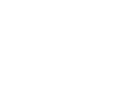

Audit Sampling Methods and Their Impact on Audit Quality
Explore how different audit sampling methods influence the overall quality and reliability of audit results.

Audit sampling is a critical component of the auditing process, enabling auditors to draw conclusions about an entire population based on a subset of data. This practice is essential for ensuring that audits are both efficient and effective, given the impracticality of examining every transaction or account.
The importance of audit sampling lies in its ability to provide reasonable assurance while managing time and resource constraints. Properly executed sampling methods can significantly enhance the reliability of audit findings, thereby bolstering overall audit quality.
Key Concepts in Audit Sampling
Audit sampling revolves around the principle of selecting and evaluating a representative subset of data to infer conclusions about the entire dataset. This approach hinges on the concept of representativeness, which ensures that the sample accurately reflects the characteristics of the population. A well-chosen sample can provide insights that are nearly as reliable as examining the entire population, making it a powerful tool in the auditor’s arsenal.
One fundamental concept in audit sampling is the notion of sampling risk. This is the risk that the sample chosen does not accurately represent the population, leading to incorrect conclusions. Sampling risk can be managed through careful planning and execution of the sampling process. Auditors must balance the need to minimize sampling risk with the practical constraints of time and resources.
Another important aspect is the distinction between sampling and non-sampling risk. While sampling risk pertains to the inherent uncertainty in using a sample, non-sampling risk arises from factors such as human error, inappropriate audit procedures, or misinterpretation of results. Effective audit sampling requires auditors to be vigilant about both types of risk to ensure the integrity of their conclusions.
Statistical vs. Non-Statistical Sampling
When auditors embark on the journey of selecting samples, they often face the choice between statistical and non-statistical sampling methods. Each approach has its own set of advantages and limitations, and the decision on which to use can significantly influence the audit’s outcome.
Statistical sampling relies on the principles of probability theory to select and evaluate samples. This method allows auditors to quantify sampling risk and make inferences about the population with a known level of confidence. Techniques such as random sampling, systematic sampling, and stratified sampling fall under this category. For instance, random sampling ensures that every item in the population has an equal chance of being selected, thereby enhancing the representativeness of the sample. Systematic sampling, on the other hand, involves selecting items at regular intervals, which can be particularly useful when dealing with large datasets.
Non-statistical sampling, in contrast, is based on the auditor’s judgment rather than mathematical probabilities. This approach can be more flexible and quicker to implement, as it allows auditors to use their professional experience to select samples that they believe are most representative of the population. Methods such as haphazard sampling and block sampling are common in this category. Haphazard sampling involves selecting items without any structured technique, relying on the auditor’s intuition. Block sampling, meanwhile, involves selecting a contiguous block of items, which can be useful in certain scenarios but may introduce bias if not carefully managed.
The choice between statistical and non-statistical sampling often hinges on the specific circumstances of the audit. Statistical sampling provides a more rigorous framework and can be particularly valuable in high-stakes audits where precision is paramount. Non-statistical sampling, while less formal, offers the advantage of speed and flexibility, making it suitable for audits with tight deadlines or limited resources.
Determining Sample Size
Determining the appropriate sample size is a nuanced process that requires a careful balance of various factors. The goal is to select a sample that is large enough to provide reliable insights while remaining manageable within the constraints of time and resources. One of the primary considerations in this process is the desired level of confidence and precision. Auditors must decide how confident they need to be in their conclusions and how much variability they are willing to accept. Higher confidence levels and lower tolerable error rates typically necessitate larger sample sizes.
Another crucial factor is the population size. While it might seem intuitive that larger populations require larger samples, the relationship is not always linear. In many cases, the sample size needed to achieve a certain level of confidence and precision does not increase proportionally with the population size. This is where statistical formulas and tables come into play, helping auditors determine the optimal sample size based on the specific characteristics of the population and the audit objectives.
The nature of the population also plays a significant role. Homogeneous populations, where items are similar in nature, often require smaller samples compared to heterogeneous populations with a high degree of variability. For example, if an auditor is examining a population of transactions that are all of similar value and type, a smaller sample might suffice. Conversely, if the transactions vary widely in amount and nature, a larger sample would be necessary to capture the diversity and ensure representativeness.
Sample Selection Techniques
Selecting the right sample is an art as much as it is a science, requiring auditors to blend methodological rigor with practical judgment. One widely used technique is random sampling, which ensures that every item in the population has an equal chance of being selected. This method is particularly effective in eliminating selection bias, thereby enhancing the representativeness of the sample. Random sampling can be executed using random number generators or specialized software like IDEA or ACL, which streamline the process and add a layer of precision.
Systematic sampling offers another robust approach, where items are selected at regular intervals from a randomly chosen starting point. This technique is especially useful when dealing with large datasets, as it simplifies the selection process while maintaining a high degree of randomness. For instance, if an auditor is examining a ledger with thousands of entries, they might select every 50th entry after a random start, ensuring a manageable yet representative sample.
Stratified sampling takes the process a step further by dividing the population into distinct subgroups or strata based on specific characteristics, such as transaction type or account size. Samples are then drawn from each stratum, ensuring that all relevant segments of the population are adequately represented. This method is particularly beneficial when the population is heterogeneous, as it allows for more precise inferences about each subgroup.
Evaluating Sample Results
Once the sample has been selected and tested, the next step is to evaluate the results. This involves analyzing the findings to determine whether they can be generalized to the entire population. Auditors must assess the nature and extent of any errors or deviations found within the sample. If the errors are isolated and minor, they may not significantly impact the overall conclusions. However, if the errors are pervasive or indicative of systemic issues, further investigation may be warranted.
The evaluation process often involves calculating error rates and comparing them to predetermined thresholds. For instance, if an auditor finds that 5% of the sampled transactions contain errors, they must determine whether this rate is acceptable based on the audit’s objectives and the materiality thresholds set at the outset. Tools like statistical software can aid in this analysis, providing confidence intervals and other metrics that help quantify the reliability of the sample results.
Impact on Audit Quality
The quality of an audit is heavily influenced by the effectiveness of the sampling methods employed. Well-executed sampling can provide a high level of assurance, enabling auditors to make informed conclusions about the entire population. Conversely, poor sampling techniques can lead to misleading results, undermining the audit’s credibility. Therefore, auditors must be meticulous in their approach, ensuring that their sampling methods are robust and appropriate for the specific audit context.
Moreover, the choice of sampling techniques and the rigor with which they are applied can affect the audit’s efficiency. Effective sampling allows auditors to focus their efforts on the most relevant areas, optimizing the use of time and resources. This not only enhances the quality of the audit but also adds value to the client by providing more accurate and actionable insights.
Crafting Effective Shareholder Reports for Modern Businesses
Modern c-suite leadership: strategy, roles, and decision-making, you may also be interested in..., comprehensive payroll internal controls for modern businesses, choosing and managing trustees: roles, duties, and strategies, creating effective engagement letters for modern practices, leadership's impact on ethical corporate culture.
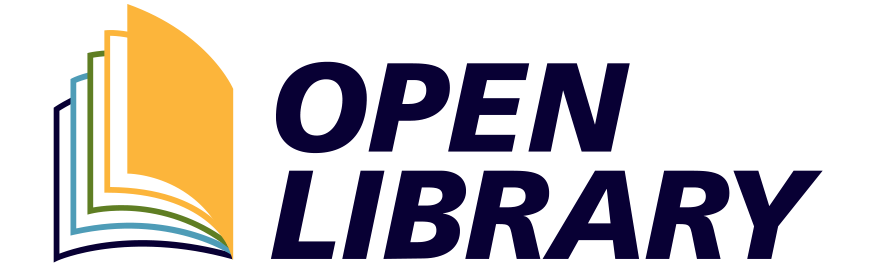
Want to create or adapt books like this? Learn more about how Pressbooks supports open publishing practices.
03. Planning an IS Audit

03.05. Effective Audit Procedures – Sampling
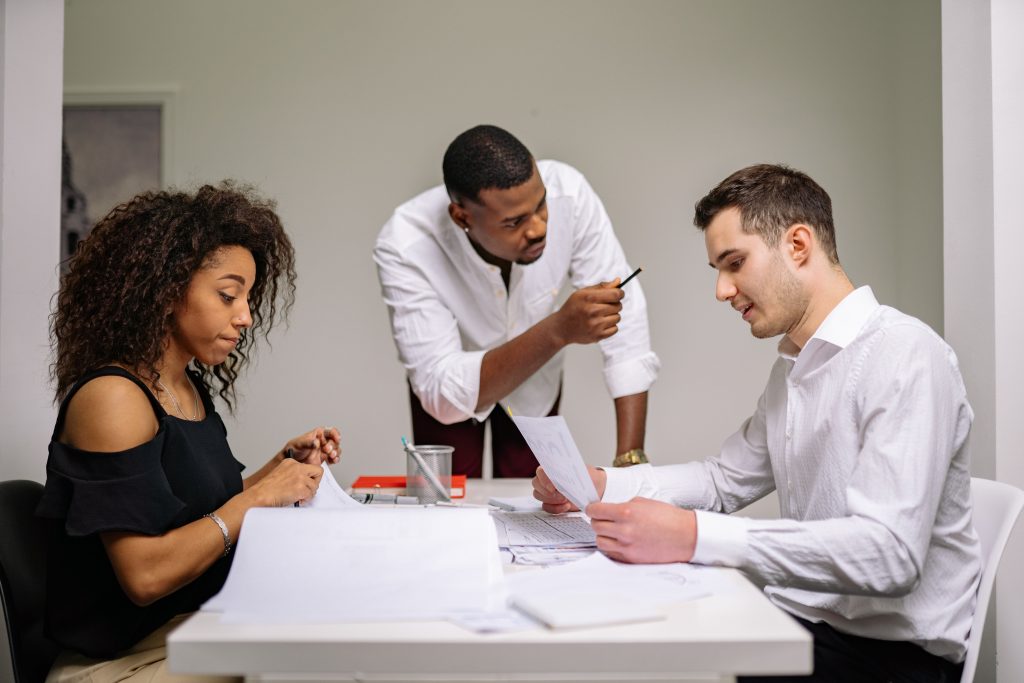
Briefly reflect on the following before we begin:
- Why do auditors often use sampling methods to gather evidence during audits?
- What are some common risks involved in selecting samples during an audit?
- How would an IS Auditor go about selecting samples during an audit?
In this section, we will explore the diverse range of sampling techniques available to IS auditors. We will do so by differentiating between statistical and non-statistical sampling methods, highlighting their respective advantages and appropriate contexts of use. We will explore judgmental sampling, a critical method where the auditor’s professional judgment plays a pivotal role in sample selection; random sampling techniques, which are fundamental to reducing bias and ensuring representativeness in the audit findings; as well as stratified sampling , showcasing how it enhances audit efficiency by categorizing data into relevant strata. Lastly, we will review the importance of software and tools in modern IS auditing, emphasizing how technology aids auditors in executing more precise and effective sampling strategies.
Next, we will discuss the key principles that help determine sufficient sample sizes in IS audits. We will also cover the concept of confidence intervals, an essential statistical tool that helps auditors understand the range within which the true value of the population parameter lies. We will cover the interplay between sample size, risk, and materiality, and how these factors influence the auditor’s decision-making process. Lastly, we will address the challenges and implications of sampling errors in IS audits, such as selection and measurement errors. We will also discuss the strategies for mitigating sampling errors that enable IS Auditors to reduce the likelihood and effect of these errors in their audit work. In doing so, evaluating and reporting sampling errors will be emphasized, highlighting the auditor’s responsibility to communicate these aspects transparently.
Sampling Methods in IS Auditing
Audit sampling emerges as a vital tool in IS auditing, where data volumes can be massive and resources are limited. It is a systematic technique used to examine a subset of data or transactions within a population to conclude the entire dataset. It allows auditors to assess the effectiveness of controls, identify anomalies, and detect errors or irregularities without the need to examine every single transaction or piece of data. This is especially crucial when dealing with extensive datasets that would be impractical to review. By selecting a representative sample, auditors can focus on areas of higher risk or greater significance, optimizing resource allocation. This ensures auditors can conduct thorough audits while efficiently managing time and resources.
Audit sampling is closely linked to risk assessment and materiality considerations as it enables IS auditors to assess the level of risk within a dataset and determine whether errors or irregularities are material enough to impact the overall audit conclusions. High-risk areas may warrant larger sample sizes or more intensive testing, while lower-risk areas may require less extensive sampling. As we know, materiality is a measure of the significance of an error or omission and guides auditors in determining how much evidence is needed. The riskier the audit area, the more evidence we require, leading to larger sample sizes. Conversely, in areas with lower risk, smaller samples may suffice. This relationship is crucial in tailoring the audit to the specific context of the audited entity.
The first method we encounter is statistical sampling . This approach relies on probability theory, ensuring that each element in the population has a known chance of being selected. Its beauty lies in its ability to provide auditors with a quantifiable measure of sampling risk. This risk, the probability that the sample may not represent the population accurately, is a fundamental concept in auditing. The three primarily used statistical sampling approaches include:
Random sampling stands on the principle of equal chance, where every item in the population is equally likely to be selected, ensuring a bias-free approach. Tools and software are often employed to aid this process, bringing in precision and efficiency that manual methods cannot match. Random sampling’s strength lies in its simplicity and fairness, making it a widely accepted method in IS auditing.
Stratified sampling enhances audit efficiency by dividing the population into subgroups or strata. This technique is particularly effective when dealing with heterogeneous populations as it ensures that each stratum is adequately represented in the sample, providing a more accurate view of the entire population.
Systematic sampling , in which an interval (i) is first calculated (population size divided by sample size), and then an item is selected from each interval by randomly selecting one item from the first interval and selecting every ith item until one item is selected from all intervals. Efficiency is a significant advantage, especially when auditing extensive datasets, as it enables auditors to review a sample while maintaining a structured approach. Systematic sampling assumes a uniform data distribution without patterns or anomalies that could skew results and is not an ideal method to use when data exhibits systematic patterns or clustering.
Contrastingly, non-statistical sampling, often used in IS auditing, does not involve this statistical theory. Here, the auditor’s professional judgment is paramount. While this method may not provide a quantifiable measure of risk, it allows for flexibility and adaptability in diverse auditing environments. It’s particularly useful when dealing with complex information systems where specific risks are identified through auditor expertise. The three primarily used non-statistical sampling approaches include the following:
- Judgmental sampling heavily relies on the auditor’s experience and knowledge. In situations where certain aspects of the system are deemed more critical, this method allows auditors to target these areas specifically. It’s an approach where intuition, honed by years of experience, plays a key role. However, auditors must remain vigilant to avoid biases that can skew the audit results.
- Block sampling begins with IS auditors partitioning the dataset into distinct blocks or groups based on specific criteria such as transaction types, periods, or data categories. Rather than randomly selecting individual items or transactions, auditors choose entire blocks for examination. The selection is guided by auditors’ judgment, considering risk, materiality, and audit focus. It allows auditors to concentrate efforts on specific areas of interest, making it suitable for targeted reviews of critical data subsets.
- Haphazard sampling allows auditors to select items without any predetermined pattern or criteria. The selection process relies on auditors’ discretion and can involve simply picking items at random or based on convenience. This approach offers a straightforward way to gather a sample for review, especially when auditors are dealing with a limited dataset or when a formal sampling method may be unnecessary due to the nature of the audit.
It is important to remember that sampling in IS auditing provides a reliable basis for making informed decisions about the information system being audited. Hence, the choice of sampling method should align with the audit’s objectives, the nature of the population, and the specific risks involved. Moreover, as technology advances, the complexity of information systems auditing has escalated, and software tools enable auditors to handle large volumes of data efficiently and accurately. They bring sophistication to sampling methods, allowing auditors to perform more complex analyses and derive more nuanced insights.
Determining the sample size in an IS audit is a critical step that balances thoroughness with efficiency. The process begins with a clear understanding of the audit’s objectives. It’s not about choosing a large sample for comprehensiveness; it’s about choosing the right size to meet our specific IT audit objectives . Understanding confidence intervals is integral to this process. A confidence interval is a range within which we expect the true value of a population parameter to fall. It’s a concept that injects a degree of scientific rigour into our audit conclusions. The width of this interval is influenced by the sample size – larger samples generally result in narrower confidence intervals, offering greater precision. However, larger samples also mean more resources and time. Thus, the auditor must strike a balance, ensuring the sample is sufficient to provide reliable results without being unnecessarily large.
In testing controls (evaluating management’s processes), the IS Auditor applies the following guidance in determining the sample size.
In performing substantive testing (evaluating the underlying activities instead of relying on management’s processes), the IS Auditor will generally test between 1% – 5% of the population with an upper cap of 500 samples. Sampling guidance may vary based on the IS Audit functions’ risk appetite and philosophy.
Sampling Errors and Their Impact on Audit Conclusions
Sampling errors occur when the selected sample does not accurately represent the entire population. This misrepresentation can lead to incorrect conclusions about the system being audited. The primary goal of IS Auditors is to provide accurate and reliable insights into the systems we examine. Sampling errors pose a significant risk to the integrity of the IS auditing work, and it is essential to recognize these errors and understand their potential impact.
There are various types of sampling errors, each with its characteristics and implications. One common type is the selection error, which arises when the method used to select the sample introduces bias. For example, choosing a non-random sample that warrants random selection can lead to skewed results. Another type is the measurement error, which occurs when there is a flaw in how information is collected or recorded. This type of error can significantly distort audit findings. The impact of sampling errors on audit quality and reliability cannot be overstated. When these errors are present, the audit conclusions drawn may be flawed, leading to misguided decisions by stakeholders. This outcome can have far-reaching consequences, especially in high-stakes environments where accurate and dependable audit results are crucial. Therefore, it is imperative for auditors to take steps to minimize the occurrence of these errors.
Mitigating sampling errors involves several strategies. Firstly, careful planning and designing of the sampling process are crucial. This planning includes selecting the appropriate sampling method and ensuring the sample size is adequate for the audit objectives. Secondly, auditors must apply their professional judgment and expertise in executing the sampling plan. This expertise involves being vigilant for signs of potential bias or inaccuracies during the sampling process. Similarly, evaluating and reporting sampling errors is another critical aspect. As auditors, we must identify and mitigate these errors and transparently communicate them in our audit reports. This transparency ensures that stakeholders are aware of the limitations of the audit findings and can interpret the results within the correct context.
In the Spotlight
For additional context on the role and importance of audit sampling, please read the article titled “Audit Sampling” [new tab] .
Henderson, K. (2023). Audit sampling. Wall Street Oasis. https://www.wallstreetoasis.com/resources/skills/accounting/what-is-audit-sampling
Key Takeaways
Let’s recap the key concepts discussed in this section by watching this video.
Source: Mehta, A.M. (2023, December 6). AIS OER ch 03 topic 05 key takeaways [Video]. https://youtu.be/os1wvwFFtqE
Knowledge Check
Review questions.
- Explain the importance of selecting the right sampling method in IS auditing. Provide an example of a situation where you would choose a statistical sampling method over a non-statistical one, and vice versa.
- Explain the concept of sample size determination in IS auditing. How does the level of risk in an audit area influence the choice of sample size?
- What are sampling errors in IS auditing, and how can they impact audit conclusions? Provide an example of a sampling error and its potential consequences in an IS audit.
- What are the key differences between statistical and non-statistical sampling methods in IS auditing, and when would you use each?
- How does an IS auditor determine the appropriate sample size for an audit, and what role do confidence intervals play in this process?
- What is a sampling error in the context of IS auditing, and how can it affect the audit’s conclusions? Give an example.
Essay Question
Sampling methods based on auditors' judgment without the use of statistical theory.
Stratified sampling enhances audit efficiency by dividing the population into subgroups or strata. and is particularly effective when dealing with heterogeneous populations as it ensures that each stratum is adequately represented in the sample, providing a more accurate view of the entire population.
Sampling methods based on probability theory, used to provide a quantifiable measure of sampling risk.
Random sampling stands on the principle of equal chance, where every item in the population is equally likely to be selected, ensuring a bias-free approach.
Systematic sampling, in which an interval (i) is first calculated (population size divided by sample size), and then an item is selected from each interval by randomly selecting one item from the first interval and selecting every ith item until one item is selected from all intervals.
Relies on the auditor's experience and knowledge. In situations where certain aspects of the system are deemed more critical, this method allows auditors to target these areas specifically.
Block sampling begins with IS auditors partitioning the dataset into distinct blocks or groups based on specific criteria such as transaction types, periods, or data categories.
Haphazard sampling allows auditors to select items without any predetermined pattern or criteria. The selection process relies on auditors' discretion and can involve simply picking items at random or based on convenience.
The specific goals and outcomes an IT audit is designed to achieve.
Testing for the actual existence of a financial statement item to ensure its validity and correctness.
The level of risk an organization is willing to accept in pursuit of its objectives.
Auditing Information Systems Copyright © 2024 by Amit M. Mehta is licensed under a Creative Commons Attribution-NonCommercial-ShareAlike 4.0 International License , except where otherwise noted.
Share This Book

IMAGES
VIDEO
COMMENTS
Both approaches require that the auditor use professional judgment in planning, performing, and evaluating a sample and in relating the evidential matter produced by the sample to other evidential matter when forming a conclusion about the related account balance or class of transactions.
judgmental sampling for illustrative case studies and 24 examples of sampling to draw inferences across the population, of which 19 were the basis for surveys. Can they provide strong evidence? In the Health area, four studies made extensive use of sampling and survey techniques to form the majority of the evidence which identified the ...
The importance of audit sampling lies in its ability to provide reasonable assurance while managing time and resource constraints. Properly executed sampling methods can significantly enhance the reliability of audit findings, thereby bolstering overall audit quality.
There are two general approaches to audit sampling: nonstatistical (targeted reviews) and statistical (probability). In selecting the approach, the audit team considers variables such as the audit budget, resources and time allocated, limitations and availability of data, and the size of sampling population.
Considered the industry-standard resource and updated as of March 1, 2014, the Audit Sampling Guide summarizes applicable requirements and practices, and delivers “how-to” advice to help...
We will cover the interplay between sample size, risk, and materiality, and how these factors influence the auditor’s decision-making process. Lastly, we will address the challenges and implications of sampling errors in IS audits, such as selection and measurement errors.