective of gender. At the same time, Mamaqi et al. (2011) included economic sectors as a control variable in their study on employability. In the current research, economic sectors refer to the family background of the participants. To be more specific, it takes the family residence in rural or urban areas of the sample into the regression model to test the effect as a control variable because students from urban families are usually in more favorable financial situations than their rural counterparts ( Yang, 2010 ).
Materials and methods
Sample and procedure.
The study was conducted at nine universities in the mainland of China. The convenience sampling method was employed to recruit participants ( Assari, 2019 ). The objectives of the study and the policies of anonymity and confidentiality were presented before the participants filled the questionnaire. At last, 646 final-year college students participated in the survey with valid questionnaire. Among these respondents, 38.1% ( N = 246) were male students, and 61.9% ( N = 400) were female undergraduates. In terms of the family background, 46.1% ( N = 348) of them were from rural households, and 53.9% ( N = 298) were from urban families. As for the branches of academic disciplines that they studied in the universities, 45% ( N = 291) were enrolled in Arts, 21.7% ( N = 140) in Engineering, 30.2% ( N = 195) in Management, and 3.1% ( N = 20) in Medicine.
Achievement motivation was measured with the Chinese version of The Achievement Motive Scale ( Gjesme and Nygard, 1970 ). It originally had two dimensions: hope for success and fear of failure. Previous studies found that The Achievement Motive Scale with 30 items was unable to guarantee an acceptable fit to a two-dimension model ( Lang and Fries, 2006 ). The current study ultimately kept the factor of “hope for success” with seven items after conducting exploratory factor analysis (EFA) and confirmatory factor analysis (CFA).
The present research adopted the Chinese version of The Morgan-Jinks Student Efficacy Scale ( Jinks and Morgan, 1999 ) to measure the construct of self-efficacy of college students. Two dimensions were included in this study: effort and context. Various studies have proved the validity and reliability of the scale in measuring students’ self-efficacy ( Magogwe and Oliver, 2007 ).
College Students’ Employability Scale ( He, 2019 ) was utilized to test undergraduates’ employability in this study. Four dimensions with 15 items in total were used: (1) application of knowledge; (2) teamwork; (3) communication and coordination; (4) self-learning; and (5) self-management.
Figure 1 illustrates the hypothesized research model.

Hypothesized research model.
Data analysis
This study conducted a three-step analysis of the data. First, SPSS 25.0 was utilized for explanatory factor analysis, descriptive analysis, correlation analysis between the studied variables, and regression analysis of the effect of the control variables. Second, AMOS 24.0 was used to conduct confirmatory factor analysis and path analysis to test the hypothesis. Third, PROCESS version 3.5 was adopted to test the mediating effect.
Common method bias test
A common method deviation can occur when data is collected by self-report scales. Harman single-factor method was adopted in this study to examine the items of achievement motivation, self-efficacy, academic performance, and employability in an exploratory factor analysis. The results showed that there were six factors with initial eigenvalues greater than 1, which explained 65.049% of variance. Only 19.544% of the variance was explained by the principal factor, which is far less than the threshold of 40% ( Liu et al., 2020 ). Therefore, Harman single-factor test indicated that common method bias was unlikely to be concerned in the present study.
Validity and reliability of the scale
The overall scale and subscales used in the present study showed good reliability and validity. The values of Cronbach’s α and McDonald’s omega were used to evaluate the internal consistency of scales. The generally accepted threshold for the Cronbach’s α coefficient states that 0.9 ≤ α is deemed excellent, 0.7 ≤ α < 0.9 good, 0.6 ≤ α < 0.7 acceptable; 0.5 ≤ α < 0.6 poor; α < 0.5 unacceptable ( Kulthanan et al., 2019 ). The Cronbach’s α value of the overall scale is 0.927. Table 1 shows that the values of each variable are greater than 0.8 and that of each subfactor greater than 0.7. The overall AM scale had a good reliability with Cronbach’s α coefficient of 0.862. The Cronbach’s α value of SE was 0.751, and that of its two subfactors are greater than 0.7. The entire EMP scale had an excellent reliability because the Cronbach’s α coefficient reached 0.950 and its five factors were all greater than 0.7. Values of McDonald’s Omega greater than 0.7 were deemed acceptable ( Sharif Nia et al., 2018 ). As shown in Table 1 , the coefficients of McDonald’s Omega were all greater than 0.7 except for the SMG because this dimension only had two items after CFA, while McDonald’s Omega estimate requires at least three items.
Reliability and validity of the scale.
AM, achievement motivation; SE, self-efficacy; EF, effort; CON, context; AP, academic performance; EMP, employability; AK, application of knowledge; TM, teamwork; CC, communication and coordination; SL, self-learning; SMG, self-management.
* a Omega cannot be estimated because the number of items for this dimension is 2.
In order to determine the construct validity, both convergent and discriminant validity were examined. A strong indication of convergent validity can be found when the average variance extracted (AVE) is at least 0.5 ( Azizi and Khatony, 2019 ) as well as the composite reliability (CR) being greater than 0.7 ( Kheirollahpour et al., 2020 ). Table 1 shows that values of AVE for each scale were greater than 0.5 and CR values are all above 0.8, which indicated a good convergent validity. A construct needs a higher AVE square root than the correlation coefficient between it and other constructs in order to have acceptable discriminate validity ( Fornell and Larcker, 1981 ). As shown in Table 2 , the square roots of achievement motivation, self-efficacy and employability are 0.715, 0.741 and 0.738, respectively. All constructs in this study had an AVE square root greater than their correlation with other constructs.
Discriminate validity of the scale.
Differences in demographic variables
The demographic variables in this study included gender, family residence, and discipline. Independent-samples T-tests and One-way ANOVA were performed concerning achievement motivation, self-efficacy, academic performance, and employability among the participated final-year undergraduates.
Table 3 shows that self-efficacy scores of male students were significantly higher ( M = 3.29, SD = 0.51) than their female counterparts ( M = 3.15, SD = 0.44), t = 3.728, p < 0.001. Academic performance scores of the participants from urban families ( M = 3.54, SD = 0.67) are significantly higher than those from rural ones ( M = 3.37, SD = 0.67), t = 3.198, p < 0.01. The scores of employability were higher for those from urban families ( M = 3.85, SD = 0.58) than those from rural households ( M = 3.68, SD = 0.70), t = 3.282, p < 0.01. Employability scores of students majoring in engineering were higher ( M = 3.87, SD = 0.66) than those in arts ( M = 3.71, SD = 0.61), management ( M = 3.83, SD = 0.66), and medicine ( M = 3.51, SD = 0.60), F = 3.857, p < 0.01. Other than that, there were no significant differences in other variables between genders, family residences, and among different branches of academic discipline ( p > 0.05).
Basic description of the variables ( N = 646).
Correlations between the variables
As shown in Table 4 , the mean values of achievement motivation, self-efficacy, and employability and their subfactors of college students were all above 3. It suggested that the college students participated in this study basically had adequate levels of achievement motivation, self-efficacy, and employability. According to Table 4 , achievement motivation had a significant correlation with undergraduates’ self-efficacy and employability (including their respective subdimensions). A noteworthy result is that although the overall self-efficacy was significantly related to employability in general, the context factor of self-efficacy only had a significant association with the application of knowledge dimension of employability. No significant correlation existed between the context factor and the other four dimensions of college students’ employability, while the effort factor of self-efficacy had significant and positive correlation with overall employability and its subfactors. Academic performance had significant and positive relation with achievement motivation, self-efficacy, employability, and their subdimensions except the context factor of self-efficacy.
Means, standard deviations and correlations between variables.
Direct effect on employability
Before testing the hypotheses, this study conducted confirmatory factor analysis via AMOS 24.0 to examine the fitness of the proposed model. The results showed that the research model had a good fit ( χ 2 /df = 3.599, GFI = 0.891, CFI = 0.926, NFI = 0.901, TLI = 0.915, SRMR = 0.0574, RMSEA = 0.063). AMOS 24.0 was also utilized to conduct path analysis to test the proposed hypothesis. As shown in Table 5 , achievement motivation can positively predict employability at 0.001 level. Thus, H1 was supported. Achievement motivation can also positively predict self-efficacy at 0.001 level. Hence, H2 was supported. The path coefficient between self-efficacy and employability was not significant ( p = 0.074 > 0.05). Therefore, H3 was rejected. Achievement motivation was tested to have positive prediction on academic performance at 0.001 level. Hence, H5 was supported. Academic performance was identified to have a positive impact on employability at 0.001 level. Therefore, H6 was supported. Self-efficacy was found to have a significant prediction on academic performance at 0.01 level ( p = 0.009). Thus, H8 was supported.
Test results of the proposed hypothesis.
AM, achievement motivation; SE, self-efficacy; AP, academic performance; EMP, employability.
The regression analysis was conducted by SPSS Version 25.0. Gender and family residence were included as control variables in the regression equation. The result showed that achievement motivation of college students generated a significant and positive impact upon their employability after controlling the influence of the participants’ gender and family background ( β = 0.551, p < 0.001).
This research adopted PROCESS Version 3.5 to examine the mediating effect of self-efficacy and academic performance on the association between achievement motivation and employability of college students. Model 6 was selected in PROCESS with Bootstrap samples of 5,000. Bias Corrected for Bootstrap CI method was chosen and the level of confidence for all confidence intervals was set at 95%. A statistically significant mediating effect occurs if the interval between BootLLCI and BootULCI does not include 0 ( Preacher and Hayes, 2008 ).
After running the macro in SPSS 25.0, the following results were generated as shown in Table 6 . It demonstrated that the total indirect effect of the prediction of achievement motivation on undergraduates’ employability was 0.34. The indirect effect of self-efficacy on the relationship between achievement motivation and college students’ employability was 0.017. The 95% confidence interval included 0 (BootLLCI = −0.005, BootULCI = 0.046), which indicated that the mediating effect is not statistically significant. Hence, H4 was rejected. Self-efficacy did not play a mediating role in the relationship between achievement motivation and undergraduates’ employability. The indirect effect of self-efficacy and academic performance on the correlation between achievement motivation and employability was 0.019. The 95% confidence interval did not include 0 (BootLLCI = 0.005, BootULCI = 0.038), which confirmed a significant mediating effect. Thus, H9 was supported in this research. Self-efficacy and academic performance had a chain mediating effect on the correlation between achievement motivation and undergraduates’ employability. The indirect effect of academic performance on the correlation between achievement motivation and employability was 0.303.0 and was not included in the 95% confidence interval (BootLLCI = 0.247, BootULCI = 0.367), which identified a significant mediating effect. Hence, H7 was supported. Academic performance functioned as a mediator in the correlation between achievement motivation and undergraduates’ employability.
Mediating effect of self-efficacy and academic performance on the correlation between achievement motivation and employability.
Direct relationships
The current study confirmed that achievement motivation has a direct impact on employability of college students. The finding could provide theoretical and practical implications for both higher education providers and receivers. As for higher education administrators and teachers, they are propelled to pay high attention to students’ psychological factors to improve the overall quality of their graduates. Systematic integration of achievement motivation cultivation into educational plans and teaching curriculum to enhance students’ employability skills ( Laguna-Sánchez et al., 2020 ) emerges as an urgent mission for the sustainability of higher education. In terms of college students, they need to be aware of the significant and positive influence of psychological attributes on their employment prospects and personal growth. Even if they encountered setbacks in academic activities or daily life on campus, they should stay motivated to achieve planned goals and acquire necessary knowledge and skills instead of “lying flat” (a recently widespread phenomenon among Chinese young people that rejects hard working and constant competition; Gao et al., 2022 ).
Many previous studies revealed that self-efficacy and achievement motivation were significantly correlated, but most of the studies examined the prediction of self-efficacy on achievement motivation ( Liu et al., 2021 ; Huang et al., 2022 ). The present study added new evidence to the argument that achievement motivation had positive impact on self-efficacy at 0.001 level in the context of higher education. Students with intrinsic desire to attain achievements turned to be confident in their abilities in interacting with environmental factors and performing positive behaviors, which showed the valid application of social cognitive theory in China’s higher education sector.
Although a variety of prior studies found that self-efficacy had a significant and positive impact on employability of college students ( Chow et al., 2019 ; Wang et al., 2022 ), the current research showed that the prediction of self-efficacy on employability was not statistically significant, which echoed with the research result by Coetzee and Oosthuizen (2012) who found self-efficacy was not significantly related to employability among adult learners enrolled in the open distance learning programs. Self-efficacy scores of the samples were relatively low ( M = 3.20, SD = 0.47) in the current study, which indicated that the participants did not have much confidence in their devotion to studies and their capabilities to accomplish academic tasks. Two dimensions of self-efficacy were examined in the questionnaire survey. The mean score of “effort” dimension was 3.45, indicating that students generally believed that they were able to obtain satisfying grades when they worked hard. The “context” dimension had the lowest score ( M = 3. 06, SD = 0.55) among all the 11 variables. It revealed that the participants had doubts about the purpose of receiving higher education and felt unsatisfied with the amount of attention received from their teachers. This might be related to the growing employment pressure on college students since they had a lot of difficulties in securing a job or even became unemployed after graduation ( Wu et al., 2022 ). Furthermore, as a result of the expansion of enrollment of higher education in China ( He et al., 2020 ), teachers have to face a large number of students in one class and teach several classes in one semester. For example, the minimum class size in Panzhihua University in Sichuan Province is 60 for compulsory courses and 120 for optional courses. The teachers there are normally assigned four different classes in each semester. As a result, teachers were unable to give sufficient attention to each student. At the same time, this research found that the context factor of self-efficacy was only positively correlated with the “application of knowledge” dimension of employability. It was not significantly related to any other factor of undergraduates’ employability. The “context” mainly refers to the external factors that influence a person’s belief in their own ability ( Beck and Quinn, 2011 ). The findings of the current research revealed that students attached more importance to their “effort” in developing employability attributes. This could be partly explained by the advancement of information technology that enables students to have access to numerous educational resources ( Li et al., 2021 ) and borderless social networking ( Smirnov, 2019 ) across the world. As long as they are determined to develop skills, they would not have much impediment in reaching external educational resources.
Academic achievements that students obtained in college had a significant impact on their employability. On one hand, students with higher academic performance are often characterized by goal-orientation, perseverance in adversity, courage in taking daunting tasks, etc. ( Lerang et al., 2019 ; Lam and Zhou, 2022 ), which are also highly-valued qualities in workplace and critical factors to ensure sustainable career success. On the other hand, higher academic performance indicates sound mastery of specific knowledge and professional skills in the given field, which are basic requirements in job responsibilities. However, higher education institutions in China are undergoing dramatic transformation. Many of them are becoming application-oriented and giving strategic priority to work-integrated learning, integration of production and education, integration of occupational qualifications, etc. ( Zhang and Chen, 2022 ). As a result, they are running risk of attaching more importance to the needs from the industries than the actual academic performance of the students. The current study highlighted the significant and positive prediction of academic performance on undergraduates’ employability. It reminds the policy-makers and administrators to put the cultivation of students’ academic achievements at the first place to ensure the sustainable development of higher education.
Mediated relations
The test results showed self-efficacy did not serve as a mediator in the relationship between achievement motivation and employability among the participants in this study. Achievement motivation was tested to have a significant prediction on self-efficacy and employability, but self-efficacy did not significantly enhance the impact of achievement motivation on undergraduates’ employability. This could be the result of the above-mentioned generally low self-efficacy scores of the participants and their uncertainties about the future after graduation. In the meanwhile, students with high level of achievement motivation would naturally mobilize cognitive, social, intellectual, and emotional resources ( Zamroni et al., 2022 ) to attain set goals and become more employable in the labor market.
Academic performance was tested to mediate the relationship between achievement motivation and employability. College students are confronted with many challenges in their academic studies, campus life, and peer competition. Achievement motivation drives students to move forward in their learning activities, daily life, and acquisition of skills, which was confirmed to have a positive prediction on undergraduates’ employability in this study and previous research ( Kamaliah et al., 2018 ). Stronger achievement motivation could keep them in a healthy mental state and equip them with positive attitudes, which, in turn, strengthens their study effects and improves their academic performance. Academic achievements are closely related to knowledge and skills that students acquired in higher education institutions, which lays a solid foundation for their professional competencies and career success ( Thiele et al., 2018 ). As a result, academic performance enhanced the effect of achievement motivation on employability in the context of tertiary education.
Chain mediating relation
The present study showed that self-efficacy and academic performance jointly mediated the impact of achievement motivation and employability of college students from the mainland of China. Based on the social cognitive theory, behavior, cognition, and environment are interconnected and mutually determined ( Almuqrin and Mutambik, 2021 ). Students’ cognitive factors have a significant influence on their behavior. Their interactions with classmates, teachers, administrators, and other environmental factors reinforced their achievement motivation, self-efficacy, academic performance, and finally, the acquisition of necessary employability skills. Strong intrinsic motive to attain achievements stimulate positive self-efficacy and generate better academic outcomes, which effectively enhances their employability. The test results underlined the significance of developing students’ self-efficacy in the pedagogical endeavors in addition to enhancing their motive to succeed because self-efficacy determines individuals’ belief in performing behaviors and, in turn, affects their efforts and prospects in accomplishing specific tasks. When students are highly motivated and also believe in their ability to achieve the goals ( Yang et al., 2021 ), they would be equipped with strong employability skills that are most sought after by employers.
This study empirically examined the relationship between achievement motivation, self-efficacy, academic performance, and employability among undergraduates. Based on the previous findings in academic literature about employability, the present research proposed and tested the hypothesis by collecting data from different universities in China. The results showed that achievement motivation positively predicted self-efficacy, academic performance, and employability of college students. Self-efficacy was tested to have no significant impact on employability of the participants and play no significant mediating role in the correlation between achievement motivation and undergraduates’ employability, while academic performance significantly mediated such relationship. The findings showed that self-efficacy and academic performance played chain mediating roles in the prediction of achievement motivation on employability of college students. After controlling gender and family residence, achievement motivation still significantly and positively affects employability of college students.
The findings of this research add new understanding to the existing literature on college students’ employability. The study identified the chain mediating effect of self-efficacy and academic performance in the relationship between undergraduates’ achievement motivation and employability and found that self-efficacy had no significant direct and indirect effect on employability in this sample. It provides timely evidence that higher education institutions should give students’ academic performance top priority against the background of current application-orientation transformation. They also need to take immediate actions to make students realize the value and meaning of attending colleges to ensure the sustainability of higher education because students have insufficient confidence in finding employment after graduation. This study has several valuable implications for future practice of improving students’ employability for higher education providers since these graduates will be the most valuable assets of organizations, highly skilled knowledge workers in the labor market, and the main force in sustainable development.
Research limitations and future research directions
Although the study contributed to the existing literature on employability of college students in a number of theoretical and practical ways, it does have several limitations that need to be considered in future studies. Firstly, the latest official data from the Ministry of Education of China released on 30 September 2021 showed that there are 1, 270 higher education institutions in Chinese mainland offering bachelor’s degrees with 12 different academic disciplines. Only 9 public schools and 4 academic disciplines were surveyed in the current research due to the limited length of time and available contacts. When respondents were recruited from different types of universities and different branches of academic disciplines, the results might be different from those in this study. As a result, it would be necessary to test the results with a wider sample of participants in order to come to more general conclusions about the relationship between achievement motivation, self-efficacy, academic performance, and employability among university students. Secondly, the study examined the employability of college students in terms of subdimensions such as application of knowledge, teamwork, communication, coordination, self-learning, and self-management. Other potential dimensions of undergraduates’ employability were not yet paid attention to in the current analysis. Future studies are encouraged to examine other aspects of college students’ employability to gain a more comprehensive assessment. Thirdly, this research relies largely on questionnaire as its main research approach. A qualitative method might be helpful in revealing the profound correlation between the studied variables and provide valuable interpretation of the research findings from different perspectives.
Data availability statement
Ethics statement.
The studies involving human participants were reviewed and approved by the Institutional Ethics Committee of the School of Foreign Languages and Cultures at Panzhihua University (approval code: no. HRECA21-006 and date of approval: 8 November 2021). The patients/participants provided their informed consent to participate in this study.
Author contributions
RP: conceptualization. XL and RP: data curation, investigation, and methodology. RP and NP: supervision and writing–review and editing. XL: writing–original draft. All authors contributed to the article and approved the submitted version.
This research was funded by the Ph.D. Research Start-up Fund of Panzhihua University (no. 035200187).
Conflict of interest
The authors declare that the research was conducted in the absence of any commercial or financial relationships that could be construed as a potential conflict of interest.
Publisher’s note
All claims expressed in this article are solely those of the authors and do not necessarily represent those of their affiliated organizations, or those of the publisher, the editors and the reviewers. Any product that may be evaluated in this article, or claim that may be made by its manufacturer, is not guaranteed or endorsed by the publisher.
- Almuqrin A., Mutambik I. (2021). The explanatory power of social cognitive theory in determining knowledge sharing among Saudi faculty . PLoS One 16 :e0248275. doi: 10.1371/journal.pone.0248275, PMID: [ PMC free article ] [ PubMed ] [ CrossRef ] [ Google Scholar ]
- Assari S. (2019). Education attainment and obesity: differential returns based on sexual orientation . Behav. Sci. 9 :16. doi: 10.3390/bs9020016, PMID: [ PMC free article ] [ PubMed ] [ CrossRef ] [ Google Scholar ]
- Azizi S. M., Khatony A. (2019). Investigating factors affecting on medical sciences students' intention to adopt mobile learning . BMC Med. Educ. 19 :381. doi: 10.1186/s12909-019-1831-4, PMID: [ PMC free article ] [ PubMed ] [ CrossRef ] [ Google Scholar ]
- Bandura A. (1977). Self-efficacy: toward a unifying theory of behavioral change . Psychol. Rev. 84 , 191–215. doi: 10.1037/0033-295X.84.2.191, PMID: [ PubMed ] [ CrossRef ] [ Google Scholar ]
- Bandura A. (1986). The explanatory and predictive scope of self-efficacy theory . J. Soc. Clin. Psychol. 4 , 359–373. doi: 10.1521/jscp.1986.4.3.359 [ CrossRef ] [ Google Scholar ]
- Bandura A. (1997). Self-efficacy: The Exercise of Control . New York, NY: Freeman. [ Google Scholar ]
- Beck V., Quinn M. (2011). The importance of health in promoting employability in the east midlands . Sociol. Res. Online 16 , 9–20. doi: 10.5153/sro.2248 [ CrossRef ] [ Google Scholar ]
- Byrne C. (2022). What determines perceived graduate employability? Exploring the effects of personal characteristics, academic achievements and graduate skills in a survey experiment . Stud. High. Educ. 47 , 159–176. doi: 10.1080/03075079.2020.1735329 [ CrossRef ] [ Google Scholar ]
- Chamorro-Premuzic T., Furnham A. (2003). Personality predicts academic performance: evidence from two longitudinal university samples . J. Res. Pers. 37 , 319–338. doi: 10.1016/S0092-6566(02)00578-0 [ CrossRef ] [ Google Scholar ]
- Chang Y., Tsai Y. (2022). The effect of university students' emotional intelligence, learning motivation and self-efficacy on their academic achievement-online English courses . Front. Psychol. 13 :818929. doi: 10.3389/fpsyg.2022.818929, PMID: [ PMC free article ] [ PubMed ] [ CrossRef ] [ Google Scholar ]
- Chhinzer N., Russo A. M. (2018). An exploration of employer perceptions of graduate student employability . Education + Training 60 , 104–120. doi: 10.1108/ET-06-2016-0111 [ CrossRef ] [ Google Scholar ]
- Chiang C. Y., Choi K. C., Ho K. M., Yu S. F. (2018). Effectiveness of nurse-led patient-centered care behavioral risk modification on secondary prevention of coronary heart disease: a systematic review . Int. J. Nurs. Stud. 84 , 28–39. doi: 10.1016/j.ijnurstu.2018.04.012, PMID: [ PubMed ] [ CrossRef ] [ Google Scholar ]
- Choi N. (2005). Self-efficacy and self-concept as predictors of college students' academic performance . Psychol. Sch. 42 , 197–205. doi: 10.1002/pits.20048 [ CrossRef ] [ Google Scholar ]
- Chow H. J., Wong S. C., Lim C. S. (2019). Examining mediating role of self-efficacy on undergraduates' perceived employability . Int. J. Acad. Res. Bus. Social Sci. 9 , 135–158. doi: 10.6007/IJARBSS/v9-i6/5929 [ CrossRef ] [ Google Scholar ]
- Chughtai A. (2018). Authentic leadership, career self-efficacy and career success: a cross-sectional study . Career Dev. Int. 23 , 595–607. doi: 10.1108/CDI-05-2018-0160 [ CrossRef ] [ Google Scholar ]
- Cifre E., Vera M., Sánchez-Cardona I., de Cuyper N. (2018). Sex, gender identity, and perceived employability among Spanish employed and unemployed youngsters . Front. Psychol. 9 :2467. doi: 10.3389/fpsyg.2018.02467, PMID: [ PMC free article ] [ PubMed ] [ CrossRef ] [ Google Scholar ]
- Coetzee M., Oosthuizen R. M. (2012). Students' sense of coherence, study engagement and self-efficacy in relation to their study and employability satisfaction . J. Psychol. Afr. 22 , 315–322. doi: 10.1080/14330237.2012.10820536 [ CrossRef ] [ Google Scholar ]
- Dacre Pool L., Sewell P. (2007). The key to employability: developing a practical model of graduate employability . Education + Training 49 , 277–289. doi: 10.1108/00400910710754435 [ CrossRef ] [ Google Scholar ]
- Damrongpanit S. (2019). From modern teaching to mathematics achievement: the mediating role of mathematics attitude, achievement motivation, and self-efficacy . Eur. J. Educ. Res. 8 , 713–727. doi: 10.12973/eu-jer.8.3.713 [ CrossRef ] [ Google Scholar ]
- Daniels L. M., Dueck B. S. (2022). Integrative and theoretical reviews of achievement motivation for school psychologists: introduction to the special issue . Can. J. Sch. Psychol. 37 , 3–22. doi: 10.1177/08295735211055333 [ CrossRef ] [ Google Scholar ]
- De Cuyper N., De Witte H. (2011). The management paradox: self-rated employability and organizational commitment and performance . Pers. Rev. 40 , 152–172. doi: 10.1108/00483481111106057 [ CrossRef ] [ Google Scholar ]
- Diao X. (2015). Zhi ye jue ce shi ye xia pin kun da xue sheng zhi ye sheng ya fu dao mo shi yan jiu [research on career counseling model for poor college students in the perspective of career decision-making] . China Adult Educ. , 41–43. doi: 10.16128/j.cnki.1005-3611.2013.01.028 [ CrossRef ] [ Google Scholar ]
- Donald W. E., Baruch Y., Ashleigh M. J. (2019). Striving for sustainable graduate careers . Career Dev. Int. 25 , 90–110. doi: 10.1108/CDI-03-2019-0079 [ CrossRef ] [ Google Scholar ]
- Dong Y., Shi H., Han Z., Zhao H., Chen J. (2019). Environmental study on participant behavior and employability of tourism majored undergraduates: taking academic achievement as the intermediary . Ekoloji 28 , 4405–4412. [ Google Scholar ]
- Downes P. E., Crawford E. R., Seibert S. E., Stoverink A. C., Campbell E. M. (2021). Referents or role models? The self-efficacy and job performance effects of perceiving higher performing peers . J. Appl. Psychol. 106 , 422–438. doi: 10.1037/apl0000519 [ PubMed ] [ CrossRef ] [ Google Scholar ]
- Eakman A. M., Kinney A. R., Schierl M. L., Henry K. L. (2019). Academic performance in student service members/veterans: effects of instructor autonomy support, academic self-efficacy and academic problems . Educ. Psychol. 39 , 1005–1026. doi: 10.1080/01443410.2019.1605048 [ CrossRef ] [ Google Scholar ]
- Endalamaw Yigermal M. (2017). The determinants of academic performance of under graduate students: in the case of Arba Minch University Chamo campus . Adv. Sci. Humanities 3 , 35–42. doi: 10.11648/j.ash.20170304.12 [ CrossRef ] [ Google Scholar ]
- Fornell C., Larcker D. F. (1981). Evaluating structural equation models with unobservable variables and measurement error . J. Mark. Res. 18 , 39–50. doi: 10.1177/002224378101800104 [ CrossRef ] [ Google Scholar ]
- Gamazo A., Martínez-Abad F. (2020). An exploration of factors linked to academic performance in PISA 2018 through data mining techniques . Front. Psychol. 11 :575167. doi: 10.3389/fpsyg.2020.575167, PMID: [ PMC free article ] [ PubMed ] [ CrossRef ] [ Google Scholar ]
- Gao R., He T., Liao Y., Liu X., Fan Y., Su Y., et al.. (2022). An investigation on the academic burden of Chinese students ranging from primary schools to universities based on a word association test in Guangdong province . Int. J. Environ. Res. Public Health 19 :2481. doi: 10.3390/ijerph19042481, PMID: [ PMC free article ] [ PubMed ] [ CrossRef ] [ Google Scholar ]
- Gatzka T., Hell B. (2018). Openness and postsecondary academic performance: a meta-analysis of facet-, aspect-, and dimension-level correlations . J. Educ. Psychol. 110 , 355–377. doi: 10.1037/edu0000194 [ CrossRef ] [ Google Scholar ]
- Gjesme T., Nygard R. (1970). Achievement-Related Motives: Theoretical Considerations and Construction of a Measuring Instrument Pedagogisk forskningsinstitutt, University of Oslo, Oslo, Norway. [ Google Scholar ]
- González-Romá V., Gamboa J. P., Peiró J. M. (2018). University graduates' employability, employment status, and job quality . J. Career Dev. 45 , 132–149. doi: 10.1177/0894845316671607 [ CrossRef ] [ Google Scholar ]
- Harvey L. (2001). Defining and measuring employability . Qual. High. Educ. 7 , 97–109. doi: 10.1080/13538320120059990 [ CrossRef ] [ Google Scholar ]
- He J. (2019). Research on the Impact of the Integration of Innovation Entrepreneurship Education with Professional Education on the Employability of College Students. Master . Xiangtan University; Xiangtan. [ Google Scholar ]
- He Y., Pei Y., Ran B., Kang J., Song Y. (2020). Analysis on the higher education sustainability in China based on the comparison between universities in China and America . Sustainability 12 :573. doi: 10.3390/su12020573 [ CrossRef ] [ Google Scholar ]
- Huang X., Wang C., Hua L., Gao Y., Zhou S., Wang X. (2022). Social context, self-efficacy, and patient-centered service behavior of medical professionals: the moderating role of achievement motivation . Front. Psych. 13 :784228. doi: 10.3389/fpsyt.2022.784228, PMID: [ PMC free article ] [ PubMed ] [ CrossRef ] [ Google Scholar ]
- Ingram N., Allen K. (2019). Talent-spotting or social magic? Inequality, cultural sorting and constructions of the ideal graduate in elite professions . Sociol. Rev. 67 , 723–740. doi: 10.1177/0038026118790949 [ CrossRef ] [ Google Scholar ]
- Ishihara T., Morita N., Nakajima T., Okita K., Sagawa M., Yamatsu K. (2018). Modeling relationships of achievement motivation and physical fitness with academic performance in Japanese schoolchildren: moderation by gender . Physiol. Behav. 194 , 66–72. doi: 10.1016/j.physbeh.2018.04.031, PMID: [ PubMed ] [ CrossRef ] [ Google Scholar ]
- Jan S. U., Anwar M. A., Warraich N. F. (2020). The relationship between emotional intelligence, library anxiety, and academic achievement among the university students . J. Librariansh. Inf. Sci. 52 , 237–248. doi: 10.1177/0961000618790629 [ CrossRef ] [ Google Scholar ]
- Jinks J., Morgan V. (1999). Children's perceived academic self-efficacy: an inventory scale . Clearing House 72 , 224–230. doi: 10.1080/00098659909599398 [ CrossRef ] [ Google Scholar ]
- Kamaliah S., Roslan S., Bakar A. R., Ghiami Z. (2018). The effect of supervised work experience on the acquisition of employability skills among Malaysian students . Higher Educ. Skills Work-Based Learn. 8 , 354–364. doi: 10.1108/HESWBL-05-2016-0028 [ CrossRef ] [ Google Scholar ]
- Kapasi A., Pei J. (2022). Mindset theory and school psychology . Can. J. Sch. Psychol. 37 , 57–74. doi: 10.1177/08295735211053961 [ CrossRef ] [ Google Scholar ]
- Kheirollahpour M. M., Danaee M. M., Merican A. F. A. F., Shariff A. A. A. A. (2020). Prediction of the influential factors on eating behaviors: a hybrid model of structural equation modelling-artificial neural networks . Sci. World J. 2020 , 1–12. doi: 10.1155/2020/4194293, PMID: [ PMC free article ] [ PubMed ] [ CrossRef ] [ Google Scholar ]
- Kulthanan K., Chularojanamontri L., Rujitharanawong C., Weerasubpong P., Weller K., Maurer M. (2019). Angioedema activity score (AAS): a valid and reliable tool to ese in Asian patients . Biomed. Res. Int. 2019 :9157895. doi: 10.1155/2019/9157895, PMID: [ PMC free article ] [ PubMed ] [ CrossRef ] [ Google Scholar ]
- Laguna-Sánchez P., Abad P., de la Fuente-Cabrero C., Calero R. (2020). A university training programme for acquiring entrepreneurial and transversal employability skills, a students' assessment . Sustainability 12 :796. doi: 10.3390/su12030796 [ CrossRef ] [ Google Scholar ]
- Lam K. K. L., Zhou M. (2022). Grit and academic achievement: a comparative cross-cultural meta-analysis . J. Educ. Psychol. 114 , 597–621. doi: 10.1037/edu0000699 [ CrossRef ] [ Google Scholar ]
- Lang J. W. B., Fries S. (2006). A revised 10-item version of the achievement motives scale . Eur. J. Psychol. Assess. 22 , 216–224. doi: 10.1027/1015-5759.22.3.216 [ CrossRef ] [ Google Scholar ]
- Lee C. G., Park S., Lee S. H., Kim H., Park J. (2018). Social cognitive theory and physical activity among Korean male high-school students . Am. J. Mens Health 12 , 973–980. doi: 10.1177/1557988318754572, PMID: [ PMC free article ] [ PubMed ] [ CrossRef ] [ Google Scholar ]
- Lerang M. S., Ertesvåg S. K., Havik T. (2019). Perceived classroom interaction, goal orientation and their association with social and academic learning outcomes . Scand. J. Educ. Res. 63 , 913–934. doi: 10.1080/00313831.2018.1466358 [ CrossRef ] [ Google Scholar ]
- Leslie E., Wilson R. (2020). Sheltering in place and domestic violence: evidence from calls for service during COVID-19 . J. Public Econ. 189 :104241. doi: 10.1016/j.jpubeco.2020.104241, PMID: [ PMC free article ] [ PubMed ] [ CrossRef ] [ Google Scholar ]
- Li G. Z., Li L. Z. (2018). Achievement motivation, self-efficacy and entrepreneurial intention: the case of minority area's college students in China . Korean-Chinese Social Sci. Stud. 16 , 123–145. doi: 10.36527/KCSSS.16.3.5 [ CrossRef ] [ Google Scholar ]
- Li C., Zhao Q., Herencsar N., Srivastava G. (2021). The design of mobile distance online education resource sharing from the perspective of man-machine cooperation . Mobile Networks Appl. 26 , 2141–2152. doi: 10.1007/s11036-021-01770-0 [ CrossRef ] [ Google Scholar ]
- Lian R., Cai W., Chen K., Shen H., Gao X., Xu B., et al.. (2021). Linking mentoring and job search behavior: a moderated mediation model . SAGE Open 11 :215824402110672. doi: 10.1177/21582440211067235 [ CrossRef ] [ Google Scholar ]
- Liu G., Cheng G., Hu J., Pan Y., Zhao S. (2020). Academic self-efficacy and postgraduate procrastination: a moderated mediation model . Front. Psychol. 11 :1752. doi: 10.3389/fpsyg.2020.01752, PMID: [ PMC free article ] [ PubMed ] [ CrossRef ] [ Google Scholar ]
- Liu X., Gao X., Ping S. (2019). Post-1990s college students academic sustainability: the role of negative emotions, achievement goals, and self-efficacy on academic performance . Sustainability 11 :775. doi: 10.3390/su11030775 [ CrossRef ] [ Google Scholar ]
- Liu S., Hong Z., Zhou W., Fang Y., Zhang L. (2021). Job-search self-efficacy and reemployment willingness among older adults: roles of achievement motivation and age . BMC Geriatr. 21 :683. doi: 10.1186/s12877-021-02645-5, PMID: [ PMC free article ] [ PubMed ] [ CrossRef ] [ Google Scholar ]
- Lou N. M. (2021). Acculturation in a postcolonial context: language, identity, cultural adaptation, and academic achievement of Macao students in mainland China . Int. J. Intercult. Relat. 85 , 213–225. doi: 10.1016/j.ijintrel.2021.10.004 [ CrossRef ] [ Google Scholar ]
- Luthans F. (2002). Positive organizational behavior: developing and managing psychological strengths . Acad. Manag. Perspect. 16 , 57–72. doi: 10.5465/ame.2002.6640181 [ CrossRef ] [ Google Scholar ]
- Macakova V., Wood C. (2022). The relationship between academic achievement, self-efficacy, implicit theories and basic psychological needs satisfaction among university students . Stud. High. Educ. 47 , 259–269. doi: 10.1080/03075079.2020.1739017 [ CrossRef ] [ Google Scholar ]
- Magogwe J. M., Oliver R. (2007). The relationship between language learning strategies, proficiency, age and self-efficacy beliefs: a study of language learners in Botswana . System 35 , 338–352. doi: 10.1016/j.system.2007.01.003 [ CrossRef ] [ Google Scholar ]
- Mamaqi X., Miguel J., Olave P. (2011). The relationship between employability and training . World Acad. Sci. Eng. Technol. 5 , 656–660. doi: 10.5281/zenodo.1330509 [ CrossRef ] [ Google Scholar ]
- Manzano-Sanchez H., Outley C., Gonzalez J. E., Matarrita-Cascante D. (2018). The influence of self-efficacy beliefs in the academic performance of Latina/o students in the United States: a systematic literature review . Hisp. J. Behav. Sci. 40 , 176–209. doi: 10.1177/0739986318761323 [ CrossRef ] [ Google Scholar ]
- Millican J., Bourner T. (2011). Student-community engagement and the changing role and context of higher education . Education + Training 53 , 89–99. doi: 10.1108/00400911111115645 [ CrossRef ] [ Google Scholar ]
- Misra R. K., Mishra P. (2016). Entrepreneurial attitude orientation and employability: mediating role of entrepreneurial personality . Int. J. Manag. Pract. 9 , 74–92. doi: 10.1504/IJMP.2016.074890 [ CrossRef ] [ Google Scholar ]
- Mornar M., Marušić I., Šabić J. (2022). Academic self-efficacy and learning strategies as mediators of the relation between personality and elementary school students' achievement . Eur. J. Psychol. Educ. [ahead of print]. doi: 10.1007/s10212-021-00576-8 [ CrossRef ] [ Google Scholar ]
- Muenks K., Yang J. S., Wigfield A. (2018). Associations between grit, motivation, and achievement in high school students . Motiv. Sci. 4 , 158–176. doi: 10.1037/mot0000076 [ CrossRef ] [ Google Scholar ]
- Nimmi P. M., Donald W. E. (2022). Modelling the interaction between serious leisure, self-perceived employability, stress, and workplace well-being: empirical insights from graduates in India . Personnel Rev. [ahead of print]. doi: 10.1108/PR-04-2021-0305 [ CrossRef ] [ Google Scholar ]
- Nofrizal N., Nirwana H., Alizamar A. (2020). The contribution of parents attention to student achievement motivation . J. Educ. Learn. Stud. 3 , 55–63. doi: 10.32698/0982 [ CrossRef ] [ Google Scholar ]
- O’Connor M. C., Paunonen S. V. (2007). Big five personality predictors of post-secondary academic performance . Personal. Individ. Differ. 43 , 971–990. doi: 10.1016/j.paid.2007.03.017 [ CrossRef ] [ Google Scholar ]
- Ouyang Y., Wang K., Zhang T., Peng L., Song G., Luo J. (2020). The influence of sports participation on body image, self-efficacy, and self-esteem in college students . Front. Psychol. 10 :3039. doi: 10.3389/fpsyg.2019.03039, PMID: [ PMC free article ] [ PubMed ] [ CrossRef ] [ Google Scholar ]
- Owusu-Agyeman Y., Fourie-Malherbe M. (2019). Students as partners in the promotion of civic engagement in higher education . Stud. High. Educ. 46 , 1241–1255. doi: 10.1080/03075079.2019.1666263 [ CrossRef ] [ Google Scholar ]
- Peng M. Y., Wang L., Yue X., Xu Y., Feng Y. (2021). A study on the influence of multi-teaching strategy intervention program on college students' absorptive capacity and employability . Front. Psychol. 12 :631958. doi: 10.3389/fpsyg.2021.631958, PMID: [ PMC free article ] [ PubMed ] [ CrossRef ] [ Google Scholar ]
- Pinto L. H., He K. (2017). “in the eyes of the beholder”: the influence of academic performance and extracurricular activities on the perceived employability of Chinese business graduates . Asia Pac. J. Hum. Resour. 57 , 503–527. doi: 10.1111/1744-7941.12200 [ CrossRef ] [ Google Scholar ]
- Poropat A. E. (2009). A meta-analysis of the five-factor model of personality and academic performance . Psychol. Bull. 135 , 322–338. doi: 10.1037/a0014996, PMID: [ PubMed ] [ CrossRef ] [ Google Scholar ]
- Preacher K. J., Hayes A. F. (2008). Asymptotic and resampling strategies for assessing and comparing indirect effects in multiple mediator models . Behav. Res. Methods 40 , 879–891. doi: 10.3758/BRM.40.3.879, PMID: [ PubMed ] [ CrossRef ] [ Google Scholar ]
- Rui Y., Nan N., Mei L.. (2011). “Study on the relationship between achievement motivation and self-efficacy of college students major in physical education,” in 2nd International Conference on Information, Communication and Education Application (ICEA 2011), Shanghai, Peoples R China .
- Sabti A. A., Md Rashid S., Nimehchisalem V., Darmi R. (2019). The impact of writing anxiety, writing achievement motivation, and writing self-efficacy on writing performance: a correlational study of Iraqi tertiary EFL learners . SAGE Open 9 :1999601868. doi: 10.1177/2158244019894289 [ CrossRef ] [ Google Scholar ]
- Schreuder D., Coetzee M. (2011). The relation between career anchors, emotional intelligence and employability satisfaction among workers in the service industry . South. Afr. Bus. Rev. 15 , 76–97. [ Google Scholar ]
- Sharif Nia H., Pahlevan Sharif S., Boyle C., Yaghoobzadeh A., Tahmasbi B., Rassool G. H., et al.. (2018). The factor structure of the spiritual well-being scale in veterans experienced chemical weapon exposure . J. Relig. Health 57 , 596–608. doi: 10.1007/s10943-017-0458-1 [ PubMed ] [ CrossRef ] [ Google Scholar ]
- She H. C., Lin H. S., Huang L. Y. (2019). Reflections on and implications of the Programme for international student assessment 2015 (PISA 2015) performance of students in Taiwan: the role of epistemic beliefs about science in scientific literacy . J. Res. Sci. Teach. 56 , 1309–1340. doi: 10.1002/tea.21553 [ CrossRef ] [ Google Scholar ]
- Shoval E., Shachaf M., Ramati-Dvir O., Shulruf B. (2021). Gender matters when sports engagement and self-efficacy interact with academic achievement . Soc. Psychol. Educ. 24 , 75–94. doi: 10.1007/s11218-020-09598-4 [ CrossRef ] [ Google Scholar ]
- Smirnov I. (2019). Schools are segregated by educational outcomes in the digital space . PLoS One 14 :e217142: e0217142. doi: 10.1371/journal.pone.0217142, PMID: [ PMC free article ] [ PubMed ] [ CrossRef ] [ Google Scholar ]
- Smith R. L., Karaman M. A., Balkin R. S., Talwar S. (2019). Psychometric properties and factor analyses of the achievement motivation measure . Br. J. Guidance Counselling 48 , 418–429. doi: 10.1080/03069885.2019.1620173 [ CrossRef ] [ Google Scholar ]
- Stajkovic A. D., Bandura A., Locke E. A., Lee D., Sergent K. (2018). Test of three conceptual models of influence of the big five personality traits and self-efficacy on academic performance: a meta-analytic path-analysis . Personal. Individ. Differ. 120 , 238–245. doi: 10.1016/j.paid.2017.08.014 [ CrossRef ] [ Google Scholar ]
- Steinmayr R., Weidinger A. F., Schwinger M., Spinath B. (2019). The importance of students' motivation for their academic achievement-replicating and extending previous findings . Front. Psychol. 10 :1730. doi: 10.3389/fpsyg.2019.01730, PMID: [ PMC free article ] [ PubMed ] [ CrossRef ] [ Google Scholar ]
- Suny I., Suardiman S. P. (2019). “Is modelling technique effective to improve achievement motivation of junior high school students,” in International Conference on Meaningful Education (ICMED), Yogyakarta, Indonesia .
- Tanjung I. B., Musa S. (2021). The impact of achievement motivation on organizational capabilities and firm performance in the creative industry context . South East Asian J. Manage. 15 , 235–250. doi: 10.21002/seam.v15i2.13602 [ CrossRef ] [ Google Scholar ]
- Tentama F., Abdillah M. H. (2019). Student employability examined from academic achievement and self-concept . Int. J. Eval. Res. Educ. 8 , 243–248. doi: 10.11591/ijere.v8i2.18128 [ CrossRef ] [ Google Scholar ]
- Thiele L., Sauer N. C., Kauffeld S. (2018). Why extraversion is not enough: the mediating role of initial peer network centrality linking personality to long-term academic performance . High. Educ. 76 , 789–805. doi: 10.1007/s10734-018-0242-5 [ CrossRef ] [ Google Scholar ]
- Torres-Roman J. S., Cruz-Avila Y., Suarez-Osorio K., Arce-Huamaní M. Á., Menez-Sanchez A., Aveiro-Róbalo T. R., et al.. (2018). Motivation towards medical career choice and academic performance in Latin American medical students: a cross-sectional study . PLoS One 13 :e205674. doi: 10.1371/journal.pone.0205674, PMID: [ PMC free article ] [ PubMed ] [ CrossRef ] [ Google Scholar ]
- Volenec Z. M., Abraham J. O., Becker A. D., Dobson A. P. (2021). Public parks and the pandemic: how park usage has been affected by COVID-19 policies . PLoS One 16 :e251799. doi: 10.1371/journal.pone.0251799, PMID: [ PMC free article ] [ PubMed ] [ CrossRef ] [ Google Scholar ]
- Wang Q., Aman M. S., Hooi L. B. (2021). Exploring talent cultivation of college student-athletes for new ventures and entrepreneurial psychology of new venture entrepreneur . Front. Psychol. , 12 :679901. doi: 10.3389/fpsyg.2021.679901, MID: [ PMC free article ] [ PubMed ] [ CrossRef ] [ Google Scholar ]
- Wang M., Guo J., Degol J. L. (2020). The role of sociocultural factors in student achievement motivation: a cross-cultural review . Adolesc. Res. Rev. 5 , 435–450. doi: 10.1007/s40894-019-00124-y [ CrossRef ] [ Google Scholar ]
- Wang D., Guo D., Song C., Hao L., Qiao Z. (2022). General self-efficacy and employability among financially underprivileged Chinese college students: the mediating role of achievement motivation and career aspirations . Front. Psychol. 12 :719771. doi: 10.3389/fpsyg.2021.719771, PMID: [ PMC free article ] [ PubMed ] [ CrossRef ] [ Google Scholar ]
- Wei Y., Cai T., Xiang G., Tan X. (2013). Achievement motivation, career decision-making self-efficacy and career maturity of college students . Chin. J. Clin. Psych. 21 , 143–145. doi: 10.16128/j.cnki.1005-3611.2013.01.028 [ CrossRef ] [ Google Scholar ]
- Werdhiastutie A., Suhariadi F., Partiwi S. G. (2020). Achievement motivation as antecedents of quality improvement of organizational human resources . Budapest Int. Res. Critics Inst. Humanities Social Sci. 3 , 747–752. doi: 10.33258/birci.v3i2.886 [ CrossRef ] [ Google Scholar ]
- White M., Becker J., du Plessis M. (2021). Unintended positive consequences of development centres in university graduates . Front. Psychol. 12 :775377. doi: 10.3389/fpsyg.2021.775377, PMID: [ PMC free article ] [ PubMed ] [ CrossRef ] [ Google Scholar ]
- Wu M., Hao X., Tian Y. (2022). Employment management policies for college graduates under COVID-19 in China: diffusion characteristics and core issues . Healthcare 10 :955. doi: 10.3390/healthcare10050955, PMID: [ PMC free article ] [ PubMed ] [ CrossRef ] [ Google Scholar ]
- Yang X. (2010). Access to higher education for rural-poor students in China . Educ. Res. Policy Prac. 9 , 193–209. doi: 10.1007/s10671-010-9084-3 [ CrossRef ] [ Google Scholar ]
- Yang K., Wu H., Yeh Y., Lin K., Wu J., Hsu Y. (2021). Implementers, designers, and disseminators of integrated STEM activities: self-efficacy and commitment . Res. Sci. Technol. Educ 1-19 . [ahead of print], 1–19. doi: 10.1080/02635143.2021.2008343 [ CrossRef ] [ Google Scholar ]
- Yawson D. E., Yamoah F. A. (2020). Understanding pedagogical essentials of employability embedded curricula for business school undergraduates: a multi-generational cohort perspective . Higher Educ. Pedagogies 5 , 360–380. doi: 10.1080/23752696.2020.1846134 [ CrossRef ] [ Google Scholar ]
- Ye R. M., Hagtvet K. (1992). Cheng jiu dong ji de ce liang yu fen xi [measurement and analysis of achievement motivation] . Psychol. Dev. Educ. 3 , 14–16. [ Google Scholar ]
- Yeager D. S., Hanselman P., Walton G. M., Murray J. S., Crosnoe R., Muller C., et al.. (2019). A national experiment reveals where a growth mindset improves achievement . Nature 573 , 364–369. doi: 10.1038/s41586-019-1466-y, PMID: [ PMC free article ] [ PubMed ] [ CrossRef ] [ Google Scholar ]
- Yokoyama S. (2019). Academic self-efficacy and academic performance in online learning: a mini review . Front. Psychol. 9 :2794. doi: 10.3389/fpsyg.2018.02794, PMID: [ PMC free article ] [ PubMed ] [ CrossRef ] [ Google Scholar ]
- Yorke M. (2006). Employability in Higher Education: What it is-What it is not . York: Higher Education Academy. [ Google Scholar ]
- Zamroni E., Awlawi A. H., Kasau M. N. R., Kholik, Usman M. (2022). Achievement motivation, emotional intelligence and self-regulation and its impact on student academic resilience in the covid-19 pandemic era: a path analysis . J. Legal Ethical Regulatory Issues 25 , 1–8. [ Google Scholar ]
- Zhang Y., Chen X. (2022). “ Application-oriented higher education in China, ” in Application-Oriented Higher Education: East-West Crosscurrents in Higher Education (Singapore: Springer; ). [ Google Scholar ]
2024 Theses Doctoral
Artificial Intelligence vs. Human Coaches: A Mixed Methods Randomized Controlled Experiment on Client Experiences and Outcomes
Barger, Amber
The rise of artificial intelligence (AI) challenges us to explore whether human-to-human relationships can extend to AI, potentially reshaping the future of coaching. The purpose of this study was to examine client perceptions of being coached by a simulated AI coach, who was embodied as a vocally conversational live-motion avatar, compared to client perceptions of a human coach. It explored if and how client ratings of coaching process measures and outcome measures aligned between the two coach treatments. In this mixed methods randomized controlled trial (RCT), 81 graduate students enrolled in the study and identified a personally relevant goal to pursue. The study deployed an alternative-treatments between-subjects design, with one-third of participants receiving coaching from simulated AI coaches, another third engaging with seasoned human coaches, and the rest forming the control group. Both treatment groups had one 60-minute session guided by the CLEAR (contract, listen, explore, action, review) coaching model to support each person to gain clarity about their goal and identify specific behaviors that could help each make progress towards their goal. Quantitative data were captured through three surveys and qualitative input was captured through open-ended survey questions and 27 debrief interviews. The study utilized a Wizard of Oz technique from human-computer interaction research, ingeniously designed to sidestep the rapid obsolescence of technology by simulating an advanced AI coaching experience where participants unknowingly interacted with professional human coaches, enabling the assessment of responses to AI coaching in the absence of fully developed autonomous AI systems. The aim was to glean insights into client reactions to a future, fully autonomous AI with the expert capabilities of a human coach. Contrary to expectations from previous literature, participants did not rate professional human coaches higher than simulated AI coaches in terms of working alliance, session value, or outcomes, which included self-rated competence and goal achievement. In fact, both coached groups made significant progress compared to the control group, with participants convincingly engaging with their respective coaches, as confirmed by a novel believability index. The findings challenge prevailing assumptions about human uniqueness in relation to technology. The rapid advancement of AI suggests a revolutionary shift in coaching, where AI could take on a central and surprisingly effective role, redefining what we thought only human coaches could do and reshaping their role in the age of AI.
- Adult education
- Artificial intelligence--Educational applications
- Graduate students
- Educational technology--Evaluation
- Education, Higher--Technological innovations
- Education, Higher--Effect of technological innovations on
This item is currently under embargo. It will be available starting 2029-05-14.
More About This Work
- DOI Copy DOI to clipboard
Can service scholarships boost academic performance? Causal evidence from China’s Free Teacher Education scholarship
- Published: 24 May 2024
Cite this article
- Qi Zheng ORCID: orcid.org/0000-0002-5290-5720 1 &
- Yan Shi 2
40 Accesses
2 Altmetric
Explore all metrics
This study provides causal evidence on the short-term impacts of the Free Teacher Education scholarship (FTE), a service scholarship for teacher candidates in China, on college academic achievement, employing a regression discontinuity design. Distinguishing itself from existing literature, the study compares academic performance within the same classrooms, drawing from a transcript dataset of around 200,000 course-level observations from a top-ranked normal university. The findings indicate that, overall, the scholarship has no significant impact on course grades or college GPA, except in specific scenarios. Heterogeneity analyses reveal that scholarship recipients from wealthier families perform slightly worse than their peers with similar family backgrounds. Additionally, a negative trend is observed in the scholarship’s impacts on course scores over time: initial performance improvement followed by a subsequent decline. These insights imply that while the FTE scholarship attracts more academically competitive students, it may simultaneously lead to unintended trade-offs in performance.
This is a preview of subscription content, log in via an institution to check access.
Access this article
Price includes VAT (Russian Federation)
Instant access to the full article PDF.
Rent this article via DeepDyve
Institutional subscriptions
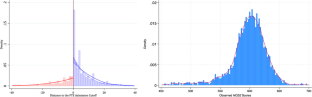
Similar content being viewed by others
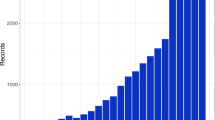
The Impact of Peer Assessment on Academic Performance: A Meta-analysis of Control Group Studies
First-year university students’ academic success: the importance of academic adjustment.
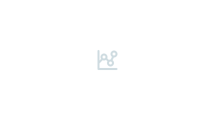
Do High School Gifted Programs Lead to Later-in-Life Success?
Data availability.
The authors accessed a restricted administrative database containing sensitive information and do not have the right to share the dataset with the public.
“Grants” and “scholarships” are often used interchangeably in this context, for consistency, this paper adopts the term “scholarships”.
Given the challenges in tracking scholarship recipients post-graduation, especially those from nationwide programs, current knowledge about the improvement in teaching effectiveness due to service scholarships is rare.
According to a nationwide survey, over one-third of Chinese college students have loans, and more than 20% struggle to cover basic college-related expenses (Li et al., 2013 ).
The NCEE scores hold validity only for the corresponding year, compelling students to weigh the risks of losing their current admission offers if they choose to retake the exam. Consequently, as Kang et al. ( 2024 ) note, the majority of students admitted to Tier 1 universities typically do not retake the test. For further comprehensive analyses and descriptions of China’s college application and admission system, interested readers can refer to works such as Chen and Kesten ( 2016 ) and Ye ( 2023 ).
In China, high school students enroll in either the art or science stream. Most departments at the sample university admit students from a single stream, except for English literacy and elementary education, which accept students from both streams. Admissions are based on the selection pool of students from the same province-major(-stream) clusters.
Applicants could still be admitted if they chose to comply with the major assignment and their scores rank above the quota limits of the university in their province. Otherwise, applicants will not be admitted and may either be matched with other universities or not receive admission at all.
In our sample university, there is no scholarship other than those provided by the government.
Given that 98.9% of students in the 2018 cohort completed the program in 4 years, the average number of courses (73.6) for this cohort should be very close to the total number of courses to obtain a bachelor’s degree in the sample university.
Student survey responses show no correlation with their FTE status, GPA, or completed credits. See Table A1 in the appendix for details.
See Table A2 in the appendix for full results.
The optimal bandwidth using MSE-optimal selector (Calonico et al., 2014 ) is 21 points.
In addition, we conducted a supplementary analysis exploring students’ underlying motivations for choosing teaching majors using additional data from the survey. This investigation covered factors such as passion for teaching, job security, flexibility, influence from close associates or previous teachers, and attraction to the FTE policy, examining their consistency around the cutoff thresholds. The analysis shows that these motivational factors account for minimal variances for scoring above the thresholds, with adjusted R-squared values ranging from 0.001 to 0.003 across various bandwidths. Notably, none of the factors reach statistical significance given other covariates (see Tables A4 and A5 in the appendix for detailed results).
Of the 1.1% of admitted teaching major students who did not enroll in the sample university, 46% had been accepted into FTE majors, indicating no significant preference for FTE scholarship among unenrolled students and implying minimal impact on the estimation.
Stepwise RD analyses, along with detailed discussions, are presented in the appendix. Figures A1 to A3 display the results of all potential combinations of covariates, while Table A7 outlines key models, underscoring the results are not sensitive to model specifications.
To ensure the power of the heterogeneity analysis, students are grouped into two income categories instead of the original five. Employing the five-category variable, despite the abundance of course-level observations, results in relatively few unique students per subgroup during local analysis.
Balance checks for subsample analysis, justifying assumption 2 should be held. Comprehensive results are presented in Tables A8 to A15 .
We also observe no significant unequal distribution among unenrolled admitted students for the by-grade analyses. For the 1st and 2nd year analyses, the sample includes all students. In the 3rd year analysis, which encompasses the 2018, 2019, and 2020 cohorts, 44% of unenrolled students were admitted to FTE majors. For the 4th year, involving the 2018 and 2019 cohorts, this figure is 45%. Due to the absence of detailed individual characteristics of unenrolled students, we lack specific insights into distribution by family income.
Full results can be found in Tables A16 and A17 in the appendix.
Concerns that the scholarships might benefit low-income students more and that unenrolled admitted students may mostly be from this group, potentially skewing estimates, are noted. However, even if all 38 unenrolled FTE students were low-income and near the cutoff points, their absence would lead to just a 2.8% sample loss within the optimal bandwidths, and 4.9% in the ± 5 bandwidths, both under the 5% threshold, suggesting minimal estimation impact. If this phenomenon is present, we would anticipate more stable and positive estimates in the low-income student analysis.
While the estimate for the polynomial specification with quadratic terms is not statistically significant, this model specification may be flawed because the polynomial terms are not statistically significant. Therefore, it may be appropriate to not refer to this model.
Note that the 4th year sample includes a limited number of students of cohort 2018 and one semester of course observations for cohort 2019 in their final year. Also, students complete most of their coursework before the last academic year. These factors may result in larger standard errors and insignificant estimates for the 4th year. The precision of estimates should improve with a larger sample size. Hence, it should be safe to infer the negative longer-term impact of the FTE scholarship on academic performance.
Bardach, L., & Klassen, R. M. (2020). Smart teachers, successful students? A systematic review of the literature on teachers’ cognitive abilities and teacher effectiveness. Educational Research Review, 30 , 100312. https://doi.org/10.1016/j.edurev.2020.100312
Article Google Scholar
Barkowski, E., Nielsen, E., Noel, H., Dodson, M., Sonnenfeld, K., Ye, C., DeMonte, E., Monahan, B., & Eccleston, M. (2018). Study of the teacher education assistance for college and higher education (TEACH) grant program. Office of Planning, Evaluation and Policy Development, US Department of Education . Retrieved from https://files.eric.ed.gov/fulltext/ED613443.pdf
Bärnighausen, T., & Bloom, D. E. (2009). “Conditional scholarships” for HIV/AIDS health workers: Educating and retaining the workforce to provide antiretroviral treatment in sub-Saharan Africa. Social Science & Medicine, 68 (3), 544–551. https://doi.org/10.1016/j.socscimed.2008.11.009
Barrow, L., Richburg-Hayes, L., Rouse, C. E., & Brock, T. (2014). Paying for performance: The education impacts of a community college scholarship program for low-income adults. Journal of Labor Economics, 32 (3), 563–599. https://doi.org/10.1086/675229
Bénabou, R., & Tirole, J. (2006). Incentives and prosocial behavior. American Economic Review, 96 (5), 1652–1678. https://doi.org/10.1257/aer.96.5.1652
Bettinger, E., Gurantz, O., Kawano, L., Sacerdote, B., & Stevens, M. (2019). The long-run impacts of financial aid: Evidence from California’s cal grant. American Economic Journal: Economic Policy, 11 (1), 64–94. https://doi.org/10.1257/pol.20170466
Blundell, R., Dearden, L., Meghir, C., & Sianesi, B. (1999). Human capital investment: The returns from education and training to the individual, the firm and the economy. Fiscal Studies, 20 (1), 1–23. https://doi.org/10.1111/j.1475-5890.1999.tb00001.x
Boatman, A., Evans, B. J., & Soliz, A. (2017). Understanding loan aversion in education: Evidence from high school seniors, community college students, and adults. AERA Open, 3 (1), 2332858416683649. https://doi.org/10.1177/2332858416683649
Brand, J. E. (2010). Civic returns to higher education: A note on heterogeneous effects. Social Forces, 89 (2), 417–433. https://doi.org/10.1353/sof.2010.0095
Calonico, S., Cattaneo, M. D., & Titiunik, R. (2014). Robust nonparametric confidence intervals for regression-discontinuity designs. Econometrica, 82 (6), 2295–2326. https://doi.org/10.3982/ECTA11757
Carruthers, C. K., & Özek, U. (2016). Losing HOPE: Financial aid and the line between college and work. Economics of Education Review, 53 , 1–15. https://doi.org/10.1016/j.econedurev.2016.03.014
Chen, Y., & Kesten, O. (2016). Chinese college admissions and school choice reforms: A theoretical analysis. Journal of Political Economy, 125 (1), 99–139. https://doi.org/10.1086/689773
Chinese Ministry of Education. (2007). The guidelines for implementing free teacher education programs at the ministry of education affiliated normal universities (Trial). Retrieved from https://www.gov.cn/zwgk/2007-05/14/content_614039.htm . ( in Chinese ).
Chinese Ministry of Education (2010). National plan for medium and long-term education reform and development (2010–2020). Retrieved from https://www.gov.cn/jrzg/2010-07/29/content_1667143.htm . (in Chinese).
Cornwell, C., Mustard, D. B., & Sridhar, D. J. (2006a). The enrollment effects of merit-based financial aid: Evidence from Georgia’s HOPE program. Journal of Labor Economics, 24 (4), 761–786. https://doi.org/10.1086/506485
Cornwell, C., Lee, K. H., & Mustard, D. B. (2006b). The effects of state-sponsored merit scholarships on course selection and major choice in college. IZA Discussion Paper No. 1953, Available at https://doi.org/10.2139/ssrn.880430
Curs, B. R., & Harper, C. E. (2012). Financial aid and first-year collegiate GPA: A regression discontinuity approach. The Review of Higher Education, 35 (4), 627–649. https://doi.org/10.1353/rhe.2012.0040
Deci, E. L., Koestner, R., & Ryan, R. M. (1999). A meta-analytic review of experiments examining the effects of extrinsic rewards on intrinsic motivation. Psychological Bulletin, 125 (6), 627.
Dee, T. S. (2004). Are there civic returns to education? Journal of Public Economics, 88 (9), 1697–1720. https://doi.org/10.1016/j.jpubeco.2003.11.002
Deming, D., & Dynarski, S. (2009). Into college, out of poverty? Policies to increase the postsecondary attainment of the poor [presented at “targeting investments in children,” September 26, 2008]. National Bureau of Economic Research Working Paper Series , No. 15387 . https://doi.org/10.3386/w15387
Dynarski, S., & Scott-Clayton, J. (2013). Financial aid policy: Lessons from research. National Bureau of Economic Research Working Paper Series , No. 18710 . https://doi.org/10.3386/w18710
Eng, A., & Matsudaira, J. (2021). Pell grants and student success: Evidence from the universe of federal aid recipients. Journal of Labor Economics, 39 (S2), S413–S454. https://doi.org/10.1086/712556
Fan, E., Meng, X., Wei, Z., & Zhao, G. (2010). Rates of return to university education: The regression discontinuity design . IZA Discussion Paper No. 4749, Available at https://doi.org/10.2139/ssrn.1552683
Feng, L., & Sass, T. R. (2018). The impact of incentives to recruit and retain teachers in “hard-to-staff” subjects. Journal of Policy Analysis and Management, 37 (1), 112–135. https://doi.org/10.1002/pam.22037
Field, E. (2009). Educational debt burden and career choice: Evidence from a financial aid experiment at NYU Law School. American Economic Journal: Applied Economics, 1 (1), 1–21. https://doi.org/10.1257/app.1.1.1
Freeman, R. B. (1997). Working for nothing: The supply of volunteer labor. Journal of Labor Economics, 15 (1, Part 2), S140–S166.
Frey, B. S., & Oberholzer-Gee, F. (1997). The cost of price incentives: An empirical analysis of motivation crowding- out. The American economic review , 87 (4), 746–755. http://www.jstor.org/stable/2951373
Frey, B. S., & Jegen, R. (2001). Motivation crowding theory. Journal of Economic Surveys, 15 (5), 589–611. https://doi.org/10.1111/1467-6419.00150
Gerber, A. S., & Green, D. P. (2012). Field experiments: Design, analysis, and interpretation . Norto: W. W.
Google Scholar
Gist, C. D., Bianco, M., & Lynn, M. (2018). Examining grow your own programs across the teacher development continuum: Mining research on teachers of color and nontraditional educator pipelines. Journal of Teacher Education, 70 (1), 13–25. https://doi.org/10.1177/0022487118787504
Han, L., & Xie, J. (2020). Can conditional grants attract better students? Evidence from Chinese teachers’ colleges. Economics of Education Review, 78 , 102034. https://doi.org/10.1016/j.econedurev.2020.102034
Hanushek, E. A., Piopiunik, M., & Wiederhold, S. (2019). The value of smarter teachers international evidence on teacher cognitive skills and student performance. Journal of Human Resources, 54 (4), 857–899.
Henry, G. T., Bastian, K. C., & Smith, A. A. (2012). Scholarships to recruit the “best and brightest” into teaching: Who is recruited, where do they teach, how effective are they, and how long do they stay? Educational Researcher, 41 (3), 83–92.
Herbaut, E., & Geven, K. (2020). What works to reduce inequalities in higher education? A systematic review of the (quasi-)experimental literature on outreach and financial aid. Research in Social Stratification and Mobility, 65 , 100442. https://doi.org/10.1016/j.rssm.2019.100442
Hillman, N. W., Tandberg, D. A., & Gross, J. P. K. (2014). Performance funding in higher education: Do financial incentives impact college completions? The Journal of Higher Education, 85 (6), 826–857. https://doi.org/10.1080/00221546.2014.11777349
Hout, M. (2012). Social and economic returns to college education in the United States. Annual Review of Sociology, 38 (1), 379–400. https://doi.org/10.1146/annurev.soc.012809.102503
Jackson, C. K. (2012). School competition and teacher labor markets: Evidence from charter school entry in North Carolina. Journal of Public Economics, 96 (5), 431–448. https://doi.org/10.1016/j.jpubeco.2011.12.006
Jacobsen, C. B., Hvitved, J., & Andersen, L. B. (2014). Command and motivation: How the perception of external internventions relates to intrinsic motivation and public service motivation. Public Administration, 92 (4), 790–806. https://doi.org/10.1111/padm.12024
Johnson, I. (2022). Merit aid and retention: Mediation and moderation. Research in Higher Education, 63 (4), 713–739. https://doi.org/10.1007/s11162-021-09661-9
Kang, C. H., & Park, Y. (2021). Private tutoring and distribution of student academic outcomes: An implication of the presence of private tutoring for educational inequality. Korean Economic Review, 37 (2), 287–326. https://doi.org/10.22841/kerdoi.2021.37.2.004
Kang, L., Lei, Z., Song, Y., & Zhang, P. (2024). Gender differences in reactions to failure in high-stakes competition: Evidence from the national college entrance exam retakes. Journal of Political Economy Microeconomics (available online). https://doi.org/10.1086/729535
Kingston, P. W., Hubbard, R., Lapp, B., Schroeder, P., & Wilson, J. (2003). Why education matters. Sociology of Education, 76 (1), 53–70. https://doi.org/10.2307/3090261
Krueger, A. B., & Lindahl, M. (2001). Education for growth: Why and for whom? Journal of Economic Literature, 39 (4), 1101–1136. https://doi.org/10.1257/jel.39.4.1101
Kunreuther, H., & Easterling, D. (1990). Are risk-benefit tradeoffs possible in siting hazardous facilities? The American Economic Review, 80 (2), 252–256.
Lepper, M. R., & Greene, D. (Eds.). (2015). The hidden costs of reward: New perspectives on the psychology of human motivation . Psychology Press.
Li, W. (2007). Family background, financial constraints and higher education attendance in China. Economics of Education Review, 26 (6), 724–734. https://doi.org/10.1016/j.econedurev.2007.09.001
Li, T., & Zhang, J. (2010). What determines employment opportunity for college graduates in China after higher education reform? China Economic Review, 21 (1), 38–50. https://doi.org/10.1016/j.chieco.2009.10.001
Li, H., Meng, L., Shi, X., & Wu, B. (2013). Poverty in China’s colleges and the targeting of financial aid. The China Quarterly, 216 , 970–992. https://doi.org/10.1017/S0305741013001082
Li, J., Shi, Z., & Xue, E. (2020). The problems, needs and strategies of rural teacher development at deep poverty areas in China: Rural schooling stakeholder perspectives. International Journal of Educational Research, 99 , 101496. https://doi.org/10.1016/j.ijer.2019.101496
Liao, W., & Yuan, R. (2017). Understand an emerging “failure” of an equality-oriented teacher policy in China: A job search perspective. International Journal of Educational Research, 81 , 71–82. https://doi.org/10.1016/j.ijer.2016.11.002
Liou, P.-Y., & Lawrenz, F. (2011). Optimizing teacher preparation loan forgiveness programs: Variables related to perceived influence. Science Education, 95 (1), 121–144. https://doi.org/10.1002/sce.20409
Long, B. T., & Riley, E. (2008). Financial aid: A broken bridge to college access? Harvard Educational Review, 77 (1), 39–63. https://doi.org/10.17763/haer.77.1.765h8777686r7357
McCrary, J. (2008). Manipulation of the running variable in the regression discontinuity design: A density test. Journal of Econometrics, 142 (2), 698–714. https://doi.org/10.1016/j.jeconom.2007.05.005
McEwan, P. J. (1999). Recruitment of rural teachers in developing countries: An economic analysis. Teaching and Teacher Education, 15 (8), 849–859. https://doi.org/10.1016/S0742-051X(99)00025-6
Mirowsky, J., & Ross, C. (2003). Education, social status, and health . Aldine de Gruyter.
Morgan, S. L., & Winship, C. (2015). Counterfactuals and causal inference . Cambridge University Press.
Nguyen, T. D., Kramer, J. W., & Evans, B. J. (2019). The effects of grant aid on student persistence and degree attainment: A systematic review and meta-analysis of the causal evidence. Review of Educational Research, 89 (6), 831–874. https://doi.org/10.3102/0034654319877156
OECD. (2005). Teachers matter: Attracting, developing and retaining effective teachers. OECD Publishing . https://doi.org/10.1787/9789264018044-en
Park, A., Shi, X., Hsieh, C.-T., & An, X. (2015). Magnet high schools and academic performance in China: A regression discontinuity design. Journal of Comparative Economics, 43 (4), 825–843. https://doi.org/10.1016/j.jce.2015.10.013
Peng, W. J., McNess, E., Thomas, S., Wu, X. R., Zhang, C., Li, J. Z., & Tian, H. S. (2014). Emerging perceptions of teacher quality and teacher development in China. International Journal of Educational Development, 34 , 77–89. https://doi.org/10.1016/j.ijedudev.2013.04.005
Peyton, D. J., van Dijk, W., & Mason-Williams, L. (2022). Meeting the moment: Impact of TEACH grant on US undergraduate education degree completion in high-need content areas. Higher Education Policy . https://doi.org/10.1057/s41307-022-00263-3
Pinto, L. H., & He, K. (2019). ‘In the eyes of the beholder’: The influence of academic performance and extracurricular activities on the perceived employability of Chinese business graduates. Asia Pacific Journal of Human Resources, 57 (4), 503–527. https://doi.org/10.1111/1744-7941.12200
Psacharopoulos, G., & Patrinos, H. A. (2018). Returns to investment in education: A decennial review of the global literature. Education Economics, 26 (5), 445–458. https://doi.org/10.1080/09645292.2018.1484426
Rockoff, J. E., Jacob, B. A., Kane, T. J., & Staiger, D. O. (2011). Can you recognize an effective teacher when you recruit one? Education Finance and Policy, 6 (1), 43–74. https://doi.org/10.1162/EDFP_a_00022
Schleicher, A. (2012). Preparing teachers and developing school leaders for the 21st century: Lessons from around the world . OECD Publishing.
Scott-Clayton, J. (2011). On money and motivation a quasi-experimental analysis of financial incentives for college achievement. Journal of Human Resources, 46 (3), 614–646. https://doi.org/10.3368/jhr.46.3.614
Shen, H., & Ziderman, A. (2009). Student loans repayment and recovery: International comparisons. Higher Education, 57 (3), 315–333. https://doi.org/10.1007/s10734-008-9146-0
Sjoquist, D. L., & Winters, J. V. (2015). State merit-based financial aid programs and college attainment. Journal of Regional Science, 55 (3), 364–390. https://doi.org/10.1111/jors.12161
Stater, M. (2009). The impact of financial aid on college GPA at three flagship public institutions. American Educational Research Journal, 46 (3), 782–815. https://doi.org/10.3102/0002831208329903
Steele, J. L., Murnane, R. J., & Willett, J. B. (2010). Do financial incentives help low-performing schools attract and keep academically talented teachers? Evidence from California. Journal of Policy Analysis and Management, 29 (3), 451–478.
Titmuss, R. (2018). The gift relationship: From human blood to social policy . Policy Press.
Wang, D., & Gao, M. (2013). Educational equality or social mobility: The value conflict between preservice teachers and the Free Teacher Education Program in China. Teaching and Teacher Education, 32 , 66–74. https://doi.org/10.1016/j.tate.2013.01.008
Wei, Y., & Zhou, S. (2019). Are better teachers more likely to move? Examining teacher mobility in rural China. The Asia-Pacific Education Researcher, 28 (2), 171–179. https://doi.org/10.1007/s40299-018-0423-0
Whitfield, J. G., Waxman, H., & Scott, T. (2016). Comparing Robert Noyce Scholars and Non- Robert Noyce Scholars Perceptions of Teaching. Journal of Research in STEM Education , 2 (2), 90–105.
Yang, P. (2010). Who gets more financial aid in China? A multilevel analysis. International Journal of Educational Development, 30 (6), 560–569. https://doi.org/10.1016/j.ijedudev.2009.12.006
Yang, P. (2011). The impact of financial aid on learning, career decisions, and employment. Chinese Education & Society, 44 (1), 27–57. https://doi.org/10.2753/CED1061-1932440102
Ye, X. (2023). Improving college choice in centralized admissions: Experimental evidence on the importance of precise predictions. Education Finance and Policy , 1–75. https://doi.org/10.1162/edfp_a_00397
Download references
Acknowledgements
We extend our heartfelt appreciation to Dr. Nicholas Hillman for his invaluable expertise and encouragement, which were instrumental in shaping this work. Our gratitude also goes to the two anonymous reviewers whose constructive and insightful comments significantly enhanced the quality of this study. We are also thankful to Dr. Daniel Collier for his generous allocation of time and valuable advice. Further, we express our thanks to our colleagues and participants at conferences and department reviews, including but not limited to Drs. Xiaoyang Ye, Ang Yu, Rian Djita, Sam Glick, Xinliang Zhang, and others, for their constructive feedback and support that have notably enriched the quality of our research.
Author information
Authors and affiliations.
Department of Educational Leadership and Policy Analysis, University of Wisconsin-Madison, 1000 Bascom Mall, Madison, WI, 53706, USA
Faculty of Education, Northeast Normal University, Changchun, Jilin Province, China
You can also search for this author in PubMed Google Scholar
Corresponding author
Correspondence to Qi Zheng .
Ethics declarations
Conflict of interest.
This study received sponsorship from the 2022 Chinese Ministry of Education (MOE) Project of Humanities and Social Sciences, titled “The Generation Mechanism and Prevention Mechanism of Teachers’ Burden Risk under the Background of Double Reduction Policy: based on the Perspective of Teachers’ Agency” (Project ID: 22YJA880044), which was granted to Professor Yan Shi. Apart from this sponsorship, we have no other conflicts of interest to declare.
Additional information
Publisher's note.
Springer Nature remains neutral with regard to jurisdictional claims in published maps and institutional affiliations.
Supplementary Information
Below is the link to the electronic supplementary material.
Supplementary file1 (DOCX 14.6 MB)
Rights and permissions.
Springer Nature or its licensor (e.g. a society or other partner) holds exclusive rights to this article under a publishing agreement with the author(s) or other rightsholder(s); author self-archiving of the accepted manuscript version of this article is solely governed by the terms of such publishing agreement and applicable law.
Reprints and permissions
About this article
Zheng, Q., Shi, Y. Can service scholarships boost academic performance? Causal evidence from China’s Free Teacher Education scholarship. High Educ (2024). https://doi.org/10.1007/s10734-024-01242-w
Download citation
Accepted : 13 May 2024
Published : 24 May 2024
DOI : https://doi.org/10.1007/s10734-024-01242-w
Share this article
Anyone you share the following link with will be able to read this content:
Sorry, a shareable link is not currently available for this article.
Provided by the Springer Nature SharedIt content-sharing initiative
- Service scholarships
- Academic achievement
- Regression discontinuity
- Transcript data
- Pre-service teachers
- Find a journal
- Publish with us
- Track your research

IMAGES
VIDEO
COMMENTS
The study of academic achievement of college students is an effective way to promote the quality of higher education process. Through a literature review related to academic achievement of college students, it is revealed that the measurement indexes of academic achievement tend to be diversified and the measurement tools are more perfect.
The literature review is of paramount im portance to upd ate the current info r- mation and trend of re search on factors that influence the acade mic perform- ance of students at the senior high ...
effects on or correlated with students' academic achievement such as learning-teaching methods (Donker, de Boer, Kontos, Dinath van Ewjk, & van der Werf, 2014), the ... In the study, a comprehensive review of related literature was conducted on the "Web of Science-All Databases" by using the phrases ("academic achievement" or "academic ...
Student success plays a vital role in educational institutions, as it is often used as a metric for the institution's performance. Early detection of students at risk, along with preventive measures, can drastically improve their success. Lately, machine learning techniques have been extensively used for prediction purpose. While there is a plethora of success stories in the literature ...
Literature Review and Hypotheses Development ... personal and intellectual ability in terms of self-management and self-efficacy to better represent the factors facing college students (Figure 1). This adjustment is justified, since the involvement of student qualities (IQ, consciousness, knowledge) is expected to be equal at the same stage of ...
Academic achievement. Academic achievement was once thought to be the most important outcome of formal educational experiences and while there is little doubt as to the vital role such achievements play in student life and later (Kell, Lubinski, & Benbow, Citation 2013 ), researchers and policy makers are ever increasingly turning to social and ...
The meta-analysis and the literature review suggest that the effect size of EI on academic performance is moderate-high (0.390). Some differences by region were attested. ... (2010). The role of emotional intelligence in predicting students' academic achievement in distance education system. Procedia - Social and Behavioral Sciences, 2(2 ...
While this is a multi-dimensional definition, authors in (York, Gibson, & Rankin, 2015) gave an amended definition concentrating on the most important six components, that is to say "Academic achievement, satisfaction, acquisition of skills and competencies, persistence, attainment of learning objectives, and career success (Fig. 3).
Sleep quality and quantity are strongly associated with academic achievement in college students (Curcio et al ... of psychosocial problems in individuals with stoma: a review of literature. Int ...
Representation of three types of study designs that can investigate the relationships between motivation and academic achievement. (1) The gray box shows that to establish that motivation causes academic achievement (top) or vice versa (bottom), experimental manipulation is needed, intervening on the predictor at time point 1, which influences the outcome at time point 2 and so on.
This paper provides a systematic literature review (SLR) of 347 papers, focusing on the: indicators of academic performance, relation between student part-time work and academic performance, and drivers of students' decision to work. ... This paper's contributions lie in systematising positive and negative impacts of student work on ...
This systematic literature review explores the impact of innovative teaching approaches on student motivation and academic achievement in online blended learning. A thorough search of five electronic databases for studies published between January 2009 and May 2023 yielded 1468 records.
The findings of this scoping review confirm Tinto's model of academic persistence by showing that a student's pre-entry attributes (e.g., prior academic achievement family), goals and commitments (e.g., motivation), social and academic experiences (social support, social and academic adjustment), integration (satisfaction with the institution ...
Scholars agree that students' academic achievement is a 'net result' of their cognitive and non-cognitive attributes (Lee & Shute, Citation 2010; Lee & Stankov, Citation 2016) as well as the sociocultural context in which the learning process takes place (Liem & McInerney, Citation 2018; Liem & Tan, Citation 2019).The present issue comprises eight papers that look into the extent to ...
The question of how learners' motivation influences their academic achievement and vice versa has been the subject of intensive research due to its theoretical relevance and important implications for the field of education. Here, we present our understanding of how influential theories of academic motivation have conceptualized reciprocal interactions between motivation and achievement and ...
A considerable amount of literature has been published on students' well-being, but empirical research has yielded ambiguous results regarding the relationship between well-being and academic achievement. Meta-analysis studies on this issue are scarce, and the available studies focus on a single domain of well-being. Meta-analyses investigating students' general well-being and academic ...
PurposeCollege students' academic achievement has crucial implications for their future success. ... College students' academic achievement has crucial implications for their future success. ... Low BJ, Hollis C, et al. Coordinated school health programs and academic achievement: a systematic review of the literature. J Sch Health. 2007;77: ...
Abstract. This paper explores the literature on the relationship between extracurricular activities and academic achievement of college students. Some studies support that extracurricular ...
This review sets the stage for further discussion of the research findings on identification practices including the efficacy of various approaches to increase the identification of gifted students in poverty. We then review research on the effectiveness of programs designed specifically to identify students with potential and support them ...
We present the first systematic review of the scientific literature on smartphone use and academic success. We synthesise the theoretical mechanisms, empirical approaches, and empirical findings described in the multidisciplinary literature to date. Our analysis of the literature reveals a predominance of empirical results supporting a negative ...
The effect of college students' reading engagement on standardized academic achievement reached 0.57, and the probability of significance was P<. 0.001, which indicates that college students' reading engagement can indeed have a positive role in promoting academic achievement.
A systematic literature review on 27 articles revealed that self-efficacy and academic performance had significant and positive relationship among Latino students in America across all levels of education and with different measuring instruments (Manzano-Sanchez et al., 2018). Thus, the following hypothesis was proposed:
Seeking academic help has a positive impact on students' ability to handle challenges, leading to improved academic success. As the academic landscape becomes more competitive, the importance of students seeking and using academic support is widely recognized for enhancing their learning experience and achievements. The main objective of this study is to review the prior literature that has ...
The intent of this systematically reviewed literature was to assess the connection between students' listening skills and their academic achievements. The researcher gathered previously ...
This study aims to explore the interrelationships between teacher competence, college students' mental health, and academic achievement, and to analyze the mediating role of mental health as well as the differences among various student groups. Through a survey of 1236 students from 45 higher education institutions in China and the application of structural equation modeling for data ...
Introduction. A lack of academic persistence among undergraduate students poses a challenge for higher education and raises concerns about dropout rates (Xavier & Meneses, Citation 2022).Those with a lower sense of belonging would be more vulnerable (Strayhorn, Citation 2012, Citation 2019) and prone to emotional and academic maladjustment (Jain & Sharma, Citation 2022; Ostrove & Long ...
The rise of artificial intelligence (AI) challenges us to explore whether human-to-human relationships can extend to AI, potentially reshaping the future of coaching. The purpose of this study was to examine client perceptions of being coached by a simulated AI coach, who was embodied as a vocally conversational live-motion avatar, compared to client perceptions of a human coach. It explored ...
This study provides causal evidence on the short-term impacts of the Free Teacher Education scholarship (FTE), a service scholarship for teacher candidates in China, on college academic achievement, employing a regression discontinuity design. Distinguishing itself from existing literature, the study compares academic performance within the same classrooms, drawing from a transcript dataset of ...