- Privacy Policy

Home » Research Results Section – Writing Guide and Examples

Research Results Section – Writing Guide and Examples
Table of Contents

Research Results
Research results refer to the findings and conclusions derived from a systematic investigation or study conducted to answer a specific question or hypothesis. These results are typically presented in a written report or paper and can include various forms of data such as numerical data, qualitative data, statistics, charts, graphs, and visual aids.
Results Section in Research
The results section of the research paper presents the findings of the study. It is the part of the paper where the researcher reports the data collected during the study and analyzes it to draw conclusions.
In the results section, the researcher should describe the data that was collected, the statistical analysis performed, and the findings of the study. It is important to be objective and not interpret the data in this section. Instead, the researcher should report the data as accurately and objectively as possible.
Structure of Research Results Section
The structure of the research results section can vary depending on the type of research conducted, but in general, it should contain the following components:
- Introduction: The introduction should provide an overview of the study, its aims, and its research questions. It should also briefly explain the methodology used to conduct the study.
- Data presentation : This section presents the data collected during the study. It may include tables, graphs, or other visual aids to help readers better understand the data. The data presented should be organized in a logical and coherent way, with headings and subheadings used to help guide the reader.
- Data analysis: In this section, the data presented in the previous section are analyzed and interpreted. The statistical tests used to analyze the data should be clearly explained, and the results of the tests should be presented in a way that is easy to understand.
- Discussion of results : This section should provide an interpretation of the results of the study, including a discussion of any unexpected findings. The discussion should also address the study’s research questions and explain how the results contribute to the field of study.
- Limitations: This section should acknowledge any limitations of the study, such as sample size, data collection methods, or other factors that may have influenced the results.
- Conclusions: The conclusions should summarize the main findings of the study and provide a final interpretation of the results. The conclusions should also address the study’s research questions and explain how the results contribute to the field of study.
- Recommendations : This section may provide recommendations for future research based on the study’s findings. It may also suggest practical applications for the study’s results in real-world settings.
Outline of Research Results Section
The following is an outline of the key components typically included in the Results section:
I. Introduction
- A brief overview of the research objectives and hypotheses
- A statement of the research question
II. Descriptive statistics
- Summary statistics (e.g., mean, standard deviation) for each variable analyzed
- Frequencies and percentages for categorical variables
III. Inferential statistics
- Results of statistical analyses, including tests of hypotheses
- Tables or figures to display statistical results
IV. Effect sizes and confidence intervals
- Effect sizes (e.g., Cohen’s d, odds ratio) to quantify the strength of the relationship between variables
- Confidence intervals to estimate the range of plausible values for the effect size
V. Subgroup analyses
- Results of analyses that examined differences between subgroups (e.g., by gender, age, treatment group)
VI. Limitations and assumptions
- Discussion of any limitations of the study and potential sources of bias
- Assumptions made in the statistical analyses
VII. Conclusions
- A summary of the key findings and their implications
- A statement of whether the hypotheses were supported or not
- Suggestions for future research
Example of Research Results Section
An Example of a Research Results Section could be:
- This study sought to examine the relationship between sleep quality and academic performance in college students.
- Hypothesis : College students who report better sleep quality will have higher GPAs than those who report poor sleep quality.
- Methodology : Participants completed a survey about their sleep habits and academic performance.
II. Participants
- Participants were college students (N=200) from a mid-sized public university in the United States.
- The sample was evenly split by gender (50% female, 50% male) and predominantly white (85%).
- Participants were recruited through flyers and online advertisements.
III. Results
- Participants who reported better sleep quality had significantly higher GPAs (M=3.5, SD=0.5) than those who reported poor sleep quality (M=2.9, SD=0.6).
- See Table 1 for a summary of the results.
- Participants who reported consistent sleep schedules had higher GPAs than those with irregular sleep schedules.
IV. Discussion
- The results support the hypothesis that better sleep quality is associated with higher academic performance in college students.
- These findings have implications for college students, as prioritizing sleep could lead to better academic outcomes.
- Limitations of the study include self-reported data and the lack of control for other variables that could impact academic performance.
V. Conclusion
- College students who prioritize sleep may see a positive impact on their academic performance.
- These findings highlight the importance of sleep in academic success.
- Future research could explore interventions to improve sleep quality in college students.
Example of Research Results in Research Paper :
Our study aimed to compare the performance of three different machine learning algorithms (Random Forest, Support Vector Machine, and Neural Network) in predicting customer churn in a telecommunications company. We collected a dataset of 10,000 customer records, with 20 predictor variables and a binary churn outcome variable.
Our analysis revealed that all three algorithms performed well in predicting customer churn, with an overall accuracy of 85%. However, the Random Forest algorithm showed the highest accuracy (88%), followed by the Support Vector Machine (86%) and the Neural Network (84%).
Furthermore, we found that the most important predictor variables for customer churn were monthly charges, contract type, and tenure. Random Forest identified monthly charges as the most important variable, while Support Vector Machine and Neural Network identified contract type as the most important.
Overall, our results suggest that machine learning algorithms can be effective in predicting customer churn in a telecommunications company, and that Random Forest is the most accurate algorithm for this task.
Example 3 :
Title : The Impact of Social Media on Body Image and Self-Esteem
Abstract : This study aimed to investigate the relationship between social media use, body image, and self-esteem among young adults. A total of 200 participants were recruited from a university and completed self-report measures of social media use, body image satisfaction, and self-esteem.
Results: The results showed that social media use was significantly associated with body image dissatisfaction and lower self-esteem. Specifically, participants who reported spending more time on social media platforms had lower levels of body image satisfaction and self-esteem compared to those who reported less social media use. Moreover, the study found that comparing oneself to others on social media was a significant predictor of body image dissatisfaction and lower self-esteem.
Conclusion : These results suggest that social media use can have negative effects on body image satisfaction and self-esteem among young adults. It is important for individuals to be mindful of their social media use and to recognize the potential negative impact it can have on their mental health. Furthermore, interventions aimed at promoting positive body image and self-esteem should take into account the role of social media in shaping these attitudes and behaviors.
Importance of Research Results
Research results are important for several reasons, including:
- Advancing knowledge: Research results can contribute to the advancement of knowledge in a particular field, whether it be in science, technology, medicine, social sciences, or humanities.
- Developing theories: Research results can help to develop or modify existing theories and create new ones.
- Improving practices: Research results can inform and improve practices in various fields, such as education, healthcare, business, and public policy.
- Identifying problems and solutions: Research results can identify problems and provide solutions to complex issues in society, including issues related to health, environment, social justice, and economics.
- Validating claims : Research results can validate or refute claims made by individuals or groups in society, such as politicians, corporations, or activists.
- Providing evidence: Research results can provide evidence to support decision-making, policy-making, and resource allocation in various fields.
How to Write Results in A Research Paper
Here are some general guidelines on how to write results in a research paper:
- Organize the results section: Start by organizing the results section in a logical and coherent manner. Divide the section into subsections if necessary, based on the research questions or hypotheses.
- Present the findings: Present the findings in a clear and concise manner. Use tables, graphs, and figures to illustrate the data and make the presentation more engaging.
- Describe the data: Describe the data in detail, including the sample size, response rate, and any missing data. Provide relevant descriptive statistics such as means, standard deviations, and ranges.
- Interpret the findings: Interpret the findings in light of the research questions or hypotheses. Discuss the implications of the findings and the extent to which they support or contradict existing theories or previous research.
- Discuss the limitations : Discuss the limitations of the study, including any potential sources of bias or confounding factors that may have affected the results.
- Compare the results : Compare the results with those of previous studies or theoretical predictions. Discuss any similarities, differences, or inconsistencies.
- Avoid redundancy: Avoid repeating information that has already been presented in the introduction or methods sections. Instead, focus on presenting new and relevant information.
- Be objective: Be objective in presenting the results, avoiding any personal biases or interpretations.
When to Write Research Results
Here are situations When to Write Research Results”
- After conducting research on the chosen topic and obtaining relevant data, organize the findings in a structured format that accurately represents the information gathered.
- Once the data has been analyzed and interpreted, and conclusions have been drawn, begin the writing process.
- Before starting to write, ensure that the research results adhere to the guidelines and requirements of the intended audience, such as a scientific journal or academic conference.
- Begin by writing an abstract that briefly summarizes the research question, methodology, findings, and conclusions.
- Follow the abstract with an introduction that provides context for the research, explains its significance, and outlines the research question and objectives.
- The next section should be a literature review that provides an overview of existing research on the topic and highlights the gaps in knowledge that the current research seeks to address.
- The methodology section should provide a detailed explanation of the research design, including the sample size, data collection methods, and analytical techniques used.
- Present the research results in a clear and concise manner, using graphs, tables, and figures to illustrate the findings.
- Discuss the implications of the research results, including how they contribute to the existing body of knowledge on the topic and what further research is needed.
- Conclude the paper by summarizing the main findings, reiterating the significance of the research, and offering suggestions for future research.
Purpose of Research Results
The purposes of Research Results are as follows:
- Informing policy and practice: Research results can provide evidence-based information to inform policy decisions, such as in the fields of healthcare, education, and environmental regulation. They can also inform best practices in fields such as business, engineering, and social work.
- Addressing societal problems : Research results can be used to help address societal problems, such as reducing poverty, improving public health, and promoting social justice.
- Generating economic benefits : Research results can lead to the development of new products, services, and technologies that can create economic value and improve quality of life.
- Supporting academic and professional development : Research results can be used to support academic and professional development by providing opportunities for students, researchers, and practitioners to learn about new findings and methodologies in their field.
- Enhancing public understanding: Research results can help to educate the public about important issues and promote scientific literacy, leading to more informed decision-making and better public policy.
- Evaluating interventions: Research results can be used to evaluate the effectiveness of interventions, such as treatments, educational programs, and social policies. This can help to identify areas where improvements are needed and guide future interventions.
- Contributing to scientific progress: Research results can contribute to the advancement of science by providing new insights and discoveries that can lead to new theories, methods, and techniques.
- Informing decision-making : Research results can provide decision-makers with the information they need to make informed decisions. This can include decision-making at the individual, organizational, or governmental levels.
- Fostering collaboration : Research results can facilitate collaboration between researchers and practitioners, leading to new partnerships, interdisciplinary approaches, and innovative solutions to complex problems.
Advantages of Research Results
Some Advantages of Research Results are as follows:
- Improved decision-making: Research results can help inform decision-making in various fields, including medicine, business, and government. For example, research on the effectiveness of different treatments for a particular disease can help doctors make informed decisions about the best course of treatment for their patients.
- Innovation : Research results can lead to the development of new technologies, products, and services. For example, research on renewable energy sources can lead to the development of new and more efficient ways to harness renewable energy.
- Economic benefits: Research results can stimulate economic growth by providing new opportunities for businesses and entrepreneurs. For example, research on new materials or manufacturing techniques can lead to the development of new products and processes that can create new jobs and boost economic activity.
- Improved quality of life: Research results can contribute to improving the quality of life for individuals and society as a whole. For example, research on the causes of a particular disease can lead to the development of new treatments and cures, improving the health and well-being of millions of people.
About the author
Muhammad Hassan
Researcher, Academic Writer, Web developer
You may also like

How to Cite Research Paper – All Formats and...

Data Collection – Methods Types and Examples

Delimitations in Research – Types, Examples and...

Research Paper Format – Types, Examples and...

Research Process – Steps, Examples and Tips

Research Design – Types, Methods and Examples
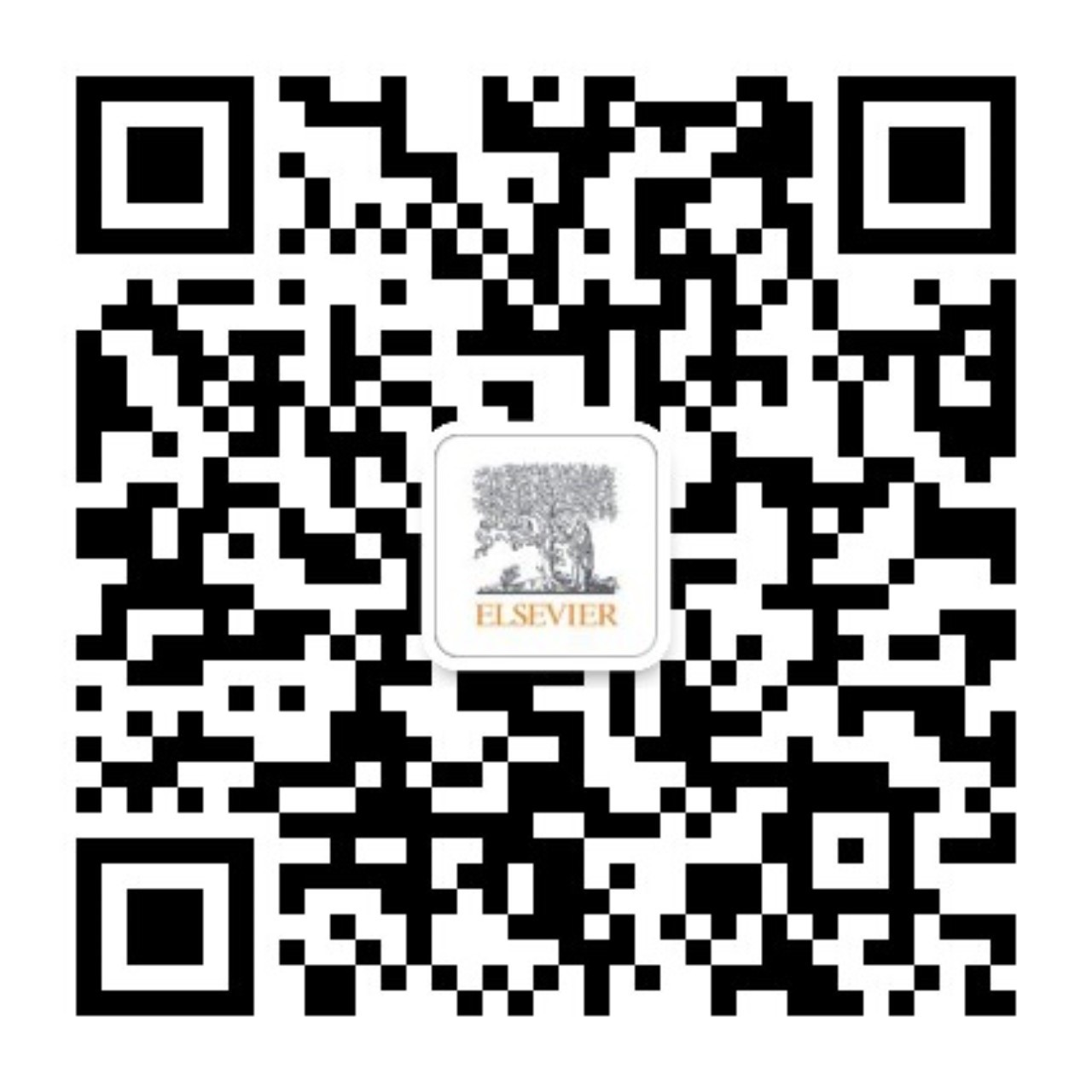
- Manuscript Preparation
How to write the results section of a research paper
- 3 minute read
- 62.2K views
Table of Contents
At its core, a research paper aims to fill a gap in the research on a given topic. As a result, the results section of the paper, which describes the key findings of the study, is often considered the core of the paper. This is the section that gets the most attention from reviewers, peers, students, and any news organization reporting on your findings. Writing a clear, concise, and logical results section is, therefore, one of the most important parts of preparing your manuscript.
Difference between results and discussion
Before delving into how to write the results section, it is important to first understand the difference between the results and discussion sections. The results section needs to detail the findings of the study. The aim of this section is not to draw connections between the different findings or to compare it to previous findings in literature—that is the purview of the discussion section. Unlike the discussion section, which can touch upon the hypothetical, the results section needs to focus on the purely factual. In some cases, it may even be preferable to club these two sections together into a single section. For example, while writing a review article, it can be worthwhile to club these two sections together, as the main results in this case are the conclusions that can be drawn from the literature.
Structure of the results section
Although the main purpose of the results section in a research paper is to report the findings, it is necessary to present an introduction and repeat the research question. This establishes a connection to the previous section of the paper and creates a smooth flow of information.
Next, the results section needs to communicate the findings of your research in a systematic manner. The section needs to be organized such that the primary research question is addressed first, then the secondary research questions. If the research addresses multiple questions, the results section must individually connect with each of the questions. This ensures clarity and minimizes confusion while reading.
Consider representing your results visually. For example, graphs, tables, and other figures can help illustrate the findings of your paper, especially if there is a large amount of data in the results.
Remember, an appealing results section can help peer reviewers better understand the merits of your research, thereby increasing your chances of publication.
Practical guidance for writing an effective results section for a research paper
- Always use simple and clear language. Avoid the use of uncertain or out-of-focus expressions.
- The findings of the study must be expressed in an objective and unbiased manner. While it is acceptable to correlate certain findings in the discussion section, it is best to avoid overinterpreting the results.
- If the research addresses more than one hypothesis, use sub-sections to describe the results. This prevents confusion and promotes understanding.
- Ensure that negative results are included in this section, even if they do not support the research hypothesis.
- Wherever possible, use illustrations like tables, figures, charts, or other visual representations to showcase the results of your research paper. Mention these illustrations in the text, but do not repeat the information that they convey.
- For statistical data, it is adequate to highlight the tests and explain their results. The initial or raw data should not be mentioned in the results section of a research paper.
The results section of a research paper is usually the most impactful section because it draws the greatest attention. Regardless of the subject of your research paper, a well-written results section is capable of generating interest in your research.
For detailed information and assistance on writing the results of a research paper, refer to Elsevier Author Services.
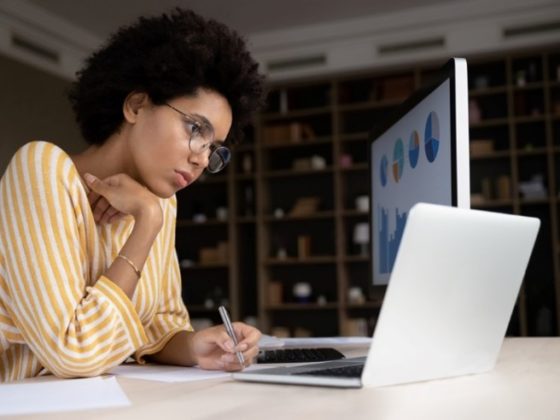
- Research Process
Writing a good review article
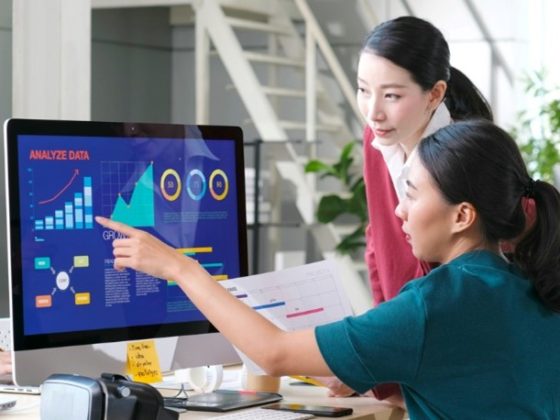
Why is data validation important in research?
You may also like.
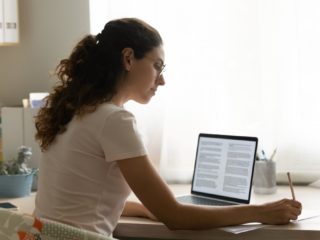
Make Hook, Line, and Sinker: The Art of Crafting Engaging Introductions
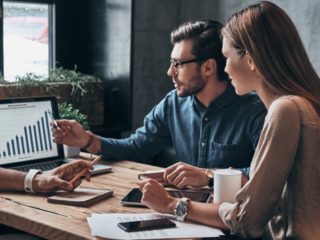
Can Describing Study Limitations Improve the Quality of Your Paper?
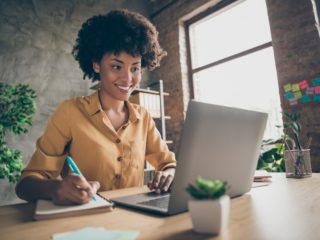
A Guide to Crafting Shorter, Impactful Sentences in Academic Writing
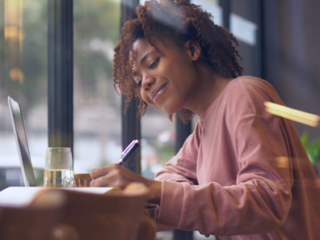
6 Steps to Write an Excellent Discussion in Your Manuscript
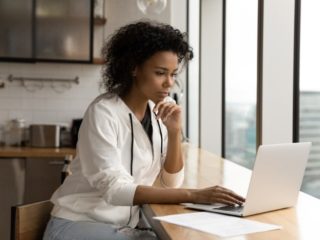
How to Write Clear and Crisp Civil Engineering Papers? Here are 5 Key Tips to Consider
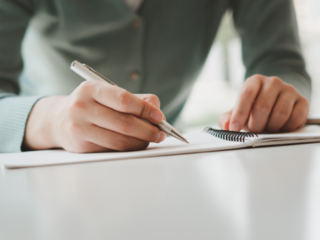
The Clear Path to An Impactful Paper: ②
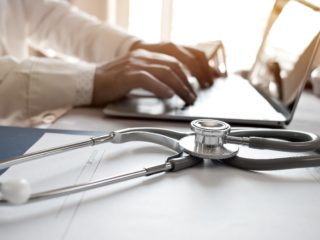
The Essentials of Writing to Communicate Research in Medicine
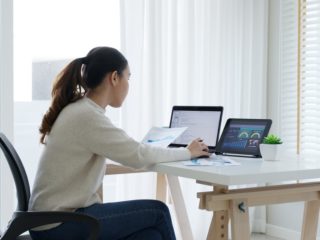
Changing Lines: Sentence Patterns in Academic Writing
Input your search keywords and press Enter.
- USC Libraries
- Research Guides
Organizing Your Social Sciences Research Paper
- 7. The Results
- Purpose of Guide
- Design Flaws to Avoid
- Independent and Dependent Variables
- Glossary of Research Terms
- Reading Research Effectively
- Narrowing a Topic Idea
- Broadening a Topic Idea
- Extending the Timeliness of a Topic Idea
- Academic Writing Style
- Applying Critical Thinking
- Choosing a Title
- Making an Outline
- Paragraph Development
- Research Process Video Series
- Executive Summary
- The C.A.R.S. Model
- Background Information
- The Research Problem/Question
- Theoretical Framework
- Citation Tracking
- Content Alert Services
- Evaluating Sources
- Primary Sources
- Secondary Sources
- Tiertiary Sources
- Scholarly vs. Popular Publications
- Qualitative Methods
- Quantitative Methods
- Insiderness
- Using Non-Textual Elements
- Limitations of the Study
- Common Grammar Mistakes
- Writing Concisely
- Avoiding Plagiarism
- Footnotes or Endnotes?
- Further Readings
- Generative AI and Writing
- USC Libraries Tutorials and Other Guides
- Bibliography
The results section is where you report the findings of your study based upon the methodology [or methodologies] you applied to gather information. The results section should state the findings of the research arranged in a logical sequence without bias or interpretation. A section describing results should be particularly detailed if your paper includes data generated from your own research.
Annesley, Thomas M. "Show Your Cards: The Results Section and the Poker Game." Clinical Chemistry 56 (July 2010): 1066-1070.
Importance of a Good Results Section
When formulating the results section, it's important to remember that the results of a study do not prove anything . Findings can only confirm or reject the hypothesis underpinning your study. However, the act of articulating the results helps you to understand the problem from within, to break it into pieces, and to view the research problem from various perspectives.
The page length of this section is set by the amount and types of data to be reported . Be concise. Use non-textual elements appropriately, such as figures and tables, to present findings more effectively. In deciding what data to describe in your results section, you must clearly distinguish information that would normally be included in a research paper from any raw data or other content that could be included as an appendix. In general, raw data that has not been summarized should not be included in the main text of your paper unless requested to do so by your professor.
Avoid providing data that is not critical to answering the research question . The background information you described in the introduction section should provide the reader with any additional context or explanation needed to understand the results. A good strategy is to always re-read the background section of your paper after you have written up your results to ensure that the reader has enough context to understand the results [and, later, how you interpreted the results in the discussion section of your paper that follows].
Bavdekar, Sandeep B. and Sneha Chandak. "Results: Unraveling the Findings." Journal of the Association of Physicians of India 63 (September 2015): 44-46; Brett, Paul. "A Genre Analysis of the Results Section of Sociology Articles." English for Specific Speakers 13 (1994): 47-59; Go to English for Specific Purposes on ScienceDirect;Burton, Neil et al. Doing Your Education Research Project . Los Angeles, CA: SAGE, 2008; Results. The Structure, Format, Content, and Style of a Journal-Style Scientific Paper. Department of Biology. Bates College; Kretchmer, Paul. Twelve Steps to Writing an Effective Results Section. San Francisco Edit; "Reporting Findings." In Making Sense of Social Research Malcolm Williams, editor. (London;: SAGE Publications, 2003) pp. 188-207.
Structure and Writing Style
I. Organization and Approach
For most research papers in the social and behavioral sciences, there are two possible ways of organizing the results . Both approaches are appropriate in how you report your findings, but use only one approach.
- Present a synopsis of the results followed by an explanation of key findings . This approach can be used to highlight important findings. For example, you may have noticed an unusual correlation between two variables during the analysis of your findings. It is appropriate to highlight this finding in the results section. However, speculating as to why this correlation exists and offering a hypothesis about what may be happening belongs in the discussion section of your paper.
- Present a result and then explain it, before presenting the next result then explaining it, and so on, then end with an overall synopsis . This is the preferred approach if you have multiple results of equal significance. It is more common in longer papers because it helps the reader to better understand each finding. In this model, it is helpful to provide a brief conclusion that ties each of the findings together and provides a narrative bridge to the discussion section of the your paper.
NOTE : Just as the literature review should be arranged under conceptual categories rather than systematically describing each source, you should also organize your findings under key themes related to addressing the research problem. This can be done under either format noted above [i.e., a thorough explanation of the key results or a sequential, thematic description and explanation of each finding].
II. Content
In general, the content of your results section should include the following:
- Introductory context for understanding the results by restating the research problem underpinning your study . This is useful in re-orientating the reader's focus back to the research problem after having read a review of the literature and your explanation of the methods used for gathering and analyzing information.
- Inclusion of non-textual elements, such as, figures, charts, photos, maps, tables, etc. to further illustrate key findings, if appropriate . Rather than relying entirely on descriptive text, consider how your findings can be presented visually. This is a helpful way of condensing a lot of data into one place that can then be referred to in the text. Consider referring to appendices if there is a lot of non-textual elements.
- A systematic description of your results, highlighting for the reader observations that are most relevant to the topic under investigation . Not all results that emerge from the methodology used to gather information may be related to answering the " So What? " question. Do not confuse observations with interpretations; observations in this context refers to highlighting important findings you discovered through a process of reviewing prior literature and gathering data.
- The page length of your results section is guided by the amount and types of data to be reported . However, focus on findings that are important and related to addressing the research problem. It is not uncommon to have unanticipated results that are not relevant to answering the research question. This is not to say that you don't acknowledge tangential findings and, in fact, can be referred to as areas for further research in the conclusion of your paper. However, spending time in the results section describing tangential findings clutters your overall results section and distracts the reader.
- A short paragraph that concludes the results section by synthesizing the key findings of the study . Highlight the most important findings you want readers to remember as they transition into the discussion section. This is particularly important if, for example, there are many results to report, the findings are complicated or unanticipated, or they are impactful or actionable in some way [i.e., able to be pursued in a feasible way applied to practice].
NOTE: Always use the past tense when referring to your study's findings. Reference to findings should always be described as having already happened because the method used to gather the information has been completed.
III. Problems to Avoid
When writing the results section, avoid doing the following :
- Discussing or interpreting your results . Save this for the discussion section of your paper, although where appropriate, you should compare or contrast specific results to those found in other studies [e.g., "Similar to the work of Smith [1990], one of the findings of this study is the strong correlation between motivation and academic achievement...."].
- Reporting background information or attempting to explain your findings. This should have been done in your introduction section, but don't panic! Often the results of a study point to the need for additional background information or to explain the topic further, so don't think you did something wrong. Writing up research is rarely a linear process. Always revise your introduction as needed.
- Ignoring negative results . A negative result generally refers to a finding that does not support the underlying assumptions of your study. Do not ignore them. Document these findings and then state in your discussion section why you believe a negative result emerged from your study. Note that negative results, and how you handle them, can give you an opportunity to write a more engaging discussion section, therefore, don't be hesitant to highlight them.
- Including raw data or intermediate calculations . Ask your professor if you need to include any raw data generated by your study, such as transcripts from interviews or data files. If raw data is to be included, place it in an appendix or set of appendices that are referred to in the text.
- Be as factual and concise as possible in reporting your findings . Do not use phrases that are vague or non-specific, such as, "appeared to be greater than other variables..." or "demonstrates promising trends that...." Subjective modifiers should be explained in the discussion section of the paper [i.e., why did one variable appear greater? Or, how does the finding demonstrate a promising trend?].
- Presenting the same data or repeating the same information more than once . If you want to highlight a particular finding, it is appropriate to do so in the results section. However, you should emphasize its significance in relation to addressing the research problem in the discussion section. Do not repeat it in your results section because you can do that in the conclusion of your paper.
- Confusing figures with tables . Be sure to properly label any non-textual elements in your paper. Don't call a chart an illustration or a figure a table. If you are not sure, go here .
Annesley, Thomas M. "Show Your Cards: The Results Section and the Poker Game." Clinical Chemistry 56 (July 2010): 1066-1070; Bavdekar, Sandeep B. and Sneha Chandak. "Results: Unraveling the Findings." Journal of the Association of Physicians of India 63 (September 2015): 44-46; Burton, Neil et al. Doing Your Education Research Project . Los Angeles, CA: SAGE, 2008; Caprette, David R. Writing Research Papers. Experimental Biosciences Resources. Rice University; Hancock, Dawson R. and Bob Algozzine. Doing Case Study Research: A Practical Guide for Beginning Researchers . 2nd ed. New York: Teachers College Press, 2011; Introduction to Nursing Research: Reporting Research Findings. Nursing Research: Open Access Nursing Research and Review Articles. (January 4, 2012); Kretchmer, Paul. Twelve Steps to Writing an Effective Results Section. San Francisco Edit ; Ng, K. H. and W. C. Peh. "Writing the Results." Singapore Medical Journal 49 (2008): 967-968; Reporting Research Findings. Wilder Research, in partnership with the Minnesota Department of Human Services. (February 2009); Results. The Structure, Format, Content, and Style of a Journal-Style Scientific Paper. Department of Biology. Bates College; Schafer, Mickey S. Writing the Results. Thesis Writing in the Sciences. Course Syllabus. University of Florida.
Writing Tip
Why Don't I Just Combine the Results Section with the Discussion Section?
It's not unusual to find articles in scholarly social science journals where the author(s) have combined a description of the findings with a discussion about their significance and implications. You could do this. However, if you are inexperienced writing research papers, consider creating two distinct sections for each section in your paper as a way to better organize your thoughts and, by extension, your paper. Think of the results section as the place where you report what your study found; think of the discussion section as the place where you interpret the information and answer the "So What?" question. As you become more skilled writing research papers, you can consider melding the results of your study with a discussion of its implications.
Driscoll, Dana Lynn and Aleksandra Kasztalska. Writing the Experimental Report: Methods, Results, and Discussion. The Writing Lab and The OWL. Purdue University.
- << Previous: Insiderness
- Next: Using Non-Textual Elements >>
- Last Updated: May 9, 2024 11:05 AM
- URL: https://libguides.usc.edu/writingguide

Organizing Academic Research Papers: 7. The Results
- Purpose of Guide
- Design Flaws to Avoid
- Glossary of Research Terms
- Narrowing a Topic Idea
- Broadening a Topic Idea
- Extending the Timeliness of a Topic Idea
- Academic Writing Style
- Choosing a Title
- Making an Outline
- Paragraph Development
- Executive Summary
- Background Information
- The Research Problem/Question
- Theoretical Framework
- Citation Tracking
- Content Alert Services
- Evaluating Sources
- Primary Sources
- Secondary Sources
- Tertiary Sources
- What Is Scholarly vs. Popular?
- Qualitative Methods
- Quantitative Methods
- Using Non-Textual Elements
- Limitations of the Study
- Common Grammar Mistakes
- Avoiding Plagiarism
- Footnotes or Endnotes?
- Further Readings
- Annotated Bibliography
- Dealing with Nervousness
- Using Visual Aids
- Grading Someone Else's Paper
- How to Manage Group Projects
- Multiple Book Review Essay
- Reviewing Collected Essays
- About Informed Consent
- Writing Field Notes
- Writing a Policy Memo
- Writing a Research Proposal
- Acknowledgements
The results section of the research paper is where you report the findings of your study based upon the information gathered as a result of the methodology [or methodologies] you applied. The results section should simply state the findings, without bias or interpretation, and arranged in a logical sequence. The results section should always be written in the past tense. A section describing results [a.k.a., "findings"] is particularly necessary if your paper includes data generated from your own research.
Importance of a Good Results Section
When formulating the results section, it's important to remember that the results of a study do not prove anything . Research results can only confirm or reject the research problem underpinning your study. However, the act of articulating the results helps you to understand the problem from within, to break it into pieces, and to view the research problem from various perspectives.
The page length of this section is set by the amount and types of data to be reported . Be concise, using non-textual elements, such as figures and tables, if appropriate, to present results more effectively. In deciding what data to describe in your results section, you must clearly distinguish material that would normally be included in a research paper from any raw data or other material that could be included as an appendix. In general, raw data should not be included in the main text of your paper unless requested to do so by your professor.
Avoid providing data that is not critical to answering the research question . The background information you described in the introduction section should provide the reader with any additional context or explanation needed to understand the results. A good rule is to always re-read the background section of your paper after you have written up your results to ensure that the reader has enough context to understand the results [and, later, how you interpreted the results in the discussion section of your paper].
Bates College; Burton, Neil et al. Doing Your Education Research Project . Los Angeles, CA: SAGE, 2008; Results . The Structure, Format, Content, and Style of a Journal-Style Scientific Paper. Department of Biology. Bates College.
Structure and Writing Style
I. Structure and Approach
For most research paper formats, there are two ways of presenting and organizing the results .
- Present the results followed by a short explanation of the findings . For example, you may have noticed an unusual correlation between two variables during the analysis of your findings. It is correct to point this out in the results section. However, speculating as to why this correlation exists, and offering a hypothesis about what may be happening, belongs in the discussion section of your paper.
- Present a section and then discuss it, before presenting the next section then discussing it, and so on . This is more common in longer papers because it helps the reader to better understand each finding. In this model, it can be helpful to provide a brief conclusion in the results section that ties each of the findings together and links to the discussion.
NOTE: The discussion section should generally follow the same format chosen in presenting and organizing the results.
II. Content
In general, the content of your results section should include the following elements:
- An introductory context for understanding the results by restating the research problem that underpins the purpose of your study.
- A summary of your key findings arranged in a logical sequence that generally follows your methodology section.
- Inclusion of non-textual elements, such as, figures, charts, photos, maps, tables, etc. to further illustrate the findings, if appropriate.
- In the text, a systematic description of your results, highlighting for the reader observations that are most relevant to the topic under investigation [remember that not all results that emerge from the methodology that you used to gather the data may be relevant].
- Use of the past tense when refering to your results.
- The page length of your results section is guided by the amount and types of data to be reported. However, focus only on findings that are important and related to addressing the research problem.
Using Non-textual Elements
- Either place figures, tables, charts, etc. within the text of the result, or include them in the back of the report--do one or the other but never do both.
- In the text, refer to each non-textual element in numbered order [e.g., Table 1, Table 2; Chart 1, Chart 2; Map 1, Map 2].
- If you place non-textual elements at the end of the report, make sure they are clearly distinguished from any attached appendix materials, such as raw data.
- Regardless of placement, each non-textual element must be numbered consecutively and complete with caption [caption goes under the figure, table, chart, etc.]
- Each non-textual element must be titled, numbered consecutively, and complete with a heading [title with description goes above the figure, table, chart, etc.].
- In proofreading your results section, be sure that each non-textual element is sufficiently complete so that it could stand on its own, separate from the text.
III. Problems to Avoid
When writing the results section, avoid doing the following :
- Discussing or interpreting your results . Save all this for the next section of your paper, although where appropriate, you should compare or contrast specific results to those found in other studies [e.g., "Similar to Smith [1990], one of the findings of this study is the strong correlation between motivation and academic achievement...."].
- Reporting background information or attempting to explain your findings ; this should have been done in your Introduction section, but don't panic! Often the results of a study point to the need to provide additional background information or to explain the topic further, so don't think you did something wrong. Revise your introduction as needed.
- Ignoring negative results . If some of your results fail to support your hypothesis, do not ignore them. Document them, then state in your discussion section why you believe a negative result emerged from your study. Note that negative results, and how you handle them, often provides you with the opportunity to write a more engaging discussion section, therefore, don't be afraid to highlight them.
- Including raw data or intermediate calculations . Ask your professor if you need to include any raw data generated by your study, such as transcripts from interviews or data files. If raw data is to be included, place it in an appendix or set of appendices that are referred to in the text.
- Be as factual and concise as possible in reporting your findings . Do not use phrases that are vague or non-specific, such as, "appeared to be greater or lesser than..." or "demonstrates promising trends that...."
- Presenting the same data or repeating the same information more than once . If you feel the need to highlight something, you will have a chance to do that in the discussion section.
- Confusing figures with tables . Be sure to properly label any non-textual elements in your paper. If you are not sure, look up the term in a dictionary.
Burton, Neil et al. Doing Your Education Research Project . Los Angeles, CA: SAGE, 2008; Caprette, David R. Writing Research Papers . Experimental Biosciences Resources. Rice University; Hancock, Dawson R. and Bob Algozzine. Doing Case Study Research: A Practical Guide for Beginning Researchers . 2nd ed. New York: Teachers College Press, 2011; Introduction to Nursing Research: Reporting Research Findings. Nursing Research: Open Access Nursing Research and Review Articles. (January 4, 2012); Reporting Research Findings. Wilder Research, in partnership with the Minnesota Department of Human Services. (February 2009); Results . The Structure, Format, Content, and Style of a Journal-Style Scientific Paper. Department of Biology. Bates College; Schafer, Mickey S. Writing the Results . Thesis Writing in the Sciences. Course Syllabus. University of Florida.
Writing Tip
Why Don't I Just Combine the Results Section with the Discussion Section?
It's not unusual to find articles in social science journals where the author(s) have combined a description of the findings from the study with a discussion about their implications. You could do this. However, if you are inexperienced writing research papers, consider creating two sections for each element in your paper as a way to better organize your thoughts and, by extension, your paper. Think of the results section as the place where you report what your study found; think of the discussion section as the place where you interpret your data and answer the "so what?" question. As you become more skilled writing research papers, you may want to meld the results of your study with a discussion of its implications.
- << Previous: Quantitative Methods
- Next: Using Non-Textual Elements >>
- Last Updated: Jul 18, 2023 11:58 AM
- URL: https://library.sacredheart.edu/c.php?g=29803
- QuickSearch
- Library Catalog
- Databases A-Z
- Publication Finder
- Course Reserves
- Citation Linker
- Digital Commons
- Our Website
Research Support
- Ask a Librarian
- Appointments
- Interlibrary Loan (ILL)
- Research Guides
- Databases by Subject
- Citation Help
Using the Library
- Reserve a Group Study Room
- Renew Books
- Honors Study Rooms
- Off-Campus Access
- Library Policies
- Library Technology
User Information
- Grad Students
- Online Students
- COVID-19 Updates
- Staff Directory
- News & Announcements
- Library Newsletter
My Accounts
- Interlibrary Loan
- Staff Site Login

FIND US ON
How to Present Results in a Research Paper
- First Online: 01 October 2023
Cite this chapter
- Aparna Mukherjee 4 ,
- Gunjan Kumar 4 &
- Rakesh Lodha 5
734 Accesses
The results section is the core of a research manuscript where the study data and analyses are presented in an organized, uncluttered manner such that the reader can easily understand and interpret the findings. This section is completely factual; there is no place for opinions or explanations from the authors. The results should correspond to the objectives of the study in an orderly manner. Self-explanatory tables and figures add value to this section and make data presentation more convenient and appealing. The results presented in this section should have a link with both the preceding methods section and the following discussion section. A well-written, articulate results section lends clarity and credibility to the research paper and the study as a whole. This chapter provides an overview and important pointers to effective drafting of the results section in a research manuscript and also in theses.
This is a preview of subscription content, log in via an institution to check access.
Access this chapter
- Available as PDF
- Read on any device
- Instant download
- Own it forever
- Available as EPUB and PDF
- Durable hardcover edition
- Dispatched in 3 to 5 business days
- Free shipping worldwide - see info
Tax calculation will be finalised at checkout
Purchases are for personal use only
Institutional subscriptions
Kallestinova ED (2011) How to write your first research paper. Yale J Biol Med 84(3):181–190
PubMed PubMed Central Google Scholar
STROBE. STROBE. [cited 2022 Nov 10]. https://www.strobe-statement.org/
Consort—Welcome to the CONSORT Website. http://www.consort-statement.org/ . Accessed 10 Nov 2022
Korevaar DA, Cohen JF, Reitsma JB, Bruns DE, Gatsonis CA, Glasziou PP et al (2016) Updating standards for reporting diagnostic accuracy: the development of STARD 2015. Res Integr Peer Rev 1(1):7
Article PubMed PubMed Central Google Scholar
Page MJ, McKenzie JE, Bossuyt PM, Boutron I, Hoffmann TC, Mulrow CD et al (2021) The PRISMA 2020 statement: an updated guideline for reporting systematic reviews. BMJ 372:n71
Page MJ, Moher D, Bossuyt PM, Boutron I, Hoffmann TC, Mulrow CD et al (2021) PRISMA 2020 explanation and elaboration: updated guidance and exemplars for reporting systematic reviews. BMJ 372:n160
Consolidated criteria for reporting qualitative research (COREQ): a 32-item checklist for interviews and focus groups | EQUATOR Network. https://www.equator-network.org/reporting-guidelines/coreq/ . Accessed 10 Nov 2022
Aggarwal R, Sahni P (2015) The results section. In: Aggarwal R, Sahni P (eds) Reporting and publishing research in the biomedical sciences, 1st edn. National Medical Journal of India, Delhi, pp 24–44
Google Scholar
Mukherjee A, Lodha R (2016) Writing the results. Indian Pediatr 53(5):409–415
Article PubMed Google Scholar
Lodha R, Randev S, Kabra SK (2016) Oral antibiotics for community acquired pneumonia with chest indrawing in children aged below five years: a systematic review. Indian Pediatr 53(6):489–495
Anderson C (2010) Presenting and evaluating qualitative research. Am J Pharm Educ 74(8):141
Roberts C, Kumar K, Finn G (2020) Navigating the qualitative manuscript writing process: some tips for authors and reviewers. BMC Med Educ 20:439
Bigby C (2015) Preparing manuscripts that report qualitative research: avoiding common pitfalls and illegitimate questions. Aust Soc Work 68(3):384–391
Article Google Scholar
Vincent BP, Kumar G, Parameswaran S, Kar SS (2019) Barriers and suggestions towards deceased organ donation in a government tertiary care teaching hospital: qualitative study using socio-ecological model framework. Indian J Transplant 13(3):194
McCormick JB, Hopkins MA (2021) Exploring public concerns for sharing and governance of personal health information: a focus group study. JAMIA Open 4(4):ooab098
Groenland -emeritus professor E. Employing the matrix method as a tool for the analysis of qualitative research data in the business domain. Rochester, NY; 2014. https://papers.ssrn.com/abstract=2495330 . Accessed 10 Nov 2022
Download references
Acknowledgments
The book chapter is derived in part from our article “Mukherjee A, Lodha R. Writing the Results. Indian Pediatr. 2016 May 8;53(5):409-15.” We thank the Editor-in-Chief of the journal “Indian Pediatrics” for the permission for the same.
Author information
Authors and affiliations.
Clinical Studies, Trials and Projection Unit, Indian Council of Medical Research, New Delhi, India
Aparna Mukherjee & Gunjan Kumar
Department of Pediatrics, All India Institute of Medical Sciences, New Delhi, India
Rakesh Lodha
You can also search for this author in PubMed Google Scholar
Corresponding author
Correspondence to Rakesh Lodha .
Editor information
Editors and affiliations.
Retired Senior Expert Pharmacologist at the Office of Cardiology, Hematology, Endocrinology, and Nephrology, Center for Drug Evaluation and Research, US Food and Drug Administration, Silver Spring, MD, USA
Gowraganahalli Jagadeesh
Professor & Director, Research Training and Publications, The Office of Research and Development, Periyar Maniammai Institute of Science & Technology (Deemed to be University), Vallam, Tamil Nadu, India
Pitchai Balakumar
Division Cardiology & Nephrology, Office of Cardiology, Hematology, Endocrinology and Nephrology, Center for Drug Evaluation and Research, US Food and Drug Administration, Silver Spring, MD, USA
Fortunato Senatore
Ethics declarations
Rights and permissions.
Reprints and permissions
Copyright information
© 2023 The Author(s), under exclusive license to Springer Nature Singapore Pte Ltd.
About this chapter
Mukherjee, A., Kumar, G., Lodha, R. (2023). How to Present Results in a Research Paper. In: Jagadeesh, G., Balakumar, P., Senatore, F. (eds) The Quintessence of Basic and Clinical Research and Scientific Publishing. Springer, Singapore. https://doi.org/10.1007/978-981-99-1284-1_44
Download citation
DOI : https://doi.org/10.1007/978-981-99-1284-1_44
Published : 01 October 2023
Publisher Name : Springer, Singapore
Print ISBN : 978-981-99-1283-4
Online ISBN : 978-981-99-1284-1
eBook Packages : Biomedical and Life Sciences Biomedical and Life Sciences (R0)
Share this chapter
Anyone you share the following link with will be able to read this content:
Sorry, a shareable link is not currently available for this article.
Provided by the Springer Nature SharedIt content-sharing initiative
- Publish with us
Policies and ethics
- Find a journal
- Track your research
- Affiliate Program

- UNITED STATES
- 台灣 (TAIWAN)
- TÜRKIYE (TURKEY)
- Academic Editing Services
- - Research Paper
- - Journal Manuscript
- - Dissertation
- - College & University Assignments
- Admissions Editing Services
- - Application Essay
- - Personal Statement
- - Recommendation Letter
- - Cover Letter
- - CV/Resume
- Business Editing Services
- - Business Documents
- - Report & Brochure
- - Website & Blog
- Writer Editing Services
- - Script & Screenplay
- Our Editors
- Client Reviews
- Editing & Proofreading Prices
- Wordvice Points
- Partner Discount
- Plagiarism Checker
- APA Citation Generator
- MLA Citation Generator
- Chicago Citation Generator
- Vancouver Citation Generator
- - APA Style
- - MLA Style
- - Chicago Style
- - Vancouver Style
- Writing & Editing Guide
- Academic Resources
- Admissions Resources
How to Write the Results/Findings Section in Research
What is the research paper Results section and what does it do?
The Results section of a scientific research paper represents the core findings of a study derived from the methods applied to gather and analyze information. It presents these findings in a logical sequence without bias or interpretation from the author, setting up the reader for later interpretation and evaluation in the Discussion section. A major purpose of the Results section is to break down the data into sentences that show its significance to the research question(s).
The Results section appears third in the section sequence in most scientific papers. It follows the presentation of the Methods and Materials and is presented before the Discussion section —although the Results and Discussion are presented together in many journals. This section answers the basic question “What did you find in your research?”
What is included in the Results section?
The Results section should include the findings of your study and ONLY the findings of your study. The findings include:
- Data presented in tables, charts, graphs, and other figures (may be placed into the text or on separate pages at the end of the manuscript)
- A contextual analysis of this data explaining its meaning in sentence form
- All data that corresponds to the central research question(s)
- All secondary findings (secondary outcomes, subgroup analyses, etc.)
If the scope of the study is broad, or if you studied a variety of variables, or if the methodology used yields a wide range of different results, the author should present only those results that are most relevant to the research question stated in the Introduction section .
As a general rule, any information that does not present the direct findings or outcome of the study should be left out of this section. Unless the journal requests that authors combine the Results and Discussion sections, explanations and interpretations should be omitted from the Results.
How are the results organized?
The best way to organize your Results section is “logically.” One logical and clear method of organizing research results is to provide them alongside the research questions—within each research question, present the type of data that addresses that research question.
Let’s look at an example. Your research question is based on a survey among patients who were treated at a hospital and received postoperative care. Let’s say your first research question is:
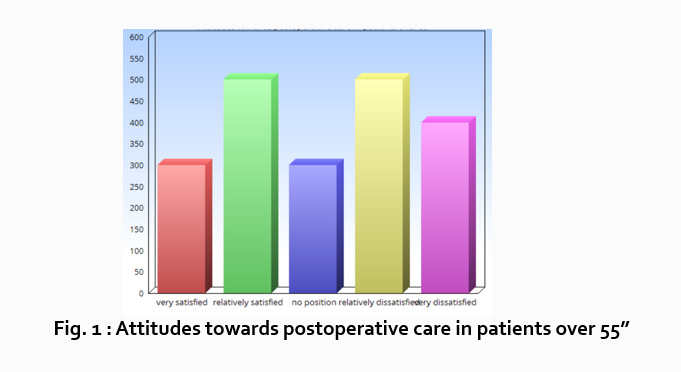
“What do hospital patients over age 55 think about postoperative care?”
This can actually be represented as a heading within your Results section, though it might be presented as a statement rather than a question:
Attitudes towards postoperative care in patients over the age of 55
Now present the results that address this specific research question first. In this case, perhaps a table illustrating data from a survey. Likert items can be included in this example. Tables can also present standard deviations, probabilities, correlation matrices, etc.
Following this, present a content analysis, in words, of one end of the spectrum of the survey or data table. In our example case, start with the POSITIVE survey responses regarding postoperative care, using descriptive phrases. For example:
“Sixty-five percent of patients over 55 responded positively to the question “ Are you satisfied with your hospital’s postoperative care ?” (Fig. 2)
Include other results such as subcategory analyses. The amount of textual description used will depend on how much interpretation of tables and figures is necessary and how many examples the reader needs in order to understand the significance of your research findings.
Next, present a content analysis of another part of the spectrum of the same research question, perhaps the NEGATIVE or NEUTRAL responses to the survey. For instance:
“As Figure 1 shows, 15 out of 60 patients in Group A responded negatively to Question 2.”
After you have assessed the data in one figure and explained it sufficiently, move on to your next research question. For example:
“How does patient satisfaction correspond to in-hospital improvements made to postoperative care?”
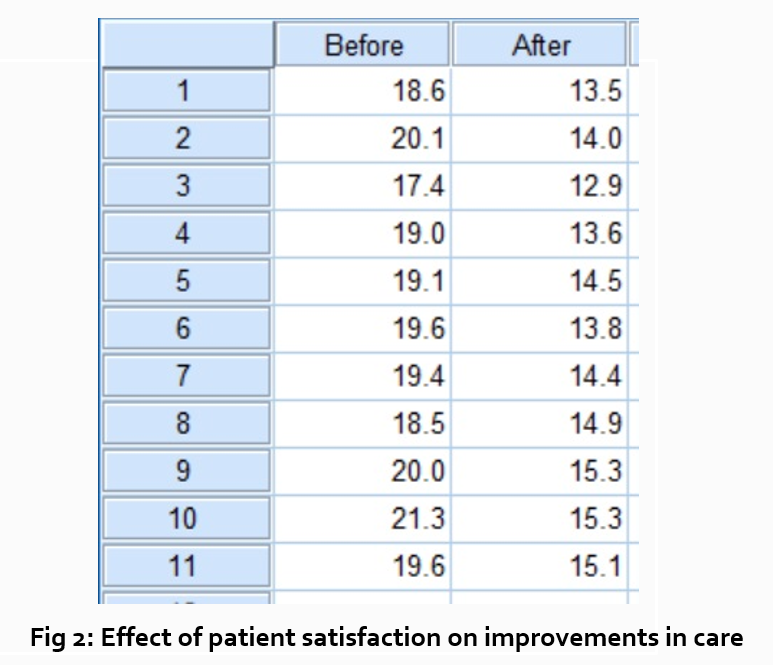
This kind of data may be presented through a figure or set of figures (for instance, a paired T-test table).
Explain the data you present, here in a table, with a concise content analysis:
“The p-value for the comparison between the before and after groups of patients was .03% (Fig. 2), indicating that the greater the dissatisfaction among patients, the more frequent the improvements that were made to postoperative care.”
Let’s examine another example of a Results section from a study on plant tolerance to heavy metal stress . In the Introduction section, the aims of the study are presented as “determining the physiological and morphological responses of Allium cepa L. towards increased cadmium toxicity” and “evaluating its potential to accumulate the metal and its associated environmental consequences.” The Results section presents data showing how these aims are achieved in tables alongside a content analysis, beginning with an overview of the findings:
“Cadmium caused inhibition of root and leave elongation, with increasing effects at higher exposure doses (Fig. 1a-c).”
The figure containing this data is cited in parentheses. Note that this author has combined three graphs into one single figure. Separating the data into separate graphs focusing on specific aspects makes it easier for the reader to assess the findings, and consolidating this information into one figure saves space and makes it easy to locate the most relevant results.
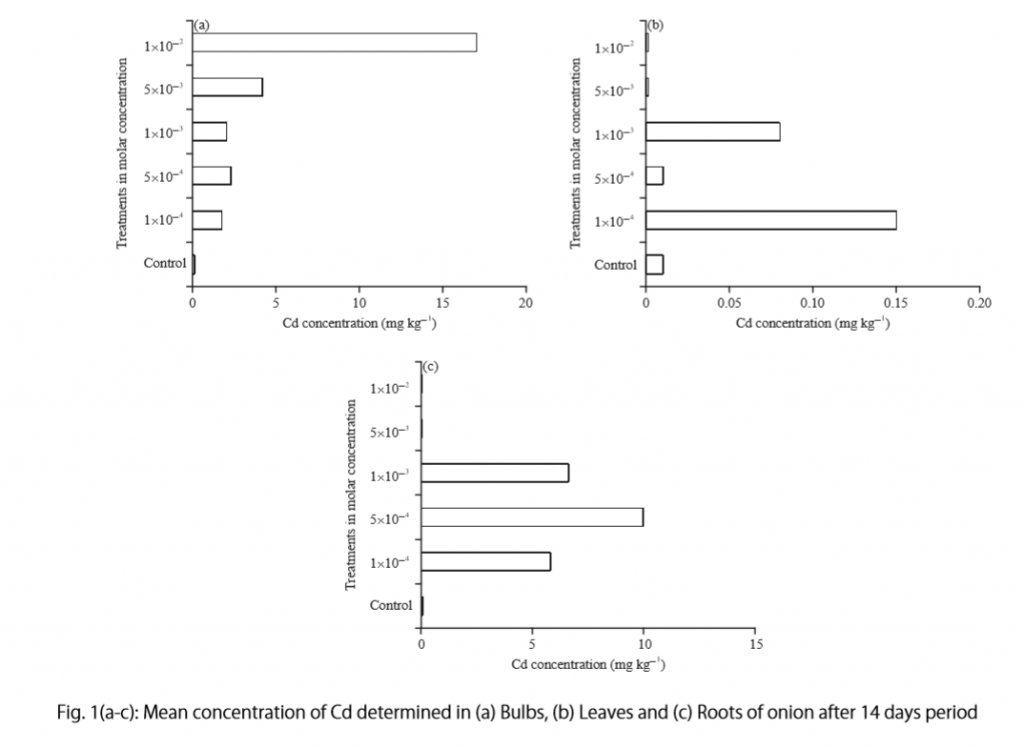
Following this overall summary, the relevant data in the tables is broken down into greater detail in text form in the Results section.
- “Results on the bio-accumulation of cadmium were found to be the highest (17.5 mg kgG1) in the bulb, when the concentration of cadmium in the solution was 1×10G2 M and lowest (0.11 mg kgG1) in the leaves when the concentration was 1×10G3 M.”
Captioning and Referencing Tables and Figures
Tables and figures are central components of your Results section and you need to carefully think about the most effective way to use graphs and tables to present your findings . Therefore, it is crucial to know how to write strong figure captions and to refer to them within the text of the Results section.
The most important advice one can give here as well as throughout the paper is to check the requirements and standards of the journal to which you are submitting your work. Every journal has its own design and layout standards, which you can find in the author instructions on the target journal’s website. Perusing a journal’s published articles will also give you an idea of the proper number, size, and complexity of your figures.
Regardless of which format you use, the figures should be placed in the order they are referenced in the Results section and be as clear and easy to understand as possible. If there are multiple variables being considered (within one or more research questions), it can be a good idea to split these up into separate figures. Subsequently, these can be referenced and analyzed under separate headings and paragraphs in the text.
To create a caption, consider the research question being asked and change it into a phrase. For instance, if one question is “Which color did participants choose?”, the caption might be “Color choice by participant group.” Or in our last research paper example, where the question was “What is the concentration of cadmium in different parts of the onion after 14 days?” the caption reads:
“Fig. 1(a-c): Mean concentration of Cd determined in (a) bulbs, (b) leaves, and (c) roots of onions after a 14-day period.”
Steps for Composing the Results Section
Because each study is unique, there is no one-size-fits-all approach when it comes to designing a strategy for structuring and writing the section of a research paper where findings are presented. The content and layout of this section will be determined by the specific area of research, the design of the study and its particular methodologies, and the guidelines of the target journal and its editors. However, the following steps can be used to compose the results of most scientific research studies and are essential for researchers who are new to preparing a manuscript for publication or who need a reminder of how to construct the Results section.
Step 1 : Consult the guidelines or instructions that the target journal or publisher provides authors and read research papers it has published, especially those with similar topics, methods, or results to your study.
- The guidelines will generally outline specific requirements for the results or findings section, and the published articles will provide sound examples of successful approaches.
- Note length limitations on restrictions on content. For instance, while many journals require the Results and Discussion sections to be separate, others do not—qualitative research papers often include results and interpretations in the same section (“Results and Discussion”).
- Reading the aims and scope in the journal’s “ guide for authors ” section and understanding the interests of its readers will be invaluable in preparing to write the Results section.
Step 2 : Consider your research results in relation to the journal’s requirements and catalogue your results.
- Focus on experimental results and other findings that are especially relevant to your research questions and objectives and include them even if they are unexpected or do not support your ideas and hypotheses.
- Catalogue your findings—use subheadings to streamline and clarify your report. This will help you avoid excessive and peripheral details as you write and also help your reader understand and remember your findings. Create appendices that might interest specialists but prove too long or distracting for other readers.
- Decide how you will structure of your results. You might match the order of the research questions and hypotheses to your results, or you could arrange them according to the order presented in the Methods section. A chronological order or even a hierarchy of importance or meaningful grouping of main themes or categories might prove effective. Consider your audience, evidence, and most importantly, the objectives of your research when choosing a structure for presenting your findings.
Step 3 : Design figures and tables to present and illustrate your data.
- Tables and figures should be numbered according to the order in which they are mentioned in the main text of the paper.
- Information in figures should be relatively self-explanatory (with the aid of captions), and their design should include all definitions and other information necessary for readers to understand the findings without reading all of the text.
- Use tables and figures as a focal point to tell a clear and informative story about your research and avoid repeating information. But remember that while figures clarify and enhance the text, they cannot replace it.
Step 4 : Draft your Results section using the findings and figures you have organized.
- The goal is to communicate this complex information as clearly and precisely as possible; precise and compact phrases and sentences are most effective.
- In the opening paragraph of this section, restate your research questions or aims to focus the reader’s attention to what the results are trying to show. It is also a good idea to summarize key findings at the end of this section to create a logical transition to the interpretation and discussion that follows.
- Try to write in the past tense and the active voice to relay the findings since the research has already been done and the agent is usually clear. This will ensure that your explanations are also clear and logical.
- Make sure that any specialized terminology or abbreviation you have used here has been defined and clarified in the Introduction section .
Step 5 : Review your draft; edit and revise until it reports results exactly as you would like to have them reported to your readers.
- Double-check the accuracy and consistency of all the data, as well as all of the visual elements included.
- Read your draft aloud to catch language errors (grammar, spelling, and mechanics), awkward phrases, and missing transitions.
- Ensure that your results are presented in the best order to focus on objectives and prepare readers for interpretations, valuations, and recommendations in the Discussion section . Look back over the paper’s Introduction and background while anticipating the Discussion and Conclusion sections to ensure that the presentation of your results is consistent and effective.
- Consider seeking additional guidance on your paper. Find additional readers to look over your Results section and see if it can be improved in any way. Peers, professors, or qualified experts can provide valuable insights.
One excellent option is to use a professional English proofreading and editing service such as Wordvice, including our paper editing service . With hundreds of qualified editors from dozens of scientific fields, Wordvice has helped thousands of authors revise their manuscripts and get accepted into their target journals. Read more about the proofreading and editing process before proceeding with getting academic editing services and manuscript editing services for your manuscript.
As the representation of your study’s data output, the Results section presents the core information in your research paper. By writing with clarity and conciseness and by highlighting and explaining the crucial findings of their study, authors increase the impact and effectiveness of their research manuscripts.
For more articles and videos on writing your research manuscript, visit Wordvice’s Resources page.
Wordvice Resources
- How to Write a Research Paper Introduction
- Which Verb Tenses to Use in a Research Paper
- How to Write an Abstract for a Research Paper
- How to Write a Research Paper Title
- Useful Phrases for Academic Writing
- Common Transition Terms in Academic Papers
- Active and Passive Voice in Research Papers
- 100+ Verbs That Will Make Your Research Writing Amazing
- Tips for Paraphrasing in Research Papers
How to Write an Effective Results Section
Affiliation.
- 1 Rothman Orthopaedics Institute, Philadelphia, PA.
- PMID: 31145152
- DOI: 10.1097/BSD.0000000000000845
Developing a well-written research paper is an important step in completing a scientific study. This paper is where the principle investigator and co-authors report the purpose, methods, findings, and conclusions of the study. A key element of writing a research paper is to clearly and objectively report the study's findings in the Results section. The Results section is where the authors inform the readers about the findings from the statistical analysis of the data collected to operationalize the study hypothesis, optimally adding novel information to the collective knowledge on the subject matter. By utilizing clear, concise, and well-organized writing techniques and visual aids in the reporting of the data, the author is able to construct a case for the research question at hand even without interpreting the data.
- Data Analysis
- Peer Review, Research*
- Publishing*
- Sample Size
How to Write the Results Section of a Research Paper
Table of Contents
Laura Moro-Martin, freelance scientific writer on Kolabtree, provides expert tips on how to write the results section of a research paper .
You have prepared a detailed −but concise− Methods section . Now it is time to write the Results of your research article. This part of the paper reports the findings of the experiments that you conducted to answer the research question(s). The Results can be considered the nucleus of a scientific article because they justify your claims, so you need to ensure that they are clear and understandable. You are telling a story −of course, a scientific story− and you want the readers to picture that same story in their minds. Let’s see how to avoid that your message ends up as in the ‘telephone game’.
The Results Section: Goals and Structure
Depending on the discipline, journal, and the nature of the study, the structure of the article can differ. We will focus on articles were the Results and Discussion appear in two separate sections, but it is possible in some cases to combine them.
In the Results section, you provide an overall description of the experiments and present the data that you obtained in a logical order, using tables and graphs as necessary. The Results section should simply state your findings without bias or interpretation. For example, in your analysis, you may have noticed a significant correlation between two variables never described before. It is correct to explain this in the Results section. However, speculation about the reasons for this correlation should go in the Discussion section of your paper.
In general, the Results section includes the following elements:
- A very short introductory context that repeats the research question and helps to understand your results.
- Report on data collection, recruitment, and/or participants. For example, in the case of clinical research, it is common to include a first table summarizing the demographic, clinical, and other relevant characteristics of the study participants.
- A systematic description of the main findings in a logical order (generally following the order of the Methods section), highlighting the most relevant results.
- Other important secondary findings, such as secondary outcomes or subgroup analyses (remember that you do not need to mention any single result).
- Visual elements, such as, figures, charts, maps, tables, etc. that summarize and illustrate the findings. These elements should be cited in the text and numbered in order. Figures and tables should be able to stand on its own without the text, which means that the legend should include enough information to understand the non-textual element.
How to Write the Results Section of a Research Paper: Tips
The first tip −applicable to other sections of the paper too− is to check and apply the requirements of the journal to which you are submitting your work.
In the Results section, you need to write concisely and objectively, leaving interpretation for the Discussion section. As always, ‘learning from others’ can help you. Select a few papers from your field, including some published in your target journal, which you consider ‘good quality’ and well written. Read them carefully and observe how the Results section is structured, the type and amount of information provided, and how the findings are exposed in a logical order. Keep an eye on visual elements, such as figures, tables, and supplementary materials. Understand what works well in those papers to effectively convey their findings, and apply it to your writing.
Your Results section needs to describe the sequence of what you did and found, the frequency of occurrence of a particular event or result, the quantities of your observations, and the causality (i.e. the relationships or connections) between the events that you observed.
To organize the results, you can try to provide them alongside the research questions. In practice, this means that you will organize this section based on the sequence of tables and figures summarizing the results of your statistical analysis. In this way, it will be easier for readers to look at and understand your findings. You need to report your statistical findings, without describing every step of your statistical analysis. Tables and figures generally report summary-level data (for example, means and standard deviations), rather than all the raw data.
Following, you can prepare the summary text to support those visual elements. You need not only to present but also to explain your findings, showing how they help to address the research question(s) and how they align with the objectives that you presented in the Introduction . Keep in mind that results do not speak for themselves, so if you do not describe them in words, the reader may perceive the findings differently from you. Build coherence along this section using goal statements and explicit reasoning (guide the reader through your reasoning, including sentences of this type: ‘In order to…, we performed….’; ‘In view of this result, we ….’, etc.).
In summary, the general steps for writing the Results section of a research article are:
- Check the guidelines of your target journal and read articles that it has published in similar topics to your study.
- Catalogue your findings in relation to the journal requirements, and design figures and tables to organize your data.
- Write the Results section following the order of figures and tables.
- Edit and revise your draft and seek additional input from colleagues or experts.
The Style of the Results Section
‘If you are out to describe the truth, leave elegance to the tailor’, Austrian physicist Ludwig Boltzmann said. Although the scope of the Results section −and of scientific papers in general− is eminently functional, this does not mean that you cannot write well. Try to improve the rhythm to move the reader along, use transitions and connectors between different sections and paragraphs, and dedicate time to revise your writing.
The Results section should be written in the past tense. Although writing in the passive voice may be tempting, the use of the active voice makes the action much more visualizable. The passive voice weakens the power of language and increases the number of words needed to say the same thing, so we recommend using the active voice as much as possible. Another tip to make your language visualizable and reduce sentence length is the use of verbal phrases instead of long nouns. For example, instead of writing ‘As shown in Table 1, there was a significant increase in gene expression’, you can say ‘As shown in Table 1, gene expression increased significantly’.
Get a Second (And Even Third) Opinion
Writing a scientific article is not an individual work. Take advantage of your co-authors by making them check the Results section and adding their comments and suggestions. Not only that, but an external opinion will help you to identify misinterpretations or errors. Ask a colleague that is not directly involved in the work to review your Results and then try to evaluate what your colleague did or did not understand. If needed, seek additional help from a qualified expert.
Common Errors to Avoid While Writing the Results Section
Several mistakes frequently occur when you write the Results section of a research paper. Here we have collected a few examples:
- Including raw results and/or endlessly repetitive data. You do not need to present every single number and calculation, but a summary of the results. If relevant, raw data can be included in supplementary materials.
- Including redundant information. If data are contained in the tables or figures, you do not need to repeat all of them in the Results section. You will have the opportunity to highlight the most relevant results in the Discussion .
- Repeating background information or methods , or introducing several sentences of introductory information (if you feel that more background information is necessary to present a result, consider inserting that information in the Introduction ).
- Results and Methods do not match . You need to explain the methodology used to obtain all the experimental observations.
- Ignoring negative results or results that do not support the conclusions. In addition to posing potential ethical concerns on your work, reviewers will not like it. You need to mention all relevant findings, even if they failed to support your predictions or hypotheses. Negative results are useful and will guide future studies on the topic. Provide your interpretation for negative results in the Discussion .
- Discussing or interpreting the results . Leave that for the Discussion , unless your target journal allows preparing one section combining Results and Discussion .
- Errors in figures/tables are varied and common . Examples of errors include using an excessive number of figures/tables (it is a good idea to select the most relevant ones and move the rest to supplementary materials), very complex figures/tables (hard-to-read figures with many subfigures or enormous tables may confuse your readers; think how these elements will be visualized in the final format of the article), difficult to interpret figures/tables (cryptic abbreviations; inadequate use of colors, axis, scales, symbols, etc.), and figures/tables that are not self-standing (figures/tables require a caption, all abbreviations used need to be explained in the legend or a footnote, and statistical tests applied are frequently reported). Do not include tables and figures that are not mentioned in the body text of your Results .
In summary, the Results section is the nucleus of your paper that justifies your claims. Take time to adequately organize it and prepare understandable figures and tables to convey your message to the reader. Good writing!
- The Structure, Format, Content, and Style of a Journal-Style Scientific Paper. https://abacus.bates.edu/~ganderso/biology/resources/writing/HTWsections.html – methods (accessed on 30th September 2020)
- Organizing Academic Research Papers: 7. The Results. https://library.sacredheart.edu/c.php?g=29803&p=185931 (accessed on 30th September 2020)
- Kendra Cherry. How to Write an APA Results Section. https://www.verywellmind.com/how-to-write-a-results-section-2795727 (accessed on 30th September 2020)
- Chapin Rodríguez. Empowering your scientific language by making it “visualizable”. http://creaducate.eu/wp-content/uploads/2019/11/tipsheet36_visualizable-lang-tip-sheet.pdf (accessed on 1st October 2020)
- IMRaD Results Discussion. https://writingcenter.gmu.edu/guides/imrad-results-discussion (accessed on 1st October 2020)
- Writing the Results Section for a Research Paper. https://wordvice.com/writing-the-results-section-for-a-research-paper/ (accessed on 1st October 2020)
- Scott L. Montgomery. The Chicago Guide to Communicating Science , Chapter 9. Second edition, The University of Chicago Press, 2017.
- Hilary Glasman-Deal . Science Research Writing for Non-Native Speakers of English, Unit 2 . Imperial College Press, 2010.
Unlock Corporate Benefits • Secure Payment Assistance • Onboarding Support • Dedicated Account Manager
Sign up with your professional email to avail special advances offered against purchase orders, seamless multi-channel payments, and extended support for agreements.
About Author
Ramya Sriram manages digital content and communications at Kolabtree (kolabtree.com), the world's largest freelancing platform for scientists. She has over a decade of experience in publishing, advertising and digital content creation.
Related Posts
Spotlight: kolabtree’s regulatory medical writer dr. nare simonyan, how healthcare writers can help your business , the benefits of outsourcing in continuing medical education (cme), leave a reply cancel reply.
Save my name, email, and website in this browser for the next time I comment.
Automated page speed optimizations for fast site performance
- How It Works
- PhD thesis writing
- Master thesis writing
- Bachelor thesis writing
- Dissertation writing service
- Dissertation abstract writing
- Thesis proposal writing
- Thesis editing service
- Thesis proofreading service
- Thesis formatting service
- Coursework writing service
- Research paper writing service
- Architecture thesis writing
- Computer science thesis writing
- Engineering thesis writing
- History thesis writing
- MBA thesis writing
- Nursing dissertation writing
- Psychology dissertation writing
- Sociology thesis writing
- Statistics dissertation writing
- Buy dissertation online
- Write my dissertation
- Cheap thesis
- Cheap dissertation
- Custom dissertation
- Dissertation help
- Pay for thesis
- Pay for dissertation
- Senior thesis
- Write my thesis
Results Section Of A Research Paper: How To Write It Properly
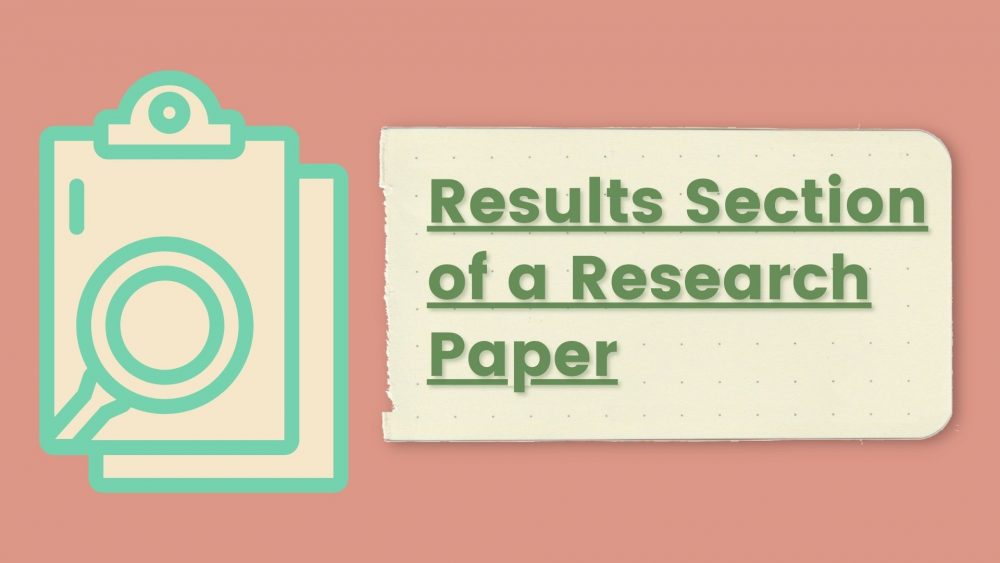
The results section of a research paper refers to the part that represents the study’s core findings from the methods that the researcher used to collect and analyze data. This section presents the results logically without interpretation or bias from the author.
Thus, this part of a research paper sets up the read for evaluation and analysis of the findings in the discussion section. Essentially, this section breaks down the information into several sentences, showing its importance to the research question. Writing results section in a research paper entails summarizing the gathered data and the performed statistical analysis. That way, the author presents or reports the results without subjective interpretation.
What Is The Results Section Of A Research Paper?
In its simplest definition, a research paper results section is where the researcher reports the findings of a study based on the applied methodology for gathering information. It’s the part where the author states the research findings in a logical sequence without interpreting them. If the research paper has data from actual research, this section should feature a detailed description of the results.
When writing a dissertation, a thesis, or any other academic paper, the result section should come third in sections’ sequence. It should follow the Methods and Materials presentation and the Discussion section comes after it. But most scientific papers present the Results and Discussion sections together. However, the results section answers the question, “What did your research uncover?”
Ideally, this section allows you to report findings in research paper, creating the basis for sufficiently justified conclusions. After writing the study findings in the results section, you interpret them in the subsequent discussion part. Therefore, your results section should report information that will justify your claims. That way, you can look back on the results section when writing the discussion part to ensure that your report supports your conclusions.
What Goes in the Results Section of a Research Paper?
This section should present results in research paper. The findings part of a research paper can differ in structure depending on the study, discipline, and journal. Nevertheless, the results section presents a description of the experiment while presenting the research results. When writing this part of your research paper, you can use graphs and tables if necessary.
However, state the findings without interpreting them. For instance, you can find a correlation between variables when analyzing data. In that case, your results section can explain this correlation without speculating about the causes of this correlation.
Here’s what to include in the results section of research paper:
A brief introductory of the context, repeating the research questions to help the readers understand the results A report about information collection, participants, and recruitment: for instance, you can include a demographic summary with the participants’ characteristics A systematic findings’ description, with a logical presentation highlighting relevant and crucial results A contextual data analysis explaining the meaning in sentences Information corresponding to the primary research questions Secondary findings like subgroup analysis and secondary outcomes Visual elements like charts, figures, tables, and maps, illustrating and summarizing the findings
Ensure that your results section cites and numbers visual elements in an orderly manner. Every table or figure should stand alone without text. That means visual elements should have adequate non-textual content to enable the audiences to understand their meanings.
If your study has a broad scope, several variables, or used methodologies that yielded different results, state the most relevant results only based on the research question you presented in your Introduction section.
The general rule is to leave out any data that doesn’t present your study’s direct outcome or findings. Unless the professor, advisor, university faulty, or your target journal requests you to combine the Results and Discussion sections, omit the interpretations and explanations of the results in this section.
How Long Should A Results Section Be?
The findings section of a research paper ranges between two and three pages, with tables, text, and figures. In most cases, universities and journals insist that this section shouldn’t exceed 1,000 words over four to nine paragraphs, usually with no references.
But a good findings section occupies 5% of the entire paper. For instance, this section should have 500 words if a dissertation has 10,000 words. If the educator didn’t specify the number of words to include in this chapter, use the data you collect to determine its length. Nevertheless, be as concise as possible by featuring only relevant results that answer your research question.
How To Write Results Section Of Research Paper
Perhaps, you have completed researching and writing the preceding sections, and you’re now wondering how to write results. By the time you’re composing this section, you already have findings or answers to your research questions. However, you don’t even know how to start a results section. And your search for guidelines landed you on this page.
Well, every research project is different and unique. That’s why researchers use different strategies when writing this section of their research papers. The scientific or academic discipline, specialization field, target journal, and the author are factors influencing how you write this section. Nevertheless, there’s a general way of writing this section, although it might differ slightly between disciplines. Here’s how to write results section in a research paper.
Check the instructions or guidelines. Check their instructions or guidelines first, whether you’re writing the research paper as part of your coursework or for an academic journal. These guidelines outline the requirements for presenting results in research papers. Also, check the published articles to know how to approach this section. When reviewing the procedures, check content restrictions and length. Essentially, learn everything you can about this section from the instructions or guidelines before you start writing. Reflect on your research findings. With instructions and guidelines in mind, reflect on your research findings to determine how to present them in your research paper. Decide on the best way to show the results so that they can answer the research question. Also, strive to clarify and streamline your report, especially with a complex and lengthy results section. You can use subheadings to avoid peripheral and excessive details. Additionally, consider breaking down the content to make it easy for the readers to understand or remember. Your hypothesis, research question, or methodologies might influence the structure of the findings sections. Nevertheless, a hierarchy of importance, chronological order, or meaningful grouping of categories or themes can be an effective way of presenting your findings. Design your visual presentations. Visual presentations improve the textual report of the research findings. Therefore, decide on the figures and styles to use in your tables, graphs, photos, and maps. However, check the instructions and guidelines of your faculty or journal to determine the visual aids you can use. Also, check what the guidelines say about their formats and design elements. Ideally, number the figures and tables according to their mention in the text. Additionally, your figures and tables should be self-explanatory. Write your findings section. Writing the results section of a research paper entails communicating the information you gathered from your study. Ideally, be as objective and factual as possible. If you gathered complex information, try to simplify and present it accurately, precisely, and clearly. Therefore, use well-structured sentences instead of complex expressions and phrases. Also, use an active voice and past tense since you’ve already done the research. Additionally, use correct spelling, grammar, and punctuation. Take your time to present the findings in the best way possible to focus your readers on your study objectives while preparing them for the coming speculations, interpretations, and recommendations. Edit Your Findings Section. Once you’ve written the results part of your paper, please go through it to ensure that you’ve presented your study findings in the best way possible. Make sure that the content of this section is factual, accurate, and without errors. You’ve taken a considerable amount of time to compose the results scientific paper audiences will find interesting to read. Therefore, take a moment to go through the draft and eliminate all errors.
Practical Tips on How to Write a Results Section of a Research Paper
The results part of a research paper aims to present the key findings objectively in a logical and orderly sequence using text and illustrative materials. A common mistake that many authors make is confusing the information in the discussion and the results sections. To avoid this, focus on presenting your research findings without interpreting them or speculating about them.
The following tips on how to write a results section should make this task easier for you:
Summarize your study results: Instead of reporting the findings in full detail, summarize them. That way, you can develop an overview of the results. Present relevant findings only: Don’t report everything you found during your research. Instead, present pertinent information only. That means taking time to analyze your results to know what your audiences want to know. Report statistical findings: When writing this section, assume that the audiences understand statistical concepts. Therefore, don’t try to explain the nitty-gritty in this section. Remember that your work is to report your study’s findings in this section. Be objective and concise: You can interpret the findings in the discussion sections. Therefore, focus on presenting the results objectively and concisely in this section. Use the suitable format: Use the correct style to present the findings depending on your study field.
Get Professional Help with the Research Section
Maybe you’re pursuing your graduate or undergraduate studies but cannot write the results part of your paper. Perhaps, you’re done researching and analyzing information, but this section proves too tricky for you to write. Well, you’re not alone because many students across the world struggle to present their research findings.
Luckily, our highly educated, talented, and experienced writers are always ready to assist such learners. If you are stuck with the results part of your paper, our professionals can help you . We offer high-quality, custom writing help online. We’re a reliable team of experts with a sterling reputation for providing comprehensive assistance to college, high school, and university learners. We deliver highly informative academic papers after conducting extensive and in-depth research. Contact us saying something like, “please do my thesis” to get quality help with your paper!
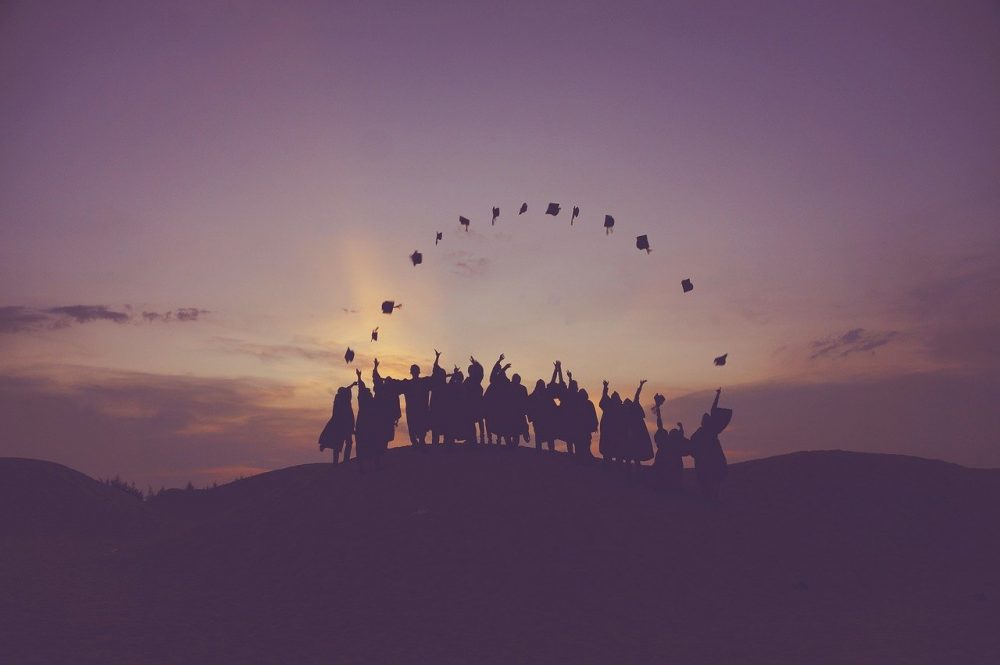
Leave a Reply Cancel reply
Your email address will not be published. Required fields are marked *
Comment * Error message
Name * Error message
Email * Error message
Save my name, email, and website in this browser for the next time I comment.
As Putin continues killing civilians, bombing kindergartens, and threatening WWIII, Ukraine fights for the world's peaceful future.
Ukraine Live Updates
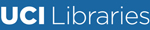
- Langson Library
- Science Library
- Grunigen Medical Library
- Law Library
- Connect From Off-Campus
- Accessibility
- Gateway Study Center
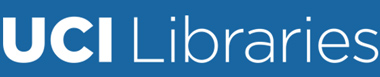
Email this link
Writing a scientific paper.
- Writing a lab report
- INTRODUCTION
Writing a "good" results section
Figures and Captions in Lab Reports
"Results Checklist" from: How to Write a Good Scientific Paper. Chris A. Mack. SPIE. 2018.
Additional tips for results sections.
- LITERATURE CITED
- Bibliography of guides to scientific writing and presenting
- Peer Review
- Presentations
- Lab Report Writing Guides on the Web
This is the core of the paper. Don't start the results sections with methods you left out of the Materials and Methods section. You need to give an overall description of the experiments and present the data you found.
- Factual statements supported by evidence. Short and sweet without excess words
- Present representative data rather than endlessly repetitive data
- Discuss variables only if they had an effect (positive or negative)
- Use meaningful statistics
- Avoid redundancy. If it is in the tables or captions you may not need to repeat it
A short article by Dr. Brett Couch and Dr. Deena Wassenberg, Biology Program, University of Minnesota
- Present the results of the paper, in logical order, using tables and graphs as necessary.
- Explain the results and show how they help to answer the research questions posed in the Introduction. Evidence does not explain itself; the results must be presented and then explained.
- Avoid: presenting results that are never discussed; presenting results in chronological order rather than logical order; ignoring results that do not support the conclusions;
- Number tables and figures separately beginning with 1 (i.e. Table 1, Table 2, Figure 1, etc.).
- Do not attempt to evaluate the results in this section. Report only what you found; hold all discussion of the significance of the results for the Discussion section.
- It is not necessary to describe every step of your statistical analyses. Scientists understand all about null hypotheses, rejection rules, and so forth and do not need to be reminded of them. Just say something like, "Honeybees did not use the flowers in proportion to their availability (X2 = 7.9, p<0.05, d.f.= 4, chi-square test)." Likewise, cite tables and figures without describing in detail how the data were manipulated. Explanations of this sort should appear in a legend or caption written on the same page as the figure or table.
- You must refer in the text to each figure or table you include in your paper.
- Tables generally should report summary-level data, such as means ± standard deviations, rather than all your raw data. A long list of all your individual observations will mean much less than a few concise, easy-to-read tables or figures that bring out the main findings of your study.
- Only use a figure (graph) when the data lend themselves to a good visual representation. Avoid using figures that show too many variables or trends at once, because they can be hard to understand.
From: https://writingcenter.gmu.edu/guides/imrad-results-discussion
- << Previous: METHODS
- Next: DISCUSSION >>
- Last Updated: Aug 4, 2023 9:33 AM
- URL: https://guides.lib.uci.edu/scientificwriting
Off-campus? Please use the Software VPN and choose the group UCIFull to access licensed content. For more information, please Click here
Software VPN is not available for guests, so they may not have access to some content when connecting from off-campus.
Training videos | Faqs

Results Section Examples and Writing Tips
Abstract | Introduction | Literature Review | Research question | Materials & Methods | Results | Discussion | Conclusion
In this blog, we look at how to write the results section of a research paper. We will go through plenty of results examples and understand how to construct a great results section for your research paper.
1. What is the purpose of the results section?

The authors report their findings in the results section. This is a relatively easy section to write. You simply have to organize your results and write them up in a logical sequence. You must present your findings without evaluating or interpreting them. You must try to illustrate your data using figures and tables to make it more accessible to the readers.
2. How should I structure my results section?
The results section of a research paper typically contains the following components:
Data pre-processing You can talk about any difficulties you encountered while collecting or processing the data.
Main findings You should present your main findings (both positive and negative) in a logical sequence.
Statistics You must use descriptive statistics and inferential statistics to present your data (mean, SD, CI etc.)
Figures and tables Use figures and tables to summarize large amounts of data in a visually pleasing way.
Trends and patterns Trends and patterns describe the general change in some variable in relation to another variable.
Reanalysis You can talk about any reanalysis of data you had to perform in order to reconfirm your findings.
3. Examples of results section
Let’s look at some examples of the results section. We will be looking at results section examples from different fields and of different formats. We have split this section into multiple components so that it is easy for you to understand.
3.1. An example of a pre-processing passage in the results section
Here is an example of the results section from an engineering research paper where the authors talk about pre-processing. The authors are saying that they performed a series of steps before conducting the actual analysis. They are saying that they filled in the missing data using linear interpolation. And then, they transformed and normalized the data. Finally, they applied data smoothing to remove any noise in the data. As you can see the authors are very transparent and are detailing everything they did to the data before the actual analysis.
The following is a brief summary of the preprocessing steps applied prior to analysis. The data were screened for outliers and such data points were set to missing. Subsequently, the missing data were filled by linear interpolation. The data were transformed using the Box method and then normalized to zero mean and unit standard deviation. The smoothing was applied at the final step to remove the noise in the data. _ Missing data _ Missing data fix _ Data normalization _ Data smoothing
3.2. An example of main findings passage in the results section
While presenting your findings you must clearly explain the following:
This is an example of the results section from a psychology research paper where authors are outlining their main findings. In this paper, the authors are investigating the effects of different types of music on people. The authors say that they found significant differences between classical and pop music in terms of memory recall. And then, they are saying that they did not find any differences in terms of emotional response. Finally, they are saying that they were quite surprised to find that both types of music fatigued the listeners at the same rate.
The results indicate significant differences between classical music and pop music in terms of their effects on memory recall and cognition (p<0.05). However, there was no significant difference across the groups for the emotional response to the music (p>0.05). It was surprising to find that both types of music elicited similar levels of fatigue in both groups (p>0.05). _ What was found? _ What was not found? _ Unexpected results
It is very clear from their tone that the last result was a bit unexpected. You can see that the authors have presented their findings in an unbiased manner without any interpretation. They have listed, the positive, the negative, and the neutral results logically in the text. This is how it should be done.
3.3. An example of using statistics in the results section
The use of relevant statistics is very important while writing your results section. It offers two benefits, number one, it will help you summarize the data in a meaningful way, and number two, it will make your text sound more credible. This results example is from a social sciences research paper investigating the relationship between social media and mental health. I want you to pay specific attention to the descriptive and inferential statistics used throughout the text.
The results of this study indicate significant differences in anxiety levels between high social media usage and moderate social media usage (p<0.05). The average time spent by high social media users (5.2 ± 2.2) was considerably higher than that of moderate social media users (3.2 ± 2.2). The odds of sleep disturbance were significantly greater for high social media users (odds ratio, 2.12; 95% CI, 1.81-2.17) compared to moderate social media users (odds ratio, 1.14; 95% CI, 1.07-1.18). _ p-values _ Standard deviation _ Odds ratio & confidence interval
The authors say that there is a significant difference in anxiety levels between high social media users and moderate social media users. Since the authors have used the word significant, they have specified the p-value of the statistical test they used to ascertain this. Then they are providing the actual values of the amount of time spent by both groups on social media. They have presented the data in the form, mean plus or minus standard deviation, which is the standard scientific way to represent this type of data. In the final statement, they talk about the odds of both groups experiencing sleep disturbances. They have provided the odds ratio along with the confidence intervals.
3.4. An example of using figures and tables in the results section
One of the important components of the results section is figures and tables. Try to present your data in figures and tables wherever necessary.

Here is a results example where authors are using figures and tables to describe their results. In the first couple of lines, they are talking about a trend in their data that relates to the change in temperature over time. They are constantly referring to the figure to get their point across to the readers. And finally, in the last sentence, they are telling the readers that the actual numerical data is provided in the table, and they can refer to it if they want. This is a standard way to use figures and tables in your research paper.
In Figure 1.1, the values are plotted as a function of time. The two peaks in the plot correspond to the maximum and minimum temperature values. The specific values obtained for each experiment are given in Table 2. _ Figure _ Figure info _ Table
3.5. An example of elaborating trends/patterns in the results section
As a researcher, it is your job to identify trends, patterns, and relationships between different variables in your data, and tell your readers about them. Because they can reveal very important evidence that you can use to answer your research questions or prove your hypothesis.
The temperature value increases until it reaches a peak value, then decreases rapidly to zero. This effect was 10 times larger at room temperature. There appears to be a positive association between temperature and time. _ Trend _ Pattern _ Interpreting the evidence
In this example, the author talks about his observation that the temperature changes over time in a certain pattern. Then the author talks about noticing the same behaviour under different conditions. Then based on the evidence, the author concludes that there is a positive association between time and temperature. The passage flows very well. You can clearly understand what the author is trying to say here.
4. Frequently Asked Questions
Most journals require separate results and discussion sections. So, it is very important that you are just reporting and describing your results without interpreting them in your results section. The interpretation of the results must be done in the discussion section.
Do not suppress negative results in your paper. Don’t worry if your experiments did not yield the results that you were expecting. Don’t try to ignore or downplay the result just because it doesn’t support your hypothesis. It doesn’t mean that your research is a failure. Negative results are as good as positive results. Actually, you have discovered a useful piece of evidence that your experiments don’t work
Here is an example of an author reporting negative and moderate results in the paper. This is perfectly fine. The authors have reported their results in the paper with full transparency and honesty. And that is how it should be.
The performance did not improve significantly with the new approach, though some marginal improvement was still achieved in terms of speed. These findings are in contrary to our original hypothesis. _ Moderate results _ Negative results
Most people skim through the paper just going through figures and tables without reading any text in the paper. So, captions should be as short as possible but detailed enough for the readers to understand the figure or table without having to read the text.
The best way to answer this question is if you want to illustrate the trends and patterns in the data, then a figure is the best option. If you want to show the actual values or present a lot of numerical information in your paper. Then, a table might be the best way to go.
The best way to answer this question is if you cannot present your data in your text in one or two lines, then you should consider putting it in a figure or a table.
You should write your results section in the past tense, because you are reporting the results of an experiment that you conducted in the past.
Similar Posts

Conclusion Section Examples and Writing Tips
In this blog, we will go through many conclusion examples and learn how to present a powerful final take-home message to your readers.

How to Handle Negative Results in your Research Paper?
In this blog, we will see how to effectively communicate negative and unexpected findings in your paper with detailed examples.
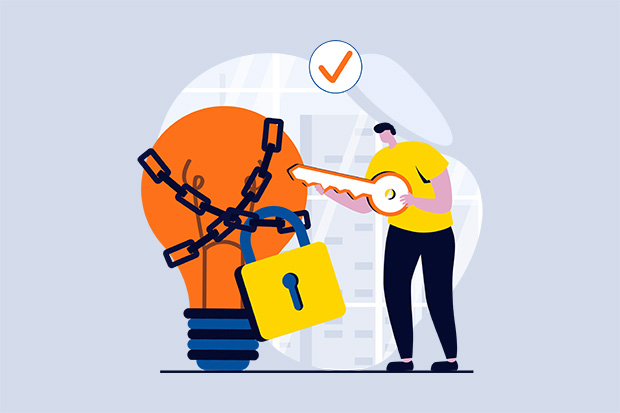
Introduction Paragraph Examples and Writing Tips
In this blog, we will go through a few introduction paragraph examples and understand how to construct a great introduction paragraph for your research paper.
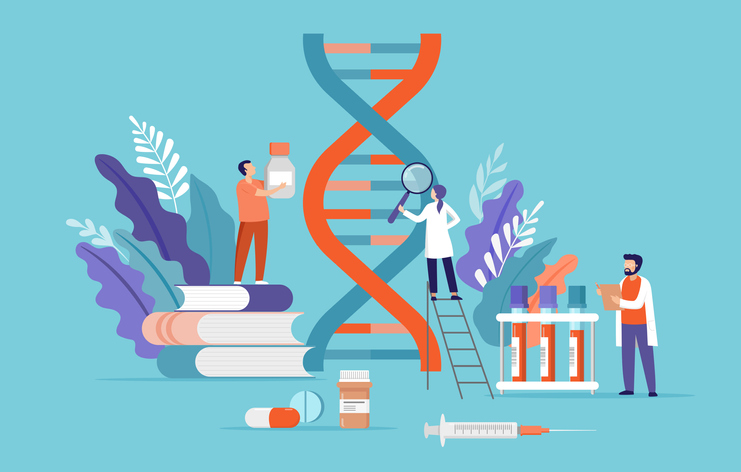
Writing a Medical Clinical Trial Research Paper – Example & Format
In this blog, we will teach you step-by-step how to write a clinical trial research paper for publication in a high quality scientific journal.

How to Make Your Study Limitations Sound Positive?
In this blog, we will look at some clever techniques to present the study limitations without reducing the impact of your work.
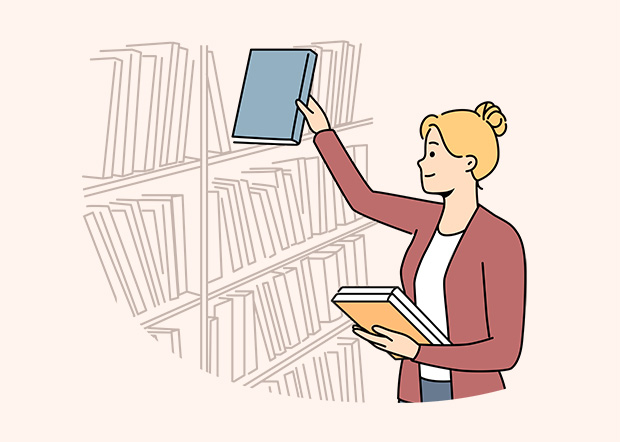
Literature Review Examples and Writing Tips
In this blog, we will go through many literature review examples and understand different ways to present past literature in your paper.
Leave a Reply Cancel reply
Your email address will not be published. Required fields are marked *
Save my name, email, and website in this browser for the next time I comment.
- 2 Share Facebook
- 1 Share Twitter
- 0 Share LinkedIn
- 2 Share Email
Research Paper Guide
Research Paper Results Section

How To Write The Results Section of A Research Paper | Steps & Examples
12 min read
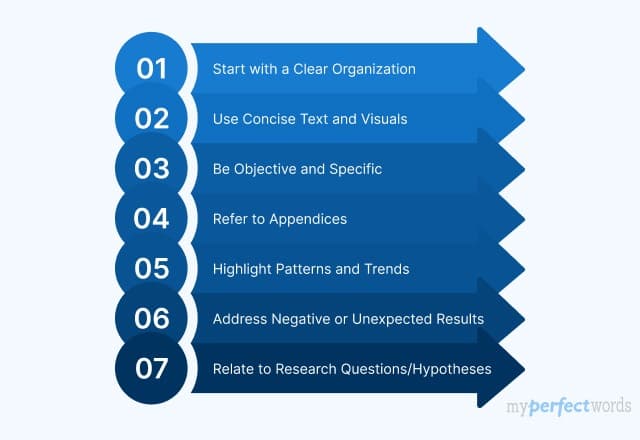
People also read
Research Paper Writing - A Step by Step Guide
Research Paper Examples - Free Sample Papers for Different Formats!
Guide to Creating Effective Research Paper Outline
Interesting Research Paper Topics for 2024
Research Proposal Writing - A Step-by-Step Guide
How to Start a Research Paper - 7 Easy Steps
How to Write an Abstract for a Research Paper - A Step by Step Guide
Writing a Literature Review For a Research Paper - A Comprehensive Guide
Qualitative Research - Methods, Types, and Examples
8 Types of Qualitative Research - Overview & Examples
Qualitative vs Quantitative Research - Learning the Basics
200+ Engaging Psychology Research Paper Topics for Students in 2024
Learn How to Write a Hypothesis in a Research Paper: Examples and Tips!
20+ Types of Research With Examples - A Detailed Guide
Understanding Quantitative Research - Types & Data Collection Techniques
230+ Sociology Research Topics & Ideas for Students
How to Cite a Research Paper - A Complete Guide
Excellent History Research Paper Topics- 300+ Ideas
A Guide on Writing the Method Section of a Research Paper - Examples & Tips
How To Write an Introduction Paragraph For a Research Paper: Learn with Examples
Crafting a Winning Research Paper Title: A Complete Guide
Writing a Research Paper Conclusion - Step-by-Step Guide
Writing a Thesis For a Research Paper - A Comprehensive Guide
How To Write A Discussion For A Research Paper | Examples & Tips
Writing a Problem Statement for a Research Paper - A Comprehensive Guide
Finding Sources For a Research Paper: A Complete Guide
A Guide on How to Edit a Research Paper
200+ Ethical Research Paper Topics to Begin With (2024)
300+ Controversial Research Paper Topics & Ideas - 2024 Edition
150+ Argumentative Research Paper Topics For You - 2024
How to Write a Research Methodology for a Research Paper
Have you ever felt stuck and confused when trying to share the results of your research in a paper? You're not alone!
Many students and researchers struggle with making their findings easy to understand while still sounding smart. It can get pretty frustrating when you're not sure how to talk about all the data and numbers in your research.
Without clear guidelines, it's easy to feel lost and unsure about how to make your results section both detailed and easy to read.
Don't worry! This guide is here to help you figure it all out.
We'll break down the process of writing a results section into simple steps. From explaining tricky stats to showing your data clearly, we've got your back.
Let's get started!

- 1. What Exactly is the Result Section of a Research Paper?
- 2. Importance of the Result Section of a Research Paper
- 3. How to Write the Results Section of a Research Paper?
- 4. Differences Between Qualitative and Quantitative Data
- 5. How To Write The Results Section of A Research Paper - Examples
- 6. Result vs Discussion Section vs Conclusion - What’s the Difference?
- 7. Mistakes to Avoid While Writing the Results Section
What Exactly is the Result Section of a Research Paper?
The results section of a research paper is where you get to showcase the findings of your study.
Think of it as the big reveal, where you share all the important information and data you've gathered. This section is crucial because it's where your reader learns about the outcomes of your research and whether your hypotheses were supported or not.
Importance of the Result Section of a Research Paper
The results section in a research paper is really important. It's like a hub where you put all the main findings and results from your study.
This part is crucial because it shows exactly what data you gathered and how you analyzed it.
In the academic world, the research results act as a kind of checkpoint. It lets other researchers and scholars review and confirm the reliability of your study methods. This helps ensure that your research is trustworthy and adds valuable information to the wider pool of knowledge in a specific field.
Also, the results section helps either prove or disprove the hypothesis you set up in your study. It's the part where the numbers and trends either support what you expected or reveal surprising insights.
What's Included in the Result Section of a Research Paper
When you're talking about the results of a research paper, it's important to know the key parts that make it clear and complete.
Let’s see what the results section of a research paper includes:
Key Points To Consider In The Result Section:
- Clarity: Present the results in a straightforward manner, making it easy for readers to comprehend.
- Objectivity: Avoid interpretation or discussion at this stage; stick to presenting the observed data.
- Consistency: Organize the results logically, following the same order as your research questions or hypotheses.
How to Write the Results Section of a Research Paper?
The results section of a research paper is crucial for conveying the outcomes of your study in a clear and organized manner.
Follow these steps to learn how to write the findings section of a research paper:
Step 1: Start With a Clear Organization
Begin the results section with a brief introductory paragraph that outlines the purpose of the results. Mention the research questions or hypotheses that the study aimed to address.
- Order of Presentation
Present your results in a systematic and coherent order. Make sure the logical sequence with the research questions or hypotheses established in the earlier sections of your paper.
This helps readers follow a logical flow and easily locate information related to specific aspects of your study.
- Use of Subheadings
Divide the Results section into meaningful subsections using clear and descriptive subheadings.
Subheadings provide a roadmap for readers, guiding them through different parts of your results.
For example, if your research involves multiple variables or distinct analyses, create subheadings for each, making it easy for readers to navigate.
Step 2: Use Concise Text and Visuals
When writing the result section, use clear and concise language to communicate your results.
Avoid unnecessary details and focus on conveying the key research findings succinctly. Present each piece of data or result once, eliminating redundancy for a streamlined presentation.
- Utilize Tables and Figures : Tables and figures should be self-explanatory, with titles and captions providing essential context.
- Choose Appropriate Detail : Strike a balance between providing essential information and avoiding information overload.
Step 3: Be Objective and Specific
Present your findings without adding personal interpretation or analysis. Focus on conveying the observed outcomes in a straightforward and unbiased manner.
- Stick to the Facts : State what was found during the study without speculating on the reasons or implications.
- Include Numerical Data : Include means, medians, standard deviations, percentages, or any other statistical measures that accurately represent your results.
- Quantify Results : Use precise numbers to quantify your findings wherever possible. This adds clarity and concreteness to your presentation.
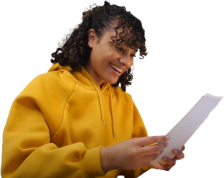
Tough Essay Due? Hire Tough Writers!
Step 4: Refer to Appendices
Evaluate the volume and complexity of your data to determine if it warrants inclusion in appendices. If your dataset is extensive and might overwhelm the main text, consider creating appendices.
Transfer detailed data, additional analyses, or supplementary information to appendices. Use separate appendices for different types of information, maintaining clarity.
- Highlight Relevance : Explain how the appendices supplement and enrich the content presented in the main text.
- Use Clear Labeling : Number or letter of each appendix for easy reference in the main text.
Step 5: Use Subheadings for Different Analyses
Recognize the various analyses conducted in your study, each addressing specific research questions or hypotheses. Introduce subheadings to distinguish and separate different analyses in the Results section.
Each subheading should correspond to a unique analysis, providing clarity to readers.
- Clearly Label Subsections : The subheading should succinctly convey the focus of the analysis without ambiguity.
- Guide Readers Effectively : The use of subheadings allows readers to locate specific information related to each analysis with ease.
Step 6: Highlight Patterns and Trends
Thoroughly examine your results to identify any recurring patterns or trends. Look for consistencies or variations in data points that are noteworthy for your study.
- Consider Multiple Analyses : Explore patterns across different analyses if applicable, ensuring a comprehensive understanding of your findings.
- Use Emphatic Language : Clearly convey the significance of identified trends in the context of your research questions.
- Connect to Research Questions: Explain how the observed trends contribute to answering the main queries of your study.
Step 7: Address Negative or Unexpected Results
Approach unexpected or negative results with transparency and honesty. Clearly state that the outcomes were not as anticipated.
Make sure to resist the temptation to downplay unexpected or negative results and acknowledge them as integral parts of the scientific process.
For Example:
Step 8: Relate to Research Questions/Hypotheses
At the last, revisit the research questions or hypotheses established at the beginning of your paper. This serves as a reminder of the primary objectives of your study.
Explicitly connect each set of findings to the corresponding research question or hypothesis. Make sure you clearly state how the results address the specific inquiries posed at the outset.
Differences Between Qualitative and Quantitative Data
Understanding the difference between qualitative and quantitative data is fundamental in research methodology.
These two distinct types of data collection offer unique insights, each contributing to a comprehensive understanding of phenomena.
In this section, we explore the key characteristics that set qualitative and quantitative data apart.
Tips for Presenting Quantitative Data in the Results Section
- Use clear and concise tables or graphs to present numerical results.
- Include descriptive statistics (mean, median, etc.) to summarize central tendencies.
Tips for Presenting Qualitative Data in the Results Section
- Summarize key themes or patterns derived from qualitative analysis.
- Use quotes or excerpts to illustrate significant findings.
Paper Due? Why Suffer? That's our Job!
How To Write The Results Section of A Research Paper - Examples
Here are a few examples that show how to write the results part of a research paper.
Results Section of a Research Paper - Apa
Results Section of a Qualitative Research Paper
Results Section of a Research Report
Result vs Discussion Section vs Conclusion - What’s the Difference?
The Results section presents a factual showcase of observed or measured outcomes.
In contrast, the discussion section goes beyond the facts, interpreting results, providing context to findings, and exploring their broader implications.
Finally, the conclusion section summarizes key points, discusses implications, and often suggests avenues for future research.
Mistakes to Avoid While Writing the Results Section
The results section of a research paper demands precision and clarity to communicate the study's outcomes effectively. However, several common mistakes can compromise the quality of this section.
To ensure an insightful results section, be mindful of the following pitfalls:
In summary, by following these tips and avoiding common mistakes, you can create a results section that meets academic standards and clearly shows why your research is important.
Remember, the results section sets the stage for the subsequent discussion. If you present your findings well, it makes your whole research paper better and easier to understand.
If you ever feel stuck while writing a research paper, especially in the results section, consider reaching out to MyPerfectWords.com!
Our experts can craft an outstanding result section that resonates with your whole research paper. We can also craft different parts of a research paper upon customized requests!
So, do not delay! Get your custom paper from our essay writing service for students now.
Frequently Asked Questions
How long should the results section of a research paper be.

The length varies, but keep it concise. Include enough information to support your discussion and conclusion without unnecessary details. A balanced Results section enhances the overall flow of your research paper.

What is the Difference between Results And Discussion Sections?
The results section presents the raw findings of a study, focusing on data and observations.
In contrast, the Discussion section interprets and analyzes these results, offering insights, context, and help readers understand the significance of the findings.

Write Essay Within 60 Seconds!

Nova Allison is a Digital Content Strategist with over eight years of experience. Nova has also worked as a technical and scientific writer. She is majorly involved in developing and reviewing online content plans that engage and resonate with audiences. Nova has a passion for writing that engages and informs her readers.

Paper Due? Why Suffer? That’s our Job!
Keep reading
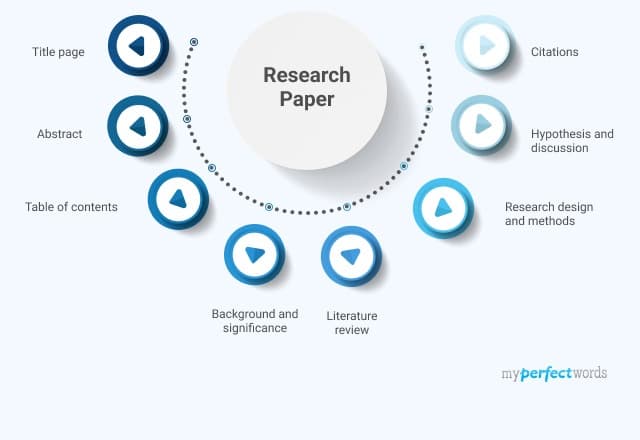
Research Paper Writing Guides
Research Paper Results Section
Last updated on: Mar 27, 2024
How To Write The Results Section of A Research Paper | Steps & Tips
By: Donna C.
Reviewed By: Rylee W.
Published on: Jan 9, 2024

Many researchers find themselves at a crossroads when it comes to crafting the results section of their research papers.
It's not just about displaying data; it's about creating a narrative that captivates readers and communicates the significance of your findings.
How do you ensure your data is not just presented but truly understood by your audience?
In this comprehensive guide, we will walk you through the process of writing an effective results section that transforms your research paper .
Whether you're struggling with data interpretation or seeking ways to make your findings resonate, our step-by-step guide will help you create outstanding result sections.
Let’s get started!
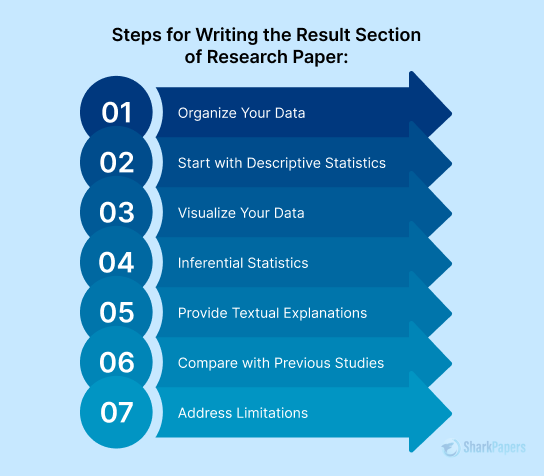
On this Page
What is the Result Section of a Research Paper?
The results section of a research paper is a critical component that presents the key findings derived from the study.
It serves as a factual and objective account of the data collected during the research process.
In this section, researchers report their observations and measurements of the hypothesis, often utilizing tables, graphs, or statistical measures to convey the information effectively.
What Does the Results Section of a Research Paper Include?
In the results section, you show off what you found in your research. Here's a quick look at what it includes:
- Data Presentation: Display your information using figures and tables.
- Descriptive Statistics: Use numbers like average and middle values to summarize your data.
- What It Means: Explain what your results say and if there are any patterns.
- Correlations and Relationships: Look for a correlation between two variables or different factors and talk about them.
- Limitations: Mention any problems or limits your study might have.
- Compare with Others: See how your findings relate to what others have discovered before.
How To Write The Results Section of A Research Paper Step-by-Step
Crafting an effective Results section is a crucial aspect of any research paper. Follow these steps to ensure your findings are presented clearly and concisely:
Step 1: Organize Your Data
Organizing your data is a critical first step in the Results section of a research paper. This involves structuring your raw data in a way that is clear, logical, and easily digestible for your readers.
- Raw Data Arrangement: Group similar data logically, such as by time or experimental conditions.
- Visual Representation: Use tables, graphs, or charts for clarity; pick the format that suits your data best.
- Clarity in Design: Ensure visuals are clear with labels, colors, and consistent formatting.
- Quick Understanding: Aim for simplicity; make sure readers can grasp the main points at a glance.
- Consistency: Keep a consistent writing style across visuals for a cohesive presentation.
Step 2: Start With Descriptive Statistics
In this section, dive into the specifics of your data through systematic description, providing a snapshot that captures both central tendencies and variability.
- Calculate the Mean: Find the average value of your data points, offering a central measure indicative of the overall trend.
- Explore the Median: Identify the middle point of your data, a robust measure that remains unaffected by extreme values.
- Assess Standard Deviation: Understand the dispersion of your data around the mean, highlighting the degree of variability.
Step 3: Visualize Your Data
Enhance understanding by incorporating visual representations. Graphs and charts can convey trends and patterns effectively, providing readers with a more intuitive grasp of your results.
- Select Appropriate Visuals: Choose the right type of graph or chart based on your data—bar charts for comparisons, line graphs for trends, and pie charts for proportions.
- Ensure Clarity: Design visuals with clear labels, legible fonts, and distinctive colors to facilitate easy interpretation.
- Highlight Trends: Emphasize significant trends or patterns in your data, guiding readers to key insights.
Step 4: Inferential Statistics
If applicable, apply inferential statistics to analyze the significance of your findings. Use tests to show statistically meaningful positive and negative results.
- Select Relevant Tests: Choose appropriate statistical tests based on your research question—ANOVA for multiple groups, t-tests for two groups, etc.
- Establish Significance Levels: Define significance levels (usually p-values) to determine whether observed differences are likely due to chance.
Step 5: Provide Textual Explanations
Accompany visual elements with clear and concise textual explanations. Explain the significance of observed trends or patterns in your visuals, ensuring readers grasp their relevance to your research problem.
Acknowledge and explain any unexpected results, offering insights into potential factors or nuances that may have influenced the outcomes.
Always tie your explanations back to your research question, emphasizing how each finding contributes to the broader understanding of the results of your study.
Step 6: Compare with Previous Studies
By discussing similarities, differences, or advancements in knowledge, you can highlight the uniqueness of your contribution to the field.
Summarize relevant findings from the literature review and review articles related to your research problem . Compare or contrast your results with those of previous studies. Discuss similarities and differences, emphasizing the novel aspects of your research.
In the end, identify any contributions your study makes to advancing knowledge in the field. Highlight how your findings build upon or challenge existing understandings.
Step 7: Address Limitations
Acknowledge and address any limitations in your study. Discuss how these limitations may have influenced your results, maintaining transparency about the potential impact on the study's outcomes.
Lastly, propose avenues for future research that could address the identified limitations, offering insights for researchers interested in building upon your study.
By following these steps, you can engage your audience, providing a comprehensive and insightful view of your research findings.
Results Section for Quantitative Research
When crafting the Results section for quantitative research, there are several key qualities that can enhance the clarity, validity, and overall impact of your presentation.
Here are the main qualities to consider for crafting a results section for quantitative research:
- Comprehensive Descriptions: Clearly state what each statistical test or measure represents and how it contributes to your study.
- Appropriate Visual Elements: Ensure that these visuals are accurately labeled, easy to interpret, and directly support the points made in the text.
- Inclusion of Key Statistical Measures: Include essential statistical measures relevant to your study, such as means, standard deviations, p-values, confidence intervals, and effect sizes.
- Concise Interpretation: Highlight the most salient patterns or trends and their implications. Save in-depth interpretation and discussion for the dedicated Discussion section.
Here is an example for quantitative results of a research paper:
Results Section Of A Quantitative Research Paper
Results Section for Qualitative Research
Writing the Results section for qualitative research involves presenting and interpreting the data collected through methods such as interviews, observations, or content analysis.
Here are the main qualities of a results section of qualitative research:
- Participant Voice: Prioritize the voices of the participants. Include direct quotes to give the participants a presence in the Results section.
- Negotiation of Bias: Acknowledge and discuss the potential biases and subjectivities that may have influenced the interpretation of the data.
- Negative Instances: Discuss instances where the data deviate from the identified themes and explore the reasons behind these variations.
- Comparison and Contrast: If applicable, compare and contrast themes across different participant groups, contexts, or time points. This adds depth to the analysis and helps in identifying patterns or variations within the data.
- Transition to Discussion: Conclude the Results section with a seamless transition to the Discussion section. Briefly summarize the main findings and indicate how they will be further explored and interpreted in the subsequent section.
Here is an example for qualitative results of a research paper:
Results Section Of A Qualitative Research Paper
Result Section Examples
Here are some examples for learning how to write the results section of a research paper.
Results Section Of A Research Paper Example
Summary Of Results In Research Example
Research Findings Example Pdf
How To Write The Results Section of A Research Paper APA
Mistakes to Avoid When Writing Results Section of Research Paper
Understanding the Results section of a research paper needs carefulness and attention to detail. To present your findings well, avoid these common mistakes:
Wrapping up, mastering the results section demands precision and clarity. By sidestepping common mistakes, you enhance the credibility of your findings.
Keep it simple, stay focused on your main question, and be transparent about limitations.
But still, if you think this work is too much for you, turn to the best paper writing service online .
We are experts who can craft an outstanding research paper as well as different sections of your research paper.
So, let experts handle all your academic affairs!
Frequently Asked Questions
What is the difference between the results and the discussion section.
Results present raw data and findings, while Discussion interprets and contextualizes results, providing explanations and exploring broader significance. Results are about "what," Discussion is about "why" and "what it means."
How Long Should the Results Section a Research Paper Be?
In general, the ideal length of this section is often several pages long, but there's no fixed rule. It's essential to balance thoroughness with conciseness.

Donna writes on a broad range of topics, but she is mostly passionate about social issues, current events, and human-interest stories. She has received high praise for her writing from both colleagues and readers alike. Donna is known in her field for creating content that is not only professional but also captivating.
Was This Blog Helpful?
Keep reading.
- Learning How to Write a Research Paper: Step-by-Step Guide
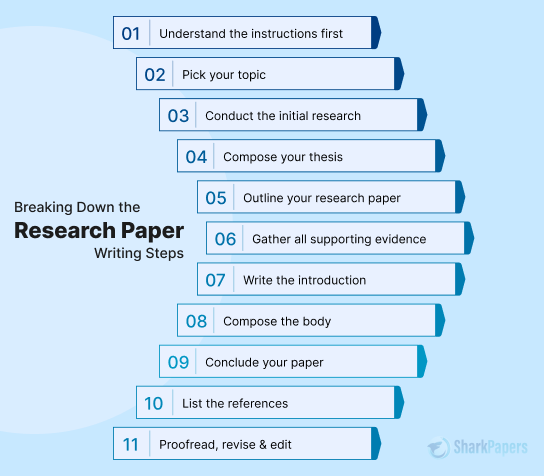
- Best 300+ Ideas For Research Paper Topics in 2024
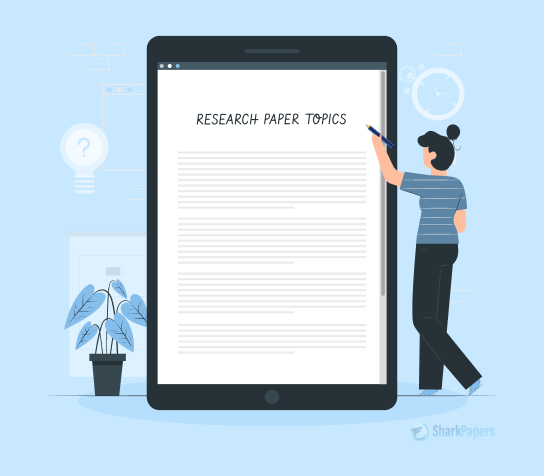
- A Complete Guide to Help You Write a Research Proposal
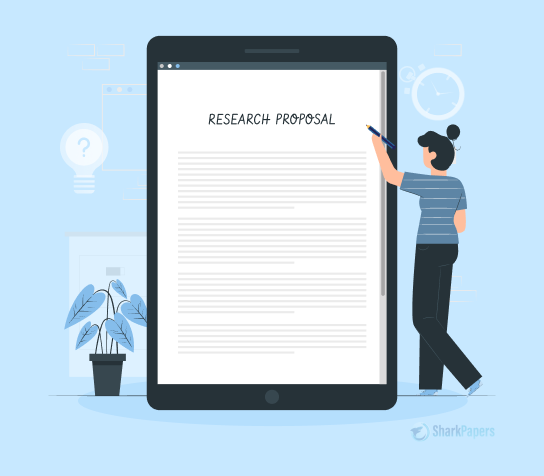
- The Definitive Guide on How to Start a Research Paper
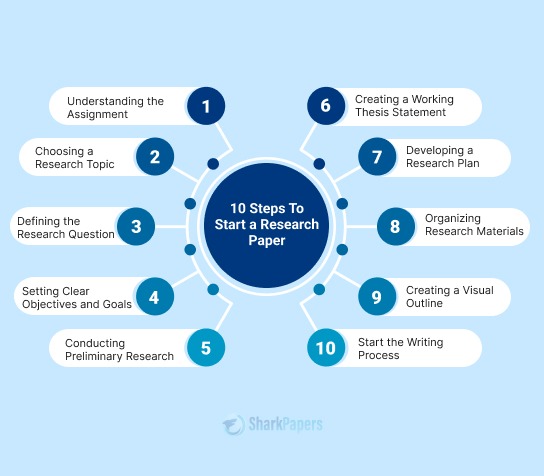
- How To Write An Introduction For A Research Paper - A Complete Guide
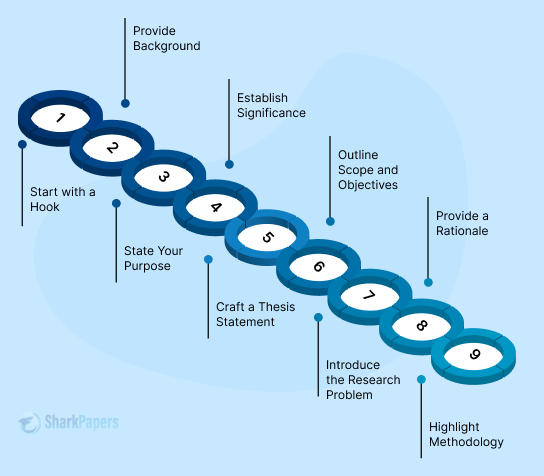
- Learn How To Write An Abstract For A Research Paper with Examples and Tips
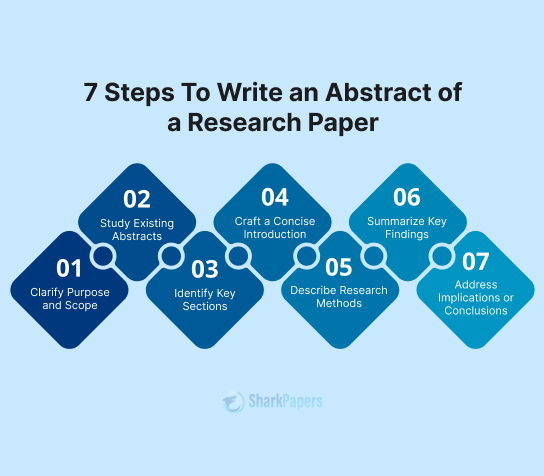
- How to Write a Literature Review for a Research Paper | A Complete Guide
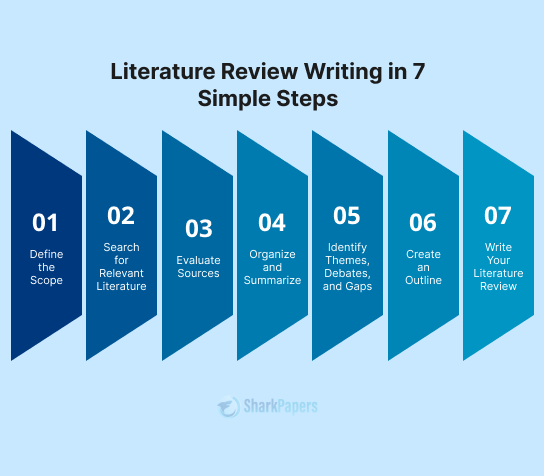
- How To Write The Methods Section of A Research Paper
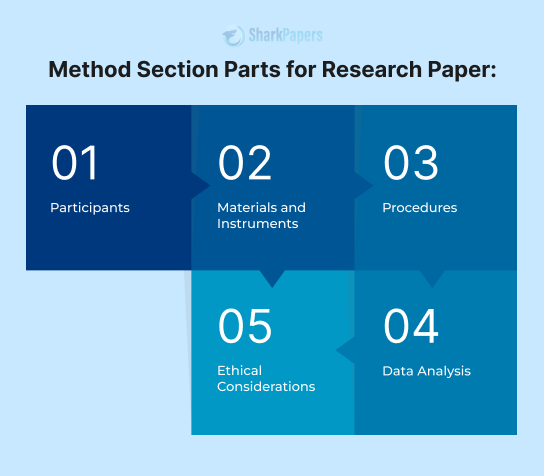
- How to Write a Research Paper Thesis: A Detailed Guide
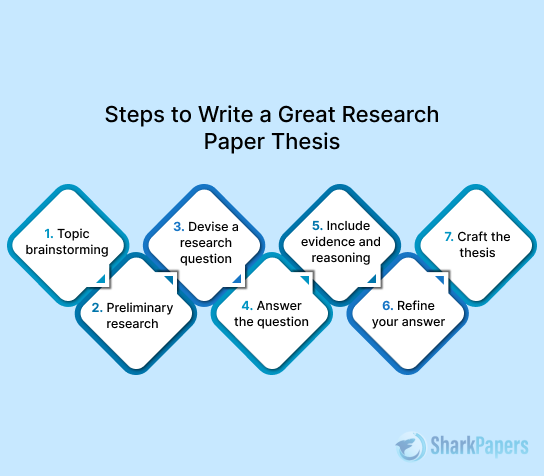
- How to Write a Research Paper Title That Stands Out
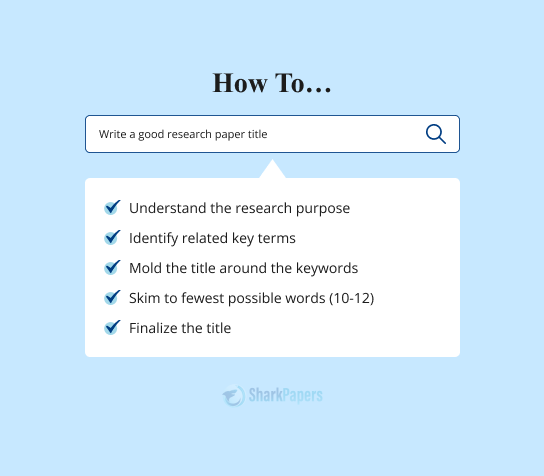
- A Detailed Guide on How To Write a Conclusion for a Research Paper
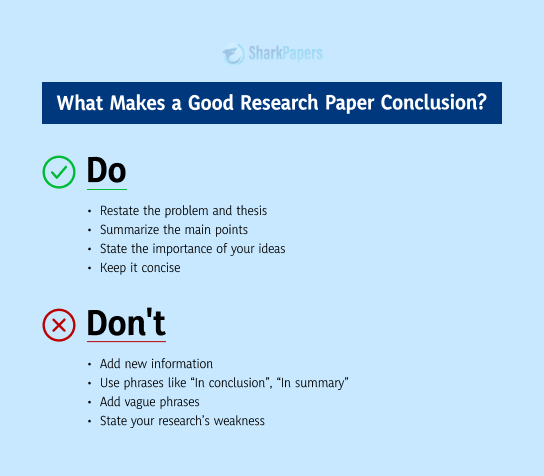
- How to Problem Statement for a Research Paper: An Easy Guide
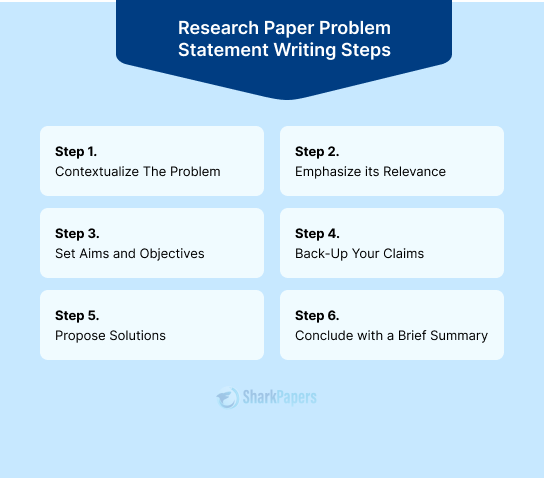
- How to Find Credible Sources for a Research Paper
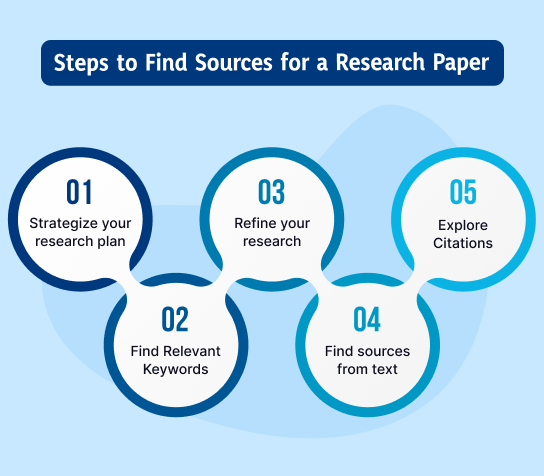
- A Detailed Guide: How to Write a Discussion for a Research Paper
)
- How To Write A Hypothesis In A Research Paper - A Simple Guide
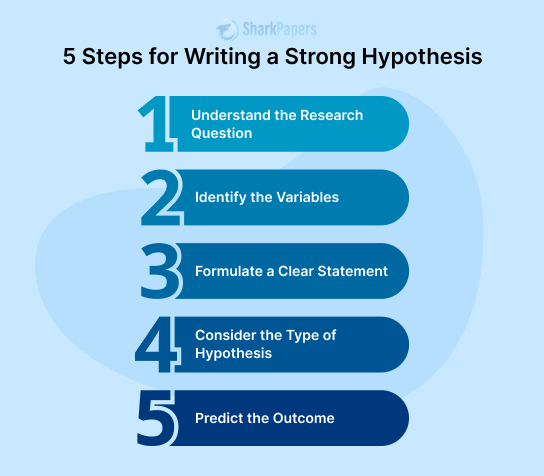
- Learn How To Cite A Research Paper in Different Formats: The Basics
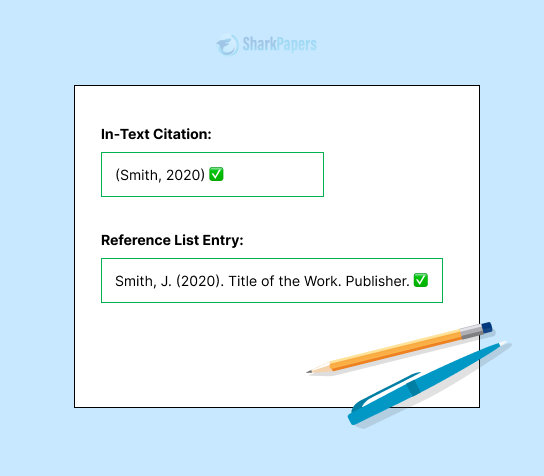
- The Ultimate List of Ethical Research Paper Topics in 2024
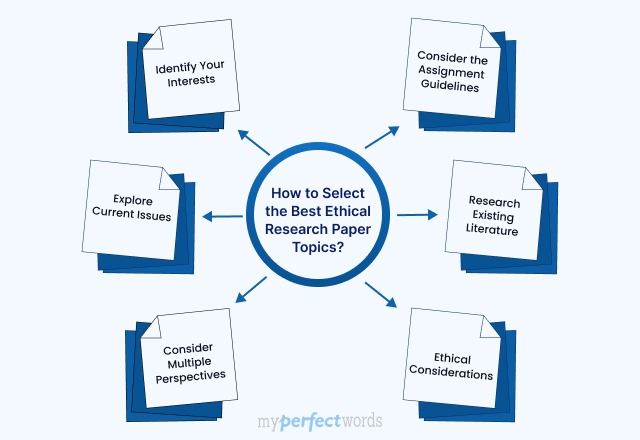
- 150+ Controversial Research Paper Topics to Get You Started
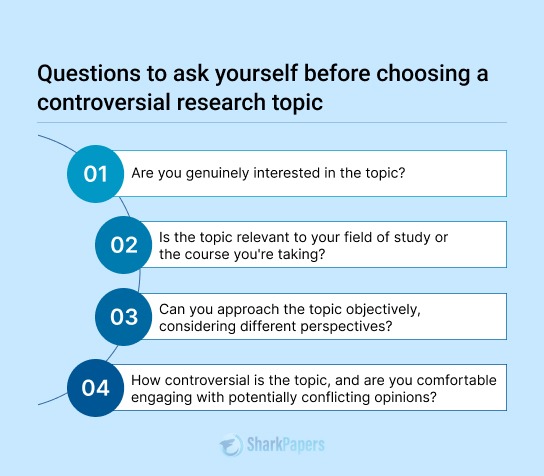
- How to Edit Research Papers With Precision: A Detailed Guide
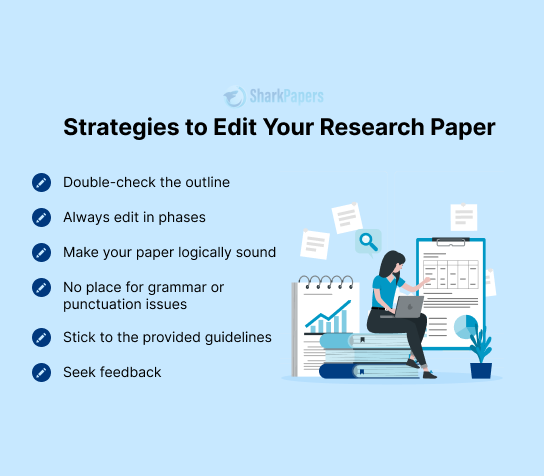
- A Comprehensive List of Argumentative Research Paper Topics
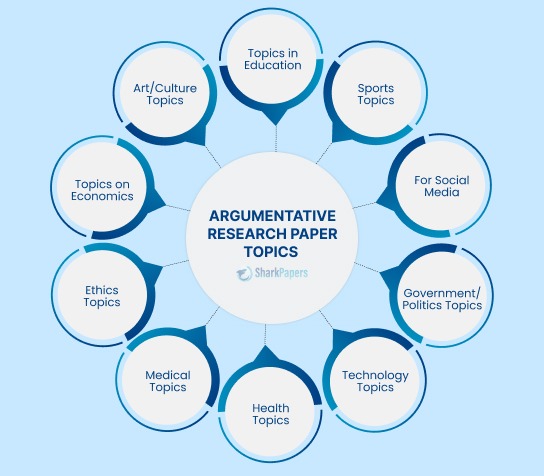
- A Detailed List of Amazing Art Research Paper Topics
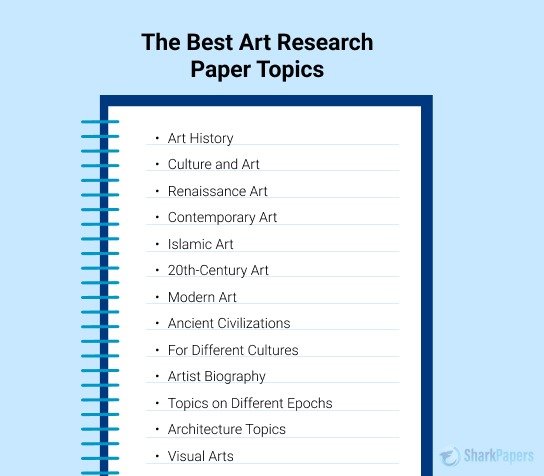
- Diverse Biology Research Paper Topics for Students: A Comprehensive List
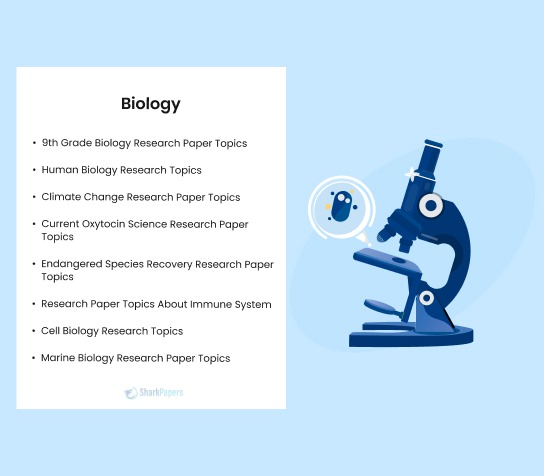
- 230 Interesting and Unique History Research Paper Topics
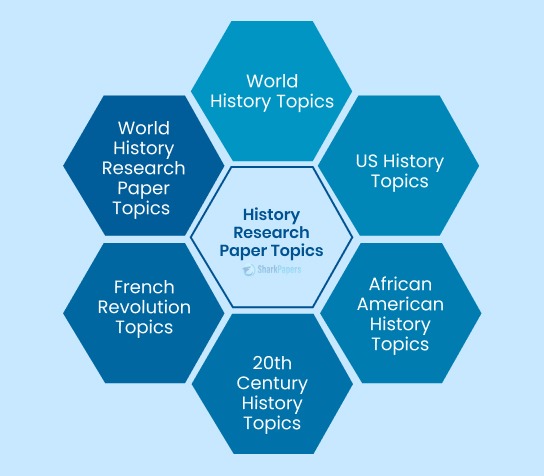
- 190 Best Business Research Paper Topics
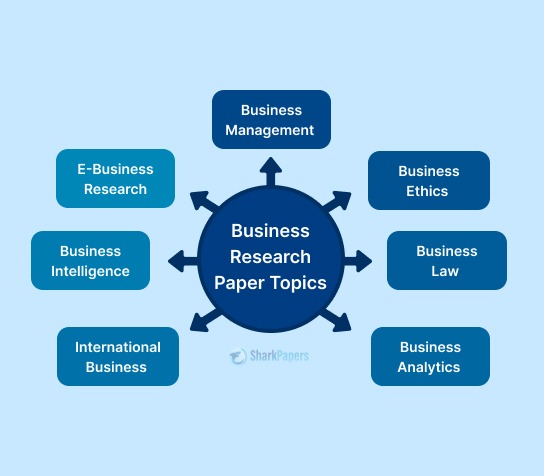
- 200+ Engaging and Novel Literature Research Paper Topics
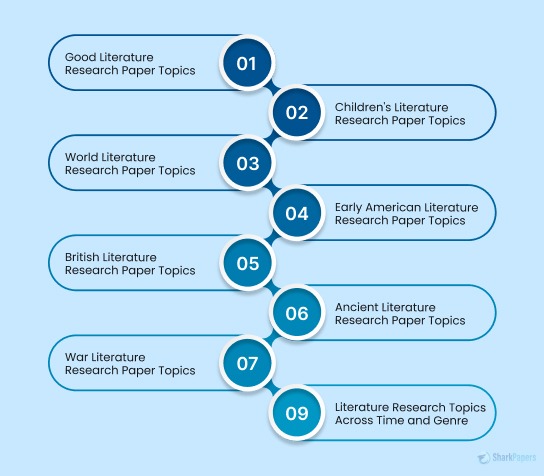
- A Guide on How to Write a Social Science Research
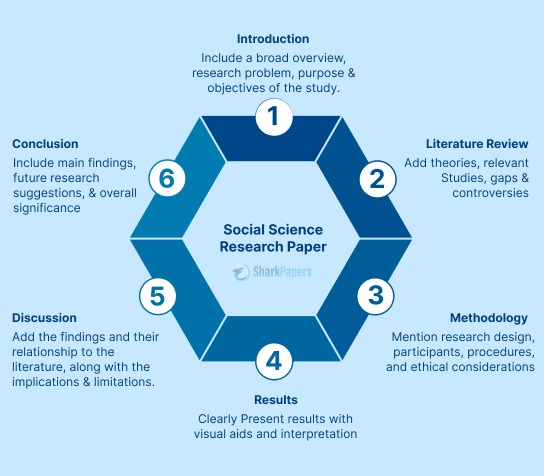
- Sociology Research Papers: Format, Outline, and Topics
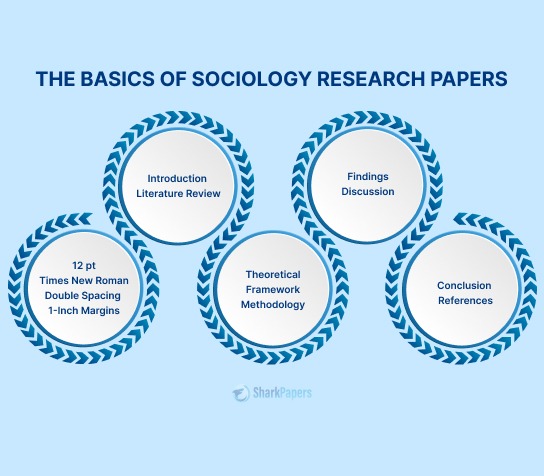
- Understanding the Basics of Biology Research Papers
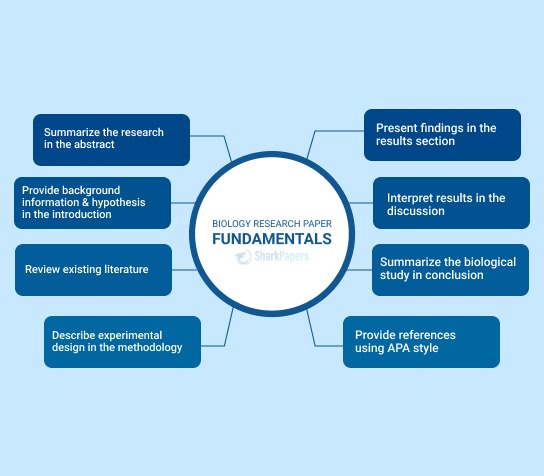
- How to Write a Psychology Research Paper: Guide with Easy Steps
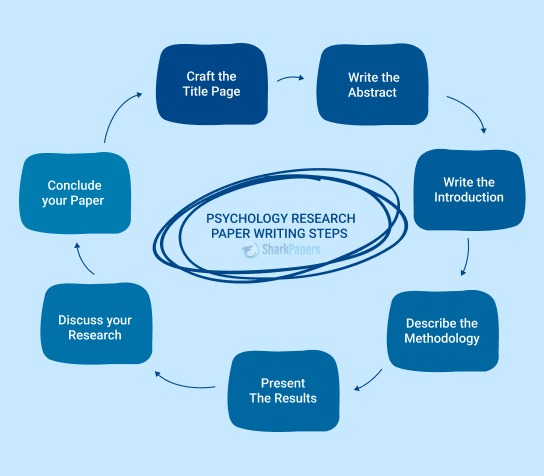
- Exploring the Different Types of Research Papers: A Guide
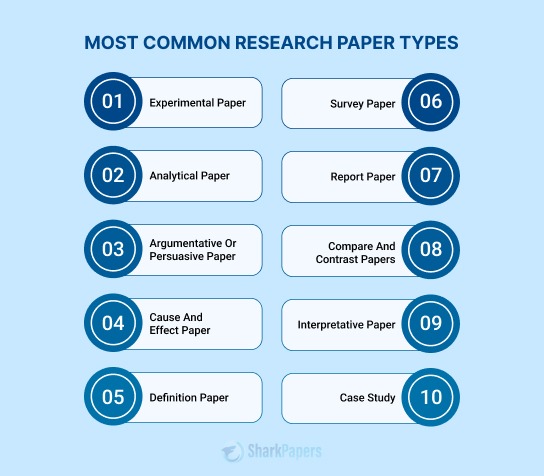
People Also Read
- scholarship essay
- press release example
- persuasive speech topics
- how to write an abstract
- graduation speech
Burdened With Assignments?
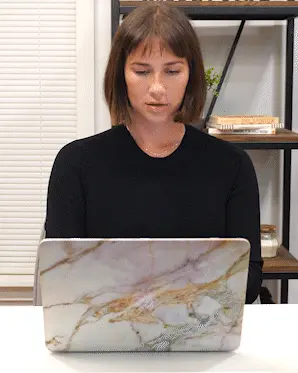
Advertisement
© 2024 - All rights reserved
2000+ SATISFIED STUDENTS
95% Satisfaction RATE
30 Days Money Back GUARANTEE
95% Success RATE

Privacy Policy | Terms & Conditions | Contact Us
© 2021 SharkPapers.com(Powered By sharkpapers.com). All rights reserved.
© 2022 Sharkpapers.com. All rights reserved.
LOGIN TO YOUR ACCOUNT
SIGN UP TO YOUR ACCOUNT
- Your phone no.
- Confirm Password
- I have read Privacy Policy and agree to the Terms and Conditions .
FORGOT PASSWORD
- SEND PASSWORD

How to Write the Results Section of A Research Paper
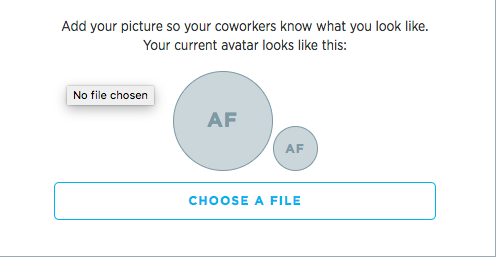
How to Evaluate Research
Writing a research paper can be a daunting experience. Whether it is your first college paper, the very first lab report you have done in high school or something you are hoping to have published, it can feel like a lot to master. Clearly, when you are doing an experiment, you want to list the results of the experiment or the research.
How do you structure the results of an experiment, and how do you communicate the results of your research? By writing clearly and answering any questions you raised earlier in your paper, you can be sure that your results section will be easily comprehensible and will bring your paper to a strong conclusion.
What Is a Research Paper?
Defined broadly, a research paper is any sort of written account of work that you undertook in order to learn more about a specific topic or set of conditions. Whether you read books in a library about ancient Egyptian hieroglyphics or wanted to conduct an experiment to see whether the sun can melt pennies, the written account of this exploration can be termed a "research paper."
In some situations, a research paper is also called a "lab report." This is usually the case when the investigation in question pertains to an experiment that was conducted in lab-like conditions. Either way, it essentially functions as a research paper.
Most research papers begin as a school assignment. While people in the academic or scientific fields and individuals who are interested in pursuing topics independently may take on research papers as a work assignment, most people's first research paper takes place while in school. Whatever you are researching, begin to think about it as an investigation. That can help you to set the paper up for a results section that will reveal what you have learned.
How to Imagine Research as an Investigation
What questions do you have about your topic? How can you get curious about the subject if it's something you at first think is boring? The key to writing the results of the research is having a question to investigate in the first place. If you need to do a research paper on something having to do with Italy, get curious about Italy. What about Italy is interesting to you? If you can choose the topic yourself, try to find something about the topic that engages you or makes you think and ask questions.
If the topic of your paper has something to do with science, and you really dislike science, this is an opportunity to get curious. Do you need to do an experiment demonstrating that sponges soak up water? Why might that be interesting or important? These are great places to begin.
Getting curious about the topic you are researching is critical. Getting curious can help you connect to the research and can make the experience more unique and interesting than it would ever have been otherwise. It can also make your research paper stand out as being distinctly yours. A lot of people can write a lazy summary of something, but only you can bring your individuality to the proceedings and use it as a lens to guide your research.
How Do I Structure a Research Paper?
The structure of a research paper or a lab report on an experiment is critical. Because research is scientific, you want to be as meticulous as possible so that all the necessary information is conveyed. You will want to begin your paper by explaining why the topic you are pursuing is worth researching. Explain why it interests you.
Explain what you hope to gain from conducting this research or this experiment. Tell the reader what your hypothesis is and explain why you've come to believe this to be true. Next, lay out your strategy or methodology. What are you going to do in this paper?
How are you planning to discover whether or not your hypothesis is correct? Explain your plan for figuring out whether your hypothesis is correct or explain the way that you decided to research the topic. Offer a list of resources that you consulted. Make it clear why you chose to go about the research in the way that you did.
What Sections Does a Research Paper Have?
Typically, a research paper has five major parts:
- Introduction
- Review of literature
The introduction is the section of the research paper where you introduce the question you are looking to investigate and explain why you are doing so. If there are statistics or quotes or other writing you have found that lends itself to supporting your investigation, you can introduce it here. For example, if you are writing about whether or not the Loch Ness monster is real, you can share quotes or statistics about the number of times that people have said they've seen it.
The next section of the paper, the review of literature, should be a synthesis of the research that you've done thus far that has informed your hypothesis. Gather and summarize the information that has led you to this point and make it clear that going into your research, you were aware of this literature, and you used it to develop your methods. In the methods section, you will begin to detail the way that you went about conducting your experiment or conducting your research.
What Is the Results and Discussion Section?
The results and discussion pieces are the two most critical parts of the research paper. This tells us in factual terms exactly what you discovered. The results section is not the place for analysis. The results section is not the place for narrative discussion or emotion either. The results section is only for the results of the research.
The results section provides the facts about what you discovered in the course of your research or experiment. The discussion section is where you can get analytical or reflective about exactly what you have discovered. This is the place where you can tell us what the results mean. Does it mean that your hypothesis was correct, or does it mean that you need to do further research or experiments before you can come to a definitive conclusion about this issue?
How Do You Write the Results of the Research?
In the results section of your paper, you need to list what you have discovered. If your experiment confirmed your hypothesis, save the discussion about that for the discussion section. The results section should simply be hard facts written in the passive voice.
Many students get confused between the active and passive voices when writing a research paper. Unlike the rest of the paper, the results section should be written in the passive voice in order to draw attention to the action and not to the person performing the action.
Once you have clearly defined what your experiment or research has yielded, you can move on to the discussion section.
How Do You Write the Discussion Section?
The discussion section is where you can analyze and make inferences about your research or your experiment. Tell the reader what it means to you now that your hypothesis was confirmed or proved to be incorrect. Moreover, what does it mean for the future of this research?
If your hypothesis was proven to be correct, can that be brought to bear on any other research or hypotheses? If your experiment was wholly inconclusive, can you say why that was? What went wrong? Is it something that could be corrected?
In What Tone Should You Write a Research Paper?
Many people who read research papers, including teachers, editors and professors, hate the passive voice. They consider the passive voice to be an example of poor writing. Many colleges have writing centers where they can help students to improve the quality of their writing, and one of the tasks they face most often is getting students out of the passive voice.
A sentence written in the active voice shows the subject acting on a direct object. "David mailed the package" is an example of a sentence in the active voice. On the contrary, a sentence written in the passive voice shows the object being acted on by a verb. An example is: "The package was mailed by David."
While technically the passive voice is not grammatically incorrect – and in some cases, given literary license, it is necessary – the passive voice is considered an example of less-than-ideal writing. Active and passive voice can change the quality of a piece of writing, particularly academic writing. If you find that you have written any sentences in your research paper in the passive voice anywhere other than in the results section, it is a good rule of thumb to go back and do a passive-to-active conversion.
What Part of My Research Paper Should Be in the Passive Voice?
The passive voice is not gramatically incorrect. It is used correctly when the intention of the sentence is to draw attention to the action and not the person performing the action. This is why when you write the results section of the research paper, you will want to employ the passive voice.
The passive voice tells us that the results of the experiment or the research are more important in this instance than the way that the research was carried out. Said another way, the results section is not about you. It is not about the way that you performed the research or the way that you set up the experiment. It is purely and simply about the results.
What Are Some Active and Passive Voice Rules?
There are some tips to make sure that you are writing in the active voice. However, keep in mind that in some cases, such as in the results section, you will need to use the passive voice. After all, if you talk about something that happened in the past or that happened to someone, you will need to use words like "was" and "had."
Sometimes, it can be effective to make something passive. For example, the phrase "the city of Rome was attacked by invaders" shows that the subject of the sentence is Rome and that is the thing that is being acted on, even if it is in a passive sense. "Invaders attacked the city of Rome" turns the focus to the invaders. A reader may well expect the following sentence to be about the invaders.
Pay close attention to the subject in the sentence. Is the subject the one carrying out the activities described in the verb? If not, go back and fix it.
Related Articles
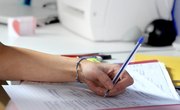
How to Write an Investigation Essay
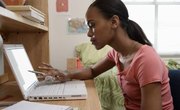
How to Make a Strong Argument in an Essay
How to Write a Lab Report
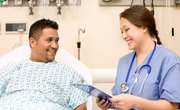
How to Write a Nursing Abstract
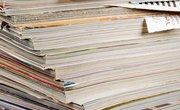
How to write a humanities paper
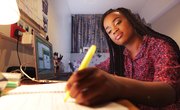
How to Do a Summary of a News Article
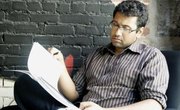
How to Write a Dissertation Summary
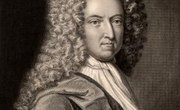
How to Write a Thesis Statement for "Robinson Crusoe"
- Towson University: Active/Passive Voice
- College of Western Idaho: What Are the Differences Between Active and Passive Voice?
- University of Wisconsin-Madison Writing Center: Use the Active Voice
- A Research Guide For Students: How to Write a Research Paper
- American Psychological Association
- Try starting this section by creating an outline of each hypothesis or research question followed by each statistical test you will use for it.
- Where relevant, state the effect size of the particular statistical test.
- Only include statistical tests that are relevant to your particular hypotheses or research questions. Excessive irrelevant statistical tests detract from the big picture and make it difficult for the reader to follow.
- Do not include specific calculations used to determine the statistic.
Ashley Friedman is a freelance writer with experience writing about education for a variety of organizations and educational institutions as well as online media sites. She has written for Pearson Education, The University of Miami, The New York City Teaching Fellows, New Visions for Public Schools, and a number of independent secondary schools. She lives in Los Angeles.

- Dissertation & Thesis Guides
- Basics of Dissertation & Thesis Writing
- How to Write a Results Section for a Dissertation or Research Paper: Guide & Examples
- Speech Topics
- Basics of Essay Writing
- Essay Topics
- Other Essays
- Main Academic Essays
- Research Paper Topics
- Basics of Research Paper Writing
- Miscellaneous
- Chicago/ Turabian
- Data & Statistics
- Methodology
- Admission Writing Tips
- Admission Advice
- Other Guides
- Student Life
- Studying Tips
- Understanding Plagiarism
- Academic Writing Tips

- Essay Guides
- Research Paper Guides
- Formatting Guides
- Basics of Research Process
- Admission Guides
How to Write a Results Section for a Dissertation or Research Paper: Guide & Examples

Table of contents

Use our free Readability checker
A results section is a crucial part of a research paper or dissertation, where you analyze your major findings. This section goes beyond simply presenting study outcomes. You should also include a comprehensive statistical analysis and interpret the collected data in detail.
Without dissertation research results, it is impossible to imagine a scientific work. Your task here is to present your study findings. What are qualitative or quantitative indicators? How to use tables and diagrams? How to describe data? Our article answers all these questions and many more. So, read further to discover how to analyze and describe your research indexes or contact or professionals for dissertation help from StudyCrumb.
What Is a Results Section of Dissertation?
The results section of a dissertation is a data statement from your research. Here you should present the main findings of your study to your readers. This section aims to show information objectively, systematically, concisely. It is allowed using text supplemented with illustrations. In general, this section's length is not limited but should include all necessary data. Interpretations or conclusions should not be included in this section. Therefore, in theory, this is one of your shortest sections. But it can also be one of the most challenging sections. The introduction presents a research topic and answers the question "why?". The Methods section explains the data collection process and answers "how?". Meanwhile, the result section shows actual data gained from experiments and tells "what?" Thus, this part plays a critical role in highlighting study's relevance. This chapter gives reader study relevance with novelty. So, you should figure out how to write it correctly. Here are main tasks that you should keep in mind while writing:
- Results answer the question "What was found in your research?"
- Results contain only your study's outcome. They do not include comments or interpretations.
- Results must always be presented accurately & objectively.
- Tables & figures are used to draw readers' attention. But the same data should never be presented in the form of a table and a figure. Don't repeat anything from a table also in text.
Dissertation: Results vs Discussion vs Conclusion
Results and discussion sections of a dissertation are often confused among researchers. Sometimes both these parts are mixed up with a conclusion for thesis . Figured out what is covered in each of these important chapters. Your readers should see that you notice how different they are. A clear understanding of differences will help you write your dissertation more effectively. 5 differences between Results VS Discussion VS Conclusion:
Wanna figure out the actual difference between discussion vs conclusion? Check out our helpful articles about Dissertation Discussion or Dissertation Conclusion.
Present Your Findings When Writing Results Section of Dissertation
Now it's time to understand how to arrange the results section of the dissertation. First, present most general findings, then narrow it down to a more specific one. Describe both qualitative & quantitative results. For example, imagine you are comparing the behavior of hamsters and mice. First, say a few words about the behavioral type of mammals that you studied. Then, mention rodents in general. At end, describe specific species of animals you carried out an experiment on.
Qualitative Results Section in Dissertation
In your dissertation results section, qualitative data may not be directly related to specific sub-questions or hypotheses. You can structure this chapter around main issues that arise when analyzing data. For each question, make a general observation of what data show. For example, you may recall recurring agreements or differences, patterns, trends. Personal answers are the basis of your research. Clarify and support these views with direct quotes. Add more information to the thesis appendix if it's needed.
Quantitative Results Section in a Dissertation
The easiest way to write a quantitative dissertation results section is to build it around a sub-question or hypothesis of your research. For each subquery, provide relevant results and include statistical analysis . Then briefly evaluate importance & reliability. Notice how each result relates to the problem or whether it supports the hypothesis. Focus on key trends, differences, and relationships between data. But don't speculate about their meaning or consequences. This should be put in the discussion vs conclusion section. Suppose your results are not directly related to answering your questions. Maybe there is additional information that helps readers understand how you collect data. In that case, you can include them in the appendix. It is often helpful to include visual elements such as graphs, charts, and tables. But only if they accurately support your results and add value.
Tables and Figures in Results Section in Dissertation
We recommend you use tables or figures in the dissertation results section correctly. Such interpretation can effectively present complex data concisely and visually. It allows readers to quickly gain a statistical overview. On the contrary, poorly designed graphs can confuse readers. That will reduce the effectiveness of your article. Here are our recommendations that help you understand how to use tables and figures:
- Make sure tables and figures are self-explanatory. Sometimes, your readers may look at tables and figures before reading the entire text. So they should make sense as separate elements.
- Do not repeat the content of tables and figures in text. Text can be used to highlight key points from tables and figures. But do not repeat every element.
- Make sure that values or information in tables and text are consistent. Make sure that abbreviations, group names, interpretations are the same as in text.
- Use clear, informative titles for tables and figures. Do not leave any table or figure without a title or legend. Otherwise, readers will not be able to understand data's meaning. Also, make sure column names, labels, figures are understandable.
- Check accuracy of data presented in tables and figures. Always double-check tables and figures to make sure numbers converge.
- Tables should not contain redundant information. Make sure tables in the article are not too crowded. If you need to provide extensive data, use Appendixes.
- Make sure images are clear. Make sure images and all parts of drawings are precise. Lettering should be in a standard font and legible against the background of the picture.
- Ask for permission to use illustrations. If you use illustrations, be sure to ask copyright holders and indicate them.
Tips on How to Write a Results Section
We have prepared several tips on how to write the results section of the dissertation! Present data collected during study objectively, logically, and concisely. Highlight most important results and organize them into specific sections. It is an excellent way to show that you have covered all the descriptive information you need. Correct usage of visual elements effectively helps your readers with understanding. So, follow main 3 rules for writing this part:
- State only actual results. Leave explanations and comments for Discussion.
- Use text, tables, and pictures to orderly highlight key results.
- Make sure that contents of tables and figures are not repeated in text.
In case you have questions about a conceptual framework in research , you will find a blog dedicated to this issue in our database.
What to Avoid When Writing the Results Section of a Dissertation
Here we will discuss how NOT to write the results section of a dissertation. Or simply, what points to avoid:
- Do not make your research too complicated. Your paper, tables, and graphs should be clearly marked and follow order. So that they can exist independently without further explanation.
- Do not include raw data. Remember, you are summarizing relevant results, not reporting them in detail. This chapter should briefly summarize your findings. Avoid complete introduction to each number and calculation.
- Do not contradict errors or false results. Explain these errors and contradictions in conclusions. This often happens when different research methods have been used.
- Do not write a conclusion or discussion. Instead, this part should contain summaries of findings.
- Do not tend to include explanations and inferences from results. Such an approach can make this chapter subjective, unclear, and confusing to the reader.
- Do not forget about novelty. Its lack is one of the main reasons for the paper's rejection.
Dissertation Results Section Example
Let's take a look at some good results section of dissertation examples. Remember that this part shows fundamental research you've done in detail. So, it has to be clear and concise, as you can see in the sample.

Final Thoughts on Writing Results Section of Dissertation
When writing a results section of a dissertation, highlight your achievements by data. The main chapter's task is to convince the reader of conclusions' validity of your research. You should not overload text with too detailed information. Never use words whose meanings you do not understand. Also, oversimplification may seem unconvincing for readers. But on the other hand, writing this part can even be fun. You can directly see your study results, which you'll interpret later. So keep going, and we wish you courage!

Writing any academic paper is long and thorough work. But StudyCrumb got you back! Our professional writers will deliver any type of work quickly and excellently!

Joe Eckel is an expert on Dissertations writing. He makes sure that each student gets precious insights on composing A-grade academic writing.

Thank you for visiting nature.com. You are using a browser version with limited support for CSS. To obtain the best experience, we recommend you use a more up to date browser (or turn off compatibility mode in Internet Explorer). In the meantime, to ensure continued support, we are displaying the site without styles and JavaScript.
- View all journals
- My Account Login
- Explore content
- About the journal
- Publish with us
- Sign up for alerts
- Open access
- Published: 30 April 2024
Nightside clouds and disequilibrium chemistry on the hot Jupiter WASP-43b
- Taylor J. Bell ORCID: orcid.org/0000-0003-4177-2149 1 , 2 ,
- Nicolas Crouzet ORCID: orcid.org/0000-0001-7866-8738 3 ,
- Patricio E. Cubillos 4 , 5 ,
- Laura Kreidberg 6 ,
- Anjali A. A. Piette ORCID: orcid.org/0000-0002-4487-5533 7 ,
- Michael T. Roman ORCID: orcid.org/0000-0001-8206-2165 8 , 9 ,
- Joanna K. Barstow ORCID: orcid.org/0000-0003-3726-5419 10 ,
- Jasmina Blecic 11 , 12 ,
- Ludmila Carone ORCID: orcid.org/0000-0001-9355-3752 5 ,
- Louis-Philippe Coulombe ORCID: orcid.org/0000-0002-2195-735X 13 ,
- Elsa Ducrot ORCID: orcid.org/0000-0002-7008-6888 14 ,
- Mark Hammond 15 ,
- João M. Mendonça 16 ,
- Julianne I. Moses ORCID: orcid.org/0000-0002-8837-0035 17 ,
- Vivien Parmentier 18 ,
- Kevin B. Stevenson ORCID: orcid.org/0000-0002-7352-7941 19 ,
- Lucas Teinturier ORCID: orcid.org/0000-0002-0797-5746 20 , 21 ,
- Michael Zhang 22 ,
- Natalie M. Batalha 23 ,
- Jacob L. Bean 22 ,
- Björn Benneke ORCID: orcid.org/0000-0001-5578-1498 13 ,
- Benjamin Charnay 20 ,
- Katy L. Chubb 24 ,
- Brice-Olivier Demory 25 , 26 ,
- Peter Gao 7 ,
- Elspeth K. H. Lee 25 ,
- Mercedes López-Morales 27 ,
- Giuseppe Morello ORCID: orcid.org/0000-0002-4262-5661 28 , 29 , 30 ,
- Emily Rauscher ORCID: orcid.org/0000-0003-3963-9672 31 ,
- David K. Sing ORCID: orcid.org/0000-0001-6050-7645 32 , 33 ,
- Xianyu Tan 15 , 34 , 35 ,
- Olivia Venot ORCID: orcid.org/0000-0003-2854-765X 36 ,
- Hannah R. Wakeford ORCID: orcid.org/0000-0003-4328-3867 37 ,
- Keshav Aggarwal ORCID: orcid.org/0000-0002-7004-8670 38 ,
- Eva-Maria Ahrer 39 , 40 ,
- Munazza K. Alam ORCID: orcid.org/0000-0003-4157-832X 7 ,
- Robin Baeyens ORCID: orcid.org/0000-0001-7578-969X 41 ,
- David Barrado ORCID: orcid.org/0000-0002-5971-9242 42 ,
- Claudio Caceres ORCID: orcid.org/0000-0002-6617-3823 43 , 44 , 45 ,
- Aarynn L. Carter 23 ,
- Sarah L. Casewell 8 ,
- Ryan C. Challener 31 ,
- Ian J. M. Crossfield 46 ,
- Leen Decin ORCID: orcid.org/0000-0002-5342-8612 47 ,
- Jean-Michel Désert 41 ,
- Ian Dobbs-Dixon 11 ,
- Achrène Dyrek 14 ,
- Néstor Espinoza 33 , 48 ,
- Adina D. Feinstein 22 , 49 ,
- Neale P. Gibson 50 ,
- Joseph Harrington ORCID: orcid.org/0000-0002-8955-8531 51 ,
- Christiane Helling 5 ,
- Renyu Hu ORCID: orcid.org/0000-0003-2215-8485 52 , 53 ,
- Nicolas Iro 54 ,
- Eliza M.-R. Kempton ORCID: orcid.org/0000-0002-1337-9051 55 ,
- Sarah Kendrew 56 ,
- Thaddeus D. Komacek ORCID: orcid.org/0000-0002-9258-5311 55 ,
- Jessica Krick 57 ,
- Pierre-Olivier Lagage 14 ,
- Jérémy Leconte 58 ,
- Monika Lendl ORCID: orcid.org/0000-0001-9699-1459 59 ,
- Neil T. Lewis 60 ,
- Joshua D. Lothringer 61 ,
- Isaac Malsky ORCID: orcid.org/0000-0003-0217-3880 31 ,
- Luigi Mancini ORCID: orcid.org/0000-0002-9428-8732 6 , 62 , 63 ,
- Megan Mansfield ORCID: orcid.org/0000-0003-4241-7413 64 ,
- Nathan J. Mayne ORCID: orcid.org/0000-0001-6707-4563 65 ,
- Thomas M. Evans-Soma ORCID: orcid.org/0000-0001-5442-1300 6 , 66 ,
- Karan Molaverdikhani ORCID: orcid.org/0000-0002-0502-0428 67 , 68 ,
- Nikolay K. Nikolov ORCID: orcid.org/0000-0002-6500-3574 48 ,
- Matthew C. Nixon ORCID: orcid.org/0000-0001-8236-5553 55 ,
- Enric Palle ORCID: orcid.org/0000-0003-0987-1593 28 ,
- Dominique J. M. Petit dit de la Roche 59 ,
- Caroline Piaulet ORCID: orcid.org/0000-0002-2875-917X 13 ,
- Diana Powell 27 ,
- Benjamin V. Rackham ORCID: orcid.org/0000-0002-3627-1676 69 , 70 ,
- Aaron D. Schneider ORCID: orcid.org/0000-0002-1448-0303 47 , 71 ,
- Maria E. Steinrueck ORCID: orcid.org/0000-0001-8342-1895 6 ,
- Jake Taylor 13 , 15 ,
- Luis Welbanks ORCID: orcid.org/0000-0003-0156-4564 72 ,
- Sergei N. Yurchenko ORCID: orcid.org/0000-0001-9286-9501 73 ,
- Xi Zhang ORCID: orcid.org/0000-0002-8706-6963 74 &
- Sebastian Zieba ORCID: orcid.org/0000-0003-0562-6750 3 , 6
Nature Astronomy ( 2024 ) Cite this article
2380 Accesses
560 Altmetric
Metrics details
Hot Jupiters are among the best-studied exoplanets, but it is still poorly understood how their chemical composition and cloud properties vary with longitude. Theoretical models predict that clouds may condense on the nightside and that molecular abundances can be driven out of equilibrium by zonal winds. Here we report a phase-resolved emission spectrum of the hot Jupiter WASP-43b measured from 5 μm to 12 μm with the JWST’s Mid-Infrared Instrument. The spectra reveal a large day–night temperature contrast (with average brightness temperatures of 1,524 ± 35 K and 863 ± 23 K, respectively) and evidence for water absorption at all orbital phases. Comparisons with three-dimensional atmospheric models show that both the phase-curve shape and emission spectra strongly suggest the presence of nightside clouds that become optically thick to thermal emission at pressures greater than ~100 mbar. The dayside is consistent with a cloudless atmosphere above the mid-infrared photosphere. Contrary to expectations from equilibrium chemistry but consistent with disequilibrium kinetics models, methane is not detected on the nightside (2 σ upper limit of 1–6 ppm, depending on model assumptions). Our results provide strong evidence that the atmosphere of WASP-43b is shaped by disequilibrium processes and provide new insights into the properties of the planet’s nightside clouds. However, the remaining discrepancies between our observations and our predictive atmospheric models emphasize the importance of further exploring the effects of clouds and disequilibrium chemistry in numerical models.
Similar content being viewed by others
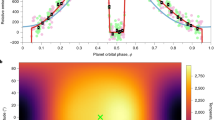
Diurnal variations in the stratosphere of the ultrahot giant exoplanet WASP-121b
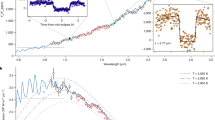
A broadband thermal emission spectrum of the ultra-hot Jupiter WASP-18b
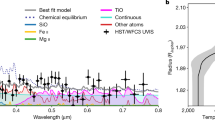
UV absorption by silicate cloud precursors in ultra-hot Jupiter WASP-178b
Hot Jupiters are tidally synchronized to their host stars, with vast differences in irradiation between the dayside and nightside. Previous observations with the Hubble Space Telescope (HST) and the Spitzer Space Telescope show that these planets have cooler nightsides and weaker hotspot offsets than expected from cloud-free three-dimensional models 1 , 2 , 3 , 4 , 5 . The main mechanism believed to be responsible for this behaviour is the presence of nightside clouds, which would hide the thermal flux of the planet and lead to a sharp longitudinal gradient in brightness temperature 3 , 4 , 6 , 7 , 8 , 9 , 10 . Other mechanisms have been proposed, such as the presence of atmospheric drag due to hydrodynamic instabilities or magnetic coupling 11 , 12 , 13 , super-stellar atmospheric metallicity 14 , 15 , or interaction between the deep winds and the photosphere 16 , but these mechanisms are less universal than the cloud hypothesis 17 , 18 .
WASP-43b, a hot Jupiter with an orbital period of just 19.5 h (ref. 19 ), is an ideal target for thermal phase-curve observations. Its host star is a K7 main-sequence star 87 pc away with metallicity close to solar and weak variability 20 . Previous measurements of the planet’s orbital phase curve in the near-infrared have revealed a large temperature contrast between the dayside and nightside hemispheres, broadly consistent with the presence of nightside clouds 3 , 21 , 22 , which could be composed of magnesium silicates (Mg 2 SiO 4 /MgSiO 2 ) and other minerals (for example, MnS, Na 2 S, metal oxides) 23 , 24 . Owing to the low nightside flux, the exact temperature and cloud properties were challenging to determine from previous observations 4 , 25 , 26 . With the mid-infrared capabilities of the JWST, we have the opportunity to measure the phase-resolved thermal spectrum with unprecedented sensitivity, particularly on the cold nightside. We observed a full orbit of WASP-43b in the 5–12 μm range with the JWST’s Mid-Infrared Instrument (MIRI) 27 in low-resolution spectroscopy (LRS) 28 slitless mode on 1 and 2 December 2022, as part of the Transiting Exoplanet Community Early Release Science Program (JWST-ERS-1366). This continuous observation lasted 26.5 h at a cadence of 10.34 s (9,216 integrations) and included a full phase curve with one transit and two eclipses.
We performed multiple independent reductions and fits to these observations (see ‘Data reduction pipelines’ and ‘Light-curve fitting’ in Methods ) to ensure robust conclusions. Our analyses all identified a strong systematic noise feature from 10.6 μm to 11.8 μm, the source of which is still unclear, and we were unable to adequately detrend these 10.6–11.8 μm data (see ‘Shadowed region effect’ in Methods ). As shown in Extended Data Fig. 1 , we also found that larger wavelength bins were required to accurately estimate our final spectral uncertainties (see ‘Spectral binning’ in Methods ). As a result, our final analyses consider only the 5–10.5 μm data, which we split into 11 channels with a constant 0.5 μm wavelength spacing. Similar to the MIRI commissioning time-series observations, our data show a strong downwards exponential ramp in the first ~60 min and a weaker ramp throughout the observation 29 (Extended Data Fig. 2 ). To minimize correlations with the phase variations, we removed the initial strong ramp by excluding the first 779 integrations (134.2 min) and then fitted a single exponential ramp model to the remaining data. A single ramp effectively removed the systematic noise, with the broadband light curve showing scatter ~1.25× the expected photon noise, while the spectroscopic light curves reach as low as ~1.1× the photon limit, probably due to improved decorrelation of wavelength-dependent systematics. Figure 1 shows the spectral light curves, broadband light curve, dayside spectra and nightside spectra from our fiducial reduction and fit.
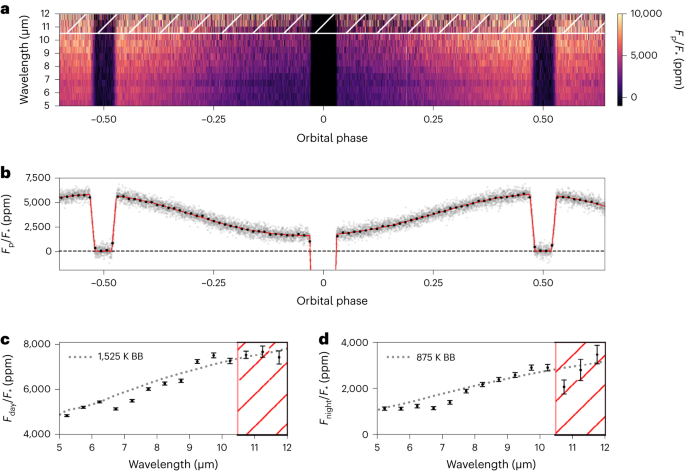
a , The observed spectroscopic light curves binned to a 0.5 μm wavelength resolution and after systematic noise removal, following the Eureka! v1 methods. The first 779 integrations have been removed from this figure and our fits as they were impacted by strongly decreasing flux. Wavelengths longer than 10.5 μm marked with a hatched region were affected by the ‘shadowed region effect’ ( Methods ) and could not be reliably reduced. b , The observed band-integrated light curve after systematic noise removal (grey points) and binned data with a cadence of 15 min (black points, with error bars smaller than the point sizes), compared with the best-fitting astrophysical model (red line). c , d , The measured dayside ( c ) and nightside ( d ) emission spectra are shown with black points and 1 σ error bars, and black-body curves (dotted line denoted as ‘BB’, assuming a PHOENIX 74 , 75 , 76 model for the star) are shown to emphasize planetary spectral features with black-body temperatures estimated by eye to match the continuum flux levels. Wavelengths longer than 10.5 μm were affected by the shadowed region effect and are unreliable.
From our Eureka! v1 analysis ( Methods ), we measure a broadband (5–10.5 μm) peak-to-trough phase variation of 4,180 ± 33 ppm with an eclipse depth of 5,752 ± 19 ppm and a nightside flux of 1,636 ± 37 ppm. Assuming a PHOENIX stellar model and marginalizing over the published stellar and system parameters 30 , the broadband dayside brightness temperature is 1,524 ± 35 K while the nightside is 863 ± 23 K. This corresponds to a day–night brightness temperature contrast of 659 ± 19 K, in agreement with the large contrasts previously observed 4 , 21 , 22 , 25 . The phase variations are well fitted by a sum of two sinusoids (the first and second harmonics), with two sinusoids preferred over a single sinusoid at 16 σ (see ‘Determining the number of sinusoid harmonics’ in Methods ) for the broadband light curve. The peak brightness of the broadband phase curve occurs at 7.34 ± 0.38° E from the substellar point (although individual reductions find offsets ranging from 7.34° E to 9.60° E), while previous studies have found offsets of 12.3 ± 1.0° E for HST Wide Field Camera 3’s (WFC3) 1.1–1.7 μm bandpass 21 , offsets ranging from 4.4° E to 12.2° E for Spitzer InfraRed Array Camera’s (IRAC) 3.6 μm filter 22 , 25 and offsets ranging from 10.4° E to 21.1° E for Spitzer/IRAC’s 4.5 μm filter 4 , 22 , 25 , 26 , 31 , 32 . Overall, these broadband data represent roughly an order of magnitude in improved precision on the eclipse depth (6×), phase-curve amplitude (6×) and phase-curve offset (10×) over individual Spitzer/IRAC 4.5 μm observations of the system 22 , 26 , 32 ; this improvement is largely driven by the JWST’s larger mirror (45×), about 12× less pointing jitter (per axis), about 4× improved stability in the width of the point spread function (PSF) along each axis and MIRI’s much broader bandpass.
Model interpretation
To interpret the measurements, we compared the observations with synthetic phase curves and emission spectra derived from general circulation models (GCMs). Simulations were gathered from five different modelling groups, amounting to 31 separate GCM realizations exploring a range of approaches and assumptions. Notably, in addition to cloud-free simulations, the majority of the GCMs modelled clouds with spatial distributions that were either fully predicted 5 , 26 , 33 or simply limited to the planet’s nightside 4 . For the predictive cloud models, simulations favoured warmer, clearer daysides with cooler, cloudier nightsides, but the precise distributions varied with assumptions regarding cloud physics and compositions. In general, models with smaller cloud particles or extended vertical distributions tended to produce thicker clouds at the pressures sensed by the observations. Details of the different models are provided in Methods .
Despite fundamental differences in the models and the parameterizations they employ, simulated phase curves derived from models that include cloud opacity on the planet’s nightside provide a better match to the observed nightside flux compared with the clear simulations (Fig. 2 ). In contrast, the observed dayside fluxes (180° orbital phase) were matched similarly well by models with and without clouds. This implies the presence of widespread clouds preferentially on the planet’s nightside with cloud optical thicknesses sufficient to suppress thermal emission and cool the thermal photosphere. Specifically, models with integrated mid-infrared cloud opacities of roughly 2–4 above the 300 mbar level (that is, blocking ~87–98% of the underlying emission), best match the observed nightside flux.
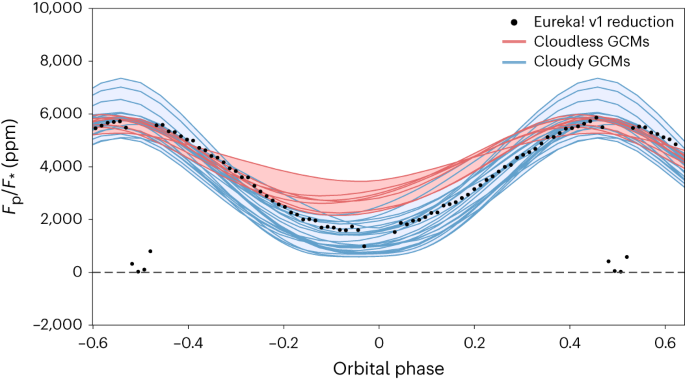
The black points show the temporally binned broadband light curve. The solid lines represent modelled phase curves derived from the 31 GCM simulations, integrated over the same wavelength range as the data, and separated into two groups based on the inclusion of clouds. The cloudless GCMs (red lines) simulated completely cloud-free skies, whereas the cloudy GCMs (blue lines) included at least some clouds on the nightside of the planet. The red and blue shaded areas span the range of all the cloudless and cloudy simulations, respectively, with the spread of values owing to differences in the various model assumptions and parameterizations. On average, the cloudless GCM phase curves have a maximum planet-to-star flux ratio of 5,703 ppm and a minimum of 2,681 ppm. This matches the observed maximum of the phase curve well but does not match its observed minimum at 1,636 ± 37 ppm. On average, the cloudy GCM phase curves have a maximum of 5,866 ppm and a minimum of 1,201 ppm, in better agreement with the observed nightside emission, but their spread of maximum values is much larger than the cloudless simulations. The cloudy models are able to suppress the nightside emission and better match the data; however, not all cloud models fit equally well and those with the optically thickest nightside clouds suppress too much emission. The models do not include the eclipse signals (phases −0.5 and 0.5) or transit signal (phase 0.0).
Including nightside clouds also improved the agreement with the measured hotspot offset (7.34± 0.38° E). While cloudless models all produced eastward offsets greater than 16.6° (25.5° on average), simulations with clouds had offsets as low as 7.6° (with a mean of 16.4°). These reduced offsets were associated with decreases in the eastwards jet speeds of up to several kilometres per second, with maximum winds of roughly 2.0–2.5 km s −1 providing the best match (see Extended Data Table 1 for further details). This modelled jet-speed reduction is probably due to a disruption in the equatorwards momentum transport 34 brought about by nightside clouds 4 , 35 , 36 . However, the resulting range of offsets seen in the suite of models suggests that this mechanism is quite sensitive to the details of cloud models, and other modelling factors (for example, atmospheric drag 11 , 12 , 16 , radiative timescales 14 , 15 , 37 ) probably still play an important role.
A comparison of the observed and modelled emission spectra further suggests that the majority of the cloud thermal opacity must be confined to pressures greater than ~10–100 mbar, because the presence of substantial cloud opacity at lower pressures dampens the modelled spectral signature amplitude below what is observed (Fig. 3 ). No distinct spectral signatures indicative of the cloud composition were evident in the observations. While no single GCM can match the emission spectra at all phases, spectra corresponding to nightside, morning and evening terminators appear qualitatively similar to GCM results that are intermediate between clear and cloudy simulations. In contrast, the absorption features indicative of water vapour (between ~5 μm and 8.5 μm) seen in the dayside emission spectrum are more consistent with an absence of cloud opacity at these mid-infrared wavelengths. Altogether, these findings represent new constraints on the spatial distribution and opacity of WASP-43b’s clouds.
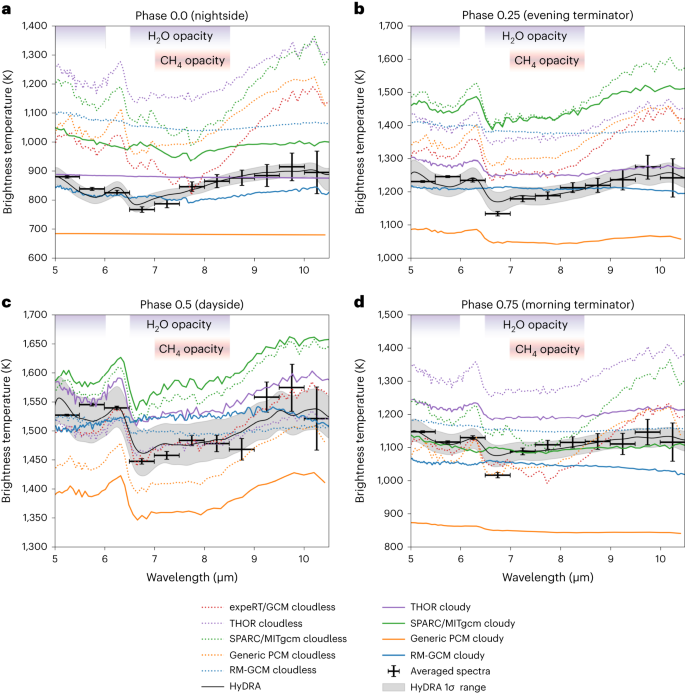
a – c , The observed emission spectrum with 1 σ error bars at phases 0.0 ( a ), 0.25 ( b ), 0.5 ( c ) and 0.75 ( d ), along with select modelled spectra derived from different cloudy and cloudless GCMs (described in Methods and listed in Extended Data Table 1 ). Although absolute brightness temperatures differ appreciably between models owing to various GCM assumptions, differences in the relative shape of the spectra are strongly dependent on the cloud and temperature structure found in the GCMs (Extended Data Fig. 7 ). Models with more isothermal profiles (like RM-GCM) or thick clouds at pressures of ≲ 10–100 mbar (like THOR cloudy, Generic PCM with 0.1 μm cloud particles) produce flatter spectra, while clearer skies yield stronger absorption features. The observed spectra from the nightside and terminators appear muted compared with the clear-model spectra, suggesting the presence of at least some clouds or weak vertical temperature gradients at pressures of ≲ 10–100 mbar. In contrast, the spectral structure produced by water vapour opacity (indicated by the purple shading) appears more consistent with models lacking clouds at these low pressures on the dayside. Under equilibrium chemistry, methane would also show an absorption feature at ~7.5–8.5 μm (shaded pink) for the colder models at phases 0.0 and 0.75. Finally, the median retrieved spectrum and 1 σ contours from the HyDRA retrieval are shown in grey.
We further characterized the chemical composition of WASP-43b’s atmosphere by applying a suite of atmospheric retrieval frameworks to the phase-resolved emission spectra. The retrievals spanned a broad range of model assumptions, including free chemical abundances versus equilibrium chemistry, different temperature profile parameterizations and different cloud models (see ‘Atmospheric retrieval models’ in Methods ). Despite these differences, the retrievals yielded consistent results for both the chemical and thermal constraints. We detected water vapour across the dayside, nightside, morning and evening hemispheres, with detection significances of up to ~3–4 σ (Extended Data Fig. 3 and Extended Data Tables 2 and 3 ). The retrieved abundances of H 2 O largely lie in the 10–10 5 ppm range for all four phases and for all the retrieval frameworks (Fig. 4 and Extended Data Fig. 4 ), broadly consistent with the value expected for a solar composition (500 ppm) as well as previous observations 22 .
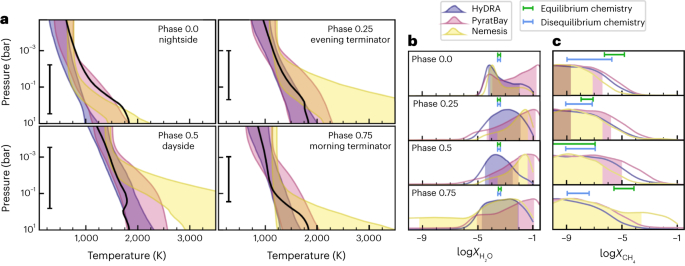
a , Temperature profile contours (68% confidence) constrained by the retrievals at each orbital phase (see legends). All frameworks produced consistent non-inverted thermal profiles that are consistent with two-dimensional radiative–convective equilibrium and photochemical models along the equator 23 (black curves) over the range of pressures probed by the observations (black bars). b , H 2 O abundance posterior distributions (volume mixing ratios). The shaded areas denote the span of the 68% confidence intervals. The green and blue bars on each panel denote the abundances predicted by equilibrium and disequilibrium chemistry solar-abundance models 23 , respectively, at the pressures probed by the observations (1–10 −3 bar, approximately). c , The same as in b but for CH 4 . The retrieved water abundances are consistent with either equilibrium or disequilibrium chemistry estimations for solar composition (500 ppm), whereas the retrieved upper limits to the CH 4 abundance are more consistent with disequilibrium chemistry predictions.
We also searched for signatures of disequilibrium chemistry in the atmosphere of WASP-43b. While CH 4 is expected to be present on the nightside under thermochemical equilibrium conditions, we did not detect CH 4 at any phase (Fig. 4 ). In the pressure range probed by the nightside spectrum (1–10 −3 bar; Extended Data Fig. 5 ), the equilibrium abundance of CH 4 is expected to vary between ~1 ppm and 100 ppm for a solar C/O ratio 23 , compared with our 95% upper limits of 1–6 ppm (Extended Data Table 2 ). The upper limits we place on the nightside CH 4 abundance are more consistent with disequilibrium models that account for vertical and horizontal transport 23 , 24 , 38 . In particular, two-dimensional photochemical models and GCMs predict the strongest depletion of CH 4 on the nightside due to strong zonal winds (>1 km s −1 ) transporting gas-phase constituents around the planet faster than the chemical reactions can maintain thermochemical equilibrium, thus ‘quenching’ and homogenizing the global composition at values more representative of dayside conditions (see also refs. 39 , 40 , 41 , 42 ). We note, however, that a low atmospheric C/O ratio and/or clouds at photospheric pressures could also lead to a non-detection of CH 4 . We also searched for signatures of NH 3 , which is predicted to have a volume mixing ratio less than 0.1–1 ppm in both equilibrium and disequilibrium chemistry models, and find that the results are inconclusive and model-dependent with the current retrieval frameworks.
Given the strong evidence for clouds from comparison with GCMs, we also searched for signatures of clouds in the atmospheric retrieval. Formally, the retrievals do not detect clouds with statistical significance, indicating that strong spectral features uniquely attributable to condensates are not visible in the data (see ‘Atmospheric retrieval models’ in Methods and Extended Data Fig. 6 ). However, the retrievals may mimic the effects of cloud opacity with a more isothermal temperature profile, as both tend to decrease the amplitude of spectral features, but the cloud-free, more isothermal temperature profile requires fewer free parameters and is therefore statistically favoured. Indeed, while the retrieved temperature profiles on the dayside and evening hemispheres agree well with the hemispherically averaged temperature profiles from the GCMs, they are more isothermal than the GCM predictions for the nightside and morning hemispheres (Extended Data Fig. 7 ). This discrepancy may hint at the presence of clouds on the nightside and morning hemispheres, consistent with the locations of clouds found in the GCMs.
Taken together, our results highlight the unique capabilities of JWST/MIRI for exoplanet atmosphere characterization. Combined with a range of atmospheric models, the observed phase curve and emission spectra provide strong evidence that the atmospheric chemistry of WASP-43b is shaped by complex disequilibrium processes and provide new constraints on the optical thickness and pressure of nightside clouds. However, while cloudy GCM predictions match the data better than cloud-free models, none of the simulations simultaneously reproduced the observed phase curve and spectra within measured uncertainties. These remaining discrepancies underscore the importance of further exploring the effects of clouds and disequilibrium chemistry in numerical models, as JWST continues to place unprecedented observational constraints on smaller and cooler planets.
Observations and quality of the data
We observed a full orbit of WASP-43b with the JWST MIRI LRS slitless mode as a part of JWST-ERS-1366. We performed target acquisition with the F1500W filter and used the SLITLESSPRISM subarray for the science observation. The science observation was taken between 1 December 2022 at 00:54:30 UT and 2 December 2022 at 03:23:36 UT, for a total of 26.5 h. We acquired 9,216 integrations, which were split into 3 exposures and 10 segments per exposure. Each integration lasts 10.34 s and is composed of 64 groups, with 1 frame per group. The LRS slitless mode reads an array of 416 × 72 pixels on the detector (the SLITLESSPRISM subarray) and uses the FASTR1 readout mode, which introduces an additional reset between integrations.
Owing to the long duration of the observation, two high-gain antenna moves occurred 8.828 h and 17.661 h after the start of the science observation. They affect only a couple of integrations that we removed from the light curves. A cross-shaped artefact is present on the two-dimensional images at the short-wavelength end due to light scattered by detector pixels 43 . It is stable over the duration of the observation but it contaminates the background and the spectral trace up to ~6 μm. This ‘cruciform’ artefact is observed in all MIRI LRS observations; a dedicated analysis is underway to estimate and mitigate its impact.
In the broadband light curve, the flux decays by ~0.1% during the first 60 min and continues to decay throughout the observation. This ramp is well modelled with 1 or 2 exponential functions after trimming the initial ~780 integrations. Without trimming any data, at least two ramps are needed. In addition, a downwards linear trend in flux is observed over the whole observation with a slope of −39 ppm per hour. These two types of drift also appear in the spectroscopic light curves. The exponential ramp amplitude in the first 60 min changes with wavelength from −0.67% in the 5–5.5 μm bin (downwards ramp) to +0.26% in the 10–10.5 μm bin (upwards ramp). The ramp becomes upwards at wavelengths longer than 7.5 μm and its timescale increases to more than 1 h at wavelengths longer than 10.5 μm. The slopes as a function of wavelength vary from −16 ppm to −52 ppm, all downwards. Such drifts (initial ramp and linear or polynomial trend) are also observed in other MIRI LRS time-series observations 29 but the strength of the trends differ for each observation. In these WASP-43b observations, we note that their characteristic parameters vary smoothly with wavelength, which may help identify their cause and build correction functions.
Over the course of the observation, the position of the spectral trace on the detector varies by 0.0036 pixels RMS (0.027 pixels peak to peak) in the spatial direction, and the Gaussian standard deviation of the spatial PSF varies by 0.00069 pixels RMS (root mean square; 0.0084 pixels peak to peak) following a sharp increase by 0.022 pixels during the first 600 integrations. Depending on the wavelength bin, that spatial drift causes noise at the level of 7–156 ppm, while variations in the PSF width cause noise at the level of 4–54 ppm (these numbers are obtained from a linear decorrelation). Overall, the MIRI instrument used in LRS slitless mode remains remarkably stable over this 26.5-h-long continuous observation and the data are of exquisite quality.
The noise in the light curve increases sharply at wavelengths beyond 10.5 μm and the transit depths obtained at these long wavelengths by different reduction pipelines are discrepant. These wavelengths were not used in the retrieval analyses and the final broadband light curve. The cause is unknown but it might be related to the fact that this region of the detector receives different illumination before the observation 44 (see ‘Shadowed region effect’ below for more details).
Data reduction pipelines
Eureka v1 reduction.
The Eureka! v1 reduction made use of version 0.9 of the Eureka! pipeline 45 , CRDS version 11.16.16 and context 1018, and jwst package version 1.8.3 46 . The gain value of 5.5 electrons per data number obtained from these CRDS reference files is known to be incorrect, and the actual gain is estimated to be ~3.1 electrons per data number although the gain may be wavelength dependent (S. Kendrew, private communication). A new reference file reflecting the updated gain is under development at STScI, which will improve the accuracy of photon-noise calculations. For the rest of this analysis, we assume a constant gain of 3.1 electrons per data number. The Eureka! control files and Eureka! parameter files files used in these analyses are available for download ( https://doi.org/10.5281/zenodo.10525170 ) and are summarized below.
Eureka! makes use of the jwst pipeline for stages 1 and 2, and both stages were run with their default settings, with the exception of increasing the stage 1 jump step’s rejection threshold to 8.0 and skipping the photom step in stage 2 because it is not necessary and can introduce additional noise for relative time-series observations. In stage 3 of Eureka!, we then rotated the MIRI/LRS slitless spectra 90° anticlockwise so that wavelength increases from left to right like the other JWST instruments to allow for easier reuse of Eureka! functions. We then extracted pixels 11–61 in the new y direction (the spatial direction) and 140–393 in the new x direction (spectral direction); pixels outside of these ranges primarily contain noise that is not useful for our reduction. Pixels marked as ‘DO_NOT_USE’ in the DQ array were then masked as were any other unflagged NaN or inf pixels. A centroid was then fit to each integration by summing along the spectral direction and fitting the resulting one-dimensional profile with a Gaussian function; the centroid from the first integration was used for determining aperture locations, while the centroids and PSF widths from all integrations were saved to be used as covariates when fitting the observations.
Our background subtraction method is tailored to mitigate several systematic effects unique to the MIRI instrument. First, MIRI/LRS observations exhibit a ‘cruciform artefact’ 43 at short wavelengths caused by scattered light within the optics; this causes bright rays of scattered light which must be sigma-clipped to avoid over-subtracting the background. In addition, MIRI/LRS observations show periodic noise in the background flux, which drifts with time 29 as well as 1/ f noise 47 , which leads to correlated noise in the cross-dispersion direction; as a result, background subtraction must be performed independently for each integration and column (row in MIRI’s rotated reference frame). Furthermore, in both these observations and the dedicated background calibration observations from JWST-COM/MIRI-1053, we found that there was a linear trend in the background flux, with the background flux increasing with increasing row index (column index in MIRI’s rotated reference frame). To robustly remove this feature, we found that it was important to either (1) use the mean from an equal number of pixels on either side of the spectral trace for each column and integration, or (2) use a linear background model for each column and integration; we adopted the former as it resulted in less noisy light curves. To summarize, for each column in each integration we subtracted the mean of the pixels separated by ≥11 pixels from the centre of the spectral trace after first masking 5 σ outliers in that column.
To compute the spatial profile for the optimal extraction of the source flux, we calculated a median frame, sigma-clipping 5 σ outliers along the time axis and smoothing along the spectral direction using a 7-pixel-wide boxcar filter. During optimal extraction, we only used the pixels within 5 pixels of the fitted centroid and masked pixels that were 10 σ discrepant with the spatial profile. Background exclusion regions ranging from 9 to 13 pixels and source aperture regions ranging from 4 to 6 pixels were considered, but our values of 11 and 5 pixels were selected as they produced the lowest median absolute deviation light curves before fitting.
Eureka! v2 reduction
The Eureka! v2 reduction followed the same procedure as the Eureka! v1 reduction except for the following differences. First, this reduction made use of version 1.8.1 of the jwst pipeline. For stage 1, we instead used a cosmic ray detection threshold of 5 and used a uniform ramp fitting weighting. For stage 2, we performed background subtraction using columns away from the trace on the left and on the right and subtracted the background for each integration 29 . Stage 3 was identical to Eureka! v1 reduction.
TEATRO reduction
We processed the data using the Transiting Exoplanet Atmosphere Tool for Reduction of Observations (TEATRO) that runs the jwst package, extracts and cleans the stellar spectra and light curves, and runs light-curve fits. We used the jwst package version 1.8.4, CRDS version 11.16.14 and context 1019. We started from the ‘uncal’ files and ran stages 1 and 2 of the pipeline. For stage 1, we set a jump rejection threshold of 6, turned off the ‘jump.flag_4_neighbors’ parameter and used the default values for all other parameters. For stage 2, we ran only the ‘AssignWcsStep’, ‘FlatFieldStep’ and ‘SourceTypeStep’; no photometric calibration was applied. The next steps were made using our own routines. We computed the background using two rectangular regions, one on each side of the spectral trace, between pixels 13 and 27 and between pixels 53 and 72 in the spatial direction, respectively. We computed the background value for each row (rows are along the spatial direction) in each region using a biweight location, averaged the two values and subtracted it from the full row. This background subtraction was done for each integration. Then, we extracted the stellar flux using aperture photometry by averaging pixels between 33 and 42 in each row to obtain the stellar spectrum at each integration. We also averaged pixels between 33 and 42 in the spatial direction and between 5 μm and 10.5 μm in the spectral direction to obtain the broadband flux. We averaged the spectra in 11 0.5-μm-wide wavelength channels. For each channel and for the broadband light curve, we normalized the light curve using the second eclipse as a reference flux, computed a running median filter using a 100-point window size, and rejected points that were more than 3 σ away from that median using a 5-iteration sigma-clipping. To limit the impact of the initial ramp on the fitting, we trim the first 779 integrations from the broadband light curve and a similar number of integrations for each channel (the exact number depends on the channel). Finally, we subtracted 1 from the normalized light curves to have the secondary eclipse flux centred on 0. These cleaned light curves were used for phase curve, eclipse and transit fits.
SPARTA reduction
We reduced the data with the open-source Simple Planetary Atmosphere Reduction Tool for Anyone (SPARTA), first introduced in ref. 48 to analyse the MIRI LRS phase curve of GJ 1214b. We started from the uncalibrated data and proceeded all the way to the final results without using any code from the jwst or Eureka! pipelines. In stage 1, we started by discarding the first five groups as well as the last group, because these groups show anomalies due to the reset switch charge decay and the last-frame effect. We fitted a slope to the up-the-ramp reads in every pixel of every integration in every exposure. We calculated the residuals of these linear fits, and for every pixel, we computed a median residual for every group across all integrations. This ‘median residual’ array has dimensions N grp × N rows × N cols . This array was subtracted from the original uncalibrated data and the up-the-ramp fit was redone, this time without discarding any groups except those that were more than 5 σ away from the best-fit line. Such outliers, which may be due to cosmic rays, were discarded and the fit recomputed until convergence. This procedure straightens out any nonlinearity in the up-the-ramp reads that is consistent across integrations, such as the reset switch charge decay, the last-frame effect or inaccuracies in the nonlinearity coefficients. After up-the-ramp fitting, we removed the background by removing the mean of columns 10–24 and 47–61 (inclusive, zero-indexed) for every row of every integration. As these two regions are of equal size and equally distant from the trace, any linear spatial trend in the background is naturally removed.
In the next step, we computed a pixel-wise median image over all integrations. This median image was used as a template to determine the position of the trace in each integration, by shifting and scaling the template until it matched the integration (and minimizes the χ 2 ). It was also used as the point spread profile for optimal extraction, after shifting in the spatial direction by the amount calculated in the previous step. Outliers more than 5 σ discrepant from the model image (which may be cosmic rays) were masked, and the optimal extraction was repeated until convergence. The z -scores image (image minus model image all divided by expected error, including photon noise and read noise) have a typical standard deviation of 0.88, compared with a theoretical minimum value of 1, indicating that the errors are being overestimated.
After optimal extraction, we gathered all the spectra and positions into one file. To reject outliers, we created a broadband light curve, detrended it by subtracting a median filter with a width 100 times less than the total data length and rejected integrations greater than 4 σ away from 0 (which may be cosmic rays). Sometimes only certain wavelengths of an integration are bad, not the entire integration. We repaired these by detrending the light curve at each wavelength, identifying 4 σ outliers and replacing them with the average of their two immediate temporal neighbours.
Spectral binning
To investigate the effects of spectral binning, we utilized the MIRI time-series commissioning observations of the transit of L168-9b (JWST-COM/MIRI-1033; ref. 29 ). L168-9b was chosen to have a clear transit signal while also having no detectable atmospheric signatures expected in its mid-infrared transmission spectrum; as a result, the observed scatter in the transmission spectrum can be used as an independent measurement of the uncertainties in the transit depths. The same procedure cannot be done on our WASP-43b science observations as there may be detectable atmospheric signatures.
Following the Eureka! reduction methods described by ref. 29 , we tried binning the L168-9b spectroscopic light curves at different resolutions and compared the observed standard deviation of the transmission spectrum with the median of the transit depth uncertainties estimated from fitting the spectral light curves. As shown in Extended Data Fig. 1 , the uncertainties in the pixel-level light curves underestimate the scatter in the transmission spectrum by a factor of about two. Because pairs of rows (in MIRI’s rotated reference frame) are reset together, it is reasonable to assume that there could be odd–even effects that would average out if combining pairs of rows; indeed, there do appear to be differences in the amplitude of the initial exponential ramp feature between odd and even rows. However, combining pairs of rows still leads to appreciable underestimation of the scatter in the transmission spectrum. Interestingly, the underestimation of the uncertainties appears to decrease with decreasing wavelength resolution. This is likely explained by wavelength-correlated noise that gets averaged out with coarse binning. A likely culprit for this wavelength-correlated noise may be the 390 Hz periodic noise observed in several MIRI subarrays, which causes clearly structured noise with a period of ~9 rows 29 (M. Ressler, private communication); this noise source is believed to be caused by MIRI’s electronics and possible mitigation strategies are still under investigation. Until the source of the excess wavelength-correlated noise is definitively determined and a noise mitigation method is developed, we recommend that MIRI/LRS observations should be binned to a fairly coarse spectral resolution as this gives better estimates of the uncertainties and also gives spectra that are closer to the photon-limited noise regime. However, we caution against quantitative extrapolations of the uncertainty underestimation to other datasets; because we do not know the source of the excess noise, we do not know how it might change with different parameters such as groups per integration or stellar magnitude.
Ultimately, for each reduction method, we binned the spectra down to a constant 0.50-μm-wavelength grid spanning 5–12 μm, giving a total of 14 spectral channels. However, as is described below, we only end up using the 11 spectral channels spanning 5–10.5 μm for science. This 0.5-μm-binning scheme combines 7 wavelengths for the shortest bin and 25 wavelengths for the longest bin, which has the added benefit of binning down the noise at longer wavelengths where there are fewer photons. However, even for this coarse of a binning scheme, we do expect there to be some additional noise beyond our estimated uncertainties on the spectrum of WASP-43b (Extended Data Fig. 1 ). As the structure of this noise source is not well understood nor is the extent to which our error bars are underestimated, our best course of action was to consider error inflation when performing spectroscopic inferences (described in more detail below).
Light-curve fitting
Detrending the initial exponential ramp.
As with other MIRI/LRS observations 29 , our spectroscopic light curves showed a strong exponential ramp at the start of the observations. As shown in Extended Data Fig. 2 , the strength and sign of the ramp varies with wavelength, changing from a strong downwards ramp at 5 μm to a nearly flat trend around 8 μm, and then becoming an upwards ramp towards longer wavelengths. From 10.6 μm to 11.8 μm, the ramp timescale became much longer and the amplitude of the ramp became much stronger; this region of the detector was previously discussed 44 and is discussed in more detail below. In general, most of the ramp’s strength had decayed within ~30–60 min, but at the precision of our data, the residual ramp signature still had an important impact on our nightside flux measurements due to the similarity of the ramp timescale with the orbital period. Unlike in the case of the MIRI/LRS commissioning observations of L168-9b 29 , we were not able to safely fit the entire dataset with a small number of exponential ramps. When fitting the entire dataset, we found that non-trivial choices about the priors for the ramp amplitudes and timescales resulted in significantly different spectra at phases 0.75 (morning hemisphere) and especially 0.0 (nightside); because the dayside spectrum is measured again near the end of the observations, it was less affected by this systematic noise.
Ultimately, we decided to conservatively discard the first 779 integrations (134.2 min), leaving only one transit duration of baseline before the first eclipse ingress began. After removing the initial 779 integrations, we found that a single exponential ramp model with broad priors that varied freely with wavelength was adequate to remove the signature. In particular, after removing the first 779 integrations we found that our dayside and nightside emission spectra were not significantly affected by (1) fitting two exponential ramps instead of one, (2) adjusting our priors on the ramp timescale to exclude rapidly decaying ramps with timescales >15 d −1 instead of >100 d −1 , (3) putting a uniform prior on the inverse timescale instead of the timescale, or (4) altering the functional form of the ramp by fitting for an exponential to which the time was raised. After removing the first ~2 h, we also found that the ramp amplitude and timescale did not vary strongly with wavelength (excluding the ‘shadowed region’ described below), although fixing these parameters to those fitted to the broadband light curve affected several points in the nightside spectrum by more than 1 σ ; we ultimately decided to leave the timescale and amplitude to vary freely with wavelength as there is no a priori reason to assume that they should be equal. With careful crafting of priors, it appeared possible to get results similar to our final spectra while removing only the first few integrations, but trimming more integrations and only using a single exponential ramp model required fewer carefully tuned prior assumptions for which we have little physical motivation.
Shadowed region effect
As was described in ref. 44 , we also identified a strong discontinuity in the spectroscopic light curves spanning pixel rows 156–220 (10.6–11.8 μm) in these observations. In this range, the temporal behaviour of the detector abruptly changes to a large-amplitude, long-timescale, upwards ramp that appears to slightly overshoot before decaying back down and approaching an equilibrium. These pixels coincide with a region of the detector between the Lyot coronagraph region and the four-quadrant phase mask region, which is unilluminated except when the dispersive element is in the optical path; as a result, we have taken to calling this unusual behaviour as the ‘shadowed region effect’. Strangely, not all MIRI/LRS observations show this behaviour, with the MIRI/LRS commissioning time-series observations 29 and the GJ 1214b phase-curve observations 48 showing no such effect. In fact, we know of only two other observations that show similar behaviour: the observation of the transit of WASP-80b (JWST-GTO-1177; T. Bell, private communication) and the observation of the phase curve of GJ 367b (JWST-GO-2508; M. Zhang, private communication). Informatively, the eclipse observation of WASP-80b taken 36 h after the WASP-80b transit using the same observing procedure (JWST-GTO-1177; T. Bell, private communication) did not show the same shadowed region effect, indicating that the effect is unlikely to be caused by stray light from nearby stars or any other factors that stayed the same between those two observations. Our best guess at this point is that the effect is related to the illumination history of the detector and the filter used by the previous MIRI observation (because the detector is illuminated at all times, even when it is not in use), but this is still under investigation and at present there is no way of predicting whether or not an observation will be impacted by the shadowed region effect. It is important to note, however, that from our limited knowledge at present that the shadowed region effect appears to be either present or not, with observations either strongly affected or seemingly completely unaffected.
Using the general methods described in the Eureka! v1 fit, we attempted to model the shadowed region effect with a combination of different ramp models, but nothing we tried was able to cleanly separate the effect from the phase variations, and there was always some clear structure left behind in the residuals of the fit. Another diagnostic that our detrending attempts were unsuccessful was that the phase offset as a function of wavelength smoothly varied around ~10° E in the unaffected region of the detector, but in the shadowed region, the phase offset would abruptly change to ~5° W; such a sharp change in a suspect region of the detector seems highly unlikely to be astrophysical in nature. As a result, we ultimately chose to exclude the three spectral bins spanning 10.5–12 μm from our retrieval efforts.
Determining the number of sinusoid harmonics
To determine the complexity of the phase-curve model required to fit the data, we used the Eureka! v1 reduction and most of the Eureka! v1 fitting methods described below, with the exception of using the dynesty 49 nested sampling algorithm (which computes the Bayesian evidence, \({{{\mathcal{Z}}}}\) ) and a batman transit and eclipse model. Within dynesty, we used 256 live points, ‘multi’ bounds, ‘rwalk’ sampling, and ran until the estimated \(d\ln ({{{\mathcal{Z}}}})\) reached 0.1. We then evaluated first-, second- and fourth-order models for the broadband light curve, excluding all third-order sinusoidal terms from the fourth-order model as these terms are not likely to be produced by the planet’s thermal radiation 50 , 51 . We then compared the Bayesian evidences of the different models following refs. 52 , 53 and found that the second-order model was significantly preferred over the first-order model at 16 σ ( \({{\Delta }}\ln ({{{\mathcal{Z}}}})=128\) ), while the second-order model was insignificantly preferred over the fourth-order model at 2.2 σ ( \({{\Delta }}\ln ({{{\mathcal{Z}}}})=1.3\) ). This is also confirmed by eye where the first-order model leaves clear phase-variation signatures in the residuals, while the residuals from the second-order model leave no noticeable phase variations behind. Finally, we also compared the phase-resolved spectra obtained from different order phase-curve models; we found that our spectra significantly changed going from a first- to second-order model (altering one or more spectral points by >1 σ ), but the fourth-order model did not significantly change the resulting phase-resolved spectra compared with the second-order. As a result, the final fits from all reductions used a second-order model. The broadband light curves obtained from the four reductions and the associated phase-curve models are shown in Supplementary Fig. 1 .
Eureka! v1 fitting methods
We first sigma-clipped any data points that were 4 σ discrepant from a smoothed version of the data (made using a boxcar filter with a width of 20 integrations) to remove any obviously errant data points while preserving the astrophysical signals like the transit.
Our astrophysical model consisted of a starry 54 transit and eclipse model, as well as a second-order sinusoidal phase-variation model. The complete astrophysical model had the form
where t is the time, F * is the received stellar flux (and includes the starry transit model), F day is the planetary flux at mid-eclipse, E ( t ) is the starry eclipse model (neglecting eclipse mapping signals for the purposes of this paper), and Ψ ( ϕ ) is the phase-variation model of the form
where ϕ is the orbital phase in radians with respect to eclipse, and AmpCos1, AmpSin1, AmpCos2 and AmpSin2 are all fitted coefficients. The second-order phase-variation terms allow for thermal variations across the face of the planet that are more gradual or steep than a simple first-order sinusoid would allow. We numerically computed dayside, morning, nightside and evening spectra using the above Ψ ( ϕ ) function at ϕ = 0, π/2, π and 3π/2, respectively. To allow the starry eclipse function to account for light travel time, we used a stellar radius ( R * ) of 0.667 R ⊙ (ref. 55 ) to convert the fitted a / R * (the scaled semi-major axis) to physical units. For our transit model, we used a reparameterized version of the quadratic limb-darkening model 56 with coefficients u 1 and u 2 uniformly constrained between 0 and 1, and used a minimally informative prior on the planet-to-star radius ratio ( R p / R * ).
Our systematics model consisted of a single exponential ramp in time to account for the idle-recovery drift documented for MIRI/LRS time-series observations 29 , a linear trend in time, and a linear trend with the spatial position and PSF width. The full systematics model can be written as
The linear trend in time is modelled as
where t l is the time with respect to the mid-point of the observations and where c 0 and c 1 are coefficients. The exponential ramp is modelled as
where t r is the time with respect to the first integration and where r 0 and r 1 are coefficients. The linear trends as a function of spatial position, y , are PSF width s y are modelled as
where f and g are coefficients. The linear trends as a function of spatial position and PSF width are with respect to the mean-subtracted spatial position and PSF width. Finally, we also fitted a multiplier (scatter mult ) to the estimated Poisson noise level for each integration to allow us to account for any noise above the photon limit as well as an incorrect value for the gain applied in stage 3.
With an initial fit to the broadband light curve (5–10.5 μm), we assumed a zero eccentricity and placed a Gaussian prior on the planet’s orbital parameters (period, P ; linear ephemeris, t 0 ; inclination, i ; and scaled semi-major axis, a / R * ) based on previously published values for the planet 30 . For the fits to the spectroscopic phase curves, we then fixed these orbital parameters to the estimated best fit from the broadband light curve fit to avoid variations in these wavelength-independent values causing spurious features in the final spectra. We fitted the observations using the No U-Turns Sampler (NUTS) from PyMC3 57 with 3 chains, each taking 6,000 tuning steps and 6,000 production draws with a target acceptance rate of 0.95. We used the Gelman–Rubin statistic 58 to ensure the chains had converged. We then used the 16th, 50th and 84th percentiles from the PyMC3 samples to estimate the best-fit values and their uncertainties.
Eureka! v2 fitting methods
For the second fit made with Eureka!, we proceeded very similarly to the Eureka! v1 fit. We clipped the light curves using a boxcar filter of 20 integrations wide with a maximum of 20 iterations and a rejection threshold of 4 σ to reject these outliers. We also modelled the phase curve using a second-order sinusoidal function, but we modelled the transit and eclipse using batman 59 instead of starry. Like in the Eureka! v1 fit, we modelled instrumental systematics with a linear polynomial model in time (equation ( 4 )), an exponential ramp (equation ( 5 )), a first-order polynomial in y position (equation ( 6 )) and a first-order polynomial in PSF width in the s y direction (equation ( 7 )).
We fitted the data using the emcee sampler 60 instead of NUTS, with 500 walkers and 1,500 steps. The jump parameters that we used were the same as in the Eureka! v1 fit: R p / R * , F day , u 1 , u 2 , AmpCos1, AmpSin1, AmpCos2, AmpSin2, c 0 , c 1 , r 0 , r 1 , f , g and scatter mult (multiplier to the estimated Poisson noise level for each integration like in the Eureka! v1 fit). We used uniform priors on u 1 and u 2 from 0 to 1, uniform priors on AmpCos1, AmpSin1, AmpCos2, AmpSin2 from −1.5 to 1.5 and broad normal priors and all other jump parameters. Convergence, mean values and uncertainties were computed in the same way as for the Eureka! v1 fit.
TEATRO fitting methods
To measure the planet’s emission as a function of longitude, we modelled the light curves with a phase-variation model, an eclipse model, a transit model and an instrument systematics model. The phase-curve model, Ψ ( t ), consists of two sinusoids: one at the planet’s orbital period, P , and one at P /2 to account for second-order variations. The eclipse model, E ( t ), and transit model, T ( t ), are computed with the exoplanet 61 package that uses the starry package 54 . We save the eclipse depth, δ e , and normalize E ( t ) to a value of 0 during the eclipse and 1 out of the eclipse, which we then call E N ( t ). We used published transit ephemerides 62 , a null eccentricity and published stellar parameters 63 . The planet-to-star radius ratio, R p / R * , impact parameter, b , and mid-transit time, t 0 , are obtained from a fit to the broadband light curve. The systematics model, S ( t ), is composed of a linear function to account for a downwards trend and an exponential function to account for the initial ramp. The full model is expressed as:
where Ψ ( t e ) is the value of Ψ at the mid-eclipse time, t e .
We fit our model to the data using a Markov chain Monte Carlo (MCMC) procedure based on the PyMC3 package 57 and gradient-based inference methods as implemented in the exoplanet package 61 . We set normal priors for t 0 , R p / R * , the stellar density ( ρ * ), a Ψ , b Ψ , c S and d S with mean values obtained from an initial nonlinear least-squares fit, a normal prior for a S with a zero mean, uniform priors for the surface brightness ratio between the planet’s dayside and the star ( s ), b , c Ψ and d Ψ , uninformative priors for the quadratic limb-darkening parameters 56 , and allowed for wide search ranges. We ran two MCMC chains with 5,000 tuning steps and 100,000 posterior samples. Convergence was obtained for all parameters (except in one case where a S was negligible and b S was unconstrained). We merged the posterior distributions of both chains and used their median and standard deviation to infer final values and uncertainties for the parameters. We also verified that the values obtained from each chain were consistent.
For the spectroscopic light-curve fits, we fixed all physical parameters to those obtained from the broadband light-curve fit except the surface brightness ratio, s , that sets the eclipse depth, we masked the transit part of the light curve, and used a similar procedure. After the fits, we calculated the eclipse depth, δ e , as s × ( R p / R * ) 2 , and computed Ψ ( t ) for the final parameters, Ψ f ( t ). The planetary flux is Ψ f ( t ) − Ψ f ( t e ) + δ e . We computed the uncertainty on the eclipse depth in two different ways: from the standard deviation of the posterior distribution of s × ( R p / R * ) 2 , and from the standard deviation of the in-eclipse points divided by \(\sqrt{{N}_{{\mathrm{e}}}}\) , where N e is the number of in-eclipse points, and took the maximum of the two. To estimate the uncertainty on the planet’s flux, we computed the 1 σ interval of Ψ ( t ) based on the posterior distributions of its parameters, computed the 1 σ uncertainty of d S , and added them in quadrature to the uncertainty on the eclipse depth to obtain more conservative uncertainties.
The spectra presented in this paper and used in the combined spectra are based on system parameters that were derived from a broadband light curve obtained in the 5–12 μm range, a transit fit in which the stellar mass and radius were fixed, a simpler additive model in which the phase curve was not turned off during the eclipse, and an MCMC run that consisted in two chains of 10,000 tuning steps and 10,000 posterior draws. Updated spectra based on system parameters derived from the broadband light curve obtained in the 5–10.5 μm range, a transit fit that has the stellar density as a free parameter, the light-curve model shown in equation ( 8 ), and two MCMC chains of 5,000 tuning steps and 100,000 posterior draws are consistent within 1 σ at every point with those shown here. As we average four reductions and inflate the uncertainties during the retrievals, the impact of these updates on our results are expected to be marginal.
SPARTA fitting methods
We use emcee 60 to fit a broadband light curve with the transit time, eclipse time, eclipse depth, four phase-curve parameters ( C 1 and D 1 for the first-order, and C 2 and D 2 for the second-order sinusoids), transit depth, a / R * , b , flux normalization, error-inflation factor, instrumental ramp amplitude ( A ) and 1/timescale ( τ ), linear slope in time ( m ) with respect to the mean of the integration times \((\overline{t})\) , and linear slope with trace y position ( c y ) as free parameters. The best-fit transit and eclipse times, a / R * and b are fixed for the spectroscopic light curves.
For the spectroscopic fits, we then use emcee to fit the free parameters: the eclipse depth, four phase-curve parameters, error-inflation factor, flux normalization, instrumental ramp amplitude and 1/timescale, linear slope with time, and linear slope with trace y position. All parameters are given uniform priors. 1/timescale is given a prior of 5–100 d −1 , but the other priors are unconstraining. In summary, the instrumental model is:
while the planetary flux model is:
where E is the eclipse depth and ω = 2π/ P is the planet’s orbital angular frequency. Note that the phase variations were set to be zero during eclipse.
Combining independent spectra
Comparing the phase-resolved spectra from each reduction (Supplementary Fig. 2 ), we see that for wavelengths below 10.5 μm, the spectra are typically consistent, while larger differences arise in the >10.5 μm region affected by the shadowed region effect. For our final, fiducial spectrum, we decided to use the median spectrum and inflated our uncertainties to account for disagreements between different reductions. The median phase-resolved spectra were computed by taking the median F p / F * per wavelength. The uncertainties were computed by taking the median uncertainty per wavelength, and then adding in quadrature the RMS between the individual reductions and the median spectrum; this minimally affects the uncertainties where there is minimal disagreement and appreciably increases the uncertainties where the larger disagreements arise.
Each reduction also computed a transmission spectrum (Supplementary Fig. 2 ), which appears quite flat (within uncertainties) with minimal differences between reductions. WASP-43b is not an excellent target for transmission spectroscopy, however, and these transmission spectra are not expected to be overly constraining.
Atmospheric forward models
GCMs were used to simulate atmospheric conditions, from which synthetic phase curves and emission spectra were forward modelled and compared with the observations. The GCMs used in this study are listed in Supplementary Table 1 , and details of each simulation are provided in Extended Data Table 1 and the following sections.
Generic PCM
The Generic Planetary Climate Model (Generic PCM) is a three-dimensional global climate model designed for modelling the atmosphere of exoplanets and for palaeoclimatic studies. The model has been used for the study of planetary atmospheres of the Solar System 64 , 65 , 66 , terrestrial exoplanets 67 , mini-Neptunes 68 and hot Jupiters 69 . The dynamical core solves the primitive equations of meteorology on a Arakawa C grid. The horizontal resolution is 64 × 48 (that is, 5.625 × 3.75°) with 40 vertical levels, equally spaced in logarithmic scale between 10 Pa and 800 bars. Along with the various parameterizations of physical processes described in refs. 64 , 65 , 66 , 67 , 68 , the Generic PCM treats clouds as radiatively active tracers of fixed radii.
The model is initialized using temperature profiles from the radiative–convective one-dimensional model Exo-REM 70 . The radiative data are computed offline using the out-of-equilibrium chemical profiles of the Exo-REM run. We use 27 frequency bins in the stellar channel (0.261–10.4 μm) and 26 in the planetary channel (0.625–324 μm), all bins including 16 k -coefficients. We start the model from a rest state (no winds), with a horizontally homogeneous temperature profile. Models are integrated for 2,000 days, which is long enough to complete the spin-up phase of the simulation above the photosphere. We do not include Rayleigh drag in our models. The simulations are performed including clouds of Mg 2 SiO 4 , with varying cloud radii (0.1, 0.5, 1, 3 and 5 μm). We also computed cloudless and Mg 2 SiO 4 models with a 10× solar metallicity and the same radii for the cloud particles. Regardless of the composition and size of the clouds, our model clearly indicates that there is no cloud formation on the dayside. Asymmetric limbs are a natural result of our model, with the eastern terminator being warmer while the western limb is cloudier and cooler. Spectral phase curves were produced using the Pytmosph3R code 71 .
SPARC/MITgcm with radiative transfer post-processing by gCMCRT
SPARC/MITgcm couples a state-of-the-art non-grey, radiative-transfer code with the MITgcm 33 . The MITgcm solves the primitive equations of dynamical meteorology on a cubed-sphere grid 72 . It is coupled to the non-grey radiative-transfer scheme based on the plane-parallel radiative-transfer code of ref. 73 . The stellar irradiation incident on WASP-43b is computed with a PHOENIX model 74 , 75 , 76 . We use previously published opacities 77 , including more recent updates 78 , 79 , and the molecular abundances are calculated assuming local chemical equilibrium 80 . In the GCM simulations, the radiative-transfer calculations are performed on 11 frequency bins ranging from 0.26 μm to 300 μm, with 8 k -coefficients per bin statistically representing the complex line-by-line opacities 3 . The strong visible absorbers TiO and VO are excluded in our k tables similar to our previous GCMs of WASP-43b 3 , 23 that best match the observed dayside emission spectrum and photometry.
Clouds in the GCM are modelled as tracers that are advected by the flow 81 and can settle under gravity. Their formation and evaporation are subjected to chemical equilibrium predictions, that is, the condensation curves of various minerals described in ref. 80 . The conversion between the condensable ‘vapour’ and clouds is treated as a simple linear relaxation over a short relaxation timescale of 100 s. The scattering and absorption of the spatial- and time-dependent clouds are included in both the thermal and visible wavelengths of the radiative transfer. A similar dynamics–cloud–radiative coupling has been developed in our previous GCMs with simplified radiative transfer and has been used to study the atmospheric dynamics of brown dwarfs 9 , 82 and ultrahot Jupiters 83 . Clouds are assumed to follow a log-normal size distribution 84 , which is described by the reference radius r 0 and a non-dimensional deviation σ : \(n(r)=\frac{{{{\mathcal{N}}}}}{\sqrt{2\uppi }\sigma r}\exp \left(-\frac{{[\ln (r/{r}_{0})]}^{2}}{2{\sigma }^{2}}\right)\) , where n ( r ) is the number density per radius bin of r and \({{{\mathcal{N}}}}\) is the total number density. σ and r 0 are free parameters and the local \({{{\mathcal{N}}}}\) is obtained from the local mass mixing ratio of clouds. The size distribution is held fixed throughout the model and is the same for all types of cloud.
Our GCMs do not explicitly impose a uniform radiative heat flux at the bottom boundary but rather relax the temperature of the lowest model layer (that is, the highest pressure layer) to a certain value over a short timescale of 100 s. This assumes that the deep GCM layer reaches the convective zone and the temperature there is set by the interior convection that ties to the interior structure of the planet. This lowest-layer temperature is in principle informed by internal structure models of WASP-43b, which are run by MESA hot Jupiter evolution modules 12 to match the present radius of WASP-43b. In most models, this lowest-layer temperature is about 2,509 K at about 510 bars. The horizontal resolution of our GCMs is typically C48, equivalent to about 1.88° per grid cell. The vertical domain is from 2 × 10 −4 bar at the top to 700 bars at the bottom and is discretized to 53 vertical layers. We typically run the simulation for over 1,200 days and average all physical quantities over the last 100 days of the simulations.
All our GCMs assume a solar composition. We performed a baseline cloudless model and one case with only MnS and Na 2 S clouds with r 0 = 3 μm, and then a few cases with MnS, Na 2 S and MgSiO 3 clouds with r 0 = 1, 1.5, 2 and 3 μm. The σ is held fixed at 0.5 in all our cloudy GCMs.
We post-process our GCM simulations with the state-of-the-art gCMCRT code, which is a publicly available hybrid Monte Carlo radiative transfer (MCRT) and ray-tracing radiative-transfer code. The model is described in detail in ref. 85 and has been applied to a range of exoplanet atmospheres 83 , 86 . gCMCRT can natively compute albedo, transmission and emission spectra at both low and high spectral resolution. gCMCRT uses custom k tables, which take cross-section data from both HELIOS-K 87 and EXOPLINES 88 . Here, we apply gCMCRT to compute low-resolution emission spectra and phase curves at R ≈ 300 from our GCM simulations. We use the three-dimensional temperature and condensate cloud tracer mixing ratio from the time-averaged end-state of each case. We assume the same cloud particle size distribution as our GCMs.
expeRT/MITgcm
The GCM expeRT/MITgcm uses the same dynamical core as SPARC/MITgcm and solves the hydrostatic primitive equations on a C32 cubed-sphere grid 72 . It resolves the atmosphere above 100 bar on 41 log-spaced cells between 1 × 10 −5 bar and 100 bar. Below 100 bar, 6 linearly spaced grid cells between 100 bar and 700 bar are added. The model expeRT/MITgcm thus resolves deep dynamics in non-inflated hot Jupiters like WASP-43b 16 , 89 .
The GCM is coupled to a non-grey radiative-transfer scheme based on petitRADTRANS 90 . Fluxes are recalculated every fourth dynamical time step. Stellar irradiation is described by the spectral fluxes from the PHOENIX model atmosphere suite 74 , 75 , 76 . The GCM operates on a precalculated grid of correlated k -binned opacities. Opacities from the ExoMol database 91 are precalculated offline on a grid of 1,000 logarithmically spaced temperature points between 100 K and 4,000 K for every vertical layer. We further include the same species as shown in ref. 89 except TiO and VO to avoid the formation of a temperature inversion in the upper atmosphere. These are: H 2 O (ref. 92 ), CH 4 (ref. 93 ), CO 2 (ref. 94 ), NH 3 (ref. 95 ), CO (ref. 96 ), H 2 S (ref. 97 ), HCN (ref. 98 ), PH 3 (ref. 99 ), FeH (ref. 100 ), Na (refs. 74 , 101 ) and K (refs. 74 , 101 ). For Rayleigh scattering, the opacities are H 2 (ref. 102 ) and He (ref. 103 ), and we add the following collision-induced absorption (CIA) opacities: H 2 –H 2 (ref. 104 ) and H 2 –He (ref. 104 ). We use for radiative-transfer calculations in the GCM the same wavelength resolution as SPARC/MITgcm (S1), but incorporate 16 instead of 8 k -coefficients. Two cloud-free WASP-43b GCM simulations were performed, one with solar and one with 10× solar element abundances. Each simulation ran for 1,500 days to ensure that the deep wind jet has fully developed. The GCM results used in this paper were time averaged over the last 100 simulation days.
Spectra and phase curves are produced from our GCM results in post-processing with petitRADTRANS 90 and prt_phasecurve 89 using a spectral resolution of R = 100 for both the phase curve and the spectra.
Originally adapted from the GCM of ref. 105 by refs. 106 , 107 , 108 , the RM-GCM model has been applied to numerous investigations of hot Jupiters and mini-Neptunes 35 , 109 , 110 , 111 . The GCM’s dynamical core solves the primitive equations of meteorology using a spectral representation of the domain, and it is coupled to a two-stream, double-grey radiative-transfer scheme based on ref. 112 . Recent updates have added aerosol scattering 35 with radiative feedback 8 , 36 . Following ref. 8 , aerosols are representative of condensate clouds and are treated as purely temperature-dependent sources of opacity, with constant mixing ratios set by the assumed solar elemental abundances. The optical thicknesses of the clouds are determined by converting the relative molecular abundances (or partial pressures) of each species into particles with prescribed densities and radii 8 . The model includes up to 13 different cloud species of various condensation temperatures, abundances and scattering properties. Places where clouds overlap have mixed properties, weighted by the optical thickness of each species.
Simulations from this GCM included a clear atmosphere and two sets of cloudy simulations. Following ref. 8 , one set of cases included 13 different species: KCl, ZnS, Na 2 S, MnS, Cr 2 O 3 , SiO 2 , Mg 2 SiO 4 , VO, Ni, Fe, Ca 2 SiO 4 , CaTiO 2 and Al 2 O 3 ; the other set omitted ZnS, Na 2 S, MnS, Fe and Ni, based on considerations of nucleation efficiency 113 . For both cloud composition scenarios, the models explored the observational consequences of variations in the cloud deck’s vertical thickness through a series of simulations with clouds tops truncated over a range of heights at 5-layer intervals (roughly a scale height), ranging from 5 to 45 layers of the 50-layer model. This effectively mimics a range of vertical mixing strengths. From the complete set published in ref. 26 , we selected a subset, with clouds of maximum vertical extents between two and nine scale heights from each of the two cloud composition scenarios.
Simulations were initialized with clear skies, no winds and no horizontal temperature gradients. We ran the simulations for over 3,500 planetary orbits, assuming tidal synchronization. Resulting temperature, wind and cloud fields of the GCM were then post-processed 114 , 115 to yield corresponding emission phase curves.
THOR 116 , 117 is an open-source GCM developed to study the atmospheres and climates of exoplanets, free from Earth- or Solar System-centric tunings. The core that solves the fluid flow equations, the dynamical core, solves the non-hydrostatic compressible Euler equations on an icosahedral grid 116 , 118 . THOR has been validated and used to simulate the atmosphere of Earth 116 , 119 , Solar System planets 120 , 121 and exoplanets 116 , 117 , 122 .
For this work, THOR used the same configuration as with previously published simulations to study the atmospheric temperature structure, cloud cover and chemistry of WASP-43b 4 , 38 , 123 . Two simulations were conducted, one with a clear atmosphere and another with a cloud structure on the nightside of the planet. To represent the radiative processes, THOR uses a simple two-band formulation calibrated to reproduce the results from more complex non-grey models on WASP-43b 3 , 124 . A simple cloud distribution on the nightside of the planet and optical cloud properties are parameterized 4 and adapted to reproduce previous HST 21 and Spitzer 4 , 22 observations. These simulations on WASP-43b with THOR have also been used to test the performance of future Ariel phase-curve observations 125 .
Both simulations, with clear and cloudy atmospheres, started with isothermal atmospheres (1,440 K, equilibrium temperature) and integrated for roughly 9,400 planetary orbits (assuming a tidally locked configuration) until a statistically steady state of the deep atmosphere thermal structure was reached. The long integration avoids biasing the results towards the set initial conditions 120 .
The multiwavelength spectra are obtained from post-processing the three-dimensional simulations with a multiwavelength radiative-transfer model 126 . The disk-averaged planet spectrum is calculated at each orbital phase by projecting the outgoing intensity for each geographical location of the observed hemisphere. The spectra include cross-sections of the main absorbers in the infrared, drawn from the ExoMOL (H 2 O (ref. 92 ), CH 4 (ref. 127 ), NH 3 (ref. 128 ), HCN (ref. 129 ) and H 2 S (ref. 97 )), HITEMP 130 (CO 2 and CO) and HITRAN 131 (C 2 H 2 ) databases. The Na and K resonance lines 132 are also added, as were H 2 –H 2 and H 2 –He CIA 104 . The atmospheric bulk composition was assumed to have solar abundance (consistent with HST/WFC3 spectrum observations), and each chemical species concentration was calculated with the FastChem model 133 . The PHOENIX models 74 , 75 , 76 were used for the WASP-43 star spectrum.
Atmospheric retrieval models
We perform atmospheric retrievals on the phase-resolved emission spectra using six different retrieval frameworks, each described in turn below. The chemical constraints from these analyses are summarized in Extended Data Tables 2 and 3 , and the spectral fits obtained are shown in Extended Data Fig. 3 . Across the six retrieval analyses, we use an error-inflation parameter to account for the effects of unknown data and/or model uncertainties. This free parameter is wavelength independent and multiplies the 1 σ error bars in the calculation of the likelihood function in the Bayesian sampling algorithm.
HyDRA retrieval framework
The HyDRA atmospheric retrieval framework 134 consists of a parametric atmospheric forward model coupled to PyMultiNest 135 , 136 , a nested sampling Bayesian parameter estimation algorithm 137 . HyDRA has been applied to hydrogen-rich atmospheres 138 , 139 , and further adapted for secondary atmospheres 140 and high-resolution spectroscopy in both one and two dimensions 141 , 142 . The input parameters for the atmospheric forward model include constant-with-depth abundances for each of the chemical species considered, six temperature profile parameters corresponding to the temperature profile model of ref. 143 , and a constant-with-wavelength multiplicative error-inflation parameter to account for model uncertainties. We additionally include a dilution parameter, A HS , for the dayside, morning and evening hemispheres, which multiplies the emission spectrum by a constant factor <1 and accounts for temperature inhomogeneities in each hemisphere 144 .
We consider opacity contributions from the chemical species that are expected to be present in hot Jupiter atmospheres and that have opacity in the MIRI LRS wavelength range: H 2 O (ref. 130 ), CH 4 (refs. 127 , 145 ), NH 3 (ref. 128 ), HCN (refs. 98 , 129 ), CO (ref. 130 ), CO 2 (ref. 130 ), C 2 H 2 (refs. 131 , 146 ), SO 2 (ref. 147 ), H 2 S (refs. 97 , 148 ) and CIA due to H 2 –H 2 and H 2 –He (ref. 104 ). The line-by-line absorption cross-sections for these species are calculated following the methods described in ref. 134 , using data from each of the references listed. We further explore retrievals with a simple silicate cloud model, which includes the modal particle size, cloud particle abundance, cloud base pressure and a pressure exponent for the drop-off of cloud particle number density with decreasing pressure. The opacity structure of the cloud is calculated using the absorption cross-sections of ref. 149 .
Given the input chemical abundances, temperature profile and cloud parameters, the forward model calculates line-by-line radiative transfer to produce the thermal emission spectrum at a resolution of R ≈ 15,000. The spectrum is then convolved to a resolution of 100, binned to the same wavelength bins as the observations and compared with the observed spectrum to calculate the likelihood of the model instance. The nested sampling algorithm explores the parameter space using 2,000 live points, and further calculates the Bayesian evidence of the retrieval model, which can be used to compare different models 52 . In particular, we calculate the detection significance of a particular chemical species by comparing retrievals that include/exclude that species, fixing the value of the error-inflation parameter to be the median retrieved value found with the full retrieval model.
Across the four phases, the only chemical species detected with statistical significance ( ≳ 3 σ ) is H 2 O. The retrieved H 2 O abundances are in the range ~30–10 4 ppm (1 σ uncertainties), with detection significances varying between ~3 σ and ~4 σ (Extended Data Table 2 ). We do not detect CH 4 at any phase, and place an upper limit of 16 ppm on the nightside CH 4 abundance, potentially indicating disequilibrium chemistry processes as described in the main text. We do not detect NH 3 at any phase either, consistent with the very low NH 3 abundances predicted by both chemical equilibrium and disequilibrium models 23 . The retrievals do not favour cloudy models over clear models with statistical significance, with extremely weak preferences of <1 σ at all phases. In addition, the posterior probability distributions for the cloud parameters are unconstrained. Extended Data Fig. 5 shows the pressure ranges of the atmospheric model probed by the observations.
PyratBay retrieval framework
PyratBay is an open-source framework that enables atmospheric modelling, spectral synthesis, and atmospheric retrievals of exoplanet observations 150 . The atmospheric model consists of parametric temperature, composition and altitude profiles as a function of pressure, for which emission and transmission spectra can be generated. The radiative-transfer module considers opacity from alkali lines 151 , Rayleigh scattering 152 , 153 , Exomol and HITEMP molecular line lists 130 , 154 pre-processed with the REPACK package 155 to extract the dominant line transitions, CIA 156 and cloud opacities. The PyratBay retrieval framework has the ability to stagger model complexity and explore a hierarchy of different model assumptions. Temperature models range from an isothermal profile to physically motivated parameterized models 143 , 157 . Composition profiles range from the simpler constant-with-altitude ‘free abundance’ to the more complex ‘chemically consistent’ retrievals, the latter accomplished via the TEA code 158 ; while cloud condensate prescriptions range from the classic ‘power law + grey’ to a ‘single-particle-size’ haze profile, a partial-coverage factor ‘patchy clouds’ 159 , and the complex parameterized Mie-scattering thermal stability cloud (TSC) model (J.B. et al., manuscript in preparation). The TSC cloud prescription, initially inspired by refs. 84 , 160 , has additional flexibility in the location of the cloud base and was further improved for this analysis (see below). The formulation utilizes a parameterized cloud shape, effective particle size and gas number density below the cloud deck, while the atmospheric mixing and settling are wrapped up inside the cloud extent and the condensate mole fraction as free parameters. This cloud model was applied to WASP-43b JWST/MIRI phase-curve simulations 23 , generated during the JWST preparatory phase, in anticipation of the actual WASP-43b JWST/MIRI observations. We showed that the TSC model has the ability to distinguish between MgSiO 3 and MnS clouds on the nightside of the planet.
For this analysis, we conducted a detailed investigation using various model assumptions. We started by exploring simple temperature prescriptions and gradually moved towards more complex ones. Initially, we considered opacity contributions from all chemical species expected to be observed in the MIRI wavelength range (H 2 O, CH 4 , NH 3 , HCN, CO, CO 2 , C 2 H 2 , SO 2 , H 2 S), but eventually focused on only those that are fit by the data. We also implemented the dilution parameter 144 and an error-inflation factor, which account for some additional model and data uncertainties. The constraints on H 2 O (together with the detection significance 161 ) and the upper limit for CH 4 for all phases are given in Extended Data Table 2 . The abundances of these species across all phases were largely model independent. However, the tentative constraints on NH 3 , which we saw in multiple phases, were strongly model dependent, and were completely erased with the inclusion of the dilution parameter and the error inflation, thus we do not report them here. WASP-43b emission spectra were computed at a resolution of R ≈ 15,000 utilizing opacity sampling of high-resolution pre-computed cross-sections ( R ≈ 10 6 ) of considered species. Furthermore, we thoroughly examined the possibility of detecting clouds in each of the four-quadrant phases, with a special emphasis on the nightside of the planet. To do this, we employed the TSC model, as in our previous analysis 23 , and explored a range of cloud species, MgSiO 3 , MnS, ZnS and KCl, that would condense under the temperature regimes expected for WASP-43b 162 (Extended Data Fig. 6 , left). We also introduced the effective standard deviation of the log-normal distribution 84 as a free parameter ( σ log ), allowing for even more flexibility in our cloud model (Extended Data Fig. 6 , right, last subpanel). To thoroughly explore the parameter space, we used two Bayesian samplers, the differential-evolution MCMC algorithm 163 , implemented following ref. 164 , and the nested sampling algorithm, implemented through PyMultiNest 135 , 136 , utilizing 15 million models and 2,000 live points, respectively. Our investigation did not provide constraints on any of the cloud parameters for any of the explored cloud condensates at any of the planetary phases, indicating the absence of detectable spectral features from clouds in the observations (Extended Data Fig. 6 , right).
NEMESIS retrieval framework
NEMESIS 165 , 166 is a free retrieval framework that uses a fast correlated- k 167 forward model, combined with either an optimal estimation or nested sampling retrieval algorithm. It has been used to perform retrievals on spectra of numerous planetary targets, both inside and outside the Solar System 168 , 169 . In this work, we use the PyMultiNest sampler 136 with 500 live points. The retrieval model presented includes four spectrally active gases, H 2 O (ref. 92 ), CO (ref. 96 ), CH 4 (ref. 93 ) and NH 3 (ref. 95 ), with k tables calculated as in ref. 91 ; we did not include CO 2 or H 2 S after initial tests indicated these were not required to fit the spectrum. All gases are assumed to be well mixed in altitude. CIA from H 2 and He is taken from refs. 156 , 170 . The spectrum is calculated at the resolution of the observation, using optimized channel integrated k tables generated from original k tables with a resolving power R = 1,000. The temperature profile is modelled as a three-parameter Guillot profile, after ref. 157 , with free parameters κ , γ and β ( α is fixed to be zero). We include a well-mixed, spectrally grey cloud with a scalable total optical depth with a cloud top at 12.5 mbar. The other retrieved parameters are a hotspot dilution factor for phases 0.25, 0.5 and 0.75, following ref. 144 , and an error-inflation term.
To calculate the detection significance for H 2 O, we run the retrieval with and without H 2 O, with all other aspects of the run identical. We then take the difference of the PyMultiNest global log-evidence values for the two scenarios, and convert from log(Bayesian evidence) to sigma following ref. 52 . The 99% upper limit for CH 4 is calculated from the equally weighted posterior distribution. We also attempt to retrieve CO and NH 3 abundances. CO is generally poorly constrained, and NH 3 is unconstrained for phases 0 and 0.75; for log(NH 3 ), we recover a 99% upper limit of −2.2 at phase 0.25 and −3.9 at phase 0.5. The cloud opacity is also generally unconstrained, with the total optical depth able to span several orders of magnitude. We stress that this model is very crude as it has only one variable cloud parameter, and further exploration of suitable cloud models for mid-infrared phase curves is warranted in future work.
SCARLET retrieval framework
We perform atmospheric retrievals on the four phase-resolved spectra using the SCARLET framework 160 , 171 . The planetary disk-integrated thermal emission, F p , is modelled for a given set of atomic/molecular abundances, temperature–pressure profile and cloud properties. We compare our model spectra with the observations by normalizing the thermal emission F p using a PHOENIX 74 , 75 , 76 stellar model spectrum with effective temperature T eff = 4,300 K and surface gravity log g = 4.50. The model spectra are computed at a resolving power of R = 15,625, convolved to the resolving power of MIRI/LRS and then binned to the 11 spectral bins (<10.5 μm) considered in the analysis, assuming the throughput to be uniform over a single bin.
The atmospheric analysis is performed considering thermochemical equilibrium, where the metallicity [M/H] ( \({{{\mathcal{U}}}}[-3,3]\) ) and carbon-to-oxygen ratio ( \({{{\mathcal{U}}}}[0,3]\) ) are free parameters that dictate the overall atmospheric composition. We use a free parameterization of the temperature–pressure profile 172 by fitting for N = 4 temperature points ( \({{{\mathcal{U}}}}[100,4400]\,{\mathrm{K}}\) ) with a constant spacing in log-pressure. The temperature–pressure profile is interpolated to the 50 layers ( P = 10 2 –10 −6 bar) considered in the model using a spline function to produce a smooth profile. We use a grid of chemical equilibrium abundances produced with FastChem2 173 to interpolate the abundance of species as a function of temperature and pressure for given values of [M/H] and C/O. The species considered in the equilibrium chemistry are H, H − (refs. 174 , 175 ), H 2 , He, H 2 O (ref. 92 ), OH (ref. 130 ), CH 4 (ref. 127 ), C 2 H 2 (ref. 176 ), CO (ref. 130 ), CO 2 (ref. 130 ), NH 3 (ref. 95 ), HCN (ref. 98 ), PH 3 (ref. 99 ), TiO (ref. 177 ) and VO (ref. 178 ). All opacities for these species are considered when computing the thermal emission. We account for potential spatial atmospheric inhomogeneities in the planetary disk that are observed at a given phase by including an area fraction parameter A HS ( \({{{\mathcal{U}}}}[0,1]\) ), which is meant to represent the possibility of a fraction of the disk contributing to most of the observed thermal emission 144 . This parameter is considered for all phases with the exception of the nightside, which is expected to be relatively uniform. Finally, we fit for an error-inflation parameter k σ ( \({{{\mathcal{U}}}}[0.1,10]\) ) to account for potential model and data uncertainty, which results in a total of 8 (7 for the nightside) free parameters. We consider 8 walkers per free parameter for the retrievals which are run for 30,000 steps. The first 18,000 steps are discarded when producing the posterior distributions of the free parameters.
PLATON retrieval framework
PLATON 179 , Planetary Atmosphere Tool for Observer Noobs, is a Bayesian retrieval tool that assumes equilibrium chemistry. We adopt the temperature–pressure profile parameterization of ref. 180 , and use the dynesty nested sampler 49 to retrieve the following free parameters: stellar radius; stellar temperature; the log metallicity, log( Z ); C/O; 5 temperature–pressure parameters (log( κ th ), log( γ ), log( γ 2 ), α , β ); and an error multiplier. The stellar radius and temperature are given Gaussian priors with means and standard deviations set by the measurements in ref. 55 : 4,400 ± 200 K and 0.667 ± 0.011 R ⊙ , respectively. The combination of the two have a similar effect to the dilution parameter of other retrieval codes, which multiplies the emission spectrum by a constant. For phase 0.0, we obtain a significantly better fit when methane opacity is set to zero (thus removing all spectral features from methane). We therefore adopt this as the fiducial model, whereas for other phases, we do not zero out any opacities.
For all retrievals, we use nested sampling with 1,000 live points. The opacities (computed at R = 10,000) and the line lists used to compute them are listed in ref. 179 . We include all 31 species in retrieval, notably including H 2 O, CO, CO 2 , CH 4 (except on the nightside), H 2 S and NH 3 .
ARCiS retrieval framework
ARCiS (Artful modelling code for exoplanet science) is an atmospheric modelling and Bayesian retrieval code 181 , 182 that utilizes the MULTINEST 135 Monte Carlo nested sampling algorithm. The code was used in previous retrievals of the atmosphere of WASP-43b in transmission 183 , using the observations of ref. 184 , and in phase-resolved emission 185 , using the observations of refs. 21 , 22 , 25 , 186 . Reference 183 found some evidence that AlO improves the fit of the transmission spectra of WASP-43b in the 1.1–1.6 μm region. We therefore include in our models for this work the following set of molecules in our free molecular retrievals: H 2 O (ref. 92 ), CO (ref. 96 ), CO 2 (ref. 94 ), NH 3 (ref. 95 ), CH 4 (ref. 93 ) and AlO (ref. 187 ). The molecular line lists are from the ExoMol 154 , 188 or HITEMP 130 databases as specified, and k tables from the ExoMolOP opacity database 91 . CIA for H 2 and He are taken from refs. 156 , 170 . We explore the inclusion of a variety of additional molecules that have available line list data with spectral features in the region of our observations, including HCN (ref. 98 ), SiO (ref. 189 ) and N 2 O (ref. 130 ). We use the Bayes factor, which is the difference between the nested sampling global log-evidence (log E ) between two models, to assess whether the inclusion of a particular parameter is statistically significant. For this, we run a retrieval with the base set of species only and another with the base set plus the molecule being assessed. The difference in log E between the two models is converted to a significance in terms of σ using the metric of ref. 52 . We explore the inclusion of a simple grey, patchy cloud model, which parameterizes cloud top pressure and degree of cloud coverage (from 0 for completely clear to 1 for completely covered). We use 1,000 live points and a sampling efficiency of 0.3 in MULTINEST for all retrievals.
We run retrievals both including and not including a retrieved error-inflation parameter. The error-inflation parameter is implemented as per ref. 190 to account for underestimated uncertainties and/or unknown missing forward model parameters. All phases apart from 0.0 retrieved a parameter that increases the observational error bars by two to three times their original values. The pressure–temperature profile parameterization of ref. 191 is used in all cases. We find evidence for the inclusion of H 2 O for all four phases, although this evidence goes from strong to weak when error inflation is included for the morning phase (0.75). We find no strong evidence for CH 4 at any phase, with 95% confidence upper limits on the log of the volume mixing ratio (VMR) of −4.9, −2.9, −3.2 and −2.2 for phases 0.0, 0.25, 0.5 and 0.75, respectively. We find some model-dependent hints of moderate evidence (based on the metric of ref. 52 ) of 4.4 σ for NH 3 at phase 0.5 (constrained to \(\log{{\mathrm{VMR}}}={-4.5}_{-0.5}^{+0.7}\) ), 3.1 σ for CO at phase 0.5 ( \(\log{{\mathrm{VMR}}}={-1.7}_{-0.7}^{+0.5}\) ) and 2.6 σ for CO at phase 0.25 ( \(\log{{\mathrm{VMR}}}={-4.0}_{-0.4}^{+0.3}\) ). However, these disappear when the error-inflation parameter is introduced. We are not able to constrain any of the cloud parameters for any phase, and so do not find a statistical reason to include our simple cloud parameterization in the models to better fit the observations.
Data availability
The data used in this paper are associated with JWST DD-ERS programme 1366 (principal investigators N.M.B., J.L.B. and K.B.S.; observation 11) and are publicly available from the Mikulski Archive for Space Telescopes ( https://mast.stsci.edu ). Additional intermediate and final results from this work are archived on Zenodo at https://doi.org/10.5281/zenodo.10525170 (ref. 192 ).
Code availability
We used the following codes to process, extract, reduce and analyse the data: STScI’s JWST Calibration pipeline 46 , Eureka! 45 , TEATRO, SPARTA 48 , Generic PCM 64 , 65 , 66 , 67 , 68 , SPARC/MITgcm 9 , 33 , 81 , 82 , expeRT/GCM 16 , 72 , 89 , RM-GCM 8 , 35 , 106 , 107 , 108 , THOR 4 , 116 , 117 , 124 , 126 , 193 , HyDRA 134 , PyratBay 150 , NEMESIS 165 , 166 , SCARLET 160 , 171 , PLATON 179 , starry 54 , exoplanet 61 , PyMC3 57 , emcee 60 , dynesty 49 , numpy 194 , astropy 195 , 196 and matplotlib 197 .
Keating, D., Cowan, N. B. & Dang, L. Uniformly hot nightside temperatures on short-period gas giants. Nat. Astron. 3 , 1092–1098 (2019).
Article ADS Google Scholar
Beatty, T. G. et al. Spitzer phase curves of KELT-1b and the signatures of nightside clouds in thermal phase observations. Astron. J. 158 , 166 (2019).
Kataria, T. et al. The atmospheric circulation of the hot Jupiter WASP-43b: comparing three-dimensional models to spectrophotometric data. Astrophys. J. 801 , 86 (2015).
Mendonça, J. M., Malik, M., Demory, B.-O. & Heng, K. Revisiting the phase curves of WASP-43b: confronting re-analyzed Spitzer data with cloudy atmospheres. Astron. J. 155 , 150 (2018).
Parmentier, V. & Crossfield, I. J. M. in Handbook of Exoplanets (eds Deeg, H. J. & Belmonte, J. A.) 1419–1440 (Springer, 2018).
Hu, R., Demory, B.-O., Seager, S., Lewis, N. & Showman, A. P. A semi-analytical model of visible-wavelength phase curves of exoplanets and applications to Kepler-7b and Kepler-10b. Astrophys. J. 802 , 51 (2015).
Shporer, A. & Hu, R. Studying atmosphere-dominated hot Jupiter Kepler phase curves: evidence that inhomogeneous atmospheric reflection is common. Astron. J. 150 , 112 (2015).
Roman, M. T. et al. Clouds in three-dimensional models of hot Jupiters over a wide range of temperatures. I. Thermal structures and broadband phase-curve predictions. Astrophys. J. 908 , 101 (2021).
Tan, X. & Showman, A. P. Atmospheric circulation of brown dwarfs and directly imaged exoplanets driven by cloud radiative feedback: effects of rotation. Mon. Not. R. Astron. Soc. 502 , 678–699 (2021).
Parmentier, V., Showman, A. P. & Fortney, J. J. The cloudy shape of hot Jupiter thermal phase curves. Mon. Not. R. Astron. Soc. 501 , 78–108 (2021).
Perna, R., Menou, K. & Rauscher, E. Magnetic drag on hot Jupiter atmospheric winds. Astrophys. J. 719 , 1421–1426 (2010).
Komacek, T. D. & Youdin, A. N. Structure and evolution of internally heated hot Jupiters. Astrophys. J. 844 , 94 (2017).
Rogers, T. M. Constraints on the magnetic field strength of HAT-P-7 b and other hot giant exoplanets. Nat. Astron. 1 , 0131 (2017).
Kataria, T., Showman, A. P., Fortney, J. J., Marley, M. S. & Freedman, R. S. The atmospheric circulation of the super Earth GJ 1214b: dependence on composition and metallicity. Astrophys. J. 785 , 92 (2014).
Zhang, X. & Showman, A. P. Effects of bulk composition on the atmospheric dynamics on close-in exoplanets. Astrophys. J. 836 , 73 (2017).
Carone, L. et al. Equatorial retrograde flow in WASP-43b elicited by deep wind jets? Mon. Not. R. Astron. Soc. 496 , 3582–3614 (2020).
Showman, A. P., Tan, X. & Parmentier, V. Atmospheric dynamics of hot giant planets and brown dwarfs. Space Sci. Rev. 216 , 139 (2020).
Helling, C. et al. Cloud property trends in hot and ultra-hot giant gas planets (WASP-43b, WASP-103b, WASP-121b, HAT-P-7b, and WASP-18b). Astron. Astrophys. 649 , A44 (2021).
Article Google Scholar
Hellier, C. et al. WASP-43b: the closest-orbiting hot Jupiter. Astron. Astrophys. 535 , L7 (2011).
Scandariato, G. et al. Phase curve and geometric albedo of WASP-43b measured with CHEOPS, TESS, and HST WFC3/UVIS. Astron. Astrophys. 668 , A17 (2022).
Stevenson, K. B. et al. Thermal structure of an exoplanet atmosphere from phase-resolved emission spectroscopy. Science 346 , 838–841 (2014).
Stevenson, K. B. et al. Spitzer phase curve constraints for WASP-43b at 3.6 and 4.5 μm. Astron. J. 153 , 68 (2017).
Venot, O. et al. Global chemistry and thermal structure models for the hot Jupiter WASP-43b and predictions for JWST. Astrophys. J. 890 , 176 (2020).
Helling, C. et al. Mineral cloud and hydrocarbon haze particles in the atmosphere of the hot Jupiter JWST target WASP-43b. Astron. Astrophys. 641 , A178 (2020).
Morello, G., Danielski, C., Dickens, D., Tremblin, P. & Lagage, P. O. An independent analysis of the Spitzer/IRAC phase curves of WASP43 b. Astron. J. 157 , 205 (2019).
Murphy, M. M. et al. A lack of variability between repeated Spitzer phase curves of WASP-43b. Astron. J. 165 , 107 (2023).
Wright, G. S. et al. The mid-infrared instrument for jwst and its in-flight performance. Publ. Astron. Soc. Pac. 135 , 048003 (2023).
Kendrew, S. et al. The Mid-Infrared Instrument for the James Webb Space Telescope, IV: the low-resolution spectrometer. Publ. Astron. Soc. Pac. 127 , 623 (2015).
Bouwman, J. et al. Spectroscopic time series performance of the Mid-infrared Instrument on the JWST. Publ. Astron. Soc. Pac. 135 , 038002 (2023).
Esposito, M. et al. The GAPS programme with HARPS-N at TNG. XIII. The orbital obliquity of three close-in massive planets hosted by dwarf K-type stars: WASP-43, HAT-P-20 and Qatar-2. Astron. Astrophys. 601 , A53 (2017).
May, E. M. & Stevenson, K. B. Introducing a new Spitzer master BLISS map to remove the instrument systematic phase-curve-parameter degeneracy, as demonstrated by a reanalysis of the 4.5 μm WASP-43b phase curve. Astron. J. 160 , 140 (2020).
Bell, T. J. et al. A comprehensive reanalysis of Spitzer’s 4.5 μm phase curves, and the phase variations of the ultra-hot Jupiters MASCARA-1b and KELT-16b. Mon. Not. R. Astron. Soc. 504 , 3316–3337 (2021).
Showman, A. P. et al. Atmospheric circulation of hot Jupiters: coupled radiative-dynamical general circulation model simulations of HD 189733b and HD 209458b. Astrophys. J. 699 , 564–584 (2009).
Showman, A. P. & Polvani, L. M. Equatorial superrotation on tidally locked exoplanets. Astrophys. J. 738 , 71 (2011).
Roman, M. & Rauscher, E. Modeling the effects of inhomogeneous aerosols on the hot Jupiter Kepler-7b’s atmospheric circulation. Astrophys. J. 850 , 17 (2017).
Roman, M. & Rauscher, E. Modeled temperature-dependent clouds with radiative feedback in hot Jupiter atmospheres. Astrophys. J. 872 , 1 (2019).
Cowan, N. B. et al. Thermal phase variations of WASP-12b: defying predictions. Astrophys. J. 747 , 82 (2012).
Mendonça, J. M., Tsai, S.-m, Malik, M., Grimm, S. L. & Heng, K. Three-dimensional circulation driving chemical disequilibrium in WASP-43b. Astrophys. J. 869 , 107 (2018).
Agúndez, M., Parmentier, V., Venot, O., Hersant, F. & Selsis, F. Pseudo 2D chemical model of hot-Jupiter atmospheres: application to HD 209458b and HD 189733b. Astron. Astrophys. 564 , A73 (2014).
Tsai, S.-M. et al. Inferring shallow surfaces on sub-Neptune exoplanets with JWST. Astrophys. J. Lett. 922 , L27 (2021).
Moses, J. I., Tremblin, P., Venot, O. & Miguel, Y. Chemical variation with altitude and longitude on exo-Neptunes: predictions for Ariel phase-curve observations. Exp. Astron. 53 , 279–322 (2022).
Baeyens, R., Konings, T., Venot, O., Carone, L. & Decin, L. Grid of pseudo-2D chemistry models for tidally locked exoplanets—II. The role of photochemistry. Mon. Not. R. Astron. Soc. 512 , 4877–4892 (2022).
Gáspár, A. et al. The quantum efficiency and diffractive image artifacts of Si:As IBC mid-IR detector arrays at 5–10 μm: implications for the JWST/MIRI detectors. Publ. Astron. Soc. Pac. 133 , 014504 (2021).
Bell, T. J. et al. A first look at the JWST MIRI/LRS phase curve of WASP-43b. Preprint at https://arxiv.org/abs/2301.06350 (2023).
Bell, T. et al. Eureka!: an end-to-end pipeline for JWST time-series observations. J. Open Source Softw. 7 , 4503 (2022).
Bushouse, H. et al. JWST calibration pipeline. GitHub https://github.com/spacetelescope/jwst (2022).
Schlawin, E. et al. JWST noise floor. I. Random error sources in JWST NIRCam time series. Astron. J. 160 , 231 (2020).
Kempton, E. M.-R. et al. A reflective, metal-rich atmosphere for GJ 1214b from its JWST phase curve. Nature 620 , 67–71 (2023).
Koposov, S. et al. joshspeagle/dynesty: v1.2.3. Zenodo https://doi.org/10.5281/zenodo.6609296 (2022).
Cowan, N. B. & Agol, E. Inverting phase functions to map exoplanets. Astrophys. J. Lett. 678 , L129 (2008).
Cowan, N. B., Chayes, V., Bouffard, É., Meynig, M. & Haggard, H. M. Odd harmonics in exoplanet photometry: weather or artifact? Mon. Not. R. Astron. Soc. 467 , 747–757 (2017).
ADS Google Scholar
Trotta, R. Bayes in the sky: Bayesian inference and model selection in cosmology. Contemp. Phys. 49 , 71–104 (2008).
Welbanks, L. & Madhusudhan, N. Aurora: a generalized retrieval framework for exoplanetary transmission spectra. Astrophys. J. 913 , 114 (2021).
Luger, R. et al. rodluger/starry: v1.2.0. Zenodo https://doi.org/10.5281/zenodo.5567790 (2021).
Bonomo, A. S. et al. The GAPS programme with HARPS-N at TNG. XIV. Investigating giant planet migration history via improved eccentricity and mass determination for 231 transiting planets. Astron. Astrophys. 602 , A107 (2017).
Kipping, D. M. Efficient, uninformative sampling of limb darkening coefficients for two-parameter laws. Mon. Not. R. Astron. Soc. 435 , 2152–2160 (2013).
Salvatier, J., Wiecki, T. V. & Fonnesbeck, C. Probabilistic programming in Python using PyMC3. PeerJ Comput. Sci. 2 , e55 (2016).
Gelman, A. & Rubin, D. B. Inference from iterative simulation using multiple sequences. Stat. Sci. 7 , 457–472 (1992).
Kreidberg, L. batman: basic transit model calculation in Python. Publ. Astron. Soc. Pac. 127 , 1161 (2015).
Foreman-Mackey, D., Hogg, D. W., Lang, D. & Goodman, J. emcee: the MCMC hammer. Publ. Astron. Soc. Pac. 125 , 306 (2013).
Foreman-Mackey, D. et al. exoplanet: gradient-based probabilistic inference for exoplanet data & other astronomical time series. J. Open Source Softw. 6 , 3285 (2021).
Ivshina, E. S. & Winn, J. N. TESS transit timing of hundreds of hot Jupiters. Astrophys. J. Suppl. Ser. 259 , 62 (2022).
Gillon, M. et al. The TRAPPIST survey of southern transiting planets. I. Thirty eclipses of the ultra-short period planet WASP-43 b. Astron. Astrophys. 542 , A4 (2012).
Turbet, M. et al. Day-night cloud asymmetry prevents early oceans on Venus but not on Earth. Nature 598 , 276–280 (2021).
Spiga, A. et al. Global climate modeling of Saturn’s atmosphere. Part II: Multi-annual high-resolution dynamical simulations. Icarus 335 , 113377 (2020).
Charnay, B., Forget, F., Tobie, G., Sotin, C. & Wordsworth, R. Titan’s past and future: 3D modeling of a pure nitrogen atmosphere and geological implications. Icarus 241 , 269–279 (2014).
Turbet, M. et al. The habitability of Proxima Centauri b: II. possible climates and observability. Astron. Astrophys. 596 , A112 (2016).
Charnay, B., Meadows, V., Misra, A., Leconte, J. & Arney, G. 3D modeling of GJ1214b’s atmosphere: formation of inhomogeneous high clouds and observational implications. Astrophys. J. 813 , L1 (2015).
Teinturier, L. et al. The radiative and dynamical impact of clouds in the atmosphere of the hot Jupiter WASP-43 b. Astron. Astrophys. 683 , A231 (2024).
Blain, D., Charnay, B. & Bézard, B. 1D atmospheric study of the temperate sub-Neptune K2-18b. Astron. Astrophys. 646 , A15 (2021).
Falco, A., Zingales, T., Pluriel, W. & Leconte, J. Toward a multidimensional analysis of transmission spectroscopy: I. Computation of transmission spectra using a 1D, 2D, or 3D atmosphere structure. Astron. Astrophys. 658 , A41 (2022).
Adcroft, A., Campin, J.-M., Hill, C. & Marshall, J. Implementation of an atmosphere ocean general circulation model on the expanded spherical cube. Mon. Weather Rev. 132 , 2845 (2004).
Marley, M. S., Gelino, C., Stephens, D., Lunine, J. I. & Freedman, R. Reflected spectra and albedos of extrasolar giant planets. I. Clear and cloudy atmospheres. Astrophys. J. 513 , 879–893 (1999).
Allard, F. & Hauschildt, P. H. Model atmospheres for M (sub)dwarf stars. I. The base model grid. Astrophys. J. 445 , 433 (1995).
Hauschildt, P. H., Allard, F. & Baron, E. The NextGen model atmosphere grid for 3000 ≤ T eff ≤ 10,000 K. Astrophys. J. 512 , 377–385 (1999).
Husser, T. O. et al. A new extensive library of PHOENIX stellar atmospheres and synthetic spectra. Astron. Astrophys. 553 , A6 (2013).
Freedman, R. S., Marley, M. S. & Lodders, K. Line and mean opacities for ultracool dwarfs and extrasolar planets. Astrophys. J. Suppl. Ser. 174 , 504–513 (2008).
Freedman, R. S. et al. Gaseous mean opacities for giant planet and ultracool dwarf atmospheres over a range of metallicities and temperatures. Astrophys. J. Suppl. Ser. 214 , 25 (2014).
Marley, M. S. et al. The Sonora brown dwarf atmosphere and evolution models. I. Model description and application to cloudless atmospheres in rainout chemical equilibrium. Astrophys. J. 920 , 85 (2021).
Visscher, C., Lodders, K. & Fegley, B. Atmospheric chemistry in giant planets, brown dwarfs, and low-mass dwarf stars. III. Iron, magnesium, and silicon. Astrophys. J. 716 , 1060–1075 (2010).
Parmentier, V., Showman, A. P. & Lian, Y. 3D mixing in hot Jupiters atmospheres—I. Application to the day/night cold trap in HD 209458b. Astron. Astrophys. 558 , A91 (2013).
Tan, X. & Showman, A. P. Atmospheric circulation of brown dwarfs and directly imaged exoplanets driven by cloud radiative feedback: global and equatorial dynamics. Mon. Not. R. Astron. Soc. 502 , 2198–2219 (2021).
Komacek, T. D., Tan, X., Gao, P. & Lee, E. K. H. Patchy nightside clouds on ultra-hot Jupiters: general circulation model simulations with radiatively active cloud tracers. Astrophys. J. 934 , 79 (2022).
Ackerman, A. S. & Marley, M. S. Precipitating condensation clouds in substellar atmospheres. Astrophys. J. 556 , 872–884 (2001).
Lee, E. K. et al. 3D radiative transfer for exoplanet atmospheres. gCMCRT: a GPU-accelerated mcrt code. Astrophys. J. 929 , 180 (2022).
Lee, E. K. et al. Simulating gas giant exoplanet atmospheres with exo-fms: comparing semigrey, picket fence, and correlated- k radiative-transfer schemes. Mon. Not. R. Astron. Soc. 506 , 2695–2711 (2021).
Grimm, S. L. et al. Helios-k 2.0 opacity calculator and open-source opacity database for exoplanetary atmospheres. Astrophys. J. Suppl. Ser. 253 , 30 (2021).
Gharib-Nezhad, E. et al. Exoplines: molecular absorption cross-section database for brown dwarf and giant exoplanet atmospheres. Astrophys. J. Suppl. Ser. 254 , 34 (2021).
Schneider, A. D. et al. Exploring the deep atmospheres of HD 209458b and WASP-43b using a non-gray general circulation model. Astron. Astrophys. 664 , A56 (2022).
Mollière, P. et al. petitRADTRANS. a Python radiative transfer package for exoplanet characterization and retrieval. Astron. Astrophys. 627 , A67 (2019).
Chubb, K. L. et al. The ExoMolOP database: cross sections and k -tables for molecules of interest in high-temperature exoplanet atmospheres. Astron. Astrophys. 646 , A21 (2021).
Polyansky, O. L. et al. ExoMol molecular line lists XXX: a complete high-accuracy line list for water. Mon. Not. R. Astron. Soc. 480 , 2597–2608 (2018).
Yurchenko, S. N., Amundsen, D. S., Tennyson, J. & Waldmann, I. P. A hybrid line list for CH 4 and hot methane continuum. Astron. Astrophys. 605 , A95 (2017).
Yurchenko, S. N., Mellor, T. M., Freedman, R. S. & Tennyson, J. ExoMol line lists—XXXIX. Ro-vibrational molecular line list for CO 2 . Mon. Not. R. Astron. Soc. 496 , 5282–5291 (2020).
Coles, P. A., Yurchenko, S. N. & Tennyson, J. ExoMol molecular line lists—XXXV. a rotation–vibration line list for hot ammonia. Mon. Not. R. Astron. Soc. 490 , 4638–4647 (2019).
Li, G. et al. Rovibrational line lists for nine isotopologues of the CO molecule in the X 1 Σ + ground electronic state. Astrophys. J. Suppl. Ser. 216 , 15 (2015).
Azzam, A. A. A., Tennyson, J., Yurchenko, S. N. & Naumenko, O. V. ExoMol molecular line lists—XVI. The rotation-vibration spectrum of hot H 2 S. Mon. Not. R. Astron. Soc. 460 , 4063–4074 (2016).
Barber, R. J. et al. ExoMol line lists—III. \n improved hot rotation-vibration line list for HCN and HNC. Mon. Not. R. Astron. Soc. 437 , 1828–1835 (2014).
Sousa-Silva, C., Al-Refaie, A. F., Tennyson, J. & Yurchenko, S. N. ExoMol line lists—VII. the rotation–vibration spectrum of phosphine up to 1500 K. Mon. Not. R. Astron. Soc. 446 , 2337–2347 (2014).
Wende, S., Reiners, A., Seifahrt, A. & Bernath, P. F. CRIRES spectroscopy and empirical line-by-line identification of FeH molecular absorption in an M dwarf. Astron. Astrophys. 523 , A58 (2010).
Piskunov, N. E., Kupka, F., Ryabchikova, T. A., Weiss, W. W. & Jeffery, C. S. VALD: the Vienna Atomic Line Data Base. Astron. Astrophys. Suppl. 112 , 525 (1995).
Dalgarno, A. & Williams, D. A. Rayleigh scattering by molecular hydrogen. Astrophys. J. 136 , 690–692 (1962).
Chan, Y. M. & Dalgarno, A. The refractive index of helium. Proc. Phys. Soc. 85 , 227–230 (1965).
Richard, C. et al. New section of the HITRAN database: collision-induced absorption (CIA). J. Quant. Spectrosc. Radiat. Transf. 113 , 1276–1285 (2012).
Hoskins, B. & Simmons, A. A multi-layer spectral model and the semi-implicit method. Q. J. R. Meteorol. Soc. 101 , 637–655 (1975).
Menou, K. & Rauscher, E. Atmospheric circulation of hot Jupiters: a shallow three-dimensional model. Astrophys. J. 700 , 887–897 (2009).
Rauscher, E. & Menou, K. Three-dimensional modeling of hot jupiter atmospheric flows. Astrophys. J. 714 , 1334 (2010).
Rauscher, E. & Menou, K. A general circulation model for gaseous exoplanets with double-gray radiative transfer. Astrophys. J. 750 , 96 (2012).
Rauscher, E. & Kempton, E. M. The atmospheric circulation and observable properties of non-synchronously rotating hot Jupiters. Astrophys. J. 790 , 79 (2014).
May, E. M. & Rauscher, E. From super-Earths to mini-Neptunes: implications of a surface on atmospheric circulation. Astrophys. J. 893 , 161 (2020).
Beltz, H. et al. Magnetic drag and 3D effects in theoretical high-resolution emission spectra of ultrahot Jupiters: the case of WASP-76b. Astron. J. 164 , 140 (2022).
Toon, O. B., McKay, C., Ackerman, T. & Santhanam, K. Rapid calculation of radiative heating rates and photodissociation rates in inhomogeneous multiple scattering atmospheres. J. Geophys. Res. Atmos. 94 , 16287–16301 (1989).
Gao, P. et al. Aerosol composition of hot giant exoplanets dominated by silicates and hydrocarbon hazes. Nat. Astron. 4 , 951–956 (2020).
Zhang, J., Kempton, E. M. R. & Rauscher, E. Constraining hot Jupiter atmospheric structure and dynamics through Doppler-shifted emission spectra. Astrophys. J. 851 , 84 (2017).
Malsky, I. et al. Modeling the high-resolution emission spectra of clear and cloudy nontransiting hot Jupiters. Astrophys. J. 923 , 62 (2021).
Mendonça, J. M., Grimm, S. L., Grosheintz, L. & Heng, K. THOR: a new and flexible global circulation model to explore planetary atmospheres. Astrophys. J. 829 , 115 (2016).
Deitrick, R. et al. THOR 2.0: major improvements to the open-source general circulation model. Astrophys. J. Suppl. Ser. 248 , 30 (2020).
Tomita, H. & Satoh, M. A new dynamical framework of nonhydrostatic global model using the icosahedral grid. Fluid Dyn. Res. 34 , 357–400 (2004).
Article ADS MathSciNet Google Scholar
Mendonça, J. M. Mass transport in a moist planetary climate model. Astron. Astrophys. 659 , A43 (2022).
Mendonça, J. M. & Buchhave, L. A. Modelling the 3D climate of Venus with OASIS. Mon. Not. R. Astron. Soc. 496 , 3512–3530 (2020).
Shao, W. D., Zhang, X., Mendonça, J. & Encrenaz, T. Local-time dependence of chemical species in the Venusian mesosphere. Planet. Sci. J. 3 , 3 (2022).
Deitrick, R. et al. The THOR + HELIOS general circulation model: multiwavelength radiative transfer with accurate scattering by clouds/hazes. Mon. Not. R. Astron. Soc. 512 , 3759–3787 (2022).
Tsai, S.-M. et al. Toward consistent modeling of atmospheric chemistry and dynamics in exoplanets: validation and generalization of the chemical relaxation method. Astrophys. J. 862 , 31 (2018).
Malik, M. et al. HELIOS: an open-source, GPU-accelerated radiative transfer code for self-consistent exoplanetary atmospheres. Astron. J. 153 , 56 (2017).
Charnay, B. et al. A survey of exoplanet phase curves with Ariel. Exp. Astron. 53 , 417–446 (2022).
Mendonça, J. M., Read, P. L., Wilson, C. F. & Lee, C. A new, fast and flexible radiative transfer method for Venus general circulation models. Planet. Space Sci. 105 , 80–93 (2015).
Yurchenko, S. N. & Tennyson, J. ExoMol line lists—IV. The rotation–vibration spectrum of methane up to 1500 K. Mon. Not. R. Astron. Soc. 440 , 1649–1661 (2014).
Yurchenko, S. N., Barber, R. J. & Tennyson, J. A variationally computed line list for hot NH 3 . Mon. Not. R. Astron. Soc. 413 , 1828–1834 (2011).
Harris, G. J., Tennyson, J., Kaminsky, B. M., Pavlenko, Y. V. & Jones, H. R. A. Improved HCN/HNC linelist, model atmospheres and synthetic spectra for WZ Cas. Mon. Not. R. Astron. Soc. 367 , 400–406 (2006).
Rothman, L. S. et al. HITEMP, the high-temperature molecular spectroscopic database. J. Quant. Spectrosc. Radiat. Transf. 111 , 2139–2150 (2010).
Rothman, L. S. et al. The HITRAN2012 molecular spectroscopic database. J. Quant. Spectrosc. Radiat. Transf. 130 , 4–50 (2013).
Draine, B. T. Physics of the Interstellar and Intergalactic Medium (Princeton Univ. Press, 2011).
Stock, J. W., Kitzmann, D., Patzer, A. B. C. & Sedlmayr, E. FastChem: a computer program for efficient complex chemical equilibrium calculations in the neutral/ionized gas phase with applications to stellar and planetary atmospheres. Mon. Not. R. Astron. Soc. 479 , 865–874 (2018).
Gandhi, S. & Madhusudhan, N. Retrieval of exoplanet emission spectra with HyDRA. Mon. Not. R. Astron. Soc. 474 , 271–288 (2018).
Feroz, F., Hobson, M. P. & Bridges, M. MULTINEST: an efficient and robust Bayesian inference tool for cosmology and particle physics. Mon. Not. R. Astron. Soc. 398 , 1601–1614 (2009).
Buchner, J. et al. X-ray spectral modelling of the AGN obscuring region in the CDFS: Bayesian model selection and catalogue. Astron. Astrophys. 564 , A125 (2014).
Skilling, J. Nested sampling for general Bayesian computation. Bayesian Anal. 1 , 833–859 (2006).
Article MathSciNet Google Scholar
Gandhi, S., Madhusudhan, N. & Mandell, A. H- and dissociation in ultra-hot Jupiters: a retrieval case study of WASP-18b. Astron. J. 159 , 232 (2020).
Piette, A. A. A. & Madhusudhan, N. Considerations for atmospheric retrieval of high-precision brown dwarf spectra. Mon. Not. R. Astron. Soc. 497 , 5136–5154 (2020).
Piette, A. A. A., Madhusudhan, N. & Mandell, A. M. HyDRo: atmospheric retrieval of rocky exoplanets in thermal emission. Mon. Not. R. Astron. Soc. 511 , 2565–2584 (2022).
Gandhi, S., Madhusudhan, N., Hawker, G. & Piette, A. HyDRA-H: simultaneous hybrid retrieval of exoplanetary emission spectra. Astron. J. 158 , 228 (2019).
Gandhi, S. et al. Spatially resolving the terminator: variation of Fe, temperature, and winds in WASP-76 b across planetary limbs and orbital phase. Mon. Not. R. Astron. Soc. 515 , 749–766 (2022).
Madhusudhan, N. & Seager, S. A temperature and abundance retrieval method for exoplanet atmospheres. Astrophys. J. 707 , 24–39 (2009).
Taylor, J. et al. Understanding and mitigating biases when studying inhomogeneous emission spectra with JWST. Mon. Not. R. Astron. Soc. 493 , 4342–4354 (2020).
Yurchenko, S. N., Tennyson, J., Barber, R. J. & Thiel, W. Vibrational transition moments of CH 4 from first principles. Journal of Molecular Spectroscopy 291 , 69–76 (2013).
Gordon, I. E. et al. The HITRAN2016 molecular spectroscopic database. J. Quant. Spectrosc. Radiat. Transf. 203 , 3–69 (2017).
Underwood, D. S. et al. ExoMol molecular line lists—XIV. The rotation–vibration spectrum of hot SO 2 . Mon. Not. R. Astron. Soc. 459 , 3890–3899 (2016).
Chubb, K. L. et al. Marvel analysis of the measured high-resolution rovibrational spectra of H 2 32 S. J. Quant. Spectrosc. Radiat. Transf. 218 , 178–186 (2018).
Pinhas, A. & Madhusudhan, N. On signatures of clouds in exoplanetary transit spectra. Mon. Not. R. Astron. Soc. 471 , 4355–4373 (2017).
Cubillos, P. E. & Blecic, J. The PyratBay framework for exoplanet atmospheric modeling: a population study of Hubble/WFC3 transmission spectra. Mon. Not. R. Astron. Soc. 505 , 2675–2702 (2021).
Burrows, A., Marley, M. S. & Sharp, C. M. The near-infrared and optical spectra of methane dwarfs and brown dwarfs. Astrophys. J. 531 , 438–446 (2000).
Kurucz, R. L. ATLAS: A Computer Program for Calculating Model Stellar Atmospheres SAO Special Report 309 (SAO, 1970).
Lecavelier Des Etangs, A., Pont, F., Vidal-Madjar, A. & Sing, D. Rayleigh scattering in the transit spectrum of HD 189733b. Astron. Astrophys. 481 , L83–L86 (2008).
Tennyson, J. et al. The ExoMol database: molecular line lists for exoplanet and other hot atmospheres. J. Mol. Spectrosc. 327 , 73–94 (2016).
Cubillos, P. E. An algorithm to compress line-transition data for radiative-transfer calculations. Astrophys. J. 850 , 32 (2017).
Borysow, A., Jorgensen, U. G. & Fu, Y. High-temperature (1000–7000 K) collision-induced absorption of H 2 pairs computed from the first principles, with application to cool and dense stellar atmospheres. J. Quant. Spectrosc. Radiat. Transf. 68 , 235–255 (2001).
Parmentier, V. & Guillot, T. A non-grey analytical model for irradiated atmospheres. I. Derivation. Astron. Astrophys. 562 , A133 (2014).
Blecic, J., Harrington, J. & Bowman, M. O. TEA: a code calculating thermochemical equilibrium abundances. Astrophys. J. Suppl. Ser. 225 , 4 (2016).
Line, M. R. & Parmentier, V. The influence of nonuniform cloud cover on transit transmission spectra. Astrophys. J. 820 , 78 (2016).
Benneke, B. Strict upper limits on the carbon-to-oxygen ratios of eight hot Jupiters from self-consistent atmospheric retrieval. Preprint at https://arxiv.org/abs/1504.07655 (2015).
Benneke, B. & Seager, S. How to distinguish between cloudy mini-Neptunes and water/volatile-dominated super-Earths. Astrophys. J. 778 , 153 (2013).
Morley, C. V. et al. Neglected clouds in T and Y dwarf atmospheres. Astrophys. J. 756 , 172 (2012).
ter Braak, C. J. F. & Vrugt, J. A. Differential evolution Markov chain with snooker updater and fewer chains. Stat. Comput. 18 , 435–446 (2008).
Cubillos, P. et al. On correlated-noise analyses applied to exoplanet light curves. Astron. J. 153 , 3 (2017).
Irwin, P. G. J. et al. The NEMESIS planetary atmosphere radiative transfer and retrieval tool. J. Quant. Spectrosc. Radiat. Transf. 109 , 1136–1150 (2008).
Krissansen-Totton, J., Garland, R., Irwin, P. & Catling, D. C. Detectability of biosignatures in anoxic atmospheres with the James Webb Space Telescope: a TRAPPIST-1e case study. Astron. J. 156 , 114 (2018).
Lacis, A. A. & Oinas, V. A description of the correlated- k distribution method for modelling nongray gaseous absorption, thermal emission, and multiple scattering in vertically inhomogeneous atmospheres. J. Geophys. Res. 96 , 9027–9064 (1991).
Barstow, J. K. Unveiling cloudy exoplanets: the influence of cloud model choices on retrieval solutions. Mon. Not. R. Astron. Soc. 497 , 4183–4195 (2020).
Irwin, P. G. J. et al. 2.5D retrieval of atmospheric properties from exoplanet phase curves: application to WASP-43b observations. Mon. Not. R. Astron. Soc. 493 , 106–125 (2020).
Borysow, A. Collision-induced absorption coefficients of H 2 pairs at temperatures from 60 K to 1000 K. Astron. Astrophys. 390 , 779–782 (2002).
Benneke, B. et al. Water vapor and clouds on the habitable-zone sub-Neptune exoplanet K2-18b. Astrophys. J. Lett. 887 , L14 (2019).
Pelletier, S. et al. Where is the water? Jupiter-like C/H ratio but strong H 2 O depletion found on τ Boötis b using SPIRou. Astron. J. 162 , 73 (2021).
Stock, J. W., Kitzmann, D. & Patzer, A. B. C. FASTCHEM 2: an improved computer program to determine the gas-phase chemical equilibrium composition for arbitrary element distributions. Mon. Not. R. Astron. Soc. 517 , 4070–4080 (2022).
Bell, K. L. & Berrington, K. A. Free-free absorption coefficient of the negative hydrogen ion. J. Phys. B 20 , 801–806 (1987).
John, T. L. Continuous absorption by the negative hydrogen ion reconsidered. Astron. Astrophys. 193 , 189–192 (1988).
Chubb, K. L., Tennyson, J. & Yurchenko, S. N. ExoMol molecular line lists—XXXVII. spectra of acetylene. Mon. Not. R. Astron. Soc. 493 , 1531–1545 (2020).
McKemmish, L. K. et al. ExoMol molecular line lists—XXXIII. The spectrum of titanium oxide. Mon. Not. R. Astron. Soc. 488 , 2836–2854 (2019).
McKemmish, L. K., Yurchenko, S. N. & Tennyson, J. ExoMol line lists—XVIII. The high-temperature spectrum of VO. Mon. Not. R. Astron. Soc. 463 , 771–793 (2016).
Zhang, M., Chachan, Y., Kempton, E. M. R., Knutson, H. A. & Chang, W. H. PLATON II: new capabilities and a comprehensive retrieval on HD 189733b transit and eclipse data. Astrophys. J. 899 , 27 (2020).
Line, M. R. & Yung, Y. L. A systematic retrieval analysis of secondary eclipse spectra. III. Diagnosing chemical disequilibrium in planetary atmospheres. Astrophys. J. 779 , 3 (2013).
Ormel, C. W. & Min, M. ARCiS framework for exoplanet atmospheres—the cloud transport model. Astron. Astrophys. 622 , A121 (2019).
Min, M., Ormel, C. W., Chubb, K., Helling, C. & Kawashima, Y. The ARCiS framework for exoplanet atmospheres: modelling philosophy and retrieval. Astron. Astrophys. 642 , A28 (2020).
Chubb, K. L., Min, M., Kawashima, Y., Helling, C. & Waldmann, I. Aluminium oxide in the atmosphere of hot Jupiter WASP-43b. Astron. Astrophys. 639 , A3 (2020).
Kreidberg, L. et al. A Precise water abundance measurement for the hot Jupiter WASP-43b. Astrophys. J. Lett. 793 , L27 (2014).
Chubb, K. L. & Min, M. Exoplanet atmosphere retrievals in 3D using phase curve data with ARCiS: application to WASP-43b. Astron. Astrophys. 665 , A2 (2022).
Blecic, J. et al. Spitzer observations of the thermal emission from WASP-43b. Astrophys. J. 781 , 116 (2014).
Patrascu, A. T., Tennyson, J. & Yurchenko, S. N. ExoMol molecular linelists: VII: the spectrum of AlO. Mon. Not. R. Astron. Soc. 449 , 3613–3619 (2015).
Tennyson, J. et al. The 2020 release of the ExoMol database: molecular line lists for exoplanet and other hot atmospheres. J. Quant. Spectrosc. Radiat. Transf. 255 , 107228 (2020).
Barton, E. J., Yurchenko, S. N. & Tennyson, J. ExoMol molecular linelists—II. The ro-vibrational spectrum of SiO. Mon. Not. R. Astron. Soc. 434 , 1469–1475 (2013).
Line, M. R., Teske, J., Burningham, B., Fortney, J. J. & Marley, M. S. Uniform atmospheric retrieval analysis of ultracool dwarfs. I. Characterizing benchmarks, GI570D and HD3651b. Astrophys. J. 807 , 183 (2015).
Guillot, T. On the radiative equilibrium of irradiated planetary atmospheres. Astron. Astrophys. 520 , A27 (2010).
Bell, T. J. et al. Products and models for "Nightside clouds and disequilibrium chemistry on the hot Jupiter WASP-43b". Zenodo https://doi.org/10.5281/zenodo.10525170 (2024).
Malik, M. et al. Self-luminous and irradiated exoplanetary atmospheres explored with HELIOS. Astron. J. 157 , 170 (2019).
Harris, C. R. et al. Array programming with NumPy. Nature 585 , 357–362 (2020).
Astropy Collaboration. Astropy: a community Python package for astronomy. Astron. Astrophys. 558 , A33 (2013).
Astropy Collaboration. The Astropy Project: building an open-science project and status of the v2.0 core package. Astron. J. 156 , 123 (2018).
Hunter, J. D. Matplotlib: a 2D graphics environment. Comput. Sci. Eng. 9 , 90–95 (2007).
Download references
Acknowledgements
T.J.B. acknowledges funding support from the NASA Next Generation Space Telescope Flight Investigations program (now JWST) via WBS 411672.07.05.05.03.02. J.K.B. is supported by a UKRI/STFC Ernest Rutherford Fellowship (grant ST/T004479/1). J.B. acknowledges the support received in part from the NYUAD IT High Performance Computing resources, services, and staff expertise. E.D. acknowledges funding as a Paris Region Fellow through the Marie Sklodowska-Curie Action. M.Z. and B.V.R. acknowledge funding from the 51 Pegasi b Fellowship. A.D.F. acknowledges support from the NSF Graduate Research Fellowship Program. M.M., D.P. and L.W. acknowledge funding from the NHFP Sagan Fellowship Program. P.E.C. is funded by the Austrian Science Fund (FWF) Erwin Schroedinger Fellowship program J4595-N. K.L.C. acknowledges funding from STFC, under project number ST/V000861/1. L.D. acknowledges funding from the KU Leuven Interdisciplinary Grant (IDN/19/028), the European Union H2020-MSCA-ITN-2019 under grant no. 860470 (CHAMELEON) and the FWO research grant G086217N. O.V. acknowledges funding from the ANR project ‘EXACT’ (ANR-21-CE49-0008-01) and from the Centre National d’Études Spatiales (CNES). L.T. and B.C. acknowledge access to the HPC resources of MesoPSL financed by the Region Ile de France and the project Equip@Meso (reference ANR-10-EQPX-29-01) of the programme Investissements d’Avenir supervised by the Agence Nationale pour la Recherche.
Author information
Authors and affiliations.
BAER Institute, NASA Ames Research Center, Moffet Field, CA, USA
Taylor J. Bell
Space Science and Astrobiology Division, NASA Ames Research Center, Moffett Field, CA, USA
Leiden Observatory, University of Leiden, Leiden, The Netherlands
Nicolas Crouzet & Sebastian Zieba
INAF - Osservatorio Astrofisico di Torino, Turin, Italy
Patricio E. Cubillos
Space Research Institute, Austrian Academy of Sciences, Graz, Austria
Patricio E. Cubillos, Ludmila Carone & Christiane Helling
Max Planck Institute for Astronomy, Heidelberg, Germany
Laura Kreidberg, Luigi Mancini, Thomas M. Evans-Soma, Maria E. Steinrueck & Sebastian Zieba
Earth and Planets Laboratory, Carnegie Institution for Science, Washington DC, USA
Anjali A. A. Piette, Peter Gao & Munazza K. Alam
School of Physics and Astronomy, University of Leicester, Leicester, UK
Michael T. Roman & Sarah L. Casewell
Universidad Adolfo Ibáñez: Peñalolén, Santiago, Chile
Michael T. Roman
School of Physical Sciences, The Open University, Milton Keynes, UK
Joanna K. Barstow
Department of Physics, New York University Abu Dhabi, Abu Dhabi, United Arab Emirates
Jasmina Blecic & Ian Dobbs-Dixon
Center for Astro, Particle and Planetary Physics (CAP3), New York University Abu Dhabi, Abu Dhabi, United Arab Emirates
Jasmina Blecic
Department of Physics and Trottier Institute for Research on Exoplanets, Université de Montréal, Montreal, Quebec, Canada
Louis-Philippe Coulombe, Björn Benneke, Caroline Piaulet & Jake Taylor
Université Paris-Saclay, Université Paris Cité, CEA, CNRS, AIM, Gif-sur-Yvette, France
Elsa Ducrot, Achrène Dyrek & Pierre-Olivier Lagage
Atmospheric, Oceanic and Planetary Physics, Department of Physics, University of Oxford, Oxford, UK
Mark Hammond, Xianyu Tan & Jake Taylor
DTU Space, Technical University of Denmark, Kongens Lyngby, Denmark
João M. Mendonça
Space Science Institute, Boulder, CO, USA
Julianne I. Moses
Université Côte d’Azur, Observatoire de la Côte d’Azur, CNRS Laboratoire Lagrange, Nice, France
Vivien Parmentier
Johns Hopkins APL, Laurel, MD, USA
Kevin B. Stevenson
LESIA, Observatoire de Paris, Université PSL, Sorbonne Université, Université Paris Cité, CNRS, Meudon, France
Lucas Teinturier & Benjamin Charnay
Laboratoire de Météorologie Dynamique, IPSL, CNRS, Sorbonne Université, Ecole Normale Supérieure, Université PSL, Ecole Polytechnique, Institut Polytechnique de Paris, Paris, France
Lucas Teinturier
Department of Astronomy and Astrophysics, University of Chicago, Chicago, IL, USA
Michael Zhang, Jacob L. Bean & Adina D. Feinstein
Department of Astronomy and Astrophysics, University of California, Santa Cruz, Santa Cruz, CA, USA
Natalie M. Batalha & Aarynn L. Carter
Centre for Exoplanet Science, University of St Andrews, St Andrews, UK
Katy L. Chubb
Center for Space and Habitability, University of Bern, Bern, Switzerland
Brice-Olivier Demory & Elspeth K. H. Lee
Space and Planetary Sciences, Institute of Physics, University of Bern, Bern, Switzerland
Brice-Olivier Demory
Center for Astrophysics | Harvard & Smithsonian, Cambridge, MA, USA
Mercedes López-Morales & Diana Powell
Instituto de Astrofsica de Canarias (IAC), Tenerife, Spain
Giuseppe Morello & Enric Palle
INAF- Palermo Astronomical Observatory, Piazza del Parlamento, Palermo, Italy
Giuseppe Morello
Department of Space, Earth and Environment, Chalmers University of Technology, Gothenburg, Sweden
Department of Astronomy, University of Michigan, Ann Arbor, MI, USA
Emily Rauscher, Ryan C. Challener & Isaac Malsky
Department of Earth and Planetary Sciences, Johns Hopkins University, Baltimore, MD, USA
David K. Sing
Department of Physics and Astronomy, Johns Hopkins University, Baltimore, MD, USA
David K. Sing & Néstor Espinoza
Tsung-Dao Lee Institute, Shanghai Jiao Tong University, Shanghai, People’s Republic of China
School of Physics and Astronomy, Shanghai Jiao Tong University, Shanghai, People’s Republic of China
Université Paris Cité and Univ Paris Est Creteil, CNRS, LISA, Paris, France
Olivia Venot
School of Physics, University of Bristol, Bristol, UK
Hannah R. Wakeford
Indian Institute of Technology, Indore, India
Keshav Aggarwal
Centre for Exoplanets and Habitability, University of Warwick, Coventry, UK
Eva-Maria Ahrer
Department of Physics, University of Warwick, Coventry, UK
Anton Pannekoek Institute for Astronomy, University of Amsterdam, Amsterdam, The Netherlands
Robin Baeyens & Jean-Michel Désert
Departamento de Astrofsica, Centro de Astrobiologa (CAB, CSIC-INTA), ESAC campus, Madrid, Spain
David Barrado
Instituto de Astrofisica, Facultad Ciencias Exactas, Universidad Andres Bello, Santiago, Chile
Claudio Caceres
Centro de Astrofisica y Tecnologias Afines (CATA), Santiago, Chile
Nucleo Milenio de Formacion Planetaria (NPF), Valparaíso, Chile
Department of Physics and Astronomy, University of Kansas, Lawrence, KS, USA
Ian J. M. Crossfield
Institute of Astronomy, Department of Physics and Astronomy, KU Leuven, Leuven, Belgium
Leen Decin & Aaron D. Schneider
Space Telescope Science Institute, Baltimore, MD, USA
Néstor Espinoza & Nikolay K. Nikolov
Department of Astrophysical and Planetary Sciences, University of Colorado Boulder, Boulder, CO, USA
Adina D. Feinstein
School of Physics, Trinity College Dublin, Dublin, Ireland
Neale P. Gibson
Planetary Sciences Group, Department of Physics and Florida Space Institute, University of Central Florida, Orlando, FL, USA
Joseph Harrington
Astrophysics Section, Jet Propulsion Laboratory, California Institute of Technology, Pasadena, CA, USA
Division of Geological and Planetary Sciences, California Institute of Technology, Pasadena, CA, USA
Institute of Planetary Research—Extrasolar Planets And Atmospheres, German Aerospace Center (DLR), Berlin, Germany
Nicolas Iro
Department of Astronomy, University of Maryland, College Park, MD, USA
Eliza M.-R. Kempton, Thaddeus D. Komacek & Matthew C. Nixon
European Space Agency, Space Telescope Science Institute, Baltimore, MD, USA
Sarah Kendrew
California Institute of Technology, IPAC, Pasadena, CA, USA
Jessica Krick
Laboratoire d’Astrophysique de Bordeaux, Université de Bordeaux, Pessac, France
Jérémy Leconte
Département d’Astronomie, Université de Genève, Sauverny, Switzerland
Monika Lendl & Dominique J. M. Petit dit de la Roche
Department of Mathematics and Statistics, University of Exeter, Exeter, UK
Neil T. Lewis
Department of Physics, Utah Valley University, Orem, UT, USA
Joshua D. Lothringer
Department of Physics, University of Rome “Tor Vergata”, Rome, Italy
Luigi Mancini
INAF - Turin Astrophysical Observatory, Turin, Italy
Steward Observatory, University of Arizona, Tucson, AZ, USA
Megan Mansfield
Department of Physics and Astronomy, Faculty of Environment, Science and Economy, University of Exeter, Exeter, UK
Nathan J. Mayne
School of Information and Physical Sciences, University of Newcastle, Callaghan, NSW, Australia
Thomas M. Evans-Soma
Universitäts-Sternwarte, Ludwig-Maximilians-Universität München, Munich, Germany
Karan Molaverdikhani
Exzellenzcluster Origins, Garching, Germany
Department of Earth, Atmospheric and Planetary Sciences, Massachusetts Institute of Technology, Cambridge, MA, USA
Benjamin V. Rackham
Kavli Institute for Astrophysics and Space Research, Massachusetts Institute of Technology, Cambridge, MA, USA
Centre for ExoLife Sciences, Niels Bohr Institute, Copenhagen, Denmark
Aaron D. Schneider
School of Earth and Space Exploration, Arizona State University, Tempe, AZ, USA
Luis Welbanks
Department of Physics and Astronomy, University College London, London, UK
Sergei N. Yurchenko
Department of Earth and Planetary Sciences, University of California, Santa Cruz, Santa Cruz, CA, USA
You can also search for this author in PubMed Google Scholar
Contributions
All authors played an appreciable role in one or more of the following: development of the original proposal, management of the project, definition of the target list and observation plan, analysis of the data, theoretical modelling, and preparation of this paper. Some specific contributions are listed as follows. N.M.B., J.L.B. and K.B.S. provided overall programme leadership and management. L.K. and N.C. coordinated the MIRI working group. L.K., V.P., K.B.S., D.K.S., E.M.-R.K., O.V. and P.E.C. made substantial contributions to the design of the programme and the observing proposal. K.B.S. generated the observing plan with input from the team. A.D., P.-O.L., R.C.C., A.L.C., G.M. and M.M. led or co-led working groups and/or contributed to important strategic planning efforts like the design and implementation of the pre-launch data challenges. P.E.C., D.K.S., R.C.C., P.-O.L. and J.B. generated simulated data for pre-launch testing of methods. L.K., T.J.B., M.T.R., N.C., V.P., A.A.A.P. and J.I.M. contributed substantially to the writing of this paper. T.J.B., N.C., M.Z. and E.D. contributed to the development of data analysis pipelines and/or provided the data analysis products used in this analysis that is, reduced the data, modelled the light curves, and/or produced the planetary spectrum. A.A.A.P. coordinated the atmospheric retrieval analysis with contributions from J.K.B., J.B., L.-P.C., M.Z., and K.L.C. M.T.R. coordinated the GCM results and interpretation with contributions from X.T., L.T., L.C., J.M.M. and I.M. T.J.B., N.C., P.E.C., J.B., L.-P.C. and M.H. generated figures for this paper. M.C.N., X.Z., B.V.R., J.K., M.L.-M., B.C., S.L.C. and R.H. provided substantial feedback to the paper, and G.M. and K.L.C. coordinated comments from all authors.
Corresponding author
Correspondence to Taylor J. Bell .
Ethics declarations
Competing interests.
The authors declare no competing interests.
Peer review
Peer review information.
Nature Astronomy thanks the anonymous reviewer(s) for their contribution to the peer review of this work
Additional information
Publisher ’ s note Springer Nature remains neutral with regard to jurisdictional claims in published maps and institutional affiliations.
Extended data
Extended data fig. 1 the underestimation of uncertainties as a function of spectral binning for the l168-9b commissioning observations..
a , The observed L168-9b transmission spectrum with 1 σ error bars for spectrally unbinned data (grey circles), 0.15 μ m bins (black squares), 0.5 μ m bins (large red circles), and a 5-12 μ m broadband bin (horizontal blue shaded region). The spectrum for wavelength pairs is not shown to avoid excessive clutter. b , The median of the transit depth uncertainties are shown with blue squares, while the observed scatter in the transmission spectrum is shown with orange circles. For unbinned data, the transmission spectrum shows about 2.5 × the scatter predicted by the fits to the individual light curves. Binning pairs of wavelengths reduces the level of underestimation of the scatter in the transmission spectrum, but considerable excess noise remains. Coarser binning schemes like the constant 0.15 μ m bins used in the MIRI time-series observation commissioning paper 29 or the 0.5 μ m bins we use in this work further reduce the level of uncertainty underestimation.
Extended Data Fig. 2 A model-independent demonstration of the initial changes in flux for the WASP-43b observations.
a , The first 120 minutes of three of our spectroscopically binned light curves of WASP-43b (with 1 σ uncertainties) showing the initial settling behaviour as a function of wavelength. A teal dashed line shows the amplitude of a -0.25% change in flux compared to the values around 120 minutes, and a magenta dotted line shows a +0.25% change. b , A summary of the ramp amplitudes, signs, and timescales for each of our wavelength bins (with 1 σ uncertainties). The teal and magenta horizontal lines are the same as those in panel a to aid in translating between the two figures. At short wavelengths, the flux sharply drops by about 0.5% within the first 30 minutes and then largely settles but does continue to decrease with time. With increasing wavelength, the strength of this initial ramp decreases and eventually changes sign, becoming an upwards ramp. Within the ‘shadowed region’ (marked in red), the light curves show a very strong upwards ramp that takes much longer (greater than about 60 minutes) to appreciably decay. It is important to note that the data in this figure also includes a small amount of astrophysical phase variations which should result in a small increase in flux of less than 0.05% per hour.
Extended Data Fig. 3 Retrieved spectra from the six retrievals.
a , Median retrieved nightside spectra for the HyDRA (dark blue line), NEMESIS (dash-dotted gold line), and PyratBay (dashed magenta line) and their 1 σ contour. The regions of higher water opacity are indicated by the purple shading at the top of the panel, with the observed rise in flux at 6.3 μ m being caused by a drop in opacity. b , c , and d , Same as panel a for the evening terminator, dayside, and morning terminator respectively. e , f , g , and h , Same as panels a, b, c, and d, for the SCARLET (dashed red line), PLATON (blue line), and ARCiS (dash-dotted green line) retrievals.
Extended Data Fig. 4 Chemically-consistent atmospheric retrievals.
Same as Figure 4 but for retrievals assuming thermochemical-equilibrium abundances consistent with the pressure-temperature profiles. a , 1 σ credible interval contours of the temperature profiles. The black curves show the predicted temperature profile from a 2D radiative-transport model46. The vertical bars show the range of pressures probed by the observations. b and c , probability posterior distributions for H 2 O and CH 4 abundances, respectively. The shaded area for each curve denotes the 1 σ credible interval of each posterior. The green and blue bars denote the abundances predicted by equilibrium and disequilibrium-chemistry models with solar abundances, respectively, at the pressures probed by the observations. Compared to the free-chemistry retrievals, the thermochemical-equilibrium retrievals on the nightside spectra produced worse fits, this is driven particularly by the higher amount of methane expected under equilibrium chemistry.
Extended Data Fig. 5 Retrieval contribution functions.
Contribution functions integrated over the data point spectral bins, at each phase ( a-d ), and for each retrieval framework. These curves show the range of pressures probed by the observation according to the atmospheric models. The enhanced opacity from the water band around 7-9 μ m makes these wavelengths probe lower pressures and hence colder temperatures, whereas the rest of the observing window probes higher pressures and higher temperatures.
Extended Data Fig. 6 PyratBay clouds exploration.
a , Cloud species that condense in the temperature regime expected for the WASP-43b nightside. Dashed lines represent vapour pressure curves 162 for each species assuming solar composition, while the coloured ranges denote the corresponding extent of the vapour pressure curves assuming 100 × sub- and super-solar atmospheric composition. The extent of the retrieved nightside contribution functions is shown in grey, and the extent of the retrieved temperature uncertainties is shown in light purple. The intersection between the contribution function and temperature ranges indicates the pressures at which we could observe cloud condensation and potentially detect their spectral features, if present in the observations. b , Panels display the retrieved posterior density plots for the explored cloud parameters of the TSC model (cloud number density, q*; effective particle size, r eff ; and the standard deviation of the log-normal distribution, \({\sigma }_{\log }\) ) for the MnS clouds. The black vertical line denotes the parameter’s median value, while the extent of the purple region denotes the 1 σ uncertainties, both given at the top left corner of the panel. Similar, fully non-constrained posteriors are retrieved for other explored cloud species, MgSiO 3 , ZnS, and KCl, suggesting the lack of observable spectral characteristics from clouds in the observed data.
Extended Data Fig. 7 A comparison of the retrieved temperature-pressure profiles to the GCM simulations.
Each of a-d shows the temperature profile retrieved by HyDRA, compared to the GCM simulations highlighted in Figure 3 and listed in Extended Data Table 1. The GCM temperature profiles are calculated at phases 0.0, 0.25, 0.5, and 0.75 by averaging over the visible hemisphere by viewing angle, to produce a one-dimensional profile that is comparable to the retrieved profile. The GCM simulations are generally warmer on the nightside than the retrieved temperatures; cloudy simulations emit from lower pressures and so match the observed lower brightness temperatures better (see the contribution functions in Extended Data Fig. 5).
Supplementary information
Supplementary information.
Supplementary Table 1 and Figs. 1 and 2.
Rights and permissions
Open Access This article is licensed under a Creative Commons Attribution 4.0 International License, which permits use, sharing, adaptation, distribution and reproduction in any medium or format, as long as you give appropriate credit to the original author(s) and the source, provide a link to the Creative Commons licence, and indicate if changes were made. The images or other third party material in this article are included in the article’s Creative Commons licence, unless indicated otherwise in a credit line to the material. If material is not included in the article’s Creative Commons licence and your intended use is not permitted by statutory regulation or exceeds the permitted use, you will need to obtain permission directly from the copyright holder. To view a copy of this licence, visit http://creativecommons.org/licenses/by/4.0/ .
Reprints and permissions
About this article
Cite this article.
Bell, T.J., Crouzet, N., Cubillos, P.E. et al. Nightside clouds and disequilibrium chemistry on the hot Jupiter WASP-43b. Nat Astron (2024). https://doi.org/10.1038/s41550-024-02230-x
Download citation
Received : 01 November 2023
Accepted : 22 February 2024
Published : 30 April 2024
DOI : https://doi.org/10.1038/s41550-024-02230-x
Share this article
Anyone you share the following link with will be able to read this content:
Sorry, a shareable link is not currently available for this article.
Provided by the Springer Nature SharedIt content-sharing initiative
Quick links
- Explore articles by subject
- Guide to authors
- Editorial policies
Sign up for the Nature Briefing newsletter — what matters in science, free to your inbox daily.

Browse Econ Literature
- Working papers
- Software components
- Book chapters
- JEL classification
More features
- Subscribe to new research
RePEc Biblio
Author registration.
- Economics Virtual Seminar Calendar NEW!
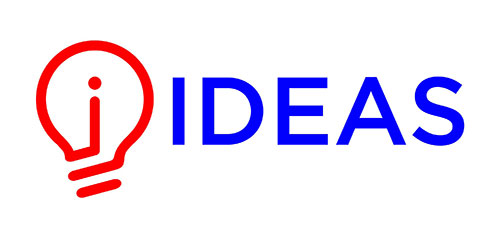
The level of digitalization in Commercial banks and bank liquidity creation
- Author & abstract
- Related works & more
Corrections
- Yang, Lingyan
Suggested Citation
Download full text from publisher.
Follow serials, authors, keywords & more
Public profiles for Economics researchers
Various research rankings in Economics
RePEc Genealogy
Who was a student of whom, using RePEc
Curated articles & papers on economics topics
Upload your paper to be listed on RePEc and IDEAS
New papers by email
Subscribe to new additions to RePEc
EconAcademics
Blog aggregator for economics research
Cases of plagiarism in Economics
About RePEc
Initiative for open bibliographies in Economics
News about RePEc
Questions about IDEAS and RePEc
RePEc volunteers
Participating archives
Publishers indexing in RePEc
Privacy statement
Found an error or omission?
Opportunities to help RePEc
Get papers listed
Have your research listed on RePEc
Open a RePEc archive
Have your institution's/publisher's output listed on RePEc
Get RePEc data
Use data assembled by RePEc
Religious Worship Attendance in America: Evidence from Cellphone Data

Religious worship is integral to the lives of millions of Americans. In this paper, I provide a descriptive analysis of religious worship attendance using geodata from smartphones for over 2 million Americans in 2019. I establish several key findings. First, 73% of people step into a religious place of worship at least once during the year on the primary day of worship (e.g. Sundays for most Christian churches). However, only 5% of Americans attend services “weekly”, far fewer than the ~22% who report to do so in surveys. The number of occasional vs. frequent attenders varies substantially by religion. I estimate that approximately 45M Americans attend worship services in a typical week of the year, but with large changes around Holidays (e.g. Easter). I document how start times, duration of attendance, and average household income all differ meaningfully across religious traditions. The intensity of religious observance correlates with a host of other activities. For example, relative to non-attenders and infrequent attenders, frequent religious attenders are less likely to go to strip clubs, liquor stores, and casinos. While cell phone data has limitations, this paper provides a unique way of understanding worship attendance and its correlates.
More Research From These Scholars
Stability of experimental results: forecasts and evidence, a note on the level of customer support by state governments: a mystery-shopping approach.
- Japanese (日本)
Our new research: Enhancing blockchain analytics through AI

Elliptic Research
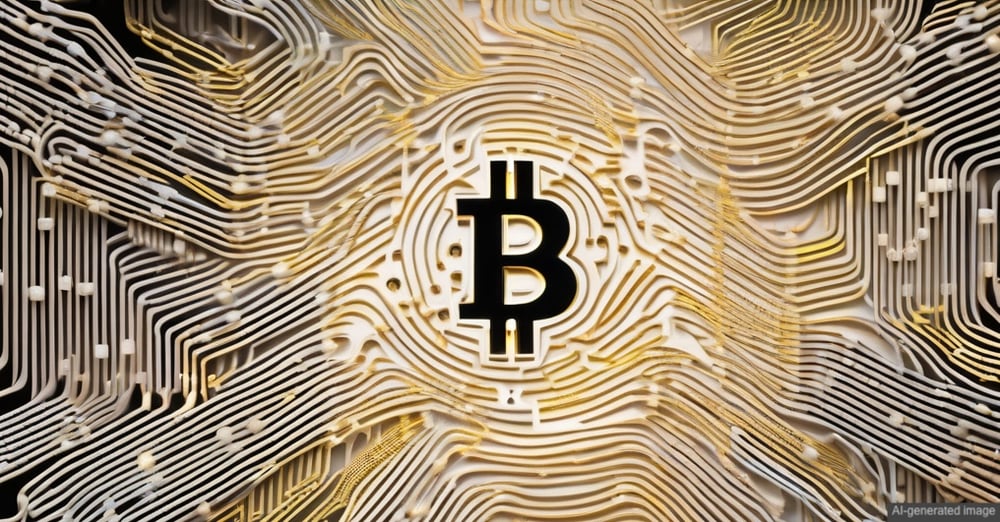
- Elliptic researchers have made advances in the use of AI to detect money laundering in Bitcoin. A new paper describing this work is co-authored with researchers from the MIT-IBM Watson AI Lab.
- A deep learning model is used to successfully identify proceeds of crime deposited at a crypto exchange, new money laundering transaction patterns and previously-unknown illicit wallets. These outputs are already being used to enhance Elliptic’s products.
- Elliptic has also made the underlying data publicly available . Containing over 200 million transactions, it will enable the wider community to develop new AI techniques for the detection of illicit cryptocurrency activity.
At Elliptic we have always pushed the boundaries of blockchain analytics, to enable our customers to more accurately and efficiently assess risk in cryptoassets. Part of this innovation has been exploring how artificial intelligence can be leveraged to improve the detection of money laundering and other financial crime on blockchains.
Blockchains provide fertile ground for machine learning techniques, thanks to the availability of both transaction data and information on the types of entities that are transacting, collected by us and others. This is in contrast to traditional finance where transaction data is typically siloed, making it challenging to apply these techniques.
Machine learning on the blockchain
We first published research on this topic in 2019, co-authored with researchers from the MIT-IBM Watson AI Lab. A machine learning model was trained to identify Bitcoin transactions made by illicit actors, such as ransomware groups or darknet marketplaces. The training data, compiled by Elliptic and containing over 200,000 bitcoin transactions, was made publicly available to encourage further experimentation and collaboration within this emerging field. That paper has now been cited nearly 400 times by researchers around the world, which demonstrates the impact it has had and continues to have in the fields of machine learning and anti-money laundering.
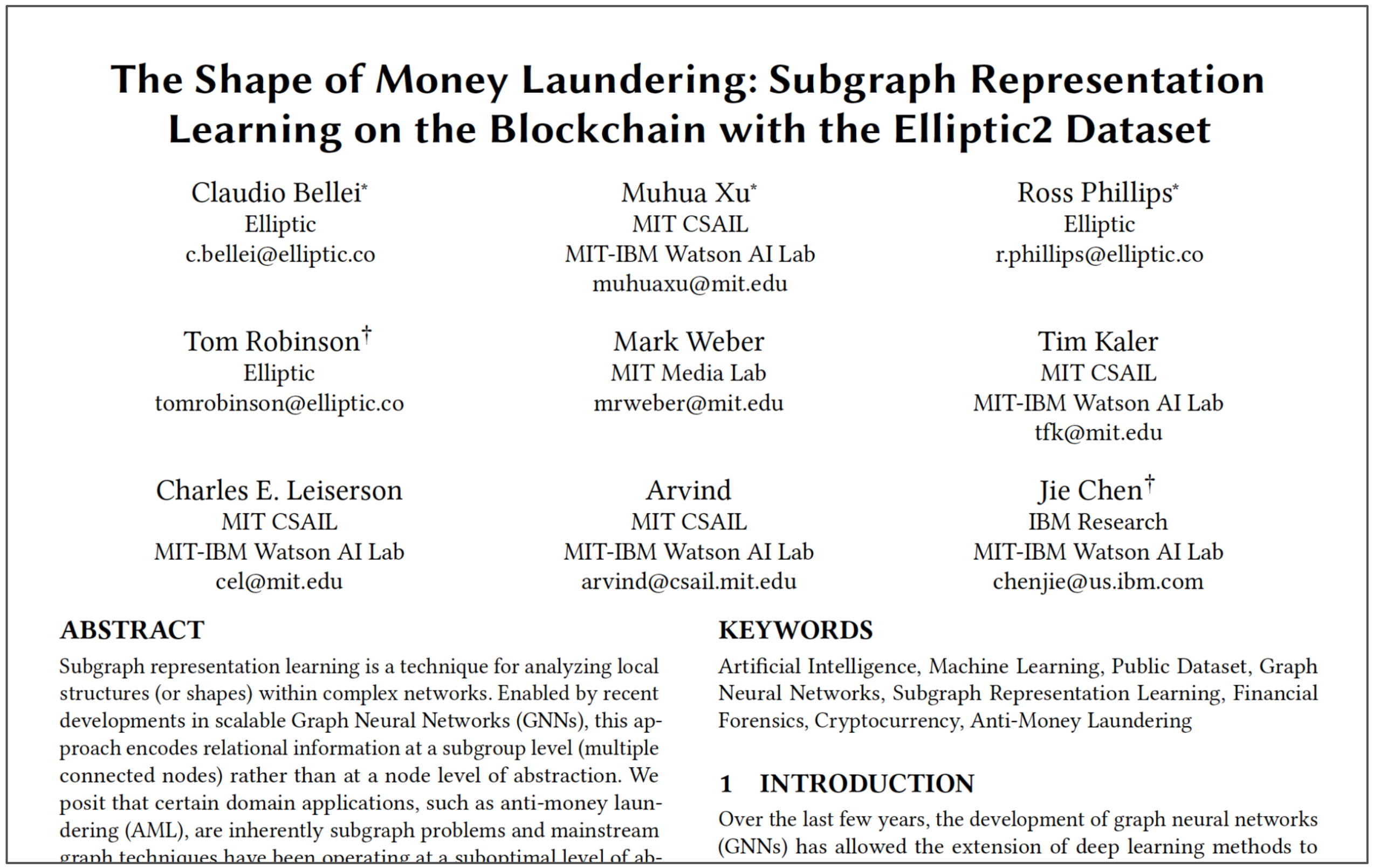
We have now released further research , applying new techniques to a much larger dataset, containing nearly 200 million transactions. This work is again co-authored by researchers from the MIT-IBM Watson AI Lab. Rather than identifying transactions made by illicit actors, a machine learning model is trained to identify “subgraphs”, chains of transactions that represent bitcoin being laundered. By identifying these subgraphs rather than illicit wallets, this approach allows us to focus on the “multi-hop” laundering process more generally rather than the on-chain behavior of specific illicit actors.
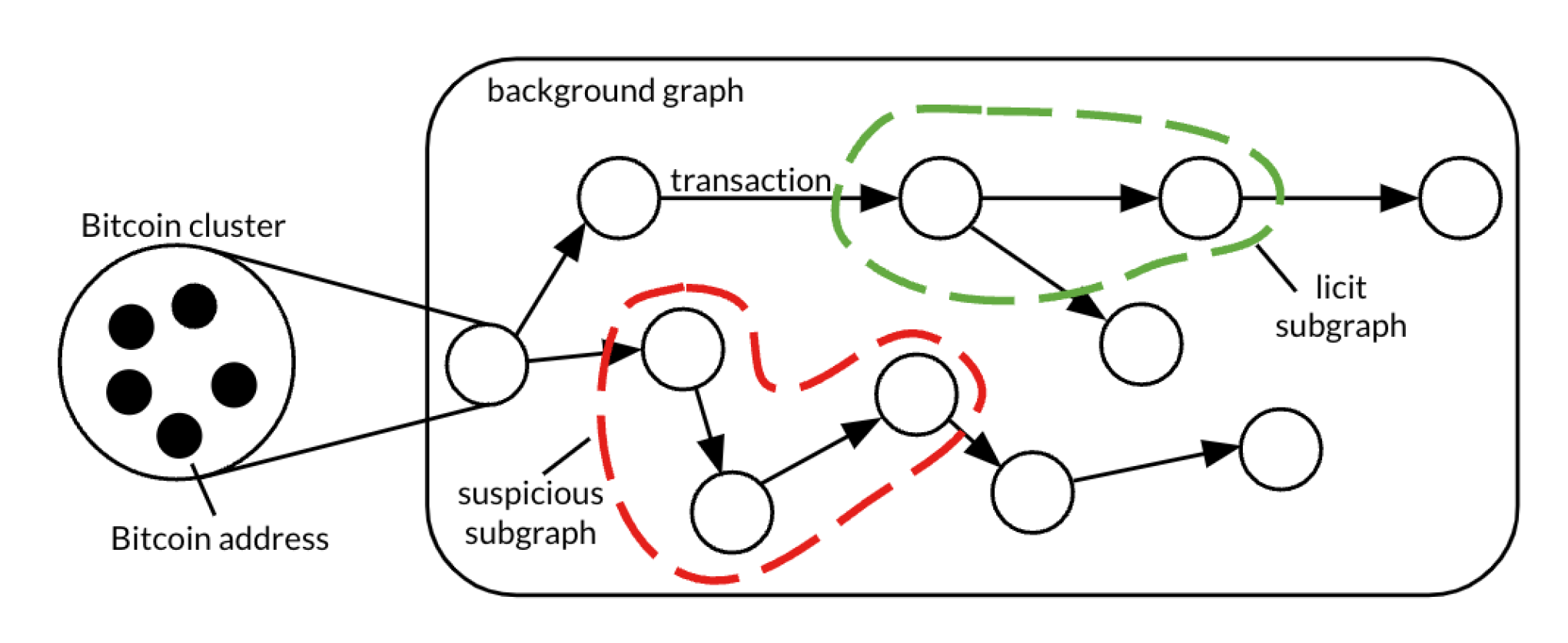
Testing our results
We worked with a cryptocurrency exchange to test whether this technique could be used to identify money laundering attempts through that business. Of 52 “money laundering” subgraphs predicted by the model and which ended with deposits to this exchange, the exchange confirmed that 14 had been received by users who had already been flagged as being linked to money laundering. On average less than one in 10,000 of these accounts are flagged as such, suggesting that the model performs very well * . Importantly, the exchange’s insights were based on off-chain information, suggesting that the model can identify money laundering that would not be identifiable using traditional blockchain analytical techniques alone.
We also investigated the types of money laundering patterns that the trained model was identifying. This revealed known money laundering patterns such as “peeling chains”, which can already be automatically detected in Elliptic’s transaction and wallet screening tools. However it also identified novel patterns such as the use of intermediary “nested services” in specific ways. Knowledge of these money laundering behaviors is of value to AML practitioners, and can be added to the suite of behaviours that can be detected with Elliptic’s tools.
.png?width=2500&height=1307&name=AI_Blog_image1_1200_627%20(1).png)
The machine learning model can also be used to help identify previously-unknown illicit wallets. When the model predicts that a given subgraph is an instance of money laundering, it implies that the funds have potentially originated from some type of illicit activity. Directed research can then be performed on these wallets to try to identify them. This approach has already enabled us to identify a number of previously unknown wallets used by illicit actors including ponzi schemes and darknet markets.
Sharing our data with the community
As well as releasing our research, we have also made the underlying data publicly available . The largest public dataset of its kind, “Elliptic2” will enable the development of new techniques for the detection of illicit cryptocurrency transactions by the wider community. It will also aid the development of the underlying graph neural network methods, used in applications including drug discovery, physics and computer vision.
This novel work demonstrates that AI methods can be applied to blockchain data to identify illicit wallets and money laundering patterns, which were previously hidden from view. This is made possible by the inherent transparency of blockchains and demonstrates that cryptoassets, far from being a haven for criminals, are far more amenable to AI-based financial crime detection than traditional financial assets. We have barely scratched the surface of what is possible in this domain, but this work has already led to benefits for Elliptic’s users. Further collaboration and data-sharing will be key to advancing these techniques further and combating financial crime in cryptoassets.
You can read the research paper in full here , and the Elliptic2 dataset is now available to access. To discuss the research, and find out more about how we are applying these new techniques to enhance our products, get in touch .
Featured Articles
Found this interesting? Share to your network.
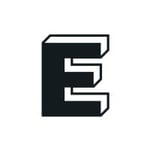
The latest deep-dives and data-driven analysis from our Research team covering their own investigations, as-its-happening monitoring of hacks and thefts, and more.
This blog is provided for general informational purposes only. By using the blog, you agree that the information on this blog does not constitute legal, financial or any other form of professional advice. No relationship is created with you, nor any duty of care assumed to you, when you use this blog. The blog is not a substitute for obtaining any legal, financial or any other form of professional advice from a suitably qualified and licensed advisor. The information on this blog may be changed without notice and is not guaranteed to be complete, accurate, correct or up-to-date.
Get the latest insights in your inbox
Poll: Biden and Trump supporters sharply divided by the media they consume
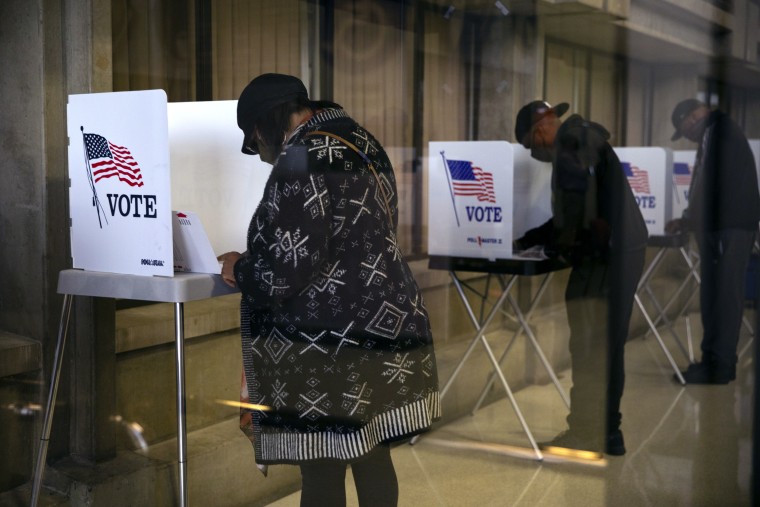
Supporters of President Joe Biden and former President Donald Trump are sharply divided across all sorts of lines, including the sources they rely on to get their news, new data from the NBC News poll shows.
Biden is the clear choice of voters who consume newspapers and national network news, while Trump does best among voters who don’t follow political news at all.
The stark differences help highlight the strategies both candidates are using as they seek another term in the White House — and shed some light on why the presidential race appears relatively stable.
The poll looked at various forms of traditional media (newspapers, national network news and cable news), as well as digital media (social media, digital websites and YouTube/Google). Among registered voters, 54% described themselves as primarily traditional news consumers, while 40% described themselves as primarily digital media consumers.
Biden holds an 11-point lead among traditional news consumers in a head-to-head presidential ballot test, with 52% support among that group to Trump’s 41%. But it’s basically a jump ball among digital media consumers, with Trump at 47% and Biden at 44%.
And Trump has a major lead among those who don’t follow political news — 53% back him, and 27% back Biden.
“It’s almost comic. If you’re one of the remaining Americans who say you read a newspaper to get news, you are voting for Biden by 49 points,” said Republican pollster Bill McInturff, who conducted the poll alongside Democratic pollster Jeff Horwitt.
The trends also extend to other questions in the poll. There's a significant difference in how traditional news consumers view Biden, while digital news consumers are far more in line with registered voters overall.
More primarily traditional news consumers have positive views of Biden (48%) than negative ones (44%). Among primarily digital news consumers, 35% view Biden positively, and 54% view him negatively. Vice President Kamala Harris' positive ratings show a similar divide, while Trump is viewed similarly by news consumers of both stripes.
And although the sample size is small, those who don't follow political news feel more positively about Trump and independent presidential candidate Robert F. Kennedy Jr. and more negatively about Biden.
Trump’s lead among those not following political news caught Horwitt’s eye amid Trump's trial on charges related to allegations he paid hush money to quash news of an alleged affair from coming out during the heat of his 2016 presidential campaign and as he faces legal jeopardy in other cases that consistently make news.
“These are voters who have tuned out information, by and large, and they know who they are supporting, and they aren’t moving,” Horwitt said.
“That’s why it’s hard to move this race based on actual news. They aren’t seeing it, and they don’t care,” he continued.
Third-party candidates also do well with this chunk of the electorate — a quarter of the 15% who say they don’t follow political news choose one of the other candidates in a five-way ballot test that includes Kennedy, Jill Stein and Cornel West. Third-party supporters also make up similar shares of those who say they get their news primarily from social media and from websites.
But voting behavior among those groups suggests that Biden's stronger showing with those traditional media consumers puts him ahead with a more reliable voting bloc.
Of those polled who could be matched to the voter file, 59% of those who voted in both 2020 and 2022 primarily consume traditional media, 40% primarily consume digital media, and just 9% don't follow political news. (The percentages add up to more than 100% because some people chose media platforms across multiple categories.)
Those who voted less frequently were more likely to say they don’t follow political news: 19% of those who voted in the last presidential election but not in 2022 and 27% who voted in neither of the last two elections say they don't follow political news.
The NBC News poll of 1,000 registered voters nationwide — 891 contacted via cellphone — was conducted April 12-16, and it has an overall margin of error of plus or minus 3.1 percentage points.

Ben Kamisar is a national political reporter for NBC News.

IMAGES
VIDEO
COMMENTS
Checklist: Research results 0 / 7. I have completed my data collection and analyzed the results. I have included all results that are relevant to my research questions. I have concisely and objectively reported each result, including relevant descriptive statistics and inferential statistics. I have stated whether each hypothesis was supported ...
Research results refer to the findings and conclusions derived from a systematic investigation or study conducted to answer a specific question or hypothesis. These results are typically presented in a written report or paper and can include various forms of data such as numerical data, qualitative data, statistics, charts, graphs, and visual aids.
1. Context. The "results section" is the heart of the paper, around which the other sections are organized ().Research is about results and the reader comes to the paper to discover the results ().In this section, authors contribute to the development of scientific literature by providing novel, hitherto unknown knowledge ().In addition to the results, this section contains data and ...
The results section of a research paper is usually the most impactful section because it draws the greatest attention. Regardless of the subject of your research paper, a well-written results section is capable of generating interest in your research. For detailed information and assistance on writing the results of a research paper, refer to ...
The results section of a research paper tells the reader what you found, while the discussion section tells the reader what your findings mean. The results section should present the facts in an academic and unbiased manner, avoiding any attempt at analyzing or interpreting the data. Think of the results section as setting the stage for the ...
The "Results section" is the third most important anatomical structure of IMRAD (Introduction, Method and Material, Result, And Discussion) frameworks, the almost universally accepted framework in many journals in the late nineteenth century. 3 Before using a structured IMRAD format, research findings in scientific papers were presented in ...
For most research papers in the social and behavioral sciences, there are two possible ways of organizing the results. Both approaches are appropriate in how you report your findings, but use only one approach. Present a synopsis of the results followed by an explanation of key findings. This approach can be used to highlight important findings.
The results section of the research paper is where you report the findings of your study based upon the information gathered as a result of the methodology [or methodologies] you applied. The results section should simply state the findings, without bias or interpretation, and arranged in a logical sequence. The results section should always be ...
The results section is the core of a research paper where the study data and analyses are presented in an organized, uncluttered manner such that the reader can easily understand and interpret the findings. It is often embellished with self-explanatory tables and figures which assist in presenting data in addition to the text.
Step 1: Consult the guidelines or instructions that the target journal or publisher provides authors and read research papers it has published, especially those with similar topics, methods, or results to your study. The guidelines will generally outline specific requirements for the results or findings section, and the published articles will ...
Developing a well-written research paper is an important step in completing a scientific study. This paper is where the principle investigator and co-authors report the purpose, methods, findings, and conclusions of the study. A key element of writing a research paper is to clearly and objectively report the study's findings in the Results section.
Build coherence along this section using goal statements and explicit reasoning (guide the reader through your reasoning, including sentences of this type: 'In order to…, we performed….'; 'In view of this result, we ….', etc.). In summary, the general steps for writing the Results section of a research article are:
The Results (also sometimes called Findings) section in an empirical research paper describes what the researcher(s) found when they analyzed their data. Its primary purpose is to use the data collected to answer the research question(s) posed in the introduction, even if the findings challenge the hypothesis.
The results section of a research paper refers to the part that represents the study's core findings from the methods that the researcher used to collect and analyze data. This section presents the results logically without interpretation or bias from the author. Thus, this part of a research paper sets up the read for evaluation and analysis ...
Present the results of the paper, in logical order, using tables and graphs as necessary. Explain the results and show how they help to answer the research questions posed in the Introduction. Evidence does not explain itself; the results must be presented and then explained. Avoid: presenting results that are never discussed; presenting ...
This is an example of the results section from a psychology research paper where authors are outlining their main findings. In this paper, the authors are investigating the effects of different types of music on people. The authors say that they found significant differences between classical and pop music in terms of memory recall.
Step 2: Use Concise Text and Visuals. When writing the result section, use clear and concise language to communicate your results. Avoid unnecessary details and focus on conveying the key research findings succinctly. Present each piece of data or result once, eliminating redundancy for a streamlined presentation.
Step 1: Organize Your Data. Organizing your data is a critical first step in the Results section of a research paper. This involves structuring your raw data in a way that is clear, logical, and easily digestible for your readers. Raw Data Arrangement: Group similar data logically, such as by time or experimental conditions.
A results section is the third component of a research paper that follows the introduction and methodology sections. This third component provides researchers with the opportunity to objectively ...
The results section is only for the results of the research. The results section provides the facts about what you discovered in the course of your research or experiment. The discussion section is where you can get analytical or reflective about exactly what you have discovered. This is the place where you can tell us what the results mean.
A results section is a crucial part of a research paper or dissertation, where you analyze your major findings. This section goes beyond simply presenting study outcomes. You should also include a comprehensive statistical analysis and interpret the collected data in detail.
Research. 1. Introduction. In this tutorial, we'll discuss presenting research results in a scientific paper. Writing a paper comes after choosing the research problem, compiling a thorough literature review, and conducting the simulations. 2. Results. Let's start with an example. Let's say we conducted an experiment analyzing the ...
Our results provide strong evidence that the atmosphere of WASP-43b is shaped by disequilibrium processes and provide new insights into the properties of the planet's nightside clouds.
Downloadable (with restrictions)! This paper takes all banking enterprises among Chinese listed companies as the research sample and examines the impact of the level of digitalization in commercial banks on bank liquidity creation through constructing an empirical econometric model. The research results show that the level of digitalization in commercial banks can significantly enhance the ...
College Board provides a variety of tools and resources to help educators prepare for test day and analyze results. Assessment Reporting for K-12 Educators View and analyze student scores for the entire SAT Suite. Sign In School Code Search Search for K-12 school codes, also known as College Entrance Examination Board (CEEB) codes. ...
In this paper, I provide a descriptive analysis of religious worship attendance using geodata from smartphones for over 2 million Americans in 2019. I establish several key findings. First, 73% of people step into a religious place of worship at least once during the year on the primary day of worship (e.g. Sundays for most Christian churches).
Elliptic researchers have made advances in the use of AI to detect money laundering in Bitcoin. A new paper describing this work is co-authored with researchers from the MIT-IBM Watson AI Lab.; A deep learning model is used to successfully identify proceeds of crime deposited at a crypto exchange, new money laundering transaction patterns and previously-unknown illicit wallets.
AP Seminar and AP Research students to submit performance tasks as final and their presentations to be scored by their AP Seminar or AP Research teachers. AP Computer Science Principles students to submit their Create performance task as final. Late Testing . Occasionally, circumstances make it necessary for students to test late.
Supporters of President Joe Biden and former President Donald Trump are sharply divided across all sorts of lines, including the sources they rely on to get their news, new data from the NBC News ...